Anvil assists in AI research to help boost pedestrian infrastructure
Researchers from Purdue University used the Anvil supercomputer in their quest to automate sidewalk identification from Google Street View images and develop sidewalk maps for neighborhoods using computer vision techniques.
Omar Faruqe Hamim is a Graduate Research Assistant at the Lyles School of Civil Engineering at Purdue University. He recently used Anvil to conduct research involving advanced computer vision techniques and machine learning algorithms, a subsect of artificial intelligence (AI). Hamim worked alongside Surendra Reddy Kancharla, a postdoctoral researcher, and under the supervision of Professor Satish Ukkusuri. Together, the team successfully created a deep learning architecture-based semantic segmentation model for mapping roads and sidewalks in developing countries from remotely sensed, open-sourced data. They were then able to automate the process of inventorying sidewalks by creating sidewalk maps of the study area on a neighborhood scale, based on the output from the developed model. All of this helps develop pedestrian infrastructure by enabling a relatively quick and efficient method of creating and maintaining a sidewalk inventory that local authorities can use for project planning.
Sidewalk mapping is an often overlooked yet crucial part of developing people-friendly cities. Walking is a major mode of transportation in urban areas, with benefits ranging from decreased fossil fuel consumption to lower obesity rates and increased quality of life and well-being. Unfortunately, without appropriately developed pedestrian infrastructure, walking in urban areas can be unsafe or even unattainable. For these reasons, a major focus for urban planners and local authorities is to improve the pedestrian infrastructure within towns and cities. However, as is the case with any resource-dependant improvement project, you have to know what you’ve got before you can fix it. An updated and accurate inventory of sidewalks is essential for pedestrian infrastructure planning. Without such an inventory, decision-makers won’t be able to identify where improvements should be made.
Many cities, in both developed and developing countries, do not have adequate sidewalk inventories. This is due to the fact that obtaining reliable and accurate data on roads and sidewalks has traditionally been a difficult and time-consuming task, requiring a hands-on approach of either sending people out to survey in-person, or having someone look through aerial images and manually mark out sidewalks. To overcome this obstacle, researchers have recently turned to the use of Google Street View (GSV) images and deep learning models to create sidewalk inventories, but this work has mainly focused on North American and European cities, in which the pedestrian infrastructure looks much different than in areas such as Southeast Asia. As such, any model created and trained on images from the former is inadequate at accurately assessing infrastructure in the latter. Hamim wanted to help correct this disparity in research, so he set his sights on Dhaka, the capital city of Bangladesh, and got to work on Anvil.
“Initially,” says Hamim, “we used a semantic segmentation model trained on Cityscapes and Mapillary images to identify sidewalks in Dhaka. But the problem with those datasets is that they were collected from Western countries, so the pedestrian infrastructure is very different from Bangladesh’s, and that led to a lot of misclassifications. So we knew that the model would not work for Dhaka.”
The first step was to improve the performance of the original, pre-trained deep-learning model (HRNet + OCR) by incorporating local images. Hamim and the team extracted over 2,500 GSV images from four distinct areas in Dhaka (one low, medium, and high-income zone, plus one test zone). These images were then annotated with labels of road, sidewalk, building, and wall. Next, the newly annotated images were used to retrain the original semantic segmentation model. Incorporating local images into the pre-trained model proved successful, drastically increasing its performance. Hamim was then able to use this data, along with correlating GPS data, to create comprehensive sidewalk maps of each area. The results of his work can be viewed below:
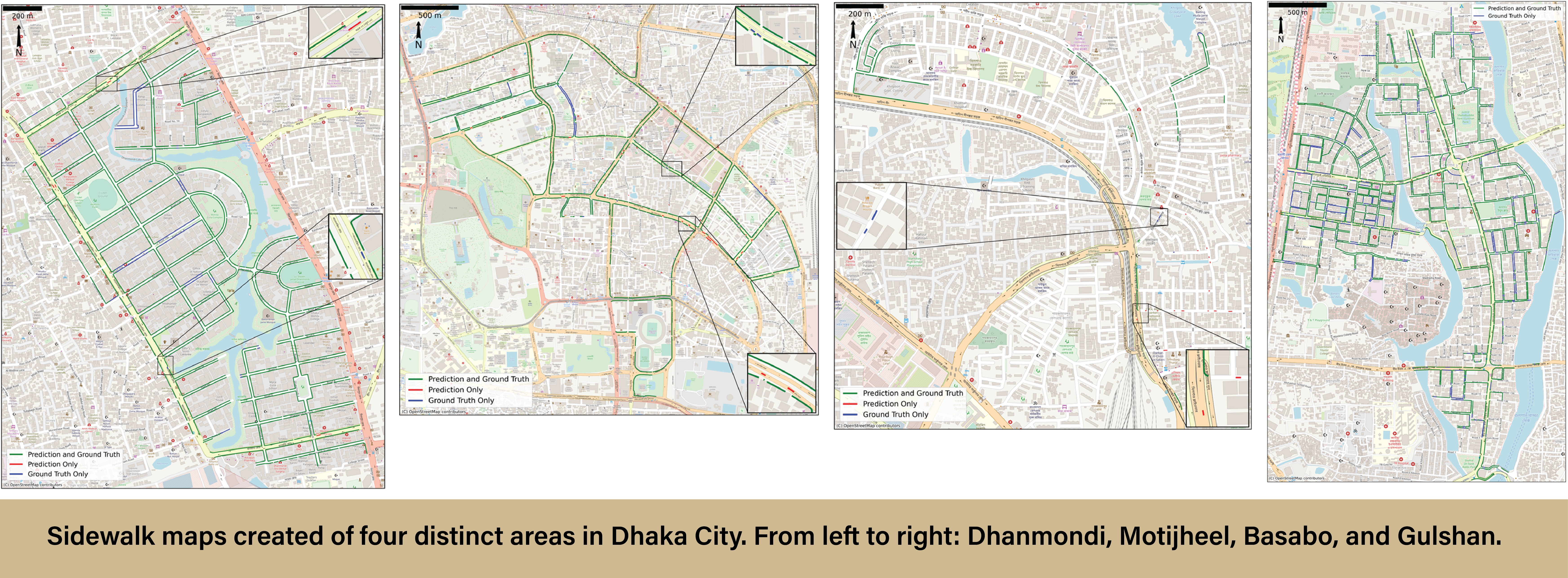
Now that Hamim has successfully created and proven the efficiency of a deep learning model for use in Southeast Asian countries, other researchers can use his model and methods to develop sidewalk maps of their respective locations.
The research conducted by Hamim and the rest of the team is computationally intensive, and, without a supercomputer, would likely never have been completed. The team chose Anvil for their project specifically due to their need for a large amount of GPUs.
“So we used HR net plus OCR,” says Hamim, “that's the semantic segmentation model. It was state-of-the-art at the time of doing the research, but the problem with that model was that it had millions of parameters and we needed a large number of GPUs to use it. So Professor Ukkusuri contacted Rajesh [Kalyanam, Senior Research Scientist at RCAC] and he helped us get set up on Anvil. We trained our model there and were able to reduce our computational time while also increasing the pixel size of our images. In our case, the images were 640 x 640 pixels, but on our lab computer we were only able to use a random pixel count of 128 x 128. On Anvil we were able to capture the whole image, which increased the performance of our model. So without the GPUs on Anvil, I would not have been able to train the model completely.”
Hamim was thrilled with the overall performance of the Anvil supercomputer, and very happy with how his AI model performed. In fact, he went on to use the newly created deep learning model to rewrite another journal publication that calculates the perceived pedestrian road safety in urban areas. To read about this project, please visit: https://www.sciencedirect.com/science/article/abs/pii/S0001457523004475?via%3Dihub
To learn more about Hamim’s initial AI modeling research, read his Sage Journals publication here: https://journals.sagepub.com/doi/10.1177/23998083231200445
Want to know more about HPC and how it can help you? Please visit our “Why HPC?” page.
Anvil is Purdue University’s most powerful supercomputer, providing researchers from diverse backgrounds with advanced computing capabilities. Built through a $10 million system acquisition grant from the National Science Foundation (NSF), Anvil supports scientific discovery by providing resources through the NSF’s Advanced Cyberinfrastructure Coordination Ecosystem: Services & Support (ACCESS), a program that serves tens of thousands of researchers across the United States.
Researchers may request access to Anvil via the ACCESS allocations process. More information about Anvil is available on Purdue’s Anvil website. Anyone with questions should contact anvil@purdue.edu. Anvil is funded under NSF award No. 2005632.
Written by: Jonathan Poole, poole43@purdue.edu