80 NVIDIA A100 GPUs recently added to Gilbreth community cluster
After expanding the Gilbreth community cluster’s GPU nodes in 2022 and yet again last year, the Rosen Center for Advanced Computing (RCAC) has once again added even more GPUs to the Gilbreth cluster to meet demand from the Purdue community.
With the recent addition of 80 new NVIDIA A100 GPUs inside 20 Dell PowerEdge xe8545 compute nodes, the Gilbreth cluster now has 411 GPUs, nearly four times its original capacity.
The Gilbreth cluster’s storage capacity was also recently doubled and upgraded to include an improved design that results in faster storage transactions and reduces researchers’ time to science.
The new nodes include NVIDIA’s NVLink technology, which allows for faster communication between the GPUs and will improve speed and access to memory for researchers who use multiple GPUs at once.
All of these upgrades to Gilbreth are part of RCAC’s ongoing investment in supporting researchers doing AI and machine learning work, and dramatically accelerating physics-based simulations. Due to the swift sell-out of recent expansions upon implementation, RCAC has proceeded to augment Gilbreth by adding nodes to effectively meet the escalating demand.
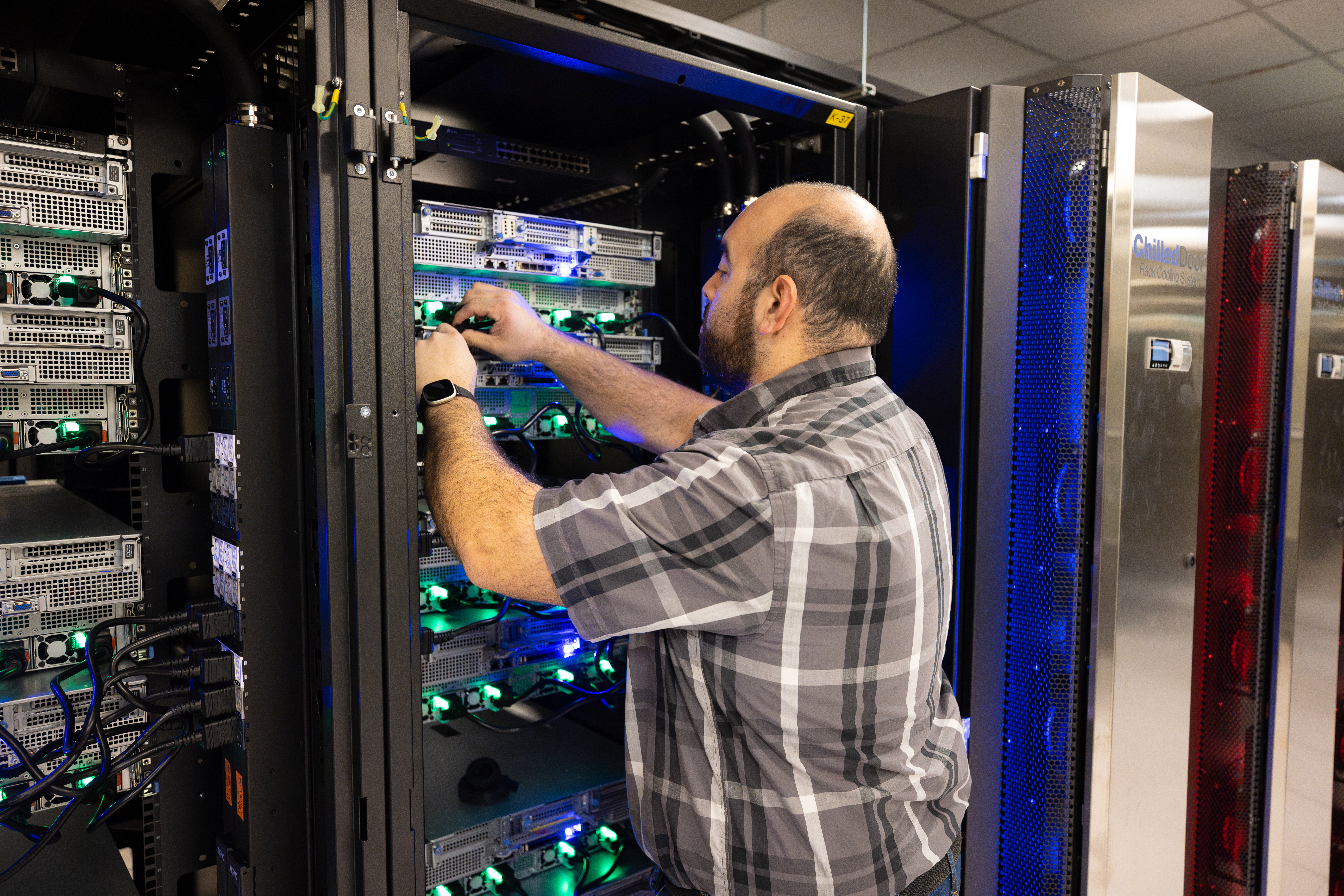
The infrastructure expansion supports RCAC’s broader strategic plan to offer AI and machine learning expertise, offer new trainings, and partner with faculty on proposals.
“Having more GPUs per node has been very beneficial,” says Shakti Wadekar, a doctoral student working with Eugenio Culurciello, a professor of biomedical engineering and the director of Purdue’s Institute for Physical AI.
Wadekar, who uses Gilbreth to train open source multimodal models, has appreciated having multiple GPUs on a single node, and looks forward to training even larger scale models with the new nodes that have four GPUs per node.
“We see much better performance when the GPUs are on the same node, because then we don’t have to take the additional step of synchronizing different nodes that are working on the same job,” adds Dwijen Chawra, a sophomore in computer science who also works in Culurciello’s lab and is focused on scaling multimodal models from a single GPU to run on multiple GPUs.
Chawra, who also works as a student worker for RCAC, tested similar nodes on his models and saw a 40-50% improvement in speed when using nodes with four GPUs over other Gilbreth nodes.
“One thing that’s special about these new GPU nodes is that NVIDIA technology allows us to pool the GPU memory to get a continuous block of larger memory for the node. The more we’re able to fit on a single node, the faster it runs – not just for training models but also for actually using and testing the model. So we can test larger models much faster using the nodes with four GPUs on them,” adds Chawra.
William Zummo, a doctoral student working in the lab of Alejandro Strachan, Reilly Professor of Materials Engineering, uses Gilbreth to conduct large-scale non-equilibrium molecular dynamics simulations to understand material failures in niobium under conditions of high strain rates.
The complexity of these simulations, involving the interactions of over 400 million atoms, necessitates the use of a cluster like Gilbreth. This extensive computational work generates approximately 13 terabytes of data files, which the team then analyzes using Gilbreth.
“Without Gilbreth and systems like it, this research would not be possible,” says Zummo.
“Recently, our group was able to achieve a 44% increase in thermal emitter efficiency, which in large part was due to the high-performance capabilities of Gilbreth. Without Gilbreth, tackling these research challenges would be infeasible,” says Michael Bezick, a sophomore in computer science working in the lab of Alexandra Boltasseva, the Ron And Dotty Garvin Tonjes Distinguished Professor Of Electrical and Computer Engineering, who is working on developing machine learning techniques to optimize the topologies of nanophotonic structures.
“This training would take significant amounts of time on a single consumer grade GPU. Parallelizing the workload across many GPUs, the industry standard in training state-of-the-art models, allows us to achieve a many-fold speedup in training, and the greater memory capacity allows us to utilize larger, deeper models,” adds Bezick.
The new A100 GPUs are offered under a similar pricing model as CPU-based community cluster systems, meaning researchers can choose between purchasing per-GPU units through either a one-time five-year charge or an annual subscription.
The economies of scale of community clusters allow Gilbreth GPUs to be priced more competitively than do-it-yourself GPU solutions, and the existing inventory allows for immediate access to nodes without a lengthy procurement process.
Researchers will have a queue specific to their lab as on other community clusters and will also have standby access to unused nodes. Access to Gilbreth can be purchased through the RCAC cluster orders website.
To learn more about Gilbreth or other Research Computing resources, contact rcac-help@purdue.edu.