Specific Applications
The following examples demonstrate job submission files for some common real-world applications. See the Generic SLURM Examples section for more examples on job submissions that can be adapted for use.
Gaussian
Gaussian is a computational chemistry software package which works on electronic structure. This section illustrates how to submit a small Gaussian job to a Slurm queue. This Gaussian example runs the Fletcher-Powell multivariable optimization.
Prepare a Gaussian input file with an appropriate filename, here named myjob.com. The final blank line is necessary:
#P TEST OPT=FP STO-3G OPTCYC=2
STO-3G FLETCHER-POWELL OPTIMIZATION OF WATER
0 1
O
H 1 R
H 1 R 2 A
R 0.96
A 104.
To submit this job, load Gaussian then run the provided script, named subg16. This job uses one compute node with 16 processor cores:
module load gaussian16
subg16 myjob -N 1 -n 16 --gres=gpu:1
View job status:
squeue -u myusername
View results in the file for Gaussian output, here named myjob.log. Only the first and last few lines appear here:
Entering Gaussian System, Link 0=/apps/cent7/gaussian/g16-A.03/g16-haswell/g16/g16
Initial command:
/apps/cent7/gaussian/g16-A.03/g16-haswell/g16/l1.exe /scratch/gilbreth/myusername/gaussian/Gau-7781.inp -scrdir=/scratch/gilbreth/myusername/gaussian/
Entering Link 1 = /apps/cent7/gaussian/g16-A.03/g16-haswell/g16/l1.exe PID= 7782.
Copyright (c) 1988,1990,1992,1993,1995,1998,2003,2009,2016,
Gaussian, Inc. All Rights Reserved.
.
.
.
Job cpu time: 0 days 0 hours 3 minutes 28.2 seconds.
Elapsed time: 0 days 0 hours 0 minutes 12.9 seconds.
File lengths (MBytes): RWF= 17 Int= 0 D2E= 0 Chk= 2 Scr= 2
Normal termination of Gaussian 16 at Tue May 1 17:12:00 2018.
real 13.85
user 202.05
sys 6.12
Machine:
gilbreth-a012.rcac.purdue.edu
gilbreth-a012.rcac.purdue.edu
gilbreth-a012.rcac.purdue.edu
gilbreth-a012.rcac.purdue.edu
gilbreth-a012.rcac.purdue.edu
gilbreth-a012.rcac.purdue.edu
gilbreth-a012.rcac.purdue.edu
gilbreth-a012.rcac.purdue.edu
Link to section 'Examples of Gaussian SLURM Job Submissions' of 'Gaussian' Examples of Gaussian SLURM Job Submissions
Submit job using 16 processor cores on a single node:
subg16 myjob -N 1 -n 16 --gres=gpu:1 -t 24:00:00 -A standby
Submit job using 16 processor cores on each of 2 nodes:
subg16 myjob -N 2 --ntasks-per-node=16 --gres=gpu:2 -t 24:00:00 -A standby
To submit a bash job, a submit script sample looks like:
#!/bin/bash
#SBATCH -A myqueuename # Queue name(use 'slist' command to find queues' name)
#SBATCH --nodes=1 # Total # of nodes
#SBATCH --ntasks=64 # Total # of MPI tasks
#SBATCH --gpus-per-node=1 # Total # of GPUs
#SBATCH --time=1:00:00 # Total run time limit (hh:mm:ss)
#SBATCH -J myjobname # Job name
#SBATCH -o myjob.o%j # Name of stdout output file
#SBATCH -e myjob.e%j # Name of stderr error file
module load gaussian16
g16 < myjob.com
For more information about Gaussian:
Machine Learning
We support several common machine learning (ML) frameworks on the community clusters through pre-installed modules. The collection of these pre-installed ML modules is referred to as ml-toolkit throughout this documentation. Currently, the following libraries are included in ML-Toolkit.
caffe cntk gym keras
mxnet opencv pytorch
tensorflow tflearn theano
Note that managing dependencies with ML applications can be non-trivial, therefore, we recommend users start by using ml-toolkit. If a custom installation is required after trying ml-toolkit, make sure to read documentation carefully.
ML-Toolkit
A set of pre-installed popular machine learning (ML) libraries, called ML-Toolkit is maintained on Gilbreth. These are Anaconda/Python-based distributions of the respective libraries. Currently, applications are supported for Python 2 and 3. Detailed instructions for searching and using the installed ML applications are presented below.
Link to section 'Instructions for using ML-Toolkit Modules' of 'ML-Toolkit' Instructions for using ML-Toolkit Modules
Link to section 'Find and Use Installed ML Packages' of 'ML-Toolkit' Find and Use Installed ML Packages
To search or load a machine learning application, you must first load one of the learning modules. The learning module loads the prerequisites (such as anaconda and cudnn) and makes ML applications visible to the user.
Step 1. Find and load a preferred learning module. Several learning modules may be available, corresponding to a specific Python version and whether the ML applications have GPU support or not. Running module load learning without specifying a version will load the version with the most recent python version. To see all available modules, run module spider learning then load the desired module.
Step 2. Find and load the desired machine learning libraries
ML packages are installed under the common application name ml-toolkit-X, where X can be cpu or gpu.
You can use the module spider ml-toolkit command to see all options and versions of each library.
Load the desired modules using the module load command. Note that both CPU and GPU options may exist for many libraries, so be sure to load the correct version. For example, if you wanted to load the most recent version of PyTorch for CPU, you would run module load ml-toolkit-cpu/pytorch
caffe cntk gym keras mxnet
opencv pytorch tensorflow tflearn theano
Step 3. You can list which ML applications are loaded in your environment using the command module list
Link to section 'Verify application import' of 'ML-Toolkit' Verify application import
Step 4. The next step is to check that you can actually use the desired ML application. You can do this by running the import command in Python. The example below tests if PyTorch has been loaded correctly.
python -c "import torch; print(torch.__version__)"
If the import operation succeeded, then you can run your own ML code. Some ML applications (such as tensorflow) print diagnostic warnings while loading -- this is the expected behavior.
If the import fails with an error, please see the troubleshooting information below.
Step 5. To load a different set of applications, unload the previously loaded applications and load the new desired applications. The example below loads Tensorflow and Keras instead of PyTorch and OpenCV.
module unload ml-toolkit-cpu/opencv
module unload ml-toolkit-cpu/pytorch
module load ml-toolkit-cpu/tensorflow
module load ml-toolkit-cpu/keras
Link to section 'Troubleshooting' of 'ML-Toolkit' Troubleshooting
ML applications depend on a wide range of Python packages and mixing multiple versions of these packages can lead to error. The following guidelines will assist you in identifying the cause of the problem.
- Check that you are using the correct version of Python with the command python --version. This should match the Python version in the loaded anaconda module.
- Start from a clean environment. Either start a new terminal session or unload all the modules using module purge. Then load the desired modules following Steps 1-2.
- Verify that PYTHONPATH does not point to undesired packages. Run the following command to print PYTHONPATH: echo $PYTHONPATH. Make sure that your Python environment is clean. Watch out for any locally installed packages that might conflict.
- If you don't see GPU devices in your code, make sure that you are using the ml-toolkit-gpu/ modules and not using their cpu versions.
- ML applications often have dependency on specific versions of Cuda and CuDNN libraries. Make sure that you have loaded the required versions using the command: module list
- Note that Caffe has a conflicting version of PyQt5. So, if you want to use Spyder (or any GUI application that uses PyQt), then you should unload the caffe module.
- Use Google search to your advantage. Copy the error message in Google and check probable causes.
More examples showing how to use ml-toolkit modules in a batch job are presented in ML Batch Jobs guide.
Link to section 'Installation of Custom ML Libraries' of 'Custom ML Packages' Installation of Custom ML Libraries
While we try to include as many common ML frameworks and versions as we can in ML-Toolkit, we recognize that there are also situations in which a custom installation may be preferable. We recommend using conda-env-mod to install and manage Python packages. Please follow the steps carefully, otherwise you may end up with a faulty installation. The example below shows how to install TensorFlow in your home directory.
Link to section 'Install' of 'Custom ML Packages' Install
Step 1: Unload all modules and start with a clean environment.
module purge
Step 2: Load the anaconda module with desired Python version.
module load anaconda
Step 2A: If the ML application requires Cuda and CuDNN, load the appropriate modules. Be sure to check that the versions you load are compatible with the desired ML package.
module load cuda
module load cudnn
Many machine-learning packages (including PyTorch and TensorFlow) now provide installation pathways that include the full cudatoolkit within the environment, making it unnecessary to load these modules.
Step 3: Create a custom anaconda environment. Make sure the python version matches the Python version in the anaconda module.
conda-env-mod create -n env_name_here
Step 4: Activate the anaconda environment by loading the modules displayed at the end of step 3.
module load use.own
module load conda-env/env_name_here-py3.8.5
Step 5: Now install the desired ML application. You can install multiple Python packages at this step using either conda or pip.
For TensorFlow (as of 2024) the recommended approach is to use pip (see tensorflow.org/install/gpu).
pip install --ignore-installed 'tensorflow[and-cuda]'
For PyTorch the recommended approach is to use conda (see pytorch.org).
conda install pytorch torchvision torchaudio pytorch-cuda=11.8 -c pytorch -c nvidia
If the installation succeeded, you can now proceed to testing and using the installed application. You must load the environment you created as well as any supporting modules (e.g., anaconda) whenever you want to use this installation. If your installation did not succeed, please refer to the troubleshooting section below as well as documentation for the desired package you are installing.
Note that loading the modules generated by conda-env-mod has different behavior than conda create env_name_here followed by source activate env_name_here. After running source activate, you may not be able to access any Python packages in anaconda or ml-toolkit modules. Therefore, using conda-env-mod is the preferred way of using your custom installations.
Link to section 'Testing the Installation' of 'Custom ML Packages' Testing the Installation
-
Verify the installation by using a simple import statement, like that listed below for TensorFlow:
python -c "import tensorflow as tf; print(tf.__version__);"
Note that a successful import of TensorFlow will print a variety of system and hardware information. This is expected.
If importing the package leads to errors, be sure to verify that all dependencies for the package have been managed, and the correct versions installed. Dependency issues between python packages are the most common cause for errors. For example, in TF, conflicts with the h5py or numpy versions are common, but upgrading those packages typically solves the problem. Managing dependencies for ML libraries can be non-trivial.
-
Next, we can test using our installation of TensorFlow for a GPU run. For this we shall use the matrix multiplication example from Tensorflow documentation.
# filename: matrixmult.py import tensorflow as tf print("Num GPUs Available: ", len(tf.config.list_physical_devices('GPU'))) tf.debugging.set_log_device_placement(True) # Place tensors on the CPU with tf.device('/CPU:0'): a = tf.constant([[1.0, 2.0, 3.0], [4.0, 5.0, 6.0]]) b = tf.constant([[1.0, 2.0], [3.0, 4.0], [5.0, 6.0]]) # Run on the GPU c = tf.matmul(a, b) print(c)
-
Run the example
$ python matrixmult.py
-
This will produce an output like:
Num GPUs Available: 3 2022-07-25 10:33:23.358919: I tensorflow/core/platform/cpu_feature_guard.cc:193] This TensorFlow binary is optimized with oneAPI Deep Neural Network Library (oneDNN) to use the following CPU instructions in performance-critical operations: AVX2 AVX512F FMA To enable them in other operations, rebuild TensorFlow with the appropriate compiler flags. 2022-07-25 10:33:26.223459: I tensorflow/core/common_runtime/gpu/gpu_device.cc:1532] Created device /job:localhost/replica:0/task:0/device:GPU:0 with 22183 MB memory: -> device: 0, name: NVIDIA A30, pci bus id: 0000:3b:00.0, compute capability: 8.0 2022-07-25 10:33:26.225495: I tensorflow/core/common_runtime/gpu/gpu_device.cc:1532] Created device /job:localhost/replica:0/task:0/device:GPU:1 with 22183 MB memory: -> device: 1, name: NVIDIA A30, pci bus id: 0000:af:00.0, compute capability: 8.0 2022-07-25 10:33:26.228514: I tensorflow/core/common_runtime/gpu/gpu_device.cc:1532] Created device /job:localhost/replica:0/task:0/device:GPU:2 with 22183 MB memory: -> device: 2, name: NVIDIA A30, pci bus id: 0000:d8:00.0, compute capability: 8.0 2022-07-25 10:33:26.933709: I tensorflow/core/common_runtime/eager/execute.cc:1323] Executing op MatMul in device /job:localhost/replica:0/task:0/device:GPU:0 2022-07-25 10:33:28.181855: I tensorflow/stream_executor/cuda/cuda_blas.cc:1786] TensorFloat-32 will be used for the matrix multiplication. This will only be logged once. tf.Tensor( [[22. 28.] [49. 64.]], shape=(2, 2), dtype=float32)
-
For more details, please refer to Tensorflow User Guide.
Link to section 'Troubleshooting' of 'Custom ML Packages' Troubleshooting
In most situations, dependencies among Python modules lead to errors. If you cannot use a Python package after installing it, please follow the steps below to find a workaround.
- Unload all the modules.
module purge
- Clean up PYTHONPATH.
unset PYTHONPATH
- Next load the modules, e.g., anaconda and your custom environment.
module load anaconda module load use.own module load conda-env/env_name_here-py3.8.5
- For GPU-enabled applications, you may also need to load the corresponding cuda/ and cudnn/ modules.
- Now try running your code again.
- A few applications only run on specific versions of Python (e.g. Python 3.6). Please check the documentation of your application if that is the case.
- If you have installed a newer version of an ml-toolkit package (e.g., a newer version of PyTorch or Tensorflow), make sure that the ml-toolkit modules are NOT loaded. In general, we recommend that you don't mix ml-toolkit modules with your custom installations.
- GPU-enabled ML applications often have dependencies on specific versions of Cuda and CuDNN. For example, Tensorflow version 1.5.0 and higher needs Cuda 9. Please check the application documentation about such dependencies.
Link to section 'Tensorboard' of 'Custom ML Packages' Tensorboard
- You can visualize data from a Tensorflow session using Tensorboard. For this, you need to save your session summary as described in the Tensorboard User Guide.
- Launch Tensorboard:
$ python -m tensorboard.main --logdir=/path/to/session/logs
- When Tensorboard is launched successfully, it will give you the URL for accessing Tensorboard.
<... build related warnings ...> TensorBoard 0.4.0 at http://gilbreth-a000.rcac.purdue.edu:6006
- Follow the printed URL to visualize your model.
- Please note that due to firewall rules, the Tensorboard URL may only be accessible from Gilbreth nodes. If you cannot access the URL directly, you can use Firefox browser in Thinlinc.
- For more details, please refer to the Tensorboard User Guide.
Link to section 'Running ML Code in a Batch Job' of 'ML Batch Jobs' Running ML Code in a Batch Job
Batch jobs allow us to automate model training without human intervention. They are also useful when you need to run a large number of simulations on the clusters. In the example below, we shall run a simple tensor_hello.py script in a batch job. We consider two situations: in the first example, we use the ML-Toolkit modules to run tensorflow, while in the second example, we use a custom installation of tensorflow (See Custom ML Packages page).
Link to section 'Using ML-Toolkit Modules' of 'ML Batch Jobs' Using ML-Toolkit Modules
Save the following code as tensor_hello.sub in the same directory where tensor_hello.py is located.
# filename: tensor_hello.sub
#SBATCH --nodes=1
#SBATCH --ntasks-per-node=1
#SBATCH --gres=gpu:1
#SBATCH --time=00:05:00
#SBATCH -A standby
#SBATCH -J hello_tensor
module purge
module load learning
module load ml-toolkit-gpu/tensorflow
module list
python tensor_hello.py
Link to section 'Using a Custom Installation' of 'ML Batch Jobs' Using a Custom Installation
Save the following code as tensor_hello.sub in the same directory where tensor_hello.py is located.
# filename: tensor_hello.sub
#SBATCH --nodes=1
#SBATCH --ntasks-per-node=1
#SBATCH --gres=gpu:1
#SBATCH --time=00:05:00
#SBATCH -A standby
#SBATCH -J hello_tensor
module purge
module load anaconda
module load cuda
module load cudnn
module load use.own
module load conda-env/my_tf_env-py3.8.5
module list
echo $PYTHONPATH
python tensor_hello.py
Link to section 'Running a Job' of 'ML Batch Jobs' Running a Job
Now you can submit the batch job using the sbatch command.
sbatch tensor_hello.sub
Once the job finishes, you will find an output file (slurm-xxxxx.out).
Matlab
MATLAB® (MATrix LABoratory) is a high-level language and interactive environment for numerical computation, visualization, and programming. MATLAB is a product of MathWorks.
MATLAB, Simulink, Compiler, and several of the optional toolboxes are available to faculty, staff, and students. To see the kind and quantity of all MATLAB licenses plus the number that you are currently using you can use the matlab_licenses command:
$ module load matlab
$ matlab_licenses
The MATLAB client can be run in the front-end for application development, however, computationally intensive jobs must be run on compute nodes.
The following sections provide several examples illustrating how to submit MATLAB jobs to a Linux compute cluster.
Matlab Script (.m File)
This section illustrates how to submit a small, serial, MATLAB program as a job to a batch queue. This MATLAB program prints the name of the run host and gets three random numbers.
Prepare a MATLAB script myscript.m, and a MATLAB function file myfunction.m:
% FILENAME: myscript.m
% Display name of compute node which ran this job.
[c name] = system('hostname');
fprintf('\n\nhostname:%s\n', name);
% Display three random numbers.
A = rand(1,3);
fprintf('%f %f %f\n', A);
quit;
% FILENAME: myfunction.m
function result = myfunction ()
% Return name of compute node which ran this job.
[c name] = system('hostname');
result = sprintf('hostname:%s', name);
% Return three random numbers.
A = rand(1,3);
r = sprintf('%f %f %f', A);
result=strvcat(result,r);
end
Also, prepare a job submission file, here named myjob.sub. Run with the name of the script:
#!/bin/bash
# FILENAME: myjob.sub
echo "myjob.sub"
# Load module, and set up environment for Matlab to run
module load matlab
unset DISPLAY
# -nodisplay: run MATLAB in text mode; X11 server not needed
# -singleCompThread: turn off implicit parallelism
# -r: read MATLAB program; use MATLAB JIT Accelerator
# Run Matlab, with the above options and specifying our .m file
matlab -nodisplay -singleCompThread -r myscript
myjob.sub
< M A T L A B (R) >
Copyright 1984-2011 The MathWorks, Inc.
R2011b (7.13.0.564) 64-bit (glnxa64)
August 13, 2011
To get started, type one of these: helpwin, helpdesk, or demo.
For product information, visit www.mathworks.com.
hostname:gilbreth-a001.rcac.purdue.edu
0.814724 0.905792 0.126987
Output shows that a processor core on one compute node (gilbreth-a001) processed the job. Output also displays the three random numbers.
For more information about MATLAB:
Implicit Parallelism
MATLAB implements implicit parallelism which is automatic multithreading of many computations, such as matrix multiplication, linear algebra, and performing the same operation on a set of numbers. This is different from the explicit parallelism of the Parallel Computing Toolbox.
MATLAB offers implicit parallelism in the form of thread-parallel enabled functions. Since these processor cores, or threads, share a common memory, many MATLAB functions contain multithreading potential. Vector operations, the particular application or algorithm, and the amount of computation (array size) contribute to the determination of whether a function runs serially or with multithreading.
When your job triggers implicit parallelism, it attempts to allocate its threads on all processor cores of the compute node on which the MATLAB client is running, including processor cores running other jobs. This competition can degrade the performance of all jobs running on the node.
When you know that you are coding a serial job but are unsure whether you are using thread-parallel enabled operations, run MATLAB with implicit parallelism turned off. Beginning with the R2009b, you can turn multithreading off by starting MATLAB with -singleCompThread:
$ matlab -nodisplay -singleCompThread -r mymatlabprogram
When you are using implicit parallelism, make sure you request exclusive access to a compute node, as MATLAB has no facility for sharing nodes.
For more information about MATLAB's implicit parallelism:
Profile Manager
MATLAB offers two kinds of profiles for parallel execution: the 'local' profile and user-defined cluster profiles. The 'local' profile runs a MATLAB job on the processor core(s) of the same compute node, or front-end, that is running the client. To run a MATLAB job on compute node(s) different from the node running the client, you must define a Cluster Profile using the Cluster Profile Manager.
To prepare a user-defined cluster profile, use the Cluster Profile Manager in the Parallel menu. This profile contains the scheduler details (queue, nodes, processors, walltime, etc.) of your job submission. Ultimately, your cluster profile will be an argument to MATLAB functions like batch().
For your convenience, a generic cluster profile is provided that can be downloaded: myslurmprofile.settings
Please note that modifications are very likely to be required to make myslurmprofile.settings work. You may need to change values for number of nodes, number of workers, walltime, and submission queue specified in the file. As well, the generic profile itself depends on the particular job scheduler on the cluster, so you may need to download or create two or more generic profiles under different names. Each time you run a job using a Cluster Profile, make sure the specific profile you are using is appropriate for the job and the cluster.
To import the profile, start a MATLAB session and select Manage Cluster Profiles... from the Parallel menu. In the Cluster Profile Manager, select Import, navigate to the folder containing the profile, select myslurmprofile.settings and click OK. Remember that the profile will need to be customized for your specific needs. If you have any questions, please contact us.
For detailed information about MATLAB's Parallel Computing Toolbox, examples, demos, and tutorials:
Parallel Computing Toolbox (parfor)
The MATLAB Parallel Computing Toolbox (PCT) extends the MATLAB language with high-level, parallel-processing features such as parallel for loops, parallel regions, message passing, distributed arrays, and parallel numerical methods. It offers a shared-memory computing environment running on the local cluster profile in addition to your MATLAB client. Moreover, the MATLAB Distributed Computing Server (DCS) scales PCT applications up to the limit of your DCS licenses.
This section illustrates the fine-grained parallelism of a parallel for loop (parfor) in a pool job.
The following examples illustrate a method for submitting a small, parallel, MATLAB program with a parallel loop (parfor statement) as a job to a queue. This MATLAB program prints the name of the run host and shows the values of variables numlabs and labindex for each iteration of the parfor loop.
This method uses the job submission command to submit a MATLAB client which calls the MATLAB batch() function with a user-defined cluster profile.
Prepare a MATLAB pool program in a MATLAB script with an appropriate filename, here named myscript.m:
% FILENAME: myscript.m
% SERIAL REGION
[c name] = system('hostname');
fprintf('SERIAL REGION: hostname:%s\n', name)
numlabs = parpool('poolsize');
fprintf(' hostname numlabs labindex iteration\n')
fprintf(' ------------------------------- ------- -------- ---------\n')
tic;
% PARALLEL LOOP
parfor i = 1:8
[c name] = system('hostname');
name = name(1:length(name)-1);
fprintf('PARALLEL LOOP: %-31s %7d %8d %9d\n', name,numlabs,labindex,i)
pause(2);
end
% SERIAL REGION
elapsed_time = toc; % get elapsed time in parallel loop
fprintf('\n')
[c name] = system('hostname');
name = name(1:length(name)-1);
fprintf('SERIAL REGION: hostname:%s\n', name)
fprintf('Elapsed time in parallel loop: %f\n', elapsed_time)
The execution of a pool job starts with a worker executing the statements of the first serial region up to the parfor block, when it pauses. A set of workers (the pool) executes the parfor block. When they finish, the first worker resumes by executing the second serial region. The code displays the names of the compute nodes running the batch session and the worker pool.
Prepare a MATLAB script that calls MATLAB function batch() which makes a four-lab pool on which to run the MATLAB code in the file myscript.m. Use an appropriate filename, here named mylclbatch.m:
% FILENAME: mylclbatch.m
!echo "mylclbatch.m"
!hostname
pjob=batch('myscript','Profile','myslurmprofile','Pool',4,'CaptureDiary',true);
wait(pjob);
diary(pjob);
quit;
Prepare a job submission file with an appropriate filename, here named myjob.sub:
#!/bin/bash
# FILENAME: myjob.sub
echo "myjob.sub"
hostname
module load matlab
unset DISPLAY
matlab -nodisplay -r mylclbatch
Submit the job as a single compute node with one processor core.
One processor core runs myjob.sub and mylclbatch.m.
Once this job starts, a second job submission is made.
myjob.sub
< M A T L A B (R) >
Copyright 1984-2013 The MathWorks, Inc.
R2013a (8.1.0.604) 64-bit (glnxa64)
February 15, 2013
To get started, type one of these: helpwin, helpdesk, or demo.
For product information, visit www.mathworks.com.
mylclbatch.mgilbreth-a000.rcac.purdue.edu
SERIAL REGION: hostname:gilbreth-a000.rcac.purdue.edu
hostname numlabs labindex iteration
------------------------------- ------- -------- ---------
PARALLEL LOOP: gilbreth-a001.rcac.purdue.edu 4 1 2
PARALLEL LOOP: gilbreth-a002.rcac.purdue.edu 4 1 4
PARALLEL LOOP: gilbreth-a001.rcac.purdue.edu 4 1 5
PARALLEL LOOP: gilbreth-a002.rcac.purdue.edu 4 1 6
PARALLEL LOOP: gilbreth-a003.rcac.purdue.edu 4 1 1
PARALLEL LOOP: gilbreth-a003.rcac.purdue.edu 4 1 3
PARALLEL LOOP: gilbreth-a004.rcac.purdue.edu 4 1 7
PARALLEL LOOP: gilbreth-a004.rcac.purdue.edu 4 1 8
SERIAL REGION: hostname:gilbreth-a001.rcac.purdue.edu
Elapsed time in parallel loop: 5.411486
To scale up this method to handle a real application, increase the wall time in the submission command to accommodate a longer running job. Secondly, increase the wall time of myslurmprofile by using the Cluster Profile Manager in the Parallel menu to enter a new wall time in the property SubmitArguments.
For more information about MATLAB Parallel Computing Toolbox:
Parallel Toolbox (spmd)
The MATLAB Parallel Computing Toolbox (PCT) extends the MATLAB language with high-level, parallel-processing features such as parallel for loops, parallel regions, message passing, distributed arrays, and parallel numerical methods. It offers a shared-memory computing environment with a maximum of eight MATLAB workers (labs, threads; versions R2009a) and 12 workers (labs, threads; version R2011a) running on the local configuration in addition to your MATLAB client. Moreover, the MATLAB Distributed Computing Server (DCS) scales PCT applications up to the limit of your DCS licenses.
This section illustrates how to submit a small, parallel, MATLAB program with a parallel region (spmd statement) as a MATLAB pool job to a batch queue.
This example uses the submission command to submit to compute nodes a MATLAB client which interprets a Matlab .m with a user-defined cluster profile which scatters the MATLAB workers onto different compute nodes. This method uses the MATLAB interpreter, the Parallel Computing Toolbox, and the Distributed Computing Server; so, it requires and checks out six licenses: one MATLAB license for the client running on the compute node, one PCT license, and four DCS licenses. Four DCS licenses run the four copies of the spmd statement. This job is completely off the front end.
Prepare a MATLAB script called myscript.m:
% FILENAME: myscript.m
% SERIAL REGION
[c name] = system('hostname');
fprintf('SERIAL REGION: hostname:%s\n', name)
p = parpool('4');
fprintf(' hostname numlabs labindex\n')
fprintf(' ------------------------------- ------- --------\n')
tic;
% PARALLEL REGION
spmd
[c name] = system('hostname');
name = name(1:length(name)-1);
fprintf('PARALLEL REGION: %-31s %7d %8d\n', name,numlabs,labindex)
pause(2);
end
% SERIAL REGION
elapsed_time = toc; % get elapsed time in parallel region
delete(p);
fprintf('\n')
[c name] = system('hostname');
name = name(1:length(name)-1);
fprintf('SERIAL REGION: hostname:%s\n', name)
fprintf('Elapsed time in parallel region: %f\n', elapsed_time)
quit;
Prepare a job submission file with an appropriate filename, here named myjob.sub. Run with the name of the script:
#!/bin/bash
# FILENAME: myjob.sub
echo "myjob.sub"
module load matlab
unset DISPLAY
matlab -nodisplay -r myscript
Run MATLAB to set the default parallel configuration to your job configuration:
$ matlab -nodisplay
>> parallel.defaultClusterProfile('myslurmprofile');
>> quit;
$
Once this job starts, a second job submission is made.
myjob.sub
< M A T L A B (R) >
Copyright 1984-2011 The MathWorks, Inc.
R2011b (7.13.0.564) 64-bit (glnxa64)
August 13, 2011
To get started, type one of these: helpwin, helpdesk, or demo.
For product information, visit www.mathworks.com.
SERIAL REGION: hostname:gilbreth-a001.rcac.purdue.edu
Starting matlabpool using the 'myslurmprofile' profile ... connected to 4 labs.
hostname numlabs labindex
------------------------------- ------- --------
Lab 2:
PARALLEL REGION: gilbreth-a002.rcac.purdue.edu 4 2
Lab 1:
PARALLEL REGION: gilbreth-a001.rcac.purdue.edu 4 1
Lab 3:
PARALLEL REGION: gilbreth-a003.rcac.purdue.edu 4 3
Lab 4:
PARALLEL REGION: gilbreth-a004.rcac.purdue.edu 4 4
Sending a stop signal to all the labs ... stopped.
SERIAL REGION: hostname:gilbreth-a001.rcac.purdue.edu
Elapsed time in parallel region: 3.382151
Output shows the name of one compute node (a001) that processed the job submission file myjob.sub and the two serial regions. The job submission scattered four processor cores (four MATLAB labs) among four different compute nodes (a001,a002,a003,a004) that processed the four parallel regions. The total elapsed time demonstrates that the jobs ran in parallel.
For more information about MATLAB Parallel Computing Toolbox:
Distributed Computing Server (parallel job)
The MATLAB Parallel Computing Toolbox (PCT) enables a parallel job via the MATLAB Distributed Computing Server (DCS). The tasks of a parallel job are identical, run simultaneously on several MATLAB workers (labs), and communicate with each other. This section illustrates an MPI-like program.
This section illustrates how to submit a small, MATLAB parallel job with four workers running one MPI-like task to a batch queue. The MATLAB program broadcasts an integer to four workers and gathers the names of the compute nodes running the workers and the lab IDs of the workers.
This example uses the job submission command to submit a Matlab script with a user-defined cluster profile which scatters the MATLAB workers onto different compute nodes. This method uses the MATLAB interpreter, the Parallel Computing Toolbox, and the Distributed Computing Server; so, it requires and checks out six licenses: one MATLAB license for the client running on the compute node, one PCT license, and four DCS licenses. Four DCS licenses run the four copies of the parallel job. This job is completely off the front end.
Prepare a MATLAB script named myscript.m :
% FILENAME: myscript.m
% Specify pool size.
% Convert the parallel job to a pool job.
parpool('4');
spmd
if labindex == 1
% Lab (rank) #1 broadcasts an integer value to other labs (ranks).
N = labBroadcast(1,int64(1000));
else
% Each lab (rank) receives the broadcast value from lab (rank) #1.
N = labBroadcast(1);
end
% Form a string with host name, total number of labs, lab ID, and broadcast value.
[c name] =system('hostname');
name = name(1:length(name)-1);
fmt = num2str(floor(log10(numlabs))+1);
str = sprintf(['%s:%d:%' fmt 'd:%d '], name,numlabs,labindex,N);
% Apply global concatenate to all str's.
% Store the concatenation of str's in the first dimension (row) and on lab #1.
result = gcat(str,1,1);
if labindex == 1
disp(result)
end
end % spmd
matlabpool close force;
quit;
Also, prepare a job submission, here named myjob.sub. Run with the name of the script:
# FILENAME: myjob.sub
echo "myjob.sub"
module load matlab
unset DISPLAY
# -nodisplay: run MATLAB in text mode; X11 server not needed
# -r: read MATLAB program; use MATLAB JIT Accelerator
matlab -nodisplay -r myscript
Run MATLAB to set the default parallel configuration to your appropriate Profile:
$ matlab -nodisplay
>> defaultParallelConfig('myslurmprofile');
>> quit;
$
Submit the job as a single compute node with one processor core.
Once this job starts, a second job submission is made.
myjob.sub
< M A T L A B (R) >
Copyright 1984-2011 The MathWorks, Inc.
R2011b (7.13.0.564) 64-bit (glnxa64)
August 13, 2011
To get started, type one of these: helpwin, helpdesk, or demo.
For product information, visit www.mathworks.com.
>Starting matlabpool using the 'myslurmprofile' configuration ... connected to 4 labs.
Lab 1:
gilbreth-a006.rcac.purdue.edu:4:1:1000
gilbreth-a007.rcac.purdue.edu:4:2:1000
gilbreth-a008.rcac.purdue.edu:4:3:1000
gilbreth-a009.rcac.purdue.edu:4:4:1000
Sending a stop signal to all the labs ... stopped.
Did not find any pre-existing parallel jobs created by matlabpool.
Output shows the name of one compute node (a006) that processed the job submission file myjob.sub. The job submission scattered four processor cores (four MATLAB labs) among four different compute nodes (a006,a007,a008,a009) that processed the four parallel regions.
To scale up this method to handle a real application, increase the wall time in the submission command to accommodate a longer running job. Secondly, increase the wall time of myslurmprofile by using the Cluster Profile Manager in the Parallel menu to enter a new wall time in the property SubmitArguments.
For more information about parallel jobs:
Python
Notice: Python 2.7 has reached end-of-life on Jan 1, 2020 (announcement). Please update your codes and your job scripts to use Python 3.
Python is a high-level, general-purpose, interpreted, dynamic programming language. We suggest using Anaconda which is a Python distribution made for large-scale data processing, predictive analytics, and scientific computing. For example, to use the default Anaconda distribution:
$ module load conda
For a full list of available Anaconda and Python modules enter:
$ module spider conda
Example Python Jobs
This section illustrates how to submit a small Python job to a SLURM queue.
Link to section 'Example 1: Hello world' of 'Example Python Jobs' Example 1: Hello world
Prepare a Python input file with an appropriate filename, here named hello.py
:
# FILENAME: hello.py
import string, sys
print("Hello, world!")
Prepare a job submission file with an appropriate filename, here named myjob.sub:
#!/bin/bash
# FILENAME: myjob.sub
module load conda
python hello.py
Hello, world!
Link to section 'Example 2: Matrix multiply' of 'Example Python Jobs' Example 2: Matrix multiply
Save the following script as matrix.py:
# Matrix multiplication program
x = [[3,1,4],[1,5,9],[2,6,5]]
y = [[3,5,8,9],[7,9,3,2],[3,8,4,6]]
result = [[sum(a*b for a,b in zip(x_row,y_col)) for y_col in zip(*y)] for x_row in x]
for r in result:
print(r)
Change the last line in the job submission file above to read:
python matrix.py
The standard output file from this job will result in the following matrix:
[28, 56, 43, 53]
[65, 122, 59, 73]
[63, 104, 54, 60]
Link to section 'Example 3: Sine wave plot using numpy and matplotlib packages' of 'Example Python Jobs' Example 3: Sine wave plot using numpy and matplotlib packages
Save the following script as sine.py:
import numpy as np
import matplotlib
matplotlib.use('Agg')
import matplotlib.pyplot as plt
x = np.linspace(-np.pi, np.pi, 201)
plt.plot(x, np.sin(x))
plt.xlabel('Angle [rad]')
plt.ylabel('sin(x)')
plt.axis('tight')
plt.savefig('sine.png')
Change your job submission file to submit this script and the job will output a png file and blank standard output and error files.
For more information about Python:
Managing Environments with Conda
Conda is a package manager in Anaconda that allows you to create and manage multiple environments where you can pick and choose which packages you want to use. To use Conda you must load an Anaconda module:
$ module load conda
Many packages are pre-installed in the global environment. To see these packages:
$ conda list
To create your own custom environment:
$ conda create --name MyEnvName python=3.8 FirstPackageName SecondPackageName -y
The --name option specifies that the environment created will be named MyEnvName. You can include as many packages as you require separated by a space. Including the -y option lets you skip the prompt to install the package. By default environments are created and stored in the $HOME/.conda directory.
To create an environment at a custom location:
$ conda create --prefix=$HOME/MyEnvName python=3.8 PackageName -y
To see a list of your environments:
$ conda env list
To remove unwanted environments:
$ conda remove --name MyEnvName --all
To add packages to your environment:
$ conda install --name MyEnvName PackageNames
To remove a package from an environment:
$ conda remove --name MyEnvName PackageName
Installing packages when creating your environment, instead of one at a time, will help you avoid dependency issues.
To activate or deactivate an environment you have created:
$ source activate MyEnvName
$ source deactivate MyEnvName
If you created your conda environment at a custom location using --prefix option, then you can activate or deactivate it using the full path.
$ source activate $HOME/MyEnvName
$ source deactivate $HOME/MyEnvName
To use a custom environment inside a job you must load the module and activate the environment inside your job submission script. Add the following lines to your submission script:
$ module load conda
$ source activate MyEnvName
For more information about Python:
Managing Packages with Pip
Pip is a Python package manager. Many Python package documentation provide pip
instructions that result in permission errors because by default pip
will install in a system-wide location and fail.
Exception:
Traceback (most recent call last):
... ... stack trace ... ...
OSError: [Errno 13] Permission denied: '/apps/cent7/anaconda/2020.07-py38/lib/python3.8/site-packages/mkl_random-1.1.1.dist-info'
If you encounter this error, it means that you cannot modify the global Python installation. We recommend installing Python packages in a conda environment. Detailed instructions for installing packages with pip can be found in our Python package installation page.
Below we list some other useful pip commands.
- Search for a package in PyPI channels:
$ pip search packageName
- Check which packages are installed globally:
$ pip list
- Check which packages you have personally installed:
$ pip list --user
- Snapshot installed packages:
$ pip freeze > requirements.txt
- You can install packages from a snapshot inside a new conda environment. Make sure to load the appropriate conda environment first.
$ pip install -r requirements.txt
For more information about Python:
Installing Packages
Installing Python packages in an Anaconda environment is recommended. One key advantage of Anaconda is that it allows users to install unrelated packages in separate self-contained environments. Individual packages can later be reinstalled or updated without impacting others. If you are unfamiliar with Conda environments, please check our Conda Guide.
To facilitate the process of creating and using Conda environments, we support a script (conda-env-mod) that generates a module file for an environment, as well as an optional Jupyter kernel to use this environment in a JupyterHub notebook.
You must load one of the anaconda modules in order to use this script.
$ module load conda
Step-by-step instructions for installing custom Python packages are presented below.
Link to section 'Step 1: Create a conda environment' of 'Installing Packages' Step 1: Create a conda environment
Users can use the conda-env-mod script to create an empty conda environment. This script needs either a name or a path for the desired environment. After the environment is created, it generates a module file for using it in future. Please note that conda-env-mod is different from the official conda-env script and supports a limited set of subcommands. Detailed instructions for using conda-env-mod can be found with the command conda-env-mod --help.
-
Example 1: Create a conda environment named mypackages in user's
$HOME
directory.$ conda-env-mod create -n mypackages
-
Example 2: Create a conda environment named mypackages at a custom location.
$ conda-env-mod create -p /depot/mylab/apps/mypackages
Please follow the on-screen instructions while the environment is being created. After finishing, the script will print the instructions to use this environment.
... ... ... Preparing transaction: ...working... done Verifying transaction: ...working... done Executing transaction: ...working... done +------------------------------------------------------+ | To use this environment, load the following modules: | | module load use.own | | module load conda-env/mypackages-py3.8.5 | +------------------------------------------------------+ Your environment "mypackages" was created successfully.
Note down the module names, as you will need to load these modules every time you want to use this environment. You may also want to add the module load lines in your jobscript, if it depends on custom Python packages.
By default, module files are generated in your $HOME/privatemodules directory. The location of module files can be customized by specifying the -m /path/to/modules option to conda-env-mod.
Note: The main differences between -p and -m are: 1) -p will change the location of packages to be installed for the env and the module file will still be located at the $HOME/privatemodules directory as defined in use.own. 2) -m will only change the location of the module file. So the method to load modules created with -m and -p are different, see Example 3 for details.
- Example 3: Create a conda environment named labpackages in your group's Data Depot space and place the module file at a shared location for the group to use.
$ conda-env-mod create -p /depot/mylab/apps/labpackages -m /depot/mylab/etc/modules ... ... ... Preparing transaction: ...working... done Verifying transaction: ...working... done Executing transaction: ...working... done +-------------------------------------------------------+ | To use this environment, load the following modules: | | module use /depot/mylab/etc/modules | | module load conda-env/labpackages-py3.8.5 | +-------------------------------------------------------+ Your environment "labpackages" was created successfully.
If you used a custom module file location, you need to run the module use command as printed by the command output above.
By default, only the environment and a module file are created (no Jupyter kernel). If you plan to use your environment in a JupyterHub notebook, you need to append a --jupyter flag to the above commands.
- Example 4: Create a Jupyter-enabled conda environment named labpackages in your group's Data Depot space and place the module file at a shared location for the group to use.
$ conda-env-mod create -p /depot/mylab/apps/labpackages -m /depot/mylab/etc/modules --jupyter ... ... ... Jupyter kernel created: "Python (My labpackages Kernel)" ... ... ... Your environment "labpackages" was created successfully.
Link to section 'Step 2: Load the conda environment' of 'Installing Packages' Step 2: Load the conda environment
-
The following instructions assume that you have used conda-env-mod script to create an environment named mypackages (Examples 1 or 2 above). If you used conda create instead, please use conda activate mypackages.
$ module load use.own $ module load conda-env/mypackages-py3.8.5
Note that the conda-env module name includes the Python version that it supports (Python 3.8.5 in this example). This is same as the Python version in the conda module.
-
If you used a custom module file location (Example 3 above), please use module use to load the conda-env module.
$ module use /depot/mylab/etc/modules $ module load conda-env/labpackages-py3.8.5
Link to section 'Step 3: Install packages' of 'Installing Packages' Step 3: Install packages
Now you can install custom packages in the environment using either conda install or pip install.
Link to section 'Installing with conda' of 'Installing Packages' Installing with conda
-
Example 1: Install OpenCV (open-source computer vision library) using conda.
$ conda install opencv
-
Example 2: Install a specific version of OpenCV using conda.
$ conda install opencv=4.5.5
-
Example 3: Install OpenCV from a specific anaconda channel.
$ conda install -c anaconda opencv
Link to section 'Installing with pip' of 'Installing Packages' Installing with pip
-
Example 4: Install pandas using pip.
$ pip install pandas
-
Example 5: Install a specific version of pandas using pip.
$ pip install pandas==1.4.3
Follow the on-screen instructions while the packages are being installed. If installation is successful, please proceed to the next section to test the packages.
Note: Do NOT run Pip with the --user argument, as that will install packages in a different location and might mess up your account environment.
Link to section 'Step 4: Test the installed packages' of 'Installing Packages' Step 4: Test the installed packages
To use the installed Python packages, you must load the module for your conda environment. If you have not loaded the conda-env module, please do so following the instructions at the end of Step 1.
$ module load use.own
$ module load conda-env/mypackages-py3.8.5
- Example 1: Test that OpenCV is available.
$ python -c "import cv2; print(cv2.__version__)"
- Example 2: Test that pandas is available.
$ python -c "import pandas; print(pandas.__version__)"
If the commands finished without errors, then the installed packages can be used in your program.
Link to section 'Additional capabilities of conda-env-mod script' of 'Installing Packages' Additional capabilities of conda-env-mod script
The conda-env-mod tool is intended to facilitate creation of a minimal Anaconda environment, matching module file and optionally a Jupyter kernel. Once created, the environment can then be accessed via familiar module load command, tuned and expanded as necessary. Additionally, the script provides several auxiliary functions to help manage environments, module files and Jupyter kernels.
General usage for the tool adheres to the following pattern:
$ conda-env-mod help
$ conda-env-mod <subcommand> <required argument> [optional arguments]
where required arguments are one of
- -n|--name ENV_NAME (name of the environment)
- -p|--prefix ENV_PATH (location of the environment)
and optional arguments further modify behavior for specific actions (e.g. -m to specify alternative location for generated module files).
Given a required name or prefix for an environment, the conda-env-mod script supports the following subcommands:
- create - to create a new environment, its corresponding module file and optional Jupyter kernel.
- delete - to delete existing environment along with its module file and Jupyter kernel.
- module - to generate just the module file for a given existing environment.
- kernel - to generate just the Jupyter kernel for a given existing environment (note that the environment has to be created with a --jupyter option).
- help - to display script usage help.
Using these subcommands, you can iteratively fine-tune your environments, module files and Jupyter kernels, as well as delete and re-create them with ease. Below we cover several commonly occurring scenarios.
Note: When you try to use conda-env-mod delete, remember to include the arguments as you create the environment (i.e. -p package_location and/or -m module_location).
Link to section 'Generating module file for an existing environment' of 'Installing Packages' Generating module file for an existing environment
If you already have an existing configured Anaconda environment and want to generate a module file for it, follow appropriate examples from Step 1 above, but use the module subcommand instead of the create one. E.g.
$ conda-env-mod module -n mypackages
and follow printed instructions on how to load this module. With an optional --jupyter flag, a Jupyter kernel will also be generated.
Note that the module name mypackages should be exactly the same with the older conda environment name. Note also that if you intend to proceed with a Jupyter kernel generation (via the --jupyter flag or a kernel subcommand later), you will have to ensure that your environment has ipython and ipykernel packages installed into it. To avoid this and other related complications, we highly recommend making a fresh environment using a suitable conda-env-mod create .... --jupyter command instead.
Link to section 'Generating Jupyter kernel for an existing environment' of 'Installing Packages' Generating Jupyter kernel for an existing environment
If you already have an existing configured Anaconda environment and want to generate a Jupyter kernel file for it, you can use the kernel subcommand. E.g.
$ conda-env-mod kernel -n mypackages
This will add a "Python (My mypackages Kernel)" item to the dropdown list of available kernels upon your next login to the JupyterHub.
Note that generated Jupiter kernels are always personal (i.e. each user has to make their own, even for shared environments). Note also that you (or the creator of the shared environment) will have to ensure that your environment has ipython and ipykernel packages installed into it.
Link to section 'Managing and using shared Python environments' of 'Installing Packages' Managing and using shared Python environments
Here is a suggested workflow for a common group-shared Anaconda environment with Jupyter capabilities:
The PI or lab software manager:
-
Creates the environment and module file (once):
$ module purge $ module load conda $ conda-env-mod create -p /depot/mylab/apps/labpackages -m /depot/mylab/etc/modules --jupyter
-
Installs required Python packages into the environment (as many times as needed):
$ module use /depot/mylab/etc/modules $ module load conda-env/labpackages-py3.8.5 $ conda install ....... # all the necessary packages
Lab members:
-
Lab members can start using the environment in their command line scripts or batch jobs simply by loading the corresponding module:
$ module use /depot/mylab/etc/modules $ module load conda-env/labpackages-py3.8.5 $ python my_data_processing_script.py .....
-
To use the environment in Jupyter notebooks, each lab member will need to create his/her own Jupyter kernel (once). This is because Jupyter kernels are private to individuals, even for shared environments.
$ module use /depot/mylab/etc/modules $ module load conda-env/labpackages-py3.8.5 $ conda-env-mod kernel -p /depot/mylab/apps/labpackages
A similar process can be devised for instructor-provided or individually-managed class software, etc.
Link to section 'Troubleshooting' of 'Installing Packages' Troubleshooting
- Python packages often fail to install or run due to dependency incompatibility with other packages. More specifically, if you previously installed packages in your home directory it is safer to clean those installations.
$ mv ~/.local ~/.local.bak $ mv ~/.cache ~/.cache.bak
- Unload all the modules.
$ module purge
- Clean up PYTHONPATH.
$ unset PYTHONPATH
- Next load the modules (e.g. anaconda) that you need.
$ module load conda/2024.02-py311 $ module load use.own $ module load conda-env/2024.02-py311
- Now try running your code again.
- Few applications only run on specific versions of Python (e.g. Python 3.6). Please check the documentation of your application if that is the case.
Installing Packages from Source
We maintain several Anaconda installations. Anaconda maintains numerous popular scientific Python libraries in a single installation. If you need a Python library not included with normal Python we recommend first checking Anaconda. For a list of modules currently installed in the Anaconda Python distribution:
$ module load conda
$ conda list
# packages in environment at /apps/spack/bell/apps/anaconda/2020.02-py37-gcc-4.8.5-u747gsx:
#
# Name Version Build Channel
_ipyw_jlab_nb_ext_conf 0.1.0 py37_0
_libgcc_mutex 0.1 main
alabaster 0.7.12 py37_0
anaconda 2020.02 py37_0
...
If you see the library in the list, you can simply import it into your Python code after loading the Anaconda module.
If you do not find the package you need, you should be able to install the library in your own Anaconda customization. First try to install it with Conda or Pip. If the package is not available from either Conda or Pip, you may be able to install it from source.
Use the following instructions as a guideline for installing packages from source. Make sure you have a download link to the software (usually it will be a tar.gz
archive file). You will substitute it on the wget line below.
We also assume that you have already created an empty conda environment as described in our Python package installation guide.
$ mkdir ~/src
$ cd ~/src
$ wget http://path/to/source/tarball/app-1.0.tar.gz
$ tar xzvf app-1.0.tar.gz
$ cd app-1.0
$ module load conda
$ module load use.own
$ module load conda-env/mypackages-py3.8.5
$ python setup.py install
$ cd ~
$ python
>>> import app
>>> quit()
The "import app" line should return without any output if installed successfully. You can then import the package in your python scripts.
If you need further help or run into any issues installing a library, contact us or drop by Coffee Hour for in-person help.
For more information about Python:
Example: Create and Use Biopython Environment with Conda
Link to section 'Using conda to create an environment that uses the biopython package' of 'Example: Create and Use Biopython Environment with Conda' Using conda to create an environment that uses the biopython package
To use Conda you must first load the anaconda module:
module load conda
Create an empty conda environment to install biopython:
conda-env-mod create -n biopython
Now activate the biopython environment:
module load use.own
module load conda-env/biopython-py3.12.5
Install the biopython packages in your environment:
conda install --channel anaconda biopython -y
Fetching package metadata ..........
Solving package specifications .........
.......
Linking packages ...
[ COMPLETE ]|################################################################
The --channel option specifies that it searches the anaconda channel for the biopython package. The -y argument is optional and allows you to skip the installation prompt. A list of packages will be displayed as they are installed.
Remember to add the following lines to your job submission script to use the custom environment in your jobs:
module load conda
module load use.own
module load conda-env/biopython-py3.12.5
If you need further help or run into any issues with creating environments, contact us or drop by Coffee Hour for in-person help.
For more information about Python:
Numpy Parallel Behavior
The widely available Numpy package is the best way to handle numerical computation in Python. The numpy
package provided by our anaconda
modules is optimized using Intel's MKL library. It will automatically parallelize many operations to make use of all the cores available on a machine.
In many contexts that would be the ideal behavior. On the cluster however that very likely is not in fact the preferred behavior because often more than one user is present on the system and/or more than one job on a node. Having multiple processes contend for those resources will actually result in lesser performance.
Setting the MKL_NUM_THREADS
or OMP_NUM_THREADS
environment variable(s) allows you to control this behavior. Our anaconda modules automatically set these variables to 1 if and only if you do not currently have that variable defined.
When submitting batch jobs it is always a good idea to be explicit rather than implicit. If you are submitting a job that you want to make use of the full resources available on the node, set one or both of these variables to the number of cores you want to allow numpy to make use of.
#!/bin/bash
module load conda
export MKL_NUM_THREADS=16
...
If you are submitting multiple jobs that you intend to be scheduled together on the same node, it is probably best to restrict numpy to a single core.
#!/bin/bash
module load conda
export MKL_NUM_THREADS=1
R
R, a GNU project, is a language and environment for data manipulation, statistics, and graphics. It is an open source version of the S programming language. R is quickly becoming the language of choice for data science due to the ease with which it can produce high quality plots and data visualizations. It is a versatile platform with a large, growing community and collection of packages.
For more general information on R visit The R Project for Statistical Computing.
Running R jobs
This section illustrates how to submit a small R job to a SLURM queue. The example job computes a Pythagorean triple.
Prepare an R input file with an appropriate filename, here named myjob.R:
# FILENAME: myjob.R
# Compute a Pythagorean triple.
a = 3
b = 4
c = sqrt(a*a + b*b)
c # display result
Prepare a job submission file with an appropriate filename, here named myjob.sub:
#!/bin/bash
# FILENAME: myjob.sub
module load r
# --vanilla:
# --no-save: do not save datasets at the end of an R session
R --vanilla --no-save < myjob.R
For other examples or R jobs:
Installing R packages
Link to section 'Challenges of Managing R Packages in the Cluster Environment' of 'Installing R packages' Challenges of Managing R Packages in the Cluster Environment
- Different clusters have different hardware and softwares. So, if you have access to multiple clusters, you must install your R packages separately for each cluster.
- Each cluster has multiple versions of R and packages installed with one version of R may not work with another version of R. So, libraries for each R version must be installed in a separate directory.
- You can define the directory where your R packages will be installed using the environment variable
R_LIBS_USER
. - For your convenience, a sample ~/.Rprofile example file is provided that can be downloaded to your cluster account and renamed into
~/.Rprofile
(or appended to one) to customize your installation preferences. Detailed instructions.
Link to section 'Installing Packages' of 'Installing R packages' Installing Packages
-
Step 0: Set up installation preferences.
Follow the steps for setting up your~/.Rprofile
preferences. This step needs to be done only once. If you have created a~/.Rprofile
file previously on Gilbreth, ignore this step. -
Step 1: Check if the package is already installed.
As part of the R installations on community clusters, a lot of R libraries are pre-installed. You can check if your package is already installed by opening an R terminal and entering the commandinstalled.packages()
. For example,module load r/4.4.1 R
installed.packages()["units",c("Package","Version")] Package Version "units" "0.8-1" quit()
If the package you are trying to use is already installed, simply load the library, e.g.,
library('units')
. Otherwise, move to the next step to install the package. -
Step 2: Load required dependencies. (if needed)
For simple packages you may not need this step. However, some R packages depend on other libraries. For example, thesf
package depends ongdal
andgeos
libraries. So, you will need to load the corresponding modules before installingsf
. Read the documentation for the package to identify which modules should be loaded.module load gdal module load geos
-
Step 3: Install the package.
Now install the desired package using the commandinstall.packages('package_name')
. R will automatically download the package and all its dependencies from CRAN and install each one. Your terminal will show the build progress and eventually show whether the package was installed successfully or not.R
install.packages('sf', repos="https://cran.case.edu/") Installing package into ‘/home/myusername/R/x86_64-pc-linux-gnu-library/4.4.1’ (as ‘lib’ is unspecified) trying URL 'https://cran.case.edu/src/contrib/sf_0.9-7.tar.gz' Content type 'application/x-gzip' length 4203095 bytes (4.0 MB) ================================================== downloaded 4.0 MB ... ... more progress messages ... ... ** testing if installed package can be loaded from final location ** testing if installed package keeps a record of temporary installation path * DONE (sf) The downloaded source packages are in ‘/tmp/RtmpSVAGio/downloaded_packages’
- Step 4: Troubleshooting. (if needed)
If Step 3 ended with an error, you need to investigate why the build failed. Most common reason for build failure is not loading the necessary modules.
Link to section 'Loading Libraries' of 'Installing R packages' Loading Libraries
Once you have packages installed you can load them with the library()
function as shown below:
library('packagename')
The package is now installed and loaded and ready to be used in R.
Link to section 'Example: Installing dplyr' of 'Installing R packages' Example: Installing dplyr
The following demonstrates installing the dplyr
package assuming the above-mentioned custom ~/.Rprofile
is in place (note its effect in the "Installing package into" information message):
module load r
R
install.packages('dplyr', repos="http://ftp.ussg.iu.edu/CRAN/")
Installing package into ‘/home/myusername/R/gilbreth/4.4.1’
(as ‘lib’ is unspecified)
...
also installing the dependencies 'crayon', 'utf8', 'bindr', 'cli', 'pillar', 'assertthat', 'bindrcpp', 'glue', 'pkgconfig', 'rlang', 'Rcpp', 'tibble', 'BH', 'plogr'
...
...
...
The downloaded source packages are in
'/tmp/RtmpHMzm9z/downloaded_packages'
library(dplyr)
Attaching package: 'dplyr'
For more information about installing R packages:
Loading Data into R
R is an environment for manipulating data. In order to manipulate data, it must be brought into the R environment. R has a function to read any file that data is stored in. Some of the most common file types like comma-separated variable(CSV) files have functions that come in the basic R packages. Other less common file types require additional packages to be installed. To read data from a CSV file into the R environment, enter the following command in the R prompt:
> read.csv(file = "path/to/data.csv", header = TRUE)
When R reads the file it creates an object that can then become the target of other functions. By default the read.csv() function will give the object the name of the .csv file. To assign a different name to the object created by read.csv enter the following in the R prompt:
> my_variable <- read.csv(file = "path/to/data.csv", header = FALSE)
To display the properties (structure) of loaded data, enter the following:
> str(my_variable)
For more functions and tutorials:
RStudio
RStudio is a graphical integrated development environment (IDE) for R. RStudio is the most popular environment for developing both R scripts and packages. RStudio is provided on most Research systems.
There are two methods to launch RStudio on the cluster: command-line and application menu icon.
Link to section 'Launch RStudio by the command-line:' of 'RStudio' Launch RStudio by the command-line:
module load gcc
module load r
module load rstudio
rstudio
Note that RStudio is a graphical program and in order to run it you must have a local X11 server running or use Thinlinc Remote Desktop environment. See the ssh X11 forwarding section for more details.
Link to section 'Launch Rstudio by the application menu icon:' of 'RStudio' Launch Rstudio by the application menu icon:
- Log into desktop.gilbreth.rcac.purdue.edu with web browser or ThinLinc client
- Click on the
Applications
drop down menu on the top left corner - Choose
Cluster Software
and thenRStudio
R and RStudio are free to download and run on your local machine. For more information about RStudio:
Setting Up R Preferences with .Rprofile
For your convenience, a sample ~/.Rprofile example file is provided that can be downloaded to your cluster account and renamed into ~/.Rprofile
(or appended to one). Follow these steps to download our recommended ~/.Rprofile
example and copy it into place:
curl -#LO https://www.rcac.purdue.edu/files/knowledge/run/examples/apps/r/Rprofile_example
mv -ib Rprofile_example ~/.Rprofile
The above installation step needs to be done only once on Gilbreth. Now load the R module and run R:
module load r/4.4.1
R
.libPaths()
[1] "/home/myusername/R/gilbreth/4.1.2-gcc-6.3.0-ymdumss"
[2] "/apps/spack/gilbreth/apps/r/4.1.2-gcc-6.3.0-ymdumss/rlib/R/library"
.libPaths()
should output something similar to above if it is set up correctly.
You are now ready to install R packages into the dedicated directory /home/myusername/R/gilbreth/4.1.2-gcc-6.3.0-ymdumss
.
Singularity
On Gilbreth, Singularity functionality is provided by Apptainer - see Apptainer section for details.
NGC (Nvidia GPU Cloud)
Link to section 'What is NGC?' of 'NGC (Nvidia GPU Cloud)' What is NGC?
Nvidia GPU cloud (NGC) is a GPU-accelerated cloud platform optimized for deep learning and scientific computing. NGC offers a comprehensive catalogue of GPU-accelerated containers, so the application runs quickly and reliably on the high performance computing environment. NGC was deployed to extend the cluster capabilities and to enable powerful software and deliver the fastest results. By utilizing Singularity and NGC, users can focus on building lean models, producing optimal solutions and gathering faster insights. For more information, please visit https://www.nvidia.com/en-us/gpu-cloud and NGC software catalog.
Link to section 'Getting Started' of 'NGC (Nvidia GPU Cloud)' Getting Started
Users can download containers from the NGC software catalog and run them directly using Singularity instructions from the corresponding container’s catalog page.
In addition, a subset of pre-downloaded NGC containers wrapped into convenient software modules are provided. These modules wrap underlying complexity and provide the same commands that are expected from non-containerized versions of each application.
On Gilbreth, type the command below to see the lists of NGC containers we deployed.
$ module load ngc
$ module avail
Link to section 'Example' of 'NGC (Nvidia GPU Cloud)' Example
This example demonstrates how to run LAMMPS with NGC modules.
First, let's prepare the run folder and download the input file for the example we are going to run.
$ cd $CLUSTER_SCRATCH
$ mkdir -p lammps_ngc
$ cd lammps_ngc
$ wget https://lammps.sandia.gov/inputs/in.lj.txt
Then load the ngc and lammps modules
$ module load ngc
$ module load lammps/29Oct2020
Finally we can set variables and start running lammps.
$ gpu_count=1
$ input=in.lj.txt
$ mpirun -n ${gpu_count} lmp -k on g ${gpu_count} -sf kk -pk kokkos cuda/aware on neigh full comm device binsize 2.8 -var x 8 -var y 4 -var z 8 -in ${input}
For more information, see each application’s NGC catalog page . For applications deployed as modules, see module help command for direct link to the relevant page (e.g. module help lammps/29Oct2020 in the above example).
Ansys Fluent
Ansys is a CAE/multiphysics engineering simulation software that utilizes finite element analysis for numerically solving a wide variety of mechanical problems. The software contains a list of packages and can simulate many structural properties such as strength, toughness, elasticity, thermal expansion, fluid dynamics as well as acoustic and electromagnetic attributes.
Link to section 'Ansys Licensing' of 'Ansys Fluent' Ansys Licensing
The Ansys licensing on our community clusters is maintained by Purdue ECN group. There are two types of licenses: teaching and research. For more information, please refer to ECN Ansys licensing page. If you are interested in purchasing your own research license, please send email to software@ecn.purdue.edu.
Link to section 'Ansys Workflow' of 'Ansys Fluent' Ansys Workflow
Ansys software consists of several sub-packages such as Workbench and Fluent. Most simulations are performed using the Ansys Workbench console, a GUI interface to manage and edit the simulation workflow. It requires X11 forwarding for remote display so a SSH client software with X11 support or a remote desktop portal is required. Please see Logging In section for more details. To ensure preferred performance, ThinLinc remote desktop connection is highly recommended.
Typically users break down larger structures into small components in geometry with each of them modeled and tested individually. A user may start by defining the dimensions of an object, adding weight, pressure, temperature, and other physical properties.
Ansys Fluent is a computational fluid dynamics (CFD) simulation software known for its advanced physics modeling capabilities and accuracy. Fluent offers unparalleled analysis capabilities and provides all the tools needed to design and optimize new equipment and to troubleshoot existing installations.
In the following sections, we provide step-by-step instructions to lead you through the process of using Fluent. We will create a classical elbow pipe model and simulate the fluid dynamics when water flows through the pipe. The project files have been generated and can be downloaded via fluent_tutorial.zip.
Link to section 'Loading Ansys Module' of 'Ansys Fluent' Loading Ansys Module
Different versions of Ansys are installed on the clusters and can be listed with module spider
or module avail
command in the terminal.
$ module avail ansys/
---------------------- Core Applications -----------------------------
ansys/2019R3 ansys/2020R1 ansys/2021R2 ansys/2022R1 (D)
Before launching Ansys Workbench, a specific version of Ansys module needs to be loaded. For example, you can module load ansys/2021R2
to use the latest Ansys 2021R2. If no version is specified, the default module -> (D) (ansys/2022R1
in this case) will be loaded. You can also check the loaded modules with module list
command.
Link to section 'Launching Ansys Workbench' of 'Ansys Fluent' Launching Ansys Workbench
Open a terminal on Gilbreth, enter rcac-runwb2
to launch Ansys Workbench.
You can also use runwb2
to launch Ansys Workbench. The main difference between runwb2
and rcac-runwb2
is that the latter sets the project folder to be in your scratch space. Ansys has an known bug that it might crash when the project folder is set to $HOME
on our systems.
Preparing Case Files for Fluent
Link to section 'Creating a Fluent fluid analysis system' of 'Preparing Case Files for Fluent' Creating a Fluent fluid analysis system
In the Ansys Workbench, create a new fluid flow analysis by double-clicking the Fluid Flow (Fluent) option under the Analysis Systems in the Toolbox on the left panel. You can also drag-and-drop the analysis system into the Project Schematic. A green dotted outline indicating a potential location for the new system initially appears in the Project Schematic. When you drag the system to one of the outlines, it turns into a red box to indicate the chosen location of the new system.
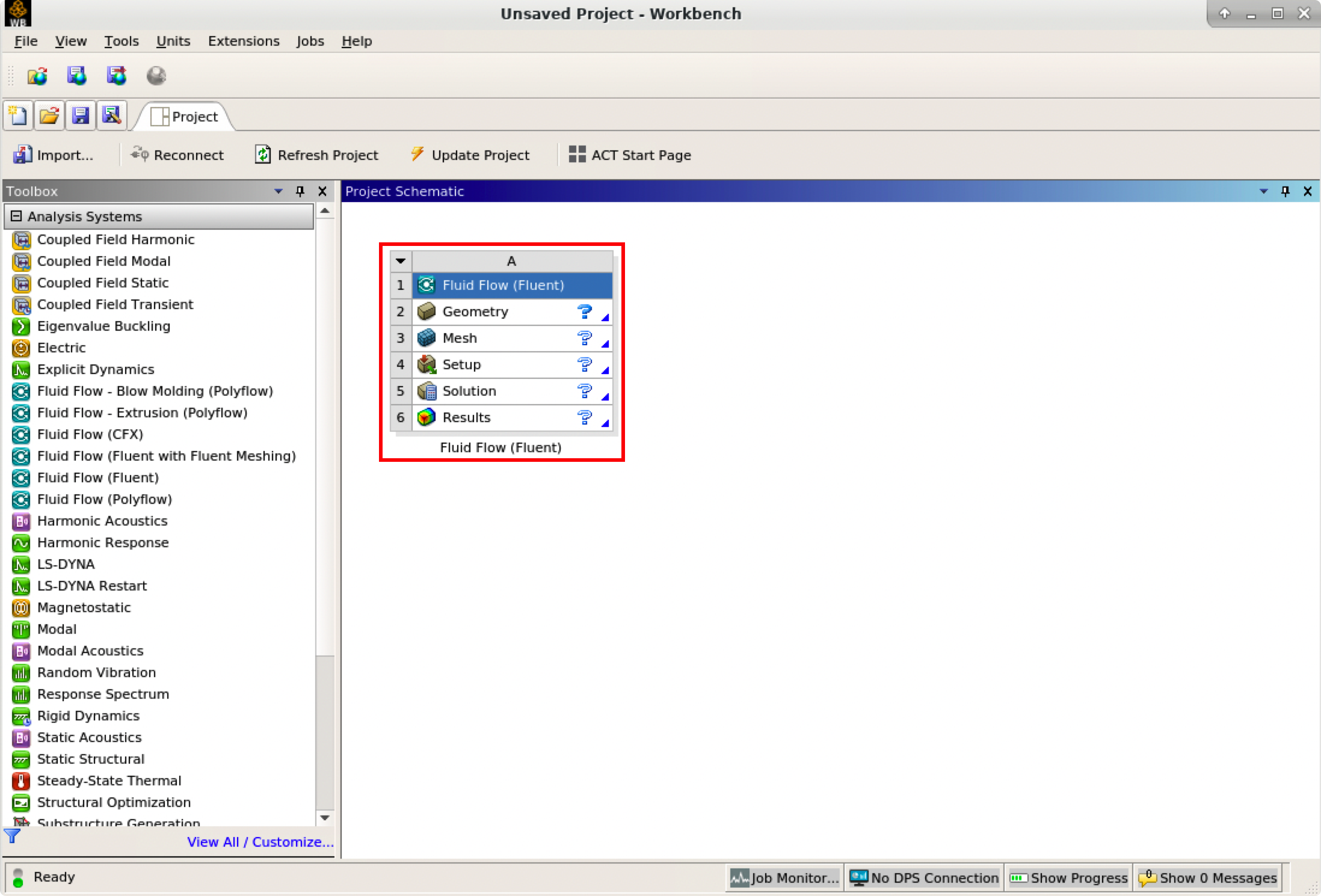
The red rectangle indicates the Fluid Flow system for Fluent, which includes all the essential workflows from “2 Geometry” to “6 Results”. You can rename it and carry out the necessary step-by-step procedures by double-clicking the corresponding cells.
It is important to save the project. Ansys Workbench saves the project with a .wbpj
extension and also all the supporting files into a folder with the same name. In this case, a file named elbow_demo.wbpj
and a folder $Ansys_PROJECT_FOLDER/elbow_demo_files/
are created in the Ansys project folder:
$ ll
total 33
drwxr-xr-x 7 myusername itap 9 Mar 3 17:47 elbow_demo_files
-rw-r--r-- 1 myusername itap 42597 Mar 3 17:47 elbow_demo.wbpj
You should always “Update Project” and save it after finishing a procedure.
Link to section 'Creating Geometry in the Ansys DesignModeler' of 'Preparing Case Files for Fluent' Creating Geometry in the Ansys DesignModeler
Create a geometry in the Ansys DesignModeler (by double-clicking “Geometry” cell in workflow), or import the appropriate geometry file (by right-clicking the Geometry cell and selecting “Import Geometry” option from the context menu).
You can use Ansys DesignModeler to create 2D/3D geometries or even draw the objects yourself. In our example, we created only half of the elbow pipe because the symmetry of the structure is taken into account to reduce the computation intensity.
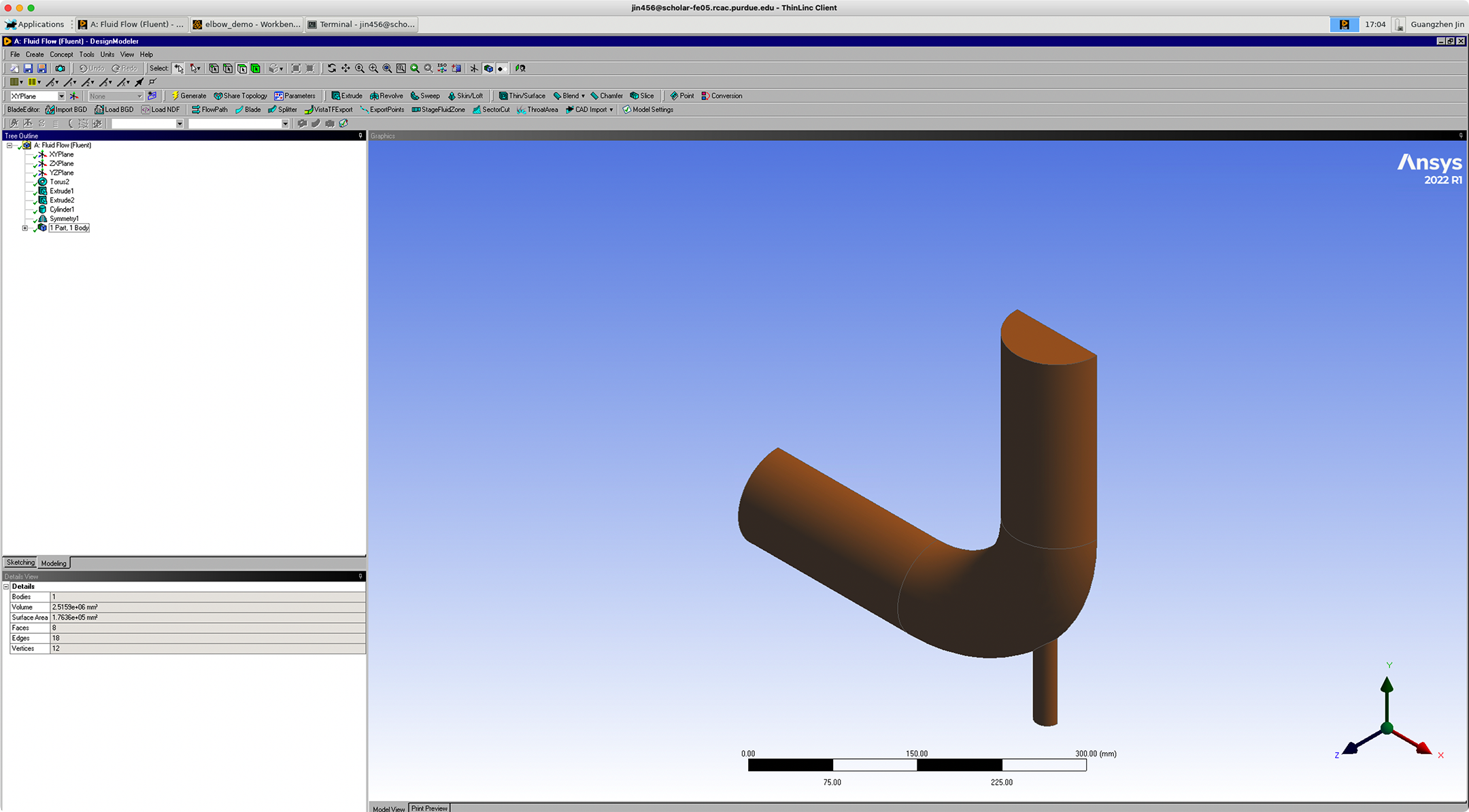
After saving the geometry, a geometry file FFF.agdb
will be created in the folder: $Ansys_PROJECT_FOLDER/elbow_demo_file/dp0/FFF/DM/
. The project in Workbench will be updated automatically.
If you import a pre-existing geometry into Ansys DesignModeler, it will also generate this file with the same filename at this location.
Link to section 'Creating mesh in the Ansys Meshing' of 'Preparing Case Files for Fluent' Creating mesh in the Ansys Meshing
Now that we have created the elbow pipe geometry, a computational mesh can be generated by the Meshing application throughout the flow volume.
With the successful creation of the geometry, there should be a green check showing the completion of “Geometry” in the Ansys Workbench. A Refresh Required icon within the “Mesh” cell indicates the mesh needs to be updated and refreshed for the system.
Then it’s time to open the Ansys Meshing application by double-clicking the “Mesh” cell and editing the mesh for the project. Generally, there are several steps we need to take to define the mesh:
- Create names for all geometry boundaries such as the inlets, outlets and fluid body. Note: You can use the strings “velocity inlet” and “pressure outlet” in the named selections (with or without hyphens or underscore characters) to allow Ansys Fluent to automatically detect and assign the corresponding boundary types accordingly. Use “Fluid” for the body to let Ansys Fluent automatically detect that the volume is a fluid zone and treat it accordingly.
- Set basic meshing parameters for the Ansys Meshing application. Here are several important parameters you may need to assign: Sizing, Quality, Body Sizing Control, Inflation.
- Select “Generate” to generate the mesh and “Update” to update the mesh into the system. Note: Once the mesh is generated, you can view the mesh statistics by opening the Statistics node in the Details of “Mesh” view. This will display information such as the number of nodes and the number of elements, which gives you a general idea for the future computational resources and time.
After generation and updating the mesh, a mesh file FFF.msh
will be generated in folder $Ansys_PROJECT_FOLDER/elbow_demo_file/dp0/FFF/MECH/
and a mesh database file FFF.mshdb
will be generated in folder $Ansys_PROJECT_FOLDER/elbow_demo_file/dp0/global/MECH/
.
Parameters used in demo case (use default if not assigned):
- Length Unit=”mm”
- Names defined for geometry:
- velocity-inlet-large (large inlet on pipe);
- velocity-inlet-small (small inlet on pipe);
- pressure-outlet (outlet on pipe);
- symmetry (symmetry surface);
- Fluid (body);
- Mesh:
- Quality: Smoothing=”high”;
- Inflation: Use Automatic Inflation=“Program Controlled”, Inflation Option=”Smooth Transition”;
- Statistics:
- Nodes=29371;
- Elements=87647.
Link to section 'Calculation with Fluent' of 'Preparing Case Files for Fluent' Calculation with Fluent
Now all the preparations have been ready for the numerical calculation in Ansys Fluent. Both “Geometry” and “Mesh” cells should have green checks on. We can set up the CFD simulation parameters in Ansys Fluent by double-clicking the “Setup” cell.
When Ansys Fluent is first started or by selecting “editing” on the “Setup” cell, the Fluent Launcher is displayed, enabling you to view and/or set certain Ansys Fluent start-up options (e.g. Precision, Parallel, Display). Note that “Dimension” is fixed to “3D” because we are using a 3D model in this project.
After the Fluent is opened, an Ansys Fluent settings file FFF.set
is written under the folder $Ansys_PROJECT_FOLDER/elbow_demo_file/dp0/FFF/Fluent/
.
Then we are going to set up all the necessary parameters for Fluent computation. Here are the key steps for the setup:
- Setting up the domain:
- Change the units for length to be consistent with the Mesh;
- Check the mesh statistics and quality;
- Setting up physics:
- Solver: “Energy”, “Viscous Model”, “Near-Wall Treatment”;
- Materials;
- Zones;
- Boundaries: Inlet, Outlet, Internal, Symmetry, Wall;
- Solving:
- Solution Methods;
- Reports;
- Initialization;
- Iterations and output frequency.
Then the calculation will be carried out and the results will be written out into FFF-1.cas.gz
under folder $Ansys_PROJECT_FOLDER/elbow_demo_file/dp0/FFF/Fluent/
.
This file contains all the settings and simulation results which can be loaded for post analysis and re-computation (more details will be introduced in the following sections). If only configurations and settings within the Fluent are needed, we can open independent Fluent or submit Fluent jobs with bash commands by loading the existing case in order to facilitate the computation process.
Parameters used in demo case (use default if not assigned):
- Domain Setup: Length Units=”mm”;
- Solver: Energy=”on”; Viscous Model=”k-epsilon”; Near-Wall Treatment=”Enhanced Wall Treatment”;
- Materials: water (Density=1000[kg/m^3]; Specific Heat=4216[J/kg-k]; Thermal Conductivity=0.677[w/m-k]; Viscosity=8e-4[kg/m-s]);
- Zones=”fluid (water)”;
- Inlet=”velocity-inlet-large” (Velocity Magnitude=0.4m/s, Specification Method=”Intensity and Hydraulic Diameter”, Turbulent Intensity=5%; Hydraulic Diameter=100mm; Thermal Temperature=293.15k) &”velocity-inlet-small” (Velocity Magnitude=1.2m/s, Specification Method=”Intensity and Hydraulic Diameter”, Turbulent Intensity=5%; Hydraulic Diameter=25mm; Thermal Temperature=313.15k); Internal=”interior-fluid”; Symmetry=”symmetry”; Wall=”wall-fluid”;
- Solution Methods: Gradient=”Green-Gauss Node Based”;
- Report: plot residual and “Facet Maximum” for “pressure-outlet”
- Hybrid Initialization;
- 300 iterations.
Case Calculating with Fluent
Link to section 'Calculation with Fluent' of 'Case Calculating with Fluent' Calculation with Fluent
Now all the files are ready for the Fluent calculations. Both “Geometry” and “Mesh” cells should have green checks. We can set up the CFD simulation parameters in the Ansys Fluent by double-clicking the “Setup” cell.
Ansys Fluent Launcher can be started by selecting “editing” on the “Setup” cell with many startup options (e.g. Precision, Parallel, Display). Note that “Dimension” is fixed to “3D” because we are using a 3D model in this project.
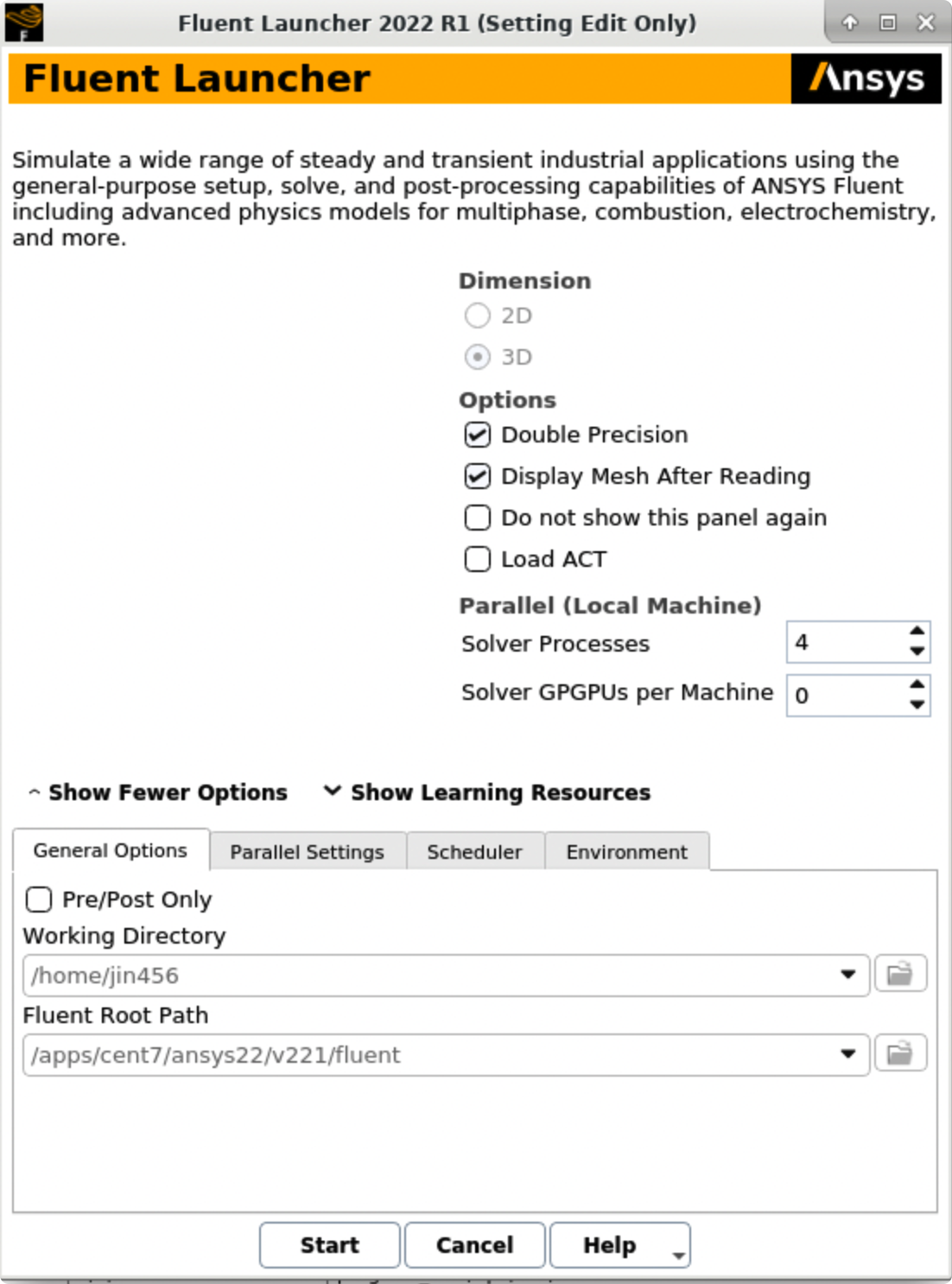
After the Fluent is opened, an Ansys Fluent settings file FFF.set
is written under the folder $Ansys_PROJECT_FOLDER/elbow_demo_file/dp0/FFF/Fluent/
.
Then we are going to set up all the necessary parameters for Fluent computation. Here are the key steps for the setup:
- Setting up the domain:
- Change the units for length to be consistent with the Mesh;
- Check the mesh statistics and quality;
- Setting up physics:
- Solver: “Energy”, “Viscous Model”, “Near-Wall Treatment”;
- Materials;
- Zones;
- Boundaries: Inlet, Outlet, Internal, Symmetry, Wall;
- Solving:
- Solution Methods;
- Reports;
- Initialization;
- Iterations and output frequency.
Then the calculation will be carried out and the results will be written out into FFF-1.cas.gz
under folder $Ansys_PROJECT_FOLDER/elbow_demo_file/dp0/FFF/Fluent/
.
This file contains all the settings and simulation results which can be loaded for post analysis and re-computation (more details will be introduced in the following sections). If only configurations and settings within the Fluent are needed, we can open independent Fluent or submit Fluent jobs with bash commands by loading the existing case in order to facilitate the computation process.
Parameters used in demo case (use default if not assigned):
- Domain Setup: Length Units=”mm”;
- Solver: Energy=”on”; Viscous Model=”k-epsilon”; Near-Wall Treatment=”Enhanced Wall Treatment”;
- Materials: water (Density=1000[kg/m^3]; Specific Heat=4216[J/kg-k]; Thermal Conductivity=0.677[w/m-k]; Viscosity=8e-4[kg/m-s]);
- Zones=”fluid (water)”;
- Inlet=”velocity-inlet-large” (Velocity Magnitude=0.4m/s, Specification Method=”Intensity and Hydraulic Diameter”, Turbulent Intensity=5%; Hydraulic Diameter=100mm; Thermal Temperature=293.15k) &”velocity-inlet-small” (Velocity Magnitude=1.2m/s, Specification Method=”Intensity and Hydraulic Diameter”, Turbulent Intensity=5%; Hydraulic Diameter=25mm; Thermal Temperature=313.15k); Internal=”interior-fluid”; Symmetry=”symmetry”; Wall=”wall-fluid”;
- Solution Methods: Gradient=”Green-Gauss Node Based”;
- Report: plot residual and “Facet Maximum” for “pressure-outlet”
- Hybrid Initialization;
- 300 iterations.
Link to section 'Results analysis' of 'Case Calculating with Fluent' Results analysis
The best methods to view and analyze the simulation should be the Ansys Fluent (directly after computation) or the Ansys CFD-Post (entering “Results” in Ansys Workbench). Both methods are straightforward so we will not cover this part in this tutorial. Here is a final simulation result showing the temperature of the symmetry after 300 iterations for reference:
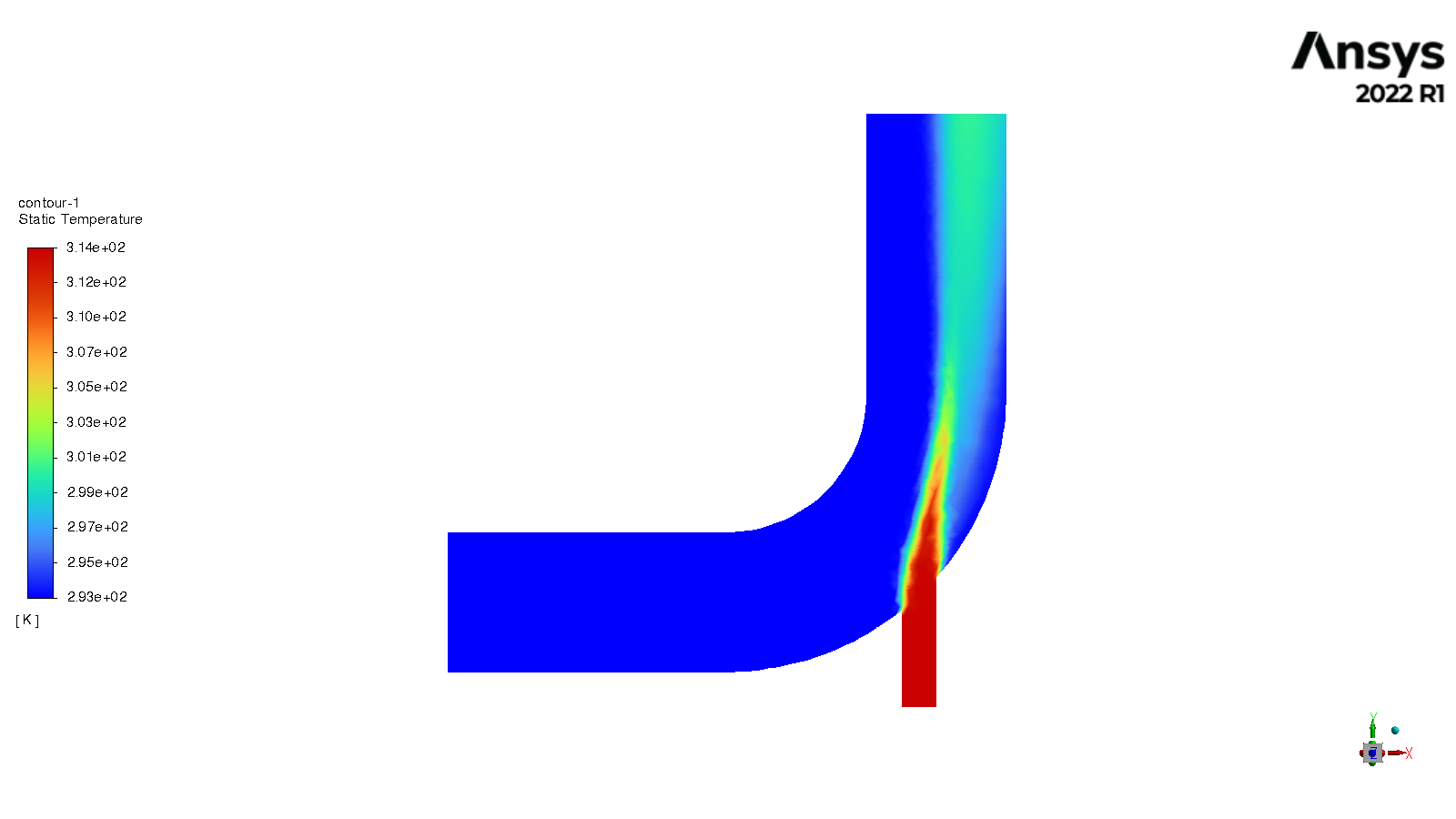
Fluent Text User Interface and Journal File
Link to section 'Fluent Text User Interface (TUI)' of 'Fluent Text User Interface and Journal File' Fluent Text User Interface (TUI)
If you pay attention to the “Console” window in the Fluent window when setting up and carrying out the calculation, corresponding commands can be found and executed one after another. Almost all the setting processes can be accomplished by the command lines, which is called Fluent Text User Interface (TUI). Here are the main commands in Fluent TUI:
adjoint/ parallel/ solve/
define/ plot/ surface/
display/ preferences/ turbo-workflow/
exit print-license-usage views/
file/ report/
mesh/ server/
For example, instead of opening a case by clicking buttons in Ansys Fluent, we can type /file read-case case_file_name.cas.gz
to open the saved case.
Link to section 'Fluent Journal Files' of 'Fluent Text User Interface and Journal File' Fluent Journal Files
A Fluent journal file is a series of TUI commands stored in a text file. The file can be written in a text editor or generated by Fluent as a transcript of the commands given to Fluent during your session.
A journal file generated by Fluent will include any GUI operations (in a TUI form, though). This is quite useful if you have a series of tasks that you need to execute, as it provides a shortcut. To record a journal file, start recording with File -> Write -> Start Journal..., perform whatever tasks you need, and then stop recording with File -> Write -> Stop Journal...
You can also write your own journal file into a text file. The basic rule for a Fluent journal file is to reproduce the TUI commands that controlled the configuration and calculation of Fluent in their order. You can add a comment in a line starting with a ;
(semicolon).
Here are some reasons why you should use a Fluent journal file:
- Using journal files with bash scripting can allow you to automate your jobs.
- Using journal files can allow you to parameterize your models easily and automatically.
- Using a journal file can set parameters you do not have in your case file e.g. autosaving.
- Using a journal file can allow you to safely save, stop and restart your jobs easily.
The order of your journal file commands is highly important. The correct sequences must be followed and some stages have multiple options e.g. different initialization methods.
Here is a sample Fluent journal file for the demo case:
;testJournal.jou
;Set the TUI version for Fluent
/file/set-tui-version "22.1"
;Read the case. The default folder
/file read-case /home/jin456/Fluent_files/tutorial_case1/elbow_files/dp0/FFF/Fluent/FFF-1.cas.gz
;Initialize the case with Hybrid Initialization
/solve/initialize/hyb-initialization
;Set Number of Iterations to 1000, Reporting Interval to 10 iterations and Profile Update Interval to 1 iteration
/solve/iterate 1000 10 1
;Outputting solver performance data upon completion of the simulation
/parallel timer usage
;Write out the simulation results.
/file write-case-data /home/jin456/Fluent_files/tutorial_case1/elbow_files/dp0/FFF/Fluent/result.cas.h5
;After computation, exit Flent
/exit
Before running this Fluent journal file, you need to make sure: 1) the ansys module has been loaded (it’s highly recommended to load the same version of Ansys when you built the case project); 2) the project case file (***.cas.gz
) has been created.
Then we can use Fluent to run this journal file by simply using:fluent 3ddp -t$NTASKS -g -i testJournal.jou
in the terminal. Here, 3d
indicates this is a 3d model, dp
indicates double precision, -t$NTASKS
tells Fluent how many Solver Processes it will take (e.g. -t4
), -g
means to run without the GUI or graphics, -i
testJournal.jou tells Fluent to read the specific journal file.
Here is a table for the available command line Options for Linux/UNIX and Windows Platforms in Ansys Fluent.
Option | Platform | Description |
---|---|---|
-cc |
all | Use the classic color scheme |
-ccp x |
Windows only | Use the Microsoft Job Scheduler where x is the head node name. |
-cnf=x |
all | Specify the hosts or machine list file |
-driver |
all | Sets the graphics driver (available drivers vary by platform - opengl or x11 or null(Linux/UNIX) - opengl or msw or null (Windows)) |
-env |
all | Show environment variables |
-fgw |
all | Disables the embedded graphics |
-g |
all | Run without the GUI or graphics (Linux/UNIX); Run with the GUI minimized (Windows) |
-gr |
all | Run without graphics |
-gu |
all | Run without the GUI but with graphics (Linux/UNIX); Run with the GUI minimized but with graphics (Windows) |
-help |
all | Display command line options |
-hidden |
Windows only | Run in batch mode |
-host_ip=host:ip |
all | Specify the IP interface to be used by the host process |
-i journal |
all | Reads the specified journal file |
-lsf |
Linux/UNIX only | Run FLUENT using LSF |
-mpi= |
all | Specify MPI implementation |
-mpitest |
all | Will launch an MPI program to collect network performance data |
-nm |
all | Do not display mesh after reading |
-pcheck |
Linux/UNIX only | Checks all nodes |
-post |
all | Run the FLUENT post-processing-only executable |
-p |
all | Choose the interconnect |
-r |
all | List all releases installed |
-rx |
all | Specify release number |
-sge |
Linux/UNIX only | Run FLUENT under Sun Grid Engine |
-sge queue |
Linux/UNIX only | Name of the queue for a given computing grid |
-sgeckpt ckpt_obj |
Linux/UNIX only | Set checkpointing object to ckpt_objfor SGE |
-sgepe fluent_pe min_n-max_n |
Linux/UNIX only | Set the parallel environment for SGE to fluent_pe, min_nand max_n are number of min and max nodes requested |
-tx |
all | Specify the number of processors x |
For more information for Fluent text user interface and journal files, please refer to Fluent FAQ.
Submitting Fluent jobs to SLURM
The Fluent simulations can also run in batch. In this section we provide an example script for submitting Fluent jobs to the SLURM scheduler. Please refer to the Running Jobs section of our user guide for detailed tutorials of submitting jobs.
#!/bin/bash
# Job script for submitting a FLUENT job on multiple cores on a single node
# Apply resources via SLURM
#SBATCH --nodes=1
#SBATCH --ntasks=4
#SBATCH --time=01:00:00
#SBATCH --job-name=fluent_test
#SBATCH -o fluent_test_%j.out
#SBATCH -e fluent_test_%j.err
# Loads Ansys and sets the application up
module purge
module load ansys/2022R1
#Initiating Fluent and reading input journal file
fluent 3ddp -t$NTASKS -g -i testJournal.jou
For more information about submitting Fluent jobs, please refer to Fluent FAQ .
Apptainer
Note: Apptainer was formerly known as Singularity and is now a part of the Linux Foundation. When migrating from Singularity see the user compatibility documentation.
Link to section 'What is Apptainer?' of 'Apptainer' What is Apptainer?
Apptainer is an open-source container platform designed to be simple, fast, and secure. It allows the portability and reproducibility of operating systems and application environments through the use of Linux containers. It gives users complete control over their environment.
Apptainer is like Docker but tuned explicitly for HPC clusters. More information is available on the project’s website.
Link to section 'Features' of 'Apptainer' Features
- Run the latest applications on an Ubuntu or Centos userland
- Gain access to the latest developer tools
- Launch MPI programs easily
- Much more
Apptainer’s user guide is available at: apptainer.org/docs/user/main/introduction.html
Link to section 'Example' of 'Apptainer' Example
Here is an example using an Ubuntu 16.04 image on Gilbreth:
apptainer exec /depot/itap/singularity/ubuntu1604.img cat /etc/lsb-release
DISTRIB_ID=Ubuntu
DISTRIB_RELEASE=16.04
DISTRIB_CODENAME=xenial
DISTRIB_DESCRIPTION="Ubuntu 16.04 LTS"
Here is another example using a Centos 7 image:
apptainer exec /depot/itap/singularity/centos7.img cat /etc/redhat-release
CentOS Linux release 7.3.1611 (Core)
Link to section 'Purdue Cluster Specific Notes' of 'Apptainer' Purdue Cluster Specific Notes
All service providers will integrate Apptainer slightly differently depending on site. The largest customization will be which default files are inserted into your images so that routine services will work.
Services we configure for your images include DNS settings and account information. File systems we overlay into your images are your home directory, scratch, Data Depot, and application file systems.
Here is a list of paths:
- /etc/resolv.conf
- /etc/hosts
- /home/$USER
- /apps
- /scratch
- /depot
This means that within the container environment these paths will be present and the same as outside the container. The /apps
, /scratch
, and /depot
directories will need to exist inside your container to work properly.
Link to section 'Creating Apptainer Images' of 'Apptainer' Creating Apptainer Images
You can build on your system or straight on the cluster (you do not need root privileges to build or run the container).
You can find information and documentation for how to install and use Apptainer on your system:
We have version 1.1.6
(or newer) on the cluster. Please note that installed versions may change throughout cluster life time, so when in doubt, please check exact version with a --version
command line flag:
apptainer --version
apptainer version 1.1.6-1
Everything you need on how to build a container is available from their user guide. Below are merely some quick tips for getting your own containers built for Gilbreth.
You can use a Definition File to both build your container and share its specification with collaborators (for the sake of reproducibility). Here is a simplistic example of such a file:
# FILENAME: Buildfile
Bootstrap: docker
From: ubuntu:18.04
%post
apt-get update && apt-get upgrade -y
mkdir /apps /depot /scratch
To build the image itself:
apptainer build ubuntu-18.04.sif Buildfile
The challenge with this approach however is that it must start from scratch if you decide to change something. In order to create a container image iteratively and interactively, you can use the --sandbox
option.
apptainer build --sandbox ubuntu-18.04 docker://ubuntu:18.04
This will not create a flat image file but a directory tree (i.e., a folder), the contents of which are the container's filesystem. In order to get a shell inside the container that allows you to modify it, user the --writable
option.
apptainer shell --writable ubuntu-18.04
Apptainer>
You can then proceed to install any libraries, software, etc. within the container. Then to create the final image file, exit
the shell and call the build
command once more on the sandbox.
apptainer build ubuntu-18.04.sif ubuntu-18.04
Finally, copy the new image to Gilbreth and run it.