Ashish
News & Events
- There are no upcoming Student Events at this time.
Meet Your Mentor!
We sat down with our 2024 Anvil REU Mentors to discuss their role at RCAC, what their REU students will be working on, and much, much more. Keep reading below to learn more about your mentor.
Ashish
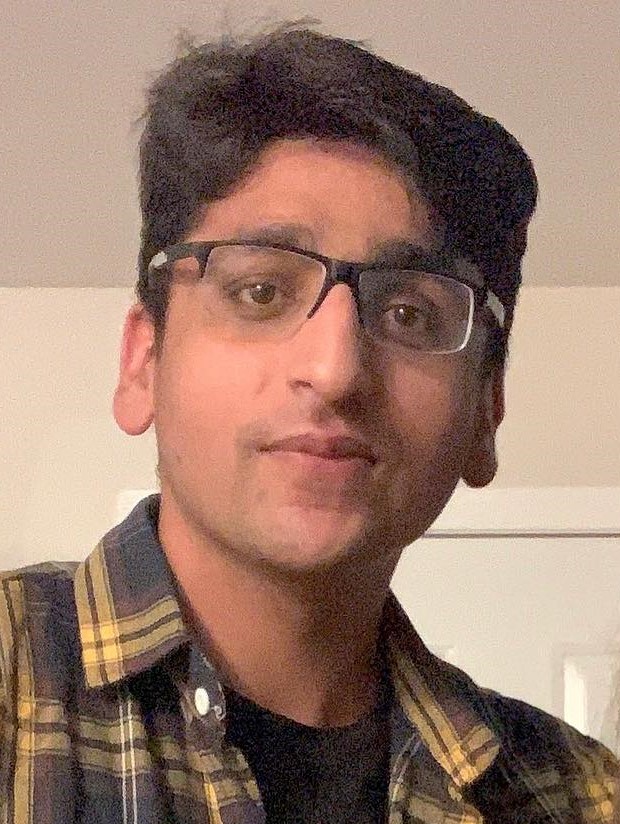
Please introduce yourself:
I am Ashish, a Senior Research Data Scientist at RCAC. My professional journey is marked by a solid statistics and machine learning foundation underpinning my data science expertise. I specialize in analyzing complex data sets, applying statistical models, and leveraging machine learning techniques to extract meaningful insights and drive innovation in my field.
What do you do?
As a Senior Research Data Scientist, I am responsible for designing and leading data analysis projects that require a deep understanding of statistics and machine learning. My job involves developing advanced predictive models, performing rigorous statistical analysis, and interpreting data to inform strategic decisions. I also stay at the forefront of advancements in AI and data science, ensuring that the latest research and best practices in these domains guide my work
Why would I come to you for help?
Individuals often seek my expertise for my extensive statistics and machine learning background, which is crucial for tackling complex data science challenges. Whether it's for advice on statistical methodologies, assistance in building and optimizing machine learning models, or insights into data-driven decision making, my comprehensive knowledge and practical experience make me a valuable resource. Moreover, my ability to clearly communicate complex technical concepts to diverse audiences further enhances my effectiveness as a mentor and collaborator in the field of data science.
What’s one professional skill you’re currently working on?
Currently, I am focusing on enhancing my deep learning skills, particularly in neural network architecture design. While I have a robust foundation in machine learning and statistics, the evolving landscape of deep learning presents new challenges and opportunities. By deepening my knowledge and practical expertise in this area, I aim to apply these advanced techniques to more complex and nuanced data problems, thereby expanding my capabilities in cutting-edge data science research and applications.
What’s your go-to productivity trick?
My go-to productivity trick is time blocking. I allocate specific blocks of time for different tasks and focus solely on one task during its allotted time. This approach helps me avoid multitasking and distractions, allowing me to dive deeply into complex data analysis or research without interruption. By dedicating focused time to each task, I ensure that I am productive and efficient, making the most of my workday.
What behavior or personality trait do you most attribute your success to, and why?
The trait I most attribute my success to is my curiosity. In the ever-evolving field of data science and AI, staying curious has driven me to learn and adapt continuously. My curiosity leads me to explore new methodologies, experiment with different techniques, and remain open to innovative ideas. This has not only kept my work dynamic and engaging but has also allowed me to stay ahead in a field where technological advancements happen rapidly. My success in data science is largely due to this relentless pursuit of knowledge and understanding, fueled by my inherent curiosity.
What led you to this career?
1). Academic Background and Interest in Mathematics and Statistics: My interest in data science began with a strong academic background in mathematics and statistics. I was always fascinated by the power of numbers and the insights they can reveal when analyzed correctly. This led me to pursue higher education in fields closely related to data analysis and statistical modeling.
2). Curiosity and Passion for Technology and Data: A natural curiosity and passion for technology and its applications in data drove me toward a career in data science. I was intrigued by how data could be used to solve real-world problems, predict outcomes, and inform decision-making processes. The interdisciplinary nature of data science, blending computer science with statistics, was particularly appealing to me
What are you currently excited about in your job?
I am particularly excited about the emerging technologies in AI and machine learning. The rapid advancements in areas like deep learning, neural networks, and natural language processing are opening up new possibilities for data analysis and predictive modeling. It's thrilling to explore these cutting-edge technologies and think about how they can be applied to solve complex problems.
What’s the biggest misconception people have about your position?
A common misconception about my role as a Senior Research Data Scientist is that it's solely focused on crunching numbers and running algorithms. While data analysis and algorithm development are certainly key aspects of the job, there's much more to it. People often overlook the importance of understanding the business context, formulating the right questions, and interpreting the results in a meaningful way. Data science is not just about applying statistical models or machine learning techniques; it's about using these tools to extract insights that can inform decision-making and solve real-world problems. Additionally, effective communication and collaboration with stakeholders and team members are crucial components of the role, which go beyond the technical aspects of data analysis.
Why did you decide to become a mentor for the REU program?
1). Passion for Teaching and Sharing Knowledge: I have always been passionate about teaching and sharing my knowledge with others. Becoming a mentor for the REU program allows me to guide and inspire undergraduates who are just beginning their journey in data science and research. It's an opportunity for me to impart the lessons I've learned and help students navigate the complexities of this field.
2). Contributing to the Growth of the Field: Data science is a rapidly evolving field, and by mentoring young researchers, I feel I can contribute to its growth. Nurturing the next generation of data scientists is crucial for the continued advancement of our field, and I take pride in being able to play a part in that.
3). Personal Fulfillment and Professional Development: Mentoring is incredibly fulfilling for me. It challenges me to stay current with the latest developments and think critically about the fundamentals of data science and machine learning. This not only benefits my mentees but also aids my own professional development.
4). Building a Stronger Research Community: I believe in the power of collaboration and community in research. By mentoring in the REU program, I can help build a stronger, more interconnected research community. It's rewarding to see students grow and contribute to the field, and I enjoy being a part of their journey.
5). Giving Back to the Academic Community: Throughout my career, I've been fortunate to have mentors who have significantly influenced my professional path. Becoming a mentor myself is a way of giving back to the academic community that has supported me. It's my way of paying it forward to the next generation of researchers.
What do you hope the REU student will get from this experience?
I hope students in the REU program gain a deeper understanding of data science and research practices. I want them to develop the ability to think independently, ask insightful questions, and seek innovative solutions to complex problems. Additionally, I hope they leave the program with a newfound confidence in their abilities and a clear vision of their future in the field
How can this help them in their college career?
Participation in the REU program can significantly enhance a student's college career. It provides a strong foundation in research that can be invaluable for their coursework and future academic projects. The experience also makes them more competitive for scholarships, advanced studies, and other academic opportunities. Furthermore, it fosters a sense of academic curiosity and a drive for continuous learning.
How can this help them in their professional career?
The skills and experience gained from the REU program are highly beneficial in a professional setting. Students learn to work collaboratively in teams, communicate effectively, and manage complex projects—skills that are in high demand in the workplace. The hands-on research experience also makes them attractive candidates for future employers and can open doors to opportunities in both academia and industry. Additionally, the networking opportunities provided by the program can lead to professional relationships that last well into their careers.
What value do you get from being a mentor?
1). Personal Satisfaction and Fulfillment: Mentoring in the REU program offers a great sense of personal satisfaction and fulfillment. Seeing students grow, learn, and develop their research skills is incredibly rewarding. It's gratifying to know that I am contributing to the development of future scientists and researchers.
2). Professional Development and Learning: Being a mentor keeps me engaged with the latest trends and developments in data science and machine learning. It challenges me to stay current and deepens my understanding of the field. Additionally, explaining complex concepts to students helps reinforce my own knowledge and sometimes provides new insights or perspectives.
3). Networking and Collaboration Opportunities: The REU program allows me to connect with other professionals and researchers in my field. These connections can lead to collaborative research projects, joint publications, and other professional opportunities. Networking with peers and being part of a community focused on research and education is highly beneficial.
4). Enhancing Teaching and Communication Skills: Mentoring students helps improve my teaching and communication skills. It requires me to explain complex topics clearly and effectively, tailor my communication to different learning styles, and provide constructive feedback. These skills are valuable in my professional life, especially when collaborating with teams or presenting research findings.
5). Contributing to the Field: By mentoring in the REU program, I am able to contribute to the field of data science in a meaningful way. Helping to train the next generation of researchers ensures the continued growth and advancement of the field. It's rewarding to be a part of shaping the future of data science and machine learning.