Purdue research team uses 100 RCAC GPUs to create urban representations of 330 US cities
In a multidisciplinary endeavor that has far-reaching implications for computer science, urban planning, digital urban forestry, ecological sciences, and related domains, a team led by Daniel Aliaga, associate professor of computer science, has leveraged the power of 100 of the Rosen Center for Advanced Computing (RCAC)’s GPUs to advance the field of deep generation of urban-related content.
Aliaga, along with Adnan Firoze, Liu He, Aocheng Li and Nikhil Makkar, all doctoral degree candidates from the same department, used the GPUs to create urban representations for 330 cities across the United States, with a significant emphasis on sustainability and the cities of the future.
The GPUs, on RCAC’s Gilbreth community cluster, were added as part of RCAC’s recent investments to better support researchers in the fields of AI and machine learning.
“The availability of the GPUs enabled us to improve algorithm development as well as run the model to enable creation of the results,” said Aliaga.
Aliaga and his team embarked on three interlinked projects using the computational power of the GPUs: urban forestry, urban layouts and historical urban layouts.
Urban Forestry
The team used the RCAC GPUs to detect trees in all of the target cities, resulting in 273 million trees throughout 8.2 million acres of urban space. The GPUs helped to explore hyperparameters and train the underlying models. Once trained, the actual inference process from satellite imagery is relatively fast. Evaluation tests demonstrated the accuracy was in the 85-97% range.
Urban Layouts
The researchers also used the GPUs to generate urban layouts from sparse data. In particular, the GPUs enabled the team to explore hyperparameters including clustering and sampling strategies to understand the huge design space and arrive at a trained deep engine that using only 2-4% of the urban layout information of a city (such as building locations, sizes and heights) can generate the entire urban layout with only a few meters, or percent, of error. The GPUs were used to optimize more than one billion parameters, using about 100 hours wall time, resulting in position errors of less than two meters, area errors of less than 20 square meters, height errors of less than half a meter, and almost zero building count error for several million buildings nationwide.
Historical Urban Layouts
The team is also using the GPUs to help in-progress research of pluralistic image completion and infilling which is useful for archaeological sites, among other applications. Unlike a current city, an ancient archaeological site only has a small percentage of the site remaining. However, this is a similar inference setup, so the same methodology can be applied. Initial results from sites in Peru, Greece, and Turkey are quite promising.
Aliaga expressed his excitement over the accomplishments of the team, stating, "Our research has pushed the boundaries of what is possible in generating urban-related content. The utilization of the RCAC GPUs has allowed us to achieve unprecedented milestones and significantly contribute to various fields, from computer science to ecological sciences."
“This use case germinated in a discussion at the RCAC Digital Twins symposium where both Daniel Aliaga and Songlin Fei presented on these three applications and their growing computational needs,” said RCAC research scientist Rajesh Kalyanam.
“It coincided with our expansion of Gilbreth and seemed like the perfect opportunity to explore the possibilities of researchers having access to this unprecedented scale of GPU acceleration at Purdue.”
“This project demonstrates a unique capacity at Purdue to push the boundaries of science via computing. The Rosen Center is proud to have contributed to this exciting research project and grateful for the investments in our infrastructure that have enabled us to support projects such as this,” adds Arman Pazouki, director of scientific applications for RCAC.
“We are excited that this project offers a model for how to enable future research in the fields of AI and machine learning.”
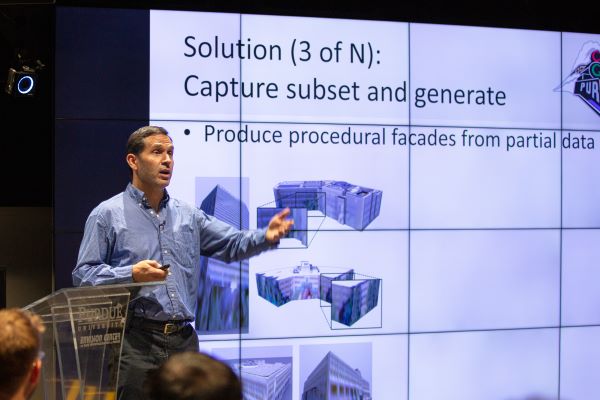
This pioneering research exemplifies the power of cutting-edge technology and collaborative interdisciplinary efforts, paving the way for a future where urban content generation becomes increasingly sophisticated and impactful.
With the recent expansion, the Gilbreth GPUs have an aggregate peak performance of 32 single-precision PetaFLOPs (one quadrillion operations per second) – doubling Gilbreth’s previous AI performance. Over the last year, Gilbreth has supported 98 principal investigators from 25 departments, enabling research ranging from computer science and electrical and computer engineering, to healthcare, energetics, and materials science.
The added resources are just a part of RCAC’s ongoing investment in supporting researchers performing AI and machine learning work. Along with the additional hardware, RCAC has full-time research scientists with AI and machine learning expertise, who offer training opportunities and are available to partner with faculty on proposals.
To learn more about Gilbreth and other Research Computing resources, contact rcac-help@purdue.edu.