Purdue’s Anvil Supercomputer Assists with COVID-19 Pandemic Response
A research group from the University of Virginia (UVA) utilized Purdue’s Anvil supercomputing cluster to help provide COVID-19 scenario modeling for local, state, federal, and university officials and departments. The team used computer models to predict what might happen with the virus, such as how it might spread, how many people could get sick and need to go to the hospital, and how vaccines might help over time. The computational power of Anvil was used to run these scenario models, which produced data that helped drive pandemic response efforts in real-time at varying levels of government.
Dr. Madhav Marathe, the Director of the Network Systems Science and Advanced Computing (NSSAC) division of the Biocomplexity Institute and Initiative at the University of Virginia, works in the field of computational epidemiology. He and his team use advanced modeling techniques and simulations to investigate large-scale biological, social, and technological systems. In this instance, the NSSAC team was tasked with simulating scenarios surrounding the COVID-19 pandemic. The scenario models produced by the team were not only used by local, state, and federal officials to help make informed decisions regarding pandemic response, but were also used to bolster the CDC’s COVID-19 Scenario Modeling Hub, a consortium combining long-term COVID-19 projections from different research teams. The importance of computational epidemiology during a pandemic cannot be overstated—more data equates to more accurate projections, and high-performance computing (HPC) is needed to run the simulations and analyze that data quickly. To quote a paper co-authored by Dr. Marathe (Marathe & Vullikanti, 2013):
“Computational models help in understanding the space-time dynamics of epidemics. Models and algorithms can be used to evaluate various intervention strategies, including pharmaceutical interventions such as vaccinations and anti-virals, as well as non-pharmaceutical interventions such as social distancing and school closures.”
Without computational epidemiology, pandemic-response teams and decision-makers would be flying blindfolded. But even with the help of supercomputers, not all datasets are created equal—the type of model you use to obtain the data matters.
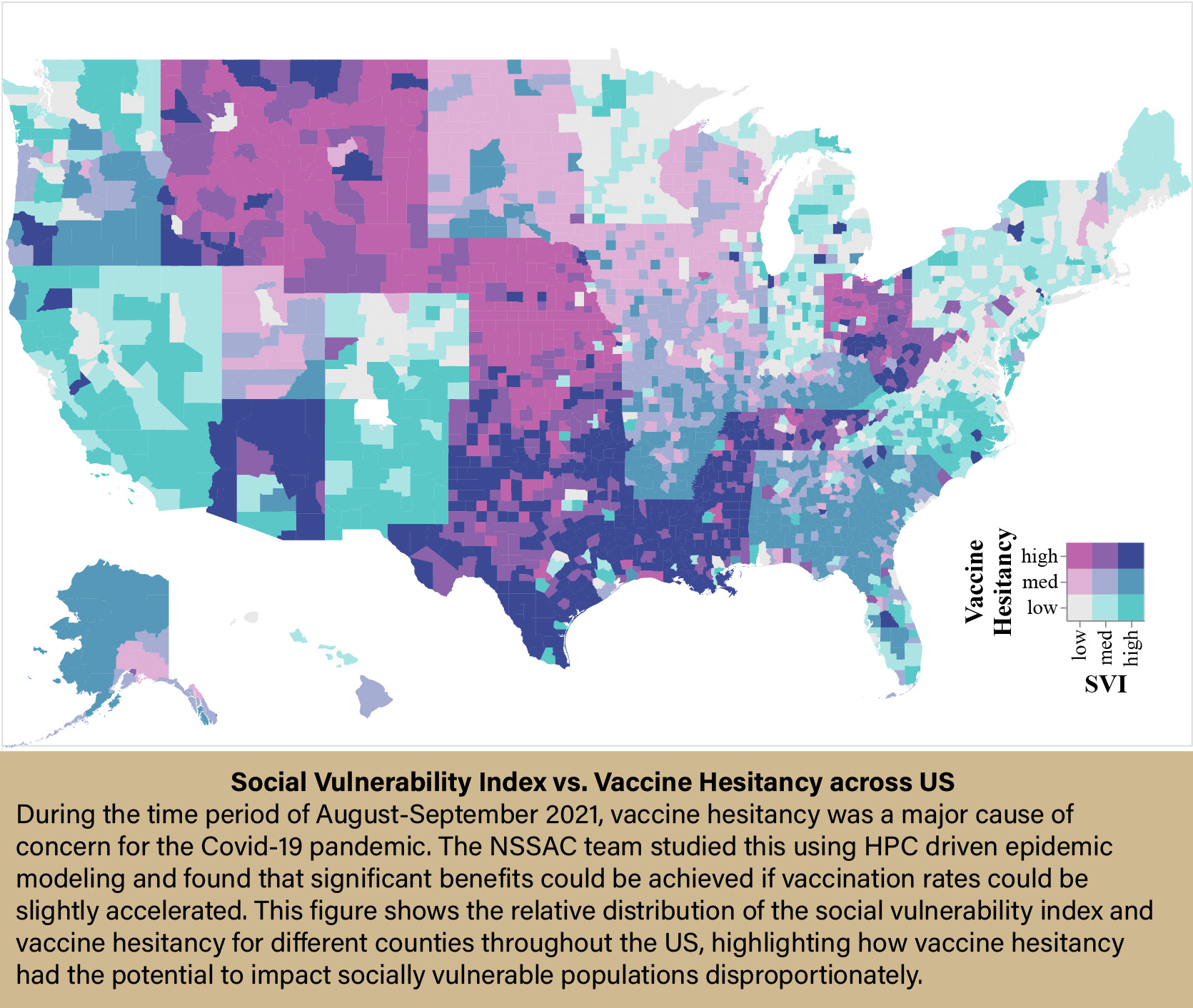
In the realm of epidemiology, three main types of modeling are used, each with its strengths and limitations: statistical-based models, compartmental mass-action models, and agent-based models. Agent-based modeling is a relative newcomer to the party. Pioneered by Marathe and his group in 2004, agent-based models take into account real-world factors, such as geography, social relationships, and individual differences—things that can have a major impact on how a disease spreads through a community but are lost in traditional epidemiology models.
“The idea is,” says Dr. Marathe, “if I want to understand how a disease spreads in a population, then I want to understand the social network that underlies this population because I want to capture the underlying heterogeneity that exists. Compartmental mass-action models treat everyone as identical. They lose all aspects of heterogeneity and asymmetry that exist in the real world.”
Agent-based modeling eliminates this oversight by representing individual people moving throughout their specific environment, behaving as normal humans would behave. These models simulate realistic populations, complete with a proper distribution of age, gender, ethnicity, job type, and geographical location. They also factor in individual behavior, such as vaccine usage or mask-wearing, which is traditionally difficult to account for, yet is a huge factor in disease spread.
“These models tell you something that is quite unique in their form. So, for instance, you can start understanding the role of network structure on the outcome—whether a dense part of the town would have more diseases than a more sparse part of the town. Or, would it affect people from some racial or ethnic background more than others because they stay together in close quarters? Or is it the case that folks who are resistant to taking vaccines are likely to be impacted somewhat disproportionately to people who have taken vaccines? These are decisions and issues where individuals and individual behavior and their situatedness in the network play a role. And this aspect is lost in aggregate models.”
Agent-based models are excellent at providing accurate, real-world data that can be used to inform policy and decision-makers on how best to respond to an evolving situation, but there is one downside—they require a massive amount of computing power.
For agent-based modeling to work, each individual accounted for within the model has to be its own data point. So when the NSSAC team set out to provide “what-if” COVID-19 scenarios for the entire population of the United States, they needed to replicate more than 300 million people with-individual level resolution, a task that required an astounding amount of computations to complete. According to Dr. Marathe, “This is not a computation that you can do on a single laptop or even a small cluster. Certainly not multiple runs. These are very, very large computations. And that’s where Anvil-like systems come in very, very handy, and are extremely useful in the process.”
To manage such heavy computations, NSSAC not only needs access to multiple high-performance computing systems, but they also need an efficient interdisciplinary team to manage the workflow.
Jiangzhuo Chen is a Research Associate Professor and a computer scientist working on epidemiology at NSSAC. Chen’s role in the project involves building the agent-based models for COVID-19, setting up the experiments, and interpreting the results. Once the models are built, Chen coordinates with Stefan Hoops, a Research Associate Professor at NSSAC, and Dustin Machi, the Senior Software Architect at NSSAC. Hoops is the author of EpiHiper, the simulation software used by the team. “EpiHiper,” says Hoops, “is a simulation tool that is highly adaptable to the policy question without any alteration. This improves the quality of the results and thus is creating trust.”
Machi takes the models and experiments that have been designed by Chen and—using the EpiHiper software created by Hoops—runs the simulations on various HPC resources, including Anvil. Machi, Hoops, and Chen, along with the rest of the team, then work to optimize and calibrate the parameters needed for successful simulations, ensuring that the simulations are set to provide the answers that they need.
Another crucial member of the team is Parantapa Bhattacharya, a research scientist at NSSAC. Due to the volume of scenarios the team needs to model, they cannot rely on one supercomputing system to complete all their simulations. Outside of the Anvil cluster, the team utilized a UVA local system named Rivanna, as well as Bridges, a supercomputer run by the Pittsburgh Supercomputing Center. Bhattacharya developed an efficient pipeline to manage the team’s resource usage, ensuring that the individual simulations get access to the resources they need. “My job,” says Bhattacharya, “is to make sure the simulation runs fast, is scalable, and we are using the resources we get as efficiently as possible.”
Here is a snapshot of how the process typically works:
- The CDC’s Scenario Modeling Hub sends the team a “What-If” scenario that needs to be simulated.
- The team creates the agent-based model. These models need to be optimized and calibrated.
- The team runs calibration and optimization simulations on their local UVA HPC resource, Rivanna. This allows them to figure out what they need for the full simulations.
- The full simulation, depending on the computational needs based on the parameters of the scenario, is then run on a more powerful HPC cluster, e.g., Anvil.
- The results from the full simulation are then gathered, analyzed, and compiled in a report, which is sent back to the CDC’s Scenario Modeling Hub.
Normally, experiments such as this could take a significant amount of time to complete. On the one hand, if you do not have access to a powerful enough HPC resource, it could take days or weeks to simply run a simulation, not to mention the amount of time it would take to interpret the terabytes of data obtained from agent-based models. The power and speed of the Anvil supercomputer proved to be extremely useful in this regard. On the other hand, even if you have access to the fastest supercomputer in the world, getting the actual timeslot on the machine to run your experiment could take way longer than is required for pandemic response situations. Oftentimes the information needed by the authorities has a very short turnaround time, and waiting in a queue for such projects is simply not an option. This is where Anvil truly shined.
The Anvil team made it a point to prioritize computing time for the NSSAC team so that the information needed to optimize vaccination strategies or minimize infections and hospitalizations was quickly delivered to the appropriate officials. This expedited process allowed the decision-makers to develop science-based responses based on real-time data, which is crucial during an evolving pandemic.
According to Machi, “Anvil was just nice to use—it was easy to get access to, and the team was great to work with, so we were able to get a configuration set that worked for our style of pipeline really well. A lot of jobs that get put onto the clusters will be just single jobs that can run, and however long they take to make it into the queue and complete is fine, but in the case of these large-scale simulations, we are doing coordinated jobs, often with a relatively small window before things are due. So if we leave ourselves to the mercy of the scheduler, then we may never get them completed in time. We got into Anvil because of this need to have multiple clusters and stuck with it because it was convenient to use and worked well for our work.”
It’s clear that having quick access to powerful HPC resources is of the utmost importance to pandemic response efforts, not only for addressing COVID-19, but also for any future pandemics that may arise. As Dr. Marathe succinctly put it:
“We need high-performance computing systems…We were here to support an evolving pandemic, in terms of operational response. This is not just a science exercise where we can wait for a while, run some experiments, do some analysis, make some interesting scientific findings, etc. We do that too, but that’s not the primary purpose. The primary purpose was to support our state, local, and federal officials as they were responding to a pandemic. And time is absolutely critical. If they need an answer in three days, then they need an answer in three days. After seven days, the results may not be that useful. So what we needed was access to [HPC] machines that were relatively easy to use, flexible in their usage, and could support this operational real-time requirement. I think this is a central question that we all have to solve should we want to prepare for the next pandemic, and I think that you folks [at Anvil] did a tremendous job in supporting our desire, and I would say, the desire of federal agencies.”
More information about the Network Systems Science and Advanced Computing (NSSAC) group’s research can be seen at https://nssac.github.io/
If you would like to learn more, information about the Anvil supercomputer can be found on Purdue’s Anvil Website.
For more information regarding HPC and how it can help you, please visit our “Why HPC?” page.
Anvil is funded under NSF award No. 2005632. Researchers may request access to Anvil via the ACCESS allocations process.
References
- Marathe M, Vullikanti AKS. Computational epidemiology. Communications of the ACM. 2013;56(7):88-96. doi:https://doi.org/10.1145/2483852.2483871
- https://nssac.github.io/
- Bhattacharya, et al. “AI-Driven Agent-Based Models to Study the Role of Vaccine Acceptance in Controlling COVID-19 Spread in the US.” 2021IEEE International Conference on Big Data (Big Data). 2021
Written by: Jonathan Poole, poole43@purdue.edu