User Guides
Compute
-Bell User Guide
Bell is a Community Cluster optimized for communities running traditional, tightly-coupled science and engineering applications.
Link to section 'Overview of Bell' of 'Overview of Bell' Overview of Bell
Bell is a Community Cluster optimized for communities running traditional, tightly-coupled science and engineering applications. Bell was built through a partnership with Dell and AMD over the summer of 2020. Bell consists of Dell compute nodes with two 64-core AMD Epyc 7662 "Rome" processors (128 cores per node) and 256 GB of memory. All nodes have 100 Gbps HDR Infiniband interconnect and a 6-year warranty.
Bell access is offered on the basis of each 64-core Rome processor, or a half-node share. To purchase access to Bell today, go to the Cluster Access Purchase page. Please subscribe to our Community Cluster Program Mailing List to stay informed on the latest purchasing developments or contact us via email at rcac-cluster-purchase@lists.purdue.edu if you have any questions.
Link to section 'Bell Namesake' of 'Overview of Bell' Bell Namesake
Bell is named in honor of Clara Bell Sessions, minority advocate and Professor and Director of Continuing Education of Nursing. More information about her life and impact on Purdue is available in a Biography of Bell.
Link to section 'Bell Specifications' of 'Overview of Bell' Bell Specifications
All Bell compute nodes have 128 processor cores and 100 Gbps Infiniband interconnects.
Front-Ends | Number of Nodes | Processors per Node | Cores per Node | Memory per Node | Retires in |
---|---|---|---|---|---|
8 | One Rome CPU @ 2.0GHz | 32 | 512 GB | 2026 |
Sub-Cluster | Number of Nodes | Processors per Node | Cores per Node | Memory per Node | Retires in |
---|---|---|---|---|---|
A | 448 | Two Rome CPUs @ 2.0GHz | 128 | 256 GB | 2026 |
B | 8 | Two Rome CPUs @ 2.0GHz | 128 | 1 TB | 2026 |
G | 4 | Two Rome CPUs @ 2.0GHz, two MI50 AMD GPUs (32GB) |
128 | 256 GB | 2026 |
G | 1 | Two Cascade Lake CPUs @ 2.90 GHz, six MI50 AMD GPUs (32GB) |
48 | 384 GB | 2026 |
Bell nodes run CentOS 7 and use Slurm (Simple Linux Utility for Resource Management) as the batch scheduler for resource and job management. The application of operating system patches occurs as security needs dictate. All nodes allow for unlimited stack usage, as well as unlimited core dump size (though disk space and server quotas may still be a limiting factor).
On Bell, the following set of compiler and message-passing library for parallel code are recommended:
- GCC 9.3.0
- OpenMPI
This compiler and these libraries are loaded by default. To load the recommended set again:
$ module load rcac
To verify what you loaded:
$ module list
Link to section 'Software catalog' of 'Overview of Bell' Software catalog
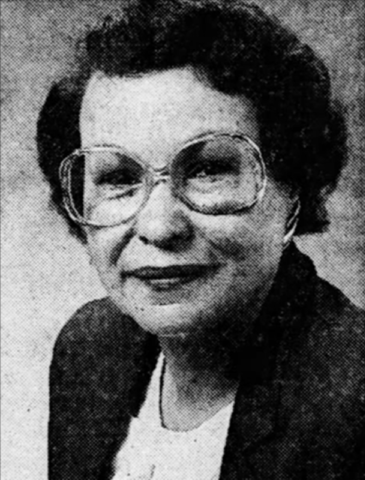
Link to section 'Clara Bell Sessions' of 'Biography of Clara Bell Sessions' Clara Bell Sessions
Clara Bell Sessions was a nursing professor and director of continuing education in nursing at Purdue University. While at Purdue, she helped establish the Minority Student Nurses' Association (MSNA) and Minority Faculty Fellows program. Bell attended Indiana State University where she earned her bachelor of science in nursing, followed by Indiana University where she earned her master's and doctoral degrees in their School of Education.
In 1981, Purdue University hired Bell as a professor of nursing and she later became the director of continuing education in nursing. Bell was dedicated to improving the experience for minority students and faculty at Purdue. She was integral in the creation of the Minority Student Nurses' Association (MSNA), now Diversity in Nursing Association, and the Minority Faculty Fellows program, the precursor of the Office of Diversity and Multicultural Affairs.
Bell was also active in national organizations. In 1992 and 1993, she co-chaired the National Congress of Black Faculty Council on Research and Education, served as a cabinet member on the Human Rights Committee of the American Nurses Association, and was a charter member of the Association of Black Nursing Faculty in Higher Education. She earned numerous service awards for her work, including service awards from the Indiana Diabetes Association, Indiana State Nurses Association's board of directors, Delta Omicron Chapter of the Nursing Honor Society of Sigma Theta Tau, and Indiana Department of Aging and Community Services.
Clara Bell Sessions died on March 3, 1996 in Terre Haute, Indiana. After her death, the Black Caucus of Faculty and Staff created the annual Clara E. Bell Academic Achievement Award for the senior in nursing or health sciences with the highest grade point average. In 2013, she was posthumously awarded the Title IX Distinguished Service Award for her contributions to gender equity in education.
Link to section 'Citations' of 'Biography of Clara Bell Sessions' Citations
Archives and Special Collections. (2020, July 28). Bell, Clara E., 1934-. Purdue University. Retrieved from: https://archives.lib.purdue.edu/agents/people/3085
Klink, A. (2020, February 4). Clara E. Bell was a trailblazing African American professor of nursing. Purdue University. Retrieved from: https://www.purdue.edu/hhs/news/2020/02/clara-e-bell-was-a-trailblazing-african-american-professor-of-nursing%EF%BB%BF/
Lythgoe, D. (2001-2020). Clara E. Stewart. The Lost Creek Settlement of Vigo County, Indiana. Retrieved from: https://www.lost-creek.org/genealogy/getperson.php?personID=I72&tree=tree2
Purdue Today. (2013, April 19). Purdue Today presenting profiles on Title IX service awardees. Purdue University. Retrieved from: https://www.purdue.edu/newsroom/purduetoday/releases/2013/Q2/purdue-today-presenting-profiles-on-title-ix-service-awardees.html
Link to section 'Accounts on Bell' of 'Accounts' Accounts on Bell
Link to section 'Obtaining an Account' of 'Accounts' Obtaining an Account
To obtain an account, you must be part of a research group which has purchased access to Bell. Refer to the Accounts / Access page for more details on how to request access.
Link to section 'Outside Collaborators' of 'Accounts' Outside Collaborators
A valid Purdue Career Account is required for access to any resource. If you do not currently have a valid Purdue Career Account you must have a current Purdue faculty or staff member file a Request for Privileges (R4P) before you can proceed.
Purchasing Nodes
RCAC operates a significant shared cluster computing infrastructure developed over several years through focused acquisitions using funds from grants, faculty startup packages, and institutional sources. These "community clusters" are now at the foundation of Purdue's research cyberinfrastructure.
We strongly encourage any Purdue faculty or staff with computational needs to join this growing community and enjoy the enormous benefits this shared infrastructure provides:
- Peace of Mind
RCAC system administrators take care of security patches, attempted hacks, operating system upgrades, and hardware repair so faculty and graduate students can concentrate on research.
- Low Overhead
RCAC data centers provide infrastructure such as networking, racks, floor space, cooling, and power.
- Cost Effective
RCAC works with vendors to obtain the best price for computing resources by pooling funds from different disciplines to leverage greater group purchasing power.
Through the Community Cluster Program, Purdue affiliates have invested several million dollars in computational and storage resources from Q4 2006 to the present with great success in both the research accomplished and the money saved on equipment purchases.
For more information or to purchase access to our latest cluster today, see the Purchase page. Have questions? contact us at rcac-cluster-purchase@lists.purdue.edu to discuss.
Logging In
To submit jobs on Bell, log in to the submission host bell.rcac.purdue.edu
via SSH. This submission host is actually 7 front-end hosts: bell-fe00
through bell-fe06
The login process randomly assigns one of these front-ends to each login to bell.rcac.purdue.edu
.
Purdue Login
Link to section 'SSH' of 'Purdue Login' SSH
- SSH to the cluster as usual.
- When asked for a password, type your password followed by "
,push
". - Your Purdue Duo client will receive a notification to approve the login.
Link to section 'Thinlinc' of 'Purdue Login' Thinlinc
- When asked for a password, type your password followed by "
,push
". - Your Purdue Duo client will receive a notification to approve the login.
- The native Thinlinc client will prompt for Duo approval twice due to the way Thinlinc works.
- The native Thinlinc client also supports key-based authentication.
Jupyterhub
The Jupyterhub service can be accessed from your web browser.
- Open a web browser and navigate to workbench.rcac.purdue.edu.
- Log in with your Purdue Career Account username and password.
Passwords
Bell supports either Purdue two-factor authentication (Purdue Login) or SSH keys.
SSH Client Software
Secure Shell or SSH is a way of establishing a secure connection between two computers. It uses public-key cryptography to authenticate the user with the remote computer and to establish a secure connection. Its usual function involves logging in to a remote machine and executing commands. There are many SSH clients available for all operating systems:
Linux / Solaris / AIX / HP-UX / Unix:
- The
ssh
command is pre-installed. Log in usingssh myusername@bell.rcac.purdue.edu
from a terminal.
Microsoft Windows:
- MobaXterm is a small, easy to use, full-featured SSH client. It includes X11 support for remote displays, SFTP capabilities, and limited SSH authentication forwarding for keys.
Mac OS X:
- The
ssh
command is pre-installed. You may start a local terminal window from "Applications->Utilities". Log in by typing the commandssh myusername@bell.rcac.purdue.edu
.
When prompted for password, enter your Purdue career account password followed by ",push
". Your Purdue Duo client will then receive a notification to approve the login.
SSH Keys
Link to section 'General overview' of 'SSH Keys' General overview
To connect to Bell using SSH keys, you must follow three high-level steps:
- Generate a key pair consisting of a private and a public key on your local machine.
- Copy the public key to the cluster and append it to
$HOME/.ssh/authorized_keys
file in your account. - Test if you can ssh from your local computer to the cluster without using your Purdue password.
Detailed steps for different operating systems and specific SSH client softwares are give below.
Link to section 'Mac and Linux:' of 'SSH Keys' Mac and Linux:
-
Run
ssh-keygen
in a terminal on your local machine. You may supply a filename and a passphrase for protecting your private key, but it is not mandatory. To accept the default settings, press Enter without specifying a filename.
Note: If you do not protect your private key with a passphrase, anyone with access to your computer could SSH to your account on Bell. -
By default, the key files will be stored in
~/.ssh/id_rsa
and~/.ssh/id_rsa.pub
on your local machine. -
Copy the contents of the public key into
$HOME/.ssh/authorized_keys
on the cluster with the following command. When asked for a password, type your password followed by ",push
". Your Purdue Duo client will receive a notification to approve the login.ssh-copy-id -i ~/.ssh/id_rsa.pub myusername@bell.rcac.purdue.edu
Note: use your actual Purdue account user name.
If your system does not have the
ssh-copy-id
command, use this instead:cat ~/.ssh/id_rsa.pub | ssh myusername@bell.rcac.purdue.edu "mkdir -p ~/.ssh && chmod 700 ~/.ssh && cat >> ~/.ssh/authorized_keys"
-
Test the new key by SSH-ing to the server. The login should now complete without asking for a password.
-
If the private key has a non-default name or location, you need to specify the key by
ssh -i my_private_key_name myusername@bell.rcac.purdue.edu
Link to section 'Windows:' of 'SSH Keys' Windows:
Programs | Instructions |
---|---|
MobaXterm | Open a local terminal and follow Linux steps |
Git Bash | Follow Linux steps |
Windows 10 PowerShell | Follow Linux steps |
Windows 10 Subsystem for Linux | Follow Linux steps |
PuTTY | Follow steps below |
PuTTY:
-
Launch PuTTYgen, keep the default key type (RSA) and length (2048-bits) and click Generate button.
The "Generate" button can be found under the "Actions" section of the PuTTY Key Generator interface. -
Once the key pair is generated:
Use the Save public key button to save the public key, e.g.
Documents\SSH_Keys\mylaptop_public_key.pub
Use the Save private key button to save the private key, e.g.
Documents\SSH_Keys\mylaptop_private_key.ppk
. When saving the private key, you can also choose a reminder comment, as well as an optional passphrase to protect your key, as shown in the image below. Note: If you do not protect your private key with a passphrase, anyone with access to your computer could SSH to your account on Bell.The PuTTY Key Generator form has inputs for the Key passphrase and optional reminder comment. From the menu of PuTTYgen, use the "Conversion -> Export OpenSSH key" tool to convert the private key into openssh format, e.g.
Documents\SSH_Keys\mylaptop_private_key.openssh
to be used later for Thinlinc. -
Configure PuTTY to use key-based authentication:
Launch PuTTY and navigate to "Connection -> SSH ->Auth" on the left panel, click Browse button under the "Authentication parameters" section and choose your private key, e.g. mylaptop_private_key.ppk
After clicking Connection -> SSH ->Auth panel, the "Browse" option can be found at the bottom of the resulting panel. Navigate back to "Session" on the left panel. Highlight "Default Settings" and click the "Save" button to ensure the change in place.
-
Connect to the cluster. When asked for a password, type your password followed by "
,push
". Your Purdue Duo client will receive a notification to approve the login. Copy the contents of public key from PuTTYgen as shown below and paste it into$HOME/.ssh/authorized_keys
. Please double-check that your text editor did not wrap or fold the pasted value (it should be one very long line).The "Public key" will look like a long string of random letters and numbers in a text box at the top of the window. - Test by connecting to the cluster. If successful, you will not be prompted for a password or receive a Duo notification. If you protected your private key with a passphrase in step 2, you will instead be prompted to enter your chosen passphrase when connecting.
Accounts on MAPS
If you are on a non-Windows system or a non-ONEPURDUE Windows machine, you can connect to MAPS via Remote Desktop. If you are connecting from off-campus, first you will need to connect to the Purdue VPN.
- Click the Windows/Start menu, click All Programs, click Accessories, then click Remote Desktop Connection. Mac users will need to download Remote Desktop for Mac. Linux users can install rdesktop from the distribution package manager.
- Once in the Remote Desktop application, click the Options button and enter the following information:
- Computer: maps01.rcac.purdue.edu or maps02.rcac.purdue.edu
- Username: ONEPURDUE\your_username
- Click Connect.
- Once logged in, you may interactively access a variety of Geospatial software.
SSH X11 Forwarding
SSH supports tunneling of X11 (X-Windows). If you have an X11 server running on your local machine, you may use X11 applications on remote systems and have their graphical displays appear on your local machine. These X11 connections are tunneled and encrypted automatically by your SSH client.
Link to section 'Installing an X11 Server' of 'SSH X11 Forwarding' Installing an X11 Server
To use X11, you will need to have a local X11 server running on your personal machine. Both free and commercial X11 servers are available for various operating systems.
Linux / Solaris / AIX / HP-UX / Unix:
- An X11 server is at the core of all graphical sessions. If you are logged in to a graphical environment on these operating systems, you are already running an X11 server.
- ThinLinc is an alternative to running an X11 server directly on your Linux computer. ThinLinc is a service that allows you to connect to a persistent remote graphical desktop session.
Microsoft Windows:
- ThinLinc is an alternative to running an X11 server directly on your Windows computer. ThinLinc is a service that allows you to connect to a persistent remote graphical desktop session.
- MobaXterm is a small, easy to use, full-featured SSH client. It includes X11 support for remote displays, SFTP capabilities, and limited SSH authentication forwarding for keys.
Mac OS X:
- X11 is available as an optional install on the Mac OS X install disks prior to 10.7/Lion. Run the installer, select the X11 option, and follow the instructions. For 10.7+ please download XQuartz.
- ThinLinc is an alternative to running an X11 server directly on your Mac computer. ThinLinc is a service that allows you to connect to a persistent remote graphical desktop session.
Link to section 'Enabling X11 Forwarding in your SSH Client' of 'SSH X11 Forwarding' Enabling X11 Forwarding in your SSH Client
Once you are running an X11 server, you will need to enable X11 forwarding/tunneling in your SSH client:
ssh
: X11 tunneling should be enabled by default. To be certain it is enabled, you may usessh -Y
.- MobaXterm: Select "New session" and "SSH." Under "Advanced SSH Settings" check the box for X11 Forwarding.
SSH will set the remote environment variable $DISPLAY
to "localhost:XX.YY"
when this is working correctly. If you had previously set your $DISPLAY
environment variable to your local IP or hostname, you must remove any set
/export
/setenv
of this variable from your login scripts. The environment variable $DISPLAY
must be left as SSH sets it, which is to a random local port address. Setting $DISPLAY
to an IP or hostname will not work.
ThinLinc
RCAC provides Cendio's ThinLinc as an alternative to running an X11 server directly on your computer. It allows you to run graphical applications or graphical interactive jobs directly on Bell through a persistent remote graphical desktop session.
ThinLinc is a service that allows you to connect to a persistent remote graphical desktop session. This service works very well over a high latency, low bandwidth, or off-campus connection compared to running an X11 server locally. It is also very helpful for Windows users who do not have an easy to use local X11 server, as little to no set up is required on your computer.
There are two ways in which to use ThinLinc: preferably through the native client or through a web browser.
Link to section 'Installing the ThinLinc native client' of 'ThinLinc' Installing the ThinLinc native client
The native ThinLinc client will offer the best experience especially over off-campus connections and is the recommended method for using ThinLinc. It is compatible with Windows, Mac OS X, and Linux.
- Download the ThinLinc client from the ThinLinc website.
- Start the ThinLinc client on your computer.
- In the client's login window, use desktop.bell.rcac.purdue.edu as the Server. Use your Purdue Career Account username and password, but append "
,push
" to your password. - Click the Connect button.
- Your Purdue Login Duo will receive a notification to approve your login.
- Continue to following section on connecting to Bell from ThinLinc.
Link to section 'Using ThinLinc through your web browser' of 'ThinLinc' Using ThinLinc through your web browser
The ThinLinc service can be accessed from your web browser as a convenience to installing the native client. This option works with no set up and is a good option for those on computers where you do not have privileges to install software. All that is required is an up-to-date web browser. Older versions of Internet Explorer may not work.
- Open a web browser and navigate to desktop.bell.rcac.purdue.edu.
- Log in with your Purdue Career Account username and password, but append "
,push
" to your password. - You may safely proceed past any warning messages from your browser.
- Your Purdue Login Duo will receive a notification to approve your login.
- Continue to the following section on connecting to Bell from ThinLinc.
Link to section 'Connecting to Bell from ThinLinc' of 'ThinLinc' Connecting to Bell from ThinLinc
- Once logged in, you will be presented with a remote Linux desktop running directly on a cluster front-end.
- Open the terminal application on the remote desktop.
- Once logged in to the Bell head node, you may use graphical editors, debuggers, software like Matlab, or run graphical interactive jobs. For example, to test the X forwarding connection issue the following command to launch the graphical editor gedit:
$ gedit
- This session will remain persistent even if you disconnect from the session. Any interactive jobs or applications you left running will continue running even if you are not connected to the session.
Link to section 'Tips for using ThinLinc native client' of 'ThinLinc' Tips for using ThinLinc native client
- To exit a full screen ThinLinc session press the F8 key on your keyboard (fn + F8 key for Mac users) and click to disconnect or exit full screen.
- Full screen mode can be disabled when connecting to a session by clicking the Options button and disabling full screen mode from the Screen tab.
Link to section 'Configure ThinLinc to use SSH Keys' of 'ThinLinc' Configure ThinLinc to use SSH Keys
- The web client does NOT support public-key authentication.
-
ThinLinc native client supports the use of an SSH key pair. For help generating and uploading keys to the cluster, see SSH Keys section in our user guide for details.
To set up SSH key authentication on the ThinLinc client:
-
Open the Options panel, and select Public key as your authentication method on the Security tab.
The "Options..." button in the ThinLinc Client can be found towards the bottom left, above the "Connect" button. -
In the options dialog, switch to the "Security" tab and select the "Public key" radio button:
The "Security" tab found in the options dialog, will be the last of available tabs. The "Public key" option can be found in the "Authentication method" options group. - Click OK to return to the ThinLinc Client login window. You should now see a Key field in place of the Password field.
-
In the Key field, type the path to your locally stored private key or click the ... button to locate and select the key on your local system. Note: If PuTTY is used to generate the SSH Key pairs, please choose the private key in the openssh format.
The ThinLinc Client login window will now display key field instead of a password field.
-
Link to section 'Rstudio Server' of 'Rstudio Server' Rstudio Server
After Aug 22, 2020, the workbench cluster will NOT support RStudio Server. Please see how to launch RStudio on bell.
File Storage and Transfer
Learn more about file storage transfer for Bell.
Link to section 'Archive and Compression' of 'Archive and Compression' Archive and Compression
There are several options for archiving and compressing groups of files or directories. The mostly commonly used options are:
Link to section 'tar' of 'Archive and Compression' tar
See the official documentation for tar for more information.
Saves many files together into a single archive file, and restores individual files from the archive. Includes automatic archive compression/decompression options and special features for incremental and full backups.
Examples:
(list contents of archive somefile.tar)
$ tar tvf somefile.tar
(extract contents of somefile.tar)
$ tar xvf somefile.tar
(extract contents of gzipped archive somefile.tar.gz)
$ tar xzvf somefile.tar.gz
(extract contents of bzip2 archive somefile.tar.bz2)
$ tar xjvf somefile.tar.bz2
(archive all ".c" files in current directory into one archive file)
$ tar cvf somefile.tar *.c
(archive and gzip-compress all files in a directory into one archive file)
$ tar czvf somefile.tar.gz somedirectory/
(archive and bzip2-compress all files in a directory into one archive file)
$ tar cjvf somefile.tar.bz2 somedirectory/
Other arguments for tar can be explored by using the man tar command.
Link to section 'gzip' of 'Archive and Compression' gzip
The standard compression system for all GNU software.
Examples:
(compress file somefile - also removes uncompressed file)
$ gzip somefile
(uncompress file somefile.gz - also removes compressed file)
$ gunzip somefile.gz
Link to section 'bzip2' of 'Archive and Compression' bzip2
See the official documentation for bzip for more information.
Strong, lossless data compressor based on the Burrows-Wheeler transform. Stronger compression than gzip.
Examples:
(compress file somefile - also removes uncompressed file)
$ bzip2 somefile
(uncompress file somefile.bz2 - also removes compressed file)
$ bunzip2 somefile.bz2
There are several other, less commonly used, options available as well:
- zip
- 7zip
- xz
Link to section 'Storage Environment Variables' of 'Storage Environment Variables' Storage Environment Variables
Several environment variables are automatically defined for you to help you manage your storage. Use environment variables instead of actual paths whenever possible to avoid problems if the specific paths to any of these change.
Name | Description |
---|---|
HOME | /home/myusername |
PWD | path to your current directory |
RCAC_SCRATCH | /scratch/bell/myusername |
By convention, environment variable names are all uppercase. You may use them on the command line or in any scripts in place of and in combination with hard-coded values:
$ ls $HOME
...
$ ls $RCAC_SCRATCH/myproject
...
To find the value of any environment variable:
$ echo $RCAC_SCRATCH
/scratch/bell/myusername
To list the values of all environment variables:
$ env
USER=myusername
HOME=/home/myusername
RCAC_SCRATCH=/scratch/bell/myusername
...
You may create or overwrite an environment variable. To pass (export) the value of a variable in bash:
$ export MYPROJECT=$RCAC_SCRATCH/myproject
To assign a value to an environment variable in either tcsh or csh:
$ setenv MYPROJECT value
Storage Options
File storage options on RCAC systems include long-term storage (home directories, depot, Fortress) and short-term storage (scratch directories, /tmp directory). Each option has different performance and intended uses, and some options vary from system to system as well. Daily snapshots of home directories are provided for a limited time for accidental deletion recovery. Scratch directories and temporary storage are not backed up and old files are regularly purged from scratch and /tmp directories. More details about each storage option appear below.
Home Directory
Home directories are provided for long-term file storage. Each user has one home directory. You should use your home directory for storing important program files, scripts, input data sets, critical results, and frequently used files. You should store infrequently used files on Fortress. Your home directory becomes your current working directory, by default, when you log in.
Your home directory physically resides on a dedicated storage system only accessible for Bell. To find the path to your home directory, first log in then immediately enter the following:
$ pwd
/home/myusername
Or from any subdirectory:
$ echo $HOME
/home/myusername
Please note that your Bell home directory and its contents are exclusive to Bell cluster, including front-end hosts and compute nodes. This home directory is not available on other RCAC machines but Bell. There is no automatic copying or synchronization between home directories, but at your discretion you can manually copy all or parts of your main home to Bell using one of the suggested methods.
Your home directory has a quota limiting the total size of files you may store within. For more information, refer to the Storage Quotas / Limits Section.
Link to section 'Lost File Recovery' of 'Home Directory' Lost File Recovery
Nightly snapshots for 7 days, weekly snapshots for 4 weeks, and monthly snapshots for 3 months are kept. This means you will find snapshots from the last 7 nights, the last 4 Sundays, and the last 3 first of the months. Files are available going back between two and three months, depending on how long ago the last first of the month was. Snapshots beyond this are not kept. For additional security, you should store another copy of your files on more permanent storage, such as the Fortress HPSS Archive.
Link to section 'Performance' of 'Home Directory' Performance
Your home directory is medium-performance, non-purged space suitable for tasks like sharing data, editing files, developing and building software, and many other uses.
Your home directory is not designed or intended for use as high-performance working space for running data-intensive jobs with heavy I/O demands.
Link to section 'Long-Term Storage' of 'Long-Term Storage' Long-Term Storage
Long-term Storage or Permanent Storage is available to users on the High Performance Storage System (HPSS), an archival storage system, called Fortress. Program files, data files and any other files which are not used often, but which must be saved, can be put in permanent storage. Fortress currently has over 10PB of capacity.
For more information about Fortress, how it works, and user guides, and how to obtain an account:
Scratch Space
Scratch directories are provided for short-term file storage only. The quota of your scratch directory is much greater than the quota of your home directory. You should use your scratch directory for storing temporary input files which your job reads or for writing temporary output files which you may examine after execution of your job. You should use your home directory and Fortress for longer-term storage or for holding critical results. The hsi and htar commands provide easy-to-use interfaces into the archive and can be used to copy files into the archive interactively or even automatically at the end of your regular job submission scripts.
Files in scratch directories are not recoverable. Files in scratch directories are not backed up. If you accidentally delete a file, a disk crashes, or old files are purged, they cannot be restored.
Files are purged from scratch directories not accessed or had content modified in 30 days. Owners of these files receive a notice one week before removal via email. Be sure to regularly check your Purdue email account or set up mail forwarding to an email account you do regularly check. For more information, please refer to our Scratch File Purging Policy.
All users may access scratch directories on Bell. To find the path to your scratch directory:
$ findscratch
/scratch/bell/myusername
The value of variable $RCAC_SCRATCH is your scratch directory path. Use this variable in any scripts. Your actual scratch directory path may change without warning, but this variable will remain current.
$ echo $RCAC_SCRATCH
/scratch/bell/myusername
Scratch directories are specific per cluster. I.e. only the /scratch/bell directory is available on Bell front-end and compute nodes. No other scratch directories are available on Bell.
Your scratch directory has a quota capping the total size and number of files you may store in it. For more information, refer to the section Storage Quotas / Limits.
Link to section 'Performance' of 'Scratch Space' Performance
Your scratch directory is located on a high-performance, large-capacity parallel filesystem engineered to provide work-area storage optimized for a wide variety of job types. It is designed to perform well with data-intensive computations, while scaling well to large numbers of simultaneous connections.
/tmp Directory
/tmp directories are provided for short-term file storage only. Each front-end and compute node has a /tmp directory. Your program may write temporary data to the /tmp
directory of the compute node on which it is running. That data is available for as long as your program is active. Once your program terminates, that temporary data is no longer available. When used properly, /tmp
may provide faster local storage to an active process than any other storage option. You should use your home directory and Fortress for longer-term storage or for holding critical results.
Backups are not performed for the /tmp
directory and removes files from /tmp
whenever space is low or whenever the system needs a reboot. In the event of a disk crash or file purge, files in /tmp
are not recoverable. You should copy any important files to more permanent storage.
Storage Quota / Limits
Some limits are imposed on your disk usage on research systems. A quota is implemented on each filesystem. Each filesystem (home directory, scratch directory, etc.) may have a different limit. If you exceed the quota, you will not be able to save new files or new data to the filesystem until you delete or move data to long-term storage.
Link to section 'Checking Quota' of 'Storage Quota / Limits' Checking Quota
To check the current quotas of your home and scratch directories check the My Quota page or use the myquota
command:
$ myquota
Type Filesystem Size Limit Use Files Limit Use
==============================================================================
home myusername 5.0GB 25.0GB 20% - - -
scratch bell 220.7GB 100.0TB 0.22% 8k 2,000k 0.43%
The columns are as follows:
- Type: indicates home or scratch directory or your depot space.
- Filesystem: name of storage option.
- Size: sum of file sizes in bytes.
- Limit: allowed maximum on sum of file sizes in bytes.
- Use: percentage of file-size limit currently in use.
- Files: number of files and directories (not the size).
- Limit: allowed maximum on number of files and directories. It is possible, though unlikely, to reach this limit and not the file-size limit if you create a large number of very small files.
- Use: percentage of file-number limit currently in use.
If you find that you reached your quota in either your home directory or your scratch file directory, obtain estimates of your disk usage. Find the top-level directories which have a high disk usage, then study the subdirectories to discover where the heaviest usage lies.
To see in a human-readable format an estimate of the disk usage of your top-level directories in your home directory:
$ du -h --max-depth=1 $HOME >myfile
32K /home/myusername/mysubdirectory_1
529M /home/myusername/mysubdirectory_2
608K /home/myusername/mysubdirectory_3
The second directory is the largest of the three, so apply command du to it.
To see in a human-readable format an estimate of the disk usage of your top-level directories in your scratch file directory:
$ du -h --max-depth=1 $RCAC_SCRATCH >myfile
160K /scratch/bell/myusername
This strategy can be very helpful in figuring out the location of your largest usage. Move unneeded files and directories to long-term storage to free space in your home and scratch directories.
Link to section 'Increasing Quota' of 'Storage Quota / Limits' Increasing Quota
Link to section 'Home Directory' of 'Storage Quota / Limits' Home Directory
If you find you need additional disk space in your home directory, please consider archiving and compressing old files and moving them to long-term storage on the Fortress HPSS Archive, or purchase the Depot space for long-term storage. Unfortunately, it is not possible to increase your home directory quota beyond it's current level.
Link to section 'Scratch Space' of 'Storage Quota / Limits' Scratch Space
If you find you need additional disk space in your scratch space, please first consider archiving and compressing old files and moving them to long-term storage on the Fortress HPSS Archive. If you are unable to do so, you may ask for a quota increase by contacting support.
Link to section 'Sharing Files from Bell' of 'Sharing' Sharing Files from Bell
Bell supports several methods for file sharing. Use the links below to learn more about these methods.
Link to section 'Sharing Data with Globus' of 'Globus' Sharing Data with Globus
Data on any RCAC resource can be shared with other users within Purdue or with collaborators at other institutions. Globus allows convenient sharing of data with outside collaborators. Data can be shared with collaborators' personal computers or directly with many other computing resources at other institutions.
To share files, login to https://transfer.rcac.purdue.edu, navigate to the endpoint (collection) of your choice, and follow instructions as described in Globus documentation on how to share data:
See also RCAC Globus presentation.
File Transfer
Bell supports several methods for file transfer. Use the links below to learn more about these methods.
SCP
SCP (Secure CoPy) is a simple way of transferring files between two machines that use the SSH protocol. SCP is available as a protocol choice in some graphical file transfer programs and also as a command line program on most Linux, Unix, and Mac OS X systems. SCP can copy single files, but will also recursively copy directory contents if given a directory name.
After Aug 17, 2020, the community clusters will not support password-based authentication for login. Methods that can be used include two-factor authentication (Purdue Login) or SSH keys. If you do not have SSH keys installed, you would need to type your Purdue Login response into the SFTP's "Password" prompt.
Link to section 'Command-line usage:' of 'SCP' Command-line usage:
You can transfer files both to and from Bell while initiating an SCP session on either some other computer or on Bell (in other words, directionality of connection and directionality of data flow are independent from each other). The scp
command appears somewhat similar to the familiar cp
command, with an extra user@host:file
syntax to denote files and directories on a remote host. Either Bell or another computer can be a remote.
-
Example: Initiating SCP session on some other computer (i.e. you are on some other computer, connecting to Bell):
(transfer TO Bell) (Individual files) $ scp sourcefile myusername@bell.rcac.purdue.edu:somedir/destinationfile $ scp sourcefile myusername@bell.rcac.purdue.edu:somedir/ (Recursive directory copy) $ scp -pr sourcedirectory/ myusername@bell.rcac.purdue.edu:somedir/ (transfer FROM Bell) (Individual files) $ scp myusername@bell.rcac.purdue.edu:somedir/sourcefile destinationfile $ scp myusername@bell.rcac.purdue.edu:somedir/sourcefile somedir/ (Recursive directory copy) $ scp -pr myusername@bell.rcac.purdue.edu:sourcedirectory somedir/
The -p flag is optional. When used, it will cause the transfer to preserve file attributes and permissions. The -r flag is required for recursive transfers of entire directories.
-
Example: Initiating SCP session on Bell (i.e. you are on Bell, connecting to some other computer):
(transfer TO Bell) (Individual files) $ scp myusername@$another.computer.example.com:sourcefile somedir/destinationfile $ scp myusername@$another.computer.example.com:sourcefile somedir/ (Recursive directory copy) $ scp -pr myusername@$another.computer.example.com:sourcedirectory/ somedir/ (transfer FROM Bell) (Individual files) $ scp somedir/sourcefile myusername@$another.computer.example.com:destinationfile $ scp somedir/sourcefile myusername@$another.computer.example.com:somedir/ (Recursive directory copy) $ scp -pr sourcedirectory myusername@$another.computer.example.com:somedir/
The -p flag is optional. When used, it will cause the transfer to preserve file attributes and permissions. The -r flag is required for recursive transfers of entire directories.
Link to section 'Software (SCP clients)' of 'SCP' Software (SCP clients)
Linux and other Unix-like systems:
- The
scp
command-line program should already be installed.
Microsoft Windows:
- MobaXterm
Free, full-featured, graphical Windows SSH, SCP, and SFTP client. - Command-line
scp
program can be installed as part of Windows Subsystem for Linux (WSL), or Git-Bash.
Mac OS X:
- The
scp
command-line program should already be installed. You may start a local terminal window from "Applications->Utilities". - Cyberduck is a full-featured and free graphical SFTP and SCP client.
Globus
Link to section 'Globus' of 'Globus' Globus
Globus, previously known as Globus Online, is a powerful and easy to use file transfer service for transferring files virtually anywhere. It works within RCAC's various research storage systems; it connects between RCAC and remote research sites running Globus; and it connects research systems to personal systems. You may use Globus to connect to your home, scratch, and Fortress storage directories. Since Globus is web-based, it works on any operating system that is connected to the internet. The Globus Personal client is available on Windows, Linux, and Mac OS X. It is primarily used as a graphical means of transfer but it can also be used over the command line.
Link to section 'Link to section 'Globus Web:' of 'Globus' Globus Web:' of 'Globus' Link to section 'Globus Web:' of 'Globus' Globus Web:
- Navigate to http://transfer.rcac.purdue.edu
- Click "Proceed" to log in with your Purdue Career Account.
- On your first login it will ask to make a connection to a Globus account. Accept the conditions.
- Now you are at the main screen. Click "File Transfer" which will bring you to a two-panel interface (if you only see one panel, you can use selector in the top-right corner to switch the view).
- You will need to select one collection and file path on one side as the source, and the second collection on the other as the destination. This can be one of several Purdue endpoints, or another University, or even your personal computer (see Personal Client section below).
The RCAC collections are as follows. A search for "Purdue" will give you several suggested results you can choose from, or you can give a more specific search.
- Home Directory storage: "Purdue Research Computing - Home Directories", however, you can start typing "Purdue" and "Home Directories" and it will suggest appropriate matches.
- Weber scratch storage: "Purdue Weber Cluster", however, you can start typing "Purdue" and "Weber and it will suggest appropriate matches. From here you will need to navigate into the first letter of your username, and then into your username.
- Research Data Depot: "Purdue Research Computing - Data Depot", a search for "Depot" should provide appropriate matches to choose from.
- Fortress: "Purdue Fortress HPSS Archive", a search for "Fortress" should provide appropriate matches to choose from.
From here, select a file or folder in either side of the two-pane window, and then use the arrows in the top-middle of the interface to instruct Globus to move files from one side to the other. You can transfer files in either direction. You will receive an email once the transfer is completed.
Link to section 'Link to section 'Globus Personal Client setup:' of 'Globus' Globus Personal Client setup:' of 'Globus' Link to section 'Globus Personal Client setup:' of 'Globus' Globus Personal Client setup:
Globus Connect Personal is a small software tool you can install to make your own computer a Globus endpoint on its own. It is useful if you need to transfer files via Globus to and from your computer directly.
- On the "Collections" page from earlier, click "Get Globus Connect Personal" or download a version for your operating system it from here: Globus Connect Personal
- Name this particular personal system and follow the setup prompts to create your Globus Connect Personal endpoint.
- Your personal system is now available as a collection within the Globus transfer interface.
Link to section 'Link to section 'Globus Command Line:' of 'Globus' Globus Command Line:' of 'Globus' Link to section 'Globus Command Line:' of 'Globus' Globus Command Line:
Globus supports command line interface, allowing advanced automation of your transfers.
To use the recommended standalone Globus CLI application (the globus command):
- First time use: issue the globus login command and follow instructions for initial login.
- Commands for interfacing with the CLI can be found via Using the Command Line Interface, as well as the Globus CLI Examples pages.
Link to section 'Link to section 'Sharing Data with Outside Collaborators' of 'Globus' Sharing Data with Outside Collaborators' of 'Globus' Link to section 'Sharing Data with Outside Collaborators' of 'Globus' Sharing Data with Outside Collaborators
Globus allows convenient sharing of data with outside collaborators. Data can be shared with collaborators' personal computers or directly with many other computing resources at other institutions. See the Globus documentation on how to share data:
For links to more information, please see Globus Support page and RCAC Globus presentation.
Windows Network Drive / SMB
SMB (Server Message Block), also known as CIFS, is an easy to use file transfer protocol that is useful for transferring files between RCAC systems and a desktop or laptop. You may use SMB to connect to your home, scratch, and Fortress storage directories. The SMB protocol is available on Windows, Linux, and Mac OS X. It is primarily used as a graphical means of transfer but it can also be used over the command line.
Note: to access Bell through SMB file sharing, you must be on a Purdue campus network or connected through VPN.
Link to section 'Windows:' of 'Windows Network Drive / SMB' Windows:
- Windows 7: Click Windows menu > Computer, then click Map Network Drive in the top bar
- Windows 8 & 10: Tap the Windows key, type computer, select This PC, click Computer > Map Network Drive in the top bar
- Windows 11: Tap the Windows key, type File Explorer, select This PC, click Computer > Map Network Drive in the top bar
- In the folder location enter the following information and click Finish:
- To access your Bell home directory, enter \\home.bell.rcac.purdue.edu\bell-home.
- To access your scratch space on Bell, enter \\scratch.bell.rcac.purdue.edu\bell-scratch. Once mapped, you will be able to navigate to your scratch directory.
- Note: Use your career account login name and password when prompted. (You will not need to add "
,push
" nor use your Purdue Duo client.) - Your home or scratch directory should now be mounted as a drive in the Computer window.
Link to section 'Mac OS X:' of 'Windows Network Drive / SMB' Mac OS X:
- In the Finder, click Go > Connect to Server
- In the Server Address enter the following information and click Connect:
- To access your Bell home directory, enter smb://home.bell.rcac.purdue.edu/bell-home.
- To access your scratch space on Bell, enter smb://scratch.bell.rcac.purdue.edu/bell-scratch. Once mapped, you will be able to navigate to your scratch directory.
- Note: Use your career account login name and password when prompted. (You will not need to add "
,push
" nor use your Purdue Duo client.) - Your home or scratch directory should now be mounted as a drive in the Computer window.
Link to section 'Linux:' of 'Windows Network Drive / SMB' Linux:
- There are several graphical methods to connect in Linux depending on your desktop environment. Once you find out how to connect to a network server on your desktop environment, choose the Samba/SMB protocol and adapt the information from the Mac OS X section to connect.
- If you would like access via samba on the command line you may install smbclient which will give you FTP-like access and can be used as shown below. For all the possible ways to connect look at the Mac OS X instructions.
smbclient //home.bell.rcac.purdue.edu/bell-home -U myusername smbclient //scratch.bell.rcac.purdue.edu/bell-scratch -U myusername
- Note: Use your career account login name and password when prompted. (You will not need to add "
,push
" nor use your Purdue Duo client.)
FTP / SFTP
FTP is not supported on any research systems because it does not allow for secure transmission of data. Use SFTP instead, as described below.
SFTP (Secure File Transfer Protocol) is a reliable way of transferring files between two machines. SFTP is available as a protocol choice in some graphical file transfer programs and also as a command-line program on most Linux, Unix, and Mac OS X systems. SFTP has more features than SCP and allows for other operations on remote files, remote directory listing, and resuming interrupted transfers. Command-line SFTP cannot recursively copy directory contents; to do so, try using SCP or graphical SFTP client.
After Aug 17, 2020, the community clusters will not support password-based authentication for login. Methods that can be used include two-factor authentication (Purdue Login) or SSH keys. If you do not have SSH keys installed, you would need to type your Purdue Login response into the SFTP's "Password" prompt.
Link to section 'Command-line usage' of 'FTP / SFTP' Command-line usage
You can transfer files both to and from Bell while initiating an SFTP session on either some other computer or on Bell (in other words, directionality of connection and directionality of data flow are independent from each other). Once the connection is established, you use put
or get
subcommands between "local" and "remote" computers. Either Bell or another computer can be a remote.
-
Example: Initiating SFTP session on some other computer (i.e. you are on another computer, connecting to Bell):
$ sftp myusername@bell.rcac.purdue.edu (transfer TO Bell) sftp> put sourcefile somedir/destinationfile sftp> put -P sourcefile somedir/ (transfer FROM Bell) sftp> get sourcefile somedir/destinationfile sftp> get -P sourcefile somedir/ sftp> exit
The -P flag is optional. When used, it will cause the transfer to preserve file attributes and permissions.
-
Example: Initiating SFTP session on Bell (i.e. you are on Bell, connecting to some other computer):
$ sftp myusername@$another.computer.example.com (transfer TO Bell) sftp> get sourcefile somedir/destinationfile sftp> get -P sourcefile somedir/ (transfer FROM Bell) sftp> put sourcefile somedir/destinationfile sftp> put -P sourcefile somedir/ sftp> exit
The -P flag is optional. When used, it will cause the transfer to preserve file attributes and permissions.
Link to section 'Software (SFTP clients)' of 'FTP / SFTP' Software (SFTP clients)
Linux and other Unix-like systems:
- The
sftp
command-line program should already be installed.
Microsoft Windows:
- MobaXterm
Free, full-featured, graphical Windows SSH, SCP, and SFTP client. - Command-line
sftp
program can be installed as part of Windows Subsystem for Linux (WSL), or Git-Bash.
Mac OS X:
- The
sftp
command-line program should already be installed. You may start a local terminal window from "Applications->Utilities". - Cyberduck is a full-featured and free graphical SFTP and SCP client.
Copying files from Purdue IT research computing home directory to Bell
The Bell home directory and its contents are specific to the Bell cluster, and are not available on other RCAC machines. For people having access to other Community Clusters and Bell, there is no automatic copying or synchronization between main and Bell home directories. At your discretion, you can manually copy all or parts of your main research computing home to Bell using one of the methods described below.
Please note that copying may fail if the size of your research computing home directory is larger than the Bell one's quota. Please check usage and limits before proceeding!
Link to section 'Complete copy' of 'Copying files from Purdue IT research computing home directory to Bell' Complete copy
For your convenience, a custom tool copy-rcac-home
is provided to simplify at-will duplication of your main research computing home directory into Bell. The tool performs a complete 1-to-1 copy using rsync -auH
(with exception of a narrow subset of system-specific service files).
To use the tool, simply type copy-rcac-home
in a terminal window on a Bell front-end or compute node:
$ copy-rcac-home
This script will copy entire contents of your main RCAC
home directory into your Bell cluster's $HOME.
Note: copying may fail if the size of your RCAC home directory
is larger than your quota on the Bell one (25GB).
BEFORE PROCEEDING, please run 'myquota' command on another
cluster to see your usage there and judge whether it would fit!
Would you like to proceed? [Y/n]:
At this stage answering yes
will proceed with copying, or you can respond with a no
(or Ctrl-C
) to cancel. See copy-rcac-home --help
for more details on the tool.
Link to section 'Partial copy' of 'Copying files from Purdue IT research computing home directory to Bell' Partial copy
Desired parts (or whole) of your research computing home directories can be copied to Bell via any of the home directories' supported transfer methods, such as SCP, SFTP, rsync, or Globus.
-
Example: recursive copying of a subdirectory from RCAC home directory into Bell home using
scp
.(if you are on Bell, use other cluster name for the remote part) $ scp -pr myothercluster.rcac.purdue.edu:somedirectory/ ~/ (if you are on another cluster, use Bell for the remote part) $ scp -pr somedirectory/ myusername@bell.rcac.purdue.edu:~/
-
Example: copying using Globus.
Search collections for "Purdue Research Computing - Home Directories" and "Purdue Bell Cluster - Home" endpoints, respectively, then transfer desired files and/or directories as usual.
Lost File Recovery
Bell is protected against accidental file deletion through a series of snapshots taken every night just after midnight. Each snapshot provides the state of your files at the time the snapshot was taken. It does so by storing only the files which have changed between snapshots. A file that has not changed between snapshots is only stored once but will appear in every snapshot. This is an efficient method of providing snapshots because the snapshot system does not have to store multiple copies of every file.
These snapshots are kept for a limited time at various intervals. RCAC keeps nightly snapshots for 7 days, weekly snapshots for 4 weeks, and monthly snapshots for 3 months. This means you will find snapshots from the last 7 nights, the last 4 Sundays, and the last 3 first of the months. Files are available going back between two and three months, depending on how long ago the last first of the month was. Snapshots beyond this are not kept.
Only files which have been saved during an overnight snapshot are recoverable. If you lose a file the same day you created it, the file is not recoverable because the snapshot system has not had a chance to save the file.
Snapshots are not a substitute for regular backups. It is the responsibility of the researchers to back up any important data to the Fortress Archive. Bell does protect against hardware failures or physical disasters through other means however these other means are also not substitutes for backups.
Files in scratch directories are not recoverable. Files in scratch directories are not backed up. If you accidentally delete a file, a disk crashes, or old files are purged, they cannot be restored.
Bell offers several ways for researchers to access snapshots of their files.
flost
If you know when you lost the file, the easiest way is to use the flost command. This tool is available from any RCAC resource. If you do not have access to a compute cluster, any Data Depot user may use an SSH client to connect to bell.rcac.purdue.edu and run this command.
To run the tool you will need to specify the location where the lost file was with the -w argument:
$ flost -w /depot/mylab
Replace mylab with the name of your lab's Bell directory. If you know more specifically where the lost file was you may provide the full path to that directory.
This tool will prompt you for the date on which you lost the file or would like to recover the file from. If the tool finds an appropriate snapshot it will provide instructions on how to search for and recover the file.
If you are not sure what date you lost the file you may try entering different dates into the flost to try to find the file or you may also manually browse the snapshots as described below.
Manual Browsing
You may also search through the snapshots by hand on the Bell filesystem if you are not sure what date you lost the file or would like to browse by hand. Snapshots can be browsed from any RCAC resource. If you do not have access to a compute cluster, any Bell user may use an SSH client to connect to bell.rcac.purdue.edu and browse from there. The snapshots are located at /depot/.snapshots
on these resources.
You can also mount the snapshot directory over Samba (or SMB, CIFS) on Windows or Mac OS X. Mount (or map) the snapshot directory in the same way as you did for your main Bell space substituting the server name and path for \\datadepot.rcac.purdue.edu\depot\.winsnaps
(Windows) or smb://datadepot.rcac.purdue.edu/depot/.winsnaps
(Mac OS X).
Once connected to the snapshot directory through SSH or Samba, you will see something similar to this:
SSH to bell.rcac.purdue.edu | Samba mount on datadepot.rcac.purdue.edu |
---|---|
|
![]() |
Each of these directories is a snapshot of the entire Bell filesystem at the timestamp encoded into the directory name. The format for this timestamp is year, two digits for month, two digits for day, followed by the time of the day.
You may cd into any of these directories where you will find the entire Bell filesystem. Use cd to continue into your lab's Bell space and then you may browse the snapshot as normal.
If you are browsing these directories over a Samba network drive you can simply drag and drop the files over into your live Data Depot folder.
Once you find the file you are looking for, use cp to copy the file back into your lab's live Bell space. Do not attempt to modify files directly in the snapshot directories.
Windows
If you use Bell through "network drives" on Windows you may recover lost files directly from within Windows:
- Open the folder that contained the lost file.
- Right click inside the window and select "Properties".
- Click on the "Previous Versions" tab.
- A list of snapshots will be displayed.
- Select the snapshot from which you wish to restore.
- In the new window, locate the file you wish to restore.
- Simply drag the file or folder to their correct locations.
In the "Previous Versions" window the list contains two columns. The first column is the timestamp on which the snapshot was taken. The second column is the date on which the selected file or folder was last modified in that snapshot. This may give you some extra clues to which snapshot contains the version of the file you are looking for.
Mac OS X
Mac OS X does not provide any way to access the Bell snapshots directly. To access the snapshots there are two options: browse the snapshots by hand through a network drive mount or use an automated command-line based tool.
To browse the snapshots by hand, follow the directions outlined in the Manual Browsing section.
To use the automated command-line tool, log into a compute cluster or into the host bell.rcac.purdue.edu (which is available to all Bell users) and use the flost tool. On Mac OS X you can use the built-in SSH terminal application to connect.
- Open the Applications folder from Finder.
- Navigate to the Utilities folder.
- Double click the Terminal application to open it.
- Type the following command when the terminal opens.
Replace myusername with your Purdue career account username and provide your password when prompted.$ ssh myusername@bell.rcac.purdue.edu
Once logged in use the flost tool as described above. The tool will guide you through the process and show you the commands necessary to retrieve your lost file.
Gateway (Open OnDemand)
Bell's Gateway is an open-source HPC portal developed by the Ohio Supercomputing Center. Open OnDemand allows one to interact with HPC resources through a web browser and easily manage files, submit jobs, and interact with graphical applications directly in a browser, all with no software to install. Bell has an instance of OnDemand available that can be accessed via gateway.bell.rcac.purdue.edu.
Link to section 'Logging In' of 'Gateway (Open OnDemand)' Logging In
To log into Gateway:
- Navigate to gateway.bell.rcac.purdue.edu
- Log in using your Career account username and Purdue Login Duo client.
On the splash page you will see a quota usage report. If you are over 90% on any of your quotas a warning will be displayed. This information will update every 10-15 minutes while you are active on Gateway.
Link to section 'Apps' of 'Gateway (Open OnDemand)' Apps
There are a number of built-in apps in Gateway that can be accessed from the top menu bar. Below are links to documentation on each app.
Interactive Apps
There are several interactive apps available through Gateway that can be accessed through the Interactive Apps dropdown menu. These apps are provided with a basic node and software configuration as a 'quick-launch' option to get your work up and running quickly. For simplicity, minimal options are provided - these apps are not intended for complex configuration/customization scenarios.
After you a submit an interactive app to the queue, Gateway will track and manage the session. Once it starts, you may connect and disconnect from the session in your browser, leaving the job running while you log out of your browser.
Each of the available apps are documented through the following links.
Compute Node Desktop
The Compute Node Desktop app will launch a graphical desktop session on a compute node. This is similar to using Thinlinc, however, this gives you a desktop directly on a compute node instead on a front-end. This app is useful if you have a custom application or application not directly available as an interactive app you would like to run inside Gateway.
To launch a desktop session on a compute node, select the Bell Compute Desktop app. From the submit form, select from the available options - the queue to which you wish to submit and the number of wallclock hours you wish to have job running. There is also a checkbox that enable a notification to your email when the job starts.
After the interactive job is submitted you will be taken to your list of active interactive app sessions. You can monitor the status of the job from here until it starts, or if you enabled the email notification, watch your Purdue email for the notification the job has started.
Once it is indicated the job has started you can connect to the desktop with the "Launch noVNC in New Tab" button. The session will be terminated after the wallclock hours you specified have elapsed or you terminate the session early with the "Delete" button from the list of sessions. Deleting the session when you are finished will free up queue resources for your lab mates and other users on the system.
Jupyter Notebook
The Notebook app will launch a Notebook session on a compute node and allow you to connect directly to it in a web browser.
To launch a Notebook session on a compute node, select the Notebook app. From the submit form, select from the available options:
- Queue: This is a dropdown menu from which you can select a queue from all of the queues to which you have permission to submit.
- Walltime: This is a field which expects a number and represents how many hours you want to keep the session running. Note that this value should not exceed the maximum value given next to the selected queue name from the queue dropdown menu.
- Number of Cores/GPUs: This is a field which expects a number and represents the number of your resources your session is requesting. Note that the amount of memory allocated for your session is proportional to the number of cores or GPUs that you request for your job, so if your session is running out of memory, consider increasing this value.
- Use Jupyter Lab: This is a checkbox which, when checked, will run Jupyter Lab instead of Jupyter Notebook. Both of these applications are interfaces to Jupyter, and you can launch Jupyter notebooks from within Jupyter Lab. Jupyter Notebook is more "barebones" while Jupyter Lab has additional features such as the ability to interact with additional file types.
- E-mail Notice: This is a checkbox which, when checked, will send you an e-mail notification to your Purdue e-mail that your session is ready when the scheduler has found resources to dedicate to your session.
After the interactive job is submitted you will be taken to your list of active interactive app sessions. You can monitor the status of the job from here until it starts, or if you enabled the email notification, watch your Purdue email for the notification the job has started.
Once it is indicated the job has started you can connect to the desktop with the "Connect to Jupyter" button. Once connected, you can create new notebooks, selecting the currently available Anaconda versions available as modules, and any personally created Notebook kernels.
Often times you may want to use one of your existing Anaconda environments within your Jupyter session to use libraries specific to your workflow. In order to do so, you must ensure that the Anaconda environment you want to use contains the Python packages "IPyKernel" and "IPython" which are packages that are required by Jupyter. When you create a Jupyter session, Open OnDemand will check through your existing Anaconda environments and create a Jupyter kernel for any Anaconda environment that contains these two packages, and you will be able to select to use that kernel from within the application.
The session will be terminated after the number of hours you specified have elapsed or you terminate the session early with the "Delete" button from the list of sessions. Deleting the session when you are finished will free up queue resources for your lab mates and other users on the system.
MATLAB
The MATLAB app will launch a MATLAB session on a compute node and allow you to connect directly to it in a web browser.
To launch a MATLAB session on a compute node, select the MATLAB app. From the submit form, select from the available options - the version of MATLAB you are interested in running, the queue to which you wish to submit, and the number of wallclock hours you wish to have job running. There is also a checkbox that enable a notification to your email when the job starts.
After the interactive job is submitted you will be taken to your list of active interactive app sessions. You can monitor the status of the job from here until it starts, or if you enabled the email notification, watch your Purdue email for the notification the job has started.
Once it is indicated the job has started you can connect to the desktop with the "Launch noVNC in New Tab" button. The session will be terminated after the wallclock hours you specified have elapsed or you terminate the session early with the "Delete" button from the list of sessions. Deleting the session when you are finished will free up queue resources for your lab mates and other users on the system.
RStudio Server
The RStudio app will launch a RStudio session on a compute node and allow you to connect directly to it in a web browser.
To launch a RStudio session on a compute node, select the RStudio app. From the submit form, select from the available options - the queue to which you wish to submit, and the number of wallclock hours you wish to have job running. There is also a checkbox that enable a notification to your email when the job starts.
After the interactive job is submitted you will be taken to your list of active interactive app sessions. You can monitor the status of the job from here until it starts, or if you enabled the email notification, watch your Purdue email for the notification the job has started.
Once it is indicated the job has started you can connect to the desktop with the "Connect to RStudio Server" button. The session will be terminated after the wallclock hours you specified have elapsed or you terminate the session early with the "Delete" button from the list of sessions. Deleting the session when you are finished will free up queue resources for your lab mates and other users on the system.
Files
The Files app will let you access your files in your Home Directory, Scratch, and Data Depot spaces. The app lets you manage create, manage, and delete files and directories from your web browser. Navigate by double clicking on folders in the file explorer or by using the file tree on the left.
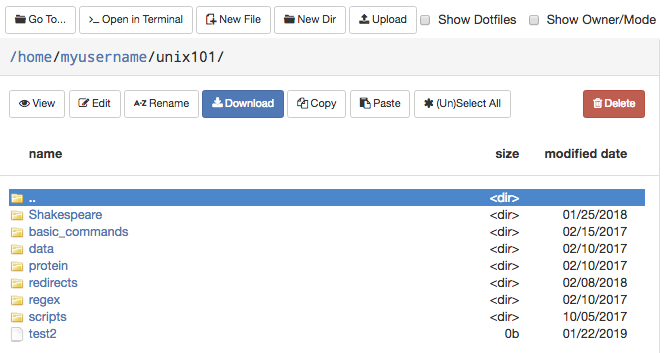
On the top row, there are buttons to:
- Go To: directly input a directory to navigate to
- Open in Terminal: launches the Shell app and navigates you to the current directory in the terminal
- New File: creates a new, empty file
- New Dir: creates a new, empty directory
- Upload: upload a file from your computer
Note: File uploads from your browser are limited to 100 GB per file. Be mindful that uploads over a few gigabytes may be unreliable through your browser, especially from off-campus connections. For very large files or off-campus transfers alternative methods such as Globus are highly recommended.
The second row of buttons lets you perform typical file management operations. The Edit button will open files in a fully fledged browser based text editor - it features syntax highlighting and vim and Emacs key bindings.
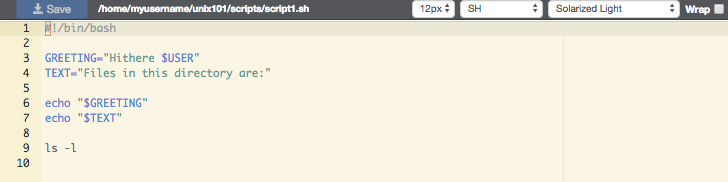
Jobs
There are two apps under the Jobs apps: Active Jobs and Job Composer. These are detailed below.
Link to section 'Active Jobs' of 'Jobs' Active Jobs
This shows you active SLURM jobs currently on the cluster. The default view will show you your current jobs, similar to squeue -u rices
. Using the button labeled "Your Jobs" in the upper right allows you to select different filters by queue (account). All accounts output by slist
will appear for you here. Using the arrow on the left hand side will expand the full job details.
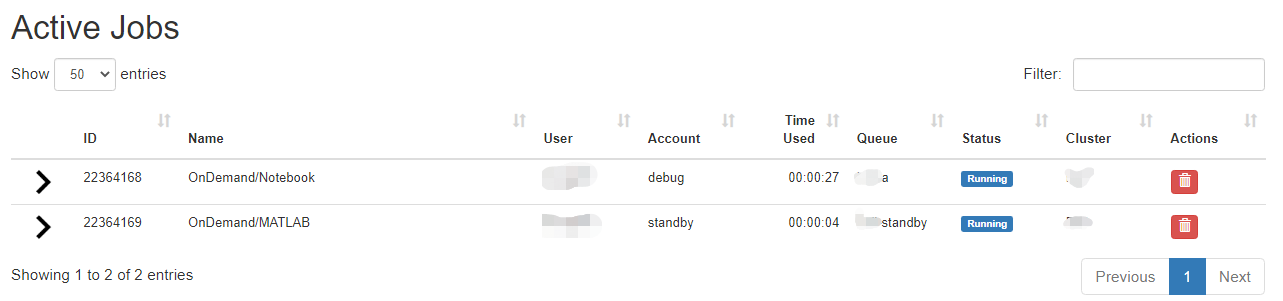
Link to section 'Job Composer' of 'Jobs' Job Composer
The Job Composer app allows you to create and submit jobs to the cluster. You can select from pre-defined templates (most of these are taken from the User Guide examples) or you can create your own templates for frequently used workflows.
Link to section 'Creating Job from Existing Template' of 'Jobs' Creating Job from Existing Template
Click "New Job" menu, then select "From Template":
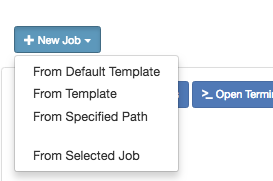
Then select from one of the available templates.
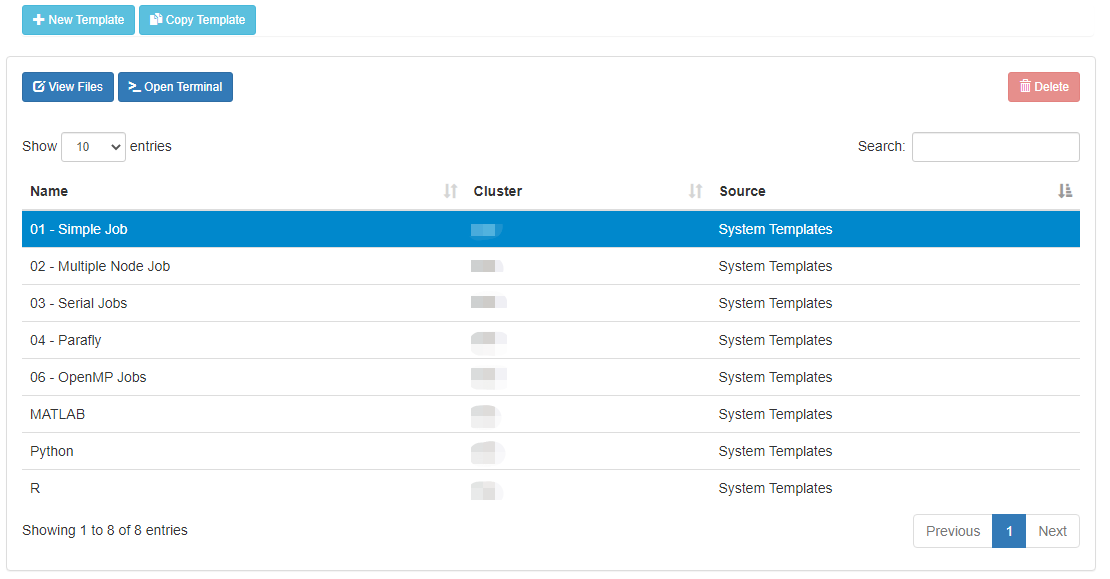
Click 'Create New Job' in second pane.
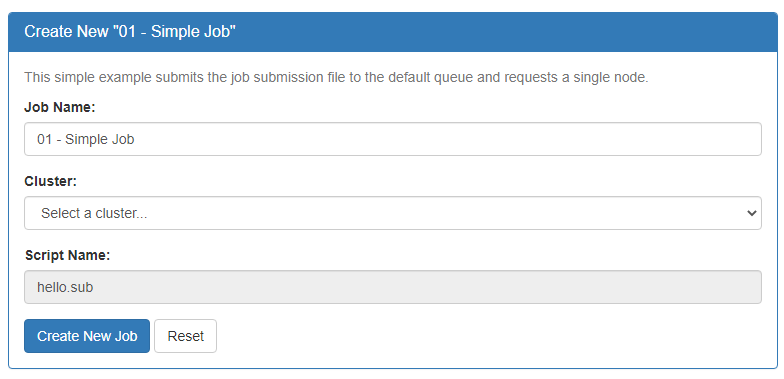
Your new job should be selected in your list of jobs. In the 'Submit Script' pane you can see the job script that was generated with an 'Open Editor' link to open the script in the built-in editor. Open the file in the editor and edit the script as necessary. By default the job will specify standby queue - this should be changed as appropriate, along with the node and walltime requests.
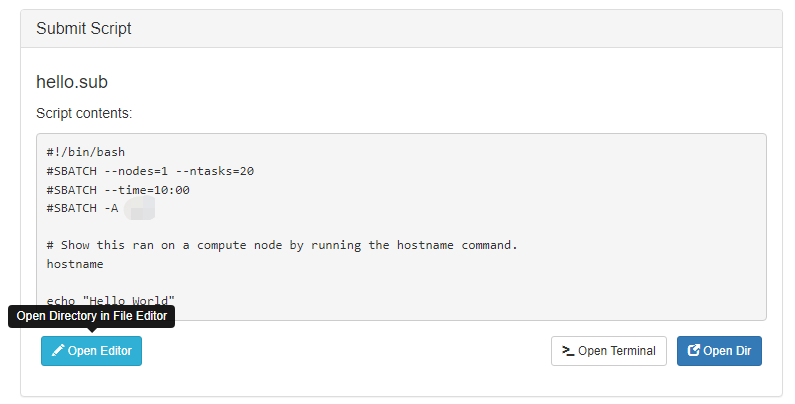
When you are finished with editing the job and are ready to submit, click the green 'Submit' button at the top of the job list. You can monitor progress from here or from the Active Jobs app. Once completed, you should see the output files appear:
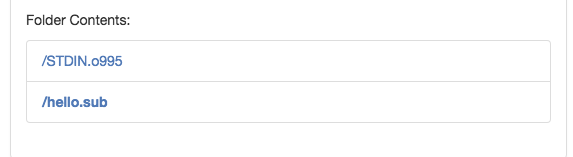
Clicking on one of the output files will open it in the file editor for your viewing.
Link to section 'Creating New Template' of 'Jobs' Creating New Template
First, prepare a template directory containing a template submission script along with any input files. Then, to import the job into the Job Composer app, click the 'Create New Template' button. Fill in the directory containing your template job script and files in the first box. Give it an appropriate name and notes.
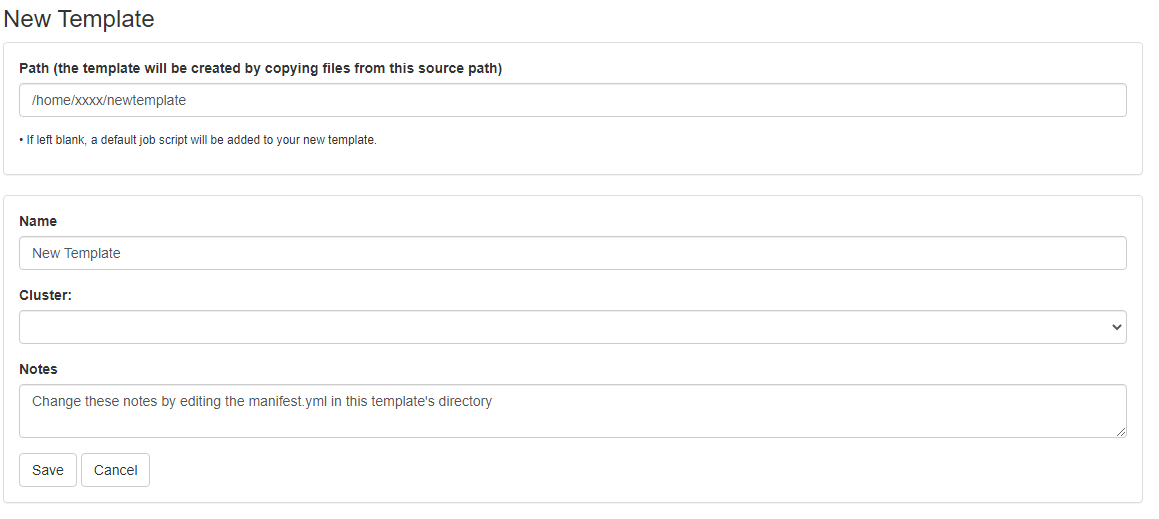
This template will now appear in your list of templates to choose from when composing jobs. You can now go create and submit a job from this new template.
Cluster Tools
The Cluster Tools menu contains cluster utilities. At the moment, only a terminal app is provided. Additional apps may be developed and provided in the future.
Link to section 'Shell Access' of 'Cluster Tools' Shell Access
Launching the shell app will provide you with a web-based terminal session on the cluster front-end. This is equivalent to using a standalone SSH client to connect to bell.rcac.purdue.edu
where you are connected to one several front-ends. The normal acceptable front-end use policy applies to access through the web-app. X11 Forwarding is not supported. Use of one of the interactive apps is recommended for graphical applications.
Software
Link to section 'Environment module' of 'Software' Environment module
Link to section 'Software catalog' of 'Software' Software catalog
Compiling Source Code
Documentation on compiling source code on Bell.
Compiling Serial Programs
A serial program is a single process which executes as a sequential stream of instructions on one processor core. Compilers capable of serial programming are available for C, C++, and versions of Fortran.
Here are a few sample serial programs:
- serial_hello.f
- serial_hello.f90
- serial_hello.f95
- serial_hello.c
-
To load a compiler, enter one of the following:
$ module load intel
$ module load gcc
Language | Intel Compiler | GNU Compiler | |
---|---|---|---|
Fortran 77 |
|
|
|
Fortran 90 |
|
|
|
Fortran 95 |
|
|
|
C |
|
|
|
C++ |
|
|
The Intel and GNU compilers will not output anything for a successful compilation. Also, the Intel compiler does not recognize the suffix ".f95".
Compiling MPI Programs
OpenMPI and Intel MPI (IMPI) are implementations of the Message-Passing Interface (MPI) standard. Libraries for these MPI implementations and compilers for C, C++, and Fortran are available on all clusters.
Language | Header Files |
---|---|
Fortran 77 |
|
Fortran 90 |
|
Fortran 95 |
|
C |
|
C++ |
|
Here are a few sample programs using MPI:
To see the available MPI libraries:
$ module avail openmpi
$ module avail impi
Language | Intel MPI | OpenMPI |
---|---|---|
Fortran 77 |
|
|
Fortran 90 |
|
|
Fortran 95 |
|
|
C |
|
|
C++ |
|
|
The Intel and GNU compilers will not output anything for a successful compilation. Also, the Intel compiler does not recognize the suffix ".f95".
Here is some more documentation from other sources on the MPI libraries:
Compiling OpenMP Programs
All compilers installed on Brown include OpenMP functionality for C, C++, and Fortran. An OpenMP program is a single process that takes advantage of a multi-core processor and its shared memory to achieve a form of parallel computing called multithreading. It distributes the work of a process over processor cores in a single compute node without the need for MPI communications.
Language | Header Files |
---|---|
Fortran 77 |
|
Fortran 90 |
|
Fortran 95 |
|
C |
|
C++ |
|
Sample programs illustrate task parallelism of OpenMP:
A sample program illustrates loop-level (data) parallelism of OpenMP:
To load a compiler, enter one of the following:
$ module load intel
$ module load gcc
Language | Intel Compiler | GNU Compiler |
---|---|---|
Fortran 77 |
|
|
Fortran 90 |
|
|
Fortran 95 |
|
|
C |
|
|
C++ |
|
|
The Intel and GNU compilers will not output anything for a successful compilation. Also, the Intel compiler does not recognize the suffix ".f95".
Here is some more documentation from other sources on OpenMP:
Compiling Hybrid Programs
A hybrid program combines both MPI and shared-memory to take advantage of compute clusters with multi-core compute nodes. Libraries for OpenMPI and Intel MPI (IMPI) and compilers which include OpenMP for C, C++, and Fortran are available.
Language | Header Files |
---|---|
Fortran 77 |
|
Fortran 90 |
|
Fortran 95 |
|
C |
|
C++ |
|
A few examples illustrate hybrid programs with task parallelism of OpenMP:
This example illustrates a hybrid program with loop-level (data) parallelism of OpenMP:
To see the available MPI libraries:
$ module avail impi
$ module avail openmpi
The following tables illustrate how to compile your hybrid (MPI/OpenMP) program. Any compiler flags accepted by Intel ifort/icc compilers are compatible with their respective MPI compiler.
Language | Command |
---|---|
Fortran 77 |
|
Fortran 90 |
|
Fortran 95 |
|
C |
|
C++ |
|
Language | Command |
---|---|
Fortran 77 |
|
Fortran 90 |
|
Fortran 95 |
|
C |
|
C++ |
|
The Intel and GNU compilers will not output anything for a successful compilation. Also, the Intel compiler does not recognize the suffix .f95
.
Intel MKL Library
Intel Math Kernel Library (MKL) contains ScaLAPACK, LAPACK, Sparse Solver, BLAS, Sparse BLAS, CBLAS, GMP, FFTs, DFTs, VSL, VML, and Interval Arithmetic routines. MKL resides in the directory stored in the environment variable MKL_HOME, after loading a version of the Intel compiler with module.
By using module load to load an Intel compiler your environment will have several variables set up to help link applications with MKL. Here are some example combinations of simplified linking options:
$ module load intel
$ echo $LINK_LAPACK
-L${MKL_HOME}/lib/intel64 -lmkl_intel_lp64 -lmkl_intel_thread -lmkl_core -liomp5 -lpthread
$ echo $LINK_LAPACK95
-L${MKL_HOME}/lib/intel64 -lmkl_lapack95_lp64 -lmkl_blas95_lp64 -lmkl_intel_lp64 -lmkl_intel_thread -lmkl_core -liomp5 -lpthread
RCAC recommends you use the provided variables to define MKL linking options in your compiling procedures. The Intel compiler modules also provide two other environment variables, LINK_LAPACK_STATIC and LINK_LAPACK95_STATIC that you may use if you need to link MKL statically.
RCAC recommends that you use dynamic linking of libguide. If so, define LD_LIBRARY_PATH such that you are using the correct version of libguide at run time. If you use static linking of libguide, then:
- If you use the Intel compilers, link in the libguide version that comes with the compiler (use the -openmp option).
- If you do not use the Intel compilers, link in the libguide version that comes with the Intel MKL above.
Here are some more documentation from other sources on the Intel MKL:
Running Jobs
There is one method for submitting jobs to Bell. You may use SLURM to submit jobs to a partition on Bell. SLURM performs job scheduling. Jobs may be any type of program. You may use either the batch or interactive mode to run your jobs. Use the batch mode for finished programs; use the interactive mode only for debugging.
In this section, you'll find a few pages describing the basics of creating and submitting SLURM jobs. As well, a number of example SLURM jobs that you may be able to adapt to your own needs.
Basics of SLURM Jobs
The Simple Linux Utility for Resource Management (SLURM) is a system providing job scheduling and job management on compute clusters. With SLURM, a user requests resources and submits a job to a queue. The system will then take jobs from queues, allocate the necessary nodes, and execute them.
Do NOT run large, long, multi-threaded, parallel, or CPU-intensive jobs on a front-end login host. All users share the front-end hosts, and running anything but the smallest test job will negatively impact everyone's ability to use Bell. Always use SLURM to submit your work as a job.
Link to section 'Submitting a Job' of 'Basics of SLURM Jobs' Submitting a Job
The main steps to submitting a job are:
Follow the links below for information on these steps, and other basic information about jobs. A number of example SLURM jobs are also available.
Queues
If you are here from our news posting and looking for informtation on our new queueing structure--head to our New Queues page. This page will be replaced with the content found there after the maintenance on July 22nd 2025.
Link to section '"mylab" Queues' of 'Queues' "mylab" Queues
Bell, as a community cluster, has one or more queues dedicated to and named after each partner who has purchased access to the cluster. These queues provide partners and their researchers with priority access to their portion of the cluster. Jobs in these queues are typically limited to 336 hours. The expectation is that any jobs submitted to your research lab queues will start within 4 hours, assuming the queue currently has enough capacity for the job (that is, your lab mates aren't using all of the cores currently).
Link to section 'Standby Queue' of 'Queues' Standby Queue
Additionally, community clusters provide a "standby" queue which is available to all cluster users. This "standby" queue allows users to utilize portions of the cluster that would otherwise be idle, but at a lower priority than partner-queue jobs, and with a relatively short time limit, to ensure "standby" jobs will not be able to tie up resources and prevent partner-queue jobs from running quickly. Jobs in standby are limited to 4 hours. There is no expectation of job start time. If the cluster is very busy with partner queue jobs, or you are requesting a very large job, jobs in standby may take hours or days to start.
Link to section 'Debug Queue' of 'Queues' Debug Queue
The debug queue allows you to quickly start small, short, interactive jobs in order to debug code, test programs, or test configurations. You are limited to one running job at a time in the queue, and you may run up to two GPUs for 30 minutes. The expectation is that debug jobs should start within a couple of minutes, assuming all of its dedicated nodes are not taken by others.
To see a list of all queues on Bell that you may submit to, use the slist command
This lists each queue you can submit to, the number of nodes allocated to the queue, how many are available to run jobs, and the maximum walltime you may request. Options to the command will give more detailed information. This command can be used to get a general idea of how busy an individual queue is and how long you may have to wait for your job to start.
Link to section ' ' of 'Queues'
Job Submission Script
To submit work to a SLURM queue, you must first create a job submission file. This job submission file is essentially a simple shell script. It will set any required environment variables, load any necessary modules, create or modify files and directories, and run any applications that you need:
#!/bin/bash
# FILENAME: myjobsubmissionfile
# Loads Matlab and sets the application up
module load matlab
# Change to the directory from which you originally submitted this job.
cd $SLURM_SUBMIT_DIR
# Runs a Matlab script named 'myscript'
matlab -nodisplay -singleCompThread -r myscript
Once your script is prepared, you are ready to submit your job.
Link to section 'Job Script Environment Variables' of 'Job Submission Script' Job Script Environment Variables
Name | Description |
---|---|
SLURM_SUBMIT_DIR | Absolute path of the current working directory when you submitted this job |
SLURM_JOBID | Job ID number assigned to this job by the batch system |
SLURM_JOB_NAME | Job name supplied by the user |
SLURM_JOB_NODELIST | Names of nodes assigned to this job |
SLURM_CLUSTER_NAME | Name of the cluster executing the job |
SLURM_SUBMIT_HOST | Hostname of the system where you submitted this job |
SLURM_JOB_PARTITION | Name of the original queue to which you submitted this job |
Submitting a Job
Once you have a job submission file, you may submit this script to SLURM using the sbatch
command. SLURM will find, or wait for, available resources matching your request and run your job there.
To submit your job to one compute node:
$ sbatch --nodes=1 myjobsubmissionfile
Slurm uses the word 'Account' and the option '-A' to specify different batch queues. To submit your job to a specific queue:
$ sbatch --nodes=1 -A standby myjobsubmissionfile
By default, each job receives 30 minutes of wall time, or clock time. If you know that your job will not need more than a certain amount of time to run, request less than the maximum wall time, as this may allow your job to run sooner. To request the 1 hour and 30 minutes of wall time:
$ sbatch -t 1:30:00 --nodes=1 -A standby myjobsubmissionfile
The --nodes
value indicates how many compute nodes you would like for your job.
Each compute node in Bell has 128 processor cores.
In some cases, you may want to request multiple nodes. To utilize multiple nodes, you will need to have a program or code that is specifically programmed to use multiple nodes such as with MPI. Simply requesting more nodes will not make your work go faster. Your code must support this ability.
To request 2 compute nodes:
$ sbatch --nodes=2 myjobsubmissionfile
By default, jobs on Bell will share nodes with other jobs.
To submit a job using 1 compute node with 4 tasks, each using the default 1 core and 1 GPU per node:
$ sbatch --nodes=1 --ntasks=4 --gpus-per-node=1 myjobsubmissionfile
If more convenient, you may also specify any command line options to sbatch
from within your job submission file, using a special form of comment:
#!/bin/sh -l
# FILENAME: myjobsubmissionfile
#SBATCH -A myqueuename
#SBATCH --nodes=1
#SBATCH --time=1:30:00
#SBATCH --job-name myjobname
# Print the hostname of the compute node on which this job is running.
/bin/hostname
If an option is present in both your job submission file and on the command line, the option on the command line will take precedence.
After you submit your job with SBATCH
, it may wait in queue for minutes, hours, or even weeks. How long it takes for a job to start depends on the specific queue, the resources and time requested, and other jobs already waiting in that queue requested as well. It is impossible to say for sure when any given job will start. For best results, request no more resources than your job requires.
Once your job is submitted, you can monitor the job status, wait for the job to complete, and check the job output.
Job Dependencies
Dependencies are an automated way of holding and releasing jobs. Jobs with a dependency are held until the condition is satisfied. Once the condition is satisfied jobs only then become eligible to run and must still queue as normal.
Job dependencies may be configured to ensure jobs start in a specified order. Jobs can be configured to run after other job state changes, such as when the job starts or the job ends.
These examples illustrate setting dependencies in several ways. Typically dependencies are set by capturing and using the job ID from the last job submitted.
To run a job after job myjobid has started:
sbatch --dependency=after:myjobid myjobsubmissionfile
To run a job after job myjobid ends without error:
sbatch --dependency=afterok:myjobid myjobsubmissionfile
To run a job after job myjobid ends with errors:
sbatch --dependency=afternotok:myjobid myjobsubmissionfile
To run a job after job myjobid ends with or without errors:
sbatch --dependency=afterany:myjobid myjobsubmissionfile
To set more complex dependencies on multiple jobs and conditions:
sbatch --dependency=after:myjobid1:myjobid2:myjobid3,afterok:myjobid4 myjobsubmissionfile
Holding a Job
Sometimes you may want to submit a job but not have it run just yet. You may be wanting to allow lab mates to cut in front of you in the queue - so hold the job until their jobs have started, and then release yours.
To place a hold on a job before it starts running, use the scontrol hold job command:
$ scontrol hold job myjobid
Once a job has started running it can not be placed on hold.
To release a hold on a job, use the scontrol release job command:
$ scontrol release job myjobid
You find the job ID using the squeue command as explained in the SLURM Job Status section.
Checking Job Status
Once a job is submitted there are several commands you can use to monitor the progress of the job.
To see your jobs, use the squeue -u command and specify your username:
(Remember, in our SLURM environment a queue is referred to as an 'Account')
squeue -u myusername
JOBID ACCOUNT NAME USER ST TIME NODES NODELIST(REASON)
182792 standby job1 myusername R 20:19 1 bell-a000
185841 standby job2 myusername R 20:19 1 bell-a001
185844 standby job3 myusername R 20:18 1 bell-a002
185847 standby job4 myusername R 20:18 1 bell-a003
To retrieve useful information about your queued or running job, use the scontrol show job command with your job's ID number. The output should look similar to the following:
scontrol show job 3519
JobId=3519 JobName=t.sub
UserId=myusername GroupId=mygroup MCS_label=N/A
Priority=3 Nice=0 Account=(null) QOS=(null)
JobState=PENDING Reason=BeginTime Dependency=(null)
Requeue=1 Restarts=0 BatchFlag=1 Reboot=0 ExitCode=0:0
RunTime=00:00:00 TimeLimit=7-00:00:00 TimeMin=N/A
SubmitTime=2019-08-29T16:56:52 EligibleTime=2019-08-29T23:30:00
AccrueTime=Unknown
StartTime=2019-08-29T23:30:00 EndTime=2019-09-05T23:30:00 Deadline=N/A
PreemptTime=None SuspendTime=None SecsPreSuspend=0
LastSchedEval=2019-08-29T16:56:52
Partition=workq AllocNode:Sid=mack-fe00:54476
ReqNodeList=(null) ExcNodeList=(null)
NodeList=(null)
NumNodes=1 NumCPUs=2 NumTasks=2 CPUs/Task=1 ReqB:S:C:T=0:0:*:*
TRES=cpu=2,node=1,billing=2
Socks/Node=* NtasksPerN:B:S:C=0:0:*:* CoreSpec=*
MinCPUsNode=1 MinMemoryNode=0 MinTmpDiskNode=0
Features=(null) DelayBoot=00:00:00
OverSubscribe=OK Contiguous=0 Licenses=(null) Network=(null)
Command=/home/myusername/jobdir/myjobfile.sub
WorkDir=/home/myusername/jobdir
StdErr=/home/myusername/jobdir/slurm-3519.out
StdIn=/dev/null
StdOut=/home/myusername/jobdir/slurm-3519.out
Power=
There are several useful bits of information in this output.
JobState
lets you know if the job is Pending, Running, Completed, or Held.RunTime and TimeLimit
will show how long the job has run and its maximum time.SubmitTime
is when the job was submitted to the cluster.NumNodes
,NumCPUs
,NumTasks
andCPUs/Task
are the number of Nodes, CPUs, Tasks, and CPUs per Task are shown.WorkDir
is the job's working directory.StdOut
andStderr
are the locations of stdout and stderr of the job, respectively.Reason
will show why aPENDING
job isn't running. The above error says that it has been requested to start at a specific, later time.
Checking Job Output
Once a job is submitted, and has started, it will write its standard output and standard error to files that you can read.
SLURM catches output written to standard output and standard error - what would be printed to your screen if you ran your program interactively. Unless you specfied otherwise, SLURM will put the output in the directory where you submitted the job in a file named slurm-
followed by the job id
, with the extension out
. For example slurm-3509.out
. Note that both stdout and stderr will be written into the same file, unless you specify otherwise.
If your program writes its own output files, those files will be created as defined by the program. This may be in the directory where the program was run, or may be defined in a configuration or input file. You will need to check the documentation for your program for more details.
Link to section 'Redirecting Job Output' of 'Checking Job Output' Redirecting Job Output
It is possible to redirect job output to somewhere other than the default location with the --error
and --output
directives:
#!/bin/bash
#SBATCH --output=/home/myusername/joboutput/myjob.out
#SBATCH --error=/home/myusername/joboutput/myjob.out
# This job prints "Hello World" to output and exits
echo "Hello World"
Canceling a Job
To stop a job before it finishes or remove it from a queue, use the scancel command:
scancel myjobid
You find the job ID using the squeue command as explained in the SLURM Job Status section.
New Queues
On Bell, the required options for job submission deviates from some of the other community clusters you might have experience using. In general every job submission will have four parts: “sbatch --ntasks=1 --cores-per-task=4 --partition=cpu --account=rcac --qos=standby
”
-
The number and type of resources you want (
--ntasks=1 --cores-per-task=4
) -
The partition where the resources are located (
--partition=cpu
) -
The account the resources should come out of (
--account=rcac
) -
The quality of service (QOS) this job expects from the resources (
--qos=standby
)
Use Case | Old Syntax | New Syntax |
---|---|---|
Submit a job to your group's account | sbatch -A mygroup |
sbatch -A mygroup -p cpu |
Submit a standby job | sbatch -A standby |
sbatch -A mygroup -p cpu -q standby |
Submit a highmem job | sbatch -A highmem |
sbatch -A mygroup -p highmem |
Submit a gpu job | sbatch -A gpu |
sbatch -A mygroup -p gpu |
Submit a multigpu job | sbatch -A multigpu |
sbatch -A mygroup -p multigpu |
If you have used other clusters, you will be familiar with the first item. If you have not, you can read about how to format the request on our job submission page. The rest of this page will focus on the last three items.
Link to section 'Partitions' of 'New Queues' Partitions
On Bell, the various types of nodes on the cluster are organized into distinct partitions. This allows jobs to different node types to be charged separately and differently. This also means that Instead of only needing to specify the account name in the job script, the desired partition must also be specified. Each of these partitions is subject to different limitations and has a specific use case that will be described below.
Link to section 'CPU Partition' of 'New Queues' CPU Partition
This partition contains the resources a group purchases access to when they purchase CPU resources on Bell and is made up of 488 Bell-A nodes. Each of these nodes contains two Zen 2 AMD EPYC 7662 64-core processors for a total of 128 cores and 256 GB of memory for a total of more than 62,000 cores in the partition. Memory in this partition is allocated proportional to your core request such that each core is given about 2 GB of memory per core requested. Submission to this partition can be accomplished by using the option: -p cpu
or --partition=cpu
.
The purchasing model for this partition allows groups to purchase high priority access to some number of cores. When an account uses resources in this account by submitting a job tagged with the normal
QOS, the cores used by that job are withdrawn from the account and deposited back into the account when the job terminates.
When using the CPU partition, jobs are tagged by the normal
QOS by default, but they can be tagged with the standby
QOS if explicitly submitted using the -q standby
or --qos=standby
option.
- Jobs tagged with the
normal
QOS are subject to the following policies:- Jobs have a high priority and should not need to wait very long before starting.
- Any cores requested by these jobs are withdrawn from the account until the job terminates.
- These jobs can run for up to two weeks at a time.
- Jobs tagged with the
standby
QOS are subject to the following policies:- Jobs have a low priority and there is no expectation of job start time. If the partition is very busy with jobs using the
normal
QOS or if you are requesting a very large job, then jobs using thestandby
QOS may take hours or days to start. - These jobs can use idle resources on the cluster and as such cores requested by these jobs are not withdrawn from the account to which they were submitted.
- These jobs can run for up to four hours at a time.
- Jobs have a low priority and there is no expectation of job start time. If the partition is very busy with jobs using the
Available QOSes: normal
, standby
Link to section 'Highmem Partition' of 'New Queues' Highmem Partition
This partition is made up of 8 Bell-B nodes which have four times as much memory as a standard Bell-A node, and access to this partition is given to all accounts on the cluster to enable work that has higher memory requirements. Each of these nodes contains two Zen 2 AMD EPYC 7662 64-core processors for a total of 128 cores and 1 TB of memory. Memory in this partition is allocated proportional to your core request such that each core is given about 8 GB of memory per core requested. Submission to this partition can be accomplished by using the option: -p highmem
or --partition=highmem
.
When using the Highmem partition, jobs are tagged by the normal
QOS by default, and this is the only QOS that is available for this partition, so there is no need to specify a QOS when using this partition. Additionally jobs are tagged by a highmem partition QOS that enforces the following policies
- There is no expectation of job start time as these nodes are a shared resources that are given as a bonus for purchasing access to high priority access to resources on Bell
- You can have 2 jobs running in this partition at once
- You can have 8 jobs submitted to thie partition at once
- Your jobs must use more than 64 of the 128 cores on the node otherwise your memory footprint would fit on a standard Bell-A node
- These jobs can run for up to 24 hours at a time.
Available QOSes: normal
Link to section 'GPU Partition' of 'New Queues' GPU Partition
This partition is made up of 4 Bell-G nodes. Each of these nodes contains two AMD MI50s and two Zen 2 AMD EPYC 7662 64-core processors for a total of 128 cores and 256GB of memory. Memory in this partition is allocated proportional to your core request such that each core is given about 3 GB of memory per core requested. You should request cores proportional to the number of GPUs you are using in this partition (i.e. if you only need one of the two GPUs, you should request half of the cores on the node) Submission to this partition can be accomplished by using the option: -p gpu
or --partition=gpu
.
When using the gpu partition, jobs are tagged by the normal
QOS by default, and this is the only QOS that is available for this partition, so there is no need to specify a QOS when using this partition. Additionally jobs are tagged by a gpu partition QOS that enforces the following policies
- There is no expectation of job start time as these nodes are a shared resources that are given as a bonus for purchasing access to high priority access to resources on Bell
- You can use up to 2 GPUs in this partition at once
- You can have 8 jobs submitted to thie partition at once
- These jobs can run for up to 24 hours at a time.
Available QOSes: normal
Link to section 'Multi-GPU Partition' of 'New Queues' Multi-GPU Partition
This partition is made up of a single Bell-X node. Each of these nodes contains six AMD MI60s and two Intel Xeon 8268 48-core processors for a total of 96 cores and 354GB of memory. Memory in this partition is allocated proportional to your core request such that each core is given about 3.5 GB of memory per core requested. You should request cores proportional to the number of GPUs you are using in this partition (i.e. if you only need one of the six GPUs, you should request 16 of the cores on the node) Submission to this partition can be accomplished by using the option: -p multigpu
or --partition=multigpu
.
When using the gpu partition, jobs are tagged by the normal
QOS by default, and this is the only QOS that is available for this partition, so there is no need to specify a QOS when using this partition. Additionally jobs are tagged by a multigpu partition QOS that enforces the following policies
- There is no expectation of job start time as these nodes are a shared resources that are given as a bonus for purchasing access to high priority access to resources on Bell
- You can use up to 6 GPUs in this partition at once
- You can have 1 jobs submitted to thie partition at once
- These jobs can run for up to 24 hours at a time.
Available QOSes: normal
Link to section 'Accounts' of 'New Queues' Accounts
On the Bell community cluster, users will have access to one or more accounts, also known as queues. These accounts are dedicated to and named after each partner who has purchased access to the cluster, and they provide partners and their researchers with priority access to their portion of the cluster. These accounts can be thought of as bank accounts that contain the resources a group has purchased access to which may include some number of cores. To see the list of accounts that you have access to on Bell as well as the resources they contain, you can use the command slist
.
On Bell, you must explicitly define the account that you want to submit to using the -A
or--account=
option.
Link to section 'Quality of Service (QOS)' of 'New Queues' Quality of Service (QOS)
On Bell, we use a Slurm concept called a Quality of Service or a QOS. A QOS can be thought of as a tag for a job that tells the scheduler how that job should be treated with respect to limits, priority, etc. The cluster administrators define the available QOSes as well as the policies for how each QOS should be treated on the cluster. A toy example of such a policy may be "no single user can have more than 200 jobs that has been tagged with a QOS named highpriority".
There are two classes of QOSes and a job can have both:
- Partition QOSes: A partition QOS is a tag that is automatically added to your job when you submit to a partition that defines a partition QOS.
- Job QOSes: A Job QOS is a tag that you explicitly give to a job using the option
-q
or--qos=
. By explicitly tagging your jobs this way, you can choose the policy that each one of your jobs should abide by. We will describe the policies for the available job QOSes in the partition section below.
As an extended metaphor, if we think of a job as a package that we need to have shipped to some destination, then the partition can be thought of as the carrier we decide to ship our package with. That carrier is going to have some company policies that dictate how you need to label/pack that package, and that company policy is like the partition QOS. It is the policy that is enforced for simply deciding to use that carrier, or in this case, deciding to submit to a particular partition.
The Job QOS can then be thought of as the various different types of shipping options that carrier might offer. You might pay extra to have that package shipped overnight. On the other hand you may choose to pay less and have your package arrive as available. Once we decide to go with a particular carrier, we are subject to their company policy, but we also have some degree of control through choosing one of their available shipping options. In the same way, when you choose to submit to a partition, you are subject to the limits enforced by the partition QOS, but you may be able to ask for your job to be handled a particular way by specifying a job QOS offered by the partition.
In order for a job to use a Job QOS, the user submitting the job must have access to the QOS, the account the job is being submitted to must accept the QOS, and the partition the job is being submitted to must accept the QOS. The below list of job QOSes are QOSes that every user and every account of Bell has access to:
normal
: Thenormal
QOS is the default job QOS on the cluster meaning if you do not explicitly list an alternative job QOS, your job will be tagged with this QOS. The policy for this QOS provides a high priority and does not add any additional limits.standby
: Thestandby
QOS must be explicitly used if desired by using the option-q standby
or--qos=standby
. The policy for this QOS gives access to idle resources on the cluster. Jobs tagged with this QOS are "low priority" jobs and are only allowed to run for up to four hours at a time, however the resources used by these jobs do not count against the resources in your Account. For users of our previous clusters, usage of this QOS replaces the previous-A standby
style of submission.
Some of these QOSes may not be available in every partition. Each of the partitions in the following section will enumerate which of these QOSes are allowed in the partition.
PBS to Slurm
This is a reference for the most common command, environment variables, and job specification options used by the workload management systems and their equivalents.
Notable Differences
-
Separate commands for Batch and Interactive jobs
Unlike PBS, in Slurm interactive jobs and batch jobs are launched with completely distinct commands.
Usesbatch [allocation request options] script
to submit a job to the batch scheduler, andsinteractive [allocation request options]
to launch an interactive job. sinteractive accepts most of the same allocation request options as sbatch does. -
No need for
cd $PBS_O_WORKDIR
In Slurm your batch job starts to run in the directory from which you submitted the script whereas in PBS/Torque you need to explicitly move back to that directory with
cd $PBS_O_WORKDIR
. -
No need to manually export environment
The environment variables that are defined in your shell session at the time that you submit the script are exported into your batch job, whereas in PBS/Torque you need to use the
-V
flag to export your environment. -
Location of output files
The output and error files are created in their final location immediately that the job begins or an error is generated, whereas in PBS/Torque temporary files are created that are only moved to the final location at the end of the job. Therefore in Slurm you can examine the output and error files from your job during its execution.
See the official Slurm Documentation for further details.
Quick Guide
This table lists the most common command, environment variables, and job specification options used by the workload management systems and their equivalents (adapted from http://www.schedmd.com/slurmdocs/rosetta.html).
User Commands | PBS/Torque | Slurm |
---|---|---|
Job submission | qsub [script_file] |
sbatch [script_file] |
Interactive Job | qsub -I |
sinteractive |
Job deletion | qdel [job_id] |
scancel [job_id] |
Job status (by job) | qstat [job_id] |
squeue [-j job_id] |
Job status (by user) | qstat -u [user_name] |
squeue [-u user_name] |
Job hold | qhold [job_id] |
scontrol hold [job_id] |
Job release | qrls [job_id] |
scontrol release [job_id] |
Queue info | qstat -Q |
squeue |
Queue access | qlist |
slist |
Node list | pbsnodes -l |
sinfo -N scontrol show nodes |
Cluster status | qstat -a |
sinfo |
GUI | xpbsmon |
sview |
Environment | PBS/Torque | Slurm |
Job ID | $PBS_JOBID |
$SLURM_JOB_ID |
Job Name | $PBS_JOBNAME |
$SLURM_JOB_NAME |
Job Queue/Account | $PBS_QUEUE |
$SLURM_JOB_ACCOUNT |
Submit Directory | $PBS_O_WORKDIR |
$SLURM_SUBMIT_DIR |
Submit Host | $PBS_O_HOST |
$SLURM_SUBMIT_HOST |
Number of nodes | $PBS_NUM_NODES |
$SLURM_JOB_NUM_NODES |
Number of Tasks | $PBS_NP |
$SLURM_NTASKS |
Number of Tasks Per Node | $PBS_NUM_PPN |
$SLURM_NTASKS_PER_NODE |
Node List (Compact) | n/a | $SLURM_JOB_NODELIST |
Node List (One Core Per Line) | LIST=$(cat $PBS_NODEFILE) |
LIST=$(srun hostname) |
Job Array Index | $PBS_ARRAYID |
$SLURM_ARRAY_TASK_ID |
Job Specification | PBS/Torque | Slurm |
Script directive | #PBS |
#SBATCH |
Queue | -q [queue] |
-A [queue] |
Node Count | -l nodes=[count] |
-N [min[-max]] |
CPU Count | -l ppn=[count] |
-n [count] Note: total, not per node |
Wall Clock Limit | -l walltime=[hh:mm:ss] |
-t [min] OR-t [hh:mm:ss] OR-t [days-hh:mm:ss] |
Standard Output FIle | -o [file_name] |
-o [file_name] |
Standard Error File | -e [file_name] |
-e [file_name] |
Combine stdout/err | -j oe (both to stdout) OR-j eo (both to stderr) |
(use -o without -e) |
Copy Environment | -V |
--export=[ALL | NONE | variables] Note: default behavior is ALL |
Copy Specific Environment Variable | -v myvar=somevalue |
--export=NONE,myvar=somevalue OR--export=ALL,myvar=somevalue |
Event Notification | -m abe |
--mail-type=[events] |
Email Address | -M [address] |
--mail-user=[address] |
Job Name | -N [name] |
--job-name=[name] |
Job Restart | -r [y|n] |
--requeue OR--no-requeue |
Working Directory | --workdir=[dir_name] |
|
Resource Sharing | -l naccesspolicy=singlejob |
--exclusive OR--shared |
Memory Size | -l mem=[MB] |
--mem=[mem][M|G|T] OR--mem-per-cpu=[mem][M|G|T] |
Account to charge | -A [account] |
-A [account] |
Tasks Per Node | -l ppn=[count] |
--tasks-per-node=[count] |
CPUs Per Task | --cpus-per-task=[count] |
|
Job Dependency | -W depend=[state:job_id] |
--depend=[state:job_id] |
Job Arrays | -t [array_spec] |
--array=[array_spec] |
Generic Resources | -l other=[resource_spec] |
--gres=[resource_spec] |
Licenses | --licenses=[license_spec] |
|
Begin Time | -A "y-m-d h:m:s" |
--begin=y-m-d[Th:m[:s]] |
See the official Slurm Documentation for further details.
Example Jobs
A number of example jobs are available for you to look over and adapt to your own needs. The first few are generic examples, and latter ones go into specifics for particular software packages.
Generic SLURM Jobs
The following examples demonstrate the basics of SLURM jobs, and are designed to cover common job request scenarios. These example jobs will need to be modified to run your application or code.
Simple Job
Every SLURM job consists of a job submission file. A job submission file contains a list of commands that run your program and a set of resource (nodes, walltime, queue) requests. The resource requests can appear in the job submission file or can be specified at submit-time as shown below.
This simple example submits the job submission file hello.sub to the standby queue on Bell and requests a single node:
#!/bin/bash
# FILENAME: hello.sub
# Show this ran on a compute node by running the hostname command.
hostname
echo "Hello World"
sbatch -A standby --nodes=1 --ntasks=1 --cpus-per-task=1 --time=00:01:00 hello.sub
Submitted batch job 3521
For a real job you would replace echo "Hello World"
with a command, or sequence of commands, that run your program.
After your job finishes running, the ls command will show a new file in your directory, the .out file:
ls -l
hello.sub
slurm-3521.out
The file slurm-3521.out contains the output and errors your program would have written to the screen if you had typed its commands at a command prompt:
cat slurm-3521.out
bell-a001.rcac.purdue.edu
Hello World
You should see the hostname of the compute node your job was executed on. Following should be the "Hello World" statement.
Multiple Node
In some cases, you may want to request multiple nodes. To utilize multiple nodes, you will need to have a program or code that is specifically programmed to use multiple nodes such as with MPI. Simply requesting more nodes will not make your work go faster. Your code must support this ability.
This example shows a request for multiple compute nodes. The job submission file contains a single command to show the names of the compute nodes allocated:
# FILENAME: myjobsubmissionfile.sub
#!/bin/bash
echo "$SLURM_JOB_NODELIST"
sbatch --nodes=2 --ntasks=256 --time=00:10:00 -A standby myjobsubmissionfile.sub
Compute nodes allocated:
bell-a[014-015]
The above example will allocate the total of 256 CPU cores across 2 nodes. Note that if your multi-node job requests fewer than each node's full 128 cores per node, by default Slurm provides no guarantee with respect to how this total is distributed between assigned nodes (i.e. the cores may not necessarily be split evenly). If you need specific arrangements of your tasks and cores, you can use --cpus-per-task= and/or --ntasks-per-node= flags. See Slurm documentation or man sbatch for more options.
Directives
So far these examples have shown submitting jobs with the resource requests on the sbatch
command line such as:
sbatch -A standby --nodes=1 --time=00:01:00 hello.sub
The resource requests can also be put into job submission file itself. Documenting the resource requests in the job submission is desirable because the job can be easily reproduced later. Details left in your command history are quickly lost. Arguments are specified with the #SBATCH
syntax:
#!/bin/bash
# FILENAME: hello.sub
#SBATCH -A standby
#SBATCH --nodes=1 --time=00:01:00
# Show this ran on a compute node by running the hostname command.
hostname
echo "Hello World"
The #SBATCH
directives must appear at the top of your submission file. SLURM will stop parsing directives as soon as it encounters a line that does not start with '#'. If you insert a directive in the middle of your script, it will be ignored.
This job can be then submitted with:
sbatch hello.sub
Specific Types of Nodes
SLURM allows running a job on specific types of compute nodes to accommodate special hardware requirements (e.g. a certain CPU or GPU type, etc.)
Cluster nodes have a set of descriptive features assigned to them, and users can specify which of these features are required by their job by using the constraint option at submission time. Only nodes having features matching the job constraints will be used to satisfy the request.
Example: a job requires a compute node in an "A" sub-cluster:
sbatch --nodes=1 --ntasks=128 --constraint=A myjobsubmissionfile.sub
Compute node allocated:
bell-a003
Feature constraints can be used for both batch and interactive jobs, as well as for individual job steps inside a job. Multiple constraints can be specified with a predefined syntax to achieve complex request logic (see detailed description of the '--constraint' option in man sbatch
or online Slurm documentation).
Refer to Detailed Hardware Specification section for list of available sub-cluster labels, their respective per-node memory sizes and other hardware details. You could also use sfeatures
command to list available constraint feature names for different node types.
Interactive Jobs
Interactive jobs are run on compute nodes, while giving you a shell to interact with. They give you the ability to type commands or use a graphical interface in the same way as if you were on a front-end login host.
To submit an interactive job, use sinteractive to run a login shell on allocated resources.
sinteractive accepts most of the same resource requests as sbatch, so to request a login shell on the cpu account while allocating 2 nodes and 128 total cores, you might do:
sinteractive -A cpu -N2 -n256
To quit your interactive job:
exit
or Ctrl-D
The above example will allocate the total of 256 CPU cores across 2 nodes. Note that if your multi-node job requests fewer than each node's full 128 cores per node, by default Slurm provides no guarantee with respect to how this total is distributed between assigned nodes (i.e. the cores may not necessarily be split evenly). If you need specific arrangements of your tasks and cores, you can use --cpus-per-task= and/or --ntasks-per-node= flags. See Slurm documentation or man salloc for more options.
Serial Jobs
This shows how to submit one of the serial programs compiled in the section Compiling Serial Programs.
Create a job submission file:
#!/bin/bash
# FILENAME: serial_hello.sub
./serial_hello
Submit the job:
sbatch --nodes=1 --ntasks=1 --time=00:01:00 serial_hello.sub
After the job completes, view results in the output file:
cat slurm-myjobid.out
Runhost:bell-a009.rcac.purdue.edu
hello, world
If the job failed to run, then view error messages in the file slurm-myjobid.out.
OpenMP
A shared-memory job is a single process that takes advantage of a multi-core processor and its shared memory to achieve parallelization.
This example shows how to submit an OpenMP program compiled in the section Compiling OpenMP Programs.
When running OpenMP programs, all threads must be on the same compute node to take advantage of shared memory. The threads cannot communicate between nodes.
To run an OpenMP program, set the environment variable OMP_NUM_THREADS to the desired number of threads:
In csh:
setenv OMP_NUM_THREADS 128
In bash:
export OMP_NUM_THREADS=128
This should almost always be equal to the number of cores on a compute node. You may want to set to another appropriate value if you are running several processes in parallel in a single job or node.
Create a job submissionfile:
#!/bin/bash
# FILENAME: omp_hello.sub
#SBATCH --nodes=1
#SBATCH --ntasks=128
#SBATCH --time=00:01:00
export OMP_NUM_THREADS=128
./omp_hello
Submit the job:
sbatch omp_hello.sub
View the results from one of the sample OpenMP programs about task parallelism:
cat omp_hello.sub.omyjobid
SERIAL REGION: Runhost:bell-a003.rcac.purdue.edu Thread:0 of 1 thread hello, world
PARALLEL REGION: Runhost:bell-a003.rcac.purdue.edu Thread:0 of 128 threads hello, world
PARALLEL REGION: Runhost:bell-a003.rcac.purdue.edu Thread:1 of 128 threads hello, world
...
If the job failed to run, then view error messages in the file slurm-myjobid.out.
If an OpenMP program uses a lot of memory and 128 threads use all of the memory of the compute node, use fewer processor cores (OpenMP threads) on that compute node.
MPI
An MPI job is a set of processes that take advantage of multiple compute nodes by communicating with each other. OpenMPI and Intel MPI (IMPI) are implementations of the MPI standard.
This section shows how to submit one of the MPI programs compiled in the section Compiling MPI Programs.
Use module load to set up the paths to access these libraries. Use module avail to see all MPI packages installed on Bell.
Create a job submission file:
#!/bin/bash
# FILENAME: mpi_hello.sub
#SBATCH --nodes=2
#SBATCH --ntasks-per-node=128
#SBATCH --time=00:01:00
#SBATCH -A standby
srun -n 256 ./mpi_hello
SLURM can run an MPI program with the srun command. The number of processes is requested with the -n option. If you do not specify the -n option, it will default to the total number of processor cores you request from SLURM.
If the code is built with OpenMPI, it can be run with a simple srun -n command. If it is built with Intel IMPI, then you also need to add the --mpi=pmi2 option: srun --mpi=pmi2 -n 256 ./mpi_hello in this example.
Submit the MPI job:
sbatch ./mpi_hello.sub
View results in the output file:
cat slurm-myjobid.out
Runhost:bell-a010.rcac.purdue.edu Rank:0 of 256 ranks hello, world
Runhost:bell-a010.rcac.purdue.edu Rank:1 of 256 ranks hello, world
...
Runhost:bell-a011.rcac.purdue.edu Rank:128 of 256 ranks hello, world
Runhost:bell-a011.rcac.purdue.edu Rank:129 of 256 ranks hello, world
...
If the job failed to run, then view error messages in the output file.
If an MPI job uses a lot of memory and 128 MPI ranks per compute node use all of the memory of the compute nodes, request more compute nodes, while keeping the total number of MPI ranks unchanged.
Submit the job with double the number of compute nodes and modify the resource request to halve the number of MPI ranks per compute node.
#!/bin/bash
# FILENAME: mpi_hello.sub
#SBATCH --nodes=4
#SBATCH --ntasks-per-node=64
#SBATCH -t 00:01:00
#SBATCH -A standby
srun -n 256 ./mpi_hello
sbatch ./mpi_hello.sub
View results in the output file:
cat slurm-myjobid.out
Runhost:bell-a10.rcac.purdue.edu Rank:0 of 256 ranks hello, world
Runhost:bell-a010.rcac.purdue.edu Rank:1 of 256 ranks hello, world
...
Runhost:bell-a011.rcac.purdue.edu Rank:64 of 256 ranks hello, world
...
Runhost:bell-a012.rcac.purdue.edu Rank:128 of 256 ranks hello, world
...
Runhost:bell-a013.rcac.purdue.edu Rank:192 of 256 ranks hello, world
...
Notes
- Use slist to determine which queues (
--account
or-A
option) are available to you. The name of the queue which is available to everyone on Bell is "standby". - Invoking an MPI program on Bell with ./program is typically wrong, since this will use only one MPI process and defeat the purpose of using MPI. Unless that is what you want (rarely the case), you should use srun or mpiexec to invoke an MPI program.
- In general, the exact order in which MPI ranks output similar write requests to an output file is random.
GPU
The Bell cluster nodes contain NVIDIA GPUs that support CUDA and OpenCL. See the detailed hardware overview for the specifics on the GPUs in Bell.
This section illustrates how to use SLURM to submit a simple GPU program.
Suppose that you named your executable file gpu_hello from the sample code gpu_hello.cu
(see the section on compiling NVIDIA GPU codes). Prepare a job submission file with an appropriate name, here named gpu_hello.sub:
#!/bin/bash
# FILENAME: gpu_hello.sub
module load cuda
host=`hostname -s`
echo $CUDA_VISIBLE_DEVICES
# Run on the first available GPU
./gpu_hello 0
Submit the job:
sbatch -A gpu --nodes=1 --gres=gpu:1 -t 00:01:00 gpu_hello.sub
Requesting a GPU from the scheduler is required.
You can specify total number of GPUs, or number of GPUs per node, or even number of GPUs per task:
sbatch -A gpu --nodes=1 --gres=gpu:1 -t 00:01:00 gpu_hello.sub
sbatch -A gpu --nodes=1 --gpus-per-node=1 -t 00:01:00 gpu_hello.sub
sbatch -A gpu --nodes=1 --gpus-per-task=1 -t 00:01:00 gpu_hello.sub
After job completion, view the new output file in your directory:
ls -l
gpu_hello
gpu_hello.cu
gpu_hello.sub
slurm-myjobid.out
View results in the file for all standard output, slurm-myjobid.out
0
hello, world
If the job failed to run, then view error messages in the file slurm-myjobid.out.
To use multiple GPUs in your job, simply specify a larger value to the GPU specification parameter. However, be aware of the number of GPUs installed on the node(s) you may be requesting. The scheduler can not allocate more GPUs than physically exist. See detailed hardware overview and output of sfeatures command for the specifics on the GPUs in Bell.
Link to section 'Collecting System Resource Utilization Data' of 'Monitoring Resources' Collecting System Resource Utilization Data
Knowing the precise resource utilization an application had during a job, such as CPU load or memory, can be incredibly useful. This is especially the case when the application isn't performing as expected.
One approach is to run a program like htop
during an interactive job and keep an eye on system resources. You can get precise time-series data from nodes associated with your job using XDmod as well, online. But these methods don't gather telemetry in an automated fashion, nor do they give you control over the resolution or format of the data.
As a matter of course, a robust implementation of some HPC workload would include resource utilization data as a diagnostic tool in the event of some failure.
The monitor
utility is a simple command line system resource monitoring tool for gathering such telemetry and is available as a module.
module load monitor
Complete documentation is available online at resource-monitor.readthedocs.io. A full manual page is also available for reference, man monitor
.
In the context of a SLURM job you will need to put this monitoring task in the background to allow the rest of your job script to proceed. Be sure to interrupt these tasks at the end of your job.
#!/bin/bash
# FILENAME: monitored_job.sh
module load monitor
# track per-code CPU load
monitor cpu percent --all-cores >cpu-percent.log &
CPU_PID=$!
# track memory usage
monitor cpu memory >cpu-memory.log &
MEM_PID=$!
# your code here
# shut down the resource monitors
kill -s INT $CPU_PID $MEM_PID
A particularly elegant solution would be to include such tools in your prologue script and have the tear down in your epilogue script.
For large distributed jobs spread across multiple nodes, mpiexec
can be used to gather telemetry from all nodes in the job. The hostname is included in each line of output so that data can be grouped as such. A concise way of constructing the needed list of hostnames in SLURM is to simply use srun hostname | sort -u
.
#!/bin/bash
# FILENAME: monitored_job.sh
module load monitor
# track all CPUs (one monitor per host)
mpiexec -machinefile <(srun hostname | sort -u) \
monitor cpu percent --all-cores >cpu-percent.log &
CPU_PID=$!
# track memory on all hosts (one monitor per host)
mpiexec -machinefile <(srun hostname | sort -u) \
monitor cpu memory >cpu-memory.log &
MEM_PID=$!
# your code here
# shut down the resource monitors
kill -s INT $CPU_PID $MEM_PID
To get resource data in a more readily computable format, the monitor
program can be told to output in CSV format with the --csv
flag.
monitor cpu memory --csv >cpu-memory.csv
For a distributed job you will need to suppress the header lines otherwise one will be created by each host.
monitor cpu memory --csv | head -1 >cpu-memory.csv
mpiexec -machinefile <(srun hostname | sort -u) \
monitor cpu memory --csv --no-header >>cpu-memory.csv
Specific Applications
The following examples demonstrate job submission files for some common real-world applications. See the Generic SLURM Examples section for more examples on job submissions that can be adapted for use.
Gaussian
Gaussian is a computational chemistry software package which works on electronic structure. This section illustrates how to submit a small Gaussian job to a Slurm queue. This Gaussian example runs the Fletcher-Powell multivariable optimization.
Prepare a Gaussian input file with an appropriate filename, here named myjob.com. The final blank line is necessary:
#P TEST OPT=FP STO-3G OPTCYC=2
STO-3G FLETCHER-POWELL OPTIMIZATION OF WATER
0 1
O
H 1 R
H 1 R 2 A
R 0.96
A 104.
To submit this job, load Gaussian then run the provided script, named subg16. This job uses one compute node with 128 processor cores:
module load gaussian16
subg16 myjob -N 1 -n 128
View job status:
squeue -u myusername
View results in the file for Gaussian output, here named myjob.log. Only the first and last few lines appear here:
Entering Gaussian System, Link 0=/apps/cent7/gaussian/g16-A.03/g16-haswell/g16/g16
Initial command:
/apps/cent7/gaussian/g16-A.03/g16-haswell/g16/l1.exe /scratch/bell/myusername/gaussian/Gau-7781.inp -scrdir=/scratch/bell/myusername/gaussian/
Entering Link 1 = /apps/cent7/gaussian/g16-A.03/g16-haswell/g16/l1.exe PID= 7782.
Copyright (c) 1988,1990,1992,1993,1995,1998,2003,2009,2016,
Gaussian, Inc. All Rights Reserved.
.
.
.
Job cpu time: 0 days 0 hours 3 minutes 28.2 seconds.
Elapsed time: 0 days 0 hours 0 minutes 12.9 seconds.
File lengths (MBytes): RWF= 17 Int= 0 D2E= 0 Chk= 2 Scr= 2
Normal termination of Gaussian 16 at Tue May 1 17:12:00 2018.
real 13.85
user 202.05
sys 6.12
Machine:
bell-a012.rcac.purdue.edu
bell-a012.rcac.purdue.edu
bell-a012.rcac.purdue.edu
bell-a012.rcac.purdue.edu
bell-a012.rcac.purdue.edu
bell-a012.rcac.purdue.edu
bell-a012.rcac.purdue.edu
bell-a012.rcac.purdue.edu
Link to section 'Examples of Gaussian SLURM Job Submissions' of 'Gaussian' Examples of Gaussian SLURM Job Submissions
Submit job using 128 processor cores on a single node:
subg16 myjob -N 1 -n 128 -t 200:00:00 -A myqueuename
Submit job using 128 processor cores on each of 2 nodes:
subg16 myjob -N 2 --ntasks-per-node=128 -t 200:00:00 -A myqueuename
To submit a bash job, a submit script sample looks like:
#!/bin/bash
#SBATCH -A myqueuename # Queue name(use 'slist' command to find queues' name)
#SBATCH --nodes=1 # Total # of nodes
#SBATCH --ntasks=64 # Total # of MPI tasks
#SBATCH --time=1:00:00 # Total run time limit (hh:mm:ss)
#SBATCH -J myjobname # Job name
#SBATCH -o myjob.o%j # Name of stdout output file
#SBATCH -e myjob.e%j # Name of stderr error file
module load gaussian16
g16 < myjob.com
For more information about Gaussian:
Machine Learning
We support several common machine learning (ML) frameworks on the community clusters through pre-installed modules. The collection of these pre-installed ML modules is referred to as ml-toolkit throughout this documentation. Currently, the following libraries are included in ML-Toolkit.
caffe cntk gym keras
mxnet opencv pytorch
tensorflow tflearn theano
Note that managing dependencies with ML applications can be non-trivial, therefore, we recommend users start by using ml-toolkit. If a custom installation is required after trying ml-toolkit, make sure to read documentation carefully.
ML-Toolkit
A set of pre-installed popular machine learning (ML) libraries, called ML-Toolkit is maintained on Bell. These are Anaconda/Python-based distributions of the respective libraries. Currently, applications are supported for Python 2 and 3. Detailed instructions for searching and using the installed ML applications are presented below.
Link to section 'Instructions for using ML-Toolkit Modules' of 'ML-Toolkit' Instructions for using ML-Toolkit Modules
Link to section 'Find and Use Installed ML Packages' of 'ML-Toolkit' Find and Use Installed ML Packages
To search or load a machine learning application, you must first load one of the learning modules. The learning module loads the prerequisites (such as anaconda and cudnn) and makes ML applications visible to the user.
Step 1. Find and load a preferred learning module. Several learning modules may be available, corresponding to a specific Python version and whether the ML applications have GPU support or not. Running module load learning without specifying a version will load the version with the most recent python version. To see all available modules, run module spider learning then load the desired module.
Step 2. Find and load the desired machine learning libraries
ML packages are installed under the common application name ml-toolkit-cpu
You can use the module spider ml-toolkit command to see all options and versions of each library.
Load the desired modules using the module load command. Note that both CPU and GPU options may exist for many libraries, so be sure to load the correct version. For example, if you wanted to load the most recent version of PyTorch for CPU, you would run module load ml-toolkit-cpu/pytorch
caffe cntk gym keras mxnet
opencv pytorch tensorflow tflearn theano
Step 3. You can list which ML applications are loaded in your environment using the command module list
Link to section 'Verify application import' of 'ML-Toolkit' Verify application import
Step 4. The next step is to check that you can actually use the desired ML application. You can do this by running the import command in Python. The example below tests if PyTorch has been loaded correctly.
python -c "import torch; print(torch.__version__)"
If the import operation succeeded, then you can run your own ML code. Some ML applications (such as tensorflow) print diagnostic warnings while loading -- this is the expected behavior.
If the import fails with an error, please see the troubleshooting information below.
Step 5. To load a different set of applications, unload the previously loaded applications and load the new desired applications. The example below loads Tensorflow and Keras instead of PyTorch and OpenCV.
module unload ml-toolkit-cpu/opencv
module unload ml-toolkit-cpu/pytorch
module load ml-toolkit-cpu/tensorflow
module load ml-toolkit-cpu/keras
Link to section 'Troubleshooting' of 'ML-Toolkit' Troubleshooting
ML applications depend on a wide range of Python packages and mixing multiple versions of these packages can lead to error. The following guidelines will assist you in identifying the cause of the problem.
- Check that you are using the correct version of Python with the command python --version. This should match the Python version in the loaded anaconda module.
- Start from a clean environment. Either start a new terminal session or unload all the modules using module purge. Then load the desired modules following Steps 1-2.
- Verify that PYTHONPATH does not point to undesired packages. Run the following command to print PYTHONPATH: echo $PYTHONPATH. Make sure that your Python environment is clean. Watch out for any locally installed packages that might conflict.
- If you don't see GPU devices in your code, make sure that you are using the ml-toolkit-gpu/ modules and not using their cpu versions.
- ML applications often have dependency on specific versions of Cuda and CuDNN libraries. Make sure that you have loaded the required versions using the command: module list
- Note that Caffe has a conflicting version of PyQt5. So, if you want to use Spyder (or any GUI application that uses PyQt), then you should unload the caffe module.
- Use Google search to your advantage. Copy the error message in Google and check probable causes.
More examples showing how to use ml-toolkit modules in a batch job are presented in ML Batch Jobs guide.
Link to section 'Running ML Code in a Batch Job' of 'ML Batch Jobs' Running ML Code in a Batch Job
Batch jobs allow us to automate model training without human intervention. They are also useful when you need to run a large number of simulations on the clusters. In the example below, we shall run a simple tensor_hello.py script in a batch job. We consider two situations: in the first example, we use the ML-Toolkit modules to run tensorflow, while in the second example, we use a custom installation of tensorflow (See Custom ML Packages page).
Link to section 'Using ML-Toolkit Modules' of 'ML Batch Jobs' Using ML-Toolkit Modules
Save the following code as tensor_hello.sub in the same directory where tensor_hello.py is located.
# filename: tensor_hello.sub
#SBATCH --nodes=1
#SBATCH --ntasks-per-node=128
#SBATCH --time=00:05:00
#SBATCH -A standby
#SBATCH -J hello_tensor
module purge
module load learning
module load ml-toolkit-cpu/tensorflow
module list
python tensor_hello.py
Link to section 'Using a Custom Installation' of 'ML Batch Jobs' Using a Custom Installation
Save the following code as tensor_hello.sub in the same directory where tensor_hello.py is located.
# filename: tensor_hello.sub
#SBATCH --nodes=1
#SBATCH --ntasks-per-node=1
#SBATCH --gres=gpu:1
#SBATCH --time=00:05:00
#SBATCH -A standby
#SBATCH -J hello_tensor
module purge
module load anaconda
module load use.own
module load conda-env/my_tf_env-py3.6.4
module list
echo $PYTHONPATH
python tensor_hello.py
Link to section 'Running a Job' of 'ML Batch Jobs' Running a Job
Now you can submit the batch job using the sbatch command.
sbatch tensor_hello.sub
Once the job finishes, you will find an output file (slurm-xxxxx.out).
Link to section 'Installation of Custom ML Libraries' of 'Custom ML Packages' Installation of Custom ML Libraries
While we try to include as many common ML frameworks and versions as we can in ML-Toolkit, we recognize that there are also situations in which a custom installation may be preferable. We recommend using conda-env-mod to install and manage Python packages. Please follow the steps carefully, otherwise you may end up with a faulty installation. The example below shows how to install TensorFlow in your home directory.
Link to section 'Install' of 'Custom ML Packages' Install
Step 1: Unload all modules and start with a clean environment.
module purge
Step 2: Load the anaconda module with desired Python version.
module load anaconda
Step 2A: If the ML application requires Cuda and CuDNN, load the appropriate modules. Be sure to check that the versions you load are compatible with the desired ML package.
module load cuda
module load cudnn
Many machine-learning packages (including PyTorch and TensorFlow) now provide installation pathways that include the full cudatoolkit within the environment, making it unnecessary to load these modules.
Step 3: Create a custom anaconda environment. Make sure the python version matches the Python version in the anaconda module.
conda-env-mod create -n env_name_here
Step 4: Activate the anaconda environment by loading the modules displayed at the end of step 3.
module load use.own
module load conda-env/env_name_here-py3.6.4
Step 5: Now install the desired ML application. You can install multiple Python packages at this step using either conda or pip.
pip install --ignore-installed tensorflow==2.6
If the installation succeeded, you can now proceed to testing and using the installed application. You must load the environment you created as well as any supporting modules (e.g., anaconda) whenever you want to use this installation. If your installation did not succeed, please refer to the troubleshooting section below as well as documentation for the desired package you are installing.
Note that loading the modules generated by conda-env-mod has different behavior than conda create env_name_here followed by source activate env_name_here. After running source activate, you may not be able to access any Python packages in anaconda or ml-toolkit modules. Therefore, using conda-env-mod is the preferred way of using your custom installations.
Link to section 'Testing the Installation' of 'Custom ML Packages' Testing the Installation
-
Verify the installation by using a simple import statement, like that listed below for TensorFlow:
python -c "import tensorflow as tf; print(tf.__version__);"
Note that a successful import of TensorFlow will print a variety of system and hardware information. This is expected.
If importing the package leads to errors, be sure to verify that all dependencies for the package have been managed, and the correct versions installed. Dependency issues between python packages are the most common cause for errors. For example, in TF, conflicts with the h5py or numpy versions are common, but upgrading those packages typically solves the problem. Managing dependencies for ML libraries can be non-trivial.
-
Link to section 'Troubleshooting' of 'Custom ML Packages' Troubleshooting
In most situations, dependencies among Python modules lead to errors. If you cannot use a Python package after installing it, please follow the steps below to find a workaround.
- Unload all the modules.
module purge
- Clean up PYTHONPATH.
unset PYTHONPATH
- Next load the modules, e.g., anaconda and your custom environment.
module load anaconda module load use.own module load conda-env/env_name_here-py3.6.4
- For GPU-enabled applications, you may also need to load the corresponding cuda/ and cudnn/ modules.
- Now try running your code again.
- A few applications only run on specific versions of Python (e.g. Python 3.6). Please check the documentation of your application if that is the case.
- If you have installed a newer version of an ml-toolkit package (e.g., a newer version of PyTorch or Tensorflow), make sure that the ml-toolkit modules are NOT loaded. In general, we recommend that you don't mix ml-toolkit modules with your custom installations.
- GPU-enabled ML applications often have dependencies on specific versions of Cuda and CuDNN. For example, Tensorflow version 1.5.0 and higher needs Cuda 9. Please check the application documentation about such dependencies.
Link to section 'Tensorboard' of 'Custom ML Packages' Tensorboard
- You can visualize data from a Tensorflow session using Tensorboard. For this, you need to save your session summary as described in the Tensorboard User Guide.
- Launch Tensorboard:
$ python -m tensorboard.main --logdir=/path/to/session/logs
- When Tensorboard is launched successfully, it will give you the URL for accessing Tensorboard.
<... build related warnings ...> TensorBoard 0.4.0 at http://bell-a000.rcac.purdue.edu:6006
- Follow the printed URL to visualize your model.
- Please note that due to firewall rules, the Tensorboard URL may only be accessible from Bell nodes. If you cannot access the URL directly, you can use Firefox browser in Thinlinc.
- For more details, please refer to the Tensorboard User Guide.
- Unload all the modules.
Matlab
MATLAB® (MATrix LABoratory) is a high-level language and interactive environment for numerical computation, visualization, and programming. MATLAB is a product of MathWorks.
MATLAB, Simulink, Compiler, and several of the optional toolboxes are available to faculty, staff, and students. To see the kind and quantity of all MATLAB licenses plus the number that you are currently using you can use the matlab_licenses command:
$ module load matlab
$ matlab_licenses
The MATLAB client can be run in the front-end for application development, however, computationally intensive jobs must be run on compute nodes.
The following sections provide several examples illustrating how to submit MATLAB jobs to a Linux compute cluster.
Matlab Script (.m File)
This section illustrates how to submit a small, serial, MATLAB program as a job to a batch queue. This MATLAB program prints the name of the run host and gets three random numbers.
Prepare a MATLAB script myscript.m, and a MATLAB function file myfunction.m:
% FILENAME: myscript.m
% Display name of compute node which ran this job.
[c name] = system('hostname');
fprintf('\n\nhostname:%s\n', name);
% Display three random numbers.
A = rand(1,3);
fprintf('%f %f %f\n', A);
quit;
% FILENAME: myfunction.m
function result = myfunction ()
% Return name of compute node which ran this job.
[c name] = system('hostname');
result = sprintf('hostname:%s', name);
% Return three random numbers.
A = rand(1,3);
r = sprintf('%f %f %f', A);
result=strvcat(result,r);
end
Also, prepare a job submission file, here named myjob.sub. Run with the name of the script:
#!/bin/bash
# FILENAME: myjob.sub
echo "myjob.sub"
# Load module, and set up environment for Matlab to run
module load matlab
unset DISPLAY
# -nodisplay: run MATLAB in text mode; X11 server not needed
# -singleCompThread: turn off implicit parallelism
# -r: read MATLAB program; use MATLAB JIT Accelerator
# Run Matlab, with the above options and specifying our .m file
matlab -nodisplay -singleCompThread -r myscript
myjob.sub
< M A T L A B (R) >
Copyright 1984-2011 The MathWorks, Inc.
R2011b (7.13.0.564) 64-bit (glnxa64)
August 13, 2011
To get started, type one of these: helpwin, helpdesk, or demo.
For product information, visit www.mathworks.com.
hostname:bell-a001.rcac.purdue.edu
0.814724 0.905792 0.126987
Output shows that a processor core on one compute node (bell-a001) processed the job. Output also displays the three random numbers.
For more information about MATLAB:
Implicit Parallelism
MATLAB implements implicit parallelism which is automatic multithreading of many computations, such as matrix multiplication, linear algebra, and performing the same operation on a set of numbers. This is different from the explicit parallelism of the Parallel Computing Toolbox.
MATLAB offers implicit parallelism in the form of thread-parallel enabled functions. Since these processor cores, or threads, share a common memory, many MATLAB functions contain multithreading potential. Vector operations, the particular application or algorithm, and the amount of computation (array size) contribute to the determination of whether a function runs serially or with multithreading.
When your job triggers implicit parallelism, it attempts to allocate its threads on all processor cores of the compute node on which the MATLAB client is running, including processor cores running other jobs. This competition can degrade the performance of all jobs running on the node.
When you know that you are coding a serial job but are unsure whether you are using thread-parallel enabled operations, run MATLAB with implicit parallelism turned off. Beginning with the R2009b, you can turn multithreading off by starting MATLAB with -singleCompThread:
$ matlab -nodisplay -singleCompThread -r mymatlabprogram
When you are using implicit parallelism, make sure you request exclusive access to a compute node, as MATLAB has no facility for sharing nodes.
For more information about MATLAB's implicit parallelism:
Profile Manager
MATLAB offers two kinds of profiles for parallel execution: the 'local' profile and user-defined cluster profiles. The 'local' profile runs a MATLAB job on the processor core(s) of the same compute node, or front-end, that is running the client. To run a MATLAB job on compute node(s) different from the node running the client, you must define a Cluster Profile using the Cluster Profile Manager.
To prepare a user-defined cluster profile, use the Cluster Profile Manager in the Parallel menu. This profile contains the scheduler details (queue, nodes, processors, walltime, etc.) of your job submission. Ultimately, your cluster profile will be an argument to MATLAB functions like batch().
For your convenience, a generic cluster profile is provided that can be downloaded: myslurmprofile.settings
Please note that modifications are very likely to be required to make myslurmprofile.settings work. You may need to change values for number of nodes, number of workers, walltime, and submission queue specified in the file. As well, the generic profile itself depends on the particular job scheduler on the cluster, so you may need to download or create two or more generic profiles under different names. Each time you run a job using a Cluster Profile, make sure the specific profile you are using is appropriate for the job and the cluster.
To import the profile, start a MATLAB session and select Manage Cluster Profiles... from the Parallel menu. In the Cluster Profile Manager, select Import, navigate to the folder containing the profile, select myslurmprofile.settings and click OK. Remember that the profile will need to be customized for your specific needs. If you have any questions, please contact us.
For detailed information about MATLAB's Parallel Computing Toolbox, examples, demos, and tutorials:
Parallel Computing Toolbox (parfor)
The MATLAB Parallel Computing Toolbox (PCT) extends the MATLAB language with high-level, parallel-processing features such as parallel for loops, parallel regions, message passing, distributed arrays, and parallel numerical methods. It offers a shared-memory computing environment running on the local cluster profile in addition to your MATLAB client. Moreover, the MATLAB Distributed Computing Server (DCS) scales PCT applications up to the limit of your DCS licenses.
This section illustrates the fine-grained parallelism of a parallel for loop (parfor) in a pool job.
The following examples illustrate a method for submitting a small, parallel, MATLAB program with a parallel loop (parfor statement) as a job to a queue. This MATLAB program prints the name of the run host and shows the values of variables numlabs and labindex for each iteration of the parfor loop.
This method uses the job submission command to submit a MATLAB client which calls the MATLAB batch() function with a user-defined cluster profile.
Prepare a MATLAB pool program in a MATLAB script with an appropriate filename, here named myscript.m:
% FILENAME: myscript.m
% SERIAL REGION
[c name] = system('hostname');
fprintf('SERIAL REGION: hostname:%s\n', name)
numlabs = parpool('poolsize');
fprintf(' hostname numlabs labindex iteration\n')
fprintf(' ------------------------------- ------- -------- ---------\n')
tic;
% PARALLEL LOOP
parfor i = 1:8
[c name] = system('hostname');
name = name(1:length(name)-1);
fprintf('PARALLEL LOOP: %-31s %7d %8d %9d\n', name,numlabs,labindex,i)
pause(2);
end
% SERIAL REGION
elapsed_time = toc; % get elapsed time in parallel loop
fprintf('\n')
[c name] = system('hostname');
name = name(1:length(name)-1);
fprintf('SERIAL REGION: hostname:%s\n', name)
fprintf('Elapsed time in parallel loop: %f\n', elapsed_time)
The execution of a pool job starts with a worker executing the statements of the first serial region up to the parfor block, when it pauses. A set of workers (the pool) executes the parfor block. When they finish, the first worker resumes by executing the second serial region. The code displays the names of the compute nodes running the batch session and the worker pool.
Prepare a MATLAB script that calls MATLAB function batch() which makes a four-lab pool on which to run the MATLAB code in the file myscript.m. Use an appropriate filename, here named mylclbatch.m:
% FILENAME: mylclbatch.m
!echo "mylclbatch.m"
!hostname
pjob=batch('myscript','Profile','myslurmprofile','Pool',4,'CaptureDiary',true);
wait(pjob);
diary(pjob);
quit;
Prepare a job submission file with an appropriate filename, here named myjob.sub:
#!/bin/bash
# FILENAME: myjob.sub
echo "myjob.sub"
hostname
module load matlab
unset DISPLAY
matlab -nodisplay -r mylclbatch
Submit the job as a single compute node with one processor core.
One processor core runs myjob.sub and mylclbatch.m.
Once this job starts, a second job submission is made.
myjob.sub
< M A T L A B (R) >
Copyright 1984-2013 The MathWorks, Inc.
R2013a (8.1.0.604) 64-bit (glnxa64)
February 15, 2013
To get started, type one of these: helpwin, helpdesk, or demo.
For product information, visit www.mathworks.com.
mylclbatch.mbell-a000.rcac.purdue.edu
SERIAL REGION: hostname:bell-a000.rcac.purdue.edu
hostname numlabs labindex iteration
------------------------------- ------- -------- ---------
PARALLEL LOOP: bell-a001.rcac.purdue.edu 4 1 2
PARALLEL LOOP: bell-a002.rcac.purdue.edu 4 1 4
PARALLEL LOOP: bell-a001.rcac.purdue.edu 4 1 5
PARALLEL LOOP: bell-a002.rcac.purdue.edu 4 1 6
PARALLEL LOOP: bell-a003.rcac.purdue.edu 4 1 1
PARALLEL LOOP: bell-a003.rcac.purdue.edu 4 1 3
PARALLEL LOOP: bell-a004.rcac.purdue.edu 4 1 7
PARALLEL LOOP: bell-a004.rcac.purdue.edu 4 1 8
SERIAL REGION: hostname:bell-a001.rcac.purdue.edu
Elapsed time in parallel loop: 5.411486
To scale up this method to handle a real application, increase the wall time in the submission command to accommodate a longer running job. Secondly, increase the wall time of myslurmprofile by using the Cluster Profile Manager in the Parallel menu to enter a new wall time in the property SubmitArguments.
For more information about MATLAB Parallel Computing Toolbox:
Parallel Toolbox (spmd)
The MATLAB Parallel Computing Toolbox (PCT) extends the MATLAB language with high-level, parallel-processing features such as parallel for loops, parallel regions, message passing, distributed arrays, and parallel numerical methods. It offers a shared-memory computing environment with a maximum of eight MATLAB workers (labs, threads; versions R2009a) and 12 workers (labs, threads; version R2011a) running on the local configuration in addition to your MATLAB client. Moreover, the MATLAB Distributed Computing Server (DCS) scales PCT applications up to the limit of your DCS licenses.
This section illustrates how to submit a small, parallel, MATLAB program with a parallel region (spmd statement) as a MATLAB pool job to a batch queue.
This example uses the submission command to submit to compute nodes a MATLAB client which interprets a Matlab .m with a user-defined cluster profile which scatters the MATLAB workers onto different compute nodes. This method uses the MATLAB interpreter, the Parallel Computing Toolbox, and the Distributed Computing Server; so, it requires and checks out six licenses: one MATLAB license for the client running on the compute node, one PCT license, and four DCS licenses. Four DCS licenses run the four copies of the spmd statement. This job is completely off the front end.
Prepare a MATLAB script called myscript.m:
% FILENAME: myscript.m
% SERIAL REGION
[c name] = system('hostname');
fprintf('SERIAL REGION: hostname:%s\n', name)
p = parpool('4');
fprintf(' hostname numlabs labindex\n')
fprintf(' ------------------------------- ------- --------\n')
tic;
% PARALLEL REGION
spmd
[c name] = system('hostname');
name = name(1:length(name)-1);
fprintf('PARALLEL REGION: %-31s %7d %8d\n', name,numlabs,labindex)
pause(2);
end
% SERIAL REGION
elapsed_time = toc; % get elapsed time in parallel region
delete(p);
fprintf('\n')
[c name] = system('hostname');
name = name(1:length(name)-1);
fprintf('SERIAL REGION: hostname:%s\n', name)
fprintf('Elapsed time in parallel region: %f\n', elapsed_time)
quit;
Prepare a job submission file with an appropriate filename, here named myjob.sub. Run with the name of the script:
#!/bin/bash
# FILENAME: myjob.sub
echo "myjob.sub"
module load matlab
unset DISPLAY
matlab -nodisplay -r myscript
Run MATLAB to set the default parallel configuration to your job configuration:
$ matlab -nodisplay
>> parallel.defaultClusterProfile('myslurmprofile');
>> quit;
$
Once this job starts, a second job submission is made.
myjob.sub
< M A T L A B (R) >
Copyright 1984-2011 The MathWorks, Inc.
R2011b (7.13.0.564) 64-bit (glnxa64)
August 13, 2011
To get started, type one of these: helpwin, helpdesk, or demo.
For product information, visit www.mathworks.com.
SERIAL REGION: hostname:bell-a001.rcac.purdue.edu
Starting matlabpool using the 'myslurmprofile' profile ... connected to 4 labs.
hostname numlabs labindex
------------------------------- ------- --------
Lab 2:
PARALLEL REGION: bell-a002.rcac.purdue.edu 4 2
Lab 1:
PARALLEL REGION: bell-a001.rcac.purdue.edu 4 1
Lab 3:
PARALLEL REGION: bell-a003.rcac.purdue.edu 4 3
Lab 4:
PARALLEL REGION: bell-a004.rcac.purdue.edu 4 4
Sending a stop signal to all the labs ... stopped.
SERIAL REGION: hostname:bell-a001.rcac.purdue.edu
Elapsed time in parallel region: 3.382151
Output shows the name of one compute node (a001) that processed the job submission file myjob.sub and the two serial regions. The job submission scattered four processor cores (four MATLAB labs) among four different compute nodes (a001,a002,a003,a004) that processed the four parallel regions. The total elapsed time demonstrates that the jobs ran in parallel.
For more information about MATLAB Parallel Computing Toolbox:
Distributed Computing Server (parallel job)
The MATLAB Parallel Computing Toolbox (PCT) enables a parallel job via the MATLAB Distributed Computing Server (DCS). The tasks of a parallel job are identical, run simultaneously on several MATLAB workers (labs), and communicate with each other. This section illustrates an MPI-like program.
This section illustrates how to submit a small, MATLAB parallel job with four workers running one MPI-like task to a batch queue. The MATLAB program broadcasts an integer to four workers and gathers the names of the compute nodes running the workers and the lab IDs of the workers.
This example uses the job submission command to submit a Matlab script with a user-defined cluster profile which scatters the MATLAB workers onto different compute nodes. This method uses the MATLAB interpreter, the Parallel Computing Toolbox, and the Distributed Computing Server; so, it requires and checks out six licenses: one MATLAB license for the client running on the compute node, one PCT license, and four DCS licenses. Four DCS licenses run the four copies of the parallel job. This job is completely off the front end.
Prepare a MATLAB script named myscript.m :
% FILENAME: myscript.m
% Specify pool size.
% Convert the parallel job to a pool job.
parpool('4');
spmd
if labindex == 1
% Lab (rank) #1 broadcasts an integer value to other labs (ranks).
N = labBroadcast(1,int64(1000));
else
% Each lab (rank) receives the broadcast value from lab (rank) #1.
N = labBroadcast(1);
end
% Form a string with host name, total number of labs, lab ID, and broadcast value.
[c name] =system('hostname');
name = name(1:length(name)-1);
fmt = num2str(floor(log10(numlabs))+1);
str = sprintf(['%s:%d:%' fmt 'd:%d '], name,numlabs,labindex,N);
% Apply global concatenate to all str's.
% Store the concatenation of str's in the first dimension (row) and on lab #1.
result = gcat(str,1,1);
if labindex == 1
disp(result)
end
end % spmd
matlabpool close force;
quit;
Also, prepare a job submission, here named myjob.sub. Run with the name of the script:
# FILENAME: myjob.sub
echo "myjob.sub"
module load matlab
unset DISPLAY
# -nodisplay: run MATLAB in text mode; X11 server not needed
# -r: read MATLAB program; use MATLAB JIT Accelerator
matlab -nodisplay -r myscript
Run MATLAB to set the default parallel configuration to your appropriate Profile:
$ matlab -nodisplay
>> defaultParallelConfig('myslurmprofile');
>> quit;
$
Submit the job as a single compute node with one processor core.
Once this job starts, a second job submission is made.
myjob.sub
< M A T L A B (R) >
Copyright 1984-2011 The MathWorks, Inc.
R2011b (7.13.0.564) 64-bit (glnxa64)
August 13, 2011
To get started, type one of these: helpwin, helpdesk, or demo.
For product information, visit www.mathworks.com.
>Starting matlabpool using the 'myslurmprofile' configuration ... connected to 4 labs.
Lab 1:
bell-a006.rcac.purdue.edu:4:1:1000
bell-a007.rcac.purdue.edu:4:2:1000
bell-a008.rcac.purdue.edu:4:3:1000
bell-a009.rcac.purdue.edu:4:4:1000
Sending a stop signal to all the labs ... stopped.
Did not find any pre-existing parallel jobs created by matlabpool.
Output shows the name of one compute node (a006) that processed the job submission file myjob.sub. The job submission scattered four processor cores (four MATLAB labs) among four different compute nodes (a006,a007,a008,a009) that processed the four parallel regions.
To scale up this method to handle a real application, increase the wall time in the submission command to accommodate a longer running job. Secondly, increase the wall time of myslurmprofile by using the Cluster Profile Manager in the Parallel menu to enter a new wall time in the property SubmitArguments.
For more information about parallel jobs:
Python
Notice: Python 2.7 has reached end-of-life on Jan 1, 2020 (announcement). Please update your codes and your job scripts to use Python 3.
Python is a high-level, general-purpose, interpreted, dynamic programming language. We suggest using Anaconda which is a Python distribution made for large-scale data processing, predictive analytics, and scientific computing. For example, to use the default Anaconda distribution:
$ module load conda
For a full list of available Anaconda and Python modules enter:
$ module spider conda
Example Python Jobs
This section illustrates how to submit a small Python job to a SLURM queue.
Link to section 'Example 1: Hello world' of 'Example Python Jobs' Example 1: Hello world
Prepare a Python input file with an appropriate filename, here named hello.py
:
# FILENAME: hello.py
import string, sys
print("Hello, world!")
Prepare a job submission file with an appropriate filename, here named myjob.sub:
#!/bin/bash
# FILENAME: myjob.sub
module load conda
python hello.py
Hello, world!
Link to section 'Example 2: Matrix multiply' of 'Example Python Jobs' Example 2: Matrix multiply
Save the following script as matrix.py:
# Matrix multiplication program
x = [[3,1,4],[1,5,9],[2,6,5]]
y = [[3,5,8,9],[7,9,3,2],[3,8,4,6]]
result = [[sum(a*b for a,b in zip(x_row,y_col)) for y_col in zip(*y)] for x_row in x]
for r in result:
print(r)
Change the last line in the job submission file above to read:
python matrix.py
The standard output file from this job will result in the following matrix:
[28, 56, 43, 53]
[65, 122, 59, 73]
[63, 104, 54, 60]
Link to section 'Example 3: Sine wave plot using numpy and matplotlib packages' of 'Example Python Jobs' Example 3: Sine wave plot using numpy and matplotlib packages
Save the following script as sine.py:
import numpy as np
import matplotlib
matplotlib.use('Agg')
import matplotlib.pyplot as plt
x = np.linspace(-np.pi, np.pi, 201)
plt.plot(x, np.sin(x))
plt.xlabel('Angle [rad]')
plt.ylabel('sin(x)')
plt.axis('tight')
plt.savefig('sine.png')
Change your job submission file to submit this script and the job will output a png file and blank standard output and error files.
For more information about Python:
Managing Environments with Conda
Conda is a package manager in Anaconda that allows you to create and manage multiple environments where you can pick and choose which packages you want to use. To use Conda you must load an Anaconda module:
$ module load conda
Many packages are pre-installed in the global environment. To see these packages:
$ conda list
To create your own custom environment:
$ conda create --name MyEnvName python=3.8 FirstPackageName SecondPackageName -y
The --name option specifies that the environment created will be named MyEnvName. You can include as many packages as you require separated by a space. Including the -y option lets you skip the prompt to install the package. By default environments are created and stored in the $HOME/.conda directory.
To create an environment at a custom location:
$ conda create --prefix=$HOME/MyEnvName python=3.8 PackageName -y
To see a list of your environments:
$ conda env list
To remove unwanted environments:
$ conda remove --name MyEnvName --all
To add packages to your environment:
$ conda install --name MyEnvName PackageNames
To remove a package from an environment:
$ conda remove --name MyEnvName PackageName
Installing packages when creating your environment, instead of one at a time, will help you avoid dependency issues.
To activate or deactivate an environment you have created:
$ source activate MyEnvName
$ source deactivate MyEnvName
If you created your conda environment at a custom location using --prefix option, then you can activate or deactivate it using the full path.
$ source activate $HOME/MyEnvName
$ source deactivate $HOME/MyEnvName
To use a custom environment inside a job you must load the module and activate the environment inside your job submission script. Add the following lines to your submission script:
$ module load conda
$ source activate MyEnvName
For more information about Python:
Managing Packages with Pip
Pip is a Python package manager. Many Python package documentation provide pip
instructions that result in permission errors because by default pip
will install in a system-wide location and fail.
Exception:
Traceback (most recent call last):
... ... stack trace ... ...
OSError: [Errno 13] Permission denied: '/apps/cent7/anaconda/2020.07-py38/lib/python3.8/site-packages/mkl_random-1.1.1.dist-info'
If you encounter this error, it means that you cannot modify the global Python installation. We recommend installing Python packages in a conda environment. Detailed instructions for installing packages with pip can be found in our Python package installation page.
Below we list some other useful pip commands.
- Search for a package in PyPI channels:
$ pip search packageName
- Check which packages are installed globally:
$ pip list
- Check which packages you have personally installed:
$ pip list --user
- Snapshot installed packages:
$ pip freeze > requirements.txt
- You can install packages from a snapshot inside a new conda environment. Make sure to load the appropriate conda environment first.
$ pip install -r requirements.txt
For more information about Python:
Installing Packages
Installing Python packages in an Anaconda environment is recommended. One key advantage of Anaconda is that it allows users to install unrelated packages in separate self-contained environments. Individual packages can later be reinstalled or updated without impacting others. If you are unfamiliar with Conda environments, please check our Conda Guide.
To facilitate the process of creating and using Conda environments, we support a script (conda-env-mod) that generates a module file for an environment, as well as an optional Jupyter kernel to use this environment in a JupyterHub notebook.
You must load one of the anaconda modules in order to use this script.
$ module load conda
Step-by-step instructions for installing custom Python packages are presented below.
Link to section 'Step 1: Create a conda environment' of 'Installing Packages' Step 1: Create a conda environment
Users can use the conda-env-mod script to create an empty conda environment. This script needs either a name or a path for the desired environment. After the environment is created, it generates a module file for using it in future. Please note that conda-env-mod is different from the official conda-env script and supports a limited set of subcommands. Detailed instructions for using conda-env-mod can be found with the command conda-env-mod --help.
-
Example 1: Create a conda environment named mypackages in user's
$HOME
directory.$ conda-env-mod create -n mypackages
-
Example 2: Create a conda environment named mypackages at a custom location.
$ conda-env-mod create -p /depot/mylab/apps/mypackages
Please follow the on-screen instructions while the environment is being created. After finishing, the script will print the instructions to use this environment.
... ... ... Preparing transaction: ...working... done Verifying transaction: ...working... done Executing transaction: ...working... done +------------------------------------------------------+ | To use this environment, load the following modules: | | module load use.own | | module load conda-env/mypackages-py3.8.5 | +------------------------------------------------------+ Your environment "mypackages" was created successfully.
Note down the module names, as you will need to load these modules every time you want to use this environment. You may also want to add the module load lines in your jobscript, if it depends on custom Python packages.
By default, module files are generated in your $HOME/privatemodules directory. The location of module files can be customized by specifying the -m /path/to/modules option to conda-env-mod.
Note: The main differences between -p and -m are: 1) -p will change the location of packages to be installed for the env and the module file will still be located at the $HOME/privatemodules directory as defined in use.own. 2) -m will only change the location of the module file. So the method to load modules created with -m and -p are different, see Example 3 for details.
- Example 3: Create a conda environment named labpackages in your group's Data Depot space and place the module file at a shared location for the group to use.
$ conda-env-mod create -p /depot/mylab/apps/labpackages -m /depot/mylab/etc/modules ... ... ... Preparing transaction: ...working... done Verifying transaction: ...working... done Executing transaction: ...working... done +-------------------------------------------------------+ | To use this environment, load the following modules: | | module use /depot/mylab/etc/modules | | module load conda-env/labpackages-py3.8.5 | +-------------------------------------------------------+ Your environment "labpackages" was created successfully.
If you used a custom module file location, you need to run the module use command as printed by the command output above.
By default, only the environment and a module file are created (no Jupyter kernel). If you plan to use your environment in a JupyterHub notebook, you need to append a --jupyter flag to the above commands.
- Example 4: Create a Jupyter-enabled conda environment named labpackages in your group's Data Depot space and place the module file at a shared location for the group to use.
$ conda-env-mod create -p /depot/mylab/apps/labpackages -m /depot/mylab/etc/modules --jupyter ... ... ... Jupyter kernel created: "Python (My labpackages Kernel)" ... ... ... Your environment "labpackages" was created successfully.
Link to section 'Step 2: Load the conda environment' of 'Installing Packages' Step 2: Load the conda environment
-
The following instructions assume that you have used conda-env-mod script to create an environment named mypackages (Examples 1 or 2 above). If you used conda create instead, please use conda activate mypackages.
$ module load use.own $ module load conda-env/mypackages-py3.8.5
Note that the conda-env module name includes the Python version that it supports (Python 3.8.5 in this example). This is same as the Python version in the conda module.
-
If you used a custom module file location (Example 3 above), please use module use to load the conda-env module.
$ module use /depot/mylab/etc/modules $ module load conda-env/labpackages-py3.8.5
Link to section 'Step 3: Install packages' of 'Installing Packages' Step 3: Install packages
Now you can install custom packages in the environment using either conda install or pip install.
Link to section 'Installing with conda' of 'Installing Packages' Installing with conda
-
Example 1: Install OpenCV (open-source computer vision library) using conda.
$ conda install opencv
-
Example 2: Install a specific version of OpenCV using conda.
$ conda install opencv=4.5.5
-
Example 3: Install OpenCV from a specific anaconda channel.
$ conda install -c anaconda opencv
Link to section 'Installing with pip' of 'Installing Packages' Installing with pip
-
Example 4: Install pandas using pip.
$ pip install pandas
-
Example 5: Install a specific version of pandas using pip.
$ pip install pandas==1.4.3
Follow the on-screen instructions while the packages are being installed. If installation is successful, please proceed to the next section to test the packages.
Note: Do NOT run Pip with the --user argument, as that will install packages in a different location and might mess up your account environment.
Link to section 'Step 4: Test the installed packages' of 'Installing Packages' Step 4: Test the installed packages
To use the installed Python packages, you must load the module for your conda environment. If you have not loaded the conda-env module, please do so following the instructions at the end of Step 1.
$ module load use.own
$ module load conda-env/mypackages-py3.8.5
- Example 1: Test that OpenCV is available.
$ python -c "import cv2; print(cv2.__version__)"
- Example 2: Test that pandas is available.
$ python -c "import pandas; print(pandas.__version__)"
If the commands finished without errors, then the installed packages can be used in your program.
Link to section 'Additional capabilities of conda-env-mod script' of 'Installing Packages' Additional capabilities of conda-env-mod script
The conda-env-mod tool is intended to facilitate creation of a minimal Anaconda environment, matching module file and optionally a Jupyter kernel. Once created, the environment can then be accessed via familiar module load command, tuned and expanded as necessary. Additionally, the script provides several auxiliary functions to help manage environments, module files and Jupyter kernels.
General usage for the tool adheres to the following pattern:
$ conda-env-mod help
$ conda-env-mod <subcommand> <required argument> [optional arguments]
where required arguments are one of
- -n|--name ENV_NAME (name of the environment)
- -p|--prefix ENV_PATH (location of the environment)
and optional arguments further modify behavior for specific actions (e.g. -m to specify alternative location for generated module files).
Given a required name or prefix for an environment, the conda-env-mod script supports the following subcommands:
- create - to create a new environment, its corresponding module file and optional Jupyter kernel.
- delete - to delete existing environment along with its module file and Jupyter kernel.
- module - to generate just the module file for a given existing environment.
- kernel - to generate just the Jupyter kernel for a given existing environment (note that the environment has to be created with a --jupyter option).
- help - to display script usage help.
Using these subcommands, you can iteratively fine-tune your environments, module files and Jupyter kernels, as well as delete and re-create them with ease. Below we cover several commonly occurring scenarios.
Note: When you try to use conda-env-mod delete, remember to include the arguments as you create the environment (i.e. -p package_location and/or -m module_location).
Link to section 'Generating module file for an existing environment' of 'Installing Packages' Generating module file for an existing environment
If you already have an existing configured Anaconda environment and want to generate a module file for it, follow appropriate examples from Step 1 above, but use the module subcommand instead of the create one. E.g.
$ conda-env-mod module -n mypackages
and follow printed instructions on how to load this module. With an optional --jupyter flag, a Jupyter kernel will also be generated.
Note that the module name mypackages should be exactly the same with the older conda environment name. Note also that if you intend to proceed with a Jupyter kernel generation (via the --jupyter flag or a kernel subcommand later), you will have to ensure that your environment has ipython and ipykernel packages installed into it. To avoid this and other related complications, we highly recommend making a fresh environment using a suitable conda-env-mod create .... --jupyter command instead.
Link to section 'Generating Jupyter kernel for an existing environment' of 'Installing Packages' Generating Jupyter kernel for an existing environment
If you already have an existing configured Anaconda environment and want to generate a Jupyter kernel file for it, you can use the kernel subcommand. E.g.
$ conda-env-mod kernel -n mypackages
This will add a "Python (My mypackages Kernel)" item to the dropdown list of available kernels upon your next login to the JupyterHub.
Note that generated Jupiter kernels are always personal (i.e. each user has to make their own, even for shared environments). Note also that you (or the creator of the shared environment) will have to ensure that your environment has ipython and ipykernel packages installed into it.
Link to section 'Managing and using shared Python environments' of 'Installing Packages' Managing and using shared Python environments
Here is a suggested workflow for a common group-shared Anaconda environment with Jupyter capabilities:
The PI or lab software manager:
-
Creates the environment and module file (once):
$ module purge $ module load conda $ conda-env-mod create -p /depot/mylab/apps/labpackages -m /depot/mylab/etc/modules --jupyter
-
Installs required Python packages into the environment (as many times as needed):
$ module use /depot/mylab/etc/modules $ module load conda-env/labpackages-py3.8.5 $ conda install ....... # all the necessary packages
Lab members:
-
Lab members can start using the environment in their command line scripts or batch jobs simply by loading the corresponding module:
$ module use /depot/mylab/etc/modules $ module load conda-env/labpackages-py3.8.5 $ python my_data_processing_script.py .....
-
To use the environment in Jupyter notebooks, each lab member will need to create his/her own Jupyter kernel (once). This is because Jupyter kernels are private to individuals, even for shared environments.
$ module use /depot/mylab/etc/modules $ module load conda-env/labpackages-py3.8.5 $ conda-env-mod kernel -p /depot/mylab/apps/labpackages
A similar process can be devised for instructor-provided or individually-managed class software, etc.
Link to section 'Troubleshooting' of 'Installing Packages' Troubleshooting
- Python packages often fail to install or run due to dependency incompatibility with other packages. More specifically, if you previously installed packages in your home directory it is safer to clean those installations.
$ mv ~/.local ~/.local.bak $ mv ~/.cache ~/.cache.bak
- Unload all the modules.
$ module purge
- Clean up PYTHONPATH.
$ unset PYTHONPATH
- Next load the modules (e.g. anaconda) that you need.
$ module load conda/2024.02-py311 $ module load use.own $ module load conda-env/2024.02-py311
- Now try running your code again.
- Few applications only run on specific versions of Python (e.g. Python 3.6). Please check the documentation of your application if that is the case.
Installing Packages from Source
We maintain several Anaconda installations. Anaconda maintains numerous popular scientific Python libraries in a single installation. If you need a Python library not included with normal Python we recommend first checking Anaconda. For a list of modules currently installed in the Anaconda Python distribution:
$ module load conda
$ conda list
# packages in environment at /apps/spack/bell/apps/anaconda/2020.02-py37-gcc-4.8.5-u747gsx:
#
# Name Version Build Channel
_ipyw_jlab_nb_ext_conf 0.1.0 py37_0
_libgcc_mutex 0.1 main
alabaster 0.7.12 py37_0
anaconda 2020.02 py37_0
...
If you see the library in the list, you can simply import it into your Python code after loading the Anaconda module.
If you do not find the package you need, you should be able to install the library in your own Anaconda customization. First try to install it with Conda or Pip. If the package is not available from either Conda or Pip, you may be able to install it from source.
Use the following instructions as a guideline for installing packages from source. Make sure you have a download link to the software (usually it will be a tar.gz
archive file). You will substitute it on the wget line below.
We also assume that you have already created an empty conda environment as described in our Python package installation guide.
$ mkdir ~/src
$ cd ~/src
$ wget http://path/to/source/tarball/app-1.0.tar.gz
$ tar xzvf app-1.0.tar.gz
$ cd app-1.0
$ module load conda
$ module load use.own
$ module load conda-env/mypackages-py3.8.5
$ python setup.py install
$ cd ~
$ python
>>> import app
>>> quit()
The "import app" line should return without any output if installed successfully. You can then import the package in your python scripts.
If you need further help or run into any issues installing a library, contact us or drop by Coffee Hour for in-person help.
For more information about Python:
Example: Create and Use Biopython Environment with Conda
Link to section 'Using conda to create an environment that uses the biopython package' of 'Example: Create and Use Biopython Environment with Conda' Using conda to create an environment that uses the biopython package
To use Conda you must first load the anaconda module:
module load conda
Create an empty conda environment to install biopython:
conda-env-mod create -n biopython
Now activate the biopython environment:
module load use.own
module load conda-env/biopython-py3.12.5
Install the biopython packages in your environment:
conda install --channel anaconda biopython -y
Fetching package metadata ..........
Solving package specifications .........
.......
Linking packages ...
[ COMPLETE ]|################################################################
The --channel option specifies that it searches the anaconda channel for the biopython package. The -y argument is optional and allows you to skip the installation prompt. A list of packages will be displayed as they are installed.
Remember to add the following lines to your job submission script to use the custom environment in your jobs:
module load conda
module load use.own
module load conda-env/biopython-py3.12.5
If you need further help or run into any issues with creating environments, contact us or drop by Coffee Hour for in-person help.
For more information about Python:
Numpy Parallel Behavior
The widely available Numpy package is the best way to handle numerical computation in Python. The numpy
package provided by our anaconda
modules is optimized using Intel's MKL library. It will automatically parallelize many operations to make use of all the cores available on a machine.
In many contexts that would be the ideal behavior. On the cluster however that very likely is not in fact the preferred behavior because often more than one user is present on the system and/or more than one job on a node. Having multiple processes contend for those resources will actually result in lesser performance.
Setting the MKL_NUM_THREADS
or OMP_NUM_THREADS
environment variable(s) allows you to control this behavior. Our anaconda modules automatically set these variables to 1 if and only if you do not currently have that variable defined.
When submitting batch jobs it is always a good idea to be explicit rather than implicit. If you are submitting a job that you want to make use of the full resources available on the node, set one or both of these variables to the number of cores you want to allow numpy to make use of.
#!/bin/bash
module load conda
export MKL_NUM_THREADS=128
...
If you are submitting multiple jobs that you intend to be scheduled together on the same node, it is probably best to restrict numpy to a single core.
#!/bin/bash
module load conda
export MKL_NUM_THREADS=1
R
R, a GNU project, is a language and environment for data manipulation, statistics, and graphics. It is an open source version of the S programming language. R is quickly becoming the language of choice for data science due to the ease with which it can produce high quality plots and data visualizations. It is a versatile platform with a large, growing community and collection of packages.
For more general information on R visit The R Project for Statistical Computing.
Running R jobs
This section illustrates how to submit a small R job to a SLURM queue. The example job computes a Pythagorean triple.
Prepare an R input file with an appropriate filename, here named myjob.R:
# FILENAME: myjob.R
# Compute a Pythagorean triple.
a = 3
b = 4
c = sqrt(a*a + b*b)
c # display result
Prepare a job submission file with an appropriate filename, here named myjob.sub:
#!/bin/bash
# FILENAME: myjob.sub
module load r
# --vanilla:
# --no-save: do not save datasets at the end of an R session
R --vanilla --no-save < myjob.R
For other examples or R jobs:
Installing R packages
Link to section 'Challenges of Managing R Packages in the Cluster Environment' of 'Installing R packages' Challenges of Managing R Packages in the Cluster Environment
- Different clusters have different hardware and softwares. So, if you have access to multiple clusters, you must install your R packages separately for each cluster.
- Each cluster has multiple versions of R and packages installed with one version of R may not work with another version of R. So, libraries for each R version must be installed in a separate directory.
- You can define the directory where your R packages will be installed using the environment variable
R_LIBS_USER
. - For your convenience, a sample ~/.Rprofile example file is provided that can be downloaded to your cluster account and renamed into
~/.Rprofile
(or appended to one) to customize your installation preferences. Detailed instructions.
Link to section 'Installing Packages' of 'Installing R packages' Installing Packages
-
Step 0: Set up installation preferences.
Follow the steps for setting up your~/.Rprofile
preferences. This step needs to be done only once. If you have created a~/.Rprofile
file previously on Bell, ignore this step. -
Step 1: Check if the package is already installed.
As part of the R installations on community clusters, a lot of R libraries are pre-installed. You can check if your package is already installed by opening an R terminal and entering the commandinstalled.packages()
. For example,module load r/4.4.1 R
installed.packages()["units",c("Package","Version")] Package Version "units" "0.8-1" quit()
If the package you are trying to use is already installed, simply load the library, e.g.,
library('units')
. Otherwise, move to the next step to install the package. -
Step 2: Load required dependencies. (if needed)
For simple packages you may not need this step. However, some R packages depend on other libraries. For example, thesf
package depends ongdal
andgeos
libraries. So, you will need to load the corresponding modules before installingsf
. Read the documentation for the package to identify which modules should be loaded.module load gdal module load geos
-
Step 3: Install the package.
Now install the desired package using the commandinstall.packages('package_name')
. R will automatically download the package and all its dependencies from CRAN and install each one. Your terminal will show the build progress and eventually show whether the package was installed successfully or not.R
install.packages('sf', repos="https://cran.case.edu/") Installing package into ‘/home/myusername/R/x86_64-pc-linux-gnu-library/4.4.1’ (as ‘lib’ is unspecified) trying URL 'https://cran.case.edu/src/contrib/sf_0.9-7.tar.gz' Content type 'application/x-gzip' length 4203095 bytes (4.0 MB) ================================================== downloaded 4.0 MB ... ... more progress messages ... ... ** testing if installed package can be loaded from final location ** testing if installed package keeps a record of temporary installation path * DONE (sf) The downloaded source packages are in ‘/tmp/RtmpSVAGio/downloaded_packages’
- Step 4: Troubleshooting. (if needed)
If Step 3 ended with an error, you need to investigate why the build failed. Most common reason for build failure is not loading the necessary modules.
Link to section 'Loading Libraries' of 'Installing R packages' Loading Libraries
Once you have packages installed you can load them with the library()
function as shown below:
library('packagename')
The package is now installed and loaded and ready to be used in R.
Link to section 'Example: Installing dplyr' of 'Installing R packages' Example: Installing dplyr
The following demonstrates installing the dplyr
package assuming the above-mentioned custom ~/.Rprofile
is in place (note its effect in the "Installing package into" information message):
module load r
R
install.packages('dplyr', repos="http://ftp.ussg.iu.edu/CRAN/")
Installing package into ‘/home/myusername/R/bell/4.4.1’
(as ‘lib’ is unspecified)
...
also installing the dependencies 'crayon', 'utf8', 'bindr', 'cli', 'pillar', 'assertthat', 'bindrcpp', 'glue', 'pkgconfig', 'rlang', 'Rcpp', 'tibble', 'BH', 'plogr'
...
...
...
The downloaded source packages are in
'/tmp/RtmpHMzm9z/downloaded_packages'
library(dplyr)
Attaching package: 'dplyr'
For more information about installing R packages:
Loading Data into R
R is an environment for manipulating data. In order to manipulate data, it must be brought into the R environment. R has a function to read any file that data is stored in. Some of the most common file types like comma-separated variable(CSV) files have functions that come in the basic R packages. Other less common file types require additional packages to be installed. To read data from a CSV file into the R environment, enter the following command in the R prompt:
> read.csv(file = "path/to/data.csv", header = TRUE)
When R reads the file it creates an object that can then become the target of other functions. By default the read.csv() function will give the object the name of the .csv file. To assign a different name to the object created by read.csv enter the following in the R prompt:
> my_variable <- read.csv(file = "path/to/data.csv", header = FALSE)
To display the properties (structure) of loaded data, enter the following:
> str(my_variable)
For more functions and tutorials:
RStudio
RStudio is a graphical integrated development environment (IDE) for R. RStudio is the most popular environment for developing both R scripts and packages. RStudio is provided on most Research systems.
There are two methods to launch RStudio on the cluster: command-line and application menu icon.
Link to section 'Launch RStudio by the command-line:' of 'RStudio' Launch RStudio by the command-line:
module load gcc
module load r
module load rstudio
rstudio
Note that RStudio is a graphical program and in order to run it you must have a local X11 server running or use Thinlinc Remote Desktop environment. See the ssh X11 forwarding section for more details.
Link to section 'Launch Rstudio by the application menu icon:' of 'RStudio' Launch Rstudio by the application menu icon:
- Log into desktop.bell.rcac.purdue.edu with web browser or ThinLinc client
- Click on the
Applications
drop down menu on the top left corner - Choose
Cluster Software
and thenRStudio
R and RStudio are free to download and run on your local machine. For more information about RStudio:
Setting Up R Preferences with .Rprofile
For your convenience, a sample ~/.Rprofile example file is provided that can be downloaded to your cluster account and renamed into ~/.Rprofile
(or appended to one). Follow these steps to download our recommended ~/.Rprofile
example and copy it into place:
curl -#LO https://www.rcac.purdue.edu/files/knowledge/run/examples/apps/r/Rprofile_example
mv -ib Rprofile_example ~/.Rprofile
The above installation step needs to be done only once on Bell. Now load the R module and run R:
module load r/4.4.1
R
.libPaths()
[1] "/home/myusername/R/bell/4.1.2-gcc-6.3.0-ymdumss"
[2] "/apps/spack/bell/apps/r/4.1.2-gcc-6.3.0-ymdumss/rlib/R/library"
.libPaths()
should output something similar to above if it is set up correctly.
You are now ready to install R packages into the dedicated directory /home/myusername/R/bell/4.1.2-gcc-6.3.0-ymdumss
.
Singularity
Note: Singularity was originally a project out of Lawrence Berkeley National Laboratory. It has now been spun off into a distinct offering under a new corporate entity under the name Sylabs Inc. This guide pertains to the open source community edition, SingularityCE.
Link to section 'What is Singularity?' of 'Singularity' What is Singularity?
Singularity is a new feature of the Community Clusters allowing the portability and reproducibility of operating system and application environments through the use of Linux containers. It gives users complete control over their environment.
Singularity is like Docker but tuned explicitly for HPC clusters. More information is available from the project’s website.
Link to section 'Features' of 'Singularity' Features
- Run the latest applications on an Ubuntu or Centos userland
- Gain access to the latest developer tools
- Launch MPI programs easily
- Much more
Singularity’s user guide is available at: sylabs.io/guides/3.8/user-guide
Link to section 'Example' of 'Singularity' Example
Here is an example using an Ubuntu 16.04 image on Bell:
singularity exec /depot/itap/singularity/ubuntu1604.img cat /etc/lsb-release
DISTRIB_ID=Ubuntu
DISTRIB_RELEASE=16.04
DISTRIB_CODENAME=xenial
DISTRIB_DESCRIPTION="Ubuntu 16.04 LTS"
Here is another example using a Centos 7 image:
singularity exec /depot/itap/singularity/centos7.img cat /etc/redhat-release
CentOS Linux release 7.2.1511 (Core)
Link to section 'Purdue Cluster Specific Notes' of 'Singularity' Purdue Cluster Specific Notes
All service providers will integrate Singularity slightly differently depending on site. The largest customization will be which default files are inserted into your images so that routine services will work.
Services we configure for your images include DNS settings and account information. File systems we overlay into your images are your home directory, scratch, Data Depot, and application file systems.
Here is a list of paths:
- /etc/resolv.conf
- /etc/hosts
- /home/$USER
- /apps
- /scratch
- /depot
This means that within the container environment these paths will be present and the same as outside the container. The /apps
, /scratch
, and /depot
directories will need to exist inside your container to work properly.
Link to section 'Creating Singularity Images' of 'Singularity' Creating Singularity Images
Due to how singularity containers work, you must have root privileges to build an image. Once you have a singularity container image built on your own system, you can copy the image file up to the cluster (you do not need root privileges to run the container).
You can find information and documentation for how to install and use singularity on your system:
We have version 3.8.0-1.el7
on the cluster. You will most likely not be able to run any container built with any singularity past that version. So be sure to follow the installation guide for version 3.8 on your system.
singularity --version
singularity version 3.8.0-1.el7
Everything you need on how to build a container is available from their user-guide. Below are merely some quick tips for getting your own containers built for Bell.
You can use a Definition File to both build your container and share its specification with collaborators (for the sake of reproducibility). Here is a simplistic example of such a file:
# FILENAME: Buildfile
Bootstrap: docker
From: ubuntu:18.04
%post
apt-get update && apt-get upgrade -y
mkdir /apps /depot /scratch
To build the image itself:
sudo singularity build ubuntu-18.04.sif Buildfile
The challenge with this approach however is that it must start from scratch if you decide to change something. In order to create a container image iteratively and interactively, you can use the --sandbox
option.
sudo singularity build --sandbox ubuntu-18.04 docker://ubuntu:18.04
This will not create a flat image file but a directory tree (i.e., a folder), the contents of which are the container's filesystem. In order to get a shell inside the container that allows you to modify it, user the --writable
option.
sudo singularity shell --writable ubuntu-18.04
Singularity: Invoking an interactive shell within container...
Singularity ubuntu-18.04.sandbox:~>
You can then proceed to install any libraries, software, etc. within the container. Then to create the final image file, exit
the shell and call the build
command once more on the sandbox.
sudo singularity build ubuntu-18.04.sif ubuntu-18.04
Finally, copy the new image to Bell and run it.
Windows
Windows virtual machines (VMs) are supported as batch jobs on HPC systems. This section illustrates how to submit a job and run a Windows instance in order to run Windows applications on the high-performance computing systems.
The following images are pre-configured and made available by staff:
- Windows 2016 Server Basic (minimal software pre-loaded)
- Windows 2016 Server GIS (GIS Software Stack pre-loaded)
The Windows VMs can be launched in two fashions:
- Menu Launcher - Point and click to start
- Command Line - Advanced and customized usage
Click each of the above links for detailed instructions on using them.
Link to section 'Software Provided in Pre-configured Virtual Machines' of 'Windows' Software Provided in Pre-configured Virtual Machines
The Windows 2016 Base server image available on Bell has the following software packages preloaded:
- Anaconda Python 2 and Python 3
- JMP 13
- Matlab R2017b
- Microsoft Office 2016
- Notepad++
- NVivo 12
- Rstudio
- Stata SE 15
- VLC Media Player
Menu Launcher
Windows VMs can be easily launched through the login/thinlinc">Thinlinc remote desktop environment.
- Log in via login/thinlinc">Thinlinc.
- Click on Applications menu in the upper left corner.
- Look under the Cluster Software menu.
- The "Windows 10" launcher will launch a VM directly on the front-end.
- Follow the dialogs to set up your VM.
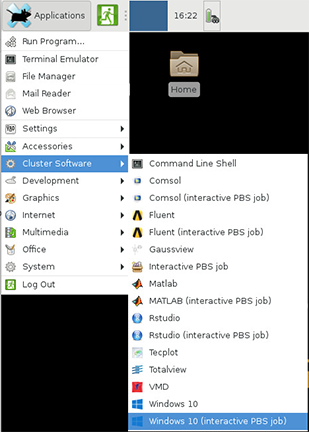
The dialog menus will walk you through setting up and loading your VM.
- You can choose to create a new image or load a saved image.
- New VMs should be saved on Scratch or Research Data Depot as they are too large for Home Directories.
- If you are using scratch, remember that scratch spaces are temporary, and be sure to safely back up your disk image somewhere permanent, such as Research Data Depot or Fortress.
You will also be prompted to select a storage space to mount on your image (Home, Scratch, or Data Depot). You can only choose one to be mounted. It will appear on a shortcut on the desktop once the VM loads.
Link to section 'Notes' of 'Menu Launcher' Notes
Using the menu launcher will launch automatically select reasonable CPU and memory values. If you wish to choose other options or work Windows VMs into scripted workflows see the section on using the command line.
Command line
If you wish to work with Windows VMs on the command line or work into scripted workflows you can interact directly with the Windows system:
Copy a Windows 2016 Server VM image to your storage. Scratch or Research Data Depot are good locations to save a VM image. If you are using scratch, remember that scratch spaces are temporary, and be sure to safely back up your disk image somewhere permanent, such as Research Data Depot or Fortress. To copy a basic image:
$ cp /apps/external/apps/windows/images/latest.qcow2 $RCAC_SCRATCH/windows.qcow2
To copy a GIS image:
$ cp /depot/itap/windows/gis/2k16.qcow2 $RCAC_SCRATCH/windows.qcow2
To launch a virtual machine in a batch job, use the "windows" script, specifying the path to your Windows virtual machine image. With no other command-line arguments, the windows script will autodetect a number cores and memory for the Windows VM. A Windows network connection will be made to your home directory. To launch:
$ windows -i $RCAC_SCRATCH/windows.qcow2
Link to section 'Command line options:' of 'Command line' Command line options:
-i <path to qcow image file> (For example, $RCAC_SCRATCH/windows-2k16.qcow2)
-m <RAM>G (For example, 32G)
-c <cores> (For example, 20)
-s <smbpath> (UNIX Path to map as a drive, for example, $RCAC_SCRATCH)
-b (If present, launches VM in background. Use VNC to connect to Windows.)
To launch a virtual machine with 32GB of RAM, 20 cores, and a network mapping to your home directory:
$ windows -i /path/to/image.qcow2 -m 32G -c 20 -s $HOME
To launch a virtual machine with 16GB of RAM, 10 cores, and a network mapping to your Data Depot space:
$ windows -i /path/to/image.qcow2 -m 16G -c 10 -s /depot/mylab
The Windows 2016 server desktop will open, and automatically log in as an administrator, so that you can install any software into the Windows virtual machine that your research requires. Changes to the image will be stored in the file specified with the -i option.
ROCm Containers Collection
Link to section 'What is ROCm Containers?' of 'ROCm Containers Collection' What is ROCm Containers?
The AMD Infinity Hub contains a collection of advanced AMD GPU software containers and deployment guides for HPC, AI & Machine Learning applications, enabling researchers to speed up their time to science. Containerized applications run quickly and reliably in the high performance computing environment with full support of AMD GPUs. A collection of Infinity Hub tools were deployed to extend cluster capabilities and to enable powerful software and deliver the fastest results. By utilizing Singularity and Infinity Hub ROCm-enabled containers, users can focus on building lean models, producing optimal solutions and gathering faster insights. For more information, please visit AMD Infinity Hub.
Link to section 'Getting Started' of 'ROCm Containers Collection' Getting Started
Users can download ROCm containers from the AMD Infinity Hub and run them directly using Singularity instructions from the corresponding container’s catalog page.
In addition, a subset of pre-downloaded ROCm containers wrapped into convenient software modules are provided. These modules wrap underlying complexity and provide the same commands that are expected from non-containerized versions of each application.
On Bell, type the command below to see the lists of ROCm containers we deployed.
module load rocmcontainers
module avail
------------ ROCm-based application container modules for AMD GPUs -------------
cp2k/20210311--h87ec1599
deepspeed/rocm4.2_ubuntu18.04_py3.6_pytorch_1.8.1
gromacs/2020.3 (D)
namd/2.15a2
openmm/7.4.2
pytorch/1.8.1-rocm4.2-ubuntu18.04-py3.6
pytorch/1.9.0-rocm4.2-ubuntu18.04-py3.6 (D)
specfem3d/20201122--h9c0626d1
specfem3d_globe/20210322--h1ee10977
tensorflow/2.5-rocm4.2-dev
[....]
Some of these modules use the container build-in MPI libraries (you may get some error messages like "Cannot load module because these module(s) are loaded: openmpi") and may require module unload openmpi
.
Link to section 'Examples of running ROCm-based containers on AMD GPUs' of 'ROCm Containers Collection' Examples of running ROCm-based containers on AMD GPUs
Examples below show how to run some containerized applications using rocmcontainers modules. In all cases, the general workflow follows the same pattern (load the rocmcontainers module; load specific application's module; run the application as if it was built natively). Additional information can be found in module help output and on each application's AMD Infinity Hub page.
Tensorflow
This example demonstrates how to run Tensorflow on AMD GPUs with rocmcontainers modules.
First, prepare the matrix multiplication example from Tensorflow documentation:
# filename: matrixmult.py
import tensorflow as tf
# Log device placement
print("Num GPUs Available: ", len(tf.config.list_physical_devices('GPU')))
tf.debugging.set_log_device_placement(True)
# Create some tensors
a = tf.constant([[1.0, 2.0, 3.0], [4.0, 5.0, 6.0]])
b = tf.constant([[1.0, 2.0], [3.0, 4.0], [5.0, 6.0]])
c = tf.matmul(a, b)
print(c)
Submit a Slurm job, making sure to request GPU-enabled queue and desired number of GPUs. For illustration purpose, the following example shows an interactive job submission, asking for one node (128 cores) in the "gpu" account with and two GPUs for 6 hours, but the same applies to your production batch jobs as well:
sinteractive -A gpu -N 1 -n 128 -t 6:00:00 --gres=gpu:2
salloc: Granted job allocation 5401130
salloc: Waiting for resource configuration
salloc: Nodes bell-g000 are ready for job
Inside the job, load necessary modules:
module load rocmcontainers
module load tensorflow/2.5-rocm4.2-dev
And run the application as usual:
python matrixmult.py
Num GPUs Available: 2
[...]
2021-09-02 21:07:34.087607: I tensorflow/core/common_runtime/gpu/gpu_device.cc:1418] Created TensorFlow device (/job:localhost/replica:0/task:0/device:GPU:0 with 32252 MB memory) -> physical GPU (device: 0, name: Vega 20, pci bus id: 0000:83:00.0)
[...]
2021-09-02 21:07:36.265167: I tensorflow/core/common_runtime/eager/execute.cc:733] Executing op MatMul in device /job:localhost/replica:0/task:0/device:GPU:0
2021-09-02 21:07:36.266755: I tensorflow/stream_executor/platform/default/dso_loader.cc:53] Successfully opened dynamic library librocblas.so
tf.Tensor(
[[22. 28.]
[49. 64.]], shape=(2, 2), dtype=float32)
For more information, see the application’s AMD Infinity Hub page. For applications deployed as modules, see module help command for a direct link to the relevant page (e.g. module help tensorflow/2.5-rocm4.2-dev in the above example).
BioContainers Collection
Link to section 'What is BioContainers?' of 'BioContainers Collection' What is BioContainers?
The BioContainers project came from the idea of using the containers-based technologies such as Docker or rkt for bioinformatics software. Having a common and controllable environment for running software could help to deal with some of the current problems during software development and distribution. BioContainers is a community-driven project that provides the infrastructure and basic guidelines to create, manage and distribute bioinformatics containers with a special focus on omics fields such as proteomics, genomics, transcriptomics and metabolomics. . For more information, please visit BioContainers project.
Link to section ' Getting Started ' of 'BioContainers Collection' Getting Started
Users can download bioinformatic containers from the BioContainers.pro and run them directly using Singularity instructions from the corresponding container’s catalog page.
Brief Singularity guide and examples are available at the Bell Singularity user guide page. Detailed Singularity user guide is available at: sylabs.io/guides/3.8/user-guide
In addition, a subset of pre-downloaded biocontainers wrapped into convenient software modules are provided. These modules wrap underlying complexity and provide the same commands that are expected from non-containerized versions of each application.
On Bell, type the command below to see the lists of biocontainers we deployed.
module load biocontainers
module avail
------------ BioContainers collection modules -------------
bamtools/2.5.1
beast2/2.6.3
bedtools/2.30.0
blast/2.11.0
bowtie2/2.4.2
bwa/0.7.17
cufflinks/2.2.1
deeptools/3.5.1
fastqc/0.11.9
faststructure/1.0
htseq/0.13.5
[....]
Link to section ' Example ' of 'BioContainers Collection' Example
This example demonstrates how to run BLASTP with the blast module. This blast module is a biocontainer wrapper for NCBI BLAST.
module load biocontainers
module load blast
blastp -query query.fasta -db nr -out output.txt -outfmt 6 -evalue 0.01
To run a job in batch mode, first prepare a job script that specifies the BioContainer modules you want to launch and the resources required to run it. Then, use the sbatch
command to submit your job script to Slurm. The following example shows the job script to use Bowtie2 in bioinformatic analysis.
#!/bin/bash
#SBATCH -A myqueuename
#SBATCH -o bowtie2_%j.txt
#SBATCH -e bowtie2_%j.err
#SBATCH --nodes=1
#SBATCH --ntasks-per-node=1
#SBATCH --cpus-per-task=8
#SBATCH --time=1:30:00
#SBATCH --job-name bowtie2
# Load the Bowtie module
module load biocontainers
module load bowtie2
# Indexing a reference genome
bowtie2-build ref.fasta ref
# Aligning paired-end reads
bowtie2 -p 8 -x ref -1 reads_1.fq -2 reads_2.fq -S align.sam
To help users get started, we provided detailed user guides for each containerized bioinformatics module on the ReadTheDocs platform
Ansys Fluent
Ansys is a CAE/multiphysics engineering simulation software that utilizes finite element analysis for numerically solving a wide variety of mechanical problems. The software contains a list of packages and can simulate many structural properties such as strength, toughness, elasticity, thermal expansion, fluid dynamics as well as acoustic and electromagnetic attributes.
Link to section 'Ansys Licensing' of 'Ansys Fluent' Ansys Licensing
The Ansys licensing on our community clusters is maintained by Purdue ECN group. There are two types of licenses: teaching and research. For more information, please refer to ECN Ansys licensing page. If you are interested in purchasing your own research license, please send email to software@ecn.purdue.edu.
Link to section 'Ansys Workflow' of 'Ansys Fluent' Ansys Workflow
Ansys software consists of several sub-packages such as Workbench and Fluent. Most simulations are performed using the Ansys Workbench console, a GUI interface to manage and edit the simulation workflow. It requires X11 forwarding for remote display so a SSH client software with X11 support or a remote desktop portal is required. Please see Logging In section for more details. To ensure preferred performance, ThinLinc remote desktop connection is highly recommended.
Typically users break down larger structures into small components in geometry with each of them modeled and tested individually. A user may start by defining the dimensions of an object, adding weight, pressure, temperature, and other physical properties.
Ansys Fluent is a computational fluid dynamics (CFD) simulation software known for its advanced physics modeling capabilities and accuracy. Fluent offers unparalleled analysis capabilities and provides all the tools needed to design and optimize new equipment and to troubleshoot existing installations.
In the following sections, we provide step-by-step instructions to lead you through the process of using Fluent. We will create a classical elbow pipe model and simulate the fluid dynamics when water flows through the pipe. The project files have been generated and can be downloaded via fluent_tutorial.zip.
Link to section 'Loading Ansys Module' of 'Ansys Fluent' Loading Ansys Module
Different versions of Ansys are installed on the clusters and can be listed with module spider
or module avail
command in the terminal.
$ module avail ansys/
---------------------- Core Applications -----------------------------
ansys/2019R3 ansys/2020R1 ansys/2021R2 ansys/2022R1 (D)
Before launching Ansys Workbench, a specific version of Ansys module needs to be loaded. For example, you can module load ansys/2021R2
to use the latest Ansys 2021R2. If no version is specified, the default module -> (D) (ansys/2022R1
in this case) will be loaded. You can also check the loaded modules with module list
command.
Link to section 'Launching Ansys Workbench' of 'Ansys Fluent' Launching Ansys Workbench
Open a terminal on Bell, enter rcac-runwb2
to launch Ansys Workbench.
You can also use runwb2
to launch Ansys Workbench. The main difference between runwb2
and rcac-runwb2
is that the latter sets the project folder to be in your scratch space. Ansys has an known bug that it might crash when the project folder is set to $HOME
on our systems.
Preparing Case Files for Fluent
Link to section 'Creating a Fluent fluid analysis system' of 'Preparing Case Files for Fluent' Creating a Fluent fluid analysis system
In the Ansys Workbench, create a new fluid flow analysis by double-clicking the Fluid Flow (Fluent) option under the Analysis Systems in the Toolbox on the left panel. You can also drag-and-drop the analysis system into the Project Schematic. A green dotted outline indicating a potential location for the new system initially appears in the Project Schematic. When you drag the system to one of the outlines, it turns into a red box to indicate the chosen location of the new system.
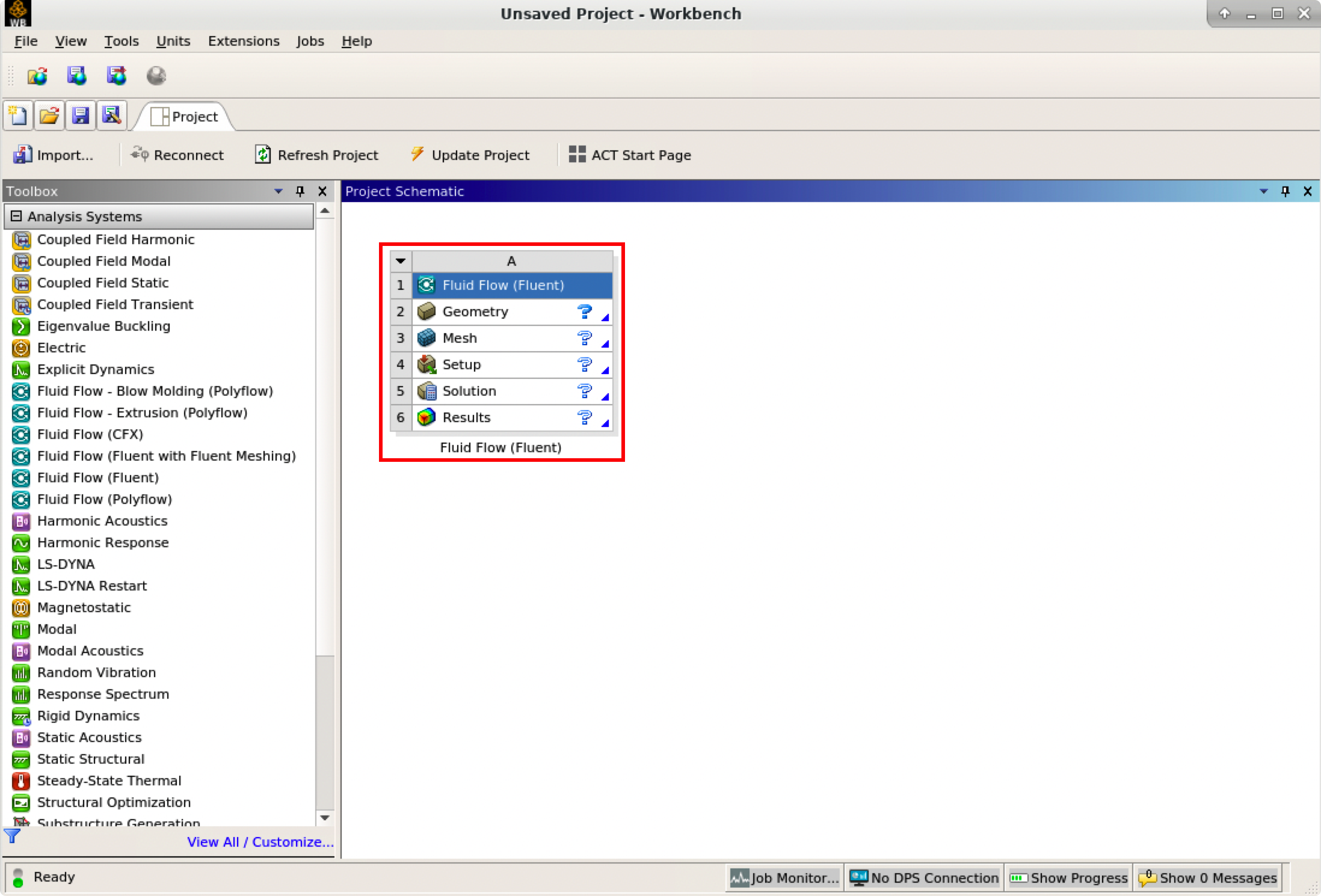
The red rectangle indicates the Fluid Flow system for Fluent, which includes all the essential workflows from “2 Geometry” to “6 Results”. You can rename it and carry out the necessary step-by-step procedures by double-clicking the corresponding cells.
It is important to save the project. Ansys Workbench saves the project with a .wbpj
extension and also all the supporting files into a folder with the same name. In this case, a file named elbow_demo.wbpj
and a folder $Ansys_PROJECT_FOLDER/elbow_demo_files/
are created in the Ansys project folder:
$ ll
total 33
drwxr-xr-x 7 myusername itap 9 Mar 3 17:47 elbow_demo_files
-rw-r--r-- 1 myusername itap 42597 Mar 3 17:47 elbow_demo.wbpj
You should always “Update Project” and save it after finishing a procedure.
Link to section 'Creating Geometry in the Ansys DesignModeler' of 'Preparing Case Files for Fluent' Creating Geometry in the Ansys DesignModeler
Create a geometry in the Ansys DesignModeler (by double-clicking “Geometry” cell in workflow), or import the appropriate geometry file (by right-clicking the Geometry cell and selecting “Import Geometry” option from the context menu).
You can use Ansys DesignModeler to create 2D/3D geometries or even draw the objects yourself. In our example, we created only half of the elbow pipe because the symmetry of the structure is taken into account to reduce the computation intensity.
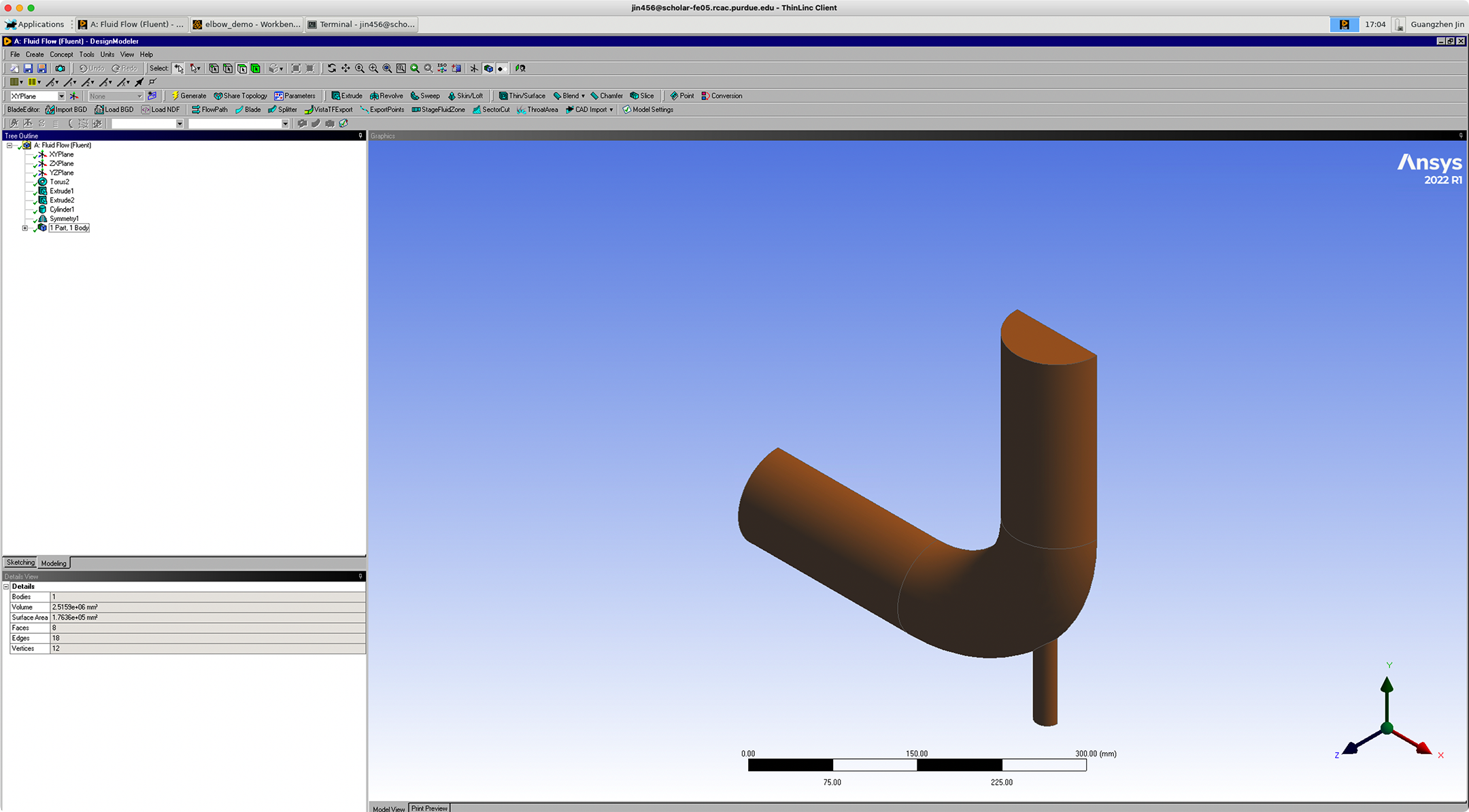
After saving the geometry, a geometry file FFF.agdb
will be created in the folder: $Ansys_PROJECT_FOLDER/elbow_demo_file/dp0/FFF/DM/
. The project in Workbench will be updated automatically.
If you import a pre-existing geometry into Ansys DesignModeler, it will also generate this file with the same filename at this location.
Link to section 'Creating mesh in the Ansys Meshing' of 'Preparing Case Files for Fluent' Creating mesh in the Ansys Meshing
Now that we have created the elbow pipe geometry, a computational mesh can be generated by the Meshing application throughout the flow volume.
With the successful creation of the geometry, there should be a green check showing the completion of “Geometry” in the Ansys Workbench. A Refresh Required icon within the “Mesh” cell indicates the mesh needs to be updated and refreshed for the system.
Then it’s time to open the Ansys Meshing application by double-clicking the “Mesh” cell and editing the mesh for the project. Generally, there are several steps we need to take to define the mesh:
- Create names for all geometry boundaries such as the inlets, outlets and fluid body. Note: You can use the strings “velocity inlet” and “pressure outlet” in the named selections (with or without hyphens or underscore characters) to allow Ansys Fluent to automatically detect and assign the corresponding boundary types accordingly. Use “Fluid” for the body to let Ansys Fluent automatically detect that the volume is a fluid zone and treat it accordingly.
- Set basic meshing parameters for the Ansys Meshing application. Here are several important parameters you may need to assign: Sizing, Quality, Body Sizing Control, Inflation.
- Select “Generate” to generate the mesh and “Update” to update the mesh into the system. Note: Once the mesh is generated, you can view the mesh statistics by opening the Statistics node in the Details of “Mesh” view. This will display information such as the number of nodes and the number of elements, which gives you a general idea for the future computational resources and time.
After generation and updating the mesh, a mesh file FFF.msh
will be generated in folder $Ansys_PROJECT_FOLDER/elbow_demo_file/dp0/FFF/MECH/
and a mesh database file FFF.mshdb
will be generated in folder $Ansys_PROJECT_FOLDER/elbow_demo_file/dp0/global/MECH/
.
Parameters used in demo case (use default if not assigned):
- Length Unit=”mm”
- Names defined for geometry:
- velocity-inlet-large (large inlet on pipe);
- velocity-inlet-small (small inlet on pipe);
- pressure-outlet (outlet on pipe);
- symmetry (symmetry surface);
- Fluid (body);
- Mesh:
- Quality: Smoothing=”high”;
- Inflation: Use Automatic Inflation=“Program Controlled”, Inflation Option=”Smooth Transition”;
- Statistics:
- Nodes=29371;
- Elements=87647.
Link to section 'Calculation with Fluent' of 'Preparing Case Files for Fluent' Calculation with Fluent
Now all the preparations have been ready for the numerical calculation in Ansys Fluent. Both “Geometry” and “Mesh” cells should have green checks on. We can set up the CFD simulation parameters in Ansys Fluent by double-clicking the “Setup” cell.
When Ansys Fluent is first started or by selecting “editing” on the “Setup” cell, the Fluent Launcher is displayed, enabling you to view and/or set certain Ansys Fluent start-up options (e.g. Precision, Parallel, Display). Note that “Dimension” is fixed to “3D” because we are using a 3D model in this project.
After the Fluent is opened, an Ansys Fluent settings file FFF.set
is written under the folder $Ansys_PROJECT_FOLDER/elbow_demo_file/dp0/FFF/Fluent/
.
Then we are going to set up all the necessary parameters for Fluent computation. Here are the key steps for the setup:
- Setting up the domain:
- Change the units for length to be consistent with the Mesh;
- Check the mesh statistics and quality;
- Setting up physics:
- Solver: “Energy”, “Viscous Model”, “Near-Wall Treatment”;
- Materials;
- Zones;
- Boundaries: Inlet, Outlet, Internal, Symmetry, Wall;
- Solving:
- Solution Methods;
- Reports;
- Initialization;
- Iterations and output frequency.
Then the calculation will be carried out and the results will be written out into FFF-1.cas.gz
under folder $Ansys_PROJECT_FOLDER/elbow_demo_file/dp0/FFF/Fluent/
.
This file contains all the settings and simulation results which can be loaded for post analysis and re-computation (more details will be introduced in the following sections). If only configurations and settings within the Fluent are needed, we can open independent Fluent or submit Fluent jobs with bash commands by loading the existing case in order to facilitate the computation process.
Parameters used in demo case (use default if not assigned):
- Domain Setup: Length Units=”mm”;
- Solver: Energy=”on”; Viscous Model=”k-epsilon”; Near-Wall Treatment=”Enhanced Wall Treatment”;
- Materials: water (Density=1000[kg/m^3]; Specific Heat=4216[J/kg-k]; Thermal Conductivity=0.677[w/m-k]; Viscosity=8e-4[kg/m-s]);
- Zones=”fluid (water)”;
- Inlet=”velocity-inlet-large” (Velocity Magnitude=0.4m/s, Specification Method=”Intensity and Hydraulic Diameter”, Turbulent Intensity=5%; Hydraulic Diameter=100mm; Thermal Temperature=293.15k) &”velocity-inlet-small” (Velocity Magnitude=1.2m/s, Specification Method=”Intensity and Hydraulic Diameter”, Turbulent Intensity=5%; Hydraulic Diameter=25mm; Thermal Temperature=313.15k); Internal=”interior-fluid”; Symmetry=”symmetry”; Wall=”wall-fluid”;
- Solution Methods: Gradient=”Green-Gauss Node Based”;
- Report: plot residual and “Facet Maximum” for “pressure-outlet”
- Hybrid Initialization;
- 300 iterations.
Case Calculating with Fluent
Link to section 'Calculation with Fluent' of 'Case Calculating with Fluent' Calculation with Fluent
Now all the files are ready for the Fluent calculations. Both “Geometry” and “Mesh” cells should have green checks. We can set up the CFD simulation parameters in the Ansys Fluent by double-clicking the “Setup” cell.
Ansys Fluent Launcher can be started by selecting “editing” on the “Setup” cell with many startup options (e.g. Precision, Parallel, Display). Note that “Dimension” is fixed to “3D” because we are using a 3D model in this project.
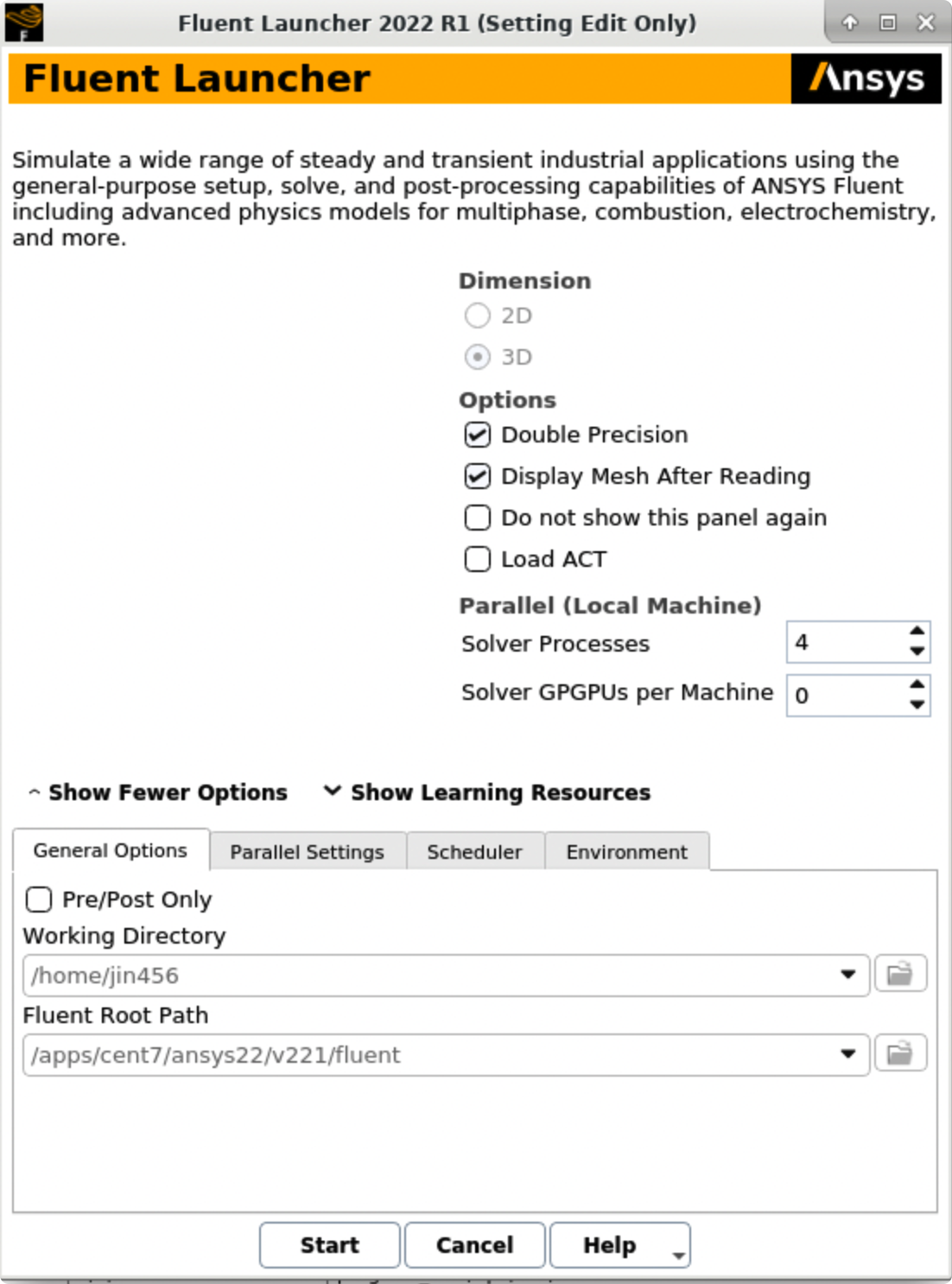
After the Fluent is opened, an Ansys Fluent settings file FFF.set
is written under the folder $Ansys_PROJECT_FOLDER/elbow_demo_file/dp0/FFF/Fluent/
.
Then we are going to set up all the necessary parameters for Fluent computation. Here are the key steps for the setup:
- Setting up the domain:
- Change the units for length to be consistent with the Mesh;
- Check the mesh statistics and quality;
- Setting up physics:
- Solver: “Energy”, “Viscous Model”, “Near-Wall Treatment”;
- Materials;
- Zones;
- Boundaries: Inlet, Outlet, Internal, Symmetry, Wall;
- Solving:
- Solution Methods;
- Reports;
- Initialization;
- Iterations and output frequency.
Then the calculation will be carried out and the results will be written out into FFF-1.cas.gz
under folder $Ansys_PROJECT_FOLDER/elbow_demo_file/dp0/FFF/Fluent/
.
This file contains all the settings and simulation results which can be loaded for post analysis and re-computation (more details will be introduced in the following sections). If only configurations and settings within the Fluent are needed, we can open independent Fluent or submit Fluent jobs with bash commands by loading the existing case in order to facilitate the computation process.
Parameters used in demo case (use default if not assigned):
- Domain Setup: Length Units=”mm”;
- Solver: Energy=”on”; Viscous Model=”k-epsilon”; Near-Wall Treatment=”Enhanced Wall Treatment”;
- Materials: water (Density=1000[kg/m^3]; Specific Heat=4216[J/kg-k]; Thermal Conductivity=0.677[w/m-k]; Viscosity=8e-4[kg/m-s]);
- Zones=”fluid (water)”;
- Inlet=”velocity-inlet-large” (Velocity Magnitude=0.4m/s, Specification Method=”Intensity and Hydraulic Diameter”, Turbulent Intensity=5%; Hydraulic Diameter=100mm; Thermal Temperature=293.15k) &”velocity-inlet-small” (Velocity Magnitude=1.2m/s, Specification Method=”Intensity and Hydraulic Diameter”, Turbulent Intensity=5%; Hydraulic Diameter=25mm; Thermal Temperature=313.15k); Internal=”interior-fluid”; Symmetry=”symmetry”; Wall=”wall-fluid”;
- Solution Methods: Gradient=”Green-Gauss Node Based”;
- Report: plot residual and “Facet Maximum” for “pressure-outlet”
- Hybrid Initialization;
- 300 iterations.
Link to section 'Results analysis' of 'Case Calculating with Fluent' Results analysis
The best methods to view and analyze the simulation should be the Ansys Fluent (directly after computation) or the Ansys CFD-Post (entering “Results” in Ansys Workbench). Both methods are straightforward so we will not cover this part in this tutorial. Here is a final simulation result showing the temperature of the symmetry after 300 iterations for reference:
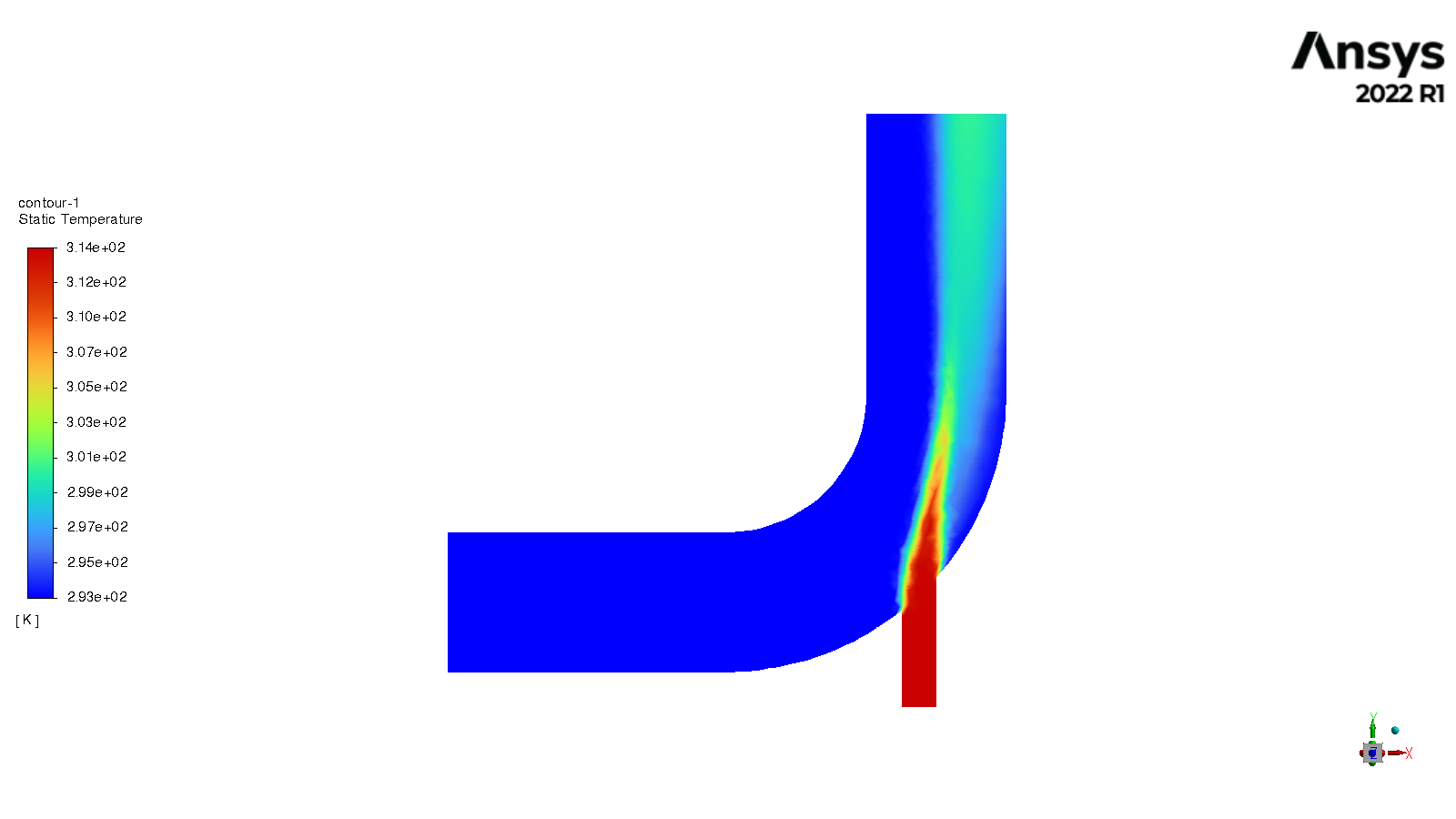
Fluent Text User Interface and Journal File
Link to section 'Fluent Text User Interface (TUI)' of 'Fluent Text User Interface and Journal File' Fluent Text User Interface (TUI)
If you pay attention to the “Console” window in the Fluent window when setting up and carrying out the calculation, corresponding commands can be found and executed one after another. Almost all the setting processes can be accomplished by the command lines, which is called Fluent Text User Interface (TUI). Here are the main commands in Fluent TUI:
adjoint/ parallel/ solve/
define/ plot/ surface/
display/ preferences/ turbo-workflow/
exit print-license-usage views/
file/ report/
mesh/ server/
For example, instead of opening a case by clicking buttons in Ansys Fluent, we can type /file read-case case_file_name.cas.gz
to open the saved case.
Link to section 'Fluent Journal Files' of 'Fluent Text User Interface and Journal File' Fluent Journal Files
A Fluent journal file is a series of TUI commands stored in a text file. The file can be written in a text editor or generated by Fluent as a transcript of the commands given to Fluent during your session.
A journal file generated by Fluent will include any GUI operations (in a TUI form, though). This is quite useful if you have a series of tasks that you need to execute, as it provides a shortcut. To record a journal file, start recording with File -> Write -> Start Journal..., perform whatever tasks you need, and then stop recording with File -> Write -> Stop Journal...
You can also write your own journal file into a text file. The basic rule for a Fluent journal file is to reproduce the TUI commands that controlled the configuration and calculation of Fluent in their order. You can add a comment in a line starting with a ;
(semicolon).
Here are some reasons why you should use a Fluent journal file:
- Using journal files with bash scripting can allow you to automate your jobs.
- Using journal files can allow you to parameterize your models easily and automatically.
- Using a journal file can set parameters you do not have in your case file e.g. autosaving.
- Using a journal file can allow you to safely save, stop and restart your jobs easily.
The order of your journal file commands is highly important. The correct sequences must be followed and some stages have multiple options e.g. different initialization methods.
Here is a sample Fluent journal file for the demo case:
;testJournal.jou
;Set the TUI version for Fluent
/file/set-tui-version "22.1"
;Read the case. The default folder
/file read-case /home/jin456/Fluent_files/tutorial_case1/elbow_files/dp0/FFF/Fluent/FFF-1.cas.gz
;Initialize the case with Hybrid Initialization
/solve/initialize/hyb-initialization
;Set Number of Iterations to 1000, Reporting Interval to 10 iterations and Profile Update Interval to 1 iteration
/solve/iterate 1000 10 1
;Outputting solver performance data upon completion of the simulation
/parallel timer usage
;Write out the simulation results.
/file write-case-data /home/jin456/Fluent_files/tutorial_case1/elbow_files/dp0/FFF/Fluent/result.cas.h5
;After computation, exit Flent
/exit
Before running this Fluent journal file, you need to make sure: 1) the ansys module has been loaded (it’s highly recommended to load the same version of Ansys when you built the case project); 2) the project case file (***.cas.gz
) has been created.
Then we can use Fluent to run this journal file by simply using:fluent 3ddp -t$NTASKS -g -i testJournal.jou
in the terminal. Here, 3d
indicates this is a 3d model, dp
indicates double precision, -t$NTASKS
tells Fluent how many Solver Processes it will take (e.g. -t4
), -g
means to run without the GUI or graphics, -i
testJournal.jou tells Fluent to read the specific journal file.
Here is a table for the available command line Options for Linux/UNIX and Windows Platforms in Ansys Fluent.
Option | Platform | Description |
---|---|---|
-cc |
all | Use the classic color scheme |
-ccp x |
Windows only | Use the Microsoft Job Scheduler where x is the head node name. |
-cnf=x |
all | Specify the hosts or machine list file |
-driver |
all | Sets the graphics driver (available drivers vary by platform - opengl or x11 or null(Linux/UNIX) - opengl or msw or null (Windows)) |
-env |
all | Show environment variables |
-fgw |
all | Disables the embedded graphics |
-g |
all | Run without the GUI or graphics (Linux/UNIX); Run with the GUI minimized (Windows) |
-gr |
all | Run without graphics |
-gu |
all | Run without the GUI but with graphics (Linux/UNIX); Run with the GUI minimized but with graphics (Windows) |
-help |
all | Display command line options |
-hidden |
Windows only | Run in batch mode |
-host_ip=host:ip |
all | Specify the IP interface to be used by the host process |
-i journal |
all | Reads the specified journal file |
-lsf |
Linux/UNIX only | Run FLUENT using LSF |
-mpi= |
all | Specify MPI implementation |
-mpitest |
all | Will launch an MPI program to collect network performance data |
-nm |
all | Do not display mesh after reading |
-pcheck |
Linux/UNIX only | Checks all nodes |
-post |
all | Run the FLUENT post-processing-only executable |
-p |
all | Choose the interconnect |
-r |
all | List all releases installed |
-rx |
all | Specify release number |
-sge |
Linux/UNIX only | Run FLUENT under Sun Grid Engine |
-sge queue |
Linux/UNIX only | Name of the queue for a given computing grid |
-sgeckpt ckpt_obj |
Linux/UNIX only | Set checkpointing object to ckpt_objfor SGE |
-sgepe fluent_pe min_n-max_n |
Linux/UNIX only | Set the parallel environment for SGE to fluent_pe, min_nand max_n are number of min and max nodes requested |
-tx |
all | Specify the number of processors x |
For more information for Fluent text user interface and journal files, please refer to Fluent FAQ.
Submitting Fluent jobs to SLURM
The Fluent simulations can also run in batch. In this section we provide an example script for submitting Fluent jobs to the SLURM scheduler. Please refer to the Running Jobs section of our user guide for detailed tutorials of submitting jobs.
#!/bin/bash
# Job script for submitting a FLUENT job on multiple cores on a single node
# Apply resources via SLURM
#SBATCH --nodes=1
#SBATCH --ntasks=4
#SBATCH --time=01:00:00
#SBATCH --job-name=fluent_test
#SBATCH -o fluent_test_%j.out
#SBATCH -e fluent_test_%j.err
# Loads Ansys and sets the application up
module purge
module load ansys/2022R1
#Initiating Fluent and reading input journal file
fluent 3ddp -t$NTASKS -g -i testJournal.jou
For more information about submitting Fluent jobs, please refer to Fluent FAQ .
Frequently Asked Questions
Some common questions, errors, and problems are categorized below. Click the Expand Topics link in the upper right to see all entries at once. You can also use the search box above to search the user guide for any issues you are seeing.
About Bell
Frequently asked questions about Bell.
Can you remove me from the Bell mailing list?
Your subscription in the Bell mailing list is tied to your account on Bell. If you are no longer using your account on Bell, your account can be deleted from the My Accounts page. Hover over the resource you wish to remove yourself from and click the red 'X' button. Your account and mailing list subscription will be removed overnight. Be sure to make a copy of any data you wish to keep first.
How is Bell different than other Community Clusters?
Bell differs from the previous Community Clusters in several significant aspects:
- Bell home directories are entirely separate from other Community Clusters home directories. There is no automatic copying or synchronization between the two. At their discretion, users can copy parts or all of the Community Clusters home directory into Bell - instructions are provided.
- Users of
hsi
andhtar
commands may encounter Fortress keytab- and authentication-related error messages due to the dedicated nature of Bell home directories. A temporary workaround is provided while a permanent solution is being developed. - Bell contains the latest generation of AMD EPYC processors, codenamed "Rome". These CPUs support AVX2 vector instructions set. When compiling your code, use of
-march=znver2
flag (for latest GCC, Clang and AOCC compilers) or-march=core-avx2
(for Intel compilers and GCC prior to 9.3) is recommended. - If your application heavily uses Intel MKL routines, setting the following environment variable is beneficial:
export MKL_DEBUG_CPU_TYPE=5
When using FFTW interface from MKL, please also set:
export MKL_CBWR=AUTO
- If you use Jupyter notebooks, JupyterHub on Bell will only be available via the OnDemand Gateway rather than the freestanding version as on previous systems. Other RCAC systems will transition to OnDemand as well, following Bell.
- A subset of Bell compute nodes contain AMD Radeon Instinct MI50 accelerator cards which can significantly improve performance of compute-intensive workloads. These can be utilized by submitting jobs to the gpu queue (add -A gpu to your job submission command).
- A selection of GPU-enabled ROCm application containers from the AMD InfinityHub collection is installed.
Do I need to do anything to my firewall to access Bell?
No firewall changes are needed to access Bell. However, to access data through Network Drives (i.e., CIFS, "Z: Drive"), you must be on a Purdue campus network or connected through VPN.
Does Bell have the same home directory as other clusters?
The Bell home directory and its contents are exclusive to Bell cluster front-end hosts and compute nodes. This home directory is not available on other RCAC machines but Bell. There is no automatic copying or synchronization between home directories.
At your discretion you can manually copy all or parts of your main research computing home to Bell using one of the suggested methods.
If you plan to use hsi
or htar
commands to access Fortress tape archive from Bell, please see also the keytab generation question for a temporary workaround to a potential caveat, while a permanent mitigation is being developed.
Logging In & Accounts
Frequently asked questions about logging in & accounts.
Errors
Common errors and solutions/work-arounds for them.
/usr/bin/xauth: error in locking authority file
Link to section 'Problem' of '/usr/bin/xauth: error in locking authority file' Problem
I receive this message when logging in:
/usr/bin/xauth: error in locking authority file
Link to section 'Solution' of '/usr/bin/xauth: error in locking authority file' Solution
Your home directory disk quota is full. You may check your quota with myquota
.
You will need to free up space in your home directory.
ncdu
command is a convenient interactive tool to examine disk usage. Consider running ncdu $HOME
to analyze where the bulk of the usage is. With this knowledge, you could then archive your data elsewhere (e.g. your research group's Data Depot space, or Fortress tape archive), or delete files you no longer need.
There are several common locations that tend to grow large over time and are merely cached downloads. The following are safe to delete if you see them in the output of ncdu $HOME
:
/home/myusername/.local/share/Trash
/home/myusername/.cache/pip
/home/myusername/.conda/pkgs
/home/myusername/.singularity/cache
My SSH connection hangs
Link to section 'Problem' of 'My SSH connection hangs' Problem
Your console hangs while trying to connect to a RCAC Server.
Link to section 'Solution' of 'My SSH connection hangs' Solution
This can happen due to various reasons. Most common reasons for hanging SSH terminals are:
- Network: If you are connected over wifi, make sure that your Internet connection is fine.
- Busy front-end server: When you connect to a cluster, you SSH to one of the front-end login nodes. Due to transient user loads, one or more of the front-ends may become unresponsive for a short while. To avoid this, try reconnecting to the cluster or wait until the login node you have connected to has reduced load.
- File system issue: If a server has issues with one or more of the file systems (
home
,scratch
, ordepot
) it may freeze your terminal. To avoid this you can connect to another front-end.
If neither of the suggestions above work, please contact support specifying the name of the server where your console is hung.
Thinlinc session frozen
Link to section 'Problem' of 'Thinlinc session frozen' Problem
Your Thinlinc session is frozen and you can not launch any commands or close the session.
Link to section 'Solution' of 'Thinlinc session frozen' Solution
This can happen due to various reasons. The most common reason is that you ran something memory-intensive inside that Thinlinc session on a front-end, so parts of the Thinlinc session got killed by Cgroups, and the entire session got stuck.
- If you are using a web-version Thinlinc remote desktop (inside the browser):
The web version does not have the capability to kill the existing session, only the standalone client does. Please install the standalone client and follow the steps below:
- If you are using a Thinlinc client:
Close the ThinLinc client, reopen the client login popup, and select
End existing session
.Select "End existing session" and try "Connect" again.
Thinlinc session unreachable
Link to section 'Problem' of 'Thinlinc session unreachable' Problem
When trying to login to Thinlinc and re-connect to your existing session, you receive an error "Your Thinlinc session is currently unreachable".
Link to section 'Solution' of 'Thinlinc session unreachable' Solution
This can happen if the specific login node your existing remote desktop session was residing on is currently offline or down, so Thinlinc can not reconnect to your existing session. Most often the session is non-recoverable at this point, so the solution is to terminate your existing Thinlinc desktop session and start a new one.
- If you are using a web-version Thinlinc remote desktop (inside the browser):
The web version does not have the capability to kill the existing session, only the standalone client does. Please install the standalone client and follow the steps below:
- If you are using a Thinlinc client:
Close the ThinLinc client, reopen the client login popup, and select
End existing session
.Select "End existing session" and try "Connect" again.
How to disable Thinlinc screensaver
Link to section 'Problem' of 'How to disable Thinlinc screensaver' Problem
Your ThinLinc desktop is locked after being idle for a while, and it asks for a password to refresh it. It means the "screensaver" and "lock screen" functions are turned on, but you want to disable these functions.
Link to section 'Solution' of 'How to disable Thinlinc screensaver' Solution
If your screen is locked, close the ThinLinc client, reopen the client login popup, and select End existing session
.
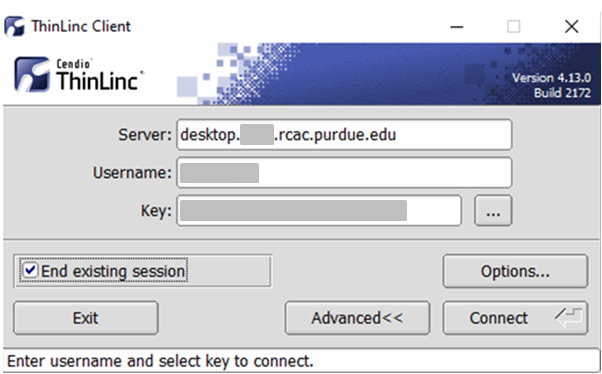
To permanently avoid screen lock issue, right click desktop and select Applications
, then settings
, and select Screensaver
.
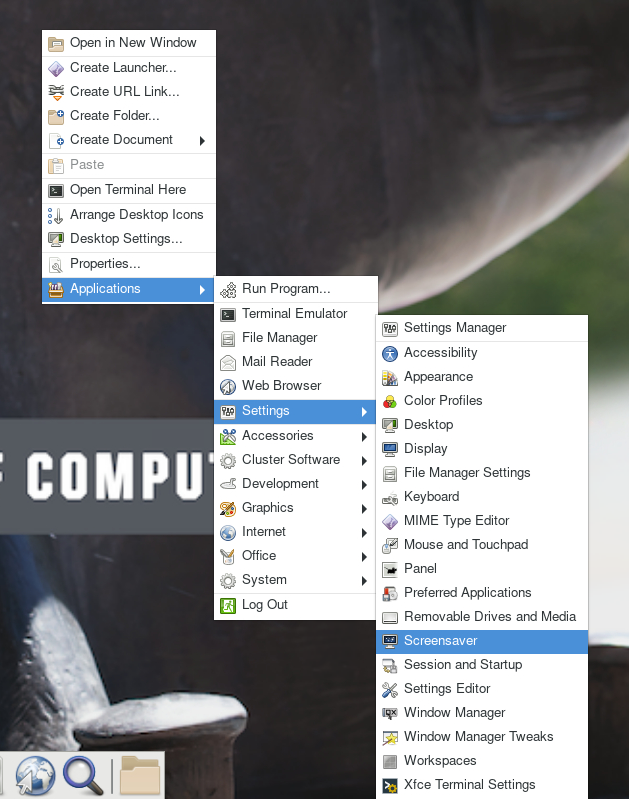
Under Screensaver, turn off the Enable Screensaver
, then under Lock Screen, turn off the Enable Lock Screen
, and close the window.
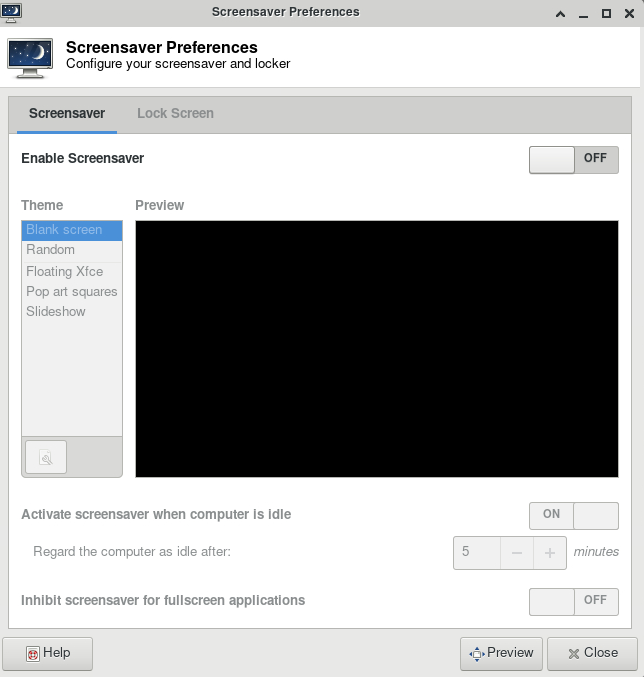
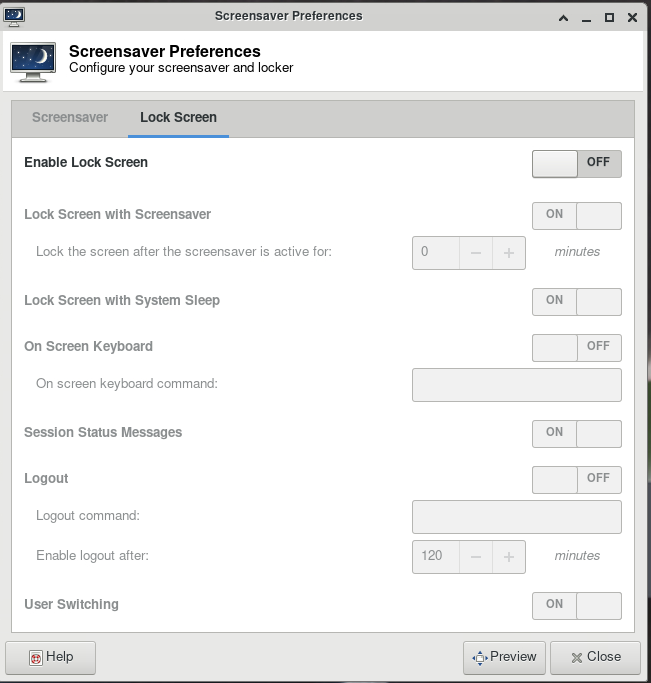
Questions
Frequently asked questions about logging in & accounts.
I worked on Bell after I graduated/left Purdue, but can not access it anymore
Link to section 'Problem' of 'I worked on Bell after I graduated/left Purdue, but can not access it anymore' Problem
You have graduated or left Purdue but continue collaboration with your Purdue colleagues. You find that your access to Purdue resources has suddenly stopped and your password is no longer accepted.
Link to section 'Solution' of 'I worked on Bell after I graduated/left Purdue, but can not access it anymore' Solution
Access to all resources depends on having a valid Purdue Career Account. Expired Career Accounts are removed twice a year, during Spring and October breaks (more details at the official page). If your Career Account was purged due to expiration, you will not be be able to access the resources.
To provide remote collaborators with valid Purdue credentials, the University provides a special procedure called Request for Privileges (R4P). If you need to continue your collaboration with your Purdue PI, the PI will have to submit or renew an R4P request on your behalf.
After your R4P is completed and Career Account is restored, please note two additional necessary steps:
-
Access: Restored Career Accounts by default do not have any RCAC resources enabled for them. Your PI will have to login to the Manage Users tool and explicitly re-enable your access by un-checking and then ticking back checkboxes for desired queues/Unix groups resources.
-
Email: Restored Career Accounts by default do not have their @purdue.edu email service enabled. While this does not preclude you from using RCAC resources, any email messages (be that generated on the clusters, or any service announcements) would not be delivered - which may cause inconvenience or loss of compute jobs. To avoid this, we recommend setting your restored @purdue.edu email service to "Forward" (to an actual address you read). The easiest way to ensure it is to go through the Account Setup process.
Jobs
Frequently asked questions related to running jobs.
Errors
Common errors and potential solutions/workarounds for them.
cannot connect to X server / cannot open display
Link to section 'Problem' of 'cannot connect to X server / cannot open display' Problem
You receive the following message after entering a command to bring up a graphical window
cannot connect to X server
cannot open display
Link to section 'Solution' of 'cannot connect to X server / cannot open display' Solution
This can happen due to multiple reasons:
- Reason: Your SSH client software does not support graphical display by itself (e.g. SecureCRT or PuTTY).
- Solution: Try using a client software like Thinlinc or MobaXterm as described in the SSH X11 Forwarding guide.
-
Reason: You did not enable X11 forwarding in your SSH connection.
-
Solution: If you are in a Windows environment, make sure that X11 forwarding is enabled in your connection settings (e.g. in MobaXterm or PuTTY). If you are in a Linux environment, try
ssh -Y -l username hostname
-
- Reason: If you are trying to open a graphical window within an interactive PBS job, make sure you are using the
-X
option withqsub
after following the previous step(s) for connecting to the front-end. Please see the example in the Interactive Jobs guide. - Reason: If none of the above apply, make sure that you are within quota of your home directory.
bash: command not found
Link to section 'Problem' of 'bash: command not found' Problem
You receive the following message after typing a command
bash: command not found
Link to section 'Solution' of 'bash: command not found' Solution
This means the system doesn't know how to find your command. Typically, you need to load a module to do it.
bash: module command not found
Link to section 'Problem' of 'bash: module command not found' Problem
You receive the following message after typing a command, e.g. module load intel
bash: module command not found
Link to section 'Solution' of 'bash: module command not found' Solution
The system cannot find the module command. You need to source the modules.sh file as below
source /etc/profile.d/modules.sh
or
#!/bin/bash -i
Close Firefox / Firefox is already running but not responding
Link to section 'Problem' of 'Close Firefox / Firefox is already running but not responding' Problem
You receive the following message after trying to launch Firefox browser inside your graphics desktop:
Close Firefox
Firefox is already running, but not responding. To open a new window,
you must first close the existing Firefox process, or restart your system.
Link to section 'Solution' of 'Close Firefox / Firefox is already running but not responding' Solution
When Firefox runs, it creates several lock files in the Firefox profile directory (inside ~/.mozilla/firefox/
folder in your home directory). If a newly-started Firefox instance detects the presence of these lock files, it complains.
This error can happen due to multiple reasons:
- Reason: You had a single Firefox process running, but it terminated abruptly without a chance to clean its lock files (e.g. the job got terminated, session ended, node crashed or rebooted, etc).
- Solution: If you are certain you do not have any other Firefox processes running elsewhere, please use the following command in a terminal window to detect and remove the lock files:
$ unlock-firefox
- Solution: If you are certain you do not have any other Firefox processes running elsewhere, please use the following command in a terminal window to detect and remove the lock files:
- Reason: You may indeed have another Firefox process (in another Thinlinc or Gateway session on this or other cluster, another front-end or compute node). With many clusters sharing common home directory, a running Firefox instance on one can affect another.
- Solution: Try finding and closing running Firefox process(es) on other nodes and clusters.
- Solution: If you must have multiple Firefoxes running simultaneously, you may be able to create separate Firefox profiles and select which one to use for each instance.
Jupyter: database is locked / can not load notebook format
Link to section 'Problem' of 'Jupyter: database is locked / can not load notebook format' Problem
You receive the following message after trying to load existing Jupyter notebooks inside your JupyterHub session:
Error loading notebook
An unknown error occurred while loading this notebook. This version can load notebook formats or earlier. See the server log for details.
Alternatively, the notebook may open but present an error when creating or saving a notebook:
Autosave Failed!
Unexpected error while saving file: MyNotebookName.ipynb database is locked
Link to section 'Solution' of 'Jupyter: database is locked / can not load notebook format' Solution
When Jupyter notebooks are opened, the server keeps track of their state in an internal database (located inside ~/.local/share/jupyter/
folder in your home directory). If a Jupyter process gets terminated abruptly (e.g. due to an out-of-memory error or a host reboot), the database lock is not cleared properly, and future instances of Jupyter detect the lock and complain.
Please follow these steps to resolve:
- Fully exit from your existing Jupyter session (close all notebooks, terminate Jupyter, log out from JupyterHub or JupyterLab, terminate OnDemand gateway's Jupyter app, etc).
- In a terminal window (SSH, Thinlinc or OnDemand gateway's terminal app) use the following command to clean up stale database locks:
$ unlock-jupyter
- Start a new Jupyter session as usual.
Questions
Frequently asked questions about jobs.
How do I know Non-uniform Memory Access (NUMA) layout on Bell?
- You can learn about processor layout on Bell nodes using the following command:
bell-a003:~$ lstopo-no-graphics
- For detailed IO connectivity:
bell-a003:~$ lstopo-no-graphics --physical --whole-io
- Please note that NUMA information is useful for advanced MPI/OpenMP/GPU optimizations. For most users, using default NUMA settings in MPI or OpenMP would give you the best performance.
Why cannot I use --mem=0 when submitting jobs?
Link to section 'Question' of 'Why cannot I use --mem=0 when submitting jobs?' Question
Why can't I specify --mem=0
for my job?
Link to section 'Answer' of 'Why cannot I use --mem=0 when submitting jobs?' Answer
We no longer support requesting unlimited memory (--mem=0
) as it has an adverse effect on the way scheduler allocates job, and could lead to large amount of nodes being blocked from usage.
Most often we suggest relying on default memory allocation (cluster-specific). But if you have to request custom amounts of memory, you can do it explicitly. For example --mem=20G
.
If you want to use the entire node's memory, you can submit the job with the --exclusive
option.
Can I extend the walltime on a job?
In some circumstances, yes. Walltime extensions must be requested of and completed by staff. Walltime extension requests will be considered on named (your advisor or research lab) queues. Standby or debug queue jobs cannot be extended.
Extension requests are at the discretion of staff based on factors such as any upcoming maintenance or resource availability. Extensions can be made past the normal maximum walltime on named queues but these jobs are subject to early termination should a conflicting maintenance downtime be scheduled.
Please be mindful of time remaining on your job when making requests and make requests at least 24 hours before the end of your job AND during business hours. We cannot guarantee jobs will be extended in time with less than 24 hours notice, after-hours, during weekends, or on a holiday.
We ask that you make accurate walltime requests during job submissions. Accurate walltimes will allow the job scheduler to efficiently and quickly schedule jobs on the cluster. Please consider that extensions can impact scheduling efficiency for all users of the cluster.
Requests can be made by contacting support. We ask that you:
- Provide numerical job IDs, cluster name, and your desired extension amount.
- Provide at least 24 hours notice before job will end (more if request is made on a weekend or holiday).
- Consider making requests during business hours. We may not be able to respond in time to requests made after-hours, on a weekend, or on a holiday.
Data
Frequently asked questions about data and data management.
How is my Data Secured on Bell?
Bell is operated in line with policies, standards, and best practices as described within Secure Purdue, and specific to RCAC Resources.
Security controls for Bell are based on ones defined in NIST cybersecurity standards.
Bell supports research at the L1 fundamental and L2 sensitive levels. Bell is not approved for storing data at the L3 restricted (covered by HIPAA) or L4 Export Controlled (ITAR), or any Controlled Unclassified Information (CUI).
For resources designed to support research with heightened security requirements, please look for resources within the REED+ Ecosystem.
Link to section 'For additional information' of 'How is my Data Secured on Bell?' For additional information
Log in with your Purdue Career Account.
Does Bell have the same home directory as other clusters?
The Bell home directory and its contents are exclusive to Bell cluster front-end hosts and compute nodes. This home directory is not available on other RCAC machines but Bell. There is no automatic copying or synchronization between home directories.
At your discretion you can manually copy all or parts of your main research computing home to Bell using one of the suggested methods.
If you plan to use hsi
or htar
commands to access Fortress tape archive from Bell, please see also the keytab generation question for a temporary workaround to a potential caveat, while a permanent mitigation is being developed.
Can I share data with outside collaborators?
Yes! Globus allows convenient sharing of data with outside collaborators. Data can be shared with collaborators' personal computers or directly with many other computing resources at other institutions. See the Globus documentation on how to share data:
HSI/HTAR: Unable to authenticate user with remote gateway (error 2 or 9)
There could be a variety of such errors, with wordings along the lines of
Could not initialize keytab on remote server.
result = -2, errno = 2rver connection
*** hpssex_OpenConnection: Unable to authenticate user with remote gateway at 128.211.138.40.1217result = -2, errno = 9
Unable to setup communication to HPSS...
ERROR (main) unable to open remote gateway server connection
HTAR: HTAR FAILED
and
*** hpssex_OpenConnection: Unable to authenticate user with remote gateway at 128.211.138.40.1217result = -11000, errno = 9
Unable to setup communication to HPSS...
*** HSI: error opening logging
Error - authentication/initialization failed
The root cause for these errors is an expired or non-existent keytab file (a special authentication token stored in your home directory). These keytabs are valid for 90 days and on most RCAC resources they are usually automatically checked and regenerated when you execute hsi
or htar
commands. However, if the keytab is invalid, or fails to generate, Fortress may be unable to authenticate you and you would see the above errors. This is especially common on those RCAC clusters that have their own dedicated home directories (such as Bell), or on standalone installations (such as if you downloaded and installed HSI and HTAR on your non-RCAC computer).
This is a temporary problem and a permanent system-wide solution is being developed. In the interim, the recommended workaround is to generate a new valid keytab file in your main research computing home directory, and then copy it to your home directory on Bell. The fortresskey
command is used to generate the keytab and can be executed on another cluster or a dedicated data management host data.rcac.purdue.edu
:
$ ssh myusername@data.rcac.purdue.edu fortresskey
$ scp -pr myusername@data.rcac.purdue.edu:~/.private $HOME
With a valid keytab in place, you should then be able to use hsi
and htar
commands to access Fortress from Bell. Note that only one keytab can be valid at any given time (i.e. if you regenerated it, you may have to copy the new keytab to all systems that you intend to use hsi
or htar
from if they do not share the main research computing home directory).
Can I access Fortress from Bell?
Yes. While Fortress directories are not directly mounted on Bell for performance and archival protection reasons, they can be accessed from Bell front-ends and nodes using any of the recommended methods of HSI, HTAR or Globus.
Software
Frequently asked questions about software.
Cannot use pip after loading ml-toolkit modules
Link to section 'Question' of 'Cannot use pip after loading ml-toolkit modules' Question
Pip throws an error after loading the machine learning modules. How can I fix it?
Link to section 'Answer' of 'Cannot use pip after loading ml-toolkit modules' Answer
Machine learning modules (tensorflow, pytorch, opencv etc.) include a version of pip that is newer than the one installed with Anaconda. As a result it will throw an error when you try to use it.
$ pip --version
Traceback (most recent call last):
File "/apps/cent7/anaconda/5.1.0-py36/bin/pip", line 7, in <module>
from pip import main
ImportError: cannot import name 'main'
The preferred way to use pip with the machine learning modules is to invoke it via Python as shown below.
$ python -m pip --version
How can I get access to Sentaurus software?
Link to section 'Question' of 'How can I get access to Sentaurus software?' Question
How can I get access to Sentaurus tools for micro- and nano-electronics design?
Link to section 'Answer' of 'How can I get access to Sentaurus software?' Answer
Sentaurus software license requires a signed NDA. Please contact Dr. Mark Johnson, Director of ECE Instructional Laboratories to complete the process.
Once the licensing process is complete and you have been added into a cae2
Unix group, you could use Sentaurus on RCAC community clusters by loading the corresponding environment module:
module load sentaurus
Julia package installation
Users do not have write permission to the default julia package installation destination. However, users can install packages into home directory under ~/.julia
.
Users can side step this by explicitly defining where to put julia packages:
$ export JULIA_DEPOT_PATH=$HOME/.julia
$ julia -e 'using Pkg; Pkg.add("PackageName")'
About Research Computing
Frequently asked questions about RCAC.
Can I get a private server from RCAC?
Link to section 'Question' of 'Can I get a private server from RCAC?' Question
Can I get a private (virtual or physical) server from RCAC?
Link to section 'Answer' of 'Can I get a private server from RCAC?' Answer
Often, researchers may want a private server to run databases, web servers, or other software. RCAC currently has Geddes, a Community Composable Platform optimized for composable, cloud-like workflows that are complementary to the batch applications run on Community Clusters. Funded by the National Science Foundation under grant OAC-2018926, Geddes consists of Dell Compute nodes with two 64-core AMD Epyc 'Rome' processors (128 cores per node).
To purchase access to Geddes today, go to the Cluster Access Purchase page. Please subscribe to our Community Cluster Program Mailing List to stay informed on the latest purchasing developments or contact us (rcac-cluster-purchase@lists.purdue.edu) if you have any questions.
Datasets
Please refer to our Federated Datasets Documentation website for up-to-date datasets on Anvil and instructions on how to use them.
Gilbreth User Guide
Gilbreth is a Community Cluster optimized for communities running GPU intensive applications such as machine learning.
Link to section 'Overview of Gilbreth' of 'Overview of Gilbreth' Overview of Gilbreth
Gilbreth is a Community Cluster optimized for communities running GPU intensive applications such as machine learning. Gilbreth consists of Dell compute nodes with Intel Xeon processors and Nvidia Tesla GPUs.
To purchase access to Gilbreth today, go to the Cluster Access Purchase page. Please subscribe to our Community Cluster Program Mailing List to stay informed on the latest purchasing developments or contact us via email at rcac-cluster-purchase@lists.purdue.edu if you have any questions.
Link to section 'Gilbreth Namesake' of 'Overview of Gilbreth' Gilbreth Namesake
Gilbreth is named in honor of Lillian Moller Gilbreth, Purdue's first female engineering professor. More information about her life and impact on Purdue is available in a Biography of Lillian Moller Gilbreth.
Link to section 'Gilbreth Detailed Hardware Specification' of 'Overview of Gilbreth' Gilbreth Detailed Hardware Specification
Gilbreth has heterogeneous hardware comprising of Nvidia V100, A100, A10, and A30 GPUs in separate sub-clusters. All the nodes are connected by 100 Gbps Infiniband interconnects. Please see the hardware specifications below for details about various node types.
Front-Ends | Number of Nodes | Cores per Node | Memory per Node | GPUs per node (GPU memory per card) | Retires in |
---|---|---|---|---|---|
With GPU | 4 | 64 | 512 GB | 1 A30 (24 GB) | 2027 |
Sub-Cluster | Number of Nodes | Cores per Node | Memory per Node | GPUs per node (GPU memory per card) | Retires in |
---|---|---|---|---|---|
B | 16 | 24 | 192 GB | 3 A30 (24 GB) | 2027 |
C | 3 | 20 | 768 GB | 4 V100 (32 GB) with NVLink | 2024 |
D | 8 | 16 | 192 GB | 3 A30 (24 GB) | 2027 |
E | 16 | 16 | 192 GB | 2 V100 (16 GB) | 2024 |
F | 5 | 40 | 192 GB | 2 V100 (32 GB) | 2025 |
G | 12 | 128 | 512 GB | 2 A100 (40 GB) | 2026 |
H | 16 | 32 | 512 GB | 3 A10 (24 GB) | 2027 |
I | 5 | 32 | 512 GB | 2 A100 (80 GB) | 2027 |
J | 2 | 128 | 1024 GB | 4 A100 (80 GB) with NVLink | 2027 |
K | 52 | 64 | 512 GB | 2 A100 (80 GB) | 2028 |
L | 2 | 64 | 512 GB | 2 H100 | 2029 |
M-Not for Sale | 2 | 96 | 2 TB | 4 H100 | 2029 |
N | 20 | 48 | 1024 GB | 4 A100 (40 GB) with NVLink | 2029 |
Gilbreth nodes run CentOS 7 and use Slurm (Simple Linux Utility for Resource Management) as the batch scheduler for resource and job management. The application of operating system patches occurs as security needs dictate. All nodes allow for unlimited stack usage, as well as unlimited core dump size (though disk space and server quotas may still be a limiting factor).
On Gilbreth, the following set of compiler, math library, and message-passing library for parallel code are recommended:
- Intel/17.0.1.132
- MKL
- Intel MPI
This compiler and these libraries are loaded by default. To load the recommended set again:
$ module load rcac
To verify what you loaded:
$ module list
Link to section 'Software catalog' of 'Overview of Gilbreth' Software catalog
Link to section 'Accounts on Gilbreth' of 'Accounts' Accounts on Gilbreth
Link to section 'Obtaining an Account' of 'Accounts' Obtaining an Account
To obtain an account, you must be part of a research group which has purchased access to Gilbreth. Refer to the Accounts / Access page for more details on how to request access.
Link to section 'Outside Collaborators' of 'Accounts' Outside Collaborators
A valid Purdue Career Account is required for access to any resource. If you do not currently have a valid Purdue Career Account you must have a current Purdue faculty or staff member file a Request for Privileges (R4P) before you can proceed.
Logging In
To submit jobs on Gilbreth, log in to the submission host gilbreth.rcac.purdue.edu
via SSH. This submission host is actually 4 front-end hosts: gilbreth-fe00
through gilbreth-fe03
The login process randomly assigns one of these front-ends to each login to gilbreth.rcac.purdue.edu
.
Purdue Login
Link to section 'SSH' of 'Purdue Login' SSH
- SSH to the cluster as usual.
- When asked for a password, type your password followed by "
,push
". - Your Purdue Duo client will receive a notification to approve the login.
Link to section 'Thinlinc' of 'Purdue Login' Thinlinc
- When asked for a password, type your password followed by "
,push
". - Your Purdue Duo client will receive a notification to approve the login.
- The native Thinlinc client will prompt for Duo approval twice due to the way Thinlinc works.
- The native Thinlinc client also supports key-based authentication.
Passwords
Gilbreth supports either Purdue two-factor authentication (Purdue Login) or SSH keys.
SSH Client Software
Secure Shell or SSH is a way of establishing a secure connection between two computers. It uses public-key cryptography to authenticate the user with the remote computer and to establish a secure connection. Its usual function involves logging in to a remote machine and executing commands. There are many SSH clients available for all operating systems:
Linux / Solaris / AIX / HP-UX / Unix:
- The
ssh
command is pre-installed. Log in usingssh myusername@gilbreth.rcac.purdue.edu
from a terminal.
Microsoft Windows:
- MobaXterm is a small, easy to use, full-featured SSH client. It includes X11 support for remote displays, SFTP capabilities, and limited SSH authentication forwarding for keys.
Mac OS X:
- The
ssh
command is pre-installed. You may start a local terminal window from "Applications->Utilities". Log in by typing the commandssh myusername@gilbreth.rcac.purdue.edu
.
When prompted for password, enter your Purdue career account password followed by ",push
". Your Purdue Duo client will then receive a notification to approve the login.
SSH Keys
Link to section 'General overview' of 'SSH Keys' General overview
To connect to Gilbreth using SSH keys, you must follow three high-level steps:
- Generate a key pair consisting of a private and a public key on your local machine.
- Copy the public key to the cluster and append it to
$HOME/.ssh/authorized_keys
file in your account. - Test if you can ssh from your local computer to the cluster without using your Purdue password.
Detailed steps for different operating systems and specific SSH client softwares are give below.
Link to section 'Mac and Linux:' of 'SSH Keys' Mac and Linux:
-
Run
ssh-keygen
in a terminal on your local machine. You may supply a filename and a passphrase for protecting your private key, but it is not mandatory. To accept the default settings, press Enter without specifying a filename.
Note: If you do not protect your private key with a passphrase, anyone with access to your computer could SSH to your account on Gilbreth. -
By default, the key files will be stored in
~/.ssh/id_rsa
and~/.ssh/id_rsa.pub
on your local machine. -
Copy the contents of the public key into
$HOME/.ssh/authorized_keys
on the cluster with the following command. When asked for a password, type your password followed by ",push
". Your Purdue Duo client will receive a notification to approve the login.ssh-copy-id -i ~/.ssh/id_rsa.pub myusername@gilbreth.rcac.purdue.edu
Note: use your actual Purdue account user name.
If your system does not have the
ssh-copy-id
command, use this instead:cat ~/.ssh/id_rsa.pub | ssh myusername@gilbreth.rcac.purdue.edu "mkdir -p ~/.ssh && chmod 700 ~/.ssh && cat >> ~/.ssh/authorized_keys"
-
Test the new key by SSH-ing to the server. The login should now complete without asking for a password.
-
If the private key has a non-default name or location, you need to specify the key by
ssh -i my_private_key_name myusername@gilbreth.rcac.purdue.edu
Link to section 'Windows:' of 'SSH Keys' Windows:
Programs | Instructions |
---|---|
MobaXterm | Open a local terminal and follow Linux steps |
Git Bash | Follow Linux steps |
Windows 10 PowerShell | Follow Linux steps |
Windows 10 Subsystem for Linux | Follow Linux steps |
PuTTY | Follow steps below |
PuTTY:
-
Launch PuTTYgen, keep the default key type (RSA) and length (2048-bits) and click Generate button.
The "Generate" button can be found under the "Actions" section of the PuTTY Key Generator interface. -
Once the key pair is generated:
Use the Save public key button to save the public key, e.g.
Documents\SSH_Keys\mylaptop_public_key.pub
Use the Save private key button to save the private key, e.g.
Documents\SSH_Keys\mylaptop_private_key.ppk
. When saving the private key, you can also choose a reminder comment, as well as an optional passphrase to protect your key, as shown in the image below. Note: If you do not protect your private key with a passphrase, anyone with access to your computer could SSH to your account on Gilbreth.The PuTTY Key Generator form has inputs for the Key passphrase and optional reminder comment. From the menu of PuTTYgen, use the "Conversion -> Export OpenSSH key" tool to convert the private key into openssh format, e.g.
Documents\SSH_Keys\mylaptop_private_key.openssh
to be used later for Thinlinc. -
Configure PuTTY to use key-based authentication:
Launch PuTTY and navigate to "Connection -> SSH ->Auth" on the left panel, click Browse button under the "Authentication parameters" section and choose your private key, e.g. mylaptop_private_key.ppk
After clicking Connection -> SSH ->Auth panel, the "Browse" option can be found at the bottom of the resulting panel. Navigate back to "Session" on the left panel. Highlight "Default Settings" and click the "Save" button to ensure the change in place.
-
Connect to the cluster. When asked for a password, type your password followed by "
,push
". Your Purdue Duo client will receive a notification to approve the login. Copy the contents of public key from PuTTYgen as shown below and paste it into$HOME/.ssh/authorized_keys
. Please double-check that your text editor did not wrap or fold the pasted value (it should be one very long line).The "Public key" will look like a long string of random letters and numbers in a text box at the top of the window. - Test by connecting to the cluster. If successful, you will not be prompted for a password or receive a Duo notification. If you protected your private key with a passphrase in step 2, you will instead be prompted to enter your chosen passphrase when connecting.
ThinLinc
RCAC provides Cendio's ThinLinc as an alternative to running an X11 server directly on your computer. It allows you to run graphical applications or graphical interactive jobs directly on Gilbreth through a persistent remote graphical desktop session.
ThinLinc is a service that allows you to connect to a persistent remote graphical desktop session. This service works very well over a high latency, low bandwidth, or off-campus connection compared to running an X11 server locally. It is also very helpful for Windows users who do not have an easy to use local X11 server, as little to no set up is required on your computer.
There are two ways in which to use ThinLinc: preferably through the native client or through a web browser.
Link to section 'Installing the ThinLinc native client' of 'ThinLinc' Installing the ThinLinc native client
The native ThinLinc client will offer the best experience especially over off-campus connections and is the recommended method for using ThinLinc. It is compatible with Windows, Mac OS X, and Linux.
- Download the ThinLinc client from the ThinLinc website.
- Start the ThinLinc client on your computer.
- In the client's login window, use desktop.gilbreth.rcac.purdue.edu as the Server. Use your Purdue Career Account username and password, but append "
,push
" to your password. - Click the Connect button.
- Your Purdue Login Duo will receive a notification to approve your login.
- Continue to following section on connecting to Gilbreth from ThinLinc.
Link to section 'Using ThinLinc through your web browser' of 'ThinLinc' Using ThinLinc through your web browser
The ThinLinc service can be accessed from your web browser as a convenience to installing the native client. This option works with no set up and is a good option for those on computers where you do not have privileges to install software. All that is required is an up-to-date web browser. Older versions of Internet Explorer may not work.
- Open a web browser and navigate to desktop.gilbreth.rcac.purdue.edu.
- Log in with your Purdue Career Account username and password, but append "
,push
" to your password. - You may safely proceed past any warning messages from your browser.
- Your Purdue Login Duo will receive a notification to approve your login.
- Continue to the following section on connecting to Gilbreth from ThinLinc.
Link to section 'Connecting to Gilbreth from ThinLinc' of 'ThinLinc' Connecting to Gilbreth from ThinLinc
- Once logged in, you will be presented with a remote Linux desktop running directly on a cluster front-end.
- Open the terminal application on the remote desktop.
- Once logged in to the Gilbreth head node, you may use graphical editors, debuggers, software like Matlab, or run graphical interactive jobs. For example, to test the X forwarding connection issue the following command to launch the graphical editor gedit:
$ gedit
- This session will remain persistent even if you disconnect from the session. Any interactive jobs or applications you left running will continue running even if you are not connected to the session.
Link to section 'Tips for using ThinLinc native client' of 'ThinLinc' Tips for using ThinLinc native client
- To exit a full screen ThinLinc session press the F8 key on your keyboard (fn + F8 key for Mac users) and click to disconnect or exit full screen.
- Full screen mode can be disabled when connecting to a session by clicking the Options button and disabling full screen mode from the Screen tab.
Link to section 'Configure ThinLinc to use SSH Keys' of 'ThinLinc' Configure ThinLinc to use SSH Keys
- The web client does NOT support public-key authentication.
-
ThinLinc native client supports the use of an SSH key pair. For help generating and uploading keys to the cluster, see SSH Keys section in our user guide for details.
To set up SSH key authentication on the ThinLinc client:
-
Open the Options panel, and select Public key as your authentication method on the Security tab.
The "Options..." button in the ThinLinc Client can be found towards the bottom left, above the "Connect" button. -
In the options dialog, switch to the "Security" tab and select the "Public key" radio button:
The "Security" tab found in the options dialog, will be the last of available tabs. The "Public key" option can be found in the "Authentication method" options group. - Click OK to return to the ThinLinc Client login window. You should now see a Key field in place of the Password field.
-
In the Key field, type the path to your locally stored private key or click the ... button to locate and select the key on your local system. Note: If PuTTY is used to generate the SSH Key pairs, please choose the private key in the openssh format.
The ThinLinc Client login window will now display key field instead of a password field.
-
SSH X11 Forwarding
SSH supports tunneling of X11 (X-Windows). If you have an X11 server running on your local machine, you may use X11 applications on remote systems and have their graphical displays appear on your local machine. These X11 connections are tunneled and encrypted automatically by your SSH client.
Link to section 'Installing an X11 Server' of 'SSH X11 Forwarding' Installing an X11 Server
To use X11, you will need to have a local X11 server running on your personal machine. Both free and commercial X11 servers are available for various operating systems.
Linux / Solaris / AIX / HP-UX / Unix:
- An X11 server is at the core of all graphical sessions. If you are logged in to a graphical environment on these operating systems, you are already running an X11 server.
- ThinLinc is an alternative to running an X11 server directly on your Linux computer. ThinLinc is a service that allows you to connect to a persistent remote graphical desktop session.
Microsoft Windows:
- ThinLinc is an alternative to running an X11 server directly on your Windows computer. ThinLinc is a service that allows you to connect to a persistent remote graphical desktop session.
- MobaXterm is a small, easy to use, full-featured SSH client. It includes X11 support for remote displays, SFTP capabilities, and limited SSH authentication forwarding for keys.
Mac OS X:
- X11 is available as an optional install on the Mac OS X install disks prior to 10.7/Lion. Run the installer, select the X11 option, and follow the instructions. For 10.7+ please download XQuartz.
- ThinLinc is an alternative to running an X11 server directly on your Mac computer. ThinLinc is a service that allows you to connect to a persistent remote graphical desktop session.
Link to section 'Enabling X11 Forwarding in your SSH Client' of 'SSH X11 Forwarding' Enabling X11 Forwarding in your SSH Client
Once you are running an X11 server, you will need to enable X11 forwarding/tunneling in your SSH client:
ssh
: X11 tunneling should be enabled by default. To be certain it is enabled, you may usessh -Y
.- MobaXterm: Select "New session" and "SSH." Under "Advanced SSH Settings" check the box for X11 Forwarding.
SSH will set the remote environment variable $DISPLAY
to "localhost:XX.YY"
when this is working correctly. If you had previously set your $DISPLAY
environment variable to your local IP or hostname, you must remove any set
/export
/setenv
of this variable from your login scripts. The environment variable $DISPLAY
must be left as SSH sets it, which is to a random local port address. Setting $DISPLAY
to an IP or hostname will not work.
Purchasing Nodes
RCAC operates a significant shared cluster computing infrastructure developed over several years through focused acquisitions using funds from grants, faculty startup packages, and institutional sources. These "community clusters" are now at the foundation of Purdue's research cyberinfrastructure.
We strongly encourage any Purdue faculty or staff with computational needs to join this growing community and enjoy the enormous benefits this shared infrastructure provides:
- Peace of Mind
RCAC system administrators take care of security patches, attempted hacks, operating system upgrades, and hardware repair so faculty and graduate students can concentrate on research.
- Low Overhead
RCAC data centers provide infrastructure such as networking, racks, floor space, cooling, and power.
- Cost Effective
RCAC works with vendors to obtain the best price for computing resources by pooling funds from different disciplines to leverage greater group purchasing power.
Through the Community Cluster Program, Purdue affiliates have invested several million dollars in computational and storage resources from Q4 2006 to the present with great success in both the research accomplished and the money saved on equipment purchases.
For more information or to purchase access to our latest cluster today, see the Purchase page. Have questions? contact us at rcac-cluster-purchase@lists.purdue.edu to discuss.
File Storage and Transfer
Learn more about file storage transfer for Gilbreth.
Link to section 'Archive and Compression' of 'Archive and Compression' Archive and Compression
There are several options for archiving and compressing groups of files or directories. The mostly commonly used options are:
Link to section 'tar' of 'Archive and Compression' tar
See the official documentation for tar for more information.
Saves many files together into a single archive file, and restores individual files from the archive. Includes automatic archive compression/decompression options and special features for incremental and full backups.
Examples:
(list contents of archive somefile.tar)
$ tar tvf somefile.tar
(extract contents of somefile.tar)
$ tar xvf somefile.tar
(extract contents of gzipped archive somefile.tar.gz)
$ tar xzvf somefile.tar.gz
(extract contents of bzip2 archive somefile.tar.bz2)
$ tar xjvf somefile.tar.bz2
(archive all ".c" files in current directory into one archive file)
$ tar cvf somefile.tar *.c
(archive and gzip-compress all files in a directory into one archive file)
$ tar czvf somefile.tar.gz somedirectory/
(archive and bzip2-compress all files in a directory into one archive file)
$ tar cjvf somefile.tar.bz2 somedirectory/
Other arguments for tar can be explored by using the man tar command.
Link to section 'gzip' of 'Archive and Compression' gzip
The standard compression system for all GNU software.
Examples:
(compress file somefile - also removes uncompressed file)
$ gzip somefile
(uncompress file somefile.gz - also removes compressed file)
$ gunzip somefile.gz
Link to section 'bzip2' of 'Archive and Compression' bzip2
See the official documentation for bzip for more information.
Strong, lossless data compressor based on the Burrows-Wheeler transform. Stronger compression than gzip.
Examples:
(compress file somefile - also removes uncompressed file)
$ bzip2 somefile
(uncompress file somefile.bz2 - also removes compressed file)
$ bunzip2 somefile.bz2
There are several other, less commonly used, options available as well:
- zip
- 7zip
- xz
Link to section 'Storage Environment Variables' of 'Storage Environment Variables' Storage Environment Variables
Several environment variables are automatically defined for you to help you manage your storage. Use environment variables instead of actual paths whenever possible to avoid problems if the specific paths to any of these change.
Name | Description |
---|---|
HOME | /home/myusername |
PWD | path to your current directory |
RCAC_SCRATCH | /scratch/gilbreth/myusername |
By convention, environment variable names are all uppercase. You may use them on the command line or in any scripts in place of and in combination with hard-coded values:
$ ls $HOME
...
$ ls $RCAC_SCRATCH/myproject
...
To find the value of any environment variable:
$ echo $RCAC_SCRATCH
/scratch/gilbreth/myusername
To list the values of all environment variables:
$ env
USER=myusername
HOME=/home/myusername
RCAC_SCRATCH=/scratch/gilbreth/myusername
...
You may create or overwrite an environment variable. To pass (export) the value of a variable in bash:
$ export MYPROJECT=$RCAC_SCRATCH/myproject
To assign a value to an environment variable in either tcsh or csh:
$ setenv MYPROJECT value
Storage Options
File storage options on RCAC systems include long-term storage (home directories, depot, Fortress) and short-term storage (scratch directories, /tmp directory). Each option has different performance and intended uses, and some options vary from system to system as well. Daily snapshots of home directories are provided for a limited time for accidental deletion recovery. Scratch directories and temporary storage are not backed up and old files are regularly purged from scratch and /tmp directories. More details about each storage option appear below.
Home Directory
Home directories are provided for long-term file storage. Each user has one home directory. You should use your home directory for storing important program files, scripts, input data sets, critical results, and frequently used files. You should store infrequently used files on Fortress. Your home directory becomes your current working directory, by default, when you log in.
Your home directory physically resides on a dedicated storage system only accessible for Gilbreth. To find the path to your home directory, first log in then immediately enter the following:
$ pwd
/home/myusername
Or from any subdirectory:
$ echo $HOME
/home/myusername
Please note that your Gilbreth home directory and its contents are exclusive to Gilbreth cluster, including front-end hosts and compute nodes. This home directory is not available on other RCAC machines but Gilbreth. There is no automatic copying or synchronization between home directories, but at your discretion you can manually copy all or parts of your main home to Gilbreth using one of the suggested methods.
Your home directory has a quota limiting the total size of files you may store within. For more information, refer to the Storage Quotas / Limits Section.
Link to section 'Lost File Recovery' of 'Home Directory' Lost File Recovery
Nightly snapshots for 7 days, weekly snapshots for 4 weeks, and monthly snapshots for 3 months are kept. This means you will find snapshots from the last 7 nights, the last 4 Sundays, and the last 3 first of the months. Files are available going back between two and three months, depending on how long ago the last first of the month was. Snapshots beyond this are not kept. For additional security, you should store another copy of your files on more permanent storage, such as the Fortress HPSS Archive.
Link to section 'Performance' of 'Home Directory' Performance
Your home directory is medium-performance, non-purged space suitable for tasks like sharing data, editing files, developing and building software, and many other uses.
Your home directory is not designed or intended for use as high-performance working space for running data-intensive jobs with heavy I/O demands.
Link to section 'Long-Term Storage' of 'Long-Term Storage' Long-Term Storage
Long-term Storage or Permanent Storage is available to users on the High Performance Storage System (HPSS), an archival storage system, called Fortress. Program files, data files and any other files which are not used often, but which must be saved, can be put in permanent storage. Fortress currently has over 10PB of capacity.
For more information about Fortress, how it works, and user guides, and how to obtain an account:
Scratch Space
Scratch directories are provided for short-term file storage only. The quota of your scratch directory is much greater than the quota of your home directory. You should use your scratch directory for storing temporary input files which your job reads or for writing temporary output files which you may examine after execution of your job. You should use your home directory and Fortress for longer-term storage or for holding critical results. The hsi and htar commands provide easy-to-use interfaces into the archive and can be used to copy files into the archive interactively or even automatically at the end of your regular job submission scripts.
Files in scratch directories are not recoverable. Files in scratch directories are not backed up. If you accidentally delete a file, a disk crashes, or old files are purged, they cannot be restored.
Files are purged from scratch directories not accessed or had content modified in 60 days. Owners of these files receive a notice one week before removal via email. Be sure to regularly check your Purdue email account or set up mail forwarding to an email account you do regularly check. For more information, please refer to our Scratch File Purging Policy.
All users may access scratch directories on Gilbreth. To find the path to your scratch directory:
$ findscratch
/scratch/gilbreth/myusername
The value of variable $RCAC_SCRATCH is your scratch directory path. Use this variable in any scripts. Your actual scratch directory path may change without warning, but this variable will remain current.
$ echo $RCAC_SCRATCH
/scratch/gilbreth/myusername
Scratch directories are specific per cluster. I.e. only the /scratch/gilbreth directory is available on Gilbreth front-end and compute nodes. No other scratch directories are available on Gilbreth.
Your scratch directory has a quota capping the total size and number of files you may store in it. For more information, refer to the section Storage Quotas / Limits.
Link to section 'Performance' of 'Scratch Space' Performance
Your scratch directory is located on a high-performance, large-capacity parallel filesystem engineered to provide work-area storage optimized for a wide variety of job types. It is designed to perform well with data-intensive computations, while scaling well to large numbers of simultaneous connections.
/tmp Directory
/tmp directories are provided for short-term file storage only. Each front-end and compute node has a /tmp directory. Your program may write temporary data to the /tmp
directory of the compute node on which it is running. That data is available for as long as your program is active. Once your program terminates, that temporary data is no longer available. When used properly, /tmp
may provide faster local storage to an active process than any other storage option. You should use your home directory and Fortress for longer-term storage or for holding critical results.
Backups are not performed for the /tmp
directory and removes files from /tmp
whenever space is low or whenever the system needs a reboot. In the event of a disk crash or file purge, files in /tmp
are not recoverable. You should copy any important files to more permanent storage.
Storage Quota / Limits
Some limits are imposed on your disk usage on research systems. A quota is implemented on each filesystem. Each filesystem (home directory, scratch directory, etc.) may have a different limit. If you exceed the quota, you will not be able to save new files or new data to the filesystem until you delete or move data to long-term storage.
Link to section 'Checking Quota' of 'Storage Quota / Limits' Checking Quota
To check the current quotas of your home and scratch directories check the My Quota page or use the myquota
command:
$ myquota
Type Filesystem Size Limit Use Files Limit Use
==============================================================================
home myusername 5.0GB 25.0GB 20% - - -
scratch gilbreth 220.7GB 100.0TB 0.22% 8k 2,000k 0.43%
The columns are as follows:
- Type: indicates home or scratch directory or your depot space.
- Filesystem: name of storage option.
- Size: sum of file sizes in bytes.
- Limit: allowed maximum on sum of file sizes in bytes.
- Use: percentage of file-size limit currently in use.
- Files: number of files and directories (not the size).
- Limit: allowed maximum on number of files and directories. It is possible, though unlikely, to reach this limit and not the file-size limit if you create a large number of very small files.
- Use: percentage of file-number limit currently in use.
If you find that you reached your quota in either your home directory or your scratch file directory, obtain estimates of your disk usage. Find the top-level directories which have a high disk usage, then study the subdirectories to discover where the heaviest usage lies.
To see in a human-readable format an estimate of the disk usage of your top-level directories in your home directory:
$ du -h --max-depth=1 $HOME >myfile
32K /home/myusername/mysubdirectory_1
529M /home/myusername/mysubdirectory_2
608K /home/myusername/mysubdirectory_3
The second directory is the largest of the three, so apply command du to it.
To see in a human-readable format an estimate of the disk usage of your top-level directories in your scratch file directory:
$ du -h --max-depth=1 $RCAC_SCRATCH >myfile
160K /scratch/gilbreth/myusername
This strategy can be very helpful in figuring out the location of your largest usage. Move unneeded files and directories to long-term storage to free space in your home and scratch directories.
Link to section 'Increasing Quota' of 'Storage Quota / Limits' Increasing Quota
Link to section 'Home Directory' of 'Storage Quota / Limits' Home Directory
If you find you need additional disk space in your home directory, please consider archiving and compressing old files and moving them to long-term storage on the Fortress HPSS Archive, or purchase the Depot space for long-term storage. Unfortunately, it is not possible to increase your home directory quota beyond it's current level.
Link to section 'Scratch Space' of 'Storage Quota / Limits' Scratch Space
If you find you need additional disk space in your scratch space, please first consider archiving and compressing old files and moving them to long-term storage on the Fortress HPSS Archive. If you are unable to do so, you may ask for a quota increase by contacting support.
Link to section 'Sharing Files from Gilbreth' of 'Sharing' Sharing Files from Gilbreth
Gilbreth supports several methods for file sharing. Use the links below to learn more about these methods.
Link to section 'Sharing Data with Globus' of 'Globus' Sharing Data with Globus
Data on any RCAC resource can be shared with other users within Purdue or with collaborators at other institutions. Globus allows convenient sharing of data with outside collaborators. Data can be shared with collaborators' personal computers or directly with many other computing resources at other institutions.
To share files, login to https://transfer.rcac.purdue.edu, navigate to the endpoint (collection) of your choice, and follow instructions as described in Globus documentation on how to share data:
See also RCAC Globus presentation.
File Transfer
Gilbreth supports several methods for file transfer. Use the links below to learn more about these methods.
SCP
SCP (Secure CoPy) is a simple way of transferring files between two machines that use the SSH protocol. SCP is available as a protocol choice in some graphical file transfer programs and also as a command line program on most Linux, Unix, and Mac OS X systems. SCP can copy single files, but will also recursively copy directory contents if given a directory name.
After Aug 17, 2020, the community clusters will not support password-based authentication for login. Methods that can be used include two-factor authentication (Purdue Login) or SSH keys. If you do not have SSH keys installed, you would need to type your Purdue Login response into the SFTP's "Password" prompt.
Link to section 'Command-line usage:' of 'SCP' Command-line usage:
You can transfer files both to and from Gilbreth while initiating an SCP session on either some other computer or on Gilbreth (in other words, directionality of connection and directionality of data flow are independent from each other). The scp
command appears somewhat similar to the familiar cp
command, with an extra user@host:file
syntax to denote files and directories on a remote host. Either Gilbreth or another computer can be a remote.
-
Example: Initiating SCP session on some other computer (i.e. you are on some other computer, connecting to Gilbreth):
(transfer TO Gilbreth) (Individual files) $ scp sourcefile myusername@gilbreth.rcac.purdue.edu:somedir/destinationfile $ scp sourcefile myusername@gilbreth.rcac.purdue.edu:somedir/ (Recursive directory copy) $ scp -pr sourcedirectory/ myusername@gilbreth.rcac.purdue.edu:somedir/ (transfer FROM Gilbreth) (Individual files) $ scp myusername@gilbreth.rcac.purdue.edu:somedir/sourcefile destinationfile $ scp myusername@gilbreth.rcac.purdue.edu:somedir/sourcefile somedir/ (Recursive directory copy) $ scp -pr myusername@gilbreth.rcac.purdue.edu:sourcedirectory somedir/
The -p flag is optional. When used, it will cause the transfer to preserve file attributes and permissions. The -r flag is required for recursive transfers of entire directories.
-
Example: Initiating SCP session on Gilbreth (i.e. you are on Gilbreth, connecting to some other computer):
(transfer TO Gilbreth) (Individual files) $ scp myusername@$another.computer.example.com:sourcefile somedir/destinationfile $ scp myusername@$another.computer.example.com:sourcefile somedir/ (Recursive directory copy) $ scp -pr myusername@$another.computer.example.com:sourcedirectory/ somedir/ (transfer FROM Gilbreth) (Individual files) $ scp somedir/sourcefile myusername@$another.computer.example.com:destinationfile $ scp somedir/sourcefile myusername@$another.computer.example.com:somedir/ (Recursive directory copy) $ scp -pr sourcedirectory myusername@$another.computer.example.com:somedir/
The -p flag is optional. When used, it will cause the transfer to preserve file attributes and permissions. The -r flag is required for recursive transfers of entire directories.
Link to section 'Software (SCP clients)' of 'SCP' Software (SCP clients)
Linux and other Unix-like systems:
- The
scp
command-line program should already be installed.
Microsoft Windows:
- MobaXterm
Free, full-featured, graphical Windows SSH, SCP, and SFTP client. - Command-line
scp
program can be installed as part of Windows Subsystem for Linux (WSL), or Git-Bash.
Mac OS X:
- The
scp
command-line program should already be installed. You may start a local terminal window from "Applications->Utilities". - Cyberduck is a full-featured and free graphical SFTP and SCP client.
Globus
Link to section 'Globus' of 'Globus' Globus
Globus, previously known as Globus Online, is a powerful and easy to use file transfer service for transferring files virtually anywhere. It works within RCAC's various research storage systems; it connects between RCAC and remote research sites running Globus; and it connects research systems to personal systems. You may use Globus to connect to your home, scratch, and Fortress storage directories. Since Globus is web-based, it works on any operating system that is connected to the internet. The Globus Personal client is available on Windows, Linux, and Mac OS X. It is primarily used as a graphical means of transfer but it can also be used over the command line.
Link to section 'Link to section 'Globus Web:' of 'Globus' Globus Web:' of 'Globus' Link to section 'Globus Web:' of 'Globus' Globus Web:
- Navigate to http://transfer.rcac.purdue.edu
- Click "Proceed" to log in with your Purdue Career Account.
- On your first login it will ask to make a connection to a Globus account. Accept the conditions.
- Now you are at the main screen. Click "File Transfer" which will bring you to a two-panel interface (if you only see one panel, you can use selector in the top-right corner to switch the view).
- You will need to select one collection and file path on one side as the source, and the second collection on the other as the destination. This can be one of several Purdue endpoints, or another University, or even your personal computer (see Personal Client section below).
The RCAC collections are as follows. A search for "Purdue" will give you several suggested results you can choose from, or you can give a more specific search.
- Home Directory storage: "Purdue Research Computing - Home Directories", however, you can start typing "Purdue" and "Home Directories" and it will suggest appropriate matches.
- Weber scratch storage: "Purdue Weber Cluster", however, you can start typing "Purdue" and "Weber and it will suggest appropriate matches. From here you will need to navigate into the first letter of your username, and then into your username.
- Research Data Depot: "Purdue Research Computing - Data Depot", a search for "Depot" should provide appropriate matches to choose from.
- Fortress: "Purdue Fortress HPSS Archive", a search for "Fortress" should provide appropriate matches to choose from.
From here, select a file or folder in either side of the two-pane window, and then use the arrows in the top-middle of the interface to instruct Globus to move files from one side to the other. You can transfer files in either direction. You will receive an email once the transfer is completed.
Link to section 'Link to section 'Globus Personal Client setup:' of 'Globus' Globus Personal Client setup:' of 'Globus' Link to section 'Globus Personal Client setup:' of 'Globus' Globus Personal Client setup:
Globus Connect Personal is a small software tool you can install to make your own computer a Globus endpoint on its own. It is useful if you need to transfer files via Globus to and from your computer directly.
- On the "Collections" page from earlier, click "Get Globus Connect Personal" or download a version for your operating system it from here: Globus Connect Personal
- Name this particular personal system and follow the setup prompts to create your Globus Connect Personal endpoint.
- Your personal system is now available as a collection within the Globus transfer interface.
Link to section 'Link to section 'Globus Command Line:' of 'Globus' Globus Command Line:' of 'Globus' Link to section 'Globus Command Line:' of 'Globus' Globus Command Line:
Globus supports command line interface, allowing advanced automation of your transfers.
To use the recommended standalone Globus CLI application (the globus command):
- First time use: issue the globus login command and follow instructions for initial login.
- Commands for interfacing with the CLI can be found via Using the Command Line Interface, as well as the Globus CLI Examples pages.
Link to section 'Link to section 'Sharing Data with Outside Collaborators' of 'Globus' Sharing Data with Outside Collaborators' of 'Globus' Link to section 'Sharing Data with Outside Collaborators' of 'Globus' Sharing Data with Outside Collaborators
Globus allows convenient sharing of data with outside collaborators. Data can be shared with collaborators' personal computers or directly with many other computing resources at other institutions. See the Globus documentation on how to share data:
For links to more information, please see Globus Support page and RCAC Globus presentation.
Windows Network Drive / SMB
SMB (Server Message Block), also known as CIFS, is an easy to use file transfer protocol that is useful for transferring files between RCAC systems and a desktop or laptop. You may use SMB to connect to your home, scratch, and Fortress storage directories. The SMB protocol is available on Windows, Linux, and Mac OS X. It is primarily used as a graphical means of transfer but it can also be used over the command line.
Note: to access Gilbreth through SMB file sharing, you must be on a Purdue campus network or connected through VPN.
Link to section 'Windows:' of 'Windows Network Drive / SMB' Windows:
- Windows 7: Click Windows menu > Computer, then click Map Network Drive in the top bar
- Windows 8 & 10: Tap the Windows key, type computer, select This PC, click Computer > Map Network Drive in the top bar
- Windows 11: Tap the Windows key, type File Explorer, select This PC, click Computer > Map Network Drive in the top bar
- In the folder location enter the following information and click Finish:
- To access your Gilbreth home directory, enter \\home.gilbreth.rcac.purdue.edu\gilbreth-home.
- To access your scratch space on Gilbreth, enter \\scratch.gilbreth.rcac.purdue.edu\gilbreth-scratch. Once mapped, you will be able to navigate to your scratch directory.
- Note: Use your career account login name and password when prompted. (You will not need to add "
,push
" nor use your Purdue Duo client.) - Your home or scratch directory should now be mounted as a drive in the Computer window.
Link to section 'Mac OS X:' of 'Windows Network Drive / SMB' Mac OS X:
- In the Finder, click Go > Connect to Server
- In the Server Address enter the following information and click Connect:
- To access your Gilbreth home directory, enter smb://home.gilbreth.rcac.purdue.edu/gilbreth-home.
- To access your scratch space on Gilbreth, enter smb://scratch.gilbreth.rcac.purdue.edu/gilbreth-scratch. Once mapped, you will be able to navigate to your scratch directory.
- Note: Use your career account login name and password when prompted. (You will not need to add "
,push
" nor use your Purdue Duo client.) - Your home or scratch directory should now be mounted as a drive in the Computer window.
Link to section 'Linux:' of 'Windows Network Drive / SMB' Linux:
- There are several graphical methods to connect in Linux depending on your desktop environment. Once you find out how to connect to a network server on your desktop environment, choose the Samba/SMB protocol and adapt the information from the Mac OS X section to connect.
- If you would like access via samba on the command line you may install smbclient which will give you FTP-like access and can be used as shown below. For all the possible ways to connect look at the Mac OS X instructions.
smbclient //home.gilbreth.rcac.purdue.edu/gilbreth-home -U myusername smbclient //scratch.gilbreth.rcac.purdue.edu/gilbreth-scratch -U myusername
- Note: Use your career account login name and password when prompted. (You will not need to add "
,push
" nor use your Purdue Duo client.)
FTP / SFTP
FTP is not supported on any research systems because it does not allow for secure transmission of data. Use SFTP instead, as described below.
SFTP (Secure File Transfer Protocol) is a reliable way of transferring files between two machines. SFTP is available as a protocol choice in some graphical file transfer programs and also as a command-line program on most Linux, Unix, and Mac OS X systems. SFTP has more features than SCP and allows for other operations on remote files, remote directory listing, and resuming interrupted transfers. Command-line SFTP cannot recursively copy directory contents; to do so, try using SCP or graphical SFTP client.
After Aug 17, 2020, the community clusters will not support password-based authentication for login. Methods that can be used include two-factor authentication (Purdue Login) or SSH keys. If you do not have SSH keys installed, you would need to type your Purdue Login response into the SFTP's "Password" prompt.
Link to section 'Command-line usage' of 'FTP / SFTP' Command-line usage
You can transfer files both to and from Gilbreth while initiating an SFTP session on either some other computer or on Gilbreth (in other words, directionality of connection and directionality of data flow are independent from each other). Once the connection is established, you use put
or get
subcommands between "local" and "remote" computers. Either Gilbreth or another computer can be a remote.
-
Example: Initiating SFTP session on some other computer (i.e. you are on another computer, connecting to Gilbreth):
$ sftp myusername@gilbreth.rcac.purdue.edu (transfer TO Gilbreth) sftp> put sourcefile somedir/destinationfile sftp> put -P sourcefile somedir/ (transfer FROM Gilbreth) sftp> get sourcefile somedir/destinationfile sftp> get -P sourcefile somedir/ sftp> exit
The -P flag is optional. When used, it will cause the transfer to preserve file attributes and permissions.
-
Example: Initiating SFTP session on Gilbreth (i.e. you are on Gilbreth, connecting to some other computer):
$ sftp myusername@$another.computer.example.com (transfer TO Gilbreth) sftp> get sourcefile somedir/destinationfile sftp> get -P sourcefile somedir/ (transfer FROM Gilbreth) sftp> put sourcefile somedir/destinationfile sftp> put -P sourcefile somedir/ sftp> exit
The -P flag is optional. When used, it will cause the transfer to preserve file attributes and permissions.
Link to section 'Software (SFTP clients)' of 'FTP / SFTP' Software (SFTP clients)
Linux and other Unix-like systems:
- The
sftp
command-line program should already be installed.
Microsoft Windows:
- MobaXterm
Free, full-featured, graphical Windows SSH, SCP, and SFTP client. - Command-line
sftp
program can be installed as part of Windows Subsystem for Linux (WSL), or Git-Bash.
Mac OS X:
- The
sftp
command-line program should already be installed. You may start a local terminal window from "Applications->Utilities". - Cyberduck is a full-featured and free graphical SFTP and SCP client.
Copying files from Purdue IT research computing home directory to Gilbreth
The Gilbreth home directory and its contents are specific to the Gilbreth cluster, and are not available on other RCAC machines. For people having access to other Community Clusters and Gilbreth, there is no automatic copying or synchronization between main and Gilbreth home directories. At your discretion, you can manually copy all or parts of your main research computing home to Gilbreth using one of the methods described below.
Please note that copying may fail if the size of your research computing home directory is larger than the Gilbreth one's quota. Please check usage and limits before proceeding!
Link to section 'Complete copy' of 'Copying files from Purdue IT research computing home directory to Gilbreth' Complete copy
For your convenience, a custom tool copy-rcac-home
is provided to simplify at-will duplication of your main research computing home directory into Gilbreth. The tool performs a complete 1-to-1 copy using rsync -auH
(with exception of a narrow subset of system-specific service files).
To use the tool, simply type copy-rcac-home
in a terminal window on a Gilbreth front-end or compute node:
$ copy-rcac-home
This script will copy entire contents of your main RCAC
home directory into your Gilbreth cluster's $HOME.
Note: copying may fail if the size of your RCAC home directory
is larger than your quota on the Gilbreth one (25GB).
BEFORE PROCEEDING, please run 'myquota' command on another
cluster to see your usage there and judge whether it would fit!
Would you like to proceed? [Y/n]:
At this stage answering yes
will proceed with copying, or you can respond with a no
(or Ctrl-C
) to cancel. See copy-rcac-home --help
for more details on the tool.
Link to section 'Partial copy' of 'Copying files from Purdue IT research computing home directory to Gilbreth' Partial copy
Desired parts (or whole) of your research computing home directories can be copied to Gilbreth via any of the home directories' supported transfer methods, such as SCP, SFTP, rsync, or Globus.
-
Example: recursive copying of a subdirectory from RCAC home directory into Gilbreth home using
scp
.(if you are on Gilbreth, use other cluster name for the remote part) $ scp -pr myothercluster.rcac.purdue.edu:somedirectory/ ~/ (if you are on another cluster, use Gilbreth for the remote part) $ scp -pr somedirectory/ myusername@gilbreth.rcac.purdue.edu:~/
-
Example: copying using Globus.
Search collections for "Purdue Research Computing - Home Directories" and "Purdue Gilbreth Cluster - Home" endpoints, respectively, then transfer desired files and/or directories as usual.
Migrating Your Current Purdue IT Research Computing Home Directory to the New Gilbreth Home Directory
In an upcoming maintenance, the Gilbreth home directory and its contents will become specific to the Gilbreth and will no longer be available on other RCAC machines. New Gilbreth home directories will be given to all Gilbreth users, and these home directories will be empty. The new home directories on Gilbreth are already available and are located at /home-new/$USER
. There will be no automatic copying or synchronization between your current Gilbreth home (also referred to as your main RCAC home directory) and your new Gilbreth home directories. At your discretion, you can manually copy all or parts of your current Gilbreth home directory to your new Gilbreth home directory using one of the methods described below.
Please note that copying may fail if the size of your main research computing home directory is larger than the new Gilbreth one's quota of 25 GB. Please check usage and limits before proceeding!
Link to section 'Complete copy' of 'Migrating Your Current Purdue IT Research Computing Home Directory to the New Gilbreth Home Directory' Complete copy
For your convenience, a custom tool copy-rcac-home
is provided to simplify at-will duplication of your main research computing home directory into Gilbreth. The tool performs a complete 1-to-1 copy using rsync -auH
(with exception of a narrow subset of system-specific service files).
To use the tool, simply type copy-rcac-home
in a terminal window on a Gilbreth front-end or compute node:
$ copy-rcac-home
This script will copy entire contents of your main RCAC
home directory into your Gilbreth cluster's $HOME.
Note: copying may fail if the size of your RCAC home directory
is larger than your quota on the Gilbreth one (25GB).
BEFORE PROCEEDING, please run 'myquota' command on another
cluster to see your usage there and judge whether it would fit!
Would you like to proceed? [Y/n]:
At this stage answering yes
will proceed with copying, or you can respond with a no
(or Ctrl-C
) to cancel. See copy-rcac-home --help
for more details on the tool.
Link to section 'Partial copy' of 'Migrating Your Current Purdue IT Research Computing Home Directory to the New Gilbreth Home Directory' Partial copy
Desired parts (or whole) of your research computing home directories can be copied to Gilbreth via any of the home directories' supported transfer methods, such as SCP, SFTP, rsync, or Globus.
- Example: recursive copying of a subdirectory from your current home directory on Gilbreth into the new Gilbreth home using
scp
andcp
.(if you are on Gilbreth) $ cp -pr somedirectory/ /home-new/$USER/ (if you are on another cluster) $ scp -pr somedirectory/ $USER@gilbreth.rcac.purdue.edu:/home-new/$USER
- Example: copying using Globus.
Search collections for "Purdue Research Computing - Home Directories" and "Purdue Gilbreth Cluster - Home Directories" endpoints, respectively, then transfer desired files and/or directories as usual. For hidden files such as a
.bashrc
file, you will need to make sure to toggle the "Show Hidden Items" button shown below.
Lost File Recovery
Gilbreth is protected against accidental file deletion through a series of snapshots taken every night just after midnight. Each snapshot provides the state of your files at the time the snapshot was taken. It does so by storing only the files which have changed between snapshots. A file that has not changed between snapshots is only stored once but will appear in every snapshot. This is an efficient method of providing snapshots because the snapshot system does not have to store multiple copies of every file.
These snapshots are kept for a limited time at various intervals. RCAC keeps nightly snapshots for 7 days, weekly snapshots for 4 weeks, and monthly snapshots for 3 months. This means you will find snapshots from the last 7 nights, the last 4 Sundays, and the last 3 first of the months. Files are available going back between two and three months, depending on how long ago the last first of the month was. Snapshots beyond this are not kept.
Only files which have been saved during an overnight snapshot are recoverable. If you lose a file the same day you created it, the file is not recoverable because the snapshot system has not had a chance to save the file.
Snapshots are not a substitute for regular backups. It is the responsibility of the researchers to back up any important data to the Fortress Archive. Gilbreth does protect against hardware failures or physical disasters through other means however these other means are also not substitutes for backups.
Files in scratch directories are not recoverable. Files in scratch directories are not backed up. If you accidentally delete a file, a disk crashes, or old files are purged, they cannot be restored.
Gilbreth offers several ways for researchers to access snapshots of their files.
flost
If you know when you lost the file, the easiest way is to use the flost command. This tool is available from any RCAC resource. If you do not have access to a compute cluster, any Data Depot user may use an SSH client to connect to gilbreth.rcac.purdue.edu and run this command.
To run the tool you will need to specify the location where the lost file was with the -w argument:
$ flost -w /depot/mylab
Replace mylab with the name of your lab's Gilbreth directory. If you know more specifically where the lost file was you may provide the full path to that directory.
This tool will prompt you for the date on which you lost the file or would like to recover the file from. If the tool finds an appropriate snapshot it will provide instructions on how to search for and recover the file.
If you are not sure what date you lost the file you may try entering different dates into the flost to try to find the file or you may also manually browse the snapshots as described below.
Manual Browsing
You may also search through the snapshots by hand on the Gilbreth filesystem if you are not sure what date you lost the file or would like to browse by hand. Snapshots can be browsed from any RCAC resource. If you do not have access to a compute cluster, any Gilbreth user may use an SSH client to connect to gilbreth.rcac.purdue.edu and browse from there. The snapshots are located at /depot/.snapshots
on these resources.
You can also mount the snapshot directory over Samba (or SMB, CIFS) on Windows or Mac OS X. Mount (or map) the snapshot directory in the same way as you did for your main Gilbreth space substituting the server name and path for \\datadepot.rcac.purdue.edu\depot\.winsnaps
(Windows) or smb://datadepot.rcac.purdue.edu/depot/.winsnaps
(Mac OS X).
Once connected to the snapshot directory through SSH or Samba, you will see something similar to this:
SSH to gilbreth.rcac.purdue.edu | Samba mount on datadepot.rcac.purdue.edu |
---|---|
|
![]() |
Each of these directories is a snapshot of the entire Gilbreth filesystem at the timestamp encoded into the directory name. The format for this timestamp is year, two digits for month, two digits for day, followed by the time of the day.
You may cd into any of these directories where you will find the entire Gilbreth filesystem. Use cd to continue into your lab's Gilbreth space and then you may browse the snapshot as normal.
If you are browsing these directories over a Samba network drive you can simply drag and drop the files over into your live Data Depot folder.
Once you find the file you are looking for, use cp to copy the file back into your lab's live Gilbreth space. Do not attempt to modify files directly in the snapshot directories.
Windows
If you use Gilbreth through "network drives" on Windows you may recover lost files directly from within Windows:
- Open the folder that contained the lost file.
- Right click inside the window and select "Properties".
- Click on the "Previous Versions" tab.
- A list of snapshots will be displayed.
- Select the snapshot from which you wish to restore.
- In the new window, locate the file you wish to restore.
- Simply drag the file or folder to their correct locations.
In the "Previous Versions" window the list contains two columns. The first column is the timestamp on which the snapshot was taken. The second column is the date on which the selected file or folder was last modified in that snapshot. This may give you some extra clues to which snapshot contains the version of the file you are looking for.
Mac OS X
Mac OS X does not provide any way to access the Gilbreth snapshots directly. To access the snapshots there are two options: browse the snapshots by hand through a network drive mount or use an automated command-line based tool.
To browse the snapshots by hand, follow the directions outlined in the Manual Browsing section.
To use the automated command-line tool, log into a compute cluster or into the host gilbreth.rcac.purdue.edu (which is available to all Gilbreth users) and use the flost tool. On Mac OS X you can use the built-in SSH terminal application to connect.
- Open the Applications folder from Finder.
- Navigate to the Utilities folder.
- Double click the Terminal application to open it.
- Type the following command when the terminal opens.
Replace myusername with your Purdue career account username and provide your password when prompted.$ ssh myusername@gilbreth.rcac.purdue.edu
Once logged in use the flost tool as described above. The tool will guide you through the process and show you the commands necessary to retrieve your lost file.
Gateway (Open OnDemand)
Gilbreth's Gateway is an open-source HPC portal developed by the Ohio Supercomputing Center. Open OnDemand allows one to interact with HPC resources through a web browser and easily manage files, submit jobs, and interact with graphical applications directly in a browser, all with no software to install. Gilbreth has an instance of OnDemand available that can be accessed via gateway.gilbreth.rcac.purdue.edu.
Link to section 'Logging In' of 'Gateway (Open OnDemand)' Logging In
To log into Gateway:
- Navigate to gateway.gilbreth.rcac.purdue.edu
- Log in using your Career account username and Purdue Login Duo client.
On the splash page you will see a quota usage report. If you are over 90% on any of your quotas a warning will be displayed. This information will update every 10-15 minutes while you are active on Gateway.
Link to section 'Apps' of 'Gateway (Open OnDemand)' Apps
There are a number of built-in apps in Gateway that can be accessed from the top menu bar. Below are links to documentation on each app.
Interactive Apps
There are several interactive apps available through Gateway that can be accessed through the Interactive Apps dropdown menu. These apps are provided with a basic node and software configuration as a 'quick-launch' option to get your work up and running quickly. For simplicity, minimal options are provided - these apps are not intended for complex configuration/customization scenarios.
After you a submit an interactive app to the queue, Gateway will track and manage the session. Once it starts, you may connect and disconnect from the session in your browser, leaving the job running while you log out of your browser.
Each of the available apps are documented through the following links.
Compute Node Desktop
The Compute Node Desktop app will launch a graphical desktop session on a compute node. This is similar to using Thinlinc, however, this gives you a desktop directly on a compute node instead on a front-end. This app is useful if you have a custom application or application not directly available as an interactive app you would like to run inside Gateway.
To launch a desktop session on a compute node, select the Gilbreth Compute Desktop app. From the submit form, select from the available options - the queue to which you wish to submit and the number of wallclock hours you wish to have job running. There is also a checkbox that enable a notification to your email when the job starts.
After the interactive job is submitted you will be taken to your list of active interactive app sessions. You can monitor the status of the job from here until it starts, or if you enabled the email notification, watch your Purdue email for the notification the job has started.
Once it is indicated the job has started you can connect to the desktop with the "Launch noVNC in New Tab" button. The session will be terminated after the wallclock hours you specified have elapsed or you terminate the session early with the "Delete" button from the list of sessions. Deleting the session when you are finished will free up queue resources for your lab mates and other users on the system.
Windows Desktop
The Windows Desktop app will launch a Windows desktop session on a compute node. This is similar to using the Windows menu launcher through Thinlinc, however, this gives you a Windows desktop directly on a compute node instead on a front-end.
To launch a Windows session on a compute node, select the Windows Desktop app. From the submit form, select from the available options - choose from the basic Windows configuration or the GIS configured image, the queue to which you wish to submit, and the number of wallclock hours you wish to have job running. There is also a checkbox that enable a notification to your email when the job starts.
This will create a file in your scratch space called windows-base.qcow2
or windows-gis.qcow2
. If the file already exists, the existing image will be restarted. You can delete or rename the image at any time through the Files App to generate a fresh image. You can only have one instance of the image running at a time or corruption will occur. There are lock files to prevent this, but be mindful of this restriction. It is also recommended you make periodic backups of the image if you are making any modifications to it.
After the interactive job is submitted you will be taken to your list of active interactive app sessions. You can monitor the status of the job from here until it starts, or if you enabled the email notification, watch your Purdue email for the notification the job has started.
Once it is indicated the job has started you can connect to the desktop with the "Launch noVNC in New Tab" button. The session will be terminated after the wallclock hours you specified have elapsed or you terminate the session early with the "Delete" button from the list of sessions. Deleting the session when you are finished will free up queue resources for your lab mates and other users on the system.
Jupyter Notebook
The Notebook app will launch a Notebook session on a compute node and allow you to connect directly to it in a web browser.
To launch a Notebook session on a compute node, select the Notebook app. From the submit form, select from the available options:
- Queue: This is a dropdown menu from which you can select a queue from all of the queues to which you have permission to submit.
- Walltime: This is a field which expects a number and represents how many hours you want to keep the session running. Note that this value should not exceed the maximum value given next to the selected queue name from the queue dropdown menu.
- Number of Cores/GPUs: This is a field which expects a number and represents the number of your resources your session is requesting. Note that the amount of memory allocated for your session is proportional to the number of cores or GPUs that you request for your job, so if your session is running out of memory, consider increasing this value.
- Use Jupyter Lab: This is a checkbox which, when checked, will run Jupyter Lab instead of Jupyter Notebook. Both of these applications are interfaces to Jupyter, and you can launch Jupyter notebooks from within Jupyter Lab. Jupyter Notebook is more "barebones" while Jupyter Lab has additional features such as the ability to interact with additional file types.
- E-mail Notice: This is a checkbox which, when checked, will send you an e-mail notification to your Purdue e-mail that your session is ready when the scheduler has found resources to dedicate to your session.
After the interactive job is submitted you will be taken to your list of active interactive app sessions. You can monitor the status of the job from here until it starts, or if you enabled the email notification, watch your Purdue email for the notification the job has started.
Once it is indicated the job has started you can connect to the desktop with the "Connect to Jupyter" button. Once connected, you can create new notebooks, selecting the currently available Anaconda versions available as modules, and any personally created Notebook kernels.
Often times you may want to use one of your existing Anaconda environments within your Jupyter session to use libraries specific to your workflow. In order to do so, you must ensure that the Anaconda environment you want to use contains the Python packages "IPyKernel" and "IPython" which are packages that are required by Jupyter. When you create a Jupyter session, Open OnDemand will check through your existing Anaconda environments and create a Jupyter kernel for any Anaconda environment that contains these two packages, and you will be able to select to use that kernel from within the application.
The session will be terminated after the number of hours you specified have elapsed or you terminate the session early with the "Delete" button from the list of sessions. Deleting the session when you are finished will free up queue resources for your lab mates and other users on the system.
Jupyter Notebook - Deep Neural Networks Demo (GPU)
The Notebook app will launch a Notebook session on a compute node and allow you to connect directly to it in a web browser. It can be used to run GPU applications such as Tensorflow and Keras. Below is a demo of this to get you started.
- Download the demo notebook to your computer.
- Launch a Notebook session from the Gateway Interactive Apps menu:
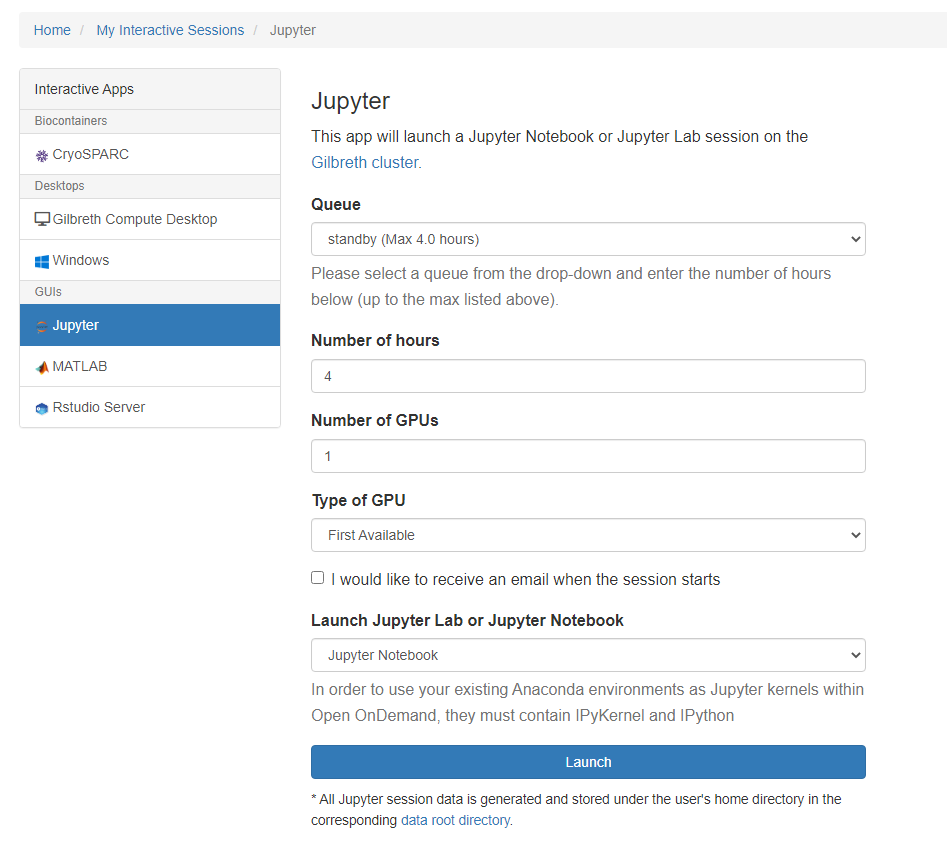
- Select the queue to which you wish to submit and enter the number of wallclock hours you require. Your notebook will be terminated after this number of hours elapses.
- Click Launch.
- Wait for your interactive session to change to Running state. This may take some time depending on how busy the queue and system is.
- Click on 'Connect to Jupyter' once the button appears.
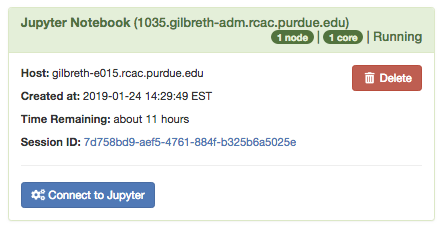
- Once in Jupyter, select 'Upload' in the upper right corner. You may wish to create a folder or change into a different directory to put the demo notebook first.
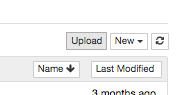
- Select the demo notebook file you downloaded earlier. Click the blue Upload button to complete the upload. Then click the
dnn.ipynb
item from the file list to launch the notebook. - You should now have the notebook loaded and you should be able to re-execute the code cells, or modify them to your needs.
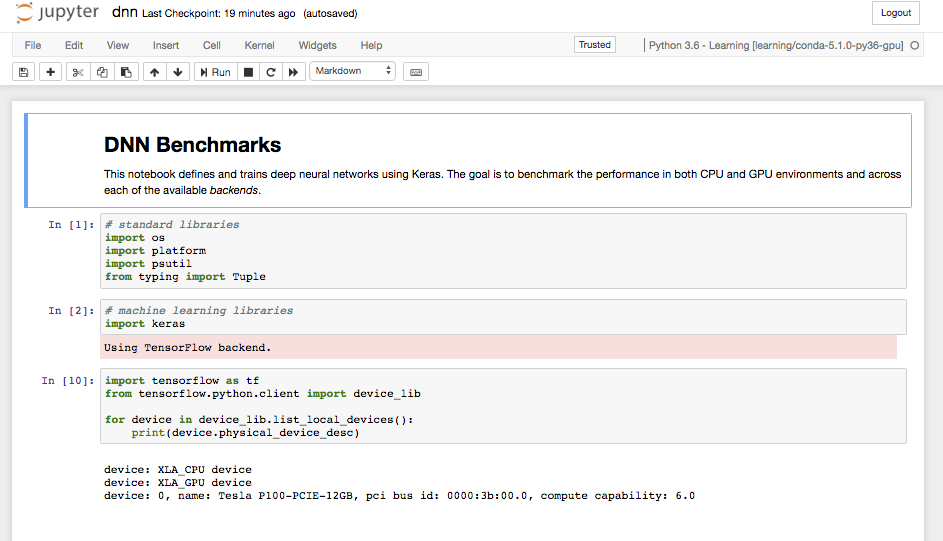
MATLAB
The MATLAB app will launch a MATLAB session on a compute node and allow you to connect directly to it in a web browser.
To launch a MATLAB session on a compute node, select the MATLAB app. From the submit form, select from the available options - the version of MATLAB you are interested in running, the queue to which you wish to submit, and the number of wallclock hours you wish to have job running. There is also a checkbox that enable a notification to your email when the job starts.
After the interactive job is submitted you will be taken to your list of active interactive app sessions. You can monitor the status of the job from here until it starts, or if you enabled the email notification, watch your Purdue email for the notification the job has started.
Once it is indicated the job has started you can connect to the desktop with the "Launch noVNC in New Tab" button. The session will be terminated after the wallclock hours you specified have elapsed or you terminate the session early with the "Delete" button from the list of sessions. Deleting the session when you are finished will free up queue resources for your lab mates and other users on the system.
RStudio Server
The RStudio app will launch a RStudio session on a compute node and allow you to connect directly to it in a web browser.
To launch a RStudio session on a compute node, select the RStudio app. From the submit form, select from the available options - the queue to which you wish to submit, and the number of wallclock hours you wish to have job running. There is also a checkbox that enable a notification to your email when the job starts.
After the interactive job is submitted you will be taken to your list of active interactive app sessions. You can monitor the status of the job from here until it starts, or if you enabled the email notification, watch your Purdue email for the notification the job has started.
Once it is indicated the job has started you can connect to the desktop with the "Connect to RStudio Server" button. The session will be terminated after the wallclock hours you specified have elapsed or you terminate the session early with the "Delete" button from the list of sessions. Deleting the session when you are finished will free up queue resources for your lab mates and other users on the system.
Files
The Files app will let you access your files in your Home Directory, Scratch, and Data Depot spaces. The app lets you manage create, manage, and delete files and directories from your web browser. Navigate by double clicking on folders in the file explorer or by using the file tree on the left.
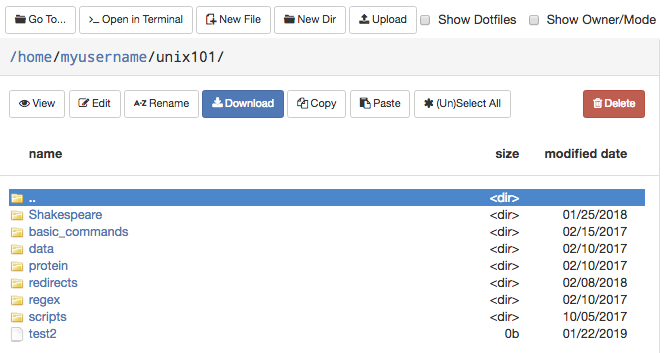
On the top row, there are buttons to:
- Go To: directly input a directory to navigate to
- Open in Terminal: launches the Shell app and navigates you to the current directory in the terminal
- New File: creates a new, empty file
- New Dir: creates a new, empty directory
- Upload: upload a file from your computer
Note: File uploads from your browser are limited to 100 GB per file. Be mindful that uploads over a few gigabytes may be unreliable through your browser, especially from off-campus connections. For very large files or off-campus transfers alternative methods such as Globus are highly recommended.
The second row of buttons lets you perform typical file management operations. The Edit button will open files in a fully fledged browser based text editor - it features syntax highlighting and vim and Emacs key bindings.
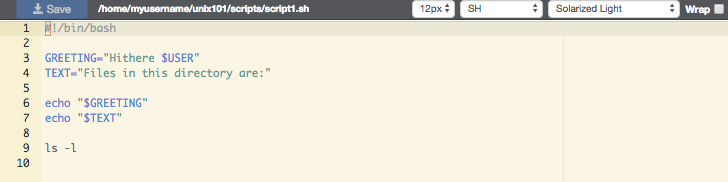
Jobs
There are two apps under the Jobs apps: Active Jobs and Job Composer. These are detailed below.
Link to section 'Active Jobs' of 'Jobs' Active Jobs
This shows you active SLURM jobs currently on the cluster. The default view will show you your current jobs, similar to squeue -u rices
. Using the button labeled "Your Jobs" in the upper right allows you to select different filters by queue (account). All accounts output by slist
will appear for you here. Using the arrow on the left hand side will expand the full job details.

Link to section 'Job Composer' of 'Jobs' Job Composer
The Job Composer app allows you to create and submit jobs to the cluster. You can select from pre-defined templates (most of these are taken from the User Guide examples) or you can create your own templates for frequently used workflows.
Link to section 'Creating Job from Existing Template' of 'Jobs' Creating Job from Existing Template
Click "New Job" menu, then select "From Template":
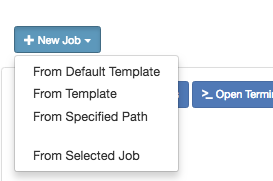
Then select from one of the available templates.
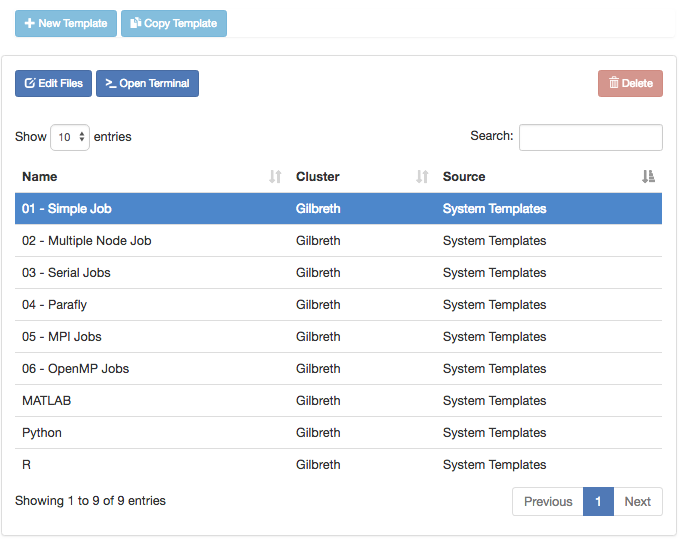
Click 'Create New Job' in second pane.
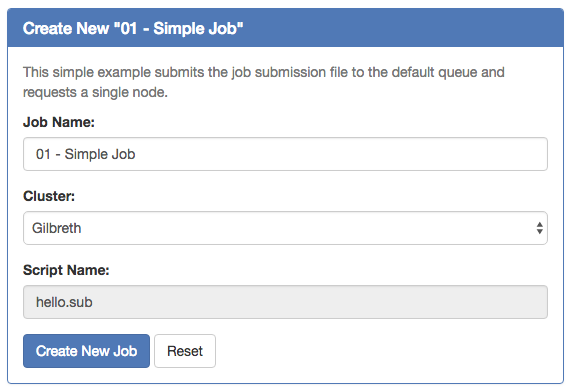
Your new job should be selected in your list of jobs. In the 'Submit Script' pane you can see the job script that was generated with an 'Open Editor' link to open the script in the built-in editor. Open the file in the editor and edit the script as necessary. By default the job will specify standby queue - this should be changed as appropriate, along with the node and walltime requests.
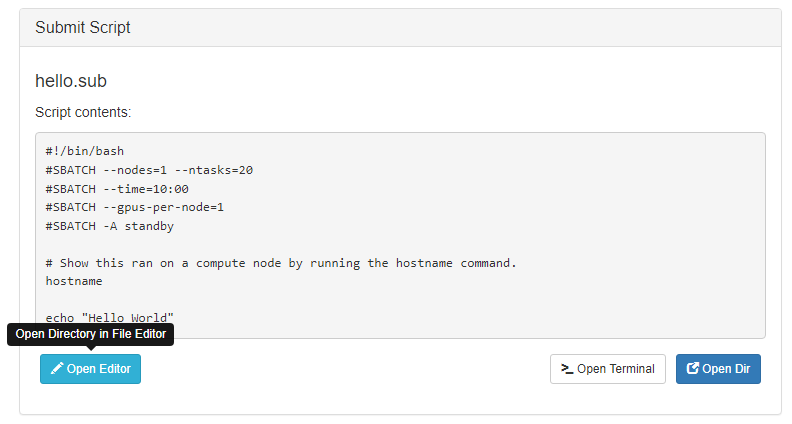
When you are finished with editing the job and are ready to submit, click the green 'Submit' button at the top of the job list. You can monitor progress from here or from the Active Jobs app. Once completed, you should see the output files appear:
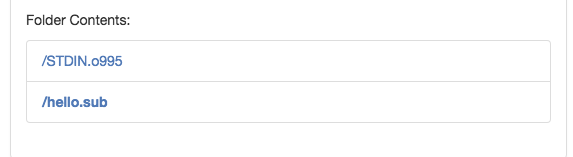
Clicking on one of the output files will open it in the file editor for your viewing.
Link to section 'Creating New Template' of 'Jobs' Creating New Template
First, prepare a template directory containing a template submission script along with any input files. Then, to import the job into the Job Composer app, click the 'Create New Template' button. Fill in the directory containing your template job script and files in the first box. Give it an appropriate name and notes.
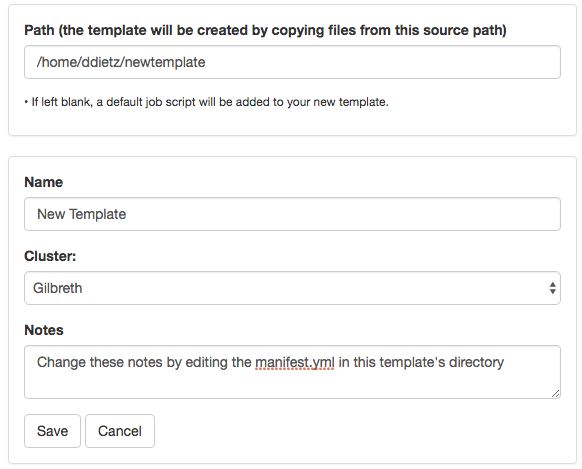
This template will now appear in your list of templates to choose from when composing jobs. You can now go create and submit a job from this new template.
Cluster Tools
The Cluster Tools menu contains cluster utilities. At the moment, only a terminal app is provided. Additional apps may be developed and provided in the future.
Link to section 'Shell Access' of 'Cluster Tools' Shell Access
Launching the shell app will provide you with a web-based terminal session on the cluster front-end. This is equivalent to using a standalone SSH client to connect to gilbreth.rcac.purdue.edu
where you are connected to one several front-ends. The normal acceptable front-end use policy applies to access through the web-app. X11 Forwarding is not supported. Use of one of the interactive apps is recommended for graphical applications.
Software
Link to section 'Environment module' of 'Software' Environment module
Link to section 'Software catalog' of 'Software' Software catalog
Compiling Source Code
Documentation on compiling source code on Gilbreth.
Compiling Serial Programs
A serial program is a single process which executes as a sequential stream of instructions on one processor core. Compilers capable of serial programming are available for C, C++, and versions of Fortran.
Here are a few sample serial programs:
- serial_hello.f
- serial_hello.f90
- serial_hello.f95
- serial_hello.c
-
To load a compiler, enter one of the following:
$ module load intel
$ module load gcc
Language | Intel Compiler | GNU Compiler | |
---|---|---|---|
Fortran 77 |
|
|
|
Fortran 90 |
|
|
|
Fortran 95 |
|
|
|
C |
|
|
|
C++ |
|
|
The Intel and GNU compilers will not output anything for a successful compilation. Also, the Intel compiler does not recognize the suffix ".f95".
Compiling MPI Programs
OpenMPI and Intel MPI (IMPI) are implementations of the Message-Passing Interface (MPI) standard. Libraries for these MPI implementations and compilers for C, C++, and Fortran are available on all clusters.
Language | Header Files |
---|---|
Fortran 77 |
|
Fortran 90 |
|
Fortran 95 |
|
C |
|
C++ |
|
Here are a few sample programs using MPI:
To see the available MPI libraries:
$ module avail openmpi
$ module avail impi
Language | Intel MPI | OpenMPI |
---|---|---|
Fortran 77 |
|
|
Fortran 90 |
|
|
Fortran 95 |
|
|
C |
|
|
C++ |
|
|
The Intel and GNU compilers will not output anything for a successful compilation. Also, the Intel compiler does not recognize the suffix ".f95".
Here is some more documentation from other sources on the MPI libraries:
Compiling OpenMP Programs
All compilers installed on Brown include OpenMP functionality for C, C++, and Fortran. An OpenMP program is a single process that takes advantage of a multi-core processor and its shared memory to achieve a form of parallel computing called multithreading. It distributes the work of a process over processor cores in a single compute node without the need for MPI communications.
Language | Header Files |
---|---|
Fortran 77 |
|
Fortran 90 |
|
Fortran 95 |
|
C |
|
C++ |
|
Sample programs illustrate task parallelism of OpenMP:
A sample program illustrates loop-level (data) parallelism of OpenMP:
To load a compiler, enter one of the following:
$ module load intel
$ module load gcc
Language | Intel Compiler | GNU Compiler |
---|---|---|
Fortran 77 |
|
|
Fortran 90 |
|
|
Fortran 95 |
|
|
C |
|
|
C++ |
|
|
The Intel and GNU compilers will not output anything for a successful compilation. Also, the Intel compiler does not recognize the suffix ".f95".
Here is some more documentation from other sources on OpenMP:
Compiling Hybrid Programs
A hybrid program combines both MPI and shared-memory to take advantage of compute clusters with multi-core compute nodes. Libraries for OpenMPI and Intel MPI (IMPI) and compilers which include OpenMP for C, C++, and Fortran are available.
Language | Header Files |
---|---|
Fortran 77 |
|
Fortran 90 |
|
Fortran 95 |
|
C |
|
C++ |
|
A few examples illustrate hybrid programs with task parallelism of OpenMP:
This example illustrates a hybrid program with loop-level (data) parallelism of OpenMP:
To see the available MPI libraries:
$ module avail impi
$ module avail openmpi
The following tables illustrate how to compile your hybrid (MPI/OpenMP) program. Any compiler flags accepted by Intel ifort/icc compilers are compatible with their respective MPI compiler.
Language | Command |
---|---|
Fortran 77 |
|
Fortran 90 |
|
Fortran 95 |
|
C |
|
C++ |
|
Language | Command |
---|---|
Fortran 77 |
|
Fortran 90 |
|
Fortran 95 |
|
C |
|
C++ |
|
The Intel and GNU compilers will not output anything for a successful compilation. Also, the Intel compiler does not recognize the suffix .f95
.
Intel MKL Library
Intel Math Kernel Library (MKL) contains ScaLAPACK, LAPACK, Sparse Solver, BLAS, Sparse BLAS, CBLAS, GMP, FFTs, DFTs, VSL, VML, and Interval Arithmetic routines. MKL resides in the directory stored in the environment variable MKL_HOME, after loading a version of the Intel compiler with module.
By using module load to load an Intel compiler your environment will have several variables set up to help link applications with MKL. Here are some example combinations of simplified linking options:
$ module load intel
$ echo $LINK_LAPACK
-L${MKL_HOME}/lib/intel64 -lmkl_intel_lp64 -lmkl_intel_thread -lmkl_core -liomp5 -lpthread
$ echo $LINK_LAPACK95
-L${MKL_HOME}/lib/intel64 -lmkl_lapack95_lp64 -lmkl_blas95_lp64 -lmkl_intel_lp64 -lmkl_intel_thread -lmkl_core -liomp5 -lpthread
RCAC recommends you use the provided variables to define MKL linking options in your compiling procedures. The Intel compiler modules also provide two other environment variables, LINK_LAPACK_STATIC and LINK_LAPACK95_STATIC that you may use if you need to link MKL statically.
RCAC recommends that you use dynamic linking of libguide. If so, define LD_LIBRARY_PATH such that you are using the correct version of libguide at run time. If you use static linking of libguide, then:
- If you use the Intel compilers, link in the libguide version that comes with the compiler (use the -openmp option).
- If you do not use the Intel compilers, link in the libguide version that comes with the Intel MKL above.
Here are some more documentation from other sources on the Intel MKL:
Compiling GPU Programs
The Gilbreth cluster nodes contain 2 GPUs that support CUDA and OpenCL. See the detailed hardware overview for the specifics on the GPUs in Gilbreth. This section focuses on using CUDA.
A simple CUDA program has a basic workflow:
- Initialize an array on the host (CPU).
- Copy array from host memory to GPU memory.
- Apply an operation to array on GPU.
- Copy array from GPU memory to host memory.
Here is a sample CUDA program:
Both front-ends and GPU-enabled compute nodes have the CUDA tools and libraries available to compile CUDA programs. To compile a CUDA program, load CUDA, and use nvcc to compile the program:
$ module load gcc/11.4.1 cuda/12.6.0
$ nvcc gpu_hello.cu -o gpu_hello
./gpu_hello
No GPU specified, using first GPUhello, world
The example illustrates only how to copy an array between a CPU and its GPU but does not perform a serious computation.
The following program times three square matrix multiplications on a CPU and on the global and shared memory of a GPU:
$ module load cuda
$ nvcc mm.cu -o mm
$ ./mm 0
speedup
-------
Elapsed time in CPU: 6555.2 milliseconds
Elapsed time in GPU (global memory): 32.9 milliseconds 199.1
Elapsed time in GPU (shared memory): 3.0 milliseconds 2191.8
For best performance, the input array or matrix must be sufficiently large to overcome the overhead in copying the input and output data to and from the GPU.
For more information about NVIDIA, CUDA, and GPUs:
Running Jobs
There is one method for submitting jobs to Gilbreth. You may use SLURM to submit jobs to a partition on Gilbreth. SLURM performs job scheduling. Jobs may be any type of program. You may use either the batch or interactive mode to run your jobs. Use the batch mode for finished programs; use the interactive mode only for debugging.
In this section, you'll find a few pages describing the basics of creating and submitting SLURM jobs. As well, a number of example SLURM jobs that you may be able to adapt to your own needs.
Basics of SLURM Jobs
The Simple Linux Utility for Resource Management (SLURM) is a system providing job scheduling and job management on compute clusters. With SLURM, a user requests resources and submits a job to a queue. The system will then take jobs from queues, allocate the necessary nodes, and execute them.
Do NOT run large, long, multi-threaded, parallel, or CPU-intensive jobs on a front-end login host. All users share the front-end hosts, and running anything but the smallest test job will negatively impact everyone's ability to use Gilbreth. Always use SLURM to submit your work as a job.
Link to section 'Submitting a Job' of 'Basics of SLURM Jobs' Submitting a Job
The main steps to submitting a job are:
Follow the links below for information on these steps, and other basic information about jobs. A number of example SLURM jobs are also available.
Queues
Link to section '"mylab" Queues' of 'Queues' "mylab" Queues
Gilbreth, as a community cluster, has one or more queues dedicated to and named after each partner who has purchased access to the cluster. These queues provide partners and their researchers with priority access to their portion of the cluster. Jobs in these queues are typically limited to 336 hours. The expectation is that any jobs submitted to your research lab queues will start within 4 hours, assuming the queue currently has enough capacity for the job (that is, your lab mates aren't using all of the cores currently).
Link to section 'Training Queue' of 'Queues' Training Queue
If your job can scale well to multiple GPUs and it requires longer than 24 hours, then use the training queue. Since the training nodes have specialty hardware and are few in number, these are restricted to users whose workloads can scale well with the number of GPUs. Please note that staff may ask you to provide evidence that your jobs can fully utilize the GPUs, before granting access to this queue. The Max wall time is 3 days, the number of jobs a user could concurrently run is 2, and the total number of consumed GPUs is 8. There are only 5 nodes in this queue, so you may have to wait a considerable amount of time before your job is scheduled.
Link to section 'Standby Queue' of 'Queues' Standby Queue
Additionally, community clusters provide a "standby" queue which is available to all cluster users. This "standby" queue allows users to utilize portions of the cluster that would otherwise be idle, but at a lower priority than partner-queue jobs, and with a relatively short time limit, to ensure "standby" jobs will not be able to tie up resources and prevent partner-queue jobs from running quickly. Jobs in standby are limited to 4 hours. There is no expectation of job start time. If the cluster is very busy with partner queue jobs, or you are requesting a very large job, jobs in standby may take hours or days to start.
Link to section 'Debug Queue' of 'Queues' Debug Queue
The debug queue allows you to quickly start small, short, interactive jobs in order to debug code, test programs, or test configurations. You are limited to one running job at a time in the queue, and you may run up to two GPUs for 30 minutes. The expectation is that debug jobs should start within a couple of minutes, assuming all of its dedicated nodes are not taken by others.
Link to section 'List of Queues' of 'Queues' List of Queues
To see a list of all queues on Gilbreth that you may submit to, use the slist command
This lists each queue you can submit to, the number of nodes allocated to the queue, how many are available to run jobs, and the maximum walltime you may request. Options to the command will give more detailed information. This command can be used to get a general idea of how busy an individual queue is and how long you may have to wait for your job to start.
The default output mode ofslist
command shows the available GPU counts in queues:
$ slist
Current Number of GPUs Node
Account Total Queue Run Free Max Walltime Type
============== ================================= ============== ======
debug 183 0 0 183 00:30:00 B,D,E,F,G,H,I
standby 183 77 55 98 04:00:00 B,D,E,F,G,H,I
training 20 0 8 12 3-00:00:00 C,J
mylab 80 0 0 80 14-00:00:00 F
To check the number of CPUs mounted on each queue, please use slist -c
command.
Link to section 'Summary of Queues' of 'Queues' Summary of Queues
Gilbreth contains several queues and heterogeneous hardware consisting of different number of cores and different GPU models. Some queues are backed by only one node type, but some queues may land on multiple node types. On queues that land on multiple node types, you will need to be mindful of your resource request. Below are the current combinations of queues, GPU types, and resources you may request.
Queue | GPU Type | Number of GPUs per node | Intended use-case | Max walltime | Max GPUs pre user concurrently | Max Jobs running per user |
---|---|---|---|---|---|---|
Standby | V100 (16 GB), V100 (32 GB), A100 (40 GB), A100 (80 GB), A10 (24 GB), A30 (24 GB) | 16 (2), 40 (2), 128 (2), 128 (2), 32 (3), 24/16 (3) | Short to moderately long jobs | 4 hours | 16 | 16 |
training | V100 (32 GB, NVLink), A100 (80GB, NVLink) | 20 (4), 128 (4) | Long jobs that can scale well to multiple GPUs, such as Deep Learning model training | 3 days | 8 | 2 |
debug | V100 (16 GB), V100 (32 GB), A100 (40 GB), A100 (80 GB), A10 (24 GB), A30 (24 GB) | 16 (2), 40 (2), 128 (2), 128 (2), 32 (3), 24/16 (3) | Quick testing | 30 mins | 2 | 1 |
"mylab" | Based on Purchase | Based on Purchase | There will be a separate queue for each type of GPU the partners have purchased. | 2 Weeks | Amount Purchased | Based on Purchase |
Job Submission Script
To submit work to a SLURM queue, you must first create a job submission file. This job submission file is essentially a simple shell script. It will set any required environment variables, load any necessary modules, create or modify files and directories, and run any applications that you need:
#!/bin/bash
# FILENAME: myjobsubmissionfile
# Loads Matlab and sets the application up
module load matlab
# Change to the directory from which you originally submitted this job.
cd $SLURM_SUBMIT_DIR
# Runs a Matlab script named 'myscript'
matlab -nodisplay -singleCompThread -r myscript
Once your script is prepared, you are ready to submit your job.
Link to section 'Job Script Environment Variables' of 'Job Submission Script' Job Script Environment Variables
Name | Description |
---|---|
SLURM_SUBMIT_DIR | Absolute path of the current working directory when you submitted this job |
SLURM_JOBID | Job ID number assigned to this job by the batch system |
SLURM_JOB_NAME | Job name supplied by the user |
SLURM_JOB_NODELIST | Names of nodes assigned to this job |
SLURM_CLUSTER_NAME | Name of the cluster executing the job |
SLURM_SUBMIT_HOST | Hostname of the system where you submitted this job |
SLURM_JOB_PARTITION | Name of the original queue to which you submitted this job |
Submitting a Job
Once you have a job submission file, you may submit this script to SLURM using the sbatch
command. SLURM will find, or wait for, available resources matching your request and run your job there.
To submit your job to one compute node:
$ sbatch --nodes=1 --gpus-per-node=1 myjobsubmissionfile
Slurm uses the word 'Account' and the option '-A' to specify different batch queues. To submit your job to a specific queue:
$ sbatch --nodes=1 --gpus-per-node=1 -A standby myjobsubmissionfile
On Gilbreth, you must specify the number of GPUs with the --gpus-per-node
option.
By default, each job receives 30 minutes of wall time, or clock time. If you know that your job will not need more than a certain amount of time to run, request less than the maximum wall time, as this may allow your job to run sooner. To request the 1 hour and 30 minutes of wall time:
$ sbatch -t 1:30:00 --nodes=1 --gpus-per-node=1 -p standby myjobsubmissionfile
The --nodes
value indicates how many compute nodes you would like for your job.
Each compute node in Gilbreth has various cores per node. Refer to the Hardware Overview and Queue Overview for details.
In some cases, you may want to request multiple nodes. To utilize multiple nodes, you will need to have a program or code that is specifically programmed to use multiple nodes such as with MPI. Simply requesting more nodes will not make your work go faster. Your code must support this ability.
To request 2 compute nodes:
$ sbatch --nodes=2 --gpus-per-node=1 myjobsubmissionfile
By default, jobs on Gilbreth will share nodes with other jobs.
To submit a job using 1 compute node with 4 tasks, each using the default 1 core and 1 GPU per node:
$ sbatch --nodes=1 --ntasks=4 --gpus-per-node=1 myjobsubmissionfile
If more convenient, you may also specify any command line options to sbatch
from within your job submission file, using a special form of comment:
#!/bin/sh -l
# FILENAME: myjobsubmissionfile
#SBATCH -A myqueuename
#SBATCH --nodes=1 --gpus-per-node=1
#SBATCH --time=1:30:00
#SBATCH --job-name myjobname
# Print the hostname of the compute node on which this job is running.
/bin/hostname
If an option is present in both your job submission file and on the command line, the option on the command line will take precedence.
After you submit your job with SBATCH
, it may wait in queue for minutes, hours, or even weeks. How long it takes for a job to start depends on the specific queue, the resources and time requested, and other jobs already waiting in that queue requested as well. It is impossible to say for sure when any given job will start. For best results, request no more resources than your job requires.
Once your job is submitted, you can monitor the job status, wait for the job to complete, and check the job output.
Job Dependencies
Dependencies are an automated way of holding and releasing jobs. Jobs with a dependency are held until the condition is satisfied. Once the condition is satisfied jobs only then become eligible to run and must still queue as normal.
Job dependencies may be configured to ensure jobs start in a specified order. Jobs can be configured to run after other job state changes, such as when the job starts or the job ends.
These examples illustrate setting dependencies in several ways. Typically dependencies are set by capturing and using the job ID from the last job submitted.
To run a job after job myjobid has started:
sbatch --dependency=after:myjobid myjobsubmissionfile
To run a job after job myjobid ends without error:
sbatch --dependency=afterok:myjobid myjobsubmissionfile
To run a job after job myjobid ends with errors:
sbatch --dependency=afternotok:myjobid myjobsubmissionfile
To run a job after job myjobid ends with or without errors:
sbatch --dependency=afterany:myjobid myjobsubmissionfile
To set more complex dependencies on multiple jobs and conditions:
sbatch --dependency=after:myjobid1:myjobid2:myjobid3,afterok:myjobid4 myjobsubmissionfile
Holding a Job
Sometimes you may want to submit a job but not have it run just yet. You may be wanting to allow lab mates to cut in front of you in the queue - so hold the job until their jobs have started, and then release yours.
To place a hold on a job before it starts running, use the scontrol hold job command:
$ scontrol hold job myjobid
Once a job has started running it can not be placed on hold.
To release a hold on a job, use the scontrol release job command:
$ scontrol release job myjobid
You find the job ID using the squeue command as explained in the SLURM Job Status section.
Checking Job Status
Once a job is submitted there are several commands you can use to monitor the progress of the job.
To see your jobs, use the squeue -u command and specify your username:
(Remember, in our SLURM environment a queue is referred to as an 'Account')
squeue -u myusername
JOBID ACCOUNT NAME USER ST TIME NODES NODELIST(REASON)
182792 standby job1 myusername R 20:19 1 gilbreth-a000
185841 standby job2 myusername R 20:19 1 gilbreth-a001
185844 standby job3 myusername R 20:18 1 gilbreth-a002
185847 standby job4 myusername R 20:18 1 gilbreth-a003
To retrieve useful information about your queued or running job, use the scontrol show job command with your job's ID number. The output should look similar to the following:
scontrol show job 3519
JobId=3519 JobName=t.sub
UserId=myusername GroupId=mygroup MCS_label=N/A
Priority=3 Nice=0 Account=(null) QOS=(null)
JobState=PENDING Reason=BeginTime Dependency=(null)
Requeue=1 Restarts=0 BatchFlag=1 Reboot=0 ExitCode=0:0
RunTime=00:00:00 TimeLimit=7-00:00:00 TimeMin=N/A
SubmitTime=2019-08-29T16:56:52 EligibleTime=2019-08-29T23:30:00
AccrueTime=Unknown
StartTime=2019-08-29T23:30:00 EndTime=2019-09-05T23:30:00 Deadline=N/A
PreemptTime=None SuspendTime=None SecsPreSuspend=0
LastSchedEval=2019-08-29T16:56:52
Partition=workq AllocNode:Sid=mack-fe00:54476
ReqNodeList=(null) ExcNodeList=(null)
NodeList=(null)
NumNodes=1 NumCPUs=2 NumTasks=2 CPUs/Task=1 ReqB:S:C:T=0:0:*:*
TRES=cpu=2,node=1,billing=2
Socks/Node=* NtasksPerN:B:S:C=0:0:*:* CoreSpec=*
MinCPUsNode=1 MinMemoryNode=0 MinTmpDiskNode=0
Features=(null) DelayBoot=00:00:00
OverSubscribe=OK Contiguous=0 Licenses=(null) Network=(null)
Command=/home/myusername/jobdir/myjobfile.sub
WorkDir=/home/myusername/jobdir
StdErr=/home/myusername/jobdir/slurm-3519.out
StdIn=/dev/null
StdOut=/home/myusername/jobdir/slurm-3519.out
Power=
There are several useful bits of information in this output.
JobState
lets you know if the job is Pending, Running, Completed, or Held.RunTime and TimeLimit
will show how long the job has run and its maximum time.SubmitTime
is when the job was submitted to the cluster.NumNodes
,NumCPUs
,NumTasks
andCPUs/Task
are the number of Nodes, CPUs, Tasks, and CPUs per Task are shown.WorkDir
is the job's working directory.StdOut
andStderr
are the locations of stdout and stderr of the job, respectively.Reason
will show why aPENDING
job isn't running. The above error says that it has been requested to start at a specific, later time.
Checking Job Output
Once a job is submitted, and has started, it will write its standard output and standard error to files that you can read.
SLURM catches output written to standard output and standard error - what would be printed to your screen if you ran your program interactively. Unless you specfied otherwise, SLURM will put the output in the directory where you submitted the job in a file named slurm-
followed by the job id
, with the extension out
. For example slurm-3509.out
. Note that both stdout and stderr will be written into the same file, unless you specify otherwise.
If your program writes its own output files, those files will be created as defined by the program. This may be in the directory where the program was run, or may be defined in a configuration or input file. You will need to check the documentation for your program for more details.
Link to section 'Redirecting Job Output' of 'Checking Job Output' Redirecting Job Output
It is possible to redirect job output to somewhere other than the default location with the --error
and --output
directives:
#!/bin/bash
#SBATCH --output=/home/myusername/joboutput/myjob.out
#SBATCH --error=/home/myusername/joboutput/myjob.out
# This job prints "Hello World" to output and exits
echo "Hello World"
Canceling a Job
To stop a job before it finishes or remove it from a queue, use the scancel command:
scancel myjobid
You find the job ID using the squeue command as explained in the SLURM Job Status section.
PBS to Slurm
This is a reference for the most common command, environment variables, and job specification options used by the workload management systems and their equivalents.
Quick Guide
This table lists the most common command, environment variables, and job specification options used by the workload management systems and their equivalents (adapted from http://www.schedmd.com/slurmdocs/rosetta.html).
User Commands | PBS/Torque | Slurm |
---|---|---|
Job submission | qsub [script_file] |
sbatch [script_file] |
Interactive Job | qsub -I |
sinteractive |
Job deletion | qdel [job_id] |
scancel [job_id] |
Job status (by job) | qstat [job_id] |
squeue [-j job_id] |
Job status (by user) | qstat -u [user_name] |
squeue [-u user_name] |
Job hold | qhold [job_id] |
scontrol hold [job_id] |
Job release | qrls [job_id] |
scontrol release [job_id] |
Queue info | qstat -Q |
squeue |
Queue access | qlist |
slist |
Node list | pbsnodes -l |
sinfo -N scontrol show nodes |
Cluster status | qstat -a |
sinfo |
GUI | xpbsmon |
sview |
Environment | PBS/Torque | Slurm |
Job ID | $PBS_JOBID |
$SLURM_JOB_ID |
Job Name | $PBS_JOBNAME |
$SLURM_JOB_NAME |
Job Queue/Account | $PBS_QUEUE |
$SLURM_JOB_ACCOUNT |
Submit Directory | $PBS_O_WORKDIR |
$SLURM_SUBMIT_DIR |
Submit Host | $PBS_O_HOST |
$SLURM_SUBMIT_HOST |
Number of nodes | $PBS_NUM_NODES |
$SLURM_JOB_NUM_NODES |
Number of Tasks | $PBS_NP |
$SLURM_NTASKS |
Number of Tasks Per Node | $PBS_NUM_PPN |
$SLURM_NTASKS_PER_NODE |
Node List (Compact) | n/a | $SLURM_JOB_NODELIST |
Node List (One Core Per Line) | LIST=$(cat $PBS_NODEFILE) |
LIST=$(srun hostname) |
Job Array Index | $PBS_ARRAYID |
$SLURM_ARRAY_TASK_ID |
Job Specification | PBS/Torque | Slurm |
Script directive | #PBS |
#SBATCH |
Queue | -q [queue] |
-A [queue] |
Node Count | -l nodes=[count] |
-N [min[-max]] |
CPU Count | -l ppn=[count] |
-n [count] Note: total, not per node |
Wall Clock Limit | -l walltime=[hh:mm:ss] |
-t [min] OR-t [hh:mm:ss] OR-t [days-hh:mm:ss] |
Standard Output FIle | -o [file_name] |
-o [file_name] |
Standard Error File | -e [file_name] |
-e [file_name] |
Combine stdout/err | -j oe (both to stdout) OR-j eo (both to stderr) |
(use -o without -e) |
Copy Environment | -V |
--export=[ALL | NONE | variables] Note: default behavior is ALL |
Copy Specific Environment Variable | -v myvar=somevalue |
--export=NONE,myvar=somevalue OR--export=ALL,myvar=somevalue |
Event Notification | -m abe |
--mail-type=[events] |
Email Address | -M [address] |
--mail-user=[address] |
Job Name | -N [name] |
--job-name=[name] |
Job Restart | -r [y|n] |
--requeue OR--no-requeue |
Working Directory | --workdir=[dir_name] |
|
Resource Sharing | -l naccesspolicy=singlejob |
--exclusive OR--shared |
Memory Size | -l mem=[MB] |
--mem=[mem][M|G|T] OR--mem-per-cpu=[mem][M|G|T] |
Account to charge | -A [account] |
-A [account] |
Tasks Per Node | -l ppn=[count] |
--tasks-per-node=[count] |
CPUs Per Task | --cpus-per-task=[count] |
|
Job Dependency | -W depend=[state:job_id] |
--depend=[state:job_id] |
Job Arrays | -t [array_spec] |
--array=[array_spec] |
Generic Resources | -l other=[resource_spec] |
--gres=[resource_spec] |
Licenses | --licenses=[license_spec] |
|
Begin Time | -A "y-m-d h:m:s" |
--begin=y-m-d[Th:m[:s]] |
See the official Slurm Documentation for further details.
Notable Differences
-
Separate commands for Batch and Interactive jobs
Unlike PBS, in Slurm interactive jobs and batch jobs are launched with completely distinct commands.
Usesbatch [allocation request options] script
to submit a job to the batch scheduler, andsinteractive [allocation request options]
to launch an interactive job. sinteractive accepts most of the same allocation request options as sbatch does. -
No need for
cd $PBS_O_WORKDIR
In Slurm your batch job starts to run in the directory from which you submitted the script whereas in PBS/Torque you need to explicitly move back to that directory with
cd $PBS_O_WORKDIR
. -
No need to manually export environment
The environment variables that are defined in your shell session at the time that you submit the script are exported into your batch job, whereas in PBS/Torque you need to use the
-V
flag to export your environment. -
Location of output files
The output and error files are created in their final location immediately that the job begins or an error is generated, whereas in PBS/Torque temporary files are created that are only moved to the final location at the end of the job. Therefore in Slurm you can examine the output and error files from your job during its execution.
See the official Slurm Documentation for further details.
Example Jobs
A number of example jobs are available for you to look over and adapt to your own needs. The first few are generic examples, and latter ones go into specifics for particular software packages.
Generic SLURM Jobs
The following examples demonstrate the basics of SLURM jobs, and are designed to cover common job request scenarios. These example jobs will need to be modified to run your application or code.
Simple Job
Every SLURM job consists of a job submission file. A job submission file contains a list of commands that run your program and a set of resource (nodes, walltime, queue) requests. The resource requests can appear in the job submission file or can be specified at submit-time as shown below.
This simple example submits the job submission file hello.sub to the standby queue on Gilbreth and requests a single node:
#!/bin/bash
# FILENAME: hello.sub
# Show this ran on a compute node by running the hostname command.
hostname
echo "Hello World"
On Gilbreth, specifying the number of GPUs requested per node is required.
sbatch -A standby --nodes=1 --ntasks=1 --cpus-per-task=1 --gpus-per-node=1 --time=00:01:00 hello.sub
Submitted batch job 3521
For a real job you would replace echo "Hello World"
with a command, or sequence of commands, that run your program.
After your job finishes running, the ls command will show a new file in your directory, the .out file:
ls -l
hello.sub
slurm-3521.out
The file slurm-3521.out contains the output and errors your program would have written to the screen if you had typed its commands at a command prompt:
cat slurm-3521.out
gilbreth-a001.rcac.purdue.edu
Hello World
You should see the hostname of the compute node your job was executed on. Following should be the "Hello World" statement.
Multiple Node
In some cases, you may want to request multiple nodes. To utilize multiple nodes, you will need to have a program or code that is specifically programmed to use multiple nodes such as with MPI. Simply requesting more nodes will not make your work go faster. Your code must support this ability.
This example shows a request for multiple compute nodes. The job submission file contains a single command to show the names of the compute nodes allocated:
# FILENAME: myjobsubmissionfile.sub
#!/bin/bash
echo "$SLURM_JOB_NODELIST"
On Gilbreth, specifying the number of GPUs requested per node is required.
sbatch --nodes=2 --ntasks=32 --gpus-per-node=1 --time=00:10:00 -A standby myjobsubmissionfile.sub
Compute nodes allocated:
gilbreth-a[014-015]
The above example will allocate the total of 32 CPU cores across 2 nodes. Note that if your multi-node job requests fewer than each node's full 16 cores per node, by default Slurm provides no guarantee with respect to how this total is distributed between assigned nodes (i.e. the cores may not necessarily be split evenly). If you need specific arrangements of your tasks and cores, you can use --cpus-per-task= and/or --ntasks-per-node= flags. See Slurm documentation or man sbatch for more options.
Directives
So far these examples have shown submitting jobs with the resource requests on the sbatch
command line such as:
sbatch -A standby --nodes=1 --gpus-per-node=1 --time=00:01:00 hello.sub
The resource requests can also be put into job submission file itself. Documenting the resource requests in the job submission is desirable because the job can be easily reproduced later. Details left in your command history are quickly lost. Arguments are specified with the #SBATCH
syntax:
#!/bin/bash
# FILENAME: hello.sub
#SBATCH -A standby
#SBATCH --nodes=1 --gpus-per-node=1 --time=00:01:00
# Show this ran on a compute node by running the hostname command.
hostname
echo "Hello World"
The #SBATCH
directives must appear at the top of your submission file. SLURM will stop parsing directives as soon as it encounters a line that does not start with '#'. If you insert a directive in the middle of your script, it will be ignored.
This job can be then submitted with:
sbatch hello.sub
Specific Types of Nodes
SLURM allows running a job on specific types of compute nodes to accommodate special hardware requirements (e.g. a certain CPU or GPU type, etc.)
Cluster nodes have a set of descriptive features assigned to them, and users can specify which of these features are required by their job by using the constraint option at submission time. Only nodes having features matching the job constraints will be used to satisfy the request.
Example: a job requires a compute node in an "A" sub-cluster:
sbatch --nodes=1 --ntasks=16 --gres=gpu:1 --constraint=A myjobsubmissionfile.sub
Compute node allocated:
gilbreth-a003
Feature constraints can be used for both batch and interactive jobs, as well as for individual job steps inside a job. Multiple constraints can be specified with a predefined syntax to achieve complex request logic (see detailed description of the '--constraint' option in man sbatch
or online Slurm documentation).
Refer to Detailed Hardware Specification section for list of available sub-cluster labels, their respective per-node memory sizes and other hardware details. You could also use sfeatures
command to list available constraint feature names for different node types.
Interactive Jobs
Interactive jobs are run on compute nodes, while giving you a shell to interact with. They give you the ability to type commands or use a graphical interface in the same way as if you were on a front-end login host.
To submit an interactive job, use sinteractive to run a login shell on allocated resources.
sinteractive accepts most of the same resource requests as sbatch, so to request a login shell on the cpu account while allocating 2 nodes and 16 total cores, you might do:
sinteractive -A cpu -N2 -n32 --gpus-per-node=1
To quit your interactive job:
exit
or Ctrl-D
The above example will allocate the total of 32 CPU cores across 2 nodes. Note that if your multi-node job requests fewer than each node's full 16 cores per node, by default Slurm provides no guarantee with respect to how this total is distributed between assigned nodes (i.e. the cores may not necessarily be split evenly). If you need specific arrangements of your tasks and cores, you can use --cpus-per-task= and/or --ntasks-per-node= flags. See Slurm documentation or man salloc for more options.
Serial Jobs
This shows how to submit one of the serial programs compiled in the section Compiling Serial Programs.
Create a job submission file:
#!/bin/bash
# FILENAME: serial_hello.sub
./serial_hello
Submit the job:
sbatch --nodes=1 --ntasks=1 --gpus-per-node=1 --time=00:01:00 serial_hello.sub
After the job completes, view results in the output file:
cat slurm-myjobid.out
Runhost:gilbreth-a009.rcac.purdue.edu
hello, world
If the job failed to run, then view error messages in the file slurm-myjobid.out.
OpenMP
A shared-memory job is a single process that takes advantage of a multi-core processor and its shared memory to achieve parallelization.
This example shows how to submit an OpenMP program compiled in the section Compiling OpenMP Programs.
When running OpenMP programs, all threads must be on the same compute node to take advantage of shared memory. The threads cannot communicate between nodes.
To run an OpenMP program, set the environment variable OMP_NUM_THREADS to the desired number of threads:
In csh:
setenv OMP_NUM_THREADS 16
In bash:
export OMP_NUM_THREADS=16
This should almost always be equal to the number of cores on a compute node. You may want to set to another appropriate value if you are running several processes in parallel in a single job or node.
Create a job submissionfile:
#!/bin/bash
# FILENAME: omp_hello.sub
#SBATCH --nodes=1
#SBATCH --ntasks=16
#SBATCH --gpus-per-node=1
#SBATCH --time=00:01:00
export OMP_NUM_THREADS=16
./omp_hello
Submit the job:
sbatch omp_hello.sub
View the results from one of the sample OpenMP programs about task parallelism:
cat omp_hello.sub.omyjobid
SERIAL REGION: Runhost:gilbreth-a003.rcac.purdue.edu Thread:0 of 1 thread hello, world
PARALLEL REGION: Runhost:gilbreth-a003.rcac.purdue.edu Thread:0 of 16 threads hello, world
PARALLEL REGION: Runhost:gilbreth-a003.rcac.purdue.edu Thread:1 of 16 threads hello, world
...
If the job failed to run, then view error messages in the file slurm-myjobid.out.
If an OpenMP program uses a lot of memory and 16 threads use all of the memory of the compute node, use fewer processor cores (OpenMP threads) on that compute node.
MPI
An MPI job is a set of processes that take advantage of multiple compute nodes by communicating with each other. OpenMPI and Intel MPI (IMPI) are implementations of the MPI standard.
This section shows how to submit one of the MPI programs compiled in the section Compiling MPI Programs.
Use module load to set up the paths to access these libraries. Use module avail to see all MPI packages installed on Gilbreth.
Create a job submission file:
#!/bin/bash
# FILENAME: mpi_hello.sub
#SBATCH --nodes=2
#SBATCH --ntasks-per-node=16
#SBATCH --gpus-per-node=1
#SBATCH --time=00:01:00
#SBATCH -A standby
srun -n 32 ./mpi_hello
SLURM can run an MPI program with the srun command. The number of processes is requested with the -n option. If you do not specify the -n option, it will default to the total number of processor cores you request from SLURM.
If the code is built with OpenMPI, it can be run with a simple srun -n command. If it is built with Intel IMPI, then you also need to add the --mpi=pmi2 option: srun --mpi=pmi2 -n 32 ./mpi_hello in this example.
Submit the MPI job:
sbatch ./mpi_hello.sub
View results in the output file:
cat slurm-myjobid.out
Runhost:gilbreth-a010.rcac.purdue.edu Rank:0 of 32 ranks hello, world
Runhost:gilbreth-a010.rcac.purdue.edu Rank:1 of 32 ranks hello, world
...
Runhost:gilbreth-a011.rcac.purdue.edu Rank:16 of 32 ranks hello, world
Runhost:gilbreth-a011.rcac.purdue.edu Rank:17 of 32 ranks hello, world
...
If the job failed to run, then view error messages in the output file.
If an MPI job uses a lot of memory and 16 MPI ranks per compute node use all of the memory of the compute nodes, request more compute nodes, while keeping the total number of MPI ranks unchanged.
Submit the job with double the number of compute nodes and modify the resource request to halve the number of MPI ranks per compute node.
#!/bin/bash
# FILENAME: mpi_hello.sub
#SBATCH --nodes=4
#SBATCH --ntasks-per-node=8
#SBATCH --gpus-per-node=1
#SBATCH -t 00:01:00
#SBATCH -A standby
srun -n 32 ./mpi_hello
sbatch ./mpi_hello.sub
View results in the output file:
cat slurm-myjobid.out
Runhost:gilbreth-a10.rcac.purdue.edu Rank:0 of 32 ranks hello, world
Runhost:gilbreth-a010.rcac.purdue.edu Rank:1 of 32 ranks hello, world
...
Runhost:gilbreth-a011.rcac.purdue.edu Rank:8 of 32 ranks hello, world
...
Runhost:gilbreth-a012.rcac.purdue.edu Rank:16 of 32 ranks hello, world
...
Runhost:gilbreth-a013.rcac.purdue.edu Rank:24 of 32 ranks hello, world
...
Notes
- Use slist to determine which queues (
--account
or-A
option) are available to you. The name of the queue which is available to everyone on Gilbreth is "standby". - Invoking an MPI program on Gilbreth with ./program is typically wrong, since this will use only one MPI process and defeat the purpose of using MPI. Unless that is what you want (rarely the case), you should use srun or mpiexec to invoke an MPI program.
- In general, the exact order in which MPI ranks output similar write requests to an output file is random.
GPU
The Gilbreth cluster nodes contain NVIDIA GPUs that support CUDA and OpenCL. See the detailed hardware overview for the specifics on the GPUs in Gilbreth.
This section illustrates how to use SLURM to submit a simple GPU program.
Suppose that you named your executable file gpu_hello from the sample code gpu_hello.cu
(see the section on compiling NVIDIA GPU codes). Prepare a job submission file with an appropriate name, here named gpu_hello.sub:
#!/bin/bash
# FILENAME: gpu_hello.sub
module load cuda
host=`hostname -s`
echo $CUDA_VISIBLE_DEVICES
# Run on the first available GPU
./gpu_hello 0
Submit the job:
sbatch -A gpu --nodes=1 --gres=gpu:1 -t 00:01:00 gpu_hello.sub
Requesting a GPU from the scheduler is required.
You can specify total number of GPUs, or number of GPUs per node, or even number of GPUs per task:
sbatch -A gpu --nodes=1 --gres=gpu:1 -t 00:01:00 gpu_hello.sub
sbatch -A gpu --nodes=1 --gpus-per-node=1 -t 00:01:00 gpu_hello.sub
sbatch -A gpu --nodes=1 --gpus-per-task=1 -t 00:01:00 gpu_hello.sub
After job completion, view the new output file in your directory:
ls -l
gpu_hello
gpu_hello.cu
gpu_hello.sub
slurm-myjobid.out
View results in the file for all standard output, slurm-myjobid.out
0
hello, world
If the job failed to run, then view error messages in the file slurm-myjobid.out.
To use multiple GPUs in your job, simply specify a larger value to the GPU specification parameter. However, be aware of the number of GPUs installed on the node(s) you may be requesting. The scheduler can not allocate more GPUs than physically exist. See detailed hardware overview and output of sfeatures command for the specifics on the GPUs in Gilbreth.
Link to section 'Collecting System Resource Utilization Data' of 'Monitoring Resources' Collecting System Resource Utilization Data
Knowing the precise resource utilization an application had during a job, such as GPU load or memory, can be incredibly useful. This is especially the case when the application isn't performing as expected.
One approach is to run a program like htop
during an interactive job and keep an eye on system resources. You can get precise time-series data from nodes associated with your job using XDmod as well, online. But these methods don't gather telemetry in an automated fashion, nor do they give you control over the resolution or format of the data.
As a matter of course, a robust implementation of some HPC workload would include resource utilization data as a diagnostic tool in the event of some failure.
The monitor
utility is a simple command line system resource monitoring tool for gathering such telemetry and is available as a module.
module load monitor
Complete documentation is available online at resource-monitor.readthedocs.io. A full manual page is also available for reference, man monitor
.
In the context of a SLURM job you will need to put this monitoring task in the background to allow the rest of your job script to proceed. Be sure to interrupt these tasks at the end of your job.
#!/bin/bash
# FILENAME: monitored_job.sh
module load monitor
# track GPU load
monitor gpu percent >gpu-percent.log &
GPU_PID=$!
# track CPU load
monitor cpu percent >cpu-percent.log &
CPU_PID=$!
# your code here
# shut down the resource monitors
kill -s INT $GPU_PID $CPU_PID
A particularly elegant solution would be to include such tools in your prologue script and have the tear down in your epilogue script.
For large distributed jobs spread across multiple nodes, mpiexec
can be used to gather telemetry from all nodes in the job. The hostname is included in each line of output so that data can be grouped as such. A concise way of constructing the needed list of hostnames in SLURM is to simply use srun hostname | sort -u
.
#!/bin/bash
# FILENAME: monitored_job.sh
module load monitor
# track all GPUs (one monitor per host)
mpiexec -machinefile <(srun hostname | sort -u) \
monitor gpu percent >gpu-percent.log &
GPU_PID=$!
# track all CPUs (one monitor per host)
mpiexec -machinefile <(srun hostname | sort -u) \
monitor cpu percent --all-cores >cpu-percent.log &
CPU_PID=$!
# your code here
# shut down the resource monitors
kill -s INT $GPU_PID $CPU_PID
To get resource data in a more readily computable format, the monitor
program can be told to output in CSV format with the --csv
flag.
monitor gpu memory --csv >gpu-memory.csv
For a distributed job you will need to suppress the header lines otherwise one will be created by each host.
monitor gpu memory --csv | head -1 >gpu-memory.csv
mpiexec -machinefile <(srun hostname | sort -u) \
monitor gpu memory --csv --no-header >>gpu-memory.csv
Specific Applications
The following examples demonstrate job submission files for some common real-world applications. See the Generic SLURM Examples section for more examples on job submissions that can be adapted for use.
Gaussian
Gaussian is a computational chemistry software package which works on electronic structure. This section illustrates how to submit a small Gaussian job to a Slurm queue. This Gaussian example runs the Fletcher-Powell multivariable optimization.
Prepare a Gaussian input file with an appropriate filename, here named myjob.com. The final blank line is necessary:
#P TEST OPT=FP STO-3G OPTCYC=2
STO-3G FLETCHER-POWELL OPTIMIZATION OF WATER
0 1
O
H 1 R
H 1 R 2 A
R 0.96
A 104.
To submit this job, load Gaussian then run the provided script, named subg16. This job uses one compute node with 16 processor cores:
module load gaussian16
subg16 myjob -N 1 -n 16 --gres=gpu:1
View job status:
squeue -u myusername
View results in the file for Gaussian output, here named myjob.log. Only the first and last few lines appear here:
Entering Gaussian System, Link 0=/apps/cent7/gaussian/g16-A.03/g16-haswell/g16/g16
Initial command:
/apps/cent7/gaussian/g16-A.03/g16-haswell/g16/l1.exe /scratch/gilbreth/myusername/gaussian/Gau-7781.inp -scrdir=/scratch/gilbreth/myusername/gaussian/
Entering Link 1 = /apps/cent7/gaussian/g16-A.03/g16-haswell/g16/l1.exe PID= 7782.
Copyright (c) 1988,1990,1992,1993,1995,1998,2003,2009,2016,
Gaussian, Inc. All Rights Reserved.
.
.
.
Job cpu time: 0 days 0 hours 3 minutes 28.2 seconds.
Elapsed time: 0 days 0 hours 0 minutes 12.9 seconds.
File lengths (MBytes): RWF= 17 Int= 0 D2E= 0 Chk= 2 Scr= 2
Normal termination of Gaussian 16 at Tue May 1 17:12:00 2018.
real 13.85
user 202.05
sys 6.12
Machine:
gilbreth-a012.rcac.purdue.edu
gilbreth-a012.rcac.purdue.edu
gilbreth-a012.rcac.purdue.edu
gilbreth-a012.rcac.purdue.edu
gilbreth-a012.rcac.purdue.edu
gilbreth-a012.rcac.purdue.edu
gilbreth-a012.rcac.purdue.edu
gilbreth-a012.rcac.purdue.edu
Link to section 'Examples of Gaussian SLURM Job Submissions' of 'Gaussian' Examples of Gaussian SLURM Job Submissions
Submit job using 16 processor cores on a single node:
subg16 myjob -N 1 -n 16 --gres=gpu:1 -t 24:00:00 -A standby
Submit job using 16 processor cores on each of 2 nodes:
subg16 myjob -N 2 --ntasks-per-node=16 --gres=gpu:2 -t 24:00:00 -A standby
To submit a bash job, a submit script sample looks like:
#!/bin/bash
#SBATCH -A myqueuename # Queue name(use 'slist' command to find queues' name)
#SBATCH --nodes=1 # Total # of nodes
#SBATCH --ntasks=64 # Total # of MPI tasks
#SBATCH --gpus-per-node=1 # Total # of GPUs
#SBATCH --time=1:00:00 # Total run time limit (hh:mm:ss)
#SBATCH -J myjobname # Job name
#SBATCH -o myjob.o%j # Name of stdout output file
#SBATCH -e myjob.e%j # Name of stderr error file
module load gaussian16
g16 < myjob.com
For more information about Gaussian:
Machine Learning
We support several common machine learning (ML) frameworks on the community clusters through pre-installed modules. The collection of these pre-installed ML modules is referred to as ml-toolkit throughout this documentation. Currently, the following libraries are included in ML-Toolkit.
caffe cntk gym keras
mxnet opencv pytorch
tensorflow tflearn theano
Note that managing dependencies with ML applications can be non-trivial, therefore, we recommend users start by using ml-toolkit. If a custom installation is required after trying ml-toolkit, make sure to read documentation carefully.
ML-Toolkit
A set of pre-installed popular machine learning (ML) libraries, called ML-Toolkit is maintained on Gilbreth. These are Anaconda/Python-based distributions of the respective libraries. Currently, applications are supported for Python 2 and 3. Detailed instructions for searching and using the installed ML applications are presented below.
Link to section 'Instructions for using ML-Toolkit Modules' of 'ML-Toolkit' Instructions for using ML-Toolkit Modules
Link to section 'Find and Use Installed ML Packages' of 'ML-Toolkit' Find and Use Installed ML Packages
To search or load a machine learning application, you must first load one of the learning modules. The learning module loads the prerequisites (such as anaconda and cudnn) and makes ML applications visible to the user.
Step 1. Find and load a preferred learning module. Several learning modules may be available, corresponding to a specific Python version and whether the ML applications have GPU support or not. Running module load learning without specifying a version will load the version with the most recent python version. To see all available modules, run module spider learning then load the desired module.
Step 2. Find and load the desired machine learning libraries
ML packages are installed under the common application name ml-toolkit-X, where X can be cpu or gpu.
You can use the module spider ml-toolkit command to see all options and versions of each library.
Load the desired modules using the module load command. Note that both CPU and GPU options may exist for many libraries, so be sure to load the correct version. For example, if you wanted to load the most recent version of PyTorch for CPU, you would run module load ml-toolkit-cpu/pytorch
caffe cntk gym keras mxnet
opencv pytorch tensorflow tflearn theano
Step 3. You can list which ML applications are loaded in your environment using the command module list
Link to section 'Verify application import' of 'ML-Toolkit' Verify application import
Step 4. The next step is to check that you can actually use the desired ML application. You can do this by running the import command in Python. The example below tests if PyTorch has been loaded correctly.
python -c "import torch; print(torch.__version__)"
If the import operation succeeded, then you can run your own ML code. Some ML applications (such as tensorflow) print diagnostic warnings while loading -- this is the expected behavior.
If the import fails with an error, please see the troubleshooting information below.
Step 5. To load a different set of applications, unload the previously loaded applications and load the new desired applications. The example below loads Tensorflow and Keras instead of PyTorch and OpenCV.
module unload ml-toolkit-cpu/opencv
module unload ml-toolkit-cpu/pytorch
module load ml-toolkit-cpu/tensorflow
module load ml-toolkit-cpu/keras
Link to section 'Troubleshooting' of 'ML-Toolkit' Troubleshooting
ML applications depend on a wide range of Python packages and mixing multiple versions of these packages can lead to error. The following guidelines will assist you in identifying the cause of the problem.
- Check that you are using the correct version of Python with the command python --version. This should match the Python version in the loaded anaconda module.
- Start from a clean environment. Either start a new terminal session or unload all the modules using module purge. Then load the desired modules following Steps 1-2.
- Verify that PYTHONPATH does not point to undesired packages. Run the following command to print PYTHONPATH: echo $PYTHONPATH. Make sure that your Python environment is clean. Watch out for any locally installed packages that might conflict.
- If you don't see GPU devices in your code, make sure that you are using the ml-toolkit-gpu/ modules and not using their cpu versions.
- ML applications often have dependency on specific versions of Cuda and CuDNN libraries. Make sure that you have loaded the required versions using the command: module list
- Note that Caffe has a conflicting version of PyQt5. So, if you want to use Spyder (or any GUI application that uses PyQt), then you should unload the caffe module.
- Use Google search to your advantage. Copy the error message in Google and check probable causes.
More examples showing how to use ml-toolkit modules in a batch job are presented in ML Batch Jobs guide.
Link to section 'Installation of Custom ML Libraries' of 'Custom ML Packages' Installation of Custom ML Libraries
While we try to include as many common ML frameworks and versions as we can in ML-Toolkit, we recognize that there are also situations in which a custom installation may be preferable. We recommend using conda-env-mod to install and manage Python packages. Please follow the steps carefully, otherwise you may end up with a faulty installation. The example below shows how to install TensorFlow in your home directory.
Link to section 'Install' of 'Custom ML Packages' Install
Step 1: Unload all modules and start with a clean environment.
module purge
Step 2: Load the anaconda module with desired Python version.
module load anaconda
Step 2A: If the ML application requires Cuda and CuDNN, load the appropriate modules. Be sure to check that the versions you load are compatible with the desired ML package.
module load cuda
module load cudnn
Many machine-learning packages (including PyTorch and TensorFlow) now provide installation pathways that include the full cudatoolkit within the environment, making it unnecessary to load these modules.
Step 3: Create a custom anaconda environment. Make sure the python version matches the Python version in the anaconda module.
conda-env-mod create -n env_name_here
Step 4: Activate the anaconda environment by loading the modules displayed at the end of step 3.
module load use.own
module load conda-env/env_name_here-py3.8.5
Step 5: Now install the desired ML application. You can install multiple Python packages at this step using either conda or pip.
For TensorFlow (as of 2024) the recommended approach is to use pip (see tensorflow.org/install/gpu).
pip install --ignore-installed 'tensorflow[and-cuda]'
For PyTorch the recommended approach is to use conda (see pytorch.org).
conda install pytorch torchvision torchaudio pytorch-cuda=11.8 -c pytorch -c nvidia
If the installation succeeded, you can now proceed to testing and using the installed application. You must load the environment you created as well as any supporting modules (e.g., anaconda) whenever you want to use this installation. If your installation did not succeed, please refer to the troubleshooting section below as well as documentation for the desired package you are installing.
Note that loading the modules generated by conda-env-mod has different behavior than conda create env_name_here followed by source activate env_name_here. After running source activate, you may not be able to access any Python packages in anaconda or ml-toolkit modules. Therefore, using conda-env-mod is the preferred way of using your custom installations.
Link to section 'Testing the Installation' of 'Custom ML Packages' Testing the Installation
-
Verify the installation by using a simple import statement, like that listed below for TensorFlow:
python -c "import tensorflow as tf; print(tf.__version__);"
Note that a successful import of TensorFlow will print a variety of system and hardware information. This is expected.
If importing the package leads to errors, be sure to verify that all dependencies for the package have been managed, and the correct versions installed. Dependency issues between python packages are the most common cause for errors. For example, in TF, conflicts with the h5py or numpy versions are common, but upgrading those packages typically solves the problem. Managing dependencies for ML libraries can be non-trivial.
-
Next, we can test using our installation of TensorFlow for a GPU run. For this we shall use the matrix multiplication example from Tensorflow documentation.
# filename: matrixmult.py import tensorflow as tf print("Num GPUs Available: ", len(tf.config.list_physical_devices('GPU'))) tf.debugging.set_log_device_placement(True) # Place tensors on the CPU with tf.device('/CPU:0'): a = tf.constant([[1.0, 2.0, 3.0], [4.0, 5.0, 6.0]]) b = tf.constant([[1.0, 2.0], [3.0, 4.0], [5.0, 6.0]]) # Run on the GPU c = tf.matmul(a, b) print(c)
-
Run the example
$ python matrixmult.py
-
This will produce an output like:
Num GPUs Available: 3 2022-07-25 10:33:23.358919: I tensorflow/core/platform/cpu_feature_guard.cc:193] This TensorFlow binary is optimized with oneAPI Deep Neural Network Library (oneDNN) to use the following CPU instructions in performance-critical operations: AVX2 AVX512F FMA To enable them in other operations, rebuild TensorFlow with the appropriate compiler flags. 2022-07-25 10:33:26.223459: I tensorflow/core/common_runtime/gpu/gpu_device.cc:1532] Created device /job:localhost/replica:0/task:0/device:GPU:0 with 22183 MB memory: -> device: 0, name: NVIDIA A30, pci bus id: 0000:3b:00.0, compute capability: 8.0 2022-07-25 10:33:26.225495: I tensorflow/core/common_runtime/gpu/gpu_device.cc:1532] Created device /job:localhost/replica:0/task:0/device:GPU:1 with 22183 MB memory: -> device: 1, name: NVIDIA A30, pci bus id: 0000:af:00.0, compute capability: 8.0 2022-07-25 10:33:26.228514: I tensorflow/core/common_runtime/gpu/gpu_device.cc:1532] Created device /job:localhost/replica:0/task:0/device:GPU:2 with 22183 MB memory: -> device: 2, name: NVIDIA A30, pci bus id: 0000:d8:00.0, compute capability: 8.0 2022-07-25 10:33:26.933709: I tensorflow/core/common_runtime/eager/execute.cc:1323] Executing op MatMul in device /job:localhost/replica:0/task:0/device:GPU:0 2022-07-25 10:33:28.181855: I tensorflow/stream_executor/cuda/cuda_blas.cc:1786] TensorFloat-32 will be used for the matrix multiplication. This will only be logged once. tf.Tensor( [[22. 28.] [49. 64.]], shape=(2, 2), dtype=float32)
-
For more details, please refer to Tensorflow User Guide.
Link to section 'Troubleshooting' of 'Custom ML Packages' Troubleshooting
In most situations, dependencies among Python modules lead to errors. If you cannot use a Python package after installing it, please follow the steps below to find a workaround.
- Unload all the modules.
module purge
- Clean up PYTHONPATH.
unset PYTHONPATH
- Next load the modules, e.g., anaconda and your custom environment.
module load anaconda module load use.own module load conda-env/env_name_here-py3.8.5
- For GPU-enabled applications, you may also need to load the corresponding cuda/ and cudnn/ modules.
- Now try running your code again.
- A few applications only run on specific versions of Python (e.g. Python 3.6). Please check the documentation of your application if that is the case.
- If you have installed a newer version of an ml-toolkit package (e.g., a newer version of PyTorch or Tensorflow), make sure that the ml-toolkit modules are NOT loaded. In general, we recommend that you don't mix ml-toolkit modules with your custom installations.
- GPU-enabled ML applications often have dependencies on specific versions of Cuda and CuDNN. For example, Tensorflow version 1.5.0 and higher needs Cuda 9. Please check the application documentation about such dependencies.
Link to section 'Tensorboard' of 'Custom ML Packages' Tensorboard
- You can visualize data from a Tensorflow session using Tensorboard. For this, you need to save your session summary as described in the Tensorboard User Guide.
- Launch Tensorboard:
$ python -m tensorboard.main --logdir=/path/to/session/logs
- When Tensorboard is launched successfully, it will give you the URL for accessing Tensorboard.
<... build related warnings ...> TensorBoard 0.4.0 at http://gilbreth-a000.rcac.purdue.edu:6006
- Follow the printed URL to visualize your model.
- Please note that due to firewall rules, the Tensorboard URL may only be accessible from Gilbreth nodes. If you cannot access the URL directly, you can use Firefox browser in Thinlinc.
- For more details, please refer to the Tensorboard User Guide.
Link to section 'Running ML Code in a Batch Job' of 'ML Batch Jobs' Running ML Code in a Batch Job
Batch jobs allow us to automate model training without human intervention. They are also useful when you need to run a large number of simulations on the clusters. In the example below, we shall run a simple tensor_hello.py script in a batch job. We consider two situations: in the first example, we use the ML-Toolkit modules to run tensorflow, while in the second example, we use a custom installation of tensorflow (See Custom ML Packages page).
Link to section 'Using ML-Toolkit Modules' of 'ML Batch Jobs' Using ML-Toolkit Modules
Save the following code as tensor_hello.sub in the same directory where tensor_hello.py is located.
# filename: tensor_hello.sub
#SBATCH --nodes=1
#SBATCH --ntasks-per-node=1
#SBATCH --gres=gpu:1
#SBATCH --time=00:05:00
#SBATCH -A standby
#SBATCH -J hello_tensor
module purge
module load learning
module load ml-toolkit-gpu/tensorflow
module list
python tensor_hello.py
Link to section 'Using a Custom Installation' of 'ML Batch Jobs' Using a Custom Installation
Save the following code as tensor_hello.sub in the same directory where tensor_hello.py is located.
# filename: tensor_hello.sub
#SBATCH --nodes=1
#SBATCH --ntasks-per-node=1
#SBATCH --gres=gpu:1
#SBATCH --time=00:05:00
#SBATCH -A standby
#SBATCH -J hello_tensor
module purge
module load anaconda
module load cuda
module load cudnn
module load use.own
module load conda-env/my_tf_env-py3.8.5
module list
echo $PYTHONPATH
python tensor_hello.py
Link to section 'Running a Job' of 'ML Batch Jobs' Running a Job
Now you can submit the batch job using the sbatch command.
sbatch tensor_hello.sub
Once the job finishes, you will find an output file (slurm-xxxxx.out).
Matlab
MATLAB® (MATrix LABoratory) is a high-level language and interactive environment for numerical computation, visualization, and programming. MATLAB is a product of MathWorks.
MATLAB, Simulink, Compiler, and several of the optional toolboxes are available to faculty, staff, and students. To see the kind and quantity of all MATLAB licenses plus the number that you are currently using you can use the matlab_licenses command:
$ module load matlab
$ matlab_licenses
The MATLAB client can be run in the front-end for application development, however, computationally intensive jobs must be run on compute nodes.
The following sections provide several examples illustrating how to submit MATLAB jobs to a Linux compute cluster.
Matlab Script (.m File)
This section illustrates how to submit a small, serial, MATLAB program as a job to a batch queue. This MATLAB program prints the name of the run host and gets three random numbers.
Prepare a MATLAB script myscript.m, and a MATLAB function file myfunction.m:
% FILENAME: myscript.m
% Display name of compute node which ran this job.
[c name] = system('hostname');
fprintf('\n\nhostname:%s\n', name);
% Display three random numbers.
A = rand(1,3);
fprintf('%f %f %f\n', A);
quit;
% FILENAME: myfunction.m
function result = myfunction ()
% Return name of compute node which ran this job.
[c name] = system('hostname');
result = sprintf('hostname:%s', name);
% Return three random numbers.
A = rand(1,3);
r = sprintf('%f %f %f', A);
result=strvcat(result,r);
end
Also, prepare a job submission file, here named myjob.sub. Run with the name of the script:
#!/bin/bash
# FILENAME: myjob.sub
echo "myjob.sub"
# Load module, and set up environment for Matlab to run
module load matlab
unset DISPLAY
# -nodisplay: run MATLAB in text mode; X11 server not needed
# -singleCompThread: turn off implicit parallelism
# -r: read MATLAB program; use MATLAB JIT Accelerator
# Run Matlab, with the above options and specifying our .m file
matlab -nodisplay -singleCompThread -r myscript
myjob.sub
< M A T L A B (R) >
Copyright 1984-2011 The MathWorks, Inc.
R2011b (7.13.0.564) 64-bit (glnxa64)
August 13, 2011
To get started, type one of these: helpwin, helpdesk, or demo.
For product information, visit www.mathworks.com.
hostname:gilbreth-a001.rcac.purdue.edu
0.814724 0.905792 0.126987
Output shows that a processor core on one compute node (gilbreth-a001) processed the job. Output also displays the three random numbers.
For more information about MATLAB:
Implicit Parallelism
MATLAB implements implicit parallelism which is automatic multithreading of many computations, such as matrix multiplication, linear algebra, and performing the same operation on a set of numbers. This is different from the explicit parallelism of the Parallel Computing Toolbox.
MATLAB offers implicit parallelism in the form of thread-parallel enabled functions. Since these processor cores, or threads, share a common memory, many MATLAB functions contain multithreading potential. Vector operations, the particular application or algorithm, and the amount of computation (array size) contribute to the determination of whether a function runs serially or with multithreading.
When your job triggers implicit parallelism, it attempts to allocate its threads on all processor cores of the compute node on which the MATLAB client is running, including processor cores running other jobs. This competition can degrade the performance of all jobs running on the node.
When you know that you are coding a serial job but are unsure whether you are using thread-parallel enabled operations, run MATLAB with implicit parallelism turned off. Beginning with the R2009b, you can turn multithreading off by starting MATLAB with -singleCompThread:
$ matlab -nodisplay -singleCompThread -r mymatlabprogram
When you are using implicit parallelism, make sure you request exclusive access to a compute node, as MATLAB has no facility for sharing nodes.
For more information about MATLAB's implicit parallelism:
Profile Manager
MATLAB offers two kinds of profiles for parallel execution: the 'local' profile and user-defined cluster profiles. The 'local' profile runs a MATLAB job on the processor core(s) of the same compute node, or front-end, that is running the client. To run a MATLAB job on compute node(s) different from the node running the client, you must define a Cluster Profile using the Cluster Profile Manager.
To prepare a user-defined cluster profile, use the Cluster Profile Manager in the Parallel menu. This profile contains the scheduler details (queue, nodes, processors, walltime, etc.) of your job submission. Ultimately, your cluster profile will be an argument to MATLAB functions like batch().
For your convenience, a generic cluster profile is provided that can be downloaded: myslurmprofile.settings
Please note that modifications are very likely to be required to make myslurmprofile.settings work. You may need to change values for number of nodes, number of workers, walltime, and submission queue specified in the file. As well, the generic profile itself depends on the particular job scheduler on the cluster, so you may need to download or create two or more generic profiles under different names. Each time you run a job using a Cluster Profile, make sure the specific profile you are using is appropriate for the job and the cluster.
To import the profile, start a MATLAB session and select Manage Cluster Profiles... from the Parallel menu. In the Cluster Profile Manager, select Import, navigate to the folder containing the profile, select myslurmprofile.settings and click OK. Remember that the profile will need to be customized for your specific needs. If you have any questions, please contact us.
For detailed information about MATLAB's Parallel Computing Toolbox, examples, demos, and tutorials:
Parallel Computing Toolbox (parfor)
The MATLAB Parallel Computing Toolbox (PCT) extends the MATLAB language with high-level, parallel-processing features such as parallel for loops, parallel regions, message passing, distributed arrays, and parallel numerical methods. It offers a shared-memory computing environment running on the local cluster profile in addition to your MATLAB client. Moreover, the MATLAB Distributed Computing Server (DCS) scales PCT applications up to the limit of your DCS licenses.
This section illustrates the fine-grained parallelism of a parallel for loop (parfor) in a pool job.
The following examples illustrate a method for submitting a small, parallel, MATLAB program with a parallel loop (parfor statement) as a job to a queue. This MATLAB program prints the name of the run host and shows the values of variables numlabs and labindex for each iteration of the parfor loop.
This method uses the job submission command to submit a MATLAB client which calls the MATLAB batch() function with a user-defined cluster profile.
Prepare a MATLAB pool program in a MATLAB script with an appropriate filename, here named myscript.m:
% FILENAME: myscript.m
% SERIAL REGION
[c name] = system('hostname');
fprintf('SERIAL REGION: hostname:%s\n', name)
numlabs = parpool('poolsize');
fprintf(' hostname numlabs labindex iteration\n')
fprintf(' ------------------------------- ------- -------- ---------\n')
tic;
% PARALLEL LOOP
parfor i = 1:8
[c name] = system('hostname');
name = name(1:length(name)-1);
fprintf('PARALLEL LOOP: %-31s %7d %8d %9d\n', name,numlabs,labindex,i)
pause(2);
end
% SERIAL REGION
elapsed_time = toc; % get elapsed time in parallel loop
fprintf('\n')
[c name] = system('hostname');
name = name(1:length(name)-1);
fprintf('SERIAL REGION: hostname:%s\n', name)
fprintf('Elapsed time in parallel loop: %f\n', elapsed_time)
The execution of a pool job starts with a worker executing the statements of the first serial region up to the parfor block, when it pauses. A set of workers (the pool) executes the parfor block. When they finish, the first worker resumes by executing the second serial region. The code displays the names of the compute nodes running the batch session and the worker pool.
Prepare a MATLAB script that calls MATLAB function batch() which makes a four-lab pool on which to run the MATLAB code in the file myscript.m. Use an appropriate filename, here named mylclbatch.m:
% FILENAME: mylclbatch.m
!echo "mylclbatch.m"
!hostname
pjob=batch('myscript','Profile','myslurmprofile','Pool',4,'CaptureDiary',true);
wait(pjob);
diary(pjob);
quit;
Prepare a job submission file with an appropriate filename, here named myjob.sub:
#!/bin/bash
# FILENAME: myjob.sub
echo "myjob.sub"
hostname
module load matlab
unset DISPLAY
matlab -nodisplay -r mylclbatch
Submit the job as a single compute node with one processor core.
One processor core runs myjob.sub and mylclbatch.m.
Once this job starts, a second job submission is made.
myjob.sub
< M A T L A B (R) >
Copyright 1984-2013 The MathWorks, Inc.
R2013a (8.1.0.604) 64-bit (glnxa64)
February 15, 2013
To get started, type one of these: helpwin, helpdesk, or demo.
For product information, visit www.mathworks.com.
mylclbatch.mgilbreth-a000.rcac.purdue.edu
SERIAL REGION: hostname:gilbreth-a000.rcac.purdue.edu
hostname numlabs labindex iteration
------------------------------- ------- -------- ---------
PARALLEL LOOP: gilbreth-a001.rcac.purdue.edu 4 1 2
PARALLEL LOOP: gilbreth-a002.rcac.purdue.edu 4 1 4
PARALLEL LOOP: gilbreth-a001.rcac.purdue.edu 4 1 5
PARALLEL LOOP: gilbreth-a002.rcac.purdue.edu 4 1 6
PARALLEL LOOP: gilbreth-a003.rcac.purdue.edu 4 1 1
PARALLEL LOOP: gilbreth-a003.rcac.purdue.edu 4 1 3
PARALLEL LOOP: gilbreth-a004.rcac.purdue.edu 4 1 7
PARALLEL LOOP: gilbreth-a004.rcac.purdue.edu 4 1 8
SERIAL REGION: hostname:gilbreth-a001.rcac.purdue.edu
Elapsed time in parallel loop: 5.411486
To scale up this method to handle a real application, increase the wall time in the submission command to accommodate a longer running job. Secondly, increase the wall time of myslurmprofile by using the Cluster Profile Manager in the Parallel menu to enter a new wall time in the property SubmitArguments.
For more information about MATLAB Parallel Computing Toolbox:
Parallel Toolbox (spmd)
The MATLAB Parallel Computing Toolbox (PCT) extends the MATLAB language with high-level, parallel-processing features such as parallel for loops, parallel regions, message passing, distributed arrays, and parallel numerical methods. It offers a shared-memory computing environment with a maximum of eight MATLAB workers (labs, threads; versions R2009a) and 12 workers (labs, threads; version R2011a) running on the local configuration in addition to your MATLAB client. Moreover, the MATLAB Distributed Computing Server (DCS) scales PCT applications up to the limit of your DCS licenses.
This section illustrates how to submit a small, parallel, MATLAB program with a parallel region (spmd statement) as a MATLAB pool job to a batch queue.
This example uses the submission command to submit to compute nodes a MATLAB client which interprets a Matlab .m with a user-defined cluster profile which scatters the MATLAB workers onto different compute nodes. This method uses the MATLAB interpreter, the Parallel Computing Toolbox, and the Distributed Computing Server; so, it requires and checks out six licenses: one MATLAB license for the client running on the compute node, one PCT license, and four DCS licenses. Four DCS licenses run the four copies of the spmd statement. This job is completely off the front end.
Prepare a MATLAB script called myscript.m:
% FILENAME: myscript.m
% SERIAL REGION
[c name] = system('hostname');
fprintf('SERIAL REGION: hostname:%s\n', name)
p = parpool('4');
fprintf(' hostname numlabs labindex\n')
fprintf(' ------------------------------- ------- --------\n')
tic;
% PARALLEL REGION
spmd
[c name] = system('hostname');
name = name(1:length(name)-1);
fprintf('PARALLEL REGION: %-31s %7d %8d\n', name,numlabs,labindex)
pause(2);
end
% SERIAL REGION
elapsed_time = toc; % get elapsed time in parallel region
delete(p);
fprintf('\n')
[c name] = system('hostname');
name = name(1:length(name)-1);
fprintf('SERIAL REGION: hostname:%s\n', name)
fprintf('Elapsed time in parallel region: %f\n', elapsed_time)
quit;
Prepare a job submission file with an appropriate filename, here named myjob.sub. Run with the name of the script:
#!/bin/bash
# FILENAME: myjob.sub
echo "myjob.sub"
module load matlab
unset DISPLAY
matlab -nodisplay -r myscript
Run MATLAB to set the default parallel configuration to your job configuration:
$ matlab -nodisplay
>> parallel.defaultClusterProfile('myslurmprofile');
>> quit;
$
Once this job starts, a second job submission is made.
myjob.sub
< M A T L A B (R) >
Copyright 1984-2011 The MathWorks, Inc.
R2011b (7.13.0.564) 64-bit (glnxa64)
August 13, 2011
To get started, type one of these: helpwin, helpdesk, or demo.
For product information, visit www.mathworks.com.
SERIAL REGION: hostname:gilbreth-a001.rcac.purdue.edu
Starting matlabpool using the 'myslurmprofile' profile ... connected to 4 labs.
hostname numlabs labindex
------------------------------- ------- --------
Lab 2:
PARALLEL REGION: gilbreth-a002.rcac.purdue.edu 4 2
Lab 1:
PARALLEL REGION: gilbreth-a001.rcac.purdue.edu 4 1
Lab 3:
PARALLEL REGION: gilbreth-a003.rcac.purdue.edu 4 3
Lab 4:
PARALLEL REGION: gilbreth-a004.rcac.purdue.edu 4 4
Sending a stop signal to all the labs ... stopped.
SERIAL REGION: hostname:gilbreth-a001.rcac.purdue.edu
Elapsed time in parallel region: 3.382151
Output shows the name of one compute node (a001) that processed the job submission file myjob.sub and the two serial regions. The job submission scattered four processor cores (four MATLAB labs) among four different compute nodes (a001,a002,a003,a004) that processed the four parallel regions. The total elapsed time demonstrates that the jobs ran in parallel.
For more information about MATLAB Parallel Computing Toolbox:
Distributed Computing Server (parallel job)
The MATLAB Parallel Computing Toolbox (PCT) enables a parallel job via the MATLAB Distributed Computing Server (DCS). The tasks of a parallel job are identical, run simultaneously on several MATLAB workers (labs), and communicate with each other. This section illustrates an MPI-like program.
This section illustrates how to submit a small, MATLAB parallel job with four workers running one MPI-like task to a batch queue. The MATLAB program broadcasts an integer to four workers and gathers the names of the compute nodes running the workers and the lab IDs of the workers.
This example uses the job submission command to submit a Matlab script with a user-defined cluster profile which scatters the MATLAB workers onto different compute nodes. This method uses the MATLAB interpreter, the Parallel Computing Toolbox, and the Distributed Computing Server; so, it requires and checks out six licenses: one MATLAB license for the client running on the compute node, one PCT license, and four DCS licenses. Four DCS licenses run the four copies of the parallel job. This job is completely off the front end.
Prepare a MATLAB script named myscript.m :
% FILENAME: myscript.m
% Specify pool size.
% Convert the parallel job to a pool job.
parpool('4');
spmd
if labindex == 1
% Lab (rank) #1 broadcasts an integer value to other labs (ranks).
N = labBroadcast(1,int64(1000));
else
% Each lab (rank) receives the broadcast value from lab (rank) #1.
N = labBroadcast(1);
end
% Form a string with host name, total number of labs, lab ID, and broadcast value.
[c name] =system('hostname');
name = name(1:length(name)-1);
fmt = num2str(floor(log10(numlabs))+1);
str = sprintf(['%s:%d:%' fmt 'd:%d '], name,numlabs,labindex,N);
% Apply global concatenate to all str's.
% Store the concatenation of str's in the first dimension (row) and on lab #1.
result = gcat(str,1,1);
if labindex == 1
disp(result)
end
end % spmd
matlabpool close force;
quit;
Also, prepare a job submission, here named myjob.sub. Run with the name of the script:
# FILENAME: myjob.sub
echo "myjob.sub"
module load matlab
unset DISPLAY
# -nodisplay: run MATLAB in text mode; X11 server not needed
# -r: read MATLAB program; use MATLAB JIT Accelerator
matlab -nodisplay -r myscript
Run MATLAB to set the default parallel configuration to your appropriate Profile:
$ matlab -nodisplay
>> defaultParallelConfig('myslurmprofile');
>> quit;
$
Submit the job as a single compute node with one processor core.
Once this job starts, a second job submission is made.
myjob.sub
< M A T L A B (R) >
Copyright 1984-2011 The MathWorks, Inc.
R2011b (7.13.0.564) 64-bit (glnxa64)
August 13, 2011
To get started, type one of these: helpwin, helpdesk, or demo.
For product information, visit www.mathworks.com.
>Starting matlabpool using the 'myslurmprofile' configuration ... connected to 4 labs.
Lab 1:
gilbreth-a006.rcac.purdue.edu:4:1:1000
gilbreth-a007.rcac.purdue.edu:4:2:1000
gilbreth-a008.rcac.purdue.edu:4:3:1000
gilbreth-a009.rcac.purdue.edu:4:4:1000
Sending a stop signal to all the labs ... stopped.
Did not find any pre-existing parallel jobs created by matlabpool.
Output shows the name of one compute node (a006) that processed the job submission file myjob.sub. The job submission scattered four processor cores (four MATLAB labs) among four different compute nodes (a006,a007,a008,a009) that processed the four parallel regions.
To scale up this method to handle a real application, increase the wall time in the submission command to accommodate a longer running job. Secondly, increase the wall time of myslurmprofile by using the Cluster Profile Manager in the Parallel menu to enter a new wall time in the property SubmitArguments.
For more information about parallel jobs:
Python
Notice: Python 2.7 has reached end-of-life on Jan 1, 2020 (announcement). Please update your codes and your job scripts to use Python 3.
Python is a high-level, general-purpose, interpreted, dynamic programming language. We suggest using Anaconda which is a Python distribution made for large-scale data processing, predictive analytics, and scientific computing. For example, to use the default Anaconda distribution:
$ module load conda
For a full list of available Anaconda and Python modules enter:
$ module spider conda
Example Python Jobs
This section illustrates how to submit a small Python job to a SLURM queue.
Link to section 'Example 1: Hello world' of 'Example Python Jobs' Example 1: Hello world
Prepare a Python input file with an appropriate filename, here named hello.py
:
# FILENAME: hello.py
import string, sys
print("Hello, world!")
Prepare a job submission file with an appropriate filename, here named myjob.sub:
#!/bin/bash
# FILENAME: myjob.sub
module load conda
python hello.py
Hello, world!
Link to section 'Example 2: Matrix multiply' of 'Example Python Jobs' Example 2: Matrix multiply
Save the following script as matrix.py:
# Matrix multiplication program
x = [[3,1,4],[1,5,9],[2,6,5]]
y = [[3,5,8,9],[7,9,3,2],[3,8,4,6]]
result = [[sum(a*b for a,b in zip(x_row,y_col)) for y_col in zip(*y)] for x_row in x]
for r in result:
print(r)
Change the last line in the job submission file above to read:
python matrix.py
The standard output file from this job will result in the following matrix:
[28, 56, 43, 53]
[65, 122, 59, 73]
[63, 104, 54, 60]
Link to section 'Example 3: Sine wave plot using numpy and matplotlib packages' of 'Example Python Jobs' Example 3: Sine wave plot using numpy and matplotlib packages
Save the following script as sine.py:
import numpy as np
import matplotlib
matplotlib.use('Agg')
import matplotlib.pyplot as plt
x = np.linspace(-np.pi, np.pi, 201)
plt.plot(x, np.sin(x))
plt.xlabel('Angle [rad]')
plt.ylabel('sin(x)')
plt.axis('tight')
plt.savefig('sine.png')
Change your job submission file to submit this script and the job will output a png file and blank standard output and error files.
For more information about Python:
Managing Environments with Conda
Conda is a package manager in Anaconda that allows you to create and manage multiple environments where you can pick and choose which packages you want to use. To use Conda you must load an Anaconda module:
$ module load conda
Many packages are pre-installed in the global environment. To see these packages:
$ conda list
To create your own custom environment:
$ conda create --name MyEnvName python=3.8 FirstPackageName SecondPackageName -y
The --name option specifies that the environment created will be named MyEnvName. You can include as many packages as you require separated by a space. Including the -y option lets you skip the prompt to install the package. By default environments are created and stored in the $HOME/.conda directory.
To create an environment at a custom location:
$ conda create --prefix=$HOME/MyEnvName python=3.8 PackageName -y
To see a list of your environments:
$ conda env list
To remove unwanted environments:
$ conda remove --name MyEnvName --all
To add packages to your environment:
$ conda install --name MyEnvName PackageNames
To remove a package from an environment:
$ conda remove --name MyEnvName PackageName
Installing packages when creating your environment, instead of one at a time, will help you avoid dependency issues.
To activate or deactivate an environment you have created:
$ source activate MyEnvName
$ source deactivate MyEnvName
If you created your conda environment at a custom location using --prefix option, then you can activate or deactivate it using the full path.
$ source activate $HOME/MyEnvName
$ source deactivate $HOME/MyEnvName
To use a custom environment inside a job you must load the module and activate the environment inside your job submission script. Add the following lines to your submission script:
$ module load conda
$ source activate MyEnvName
For more information about Python:
Managing Packages with Pip
Pip is a Python package manager. Many Python package documentation provide pip
instructions that result in permission errors because by default pip
will install in a system-wide location and fail.
Exception:
Traceback (most recent call last):
... ... stack trace ... ...
OSError: [Errno 13] Permission denied: '/apps/cent7/anaconda/2020.07-py38/lib/python3.8/site-packages/mkl_random-1.1.1.dist-info'
If you encounter this error, it means that you cannot modify the global Python installation. We recommend installing Python packages in a conda environment. Detailed instructions for installing packages with pip can be found in our Python package installation page.
Below we list some other useful pip commands.
- Search for a package in PyPI channels:
$ pip search packageName
- Check which packages are installed globally:
$ pip list
- Check which packages you have personally installed:
$ pip list --user
- Snapshot installed packages:
$ pip freeze > requirements.txt
- You can install packages from a snapshot inside a new conda environment. Make sure to load the appropriate conda environment first.
$ pip install -r requirements.txt
For more information about Python:
Installing Packages
Installing Python packages in an Anaconda environment is recommended. One key advantage of Anaconda is that it allows users to install unrelated packages in separate self-contained environments. Individual packages can later be reinstalled or updated without impacting others. If you are unfamiliar with Conda environments, please check our Conda Guide.
To facilitate the process of creating and using Conda environments, we support a script (conda-env-mod) that generates a module file for an environment, as well as an optional Jupyter kernel to use this environment in a JupyterHub notebook.
You must load one of the anaconda modules in order to use this script.
$ module load conda
Step-by-step instructions for installing custom Python packages are presented below.
Link to section 'Step 1: Create a conda environment' of 'Installing Packages' Step 1: Create a conda environment
Users can use the conda-env-mod script to create an empty conda environment. This script needs either a name or a path for the desired environment. After the environment is created, it generates a module file for using it in future. Please note that conda-env-mod is different from the official conda-env script and supports a limited set of subcommands. Detailed instructions for using conda-env-mod can be found with the command conda-env-mod --help.
-
Example 1: Create a conda environment named mypackages in user's
$HOME
directory.$ conda-env-mod create -n mypackages
-
Example 2: Create a conda environment named mypackages at a custom location.
$ conda-env-mod create -p /depot/mylab/apps/mypackages
Please follow the on-screen instructions while the environment is being created. After finishing, the script will print the instructions to use this environment.
... ... ... Preparing transaction: ...working... done Verifying transaction: ...working... done Executing transaction: ...working... done +------------------------------------------------------+ | To use this environment, load the following modules: | | module load use.own | | module load conda-env/mypackages-py3.8.5 | +------------------------------------------------------+ Your environment "mypackages" was created successfully.
Note down the module names, as you will need to load these modules every time you want to use this environment. You may also want to add the module load lines in your jobscript, if it depends on custom Python packages.
By default, module files are generated in your $HOME/privatemodules directory. The location of module files can be customized by specifying the -m /path/to/modules option to conda-env-mod.
Note: The main differences between -p and -m are: 1) -p will change the location of packages to be installed for the env and the module file will still be located at the $HOME/privatemodules directory as defined in use.own. 2) -m will only change the location of the module file. So the method to load modules created with -m and -p are different, see Example 3 for details.
- Example 3: Create a conda environment named labpackages in your group's Data Depot space and place the module file at a shared location for the group to use.
$ conda-env-mod create -p /depot/mylab/apps/labpackages -m /depot/mylab/etc/modules ... ... ... Preparing transaction: ...working... done Verifying transaction: ...working... done Executing transaction: ...working... done +-------------------------------------------------------+ | To use this environment, load the following modules: | | module use /depot/mylab/etc/modules | | module load conda-env/labpackages-py3.8.5 | +-------------------------------------------------------+ Your environment "labpackages" was created successfully.
If you used a custom module file location, you need to run the module use command as printed by the command output above.
By default, only the environment and a module file are created (no Jupyter kernel). If you plan to use your environment in a JupyterHub notebook, you need to append a --jupyter flag to the above commands.
- Example 4: Create a Jupyter-enabled conda environment named labpackages in your group's Data Depot space and place the module file at a shared location for the group to use.
$ conda-env-mod create -p /depot/mylab/apps/labpackages -m /depot/mylab/etc/modules --jupyter ... ... ... Jupyter kernel created: "Python (My labpackages Kernel)" ... ... ... Your environment "labpackages" was created successfully.
Link to section 'Step 2: Load the conda environment' of 'Installing Packages' Step 2: Load the conda environment
-
The following instructions assume that you have used conda-env-mod script to create an environment named mypackages (Examples 1 or 2 above). If you used conda create instead, please use conda activate mypackages.
$ module load use.own $ module load conda-env/mypackages-py3.8.5
Note that the conda-env module name includes the Python version that it supports (Python 3.8.5 in this example). This is same as the Python version in the conda module.
-
If you used a custom module file location (Example 3 above), please use module use to load the conda-env module.
$ module use /depot/mylab/etc/modules $ module load conda-env/labpackages-py3.8.5
Link to section 'Step 3: Install packages' of 'Installing Packages' Step 3: Install packages
Now you can install custom packages in the environment using either conda install or pip install.
Link to section 'Installing with conda' of 'Installing Packages' Installing with conda
-
Example 1: Install OpenCV (open-source computer vision library) using conda.
$ conda install opencv
-
Example 2: Install a specific version of OpenCV using conda.
$ conda install opencv=4.5.5
-
Example 3: Install OpenCV from a specific anaconda channel.
$ conda install -c anaconda opencv
Link to section 'Installing with pip' of 'Installing Packages' Installing with pip
-
Example 4: Install pandas using pip.
$ pip install pandas
-
Example 5: Install a specific version of pandas using pip.
$ pip install pandas==1.4.3
Follow the on-screen instructions while the packages are being installed. If installation is successful, please proceed to the next section to test the packages.
Note: Do NOT run Pip with the --user argument, as that will install packages in a different location and might mess up your account environment.
Link to section 'Step 4: Test the installed packages' of 'Installing Packages' Step 4: Test the installed packages
To use the installed Python packages, you must load the module for your conda environment. If you have not loaded the conda-env module, please do so following the instructions at the end of Step 1.
$ module load use.own
$ module load conda-env/mypackages-py3.8.5
- Example 1: Test that OpenCV is available.
$ python -c "import cv2; print(cv2.__version__)"
- Example 2: Test that pandas is available.
$ python -c "import pandas; print(pandas.__version__)"
If the commands finished without errors, then the installed packages can be used in your program.
Link to section 'Additional capabilities of conda-env-mod script' of 'Installing Packages' Additional capabilities of conda-env-mod script
The conda-env-mod tool is intended to facilitate creation of a minimal Anaconda environment, matching module file and optionally a Jupyter kernel. Once created, the environment can then be accessed via familiar module load command, tuned and expanded as necessary. Additionally, the script provides several auxiliary functions to help manage environments, module files and Jupyter kernels.
General usage for the tool adheres to the following pattern:
$ conda-env-mod help
$ conda-env-mod <subcommand> <required argument> [optional arguments]
where required arguments are one of
- -n|--name ENV_NAME (name of the environment)
- -p|--prefix ENV_PATH (location of the environment)
and optional arguments further modify behavior for specific actions (e.g. -m to specify alternative location for generated module files).
Given a required name or prefix for an environment, the conda-env-mod script supports the following subcommands:
- create - to create a new environment, its corresponding module file and optional Jupyter kernel.
- delete - to delete existing environment along with its module file and Jupyter kernel.
- module - to generate just the module file for a given existing environment.
- kernel - to generate just the Jupyter kernel for a given existing environment (note that the environment has to be created with a --jupyter option).
- help - to display script usage help.
Using these subcommands, you can iteratively fine-tune your environments, module files and Jupyter kernels, as well as delete and re-create them with ease. Below we cover several commonly occurring scenarios.
Note: When you try to use conda-env-mod delete, remember to include the arguments as you create the environment (i.e. -p package_location and/or -m module_location).
Link to section 'Generating module file for an existing environment' of 'Installing Packages' Generating module file for an existing environment
If you already have an existing configured Anaconda environment and want to generate a module file for it, follow appropriate examples from Step 1 above, but use the module subcommand instead of the create one. E.g.
$ conda-env-mod module -n mypackages
and follow printed instructions on how to load this module. With an optional --jupyter flag, a Jupyter kernel will also be generated.
Note that the module name mypackages should be exactly the same with the older conda environment name. Note also that if you intend to proceed with a Jupyter kernel generation (via the --jupyter flag or a kernel subcommand later), you will have to ensure that your environment has ipython and ipykernel packages installed into it. To avoid this and other related complications, we highly recommend making a fresh environment using a suitable conda-env-mod create .... --jupyter command instead.
Link to section 'Generating Jupyter kernel for an existing environment' of 'Installing Packages' Generating Jupyter kernel for an existing environment
If you already have an existing configured Anaconda environment and want to generate a Jupyter kernel file for it, you can use the kernel subcommand. E.g.
$ conda-env-mod kernel -n mypackages
This will add a "Python (My mypackages Kernel)" item to the dropdown list of available kernels upon your next login to the JupyterHub.
Note that generated Jupiter kernels are always personal (i.e. each user has to make their own, even for shared environments). Note also that you (or the creator of the shared environment) will have to ensure that your environment has ipython and ipykernel packages installed into it.
Link to section 'Managing and using shared Python environments' of 'Installing Packages' Managing and using shared Python environments
Here is a suggested workflow for a common group-shared Anaconda environment with Jupyter capabilities:
The PI or lab software manager:
-
Creates the environment and module file (once):
$ module purge $ module load conda $ conda-env-mod create -p /depot/mylab/apps/labpackages -m /depot/mylab/etc/modules --jupyter
-
Installs required Python packages into the environment (as many times as needed):
$ module use /depot/mylab/etc/modules $ module load conda-env/labpackages-py3.8.5 $ conda install ....... # all the necessary packages
Lab members:
-
Lab members can start using the environment in their command line scripts or batch jobs simply by loading the corresponding module:
$ module use /depot/mylab/etc/modules $ module load conda-env/labpackages-py3.8.5 $ python my_data_processing_script.py .....
-
To use the environment in Jupyter notebooks, each lab member will need to create his/her own Jupyter kernel (once). This is because Jupyter kernels are private to individuals, even for shared environments.
$ module use /depot/mylab/etc/modules $ module load conda-env/labpackages-py3.8.5 $ conda-env-mod kernel -p /depot/mylab/apps/labpackages
A similar process can be devised for instructor-provided or individually-managed class software, etc.
Link to section 'Troubleshooting' of 'Installing Packages' Troubleshooting
- Python packages often fail to install or run due to dependency incompatibility with other packages. More specifically, if you previously installed packages in your home directory it is safer to clean those installations.
$ mv ~/.local ~/.local.bak $ mv ~/.cache ~/.cache.bak
- Unload all the modules.
$ module purge
- Clean up PYTHONPATH.
$ unset PYTHONPATH
- Next load the modules (e.g. anaconda) that you need.
$ module load conda/2024.02-py311 $ module load use.own $ module load conda-env/2024.02-py311
- Now try running your code again.
- Few applications only run on specific versions of Python (e.g. Python 3.6). Please check the documentation of your application if that is the case.
Installing Packages from Source
We maintain several Anaconda installations. Anaconda maintains numerous popular scientific Python libraries in a single installation. If you need a Python library not included with normal Python we recommend first checking Anaconda. For a list of modules currently installed in the Anaconda Python distribution:
$ module load conda
$ conda list
# packages in environment at /apps/spack/bell/apps/anaconda/2020.02-py37-gcc-4.8.5-u747gsx:
#
# Name Version Build Channel
_ipyw_jlab_nb_ext_conf 0.1.0 py37_0
_libgcc_mutex 0.1 main
alabaster 0.7.12 py37_0
anaconda 2020.02 py37_0
...
If you see the library in the list, you can simply import it into your Python code after loading the Anaconda module.
If you do not find the package you need, you should be able to install the library in your own Anaconda customization. First try to install it with Conda or Pip. If the package is not available from either Conda or Pip, you may be able to install it from source.
Use the following instructions as a guideline for installing packages from source. Make sure you have a download link to the software (usually it will be a tar.gz
archive file). You will substitute it on the wget line below.
We also assume that you have already created an empty conda environment as described in our Python package installation guide.
$ mkdir ~/src
$ cd ~/src
$ wget http://path/to/source/tarball/app-1.0.tar.gz
$ tar xzvf app-1.0.tar.gz
$ cd app-1.0
$ module load conda
$ module load use.own
$ module load conda-env/mypackages-py3.8.5
$ python setup.py install
$ cd ~
$ python
>>> import app
>>> quit()
The "import app" line should return without any output if installed successfully. You can then import the package in your python scripts.
If you need further help or run into any issues installing a library, contact us or drop by Coffee Hour for in-person help.
For more information about Python:
Example: Create and Use Biopython Environment with Conda
Link to section 'Using conda to create an environment that uses the biopython package' of 'Example: Create and Use Biopython Environment with Conda' Using conda to create an environment that uses the biopython package
To use Conda you must first load the anaconda module:
module load conda
Create an empty conda environment to install biopython:
conda-env-mod create -n biopython
Now activate the biopython environment:
module load use.own
module load conda-env/biopython-py3.12.5
Install the biopython packages in your environment:
conda install --channel anaconda biopython -y
Fetching package metadata ..........
Solving package specifications .........
.......
Linking packages ...
[ COMPLETE ]|################################################################
The --channel option specifies that it searches the anaconda channel for the biopython package. The -y argument is optional and allows you to skip the installation prompt. A list of packages will be displayed as they are installed.
Remember to add the following lines to your job submission script to use the custom environment in your jobs:
module load conda
module load use.own
module load conda-env/biopython-py3.12.5
If you need further help or run into any issues with creating environments, contact us or drop by Coffee Hour for in-person help.
For more information about Python:
Numpy Parallel Behavior
The widely available Numpy package is the best way to handle numerical computation in Python. The numpy
package provided by our anaconda
modules is optimized using Intel's MKL library. It will automatically parallelize many operations to make use of all the cores available on a machine.
In many contexts that would be the ideal behavior. On the cluster however that very likely is not in fact the preferred behavior because often more than one user is present on the system and/or more than one job on a node. Having multiple processes contend for those resources will actually result in lesser performance.
Setting the MKL_NUM_THREADS
or OMP_NUM_THREADS
environment variable(s) allows you to control this behavior. Our anaconda modules automatically set these variables to 1 if and only if you do not currently have that variable defined.
When submitting batch jobs it is always a good idea to be explicit rather than implicit. If you are submitting a job that you want to make use of the full resources available on the node, set one or both of these variables to the number of cores you want to allow numpy to make use of.
#!/bin/bash
module load conda
export MKL_NUM_THREADS=16
...
If you are submitting multiple jobs that you intend to be scheduled together on the same node, it is probably best to restrict numpy to a single core.
#!/bin/bash
module load conda
export MKL_NUM_THREADS=1
R
R, a GNU project, is a language and environment for data manipulation, statistics, and graphics. It is an open source version of the S programming language. R is quickly becoming the language of choice for data science due to the ease with which it can produce high quality plots and data visualizations. It is a versatile platform with a large, growing community and collection of packages.
For more general information on R visit The R Project for Statistical Computing.
Running R jobs
This section illustrates how to submit a small R job to a SLURM queue. The example job computes a Pythagorean triple.
Prepare an R input file with an appropriate filename, here named myjob.R:
# FILENAME: myjob.R
# Compute a Pythagorean triple.
a = 3
b = 4
c = sqrt(a*a + b*b)
c # display result
Prepare a job submission file with an appropriate filename, here named myjob.sub:
#!/bin/bash
# FILENAME: myjob.sub
module load r
# --vanilla:
# --no-save: do not save datasets at the end of an R session
R --vanilla --no-save < myjob.R
For other examples or R jobs:
Installing R packages
Link to section 'Challenges of Managing R Packages in the Cluster Environment' of 'Installing R packages' Challenges of Managing R Packages in the Cluster Environment
- Different clusters have different hardware and softwares. So, if you have access to multiple clusters, you must install your R packages separately for each cluster.
- Each cluster has multiple versions of R and packages installed with one version of R may not work with another version of R. So, libraries for each R version must be installed in a separate directory.
- You can define the directory where your R packages will be installed using the environment variable
R_LIBS_USER
. - For your convenience, a sample ~/.Rprofile example file is provided that can be downloaded to your cluster account and renamed into
~/.Rprofile
(or appended to one) to customize your installation preferences. Detailed instructions.
Link to section 'Installing Packages' of 'Installing R packages' Installing Packages
-
Step 0: Set up installation preferences.
Follow the steps for setting up your~/.Rprofile
preferences. This step needs to be done only once. If you have created a~/.Rprofile
file previously on Gilbreth, ignore this step. -
Step 1: Check if the package is already installed.
As part of the R installations on community clusters, a lot of R libraries are pre-installed. You can check if your package is already installed by opening an R terminal and entering the commandinstalled.packages()
. For example,module load r/4.4.1 R
installed.packages()["units",c("Package","Version")] Package Version "units" "0.8-1" quit()
If the package you are trying to use is already installed, simply load the library, e.g.,
library('units')
. Otherwise, move to the next step to install the package. -
Step 2: Load required dependencies. (if needed)
For simple packages you may not need this step. However, some R packages depend on other libraries. For example, thesf
package depends ongdal
andgeos
libraries. So, you will need to load the corresponding modules before installingsf
. Read the documentation for the package to identify which modules should be loaded.module load gdal module load geos
-
Step 3: Install the package.
Now install the desired package using the commandinstall.packages('package_name')
. R will automatically download the package and all its dependencies from CRAN and install each one. Your terminal will show the build progress and eventually show whether the package was installed successfully or not.R
install.packages('sf', repos="https://cran.case.edu/") Installing package into ‘/home/myusername/R/x86_64-pc-linux-gnu-library/4.4.1’ (as ‘lib’ is unspecified) trying URL 'https://cran.case.edu/src/contrib/sf_0.9-7.tar.gz' Content type 'application/x-gzip' length 4203095 bytes (4.0 MB) ================================================== downloaded 4.0 MB ... ... more progress messages ... ... ** testing if installed package can be loaded from final location ** testing if installed package keeps a record of temporary installation path * DONE (sf) The downloaded source packages are in ‘/tmp/RtmpSVAGio/downloaded_packages’
- Step 4: Troubleshooting. (if needed)
If Step 3 ended with an error, you need to investigate why the build failed. Most common reason for build failure is not loading the necessary modules.
Link to section 'Loading Libraries' of 'Installing R packages' Loading Libraries
Once you have packages installed you can load them with the library()
function as shown below:
library('packagename')
The package is now installed and loaded and ready to be used in R.
Link to section 'Example: Installing dplyr' of 'Installing R packages' Example: Installing dplyr
The following demonstrates installing the dplyr
package assuming the above-mentioned custom ~/.Rprofile
is in place (note its effect in the "Installing package into" information message):
module load r
R
install.packages('dplyr', repos="http://ftp.ussg.iu.edu/CRAN/")
Installing package into ‘/home/myusername/R/gilbreth/4.4.1’
(as ‘lib’ is unspecified)
...
also installing the dependencies 'crayon', 'utf8', 'bindr', 'cli', 'pillar', 'assertthat', 'bindrcpp', 'glue', 'pkgconfig', 'rlang', 'Rcpp', 'tibble', 'BH', 'plogr'
...
...
...
The downloaded source packages are in
'/tmp/RtmpHMzm9z/downloaded_packages'
library(dplyr)
Attaching package: 'dplyr'
For more information about installing R packages:
Loading Data into R
R is an environment for manipulating data. In order to manipulate data, it must be brought into the R environment. R has a function to read any file that data is stored in. Some of the most common file types like comma-separated variable(CSV) files have functions that come in the basic R packages. Other less common file types require additional packages to be installed. To read data from a CSV file into the R environment, enter the following command in the R prompt:
> read.csv(file = "path/to/data.csv", header = TRUE)
When R reads the file it creates an object that can then become the target of other functions. By default the read.csv() function will give the object the name of the .csv file. To assign a different name to the object created by read.csv enter the following in the R prompt:
> my_variable <- read.csv(file = "path/to/data.csv", header = FALSE)
To display the properties (structure) of loaded data, enter the following:
> str(my_variable)
For more functions and tutorials:
RStudio
RStudio is a graphical integrated development environment (IDE) for R. RStudio is the most popular environment for developing both R scripts and packages. RStudio is provided on most Research systems.
There are two methods to launch RStudio on the cluster: command-line and application menu icon.
Link to section 'Launch RStudio by the command-line:' of 'RStudio' Launch RStudio by the command-line:
module load gcc
module load r
module load rstudio
rstudio
Note that RStudio is a graphical program and in order to run it you must have a local X11 server running or use Thinlinc Remote Desktop environment. See the ssh X11 forwarding section for more details.
Link to section 'Launch Rstudio by the application menu icon:' of 'RStudio' Launch Rstudio by the application menu icon:
- Log into desktop.gilbreth.rcac.purdue.edu with web browser or ThinLinc client
- Click on the
Applications
drop down menu on the top left corner - Choose
Cluster Software
and thenRStudio
R and RStudio are free to download and run on your local machine. For more information about RStudio:
Setting Up R Preferences with .Rprofile
For your convenience, a sample ~/.Rprofile example file is provided that can be downloaded to your cluster account and renamed into ~/.Rprofile
(or appended to one). Follow these steps to download our recommended ~/.Rprofile
example and copy it into place:
curl -#LO https://www.rcac.purdue.edu/files/knowledge/run/examples/apps/r/Rprofile_example
mv -ib Rprofile_example ~/.Rprofile
The above installation step needs to be done only once on Gilbreth. Now load the R module and run R:
module load r/4.4.1
R
.libPaths()
[1] "/home/myusername/R/gilbreth/4.1.2-gcc-6.3.0-ymdumss"
[2] "/apps/spack/gilbreth/apps/r/4.1.2-gcc-6.3.0-ymdumss/rlib/R/library"
.libPaths()
should output something similar to above if it is set up correctly.
You are now ready to install R packages into the dedicated directory /home/myusername/R/gilbreth/4.1.2-gcc-6.3.0-ymdumss
.
Singularity
On Gilbreth, Singularity functionality is provided by Apptainer - see Apptainer section for details.
NGC (Nvidia GPU Cloud)
Link to section 'What is NGC?' of 'NGC (Nvidia GPU Cloud)' What is NGC?
Nvidia GPU cloud (NGC) is a GPU-accelerated cloud platform optimized for deep learning and scientific computing. NGC offers a comprehensive catalogue of GPU-accelerated containers, so the application runs quickly and reliably on the high performance computing environment. NGC was deployed to extend the cluster capabilities and to enable powerful software and deliver the fastest results. By utilizing Singularity and NGC, users can focus on building lean models, producing optimal solutions and gathering faster insights. For more information, please visit https://www.nvidia.com/en-us/gpu-cloud and NGC software catalog.
Link to section 'Getting Started' of 'NGC (Nvidia GPU Cloud)' Getting Started
Users can download containers from the NGC software catalog and run them directly using Singularity instructions from the corresponding container’s catalog page.
In addition, a subset of pre-downloaded NGC containers wrapped into convenient software modules are provided. These modules wrap underlying complexity and provide the same commands that are expected from non-containerized versions of each application.
On Gilbreth, type the command below to see the lists of NGC containers we deployed.
$ module load ngc
$ module avail
Link to section 'Example' of 'NGC (Nvidia GPU Cloud)' Example
This example demonstrates how to run LAMMPS with NGC modules.
First, let's prepare the run folder and download the input file for the example we are going to run.
$ cd $CLUSTER_SCRATCH
$ mkdir -p lammps_ngc
$ cd lammps_ngc
$ wget https://lammps.sandia.gov/inputs/in.lj.txt
Then load the ngc and lammps modules
$ module load ngc
$ module load lammps/29Oct2020
Finally we can set variables and start running lammps.
$ gpu_count=1
$ input=in.lj.txt
$ mpirun -n ${gpu_count} lmp -k on g ${gpu_count} -sf kk -pk kokkos cuda/aware on neigh full comm device binsize 2.8 -var x 8 -var y 4 -var z 8 -in ${input}
For more information, see each application’s NGC catalog page . For applications deployed as modules, see module help command for direct link to the relevant page (e.g. module help lammps/29Oct2020 in the above example).
Ansys Fluent
Ansys is a CAE/multiphysics engineering simulation software that utilizes finite element analysis for numerically solving a wide variety of mechanical problems. The software contains a list of packages and can simulate many structural properties such as strength, toughness, elasticity, thermal expansion, fluid dynamics as well as acoustic and electromagnetic attributes.
Link to section 'Ansys Licensing' of 'Ansys Fluent' Ansys Licensing
The Ansys licensing on our community clusters is maintained by Purdue ECN group. There are two types of licenses: teaching and research. For more information, please refer to ECN Ansys licensing page. If you are interested in purchasing your own research license, please send email to software@ecn.purdue.edu.
Link to section 'Ansys Workflow' of 'Ansys Fluent' Ansys Workflow
Ansys software consists of several sub-packages such as Workbench and Fluent. Most simulations are performed using the Ansys Workbench console, a GUI interface to manage and edit the simulation workflow. It requires X11 forwarding for remote display so a SSH client software with X11 support or a remote desktop portal is required. Please see Logging In section for more details. To ensure preferred performance, ThinLinc remote desktop connection is highly recommended.
Typically users break down larger structures into small components in geometry with each of them modeled and tested individually. A user may start by defining the dimensions of an object, adding weight, pressure, temperature, and other physical properties.
Ansys Fluent is a computational fluid dynamics (CFD) simulation software known for its advanced physics modeling capabilities and accuracy. Fluent offers unparalleled analysis capabilities and provides all the tools needed to design and optimize new equipment and to troubleshoot existing installations.
In the following sections, we provide step-by-step instructions to lead you through the process of using Fluent. We will create a classical elbow pipe model and simulate the fluid dynamics when water flows through the pipe. The project files have been generated and can be downloaded via fluent_tutorial.zip.
Link to section 'Loading Ansys Module' of 'Ansys Fluent' Loading Ansys Module
Different versions of Ansys are installed on the clusters and can be listed with module spider
or module avail
command in the terminal.
$ module avail ansys/
---------------------- Core Applications -----------------------------
ansys/2019R3 ansys/2020R1 ansys/2021R2 ansys/2022R1 (D)
Before launching Ansys Workbench, a specific version of Ansys module needs to be loaded. For example, you can module load ansys/2021R2
to use the latest Ansys 2021R2. If no version is specified, the default module -> (D) (ansys/2022R1
in this case) will be loaded. You can also check the loaded modules with module list
command.
Link to section 'Launching Ansys Workbench' of 'Ansys Fluent' Launching Ansys Workbench
Open a terminal on Gilbreth, enter rcac-runwb2
to launch Ansys Workbench.
You can also use runwb2
to launch Ansys Workbench. The main difference between runwb2
and rcac-runwb2
is that the latter sets the project folder to be in your scratch space. Ansys has an known bug that it might crash when the project folder is set to $HOME
on our systems.
Preparing Case Files for Fluent
Link to section 'Creating a Fluent fluid analysis system' of 'Preparing Case Files for Fluent' Creating a Fluent fluid analysis system
In the Ansys Workbench, create a new fluid flow analysis by double-clicking the Fluid Flow (Fluent) option under the Analysis Systems in the Toolbox on the left panel. You can also drag-and-drop the analysis system into the Project Schematic. A green dotted outline indicating a potential location for the new system initially appears in the Project Schematic. When you drag the system to one of the outlines, it turns into a red box to indicate the chosen location of the new system.
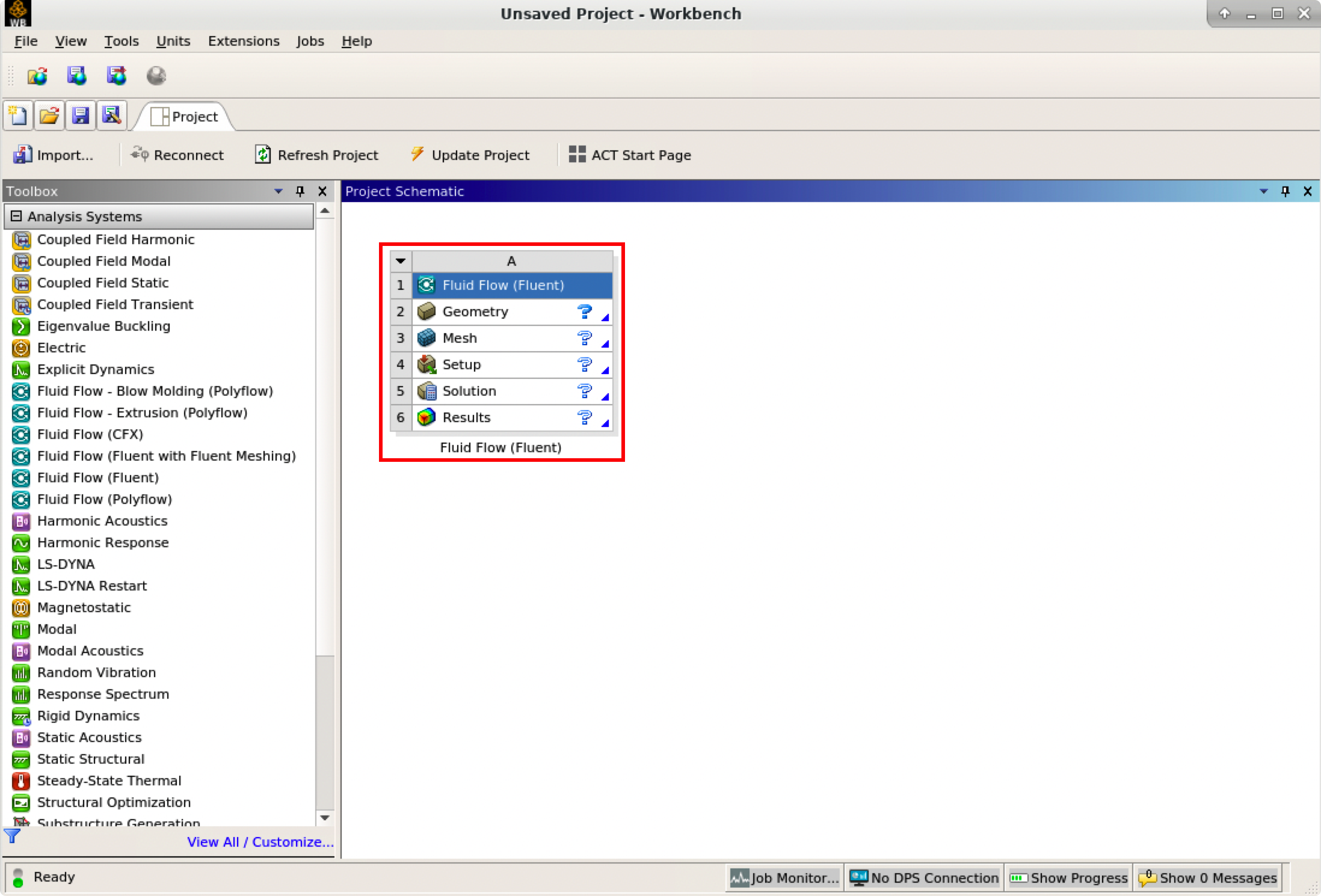
The red rectangle indicates the Fluid Flow system for Fluent, which includes all the essential workflows from “2 Geometry” to “6 Results”. You can rename it and carry out the necessary step-by-step procedures by double-clicking the corresponding cells.
It is important to save the project. Ansys Workbench saves the project with a .wbpj
extension and also all the supporting files into a folder with the same name. In this case, a file named elbow_demo.wbpj
and a folder $Ansys_PROJECT_FOLDER/elbow_demo_files/
are created in the Ansys project folder:
$ ll
total 33
drwxr-xr-x 7 myusername itap 9 Mar 3 17:47 elbow_demo_files
-rw-r--r-- 1 myusername itap 42597 Mar 3 17:47 elbow_demo.wbpj
You should always “Update Project” and save it after finishing a procedure.
Link to section 'Creating Geometry in the Ansys DesignModeler' of 'Preparing Case Files for Fluent' Creating Geometry in the Ansys DesignModeler
Create a geometry in the Ansys DesignModeler (by double-clicking “Geometry” cell in workflow), or import the appropriate geometry file (by right-clicking the Geometry cell and selecting “Import Geometry” option from the context menu).
You can use Ansys DesignModeler to create 2D/3D geometries or even draw the objects yourself. In our example, we created only half of the elbow pipe because the symmetry of the structure is taken into account to reduce the computation intensity.
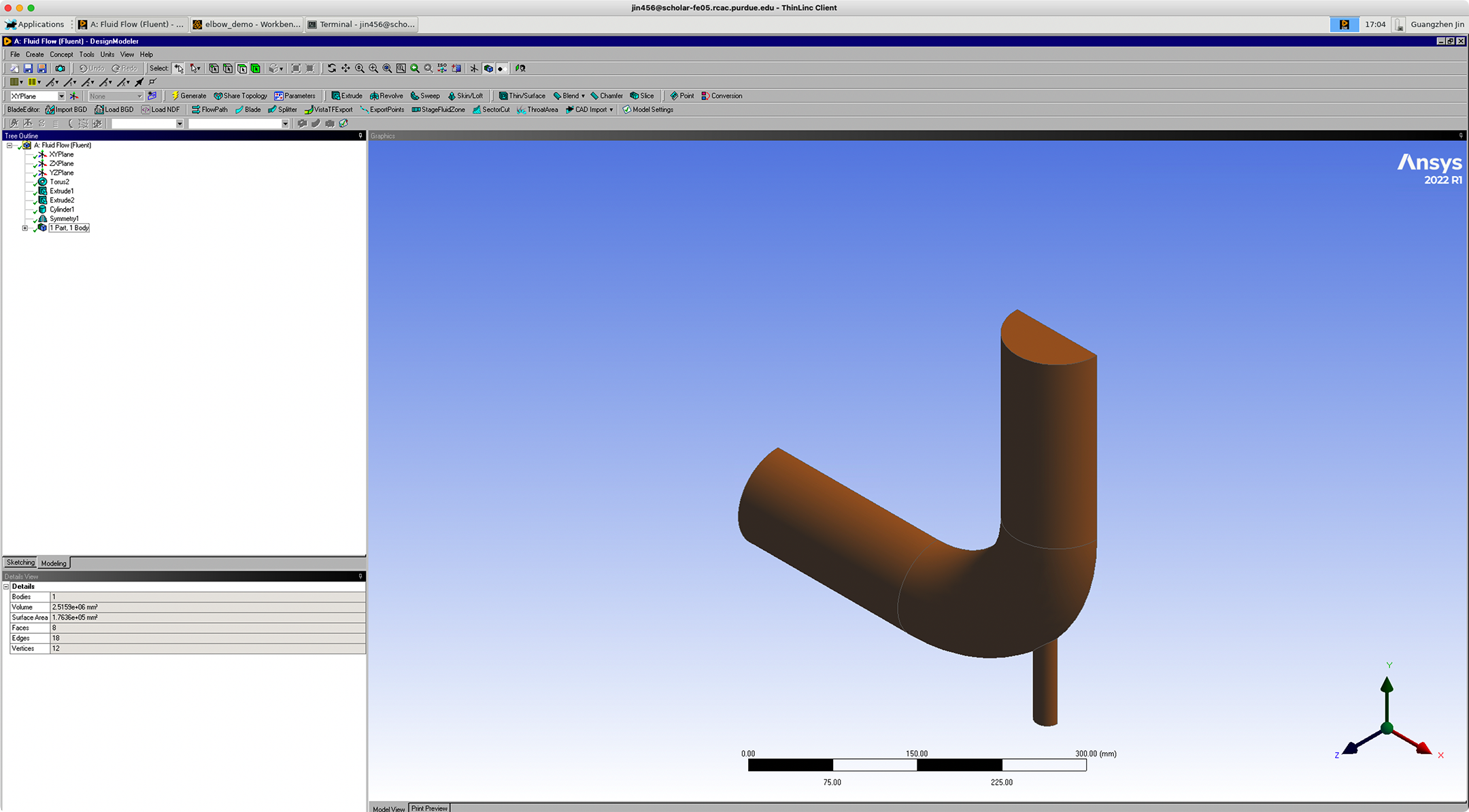
After saving the geometry, a geometry file FFF.agdb
will be created in the folder: $Ansys_PROJECT_FOLDER/elbow_demo_file/dp0/FFF/DM/
. The project in Workbench will be updated automatically.
If you import a pre-existing geometry into Ansys DesignModeler, it will also generate this file with the same filename at this location.
Link to section 'Creating mesh in the Ansys Meshing' of 'Preparing Case Files for Fluent' Creating mesh in the Ansys Meshing
Now that we have created the elbow pipe geometry, a computational mesh can be generated by the Meshing application throughout the flow volume.
With the successful creation of the geometry, there should be a green check showing the completion of “Geometry” in the Ansys Workbench. A Refresh Required icon within the “Mesh” cell indicates the mesh needs to be updated and refreshed for the system.
Then it’s time to open the Ansys Meshing application by double-clicking the “Mesh” cell and editing the mesh for the project. Generally, there are several steps we need to take to define the mesh:
- Create names for all geometry boundaries such as the inlets, outlets and fluid body. Note: You can use the strings “velocity inlet” and “pressure outlet” in the named selections (with or without hyphens or underscore characters) to allow Ansys Fluent to automatically detect and assign the corresponding boundary types accordingly. Use “Fluid” for the body to let Ansys Fluent automatically detect that the volume is a fluid zone and treat it accordingly.
- Set basic meshing parameters for the Ansys Meshing application. Here are several important parameters you may need to assign: Sizing, Quality, Body Sizing Control, Inflation.
- Select “Generate” to generate the mesh and “Update” to update the mesh into the system. Note: Once the mesh is generated, you can view the mesh statistics by opening the Statistics node in the Details of “Mesh” view. This will display information such as the number of nodes and the number of elements, which gives you a general idea for the future computational resources and time.
After generation and updating the mesh, a mesh file FFF.msh
will be generated in folder $Ansys_PROJECT_FOLDER/elbow_demo_file/dp0/FFF/MECH/
and a mesh database file FFF.mshdb
will be generated in folder $Ansys_PROJECT_FOLDER/elbow_demo_file/dp0/global/MECH/
.
Parameters used in demo case (use default if not assigned):
- Length Unit=”mm”
- Names defined for geometry:
- velocity-inlet-large (large inlet on pipe);
- velocity-inlet-small (small inlet on pipe);
- pressure-outlet (outlet on pipe);
- symmetry (symmetry surface);
- Fluid (body);
- Mesh:
- Quality: Smoothing=”high”;
- Inflation: Use Automatic Inflation=“Program Controlled”, Inflation Option=”Smooth Transition”;
- Statistics:
- Nodes=29371;
- Elements=87647.
Link to section 'Calculation with Fluent' of 'Preparing Case Files for Fluent' Calculation with Fluent
Now all the preparations have been ready for the numerical calculation in Ansys Fluent. Both “Geometry” and “Mesh” cells should have green checks on. We can set up the CFD simulation parameters in Ansys Fluent by double-clicking the “Setup” cell.
When Ansys Fluent is first started or by selecting “editing” on the “Setup” cell, the Fluent Launcher is displayed, enabling you to view and/or set certain Ansys Fluent start-up options (e.g. Precision, Parallel, Display). Note that “Dimension” is fixed to “3D” because we are using a 3D model in this project.
After the Fluent is opened, an Ansys Fluent settings file FFF.set
is written under the folder $Ansys_PROJECT_FOLDER/elbow_demo_file/dp0/FFF/Fluent/
.
Then we are going to set up all the necessary parameters for Fluent computation. Here are the key steps for the setup:
- Setting up the domain:
- Change the units for length to be consistent with the Mesh;
- Check the mesh statistics and quality;
- Setting up physics:
- Solver: “Energy”, “Viscous Model”, “Near-Wall Treatment”;
- Materials;
- Zones;
- Boundaries: Inlet, Outlet, Internal, Symmetry, Wall;
- Solving:
- Solution Methods;
- Reports;
- Initialization;
- Iterations and output frequency.
Then the calculation will be carried out and the results will be written out into FFF-1.cas.gz
under folder $Ansys_PROJECT_FOLDER/elbow_demo_file/dp0/FFF/Fluent/
.
This file contains all the settings and simulation results which can be loaded for post analysis and re-computation (more details will be introduced in the following sections). If only configurations and settings within the Fluent are needed, we can open independent Fluent or submit Fluent jobs with bash commands by loading the existing case in order to facilitate the computation process.
Parameters used in demo case (use default if not assigned):
- Domain Setup: Length Units=”mm”;
- Solver: Energy=”on”; Viscous Model=”k-epsilon”; Near-Wall Treatment=”Enhanced Wall Treatment”;
- Materials: water (Density=1000[kg/m^3]; Specific Heat=4216[J/kg-k]; Thermal Conductivity=0.677[w/m-k]; Viscosity=8e-4[kg/m-s]);
- Zones=”fluid (water)”;
- Inlet=”velocity-inlet-large” (Velocity Magnitude=0.4m/s, Specification Method=”Intensity and Hydraulic Diameter”, Turbulent Intensity=5%; Hydraulic Diameter=100mm; Thermal Temperature=293.15k) &”velocity-inlet-small” (Velocity Magnitude=1.2m/s, Specification Method=”Intensity and Hydraulic Diameter”, Turbulent Intensity=5%; Hydraulic Diameter=25mm; Thermal Temperature=313.15k); Internal=”interior-fluid”; Symmetry=”symmetry”; Wall=”wall-fluid”;
- Solution Methods: Gradient=”Green-Gauss Node Based”;
- Report: plot residual and “Facet Maximum” for “pressure-outlet”
- Hybrid Initialization;
- 300 iterations.
Case Calculating with Fluent
Link to section 'Calculation with Fluent' of 'Case Calculating with Fluent' Calculation with Fluent
Now all the files are ready for the Fluent calculations. Both “Geometry” and “Mesh” cells should have green checks. We can set up the CFD simulation parameters in the Ansys Fluent by double-clicking the “Setup” cell.
Ansys Fluent Launcher can be started by selecting “editing” on the “Setup” cell with many startup options (e.g. Precision, Parallel, Display). Note that “Dimension” is fixed to “3D” because we are using a 3D model in this project.
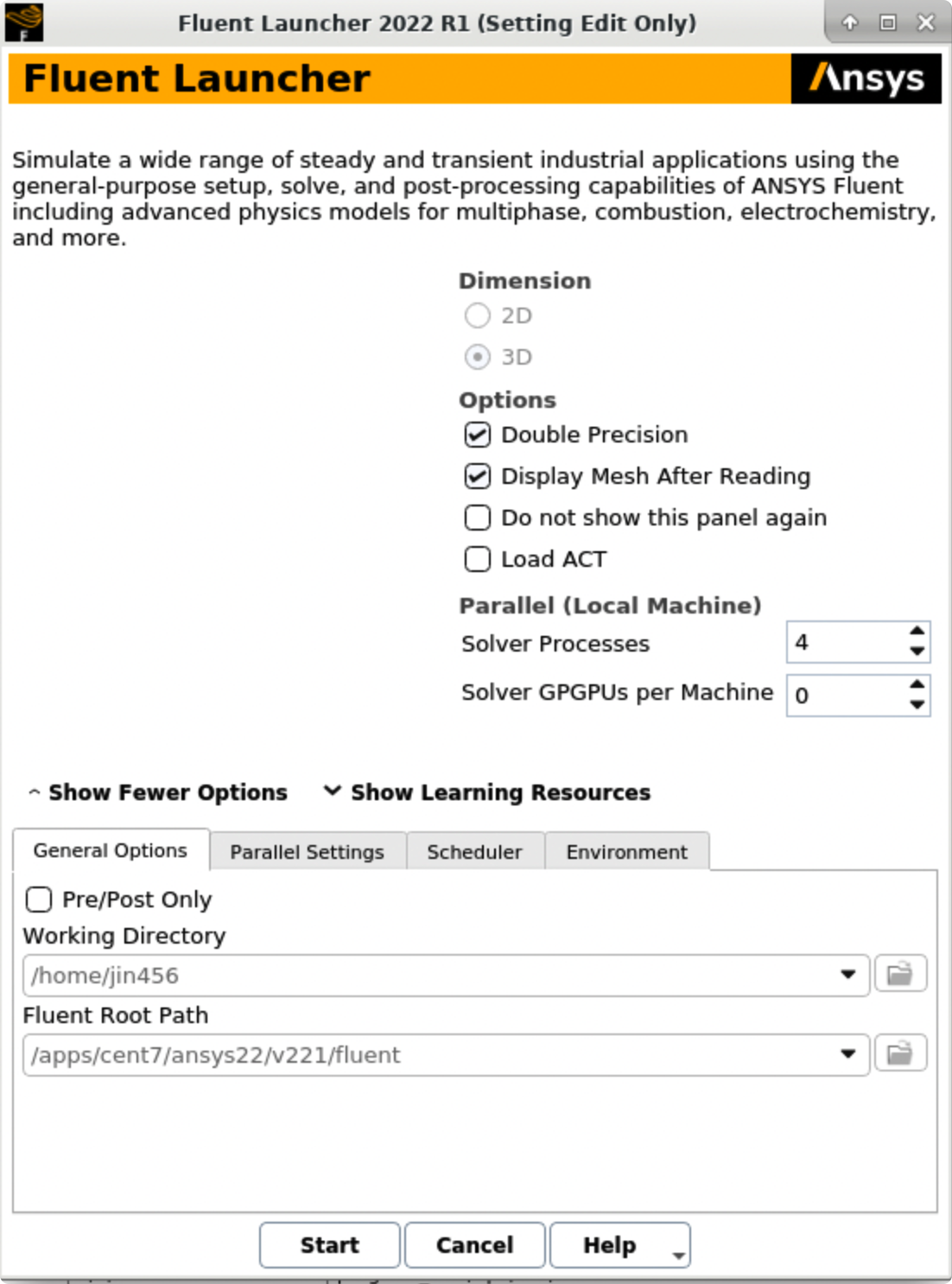
After the Fluent is opened, an Ansys Fluent settings file FFF.set
is written under the folder $Ansys_PROJECT_FOLDER/elbow_demo_file/dp0/FFF/Fluent/
.
Then we are going to set up all the necessary parameters for Fluent computation. Here are the key steps for the setup:
- Setting up the domain:
- Change the units for length to be consistent with the Mesh;
- Check the mesh statistics and quality;
- Setting up physics:
- Solver: “Energy”, “Viscous Model”, “Near-Wall Treatment”;
- Materials;
- Zones;
- Boundaries: Inlet, Outlet, Internal, Symmetry, Wall;
- Solving:
- Solution Methods;
- Reports;
- Initialization;
- Iterations and output frequency.
Then the calculation will be carried out and the results will be written out into FFF-1.cas.gz
under folder $Ansys_PROJECT_FOLDER/elbow_demo_file/dp0/FFF/Fluent/
.
This file contains all the settings and simulation results which can be loaded for post analysis and re-computation (more details will be introduced in the following sections). If only configurations and settings within the Fluent are needed, we can open independent Fluent or submit Fluent jobs with bash commands by loading the existing case in order to facilitate the computation process.
Parameters used in demo case (use default if not assigned):
- Domain Setup: Length Units=”mm”;
- Solver: Energy=”on”; Viscous Model=”k-epsilon”; Near-Wall Treatment=”Enhanced Wall Treatment”;
- Materials: water (Density=1000[kg/m^3]; Specific Heat=4216[J/kg-k]; Thermal Conductivity=0.677[w/m-k]; Viscosity=8e-4[kg/m-s]);
- Zones=”fluid (water)”;
- Inlet=”velocity-inlet-large” (Velocity Magnitude=0.4m/s, Specification Method=”Intensity and Hydraulic Diameter”, Turbulent Intensity=5%; Hydraulic Diameter=100mm; Thermal Temperature=293.15k) &”velocity-inlet-small” (Velocity Magnitude=1.2m/s, Specification Method=”Intensity and Hydraulic Diameter”, Turbulent Intensity=5%; Hydraulic Diameter=25mm; Thermal Temperature=313.15k); Internal=”interior-fluid”; Symmetry=”symmetry”; Wall=”wall-fluid”;
- Solution Methods: Gradient=”Green-Gauss Node Based”;
- Report: plot residual and “Facet Maximum” for “pressure-outlet”
- Hybrid Initialization;
- 300 iterations.
Link to section 'Results analysis' of 'Case Calculating with Fluent' Results analysis
The best methods to view and analyze the simulation should be the Ansys Fluent (directly after computation) or the Ansys CFD-Post (entering “Results” in Ansys Workbench). Both methods are straightforward so we will not cover this part in this tutorial. Here is a final simulation result showing the temperature of the symmetry after 300 iterations for reference:
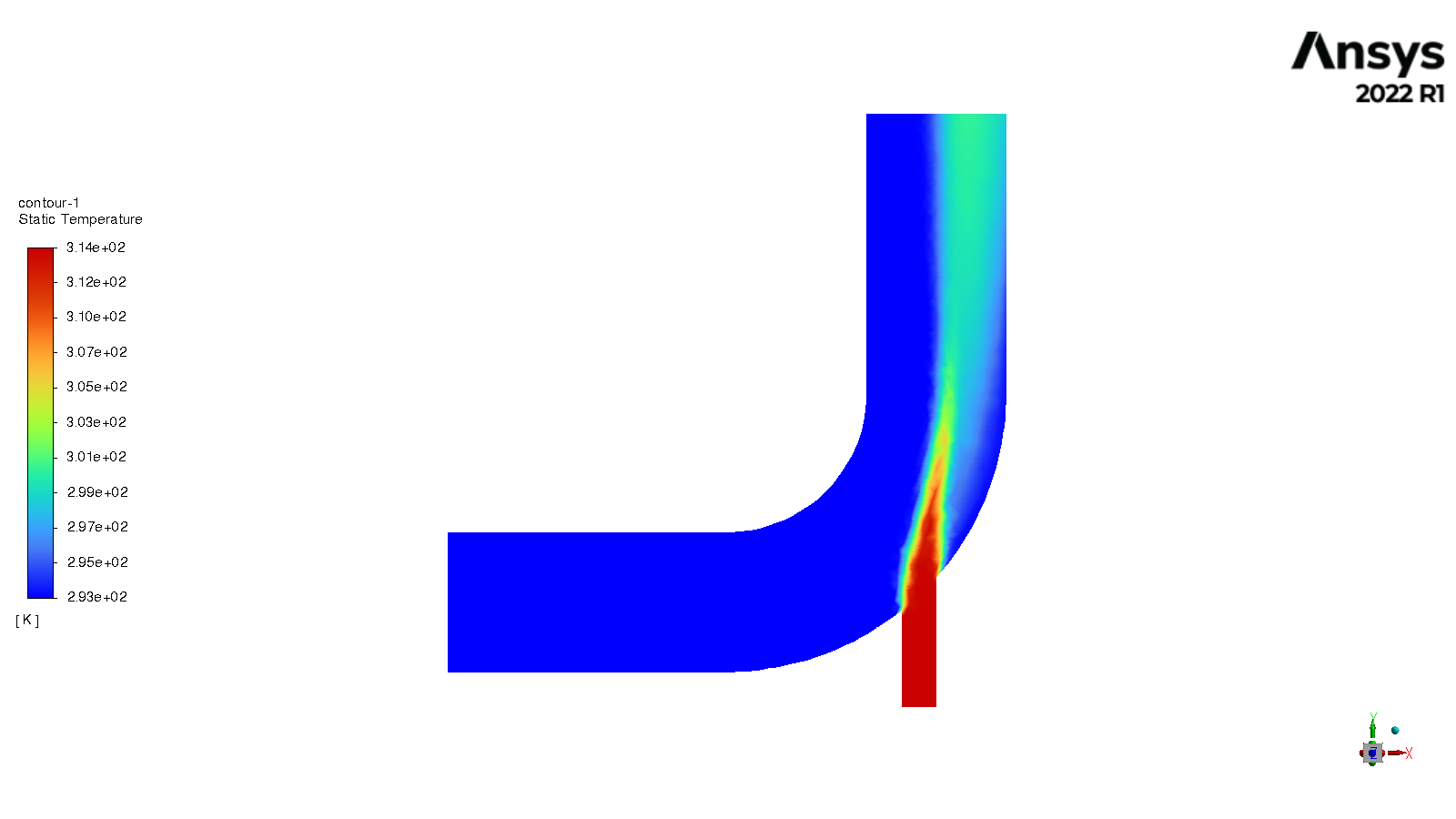
Fluent Text User Interface and Journal File
Link to section 'Fluent Text User Interface (TUI)' of 'Fluent Text User Interface and Journal File' Fluent Text User Interface (TUI)
If you pay attention to the “Console” window in the Fluent window when setting up and carrying out the calculation, corresponding commands can be found and executed one after another. Almost all the setting processes can be accomplished by the command lines, which is called Fluent Text User Interface (TUI). Here are the main commands in Fluent TUI:
adjoint/ parallel/ solve/
define/ plot/ surface/
display/ preferences/ turbo-workflow/
exit print-license-usage views/
file/ report/
mesh/ server/
For example, instead of opening a case by clicking buttons in Ansys Fluent, we can type /file read-case case_file_name.cas.gz
to open the saved case.
Link to section 'Fluent Journal Files' of 'Fluent Text User Interface and Journal File' Fluent Journal Files
A Fluent journal file is a series of TUI commands stored in a text file. The file can be written in a text editor or generated by Fluent as a transcript of the commands given to Fluent during your session.
A journal file generated by Fluent will include any GUI operations (in a TUI form, though). This is quite useful if you have a series of tasks that you need to execute, as it provides a shortcut. To record a journal file, start recording with File -> Write -> Start Journal..., perform whatever tasks you need, and then stop recording with File -> Write -> Stop Journal...
You can also write your own journal file into a text file. The basic rule for a Fluent journal file is to reproduce the TUI commands that controlled the configuration and calculation of Fluent in their order. You can add a comment in a line starting with a ;
(semicolon).
Here are some reasons why you should use a Fluent journal file:
- Using journal files with bash scripting can allow you to automate your jobs.
- Using journal files can allow you to parameterize your models easily and automatically.
- Using a journal file can set parameters you do not have in your case file e.g. autosaving.
- Using a journal file can allow you to safely save, stop and restart your jobs easily.
The order of your journal file commands is highly important. The correct sequences must be followed and some stages have multiple options e.g. different initialization methods.
Here is a sample Fluent journal file for the demo case:
;testJournal.jou
;Set the TUI version for Fluent
/file/set-tui-version "22.1"
;Read the case. The default folder
/file read-case /home/jin456/Fluent_files/tutorial_case1/elbow_files/dp0/FFF/Fluent/FFF-1.cas.gz
;Initialize the case with Hybrid Initialization
/solve/initialize/hyb-initialization
;Set Number of Iterations to 1000, Reporting Interval to 10 iterations and Profile Update Interval to 1 iteration
/solve/iterate 1000 10 1
;Outputting solver performance data upon completion of the simulation
/parallel timer usage
;Write out the simulation results.
/file write-case-data /home/jin456/Fluent_files/tutorial_case1/elbow_files/dp0/FFF/Fluent/result.cas.h5
;After computation, exit Flent
/exit
Before running this Fluent journal file, you need to make sure: 1) the ansys module has been loaded (it’s highly recommended to load the same version of Ansys when you built the case project); 2) the project case file (***.cas.gz
) has been created.
Then we can use Fluent to run this journal file by simply using:fluent 3ddp -t$NTASKS -g -i testJournal.jou
in the terminal. Here, 3d
indicates this is a 3d model, dp
indicates double precision, -t$NTASKS
tells Fluent how many Solver Processes it will take (e.g. -t4
), -g
means to run without the GUI or graphics, -i
testJournal.jou tells Fluent to read the specific journal file.
Here is a table for the available command line Options for Linux/UNIX and Windows Platforms in Ansys Fluent.
Option | Platform | Description |
---|---|---|
-cc |
all | Use the classic color scheme |
-ccp x |
Windows only | Use the Microsoft Job Scheduler where x is the head node name. |
-cnf=x |
all | Specify the hosts or machine list file |
-driver |
all | Sets the graphics driver (available drivers vary by platform - opengl or x11 or null(Linux/UNIX) - opengl or msw or null (Windows)) |
-env |
all | Show environment variables |
-fgw |
all | Disables the embedded graphics |
-g |
all | Run without the GUI or graphics (Linux/UNIX); Run with the GUI minimized (Windows) |
-gr |
all | Run without graphics |
-gu |
all | Run without the GUI but with graphics (Linux/UNIX); Run with the GUI minimized but with graphics (Windows) |
-help |
all | Display command line options |
-hidden |
Windows only | Run in batch mode |
-host_ip=host:ip |
all | Specify the IP interface to be used by the host process |
-i journal |
all | Reads the specified journal file |
-lsf |
Linux/UNIX only | Run FLUENT using LSF |
-mpi= |
all | Specify MPI implementation |
-mpitest |
all | Will launch an MPI program to collect network performance data |
-nm |
all | Do not display mesh after reading |
-pcheck |
Linux/UNIX only | Checks all nodes |
-post |
all | Run the FLUENT post-processing-only executable |
-p |
all | Choose the interconnect |
-r |
all | List all releases installed |
-rx |
all | Specify release number |
-sge |
Linux/UNIX only | Run FLUENT under Sun Grid Engine |
-sge queue |
Linux/UNIX only | Name of the queue for a given computing grid |
-sgeckpt ckpt_obj |
Linux/UNIX only | Set checkpointing object to ckpt_objfor SGE |
-sgepe fluent_pe min_n-max_n |
Linux/UNIX only | Set the parallel environment for SGE to fluent_pe, min_nand max_n are number of min and max nodes requested |
-tx |
all | Specify the number of processors x |
For more information for Fluent text user interface and journal files, please refer to Fluent FAQ.
Submitting Fluent jobs to SLURM
The Fluent simulations can also run in batch. In this section we provide an example script for submitting Fluent jobs to the SLURM scheduler. Please refer to the Running Jobs section of our user guide for detailed tutorials of submitting jobs.
#!/bin/bash
# Job script for submitting a FLUENT job on multiple cores on a single node
# Apply resources via SLURM
#SBATCH --nodes=1
#SBATCH --ntasks=4
#SBATCH --time=01:00:00
#SBATCH --job-name=fluent_test
#SBATCH -o fluent_test_%j.out
#SBATCH -e fluent_test_%j.err
# Loads Ansys and sets the application up
module purge
module load ansys/2022R1
#Initiating Fluent and reading input journal file
fluent 3ddp -t$NTASKS -g -i testJournal.jou
For more information about submitting Fluent jobs, please refer to Fluent FAQ .
Apptainer
Note: Apptainer was formerly known as Singularity and is now a part of the Linux Foundation. When migrating from Singularity see the user compatibility documentation.
Link to section 'What is Apptainer?' of 'Apptainer' What is Apptainer?
Apptainer is an open-source container platform designed to be simple, fast, and secure. It allows the portability and reproducibility of operating systems and application environments through the use of Linux containers. It gives users complete control over their environment.
Apptainer is like Docker but tuned explicitly for HPC clusters. More information is available on the project’s website.
Link to section 'Features' of 'Apptainer' Features
- Run the latest applications on an Ubuntu or Centos userland
- Gain access to the latest developer tools
- Launch MPI programs easily
- Much more
Apptainer’s user guide is available at: apptainer.org/docs/user/main/introduction.html
Link to section 'Example' of 'Apptainer' Example
Here is an example using an Ubuntu 16.04 image on Gilbreth:
apptainer exec /depot/itap/singularity/ubuntu1604.img cat /etc/lsb-release
DISTRIB_ID=Ubuntu
DISTRIB_RELEASE=16.04
DISTRIB_CODENAME=xenial
DISTRIB_DESCRIPTION="Ubuntu 16.04 LTS"
Here is another example using a Centos 7 image:
apptainer exec /depot/itap/singularity/centos7.img cat /etc/redhat-release
CentOS Linux release 7.3.1611 (Core)
Link to section 'Purdue Cluster Specific Notes' of 'Apptainer' Purdue Cluster Specific Notes
All service providers will integrate Apptainer slightly differently depending on site. The largest customization will be which default files are inserted into your images so that routine services will work.
Services we configure for your images include DNS settings and account information. File systems we overlay into your images are your home directory, scratch, Data Depot, and application file systems.
Here is a list of paths:
- /etc/resolv.conf
- /etc/hosts
- /home/$USER
- /apps
- /scratch
- /depot
This means that within the container environment these paths will be present and the same as outside the container. The /apps
, /scratch
, and /depot
directories will need to exist inside your container to work properly.
Link to section 'Creating Apptainer Images' of 'Apptainer' Creating Apptainer Images
You can build on your system or straight on the cluster (you do not need root privileges to build or run the container).
You can find information and documentation for how to install and use Apptainer on your system:
We have version 1.1.6
(or newer) on the cluster. Please note that installed versions may change throughout cluster life time, so when in doubt, please check exact version with a --version
command line flag:
apptainer --version
apptainer version 1.1.6-1
Everything you need on how to build a container is available from their user guide. Below are merely some quick tips for getting your own containers built for Gilbreth.
You can use a Definition File to both build your container and share its specification with collaborators (for the sake of reproducibility). Here is a simplistic example of such a file:
# FILENAME: Buildfile
Bootstrap: docker
From: ubuntu:18.04
%post
apt-get update && apt-get upgrade -y
mkdir /apps /depot /scratch
To build the image itself:
apptainer build ubuntu-18.04.sif Buildfile
The challenge with this approach however is that it must start from scratch if you decide to change something. In order to create a container image iteratively and interactively, you can use the --sandbox
option.
apptainer build --sandbox ubuntu-18.04 docker://ubuntu:18.04
This will not create a flat image file but a directory tree (i.e., a folder), the contents of which are the container's filesystem. In order to get a shell inside the container that allows you to modify it, user the --writable
option.
apptainer shell --writable ubuntu-18.04
Apptainer>
You can then proceed to install any libraries, software, etc. within the container. Then to create the final image file, exit
the shell and call the build
command once more on the sandbox.
apptainer build ubuntu-18.04.sif ubuntu-18.04
Finally, copy the new image to Gilbreth and run it.
GPU Usage Monitoring
Link to section 'What is it?' of 'GPU Usage Monitoring' What is it?
To ensure that GPUs are effectively utilized on our cluster, we log and store the power, memory, and utilization of every GPU on our cluster every 10 seconds. RCAC uses this data to identify users that request large amounts of GPU hours but do not actually use them.
To assist users in optimizing their usage of requested GPU resources, we have created a GPU usage monitor tool that allows users to track their GPU utilization for jobs that they have submitted. The purpose of this tool is to provide users an interface to identify jobs or GPUs that they have requested but are not being effectively utilized.
Link to section 'How to use' of 'GPU Usage Monitoring' How to use
Our GPU Usage monitor is currently implemented on Gautschi
and Gilbreth
, and is available at the following location:
/apps/external/gpu_util/get_gpu_util
Link to section 'Seeing Logged Jobs' of 'GPU Usage Monitoring' Seeing Logged Jobs
You can check all the jobs that you've run with the -u
or --user_jobs
flag:
/apps/external/gpu_util/get_gpu_util --user_jobs
This will print a table of all the GPU jobs you have submitted to the cluster, with the job ID, start and end times, and the number of GPUs that that job had allocated to it:
It should be noted that we collect GPU usage information every 3 - 3.5 hours. If you have recently submitted a job, your job may not be available yet.
Link to section 'Inspecting a specific job' of 'GPU Usage Monitoring' Inspecting a specific job
If you want to view the GPU utilization of a specific job, you can use the -j
or --job_id
flag. For example, to see the utilization of job 32083, you may run the following:
/apps/external/gpu_util/get_gpu_util --job_id 32083
This will print a table of the GPU memory and utilization for each GPU that was allocated for the specified job:

Link to section 'Plotting Data' of 'GPU Usage Monitoring' Plotting Data
It is also possible to plot the GPU utilization and memory usage over time with the -p
or the --plot
flag:
/apps/external/gpu_util/get_gpu_util --job_id 32083 --plot
This will print two graphs. The first will be a 2-minute rolling average of the GPU utilization over time, and the second will be the percentage of memory used over time. Each GPU will be colored differently:
Link to section 'Saving GPU Data' of 'GPU Usage Monitoring' Saving GPU Data
If you would like to save the raw GPU utilization and memory usage data for your job, you can use the -s
or --save
flag:
/apps/external/gpu_util/get_gpu_util --job_id 32083 --save
This will download the GPU data for a specific job as:
{job_id}_gpu_usage.csv
Link to section 'Checking Account Usage (Group Managers only)' of 'GPU Usage Monitoring' Checking Account Usage (Group Managers only)
If you are a manager of a specific group, you are able to query all accounts owned by that group. You can check the usage of all members of your account over the previous week by running:
/apps/external/gpu_util/get_gpu_util -A accountname
If you would like a breakdown of the individual jobs ran within the past week, you can add the --records
flag:
/apps/external/gpu_util/get_gpu_util -A accountname --records
Further, the --job {jobid}
flag will also allow you to query the utilization of individual jobs that were ran on an account that you manage.
Link to section 'FAQ/Troubleshooting' of 'GPU Usage Monitoring' FAQ/Troubleshooting
- "Why doesn't my job show up in the list?"
- Although we log GPU utilization every 10 seconds, we only actually collect it every 3-3.5 hours. If you check later, your job should be listed.
- "Can other people see my data?"
- No, our interface only allows users to check the utilization of their own jobs.
- "Is this a good way to see the GPU Hours balance?"
- No, the primary purpose of this tool is to check that GPUs are actually being used, not to track billed GPU hours. To see the total GPU hours balance, please use the
slist
command.
- No, the primary purpose of this tool is to check that GPUs are actually being used, not to track billed GPU hours. To see the total GPU hours balance, please use the
Frequently Asked Questions
Some common questions, errors, and problems are categorized below. Click the Expand Topics link in the upper right to see all entries at once. You can also use the search box above to search the user guide for any issues you are seeing.
About Gilbreth
Frequently asked questions about Gilbreth.
Can you remove me from the Gilbreth mailing list?
Your subscription in the Gilbreth mailing list is tied to your account on Gilbreth. If you are no longer using your account on Gilbreth, your account can be deleted from the My Accounts page. Hover over the resource you wish to remove yourself from and click the red 'X' button. Your account and mailing list subscription will be removed overnight. Be sure to make a copy of any data you wish to keep first.
How is Gilbreth different than other Community Clusters?
Gilbreth differs from the previous Community Clusters in many significant aspects:
- Each Gilbreth compute nodes are equipped with a variety of Nvidia Tesla GPU accelerator cards which can significantly improve performance of compute-intensive workloads.
- Each Gilbreth front-end contains one Nvidia Tesla A30 accelerator card. This makes GPU code development and testing much simpler.
- GPU-enabled applications have both non-gpu and gpu-enabled versions installed. Typically, gpu-enabled versions are tagged with gpu in their module name, e.g., lammps/31Mar17_gpu is the GPU-enabled version of LAMMPS, while lammps/31Mar17 is the non-gpu version of LAMMPS.
- An exception to the above rule is that for licensed softwares like Abaqus, Ansys, and Matlab, a single module contains both non-gpu and gpu-enabled versions.
- A selection of GPU-enabled application containers from the Nvidia GPU Cloud (NGC) collection is installed.
Do I need to do anything to my firewall to access Gilbreth?
No firewall changes are needed to access Gilbreth. However, to access data through Network Drives (i.e., CIFS, "Z: Drive"), you must be on a Purdue campus network or connected through VPN.
Logging In & Accounts
Frequently asked questions about logging in & accounts.
Errors
Common errors and solutions/work-arounds for them.
Account creation failed
An email came into rcac-help from the automated account checker that an account creation failed. There are a few scenarios that can cause this. There are a few things to check.
Link to section 'Account not created' of 'Account creation failed' Account not created
First check what resource they were added to and the corresponding role status from the User Search page.
Take the following steps for these scenarios:
Link to section 'No Role' of 'Account creation failed' No Role
This means either our website failed and didn't add the role (rare, but there is a known bug where when a faculty requests Radon/Hathi for themselves it fails) or IAMO rejected the role.
You can try manually adding the role through the tool and see if it rejects it again, or ask IAMO about the status and if the role can be added (see below).
Link to section 'Role Pending' of 'Account creation failed' Role Pending
This means two things: IAMO's overnight process failed or the account was added just past the cutoff for the overnight process, but before the account check run.
In the former scenario, something went wrong on IAMO's side. Usually Ben is on top of things and gets things sorted quickly when he gets in the morning, but if it's afternoon and it's still not there ask IAMO about it.
For the latter scenario, there is a very narrow window when users can be added and trigger a false alarm (something like ~4-5am). It's rare, but it happens from time to time when we have a night owl/early bird faculty (or traveling abroad).
Link to section 'Role Ready' of 'Account creation failed' Role Ready
The are two scenarios here: IAMO's overnight process failed and has already been fixed or the transd is broken on our end.
In the first scenario, there probably isn't anything to do. You can verify their account with ldapsearch -x uid=USERNAME | grep host
and see if the have the proper host entry. If they do, they should be able to log in.
In the second scenario, the next step would be to investigate the transd. The transd translates packets from IAMO into accounts on our systems. Log into xenon.rcac and look at /var/log/transd_log. Is there recent activity at the end of log? If the end of the log is stale, something is probably stuck, like a full disk or some such. In this case, assign ticket to systems and ask them to look at it. If it has recent activity, you should be able to grep the log for the username and look for account entries for them. If the transd is running further investigation is probably needed.
Link to section 'Asking IAMO' of 'Account creation failed' Asking IAMO
The Footprints queue for IAMO is ITAP_IDENTITY_MANAGEMENT. Ben Lewis and Scott Morris are familiar with our web app, and should be familiar with seeing this "account failed" emails. If they come back and say the account is expired/graduated/etc contact the faculty separately with this information (see below). Otherwise Ben should be able to push accounts or unjam the logjam.
Link to section 'Login Shell /opt/acmaint-3.10/etc/disable is invalid.' of 'Account creation failed' Login Shell /opt/acmaint-3.10/etc/disable is invalid.
This means the user account is no longer valid, ie, they graduated. Remove the account from the Manage User page, and inform the faculty separately (don't use the FP ticket) that added them that we were unable to create an account for the user. Good to verify with PI about student's graudation status (usually that'll ring some bells with the faculty). They will need to have an Request for Privileges (R4P) filed, and then they can re-add the account once complete. If the faculty thinks the student should be valid, ask IAMO about the status. They may have been very recently added back, or had some other issue.
/usr/bin/xauth: error in locking authority file
Link to section 'Problem' of '/usr/bin/xauth: error in locking authority file' Problem
I receive this message when logging in:
/usr/bin/xauth: error in locking authority file
Link to section 'Solution' of '/usr/bin/xauth: error in locking authority file' Solution
Your home directory disk quota is full. You may check your quota with myquota
.
You will need to free up space in your home directory.
ncdu
command is a convenient interactive tool to examine disk usage. Consider running ncdu $HOME
to analyze where the bulk of the usage is. With this knowledge, you could then archive your data elsewhere (e.g. your research group's Data Depot space, or Fortress tape archive), or delete files you no longer need.
There are several common locations that tend to grow large over time and are merely cached downloads. The following are safe to delete if you see them in the output of ncdu $HOME
:
/home/myusername/.local/share/Trash
/home/myusername/.cache/pip
/home/myusername/.conda/pkgs
/home/myusername/.singularity/cache
My SSH connection hangs
Link to section 'Problem' of 'My SSH connection hangs' Problem
Your console hangs while trying to connect to a RCAC Server.
Link to section 'Solution' of 'My SSH connection hangs' Solution
This can happen due to various reasons. Most common reasons for hanging SSH terminals are:
- Network: If you are connected over wifi, make sure that your Internet connection is fine.
- Busy front-end server: When you connect to a cluster, you SSH to one of the front-end login nodes. Due to transient user loads, one or more of the front-ends may become unresponsive for a short while. To avoid this, try reconnecting to the cluster or wait until the login node you have connected to has reduced load.
- File system issue: If a server has issues with one or more of the file systems (
home
,scratch
, ordepot
) it may freeze your terminal. To avoid this you can connect to another front-end.
If neither of the suggestions above work, please contact support specifying the name of the server where your console is hung.
Thinlinc session frozen
Link to section 'Problem' of 'Thinlinc session frozen' Problem
Your Thinlinc session is frozen and you can not launch any commands or close the session.
Link to section 'Solution' of 'Thinlinc session frozen' Solution
This can happen due to various reasons. The most common reason is that you ran something memory-intensive inside that Thinlinc session on a front-end, so parts of the Thinlinc session got killed by Cgroups, and the entire session got stuck.
- If you are using a web-version Thinlinc remote desktop (inside the browser):
The web version does not have the capability to kill the existing session, only the standalone client does. Please install the standalone client and follow the steps below:
- If you are using a Thinlinc client:
Close the ThinLinc client, reopen the client login popup, and select
End existing session
.Select "End existing session" and try "Connect" again.
Thinlinc session unreachable
Link to section 'Problem' of 'Thinlinc session unreachable' Problem
When trying to login to Thinlinc and re-connect to your existing session, you receive an error "Your Thinlinc session is currently unreachable".
Link to section 'Solution' of 'Thinlinc session unreachable' Solution
This can happen if the specific login node your existing remote desktop session was residing on is currently offline or down, so Thinlinc can not reconnect to your existing session. Most often the session is non-recoverable at this point, so the solution is to terminate your existing Thinlinc desktop session and start a new one.
- If you are using a web-version Thinlinc remote desktop (inside the browser):
The web version does not have the capability to kill the existing session, only the standalone client does. Please install the standalone client and follow the steps below:
- If you are using a Thinlinc client:
Close the ThinLinc client, reopen the client login popup, and select
End existing session
.Select "End existing session" and try "Connect" again.
How to disable Thinlinc screensaver
Link to section 'Problem' of 'How to disable Thinlinc screensaver' Problem
Your ThinLinc desktop is locked after being idle for a while, and it asks for a password to refresh it. It means the "screensaver" and "lock screen" functions are turned on, but you want to disable these functions.
Link to section 'Solution' of 'How to disable Thinlinc screensaver' Solution
If your screen is locked, close the ThinLinc client, reopen the client login popup, and select End existing session
.
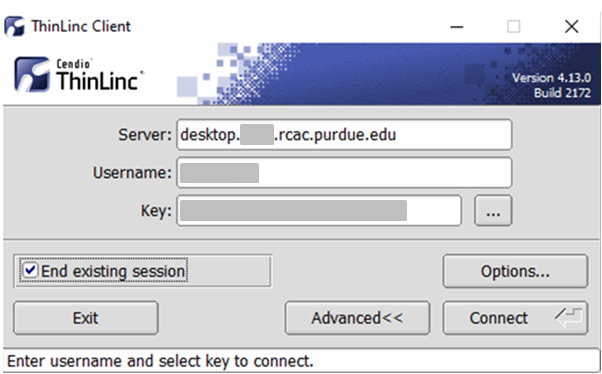
To permanently avoid screen lock issue, right click desktop and select Applications
, then settings
, and select Screensaver
.
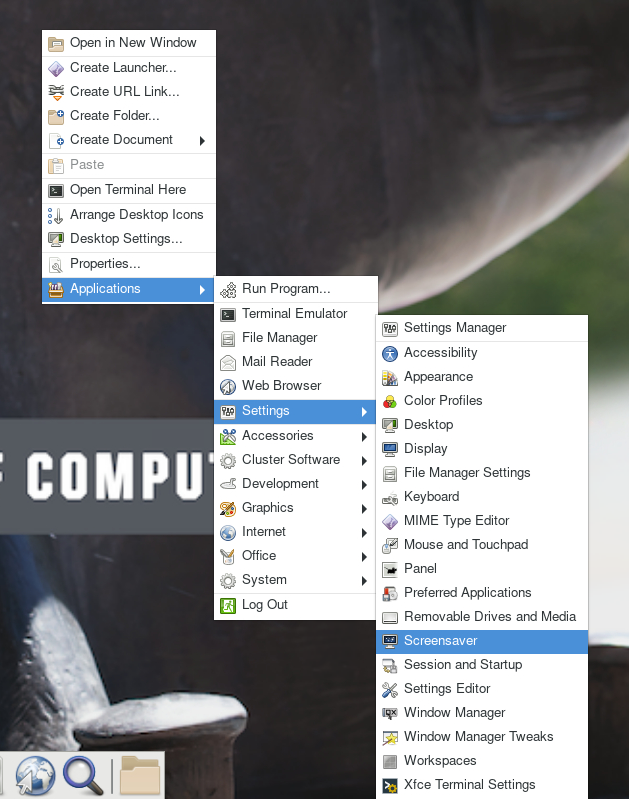
Under Screensaver, turn off the Enable Screensaver
, then under Lock Screen, turn off the Enable Lock Screen
, and close the window.
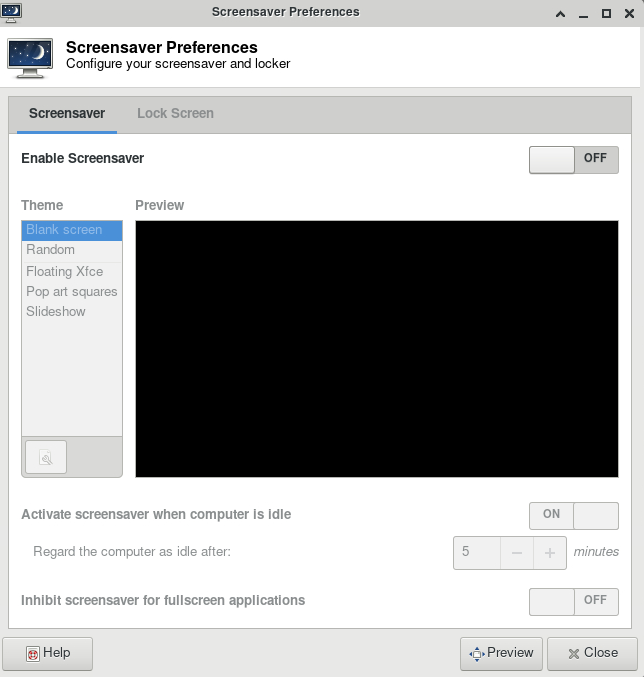
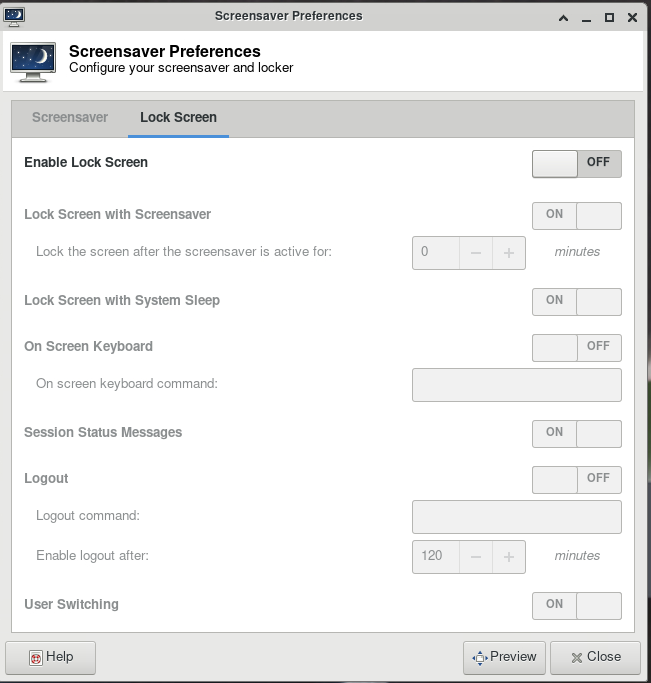
Questions
Frequently asked questions about logging in & accounts.
I worked on Gilbreth after I graduated/left Purdue, but can not access it anymore
Link to section 'Problem' of 'I worked on Gilbreth after I graduated/left Purdue, but can not access it anymore' Problem
You have graduated or left Purdue but continue collaboration with your Purdue colleagues. You find that your access to Purdue resources has suddenly stopped and your password is no longer accepted.
Link to section 'Solution' of 'I worked on Gilbreth after I graduated/left Purdue, but can not access it anymore' Solution
Access to all resources depends on having a valid Purdue Career Account. Expired Career Accounts are removed twice a year, during Spring and October breaks (more details at the official page). If your Career Account was purged due to expiration, you will not be be able to access the resources.
To provide remote collaborators with valid Purdue credentials, the University provides a special procedure called Request for Privileges (R4P). If you need to continue your collaboration with your Purdue PI, the PI will have to submit or renew an R4P request on your behalf.
After your R4P is completed and Career Account is restored, please note two additional necessary steps:
-
Access: Restored Career Accounts by default do not have any RCAC resources enabled for them. Your PI will have to login to the Manage Users tool and explicitly re-enable your access by un-checking and then ticking back checkboxes for desired queues/Unix groups resources.
-
Email: Restored Career Accounts by default do not have their @purdue.edu email service enabled. While this does not preclude you from using RCAC resources, any email messages (be that generated on the clusters, or any service announcements) would not be delivered - which may cause inconvenience or loss of compute jobs. To avoid this, we recommend setting your restored @purdue.edu email service to "Forward" (to an actual address you read). The easiest way to ensure it is to go through the Account Setup process.
Can I manage my Login Activity in Box?
In Box under your account settings, click the "Security" tab. You can review and remove sessions.
Jobs
Frequently asked questions related to running jobs.
Errors
Common errors and potential solutions/workarounds for them.
cannot connect to X server / cannot open display
Link to section 'Problem' of 'cannot connect to X server / cannot open display' Problem
You receive the following message after entering a command to bring up a graphical window
cannot connect to X server
cannot open display
Link to section 'Solution' of 'cannot connect to X server / cannot open display' Solution
This can happen due to multiple reasons:
- Reason: Your SSH client software does not support graphical display by itself (e.g. SecureCRT or PuTTY).
- Solution: Try using a client software like Thinlinc or MobaXterm as described in the SSH X11 Forwarding guide.
-
Reason: You did not enable X11 forwarding in your SSH connection.
-
Solution: If you are in a Windows environment, make sure that X11 forwarding is enabled in your connection settings (e.g. in MobaXterm or PuTTY). If you are in a Linux environment, try
ssh -Y -l username hostname
-
- Reason: If you are trying to open a graphical window within an interactive PBS job, make sure you are using the
-X
option withqsub
after following the previous step(s) for connecting to the front-end. Please see the example in the Interactive Jobs guide. - Reason: If none of the above apply, make sure that you are within quota of your home directory.
bash: command not found
Link to section 'Problem' of 'bash: command not found' Problem
You receive the following message after typing a command
bash: command not found
Link to section 'Solution' of 'bash: command not found' Solution
This means the system doesn't know how to find your command. Typically, you need to load a module to do it.
bash: module command not found
Link to section 'Problem' of 'bash: module command not found' Problem
You receive the following message after typing a command, e.g. module load intel
bash: module command not found
Link to section 'Solution' of 'bash: module command not found' Solution
The system cannot find the module command. You need to source the modules.sh file as below
source /etc/profile.d/modules.sh
or
#!/bin/bash -i
Close Firefox / Firefox is already running but not responding
Link to section 'Problem' of 'Close Firefox / Firefox is already running but not responding' Problem
You receive the following message after trying to launch Firefox browser inside your graphics desktop:
Close Firefox
Firefox is already running, but not responding. To open a new window,
you must first close the existing Firefox process, or restart your system.
Link to section 'Solution' of 'Close Firefox / Firefox is already running but not responding' Solution
When Firefox runs, it creates several lock files in the Firefox profile directory (inside ~/.mozilla/firefox/
folder in your home directory). If a newly-started Firefox instance detects the presence of these lock files, it complains.
This error can happen due to multiple reasons:
- Reason: You had a single Firefox process running, but it terminated abruptly without a chance to clean its lock files (e.g. the job got terminated, session ended, node crashed or rebooted, etc).
- Solution: If you are certain you do not have any other Firefox processes running elsewhere, please use the following command in a terminal window to detect and remove the lock files:
$ unlock-firefox
- Solution: If you are certain you do not have any other Firefox processes running elsewhere, please use the following command in a terminal window to detect and remove the lock files:
- Reason: You may indeed have another Firefox process (in another Thinlinc or Gateway session on this or other cluster, another front-end or compute node). With many clusters sharing common home directory, a running Firefox instance on one can affect another.
- Solution: Try finding and closing running Firefox process(es) on other nodes and clusters.
- Solution: If you must have multiple Firefoxes running simultaneously, you may be able to create separate Firefox profiles and select which one to use for each instance.
Jupyter: database is locked / can not load notebook format
Link to section 'Problem' of 'Jupyter: database is locked / can not load notebook format' Problem
You receive the following message after trying to load existing Jupyter notebooks inside your JupyterHub session:
Error loading notebook
An unknown error occurred while loading this notebook. This version can load notebook formats or earlier. See the server log for details.
Alternatively, the notebook may open but present an error when creating or saving a notebook:
Autosave Failed!
Unexpected error while saving file: MyNotebookName.ipynb database is locked
Link to section 'Solution' of 'Jupyter: database is locked / can not load notebook format' Solution
When Jupyter notebooks are opened, the server keeps track of their state in an internal database (located inside ~/.local/share/jupyter/
folder in your home directory). If a Jupyter process gets terminated abruptly (e.g. due to an out-of-memory error or a host reboot), the database lock is not cleared properly, and future instances of Jupyter detect the lock and complain.
Please follow these steps to resolve:
- Fully exit from your existing Jupyter session (close all notebooks, terminate Jupyter, log out from JupyterHub or JupyterLab, terminate OnDemand gateway's Jupyter app, etc).
- In a terminal window (SSH, Thinlinc or OnDemand gateway's terminal app) use the following command to clean up stale database locks:
$ unlock-jupyter
- Start a new Jupyter session as usual.
Questions
Frequently asked questions about jobs.
How do I know Non-uniform Memory Access (NUMA) layout on Gilbreth?
- You can learn about processor layout on Gilbreth nodes using the following command:
gilbreth-a003:~$ lstopo-no-graphics
- For detailed IO connectivity:
gilbreth-a003:~$ lstopo-no-graphics --physical --whole-io
- Please note that NUMA information is useful for advanced MPI/OpenMP/GPU optimizations. For most users, using default NUMA settings in MPI or OpenMP would give you the best performance.
Why cannot I use --mem=0 when submitting jobs?
Link to section 'Question' of 'Why cannot I use --mem=0 when submitting jobs?' Question
Why can't I specify --mem=0
for my job?
Link to section 'Answer' of 'Why cannot I use --mem=0 when submitting jobs?' Answer
We no longer support requesting unlimited memory (--mem=0
) as it has an adverse effect on the way scheduler allocates job, and could lead to large amount of nodes being blocked from usage.
Most often we suggest relying on default memory allocation (cluster-specific). But if you have to request custom amounts of memory, you can do it explicitly. For example --mem=20G
.
If you want to use the entire node's memory, you can submit the job with the --exclusive
option.
Can I extend the walltime on a job?
In some circumstances, yes. Walltime extensions must be requested of and completed by staff. Walltime extension requests will be considered on named (your advisor or research lab) queues. Standby or debug queue jobs cannot be extended.
Extension requests are at the discretion of staff based on factors such as any upcoming maintenance or resource availability. Extensions can be made past the normal maximum walltime on named queues but these jobs are subject to early termination should a conflicting maintenance downtime be scheduled.
Please be mindful of time remaining on your job when making requests and make requests at least 24 hours before the end of your job AND during business hours. We cannot guarantee jobs will be extended in time with less than 24 hours notice, after-hours, during weekends, or on a holiday.
We ask that you make accurate walltime requests during job submissions. Accurate walltimes will allow the job scheduler to efficiently and quickly schedule jobs on the cluster. Please consider that extensions can impact scheduling efficiency for all users of the cluster.
Requests can be made by contacting support. We ask that you:
- Provide numerical job IDs, cluster name, and your desired extension amount.
- Provide at least 24 hours notice before job will end (more if request is made on a weekend or holiday).
- Consider making requests during business hours. We may not be able to respond in time to requests made after-hours, on a weekend, or on a holiday.
Data
Frequently asked questions about data and data management.
How is my Data Secured on Gilbreth?
Gilbreth is operated in line with policies, standards, and best practices as described within Secure Purdue, and specific to RCAC Resources.
Security controls for Gilbreth are based on ones defined in NIST cybersecurity standards.
Gilbreth supports research at the L1 fundamental and L2 sensitive levels. Gilbreth is not approved for storing data at the L3 restricted (covered by HIPAA) or L4 Export Controlled (ITAR), or any Controlled Unclassified Information (CUI).
For resources designed to support research with heightened security requirements, please look for resources within the REED+ Ecosystem.
Link to section 'For additional information' of 'How is my Data Secured on Gilbreth?' For additional information
Log in with your Purdue Career Account.
Can I share data with outside collaborators?
Yes! Globus allows convenient sharing of data with outside collaborators. Data can be shared with collaborators' personal computers or directly with many other computing resources at other institutions. See the Globus documentation on how to share data:
Can I access Fortress from Gilbreth?
Yes. While Fortress directories are not directly mounted on Gilbreth for performance and archival protection reasons, they can be accessed from Gilbreth front-ends and nodes using any of the recommended methods of HSI, HTAR or Globus.
Software
Frequently asked questions about software.
Cannot use pip after loading ml-toolkit modules
Link to section 'Question' of 'Cannot use pip after loading ml-toolkit modules' Question
Pip throws an error after loading the machine learning modules. How can I fix it?
Link to section 'Answer' of 'Cannot use pip after loading ml-toolkit modules' Answer
Machine learning modules (tensorflow, pytorch, opencv etc.) include a version of pip that is newer than the one installed with Anaconda. As a result it will throw an error when you try to use it.
$ pip --version
Traceback (most recent call last):
File "/apps/cent7/anaconda/5.1.0-py36/bin/pip", line 7, in <module>
from pip import main
ImportError: cannot import name 'main'
The preferred way to use pip with the machine learning modules is to invoke it via Python as shown below.
$ python -m pip --version
How can I get access to Sentaurus software?
Link to section 'Question' of 'How can I get access to Sentaurus software?' Question
How can I get access to Sentaurus tools for micro- and nano-electronics design?
Link to section 'Answer' of 'How can I get access to Sentaurus software?' Answer
Sentaurus software license requires a signed NDA. Please contact Dr. Mark Johnson, Director of ECE Instructional Laboratories to complete the process.
Once the licensing process is complete and you have been added into a cae2
Unix group, you could use Sentaurus on RCAC community clusters by loading the corresponding environment module:
module load sentaurus
Julia package installation
Users do not have write permission to the default julia package installation destination. However, users can install packages into home directory under ~/.julia
.
Users can side step this by explicitly defining where to put julia packages:
$ export JULIA_DEPOT_PATH=$HOME/.julia
$ julia -e 'using Pkg; Pkg.add("PackageName")'
About Research Computing
Frequently asked questions about RCAC.
Can I get a private server from RCAC?
Link to section 'Question' of 'Can I get a private server from RCAC?' Question
Can I get a private (virtual or physical) server from RCAC?
Link to section 'Answer' of 'Can I get a private server from RCAC?' Answer
Often, researchers may want a private server to run databases, web servers, or other software. RCAC currently has Geddes, a Community Composable Platform optimized for composable, cloud-like workflows that are complementary to the batch applications run on Community Clusters. Funded by the National Science Foundation under grant OAC-2018926, Geddes consists of Dell Compute nodes with two 64-core AMD Epyc 'Rome' processors (128 cores per node).
To purchase access to Geddes today, go to the Cluster Access Purchase page. Please subscribe to our Community Cluster Program Mailing List to stay informed on the latest purchasing developments or contact us (rcac-cluster-purchase@lists.purdue.edu) if you have any questions.
Biography of Lillian Moller Gilbreth
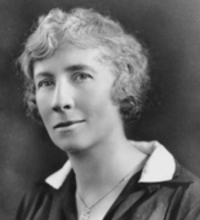
Lillian Moller Gilbreth was an industrial engineer and efficiency expert who became Purdue’s first female engineering professor when she joined the faculty in 1935.
Professor Gilbreth’s research focused on combining psychology and engineering to improve efficiency in the workplace and home, and she pioneered the field now known as ergonomics. To improve household efficiency, she invented a number of kitchen devices, including the foot pedal trash can, refrigerator door shelves and the electric mixer.
Among many other honors, she was the first woman elected to the National Academy of Engineering (1965), the second female member of the American Society of Mechanical Engineers (1926) and the first woman to receive the Hoover Medal (1966). In 2001, the National Academy of Engineering established the Gilbreth Lectures in her honor as a means of recognizing outstanding young American engineers. She received more than 20 honorary degrees.
Professor Gilbreth’s family life with her husband and research collaborator Frank and their 12 children is the subject of the autobiographical novels “Cheaper by the Dozen” and “Belles on Their Toes,” which were written by two of their children and describe how the Gilbreths applied their efficiency studies in their home. The novels were made into popular films starring Myrna Loy.
Professor Gilbreth was born in Oakland, California and earned her bachelor’s degree in English literature from the University of California-Berkeley in 1900. She began studying for a master’s degree at Columbia University, but an illness forced her to return home and she earned her master’s degree in literature from Berkeley in 1902. When she received a doctorate in applied psychology from Brown University in 1915, she became the first mother to receive a doctorate from the university.
With her husband Frank, Professor Gilbreth developed a new way of performing time and motion studies, which break tasks into steps to evaluate the efficiency of workplace processes. The Gilbreths used a video camera to record work processes, and studying the films allowed them to better design equipment to improve efficiency and reduce workers’ fatigue, a concept that eventually developed into the field of ergonomics.
After Frank Gilbreth’s death in 1924, Professor Gilbreth succeeded him as a visiting lecturer at Purdue. In 1935, she became a professor of management in Purdue’s School of Mechanical Engineering. She was the first female engineering professor at Purdue and, by some accounts, the first female engineering professor in the country. She was promoted to full professor in 1940 and remained at Purdue until her retirement in 1948.
Purdue Libraries’ Archives and Special Collections is home to the books, working papers and family archives of Lillian and Frank Gilbreth. Researchers from around the world visit every year to study the Gilbreths’ papers.
Datasets
Please refer to our Federated Datasets Documentation website for up-to-date datasets on Anvil and instructions on how to use them.
Weber User Guide
Weber is Purdue's new specialty high performance computing cluster for data, applications, and research which are covered by export control regulations such as EAR, ITAR, or requiring compliance with the NIST SP 800-171.
Note: Weber user guide requires login to access.
Link to section 'Overview of Weber' of 'Overview of Weber' Overview of Weber
Weber is Purdue's specialty high performance computing cluster deployed in 2019 for data, applications, and research under export control regulations such as EAR, ITAR, or requiring compliance with the NIST SP 800-171.
For purchase access questions, please contact the Export Controls office at exportcontrols@purdue.edu
For technical questions, please contact RCAC at rcac-help@purdue.edu
Link to section 'Weber Namesake' of 'Overview of Weber' Weber Namesake
Weber is named in honor of Mary Ellen Weber, scientist and former astronaut. More information about her life and impact on Purdue is available in a Biography of Weber.
Link to section 'Weber Specifications' of 'Overview of Weber' Weber Specifications
Weber consists of Dell compute nodes with two 64-core AMD EPYC 7713 processors, and Dell GPU nodes with two 8-core Intel Xeon 4110 processors and a Tesla V100 GPU. All nodes have 56 Gbps EDR Infiniband Interconnect.
All Weber nodes have 20 processor cores, 64 GB of RAM, and 56 Gbps Infiniband interconnects.
Front-Ends | Number of Nodes | Processors per Node | Cores per Node | Memory per Node | Retires in |
---|---|---|---|---|---|
2 | Two AMD EPYC 7702P @ 2.00GHz | 128 | 256 GB | 2024 |
Sub-Cluster | Number of Nodes | Processors per Node | Cores per Node | Memory per Node | Retires in |
---|---|---|---|---|---|
A | 15 | Two AMD EPYC 7713 @ 2.00GHz | 128 | 256 GB | 2027 |
G | 2 | Two Intel Xeon 4110 @ 2.10GHz | 16 | 196 GB | 2024 |
Weber nodes run CentOS 7 and use SLURM as the batch system for resource and job management. The application of operating system patches occurs as security needs dictate. All nodes allow for unlimited stack usage, as well as unlimited core dump size (though disk space and server quotas may still be a limiting factor).
On Weber, the following set of compiler, math library, and message-passing library for parallel code are recommended:
- Intel
- MKL
- Intel MPI
This compiler and these libraries are loaded by default. To load the recommended set again:
$ module load rcac
To verify what you loaded:
$ module list
Biography of Mary Ellen Weber
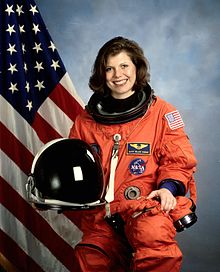
Mary Ellen Weber is a Purdue alumna, astronaut, chemist, business executive and speaker.
Dr. Weber grew up in Ohio and earned her bachelor's degree in chemical engineering with honors from Purdue in 1984. She went on to earn a doctorate in physical chemistry from the University of California-Berkeley in 1988 and a master of business administration degree from Southern Methodist University in 2002.
Dr. Weber was selected by NASA to become an astronaut in 1992. She served on two space shuttle missions, STS-70 Discovery in 1995 and STS-101 Atlantis in 2000, traveling a total of 297 earth orbits and 7.8 million miles. On the Discovery mission, Dr. Weber successfully deployed a $200 million NASA communications satellite to its orbit 22,000 miles above Earth and performed biotechnology research related to colon cancer.
On the Atlantis mission, which was the third shuttle mission devoted to the construction of the International Space Station, Dr. Weber operated the shuttle's 60-foot robotic arm to maneuver spacewalking crewmembers along the Station's surface and directed the transfer of more than three thousand pounds of equipment.
In addition to her work in the Astronaut Corps, Dr. Weber held a variety of other positions within NASA, including working as the Legislative Affairs liaison at NASA headquarters in Washington, D.C. She is the recipient of the NASA Exceptional Service Medal.
After leaving NASA, Dr. Weber was the Vice President for Government Affairs and Policy for nine years at the University of Texas Southwestern Medical Center in Dallas, Texas. She is the founder of Stellar Strategies, LLC, consulting in strategic communications, technology innovation and high-risk operations. She has over 20 years of experience as a speaker and has been a keynote speaker at many conferences and a frequent TV news guest.
Dr. Weber is an active competitive skydiver, who has logged nearly 6,000 skydives and won two dozen medals at the U.S. National Skydiving Championships.
Globus HA Consent
WARNING: UNAUTHORIZED ACCESS TO THIS SYSTEM IS PROHIBITED. This Information System (IS) contains the property of Purdue University and is for authorized use only. This IS may contain federal contract information (FCI) and controlled unclassified information (CUI). By using this IS (which includes any device attached to this IS), you consent to the following conditions:
- Purdue University routinely intercepts and monitors communications on this IS.
- At any time, Purdue University may inspect and seize data stored on this IS.
- Communications using, or data stored on, this IS are not private, are subject to routine monitoring, interception, and search, and may be disclosed or used for any Purdue University authorized purpose.
- This IS includes security measures (e.g., authentication and access controls) to protect Purdue University interests, not for your personal benefit or privacy.
- Unauthorized use of this IS is subject to criminal and civil penalties.
Scholar User Guide
Scholar is a small computer cluster, suitable for classroom learning about high performance computing (HPC).
Link to section 'Overview of Scholar' of 'Overview of Scholar' Overview of Scholar
Scholar is a small computer cluster, suitable for classroom learning about high performance computing (HPC). It consists of 6 interactive login servers and 16 batch worker nodes.
It can be accessed as a typical cluster, with a job scheduler distributing batch jobs onto its worker nodes, or as an interactive resource, with software packages available through a desktop-like environment on its login servers.
If you have a class that you think will benefit from the use of Scholar, you can schedule it for your class through our Class Account Request page. You only need to register your class itself. All students who register for the class will automatically get login privileges to the Scholar cluster.
As a batch resource, the cluster has access to typical HPC software packages and tool chains; as an interactive resource, Scholar provides a Linux remote desktop, or a Jupyter notebook server, or an R Studio server. Jupyter and R Studio can be used by students without any reliance on Linux knowledge or experience.
Link to section 'Scholar Specifications' of 'Overview of Scholar' Scholar Specifications
Front-Ends | Number of Nodes | Processors per Node | Cores per Node | Memory per Node | Retires in |
---|---|---|---|---|---|
No GPU | 3 | Two AMD EPYC 9634 ("Genoa") 84-Core Processors | 168 | 384 GB | 2029 |
With GPU | 3 | Two Intel Xeon Gold 6126 ("Skylake") 12-Core Processors with one NVIDIA Tesla V100 32GB GPU | 24 | 768 GB | 2027 |
Sub-Cluster | Number of Nodes | Processors per Node | Cores per Node | Memory per Node | Retires in |
---|---|---|---|---|---|
A | 4 | Two AMD EPYC 7713 ("Milan") 64-Core Processors | 128 | 256 GB | 2027 |
B | 3 | One AMD EPYC 7702P ("Rome") 64-Core Processor | 64 | 256 GB | 2026 |
G | 4 | Two Intel Xeon Silver 4110 ("Skylake") 8-Core Processors with one NVIDIA Tesla V100 16GB GPU | 16 | 192 GB | 2027 |
H | 2 | Two AMD EPYC 7543 3rd generation ("Milan") 32-Core Processors with two NVIDIA A30 24GB GPUs | 64 | 512 GB | 2027 |
H-MIG | 2 | Two AMD EPYC 7543 3rd generation ("Milan") 32-Core Processors with eight 6GB Multi-Instance GPUs (MIGs) configured from two NVIDIA A30 24GB GPUs. | 64 | 512 GB | 2027 |
I-MIG | 1 | Two AMD EPYC 9554 ("Genoa") 64-Core Processors with four 6GB Multi-Instance GPUs (MIGs) configured from one NVIDIA A30 24GB GPU | 128 | 384 GB | 2029 |
J | 4 | Two Intel Xeon Gold 6126 ("Skylake") 12-Core Processors with two NVIDIA A40 48GB GPUs | 24 | 192 GB | 2029 |
Faculty who would like to know more about Scholar, please read the Faculty Guide
Link to section 'Software catalog' of 'Overview of Scholar' Software catalog
Link to section 'Accounts on Scholar' of 'Accounts' Accounts on Scholar
Link to section 'Obtaining an Account' of 'Accounts' Obtaining an Account
All Purdue faculty may request access to Scholar for use in the classroom. Please use the Accounts for Classes tool to create accounts for your class. You will need to select the semester and CRN of the class. All students registered in that class will be added once the request is fulfilled. You may add additional instructors or TAs from the same tool.
Link to section 'Outside Collaborators' of 'Accounts' Outside Collaborators
A valid Purdue Career Account is required for access to any resource. If you do not currently have a valid Purdue Career Account you must have a current Purdue faculty or staff member file a Request for Privileges (R4P) before you can proceed.
Logging In
To submit jobs on Scholar, log in to the submission host scholar.rcac.purdue.edu
via SSH. This submission host is actually 7 front-end hosts: scholar-fe00
through scholar-fe06
The login process randomly assigns one of these front-ends to each login to scholar.rcac.purdue.edu
.
To submit jobs on Scholar front ends with local GPUs, log in to gpu.scholar.rcac.purdue.edu
via SSH.
Purdue Login
Link to section 'SSH' of 'Purdue Login' SSH
- SSH to the cluster as usual.
- When asked for a password, type your password followed by "
,push
". - Your Purdue Duo client will receive a notification to approve the login.
Link to section 'Thinlinc' of 'Purdue Login' Thinlinc
- When asked for a password, type your password followed by "
,push
". - Your Purdue Duo client will receive a notification to approve the login.
- The native Thinlinc client will prompt for Duo approval twice due to the way Thinlinc works.
- The native Thinlinc client also supports key-based authentication.
Passwords
Scholar supports either Purdue two-factor authentication (Purdue Login) or SSH keys.
SSH Client Software
Secure Shell or SSH is a way of establishing a secure connection between two computers. It uses public-key cryptography to authenticate the user with the remote computer and to establish a secure connection. Its usual function involves logging in to a remote machine and executing commands. There are many SSH clients available for all operating systems:
Linux / Solaris / AIX / HP-UX / Unix:
- The
ssh
command is pre-installed. Log in usingssh myusername@scholar.rcac.purdue.edu
from a terminal.
Microsoft Windows:
- MobaXterm is a small, easy to use, full-featured SSH client. It includes X11 support for remote displays, SFTP capabilities, and limited SSH authentication forwarding for keys.
Mac OS X:
- The
ssh
command is pre-installed. You may start a local terminal window from "Applications->Utilities". Log in by typing the commandssh myusername@scholar.rcac.purdue.edu
.
When prompted for password, enter your Purdue career account password followed by ",push
". Your Purdue Duo client will then receive a notification to approve the login.
SSH Keys
Link to section 'General overview' of 'SSH Keys' General overview
To connect to Scholar using SSH keys, you must follow three high-level steps:
- Generate a key pair consisting of a private and a public key on your local machine.
- Copy the public key to the cluster and append it to
$HOME/.ssh/authorized_keys
file in your account. - Test if you can ssh from your local computer to the cluster without using your Purdue password.
Detailed steps for different operating systems and specific SSH client softwares are give below.
Link to section 'Mac and Linux:' of 'SSH Keys' Mac and Linux:
-
Run
ssh-keygen
in a terminal on your local machine. You may supply a filename and a passphrase for protecting your private key, but it is not mandatory. To accept the default settings, press Enter without specifying a filename.
Note: If you do not protect your private key with a passphrase, anyone with access to your computer could SSH to your account on Scholar. -
By default, the key files will be stored in
~/.ssh/id_rsa
and~/.ssh/id_rsa.pub
on your local machine. -
Copy the contents of the public key into
$HOME/.ssh/authorized_keys
on the cluster with the following command. When asked for a password, type your password followed by ",push
". Your Purdue Duo client will receive a notification to approve the login.ssh-copy-id -i ~/.ssh/id_rsa.pub myusername@scholar.rcac.purdue.edu
Note: use your actual Purdue account user name.
If your system does not have the
ssh-copy-id
command, use this instead:cat ~/.ssh/id_rsa.pub | ssh myusername@scholar.rcac.purdue.edu "mkdir -p ~/.ssh && chmod 700 ~/.ssh && cat >> ~/.ssh/authorized_keys"
-
Test the new key by SSH-ing to the server. The login should now complete without asking for a password.
-
If the private key has a non-default name or location, you need to specify the key by
ssh -i my_private_key_name myusername@scholar.rcac.purdue.edu
Link to section 'Windows:' of 'SSH Keys' Windows:
Programs | Instructions |
---|---|
MobaXterm | Open a local terminal and follow Linux steps |
Git Bash | Follow Linux steps |
Windows 10 PowerShell | Follow Linux steps |
Windows 10 Subsystem for Linux | Follow Linux steps |
PuTTY | Follow steps below |
PuTTY:
-
Launch PuTTYgen, keep the default key type (RSA) and length (2048-bits) and click Generate button.
The "Generate" button can be found under the "Actions" section of the PuTTY Key Generator interface. -
Once the key pair is generated:
Use the Save public key button to save the public key, e.g.
Documents\SSH_Keys\mylaptop_public_key.pub
Use the Save private key button to save the private key, e.g.
Documents\SSH_Keys\mylaptop_private_key.ppk
. When saving the private key, you can also choose a reminder comment, as well as an optional passphrase to protect your key, as shown in the image below. Note: If you do not protect your private key with a passphrase, anyone with access to your computer could SSH to your account on Scholar.The PuTTY Key Generator form has inputs for the Key passphrase and optional reminder comment. From the menu of PuTTYgen, use the "Conversion -> Export OpenSSH key" tool to convert the private key into openssh format, e.g.
Documents\SSH_Keys\mylaptop_private_key.openssh
to be used later for Thinlinc. -
Configure PuTTY to use key-based authentication:
Launch PuTTY and navigate to "Connection -> SSH ->Auth" on the left panel, click Browse button under the "Authentication parameters" section and choose your private key, e.g. mylaptop_private_key.ppk
After clicking Connection -> SSH ->Auth panel, the "Browse" option can be found at the bottom of the resulting panel. Navigate back to "Session" on the left panel. Highlight "Default Settings" and click the "Save" button to ensure the change in place.
-
Connect to the cluster. When asked for a password, type your password followed by "
,push
". Your Purdue Duo client will receive a notification to approve the login. Copy the contents of public key from PuTTYgen as shown below and paste it into$HOME/.ssh/authorized_keys
. Please double-check that your text editor did not wrap or fold the pasted value (it should be one very long line).The "Public key" will look like a long string of random letters and numbers in a text box at the top of the window. - Test by connecting to the cluster. If successful, you will not be prompted for a password or receive a Duo notification. If you protected your private key with a passphrase in step 2, you will instead be prompted to enter your chosen passphrase when connecting.
ThinLinc
RCAC provides Cendio's ThinLinc as an alternative to running an X11 server directly on your computer. It allows you to run graphical applications or graphical interactive jobs directly on Scholar through a persistent remote graphical desktop session.
ThinLinc is a service that allows you to connect to a persistent remote graphical desktop session. This service works very well over a high latency, low bandwidth, or off-campus connection compared to running an X11 server locally. It is also very helpful for Windows users who do not have an easy to use local X11 server, as little to no set up is required on your computer.
There are two ways in which to use ThinLinc: preferably through the native client or through a web browser.
Link to section 'Installing the ThinLinc native client' of 'ThinLinc' Installing the ThinLinc native client
The native ThinLinc client will offer the best experience especially over off-campus connections and is the recommended method for using ThinLinc. It is compatible with Windows, Mac OS X, and Linux.
- Download the ThinLinc client from the ThinLinc website.
- Start the ThinLinc client on your computer.
- In the client's login window, use desktop.scholar.rcac.purdue.edu as the Server. Use your Purdue Career Account username and password, but append "
,push
" to your password. - Click the Connect button.
- Your Purdue Login Duo will receive a notification to approve your login.
- Continue to following section on connecting to Scholar from ThinLinc.
Link to section 'Using ThinLinc through your web browser' of 'ThinLinc' Using ThinLinc through your web browser
The ThinLinc service can be accessed from your web browser as a convenience to installing the native client. This option works with no set up and is a good option for those on computers where you do not have privileges to install software. All that is required is an up-to-date web browser. Older versions of Internet Explorer may not work.
- Open a web browser and navigate to desktop.scholar.rcac.purdue.edu.
- Log in with your Purdue Career Account username and password, but append "
,push
" to your password. - You may safely proceed past any warning messages from your browser.
- Your Purdue Login Duo will receive a notification to approve your login.
- Continue to the following section on connecting to Scholar from ThinLinc.
Link to section 'Connecting to Scholar from ThinLinc' of 'ThinLinc' Connecting to Scholar from ThinLinc
- Once logged in, you will be presented with a remote Linux desktop running directly on a cluster front-end.
- Open the terminal application on the remote desktop.
- Once logged in to the Scholar head node, you may use graphical editors, debuggers, software like Matlab, or run graphical interactive jobs. For example, to test the X forwarding connection issue the following command to launch the graphical editor gedit:
$ gedit
- This session will remain persistent even if you disconnect from the session. Any interactive jobs or applications you left running will continue running even if you are not connected to the session.
Link to section 'Tips for using ThinLinc native client' of 'ThinLinc' Tips for using ThinLinc native client
- To exit a full screen ThinLinc session press the F8 key on your keyboard (fn + F8 key for Mac users) and click to disconnect or exit full screen.
- Full screen mode can be disabled when connecting to a session by clicking the Options button and disabling full screen mode from the Screen tab.
Link to section 'Configure ThinLinc to use SSH Keys' of 'ThinLinc' Configure ThinLinc to use SSH Keys
- The web client does NOT support public-key authentication.
-
ThinLinc native client supports the use of an SSH key pair. For help generating and uploading keys to the cluster, see SSH Keys section in our user guide for details.
To set up SSH key authentication on the ThinLinc client:
-
Open the Options panel, and select Public key as your authentication method on the Security tab.
The "Options..." button in the ThinLinc Client can be found towards the bottom left, above the "Connect" button. -
In the options dialog, switch to the "Security" tab and select the "Public key" radio button:
The "Security" tab found in the options dialog, will be the last of available tabs. The "Public key" option can be found in the "Authentication method" options group. - Click OK to return to the ThinLinc Client login window. You should now see a Key field in place of the Password field.
-
In the Key field, type the path to your locally stored private key or click the ... button to locate and select the key on your local system. Note: If PuTTY is used to generate the SSH Key pairs, please choose the private key in the openssh format.
The ThinLinc Client login window will now display key field instead of a password field.
-
SSH X11 Forwarding
SSH supports tunneling of X11 (X-Windows). If you have an X11 server running on your local machine, you may use X11 applications on remote systems and have their graphical displays appear on your local machine. These X11 connections are tunneled and encrypted automatically by your SSH client.
Link to section 'Installing an X11 Server' of 'SSH X11 Forwarding' Installing an X11 Server
To use X11, you will need to have a local X11 server running on your personal machine. Both free and commercial X11 servers are available for various operating systems.
Linux / Solaris / AIX / HP-UX / Unix:
- An X11 server is at the core of all graphical sessions. If you are logged in to a graphical environment on these operating systems, you are already running an X11 server.
- ThinLinc is an alternative to running an X11 server directly on your Linux computer. ThinLinc is a service that allows you to connect to a persistent remote graphical desktop session.
Microsoft Windows:
- ThinLinc is an alternative to running an X11 server directly on your Windows computer. ThinLinc is a service that allows you to connect to a persistent remote graphical desktop session.
- MobaXterm is a small, easy to use, full-featured SSH client. It includes X11 support for remote displays, SFTP capabilities, and limited SSH authentication forwarding for keys.
Mac OS X:
- X11 is available as an optional install on the Mac OS X install disks prior to 10.7/Lion. Run the installer, select the X11 option, and follow the instructions. For 10.7+ please download XQuartz.
- ThinLinc is an alternative to running an X11 server directly on your Mac computer. ThinLinc is a service that allows you to connect to a persistent remote graphical desktop session.
Link to section 'Enabling X11 Forwarding in your SSH Client' of 'SSH X11 Forwarding' Enabling X11 Forwarding in your SSH Client
Once you are running an X11 server, you will need to enable X11 forwarding/tunneling in your SSH client:
ssh
: X11 tunneling should be enabled by default. To be certain it is enabled, you may usessh -Y
.- MobaXterm: Select "New session" and "SSH." Under "Advanced SSH Settings" check the box for X11 Forwarding.
SSH will set the remote environment variable $DISPLAY
to "localhost:XX.YY"
when this is working correctly. If you had previously set your $DISPLAY
environment variable to your local IP or hostname, you must remove any set
/export
/setenv
of this variable from your login scripts. The environment variable $DISPLAY
must be left as SSH sets it, which is to a random local port address. Setting $DISPLAY
to an IP or hostname will not work.
Purchasing Nodes
RCAC operates a significant shared cluster computing infrastructure developed over several years through focused acquisitions using funds from grants, faculty startup packages, and institutional sources. These "community clusters" are now at the foundation of Purdue's research cyberinfrastructure.
We strongly encourage any Purdue faculty or staff with computational needs to join this growing community and enjoy the enormous benefits this shared infrastructure provides:
- Peace of Mind
RCAC system administrators take care of security patches, attempted hacks, operating system upgrades, and hardware repair so faculty and graduate students can concentrate on research.
- Low Overhead
RCAC data centers provide infrastructure such as networking, racks, floor space, cooling, and power.
- Cost Effective
RCAC works with vendors to obtain the best price for computing resources by pooling funds from different disciplines to leverage greater group purchasing power.
Through the Community Cluster Program, Purdue affiliates have invested several million dollars in computational and storage resources from Q4 2006 to the present with great success in both the research accomplished and the money saved on equipment purchases.
For more information or to purchase access to our latest cluster today, see the Purchase page. Have questions? contact us at rcac-cluster-purchase@lists.purdue.edu to discuss.
File Storage and Transfer
Learn more about file storage transfer for Scholar.
Link to section 'Archive and Compression' of 'Archive and Compression' Archive and Compression
There are several options for archiving and compressing groups of files or directories. The mostly commonly used options are:
Link to section 'tar' of 'Archive and Compression' tar
See the official documentation for tar for more information.
Saves many files together into a single archive file, and restores individual files from the archive. Includes automatic archive compression/decompression options and special features for incremental and full backups.
Examples:
(list contents of archive somefile.tar)
$ tar tvf somefile.tar
(extract contents of somefile.tar)
$ tar xvf somefile.tar
(extract contents of gzipped archive somefile.tar.gz)
$ tar xzvf somefile.tar.gz
(extract contents of bzip2 archive somefile.tar.bz2)
$ tar xjvf somefile.tar.bz2
(archive all ".c" files in current directory into one archive file)
$ tar cvf somefile.tar *.c
(archive and gzip-compress all files in a directory into one archive file)
$ tar czvf somefile.tar.gz somedirectory/
(archive and bzip2-compress all files in a directory into one archive file)
$ tar cjvf somefile.tar.bz2 somedirectory/
Other arguments for tar can be explored by using the man tar command.
Link to section 'gzip' of 'Archive and Compression' gzip
The standard compression system for all GNU software.
Examples:
(compress file somefile - also removes uncompressed file)
$ gzip somefile
(uncompress file somefile.gz - also removes compressed file)
$ gunzip somefile.gz
Link to section 'bzip2' of 'Archive and Compression' bzip2
See the official documentation for bzip for more information.
Strong, lossless data compressor based on the Burrows-Wheeler transform. Stronger compression than gzip.
Examples:
(compress file somefile - also removes uncompressed file)
$ bzip2 somefile
(uncompress file somefile.bz2 - also removes compressed file)
$ bunzip2 somefile.bz2
There are several other, less commonly used, options available as well:
- zip
- 7zip
- xz
Link to section 'Storage Environment Variables' of 'Storage Environment Variables' Storage Environment Variables
Several environment variables are automatically defined for you to help you manage your storage. Use environment variables instead of actual paths whenever possible to avoid problems if the specific paths to any of these change.
Name | Description |
---|---|
HOME | /home/myusername |
PWD | path to your current directory |
RCAC_SCRATCH | /scratch/scholar/myusername |
By convention, environment variable names are all uppercase. You may use them on the command line or in any scripts in place of and in combination with hard-coded values:
$ ls $HOME
...
$ ls $RCAC_SCRATCH/myproject
...
To find the value of any environment variable:
$ echo $RCAC_SCRATCH
/scratch/scholar/myusername
To list the values of all environment variables:
$ env
USER=myusername
HOME=/home/myusername
RCAC_SCRATCH=/scratch/scholar/myusername
...
You may create or overwrite an environment variable. To pass (export) the value of a variable in bash:
$ export MYPROJECT=$RCAC_SCRATCH/myproject
To assign a value to an environment variable in either tcsh or csh:
$ setenv MYPROJECT value
Storage Options
File storage options on RCAC systems include long-term storage (home directories, depot, Fortress) and short-term storage (scratch directories, /tmp directory). Each option has different performance and intended uses, and some options vary from system to system as well. Daily snapshots of home directories are provided for a limited time for accidental deletion recovery. Scratch directories and temporary storage are not backed up and old files are regularly purged from scratch and /tmp directories. More details about each storage option appear below.
Home Directory
Home directories are provided for long-term file storage. Each user has one home directory. You should use your home directory for storing important program files, scripts, input data sets, critical results, and frequently used files. You should store infrequently used files on Fortress. Your home directory becomes your current working directory, by default, when you log in.
Your home directory physically resides on a dedicated storage system only accessible for Scholar. To find the path to your home directory, first log in then immediately enter the following:
$ pwd
/home/myusername
Or from any subdirectory:
$ echo $HOME
/home/myusername
Please note that your Scholar home directory and its contents are exclusive to Scholar cluster, including front-end hosts and compute nodes. This home directory is not available on other RCAC machines but Scholar. There is no automatic copying or synchronization between home directories, but at your discretion you can manually copy all or parts of your main home to Scholar using one of the suggested methods.
Your home directory has a quota limiting the total size of files you may store within. For more information, refer to the Storage Quotas / Limits Section.
Link to section 'Lost File Recovery' of 'Home Directory' Lost File Recovery
Nightly snapshots for 7 days, weekly snapshots for 4 weeks, and monthly snapshots for 3 months are kept. This means you will find snapshots from the last 7 nights, the last 4 Sundays, and the last 3 first of the months. Files are available going back between two and three months, depending on how long ago the last first of the month was. Snapshots beyond this are not kept. For additional security, you should store another copy of your files on more permanent storage, such as the Fortress HPSS Archive.
Link to section 'Performance' of 'Home Directory' Performance
Your home directory is medium-performance, non-purged space suitable for tasks like sharing data, editing files, developing and building software, and many other uses.
Your home directory is not designed or intended for use as high-performance working space for running data-intensive jobs with heavy I/O demands.
Link to section 'Long-Term Storage' of 'Long-Term Storage' Long-Term Storage
Long-term Storage or Permanent Storage is available to users on the High Performance Storage System (HPSS), an archival storage system, called Fortress. Program files, data files and any other files which are not used often, but which must be saved, can be put in permanent storage. Fortress currently has over 10PB of capacity.
For more information about Fortress, how it works, and user guides, and how to obtain an account:
Scratch Space
Scratch directories are provided for short-term file storage only. The quota of your scratch directory is much greater than the quota of your home directory. You should use your scratch directory for storing temporary input files which your job reads or for writing temporary output files which you may examine after execution of your job. You should use your home directory and Fortress for longer-term storage or for holding critical results. The hsi and htar commands provide easy-to-use interfaces into the archive and can be used to copy files into the archive interactively or even automatically at the end of your regular job submission scripts.
Files in scratch directories are not recoverable. Files in scratch directories are not backed up. If you accidentally delete a file, a disk crashes, or old files are purged, they cannot be restored.
Files are purged from scratch directories not accessed or had content modified in 60 days. Owners of these files receive a notice one week before removal via email. Be sure to regularly check your Purdue email account or set up mail forwarding to an email account you do regularly check. For more information, please refer to our Scratch File Purging Policy.
All users may access scratch directories on Scholar. To find the path to your scratch directory:
$ findscratch
/scratch/scholar/myusername
The value of variable $RCAC_SCRATCH is your scratch directory path. Use this variable in any scripts. Your actual scratch directory path may change without warning, but this variable will remain current.
$ echo $RCAC_SCRATCH
/scratch/scholar/myusername
Scratch directories are specific per cluster. I.e. only the /scratch/scholar directory is available on Scholar front-end and compute nodes. No other scratch directories are available on Scholar.
Your scratch directory has a quota capping the total size and number of files you may store in it. For more information, refer to the section Storage Quotas / Limits.
Link to section 'Performance' of 'Scratch Space' Performance
Your scratch directory is located on a high-performance, large-capacity parallel filesystem engineered to provide work-area storage optimized for a wide variety of job types. It is designed to perform well with data-intensive computations, while scaling well to large numbers of simultaneous connections.
/tmp Directory
/tmp directories are provided for short-term file storage only. Each front-end and compute node has a /tmp directory. Your program may write temporary data to the /tmp
directory of the compute node on which it is running. That data is available for as long as your program is active. Once your program terminates, that temporary data is no longer available. When used properly, /tmp
may provide faster local storage to an active process than any other storage option. You should use your home directory and Fortress for longer-term storage or for holding critical results.
Backups are not performed for the /tmp
directory and removes files from /tmp
whenever space is low or whenever the system needs a reboot. In the event of a disk crash or file purge, files in /tmp
are not recoverable. You should copy any important files to more permanent storage.
Link to section 'Sharing Files from Scholar' of 'Sharing' Sharing Files from Scholar
Scholar supports several methods for file sharing. Use the links below to learn more about these methods.
Link to section 'Sharing Data with Globus' of 'Globus' Sharing Data with Globus
Data on any RCAC resource can be shared with other users within Purdue or with collaborators at other institutions. Globus allows convenient sharing of data with outside collaborators. Data can be shared with collaborators' personal computers or directly with many other computing resources at other institutions.
To share files, login to https://transfer.rcac.purdue.edu, navigate to the endpoint (collection) of your choice, and follow instructions as described in Globus documentation on how to share data:
See also RCAC Globus presentation.
File Transfer
Scholar supports several methods for file transfer. Use the links below to learn more about these methods.
SCP
SCP (Secure CoPy) is a simple way of transferring files between two machines that use the SSH protocol. SCP is available as a protocol choice in some graphical file transfer programs and also as a command line program on most Linux, Unix, and Mac OS X systems. SCP can copy single files, but will also recursively copy directory contents if given a directory name.
After Aug 17, 2020, the community clusters will not support password-based authentication for login. Methods that can be used include two-factor authentication (Purdue Login) or SSH keys. If you do not have SSH keys installed, you would need to type your Purdue Login response into the SFTP's "Password" prompt.
Link to section 'Command-line usage:' of 'SCP' Command-line usage:
You can transfer files both to and from Scholar while initiating an SCP session on either some other computer or on Scholar (in other words, directionality of connection and directionality of data flow are independent from each other). The scp
command appears somewhat similar to the familiar cp
command, with an extra user@host:file
syntax to denote files and directories on a remote host. Either Scholar or another computer can be a remote.
-
Example: Initiating SCP session on some other computer (i.e. you are on some other computer, connecting to Scholar):
(transfer TO Scholar) (Individual files) $ scp sourcefile myusername@scholar.rcac.purdue.edu:somedir/destinationfile $ scp sourcefile myusername@scholar.rcac.purdue.edu:somedir/ (Recursive directory copy) $ scp -pr sourcedirectory/ myusername@scholar.rcac.purdue.edu:somedir/ (transfer FROM Scholar) (Individual files) $ scp myusername@scholar.rcac.purdue.edu:somedir/sourcefile destinationfile $ scp myusername@scholar.rcac.purdue.edu:somedir/sourcefile somedir/ (Recursive directory copy) $ scp -pr myusername@scholar.rcac.purdue.edu:sourcedirectory somedir/
The -p flag is optional. When used, it will cause the transfer to preserve file attributes and permissions. The -r flag is required for recursive transfers of entire directories.
-
Example: Initiating SCP session on Scholar (i.e. you are on Scholar, connecting to some other computer):
(transfer TO Scholar) (Individual files) $ scp myusername@$another.computer.example.com:sourcefile somedir/destinationfile $ scp myusername@$another.computer.example.com:sourcefile somedir/ (Recursive directory copy) $ scp -pr myusername@$another.computer.example.com:sourcedirectory/ somedir/ (transfer FROM Scholar) (Individual files) $ scp somedir/sourcefile myusername@$another.computer.example.com:destinationfile $ scp somedir/sourcefile myusername@$another.computer.example.com:somedir/ (Recursive directory copy) $ scp -pr sourcedirectory myusername@$another.computer.example.com:somedir/
The -p flag is optional. When used, it will cause the transfer to preserve file attributes and permissions. The -r flag is required for recursive transfers of entire directories.
Link to section 'Software (SCP clients)' of 'SCP' Software (SCP clients)
Linux and other Unix-like systems:
- The
scp
command-line program should already be installed.
Microsoft Windows:
- MobaXterm
Free, full-featured, graphical Windows SSH, SCP, and SFTP client. - Command-line
scp
program can be installed as part of Windows Subsystem for Linux (WSL), or Git-Bash.
Mac OS X:
- The
scp
command-line program should already be installed. You may start a local terminal window from "Applications->Utilities". - Cyberduck is a full-featured and free graphical SFTP and SCP client.
Globus
Link to section 'Globus' of 'Globus' Globus
Globus, previously known as Globus Online, is a powerful and easy to use file transfer service for transferring files virtually anywhere. It works within RCAC's various research storage systems; it connects between RCAC and remote research sites running Globus; and it connects research systems to personal systems. You may use Globus to connect to your home, scratch, and Fortress storage directories. Since Globus is web-based, it works on any operating system that is connected to the internet. The Globus Personal client is available on Windows, Linux, and Mac OS X. It is primarily used as a graphical means of transfer but it can also be used over the command line.
Link to section 'Link to section 'Globus Web:' of 'Globus' Globus Web:' of 'Globus' Link to section 'Globus Web:' of 'Globus' Globus Web:
- Navigate to http://transfer.rcac.purdue.edu
- Click "Proceed" to log in with your Purdue Career Account.
- On your first login it will ask to make a connection to a Globus account. Accept the conditions.
- Now you are at the main screen. Click "File Transfer" which will bring you to a two-panel interface (if you only see one panel, you can use selector in the top-right corner to switch the view).
- You will need to select one collection and file path on one side as the source, and the second collection on the other as the destination. This can be one of several Purdue endpoints, or another University, or even your personal computer (see Personal Client section below).
The RCAC collections are as follows. A search for "Purdue" will give you several suggested results you can choose from, or you can give a more specific search.
- Home Directory storage: "Purdue Research Computing - Home Directories", however, you can start typing "Purdue" and "Home Directories" and it will suggest appropriate matches.
- Weber scratch storage: "Purdue Weber Cluster", however, you can start typing "Purdue" and "Weber and it will suggest appropriate matches. From here you will need to navigate into the first letter of your username, and then into your username.
- Research Data Depot: "Purdue Research Computing - Data Depot", a search for "Depot" should provide appropriate matches to choose from.
- Fortress: "Purdue Fortress HPSS Archive", a search for "Fortress" should provide appropriate matches to choose from.
From here, select a file or folder in either side of the two-pane window, and then use the arrows in the top-middle of the interface to instruct Globus to move files from one side to the other. You can transfer files in either direction. You will receive an email once the transfer is completed.
Link to section 'Link to section 'Globus Personal Client setup:' of 'Globus' Globus Personal Client setup:' of 'Globus' Link to section 'Globus Personal Client setup:' of 'Globus' Globus Personal Client setup:
Globus Connect Personal is a small software tool you can install to make your own computer a Globus endpoint on its own. It is useful if you need to transfer files via Globus to and from your computer directly.
- On the "Collections" page from earlier, click "Get Globus Connect Personal" or download a version for your operating system it from here: Globus Connect Personal
- Name this particular personal system and follow the setup prompts to create your Globus Connect Personal endpoint.
- Your personal system is now available as a collection within the Globus transfer interface.
Link to section 'Link to section 'Globus Command Line:' of 'Globus' Globus Command Line:' of 'Globus' Link to section 'Globus Command Line:' of 'Globus' Globus Command Line:
Globus supports command line interface, allowing advanced automation of your transfers.
To use the recommended standalone Globus CLI application (the globus command):
- First time use: issue the globus login command and follow instructions for initial login.
- Commands for interfacing with the CLI can be found via Using the Command Line Interface, as well as the Globus CLI Examples pages.
Link to section 'Link to section 'Sharing Data with Outside Collaborators' of 'Globus' Sharing Data with Outside Collaborators' of 'Globus' Link to section 'Sharing Data with Outside Collaborators' of 'Globus' Sharing Data with Outside Collaborators
Globus allows convenient sharing of data with outside collaborators. Data can be shared with collaborators' personal computers or directly with many other computing resources at other institutions. See the Globus documentation on how to share data:
For links to more information, please see Globus Support page and RCAC Globus presentation.
Windows Network Drive / SMB
SMB (Server Message Block), also known as CIFS, is an easy to use file transfer protocol that is useful for transferring files between RCAC systems and a desktop or laptop. You may use SMB to connect to your home, scratch, and Fortress storage directories. The SMB protocol is available on Windows, Linux, and Mac OS X. It is primarily used as a graphical means of transfer but it can also be used over the command line.
Note: to access Scholar through SMB file sharing, you must be on a Purdue campus network or connected through VPN.
Link to section 'Windows:' of 'Windows Network Drive / SMB' Windows:
- Windows 7: Click Windows menu > Computer, then click Map Network Drive in the top bar
- Windows 8 & 10: Tap the Windows key, type computer, select This PC, click Computer > Map Network Drive in the top bar
- Windows 11: Tap the Windows key, type File Explorer, select This PC, click Computer > Map Network Drive in the top bar
- In the folder location enter the following information and click Finish:
- To access your Scholar home directory, enter \\home.scholar.rcac.purdue.edu\scholar-home.
- To access your scratch space on Scholar, enter \\scratch.scholar.rcac.purdue.edu\scholar-scratch. Once mapped, you will be able to navigate to your scratch directory.
- Note: Use your career account login name and password when prompted. (You will not need to add "
,push
" nor use your Purdue Duo client.) - Your home or scratch directory should now be mounted as a drive in the Computer window.
Link to section 'Mac OS X:' of 'Windows Network Drive / SMB' Mac OS X:
- In the Finder, click Go > Connect to Server
- In the Server Address enter the following information and click Connect:
- To access your Scholar home directory, enter smb://home.scholar.rcac.purdue.edu/scholar-home.
- To access your scratch space on Scholar, enter smb://scratch.scholar.rcac.purdue.edu/scholar-scratch. Once mapped, you will be able to navigate to your scratch directory.
- Note: Use your career account login name and password when prompted. (You will not need to add "
,push
" nor use your Purdue Duo client.) - Your home or scratch directory should now be mounted as a drive in the Computer window.
Link to section 'Linux:' of 'Windows Network Drive / SMB' Linux:
- There are several graphical methods to connect in Linux depending on your desktop environment. Once you find out how to connect to a network server on your desktop environment, choose the Samba/SMB protocol and adapt the information from the Mac OS X section to connect.
- If you would like access via samba on the command line you may install smbclient which will give you FTP-like access and can be used as shown below. For all the possible ways to connect look at the Mac OS X instructions.
smbclient //home.scholar.rcac.purdue.edu/scholar-home -U myusername smbclient //scratch.scholar.rcac.purdue.edu/scholar-scratch -U myusername
- Note: Use your career account login name and password when prompted. (You will not need to add "
,push
" nor use your Purdue Duo client.)
FTP / SFTP
FTP is not supported on any research systems because it does not allow for secure transmission of data. Use SFTP instead, as described below.
SFTP (Secure File Transfer Protocol) is a reliable way of transferring files between two machines. SFTP is available as a protocol choice in some graphical file transfer programs and also as a command-line program on most Linux, Unix, and Mac OS X systems. SFTP has more features than SCP and allows for other operations on remote files, remote directory listing, and resuming interrupted transfers. Command-line SFTP cannot recursively copy directory contents; to do so, try using SCP or graphical SFTP client.
After Aug 17, 2020, the community clusters will not support password-based authentication for login. Methods that can be used include two-factor authentication (Purdue Login) or SSH keys. If you do not have SSH keys installed, you would need to type your Purdue Login response into the SFTP's "Password" prompt.
Link to section 'Command-line usage' of 'FTP / SFTP' Command-line usage
You can transfer files both to and from Scholar while initiating an SFTP session on either some other computer or on Scholar (in other words, directionality of connection and directionality of data flow are independent from each other). Once the connection is established, you use put
or get
subcommands between "local" and "remote" computers. Either Scholar or another computer can be a remote.
-
Example: Initiating SFTP session on some other computer (i.e. you are on another computer, connecting to Scholar):
$ sftp myusername@scholar.rcac.purdue.edu (transfer TO Scholar) sftp> put sourcefile somedir/destinationfile sftp> put -P sourcefile somedir/ (transfer FROM Scholar) sftp> get sourcefile somedir/destinationfile sftp> get -P sourcefile somedir/ sftp> exit
The -P flag is optional. When used, it will cause the transfer to preserve file attributes and permissions.
-
Example: Initiating SFTP session on Scholar (i.e. you are on Scholar, connecting to some other computer):
$ sftp myusername@$another.computer.example.com (transfer TO Scholar) sftp> get sourcefile somedir/destinationfile sftp> get -P sourcefile somedir/ (transfer FROM Scholar) sftp> put sourcefile somedir/destinationfile sftp> put -P sourcefile somedir/ sftp> exit
The -P flag is optional. When used, it will cause the transfer to preserve file attributes and permissions.
Link to section 'Software (SFTP clients)' of 'FTP / SFTP' Software (SFTP clients)
Linux and other Unix-like systems:
- The
sftp
command-line program should already be installed.
Microsoft Windows:
- MobaXterm
Free, full-featured, graphical Windows SSH, SCP, and SFTP client. - Command-line
sftp
program can be installed as part of Windows Subsystem for Linux (WSL), or Git-Bash.
Mac OS X:
- The
sftp
command-line program should already be installed. You may start a local terminal window from "Applications->Utilities". - Cyberduck is a full-featured and free graphical SFTP and SCP client.
Copying files from Purdue IT research computing home directory to Scholar
The Scholar home directory and its contents are specific to the Scholar cluster, and are not available on other RCAC machines. For people having access to other Community Clusters and Scholar, there is no automatic copying or synchronization between main and Scholar home directories. At your discretion, you can manually copy all or parts of your main research computing home to Scholar using one of the methods described below.
Please note that copying may fail if the size of your research computing home directory is larger than the Scholar one's quota. Please check usage and limits before proceeding!
Link to section 'Complete copy' of 'Copying files from Purdue IT research computing home directory to Scholar' Complete copy
For your convenience, a custom tool copy-rcac-home
is provided to simplify at-will duplication of your main research computing home directory into Scholar. The tool performs a complete 1-to-1 copy using rsync -auH
(with exception of a narrow subset of system-specific service files).
To use the tool, simply type copy-rcac-home
in a terminal window on a Scholar front-end or compute node:
$ copy-rcac-home
This script will copy entire contents of your main RCAC
home directory into your Scholar cluster's $HOME.
Note: copying may fail if the size of your RCAC home directory
is larger than your quota on the Scholar one (25GB).
BEFORE PROCEEDING, please run 'myquota' command on another
cluster to see your usage there and judge whether it would fit!
Would you like to proceed? [Y/n]:
At this stage answering yes
will proceed with copying, or you can respond with a no
(or Ctrl-C
) to cancel. See copy-rcac-home --help
for more details on the tool.
Link to section 'Partial copy' of 'Copying files from Purdue IT research computing home directory to Scholar' Partial copy
Desired parts (or whole) of your research computing home directories can be copied to Scholar via any of the home directories' supported transfer methods, such as SCP, SFTP, rsync, or Globus.
-
Example: recursive copying of a subdirectory from RCAC home directory into Scholar home using
scp
.(if you are on Scholar, use other cluster name for the remote part) $ scp -pr myothercluster.rcac.purdue.edu:somedirectory/ ~/ (if you are on another cluster, use Scholar for the remote part) $ scp -pr somedirectory/ myusername@scholar.rcac.purdue.edu:~/
-
Example: copying using Globus.
Search collections for "Purdue Research Computing - Home Directories" and "Purdue Scholar Cluster - Home" endpoints, respectively, then transfer desired files and/or directories as usual.
Storage Quota / Limits
Some limits are imposed on your disk usage on research systems. A quota is implemented on each filesystem. Each filesystem (home directory, scratch directory, etc.) may have a different limit. If you exceed the quota, you will not be able to save new files or new data to the filesystem until you delete or move data to long-term storage.
Link to section 'Checking Quota' of 'Storage Quota / Limits' Checking Quota
To check the current quotas of your home and scratch directories check the My Quota page or use the myquota
command:
$ myquota
Type Filesystem Size Limit Use Files Limit Use
==============================================================================
home myusername 5.0GB 25.0GB 20% - - -
scratch scholar 220.7GB 100.0TB 0.22% 8k 2,000k 0.43%
The columns are as follows:
- Type: indicates home or scratch directory or your depot space.
- Filesystem: name of storage option.
- Size: sum of file sizes in bytes.
- Limit: allowed maximum on sum of file sizes in bytes.
- Use: percentage of file-size limit currently in use.
- Files: number of files and directories (not the size).
- Limit: allowed maximum on number of files and directories. It is possible, though unlikely, to reach this limit and not the file-size limit if you create a large number of very small files.
- Use: percentage of file-number limit currently in use.
If you find that you reached your quota in either your home directory or your scratch file directory, obtain estimates of your disk usage. Find the top-level directories which have a high disk usage, then study the subdirectories to discover where the heaviest usage lies.
To see in a human-readable format an estimate of the disk usage of your top-level directories in your home directory:
$ du -h --max-depth=1 $HOME >myfile
32K /home/myusername/mysubdirectory_1
529M /home/myusername/mysubdirectory_2
608K /home/myusername/mysubdirectory_3
The second directory is the largest of the three, so apply command du to it.
To see in a human-readable format an estimate of the disk usage of your top-level directories in your scratch file directory:
$ du -h --max-depth=1 $RCAC_SCRATCH >myfile
160K /scratch/scholar/myusername
This strategy can be very helpful in figuring out the location of your largest usage. Move unneeded files and directories to long-term storage to free space in your home and scratch directories.
Link to section 'Increasing Quota' of 'Storage Quota / Limits' Increasing Quota
Link to section 'Home Directory' of 'Storage Quota / Limits' Home Directory
If you find you need additional disk space in your home directory, please consider archiving and compressing old files and moving them to long-term storage on the Fortress HPSS Archive, or purchase the Depot space for long-term storage. Unfortunately, it is not possible to increase your home directory quota beyond it's current level.
Link to section 'Scratch Space' of 'Storage Quota / Limits' Scratch Space
If you find you need additional disk space in your scratch space, please first consider archiving and compressing old files and moving them to long-term storage on the Fortress HPSS Archive. If you are unable to do so, you may ask for a quota increase by contacting support.
Lost File Recovery
Scholar is protected against accidental file deletion through a series of snapshots taken every night just after midnight. Each snapshot provides the state of your files at the time the snapshot was taken. It does so by storing only the files which have changed between snapshots. A file that has not changed between snapshots is only stored once but will appear in every snapshot. This is an efficient method of providing snapshots because the snapshot system does not have to store multiple copies of every file.
These snapshots are kept for a limited time at various intervals. RCAC keeps nightly snapshots for 7 days, weekly snapshots for 4 weeks, and monthly snapshots for 3 months. This means you will find snapshots from the last 7 nights, the last 4 Sundays, and the last 3 first of the months. Files are available going back between two and three months, depending on how long ago the last first of the month was. Snapshots beyond this are not kept.
Only files which have been saved during an overnight snapshot are recoverable. If you lose a file the same day you created it, the file is not recoverable because the snapshot system has not had a chance to save the file.
Snapshots are not a substitute for regular backups. It is the responsibility of the researchers to back up any important data to the Fortress Archive. Scholar does protect against hardware failures or physical disasters through other means however these other means are also not substitutes for backups.
Files in scratch directories are not recoverable. Files in scratch directories are not backed up. If you accidentally delete a file, a disk crashes, or old files are purged, they cannot be restored.
Scholar offers several ways for researchers to access snapshots of their files.
flost
If you know when you lost the file, the easiest way is to use the flost command. This tool is available from any RCAC resource. If you do not have access to a compute cluster, any Data Depot user may use an SSH client to connect to scholar.rcac.purdue.edu and run this command.
To run the tool you will need to specify the location where the lost file was with the -w argument:
$ flost -w /depot/mylab
Replace mylab with the name of your lab's Scholar directory. If you know more specifically where the lost file was you may provide the full path to that directory.
This tool will prompt you for the date on which you lost the file or would like to recover the file from. If the tool finds an appropriate snapshot it will provide instructions on how to search for and recover the file.
If you are not sure what date you lost the file you may try entering different dates into the flost to try to find the file or you may also manually browse the snapshots as described below.
Manual Browsing
You may also search through the snapshots by hand on the Scholar filesystem if you are not sure what date you lost the file or would like to browse by hand. Snapshots can be browsed from any RCAC resource. If you do not have access to a compute cluster, any Scholar user may use an SSH client to connect to scholar.rcac.purdue.edu and browse from there. The snapshots are located at /depot/.snapshots
on these resources.
You can also mount the snapshot directory over Samba (or SMB, CIFS) on Windows or Mac OS X. Mount (or map) the snapshot directory in the same way as you did for your main Scholar space substituting the server name and path for \\datadepot.rcac.purdue.edu\depot\.winsnaps
(Windows) or smb://datadepot.rcac.purdue.edu/depot/.winsnaps
(Mac OS X).
Once connected to the snapshot directory through SSH or Samba, you will see something similar to this:
SSH to scholar.rcac.purdue.edu | Samba mount on datadepot.rcac.purdue.edu |
---|---|
|
![]() |
Each of these directories is a snapshot of the entire Scholar filesystem at the timestamp encoded into the directory name. The format for this timestamp is year, two digits for month, two digits for day, followed by the time of the day.
You may cd into any of these directories where you will find the entire Scholar filesystem. Use cd to continue into your lab's Scholar space and then you may browse the snapshot as normal.
If you are browsing these directories over a Samba network drive you can simply drag and drop the files over into your live Data Depot folder.
Once you find the file you are looking for, use cp to copy the file back into your lab's live Scholar space. Do not attempt to modify files directly in the snapshot directories.
Windows
If you use Scholar through "network drives" on Windows you may recover lost files directly from within Windows:
- Open the folder that contained the lost file.
- Right click inside the window and select "Properties".
- Click on the "Previous Versions" tab.
- A list of snapshots will be displayed.
- Select the snapshot from which you wish to restore.
- In the new window, locate the file you wish to restore.
- Simply drag the file or folder to their correct locations.
In the "Previous Versions" window the list contains two columns. The first column is the timestamp on which the snapshot was taken. The second column is the date on which the selected file or folder was last modified in that snapshot. This may give you some extra clues to which snapshot contains the version of the file you are looking for.
Mac OS X
Mac OS X does not provide any way to access the Scholar snapshots directly. To access the snapshots there are two options: browse the snapshots by hand through a network drive mount or use an automated command-line based tool.
To browse the snapshots by hand, follow the directions outlined in the Manual Browsing section.
To use the automated command-line tool, log into a compute cluster or into the host scholar.rcac.purdue.edu (which is available to all Scholar users) and use the flost tool. On Mac OS X you can use the built-in SSH terminal application to connect.
- Open the Applications folder from Finder.
- Navigate to the Utilities folder.
- Double click the Terminal application to open it.
- Type the following command when the terminal opens.
Replace myusername with your Purdue career account username and provide your password when prompted.$ ssh myusername@scholar.rcac.purdue.edu
Once logged in use the flost tool as described above. The tool will guide you through the process and show you the commands necessary to retrieve your lost file.
Gateway (Open OnDemand)
Scholar's Gateway is an open-source HPC portal developed by the Ohio Supercomputing Center. Open OnDemand allows one to interact with HPC resources through a web browser and easily manage files, submit jobs, and interact with graphical applications directly in a browser, all with no software to install. Scholar has an instance of OnDemand available that can be accessed via gateway.scholar.rcac.purdue.edu.
Link to section 'Logging In' of 'Gateway (Open OnDemand)' Logging In
To log into Gateway:
- Navigate to gateway.scholar.rcac.purdue.edu
- Log in using your Career account username and Purdue Login Duo client.
On the splash page you will see a quota usage report. If you are over 90% on any of your quotas a warning will be displayed. This information will update every 10-15 minutes while you are active on Gateway.
Link to section 'Apps' of 'Gateway (Open OnDemand)' Apps
There are a number of built-in apps in Gateway that can be accessed from the top menu bar. Below are links to documentation on each app.
Interactive Apps
There are several interactive apps available through Gateway that can be accessed through the Interactive Apps dropdown menu. These apps are provided with a basic node and software configuration as a 'quick-launch' option to get your work up and running quickly. For simplicity, minimal options are provided - these apps are not intended for complex configuration/customization scenarios.
After you a submit an interactive app to the queue, Gateway will track and manage the session. Once it starts, you may connect and disconnect from the session in your browser, leaving the job running while you log out of your browser.
Each of the available apps are documented through the following links.
Compute Node Desktop
The Compute Node Desktop app will launch a graphical desktop session on a compute node. This is similar to using Thinlinc, however, this gives you a desktop directly on a compute node instead on a front-end. This app is useful if you have a custom application or application not directly available as an interactive app you would like to run inside Gateway.
To launch a desktop session on a compute node, select the Scholar Compute Desktop app. From the submit form, select from the available options - the queue to which you wish to submit and the number of wallclock hours you wish to have job running. There is also a checkbox that enable a notification to your email when the job starts.
After the interactive job is submitted you will be taken to your list of active interactive app sessions. You can monitor the status of the job from here until it starts, or if you enabled the email notification, watch your Purdue email for the notification the job has started.
Once it is indicated the job has started you can connect to the desktop with the "Launch noVNC in New Tab" button. The session will be terminated after the wallclock hours you specified have elapsed or you terminate the session early with the "Delete" button from the list of sessions. Deleting the session when you are finished will free up queue resources for your lab mates and other users on the system.
Windows Desktop
The Windows Desktop app will launch a Windows desktop session on a compute node. This is similar to using the Windows menu launcher through Thinlinc, however, this gives you a Windows desktop directly on a compute node instead on a front-end.
To launch a Windows session on a compute node, select the Windows Desktop app. From the submit form, select from the available options - choose from the basic Windows configuration or the GIS configured image, the queue to which you wish to submit, and the number of wallclock hours you wish to have job running. There is also a checkbox that enable a notification to your email when the job starts.
This will create a file in your scratch space called windows-base.qcow2
or windows-gis.qcow2
. If the file already exists, the existing image will be restarted. You can delete or rename the image at any time through the Files App to generate a fresh image. You can only have one instance of the image running at a time or corruption will occur. There are lock files to prevent this, but be mindful of this restriction. It is also recommended you make periodic backups of the image if you are making any modifications to it.
After the interactive job is submitted you will be taken to your list of active interactive app sessions. You can monitor the status of the job from here until it starts, or if you enabled the email notification, watch your Purdue email for the notification the job has started.
Once it is indicated the job has started you can connect to the desktop with the "Launch noVNC in New Tab" button. The session will be terminated after the wallclock hours you specified have elapsed or you terminate the session early with the "Delete" button from the list of sessions. Deleting the session when you are finished will free up queue resources for your lab mates and other users on the system.
Jupyter Notebook
The Notebook app will launch a Notebook session on a compute node and allow you to connect directly to it in a web browser.
To launch a Notebook session on a compute node, select the Notebook app. From the submit form, select from the available options:
- Queue: This is a dropdown menu from which you can select a queue from all of the queues to which you have permission to submit.
- Walltime: This is a field which expects a number and represents how many hours you want to keep the session running. Note that this value should not exceed the maximum value given next to the selected queue name from the queue dropdown menu.
- Number of Cores/GPUs: This is a field which expects a number and represents the number of your resources your session is requesting. Note that the amount of memory allocated for your session is proportional to the number of cores or GPUs that you request for your job, so if your session is running out of memory, consider increasing this value.
- Use Jupyter Lab: This is a checkbox which, when checked, will run Jupyter Lab instead of Jupyter Notebook. Both of these applications are interfaces to Jupyter, and you can launch Jupyter notebooks from within Jupyter Lab. Jupyter Notebook is more "barebones" while Jupyter Lab has additional features such as the ability to interact with additional file types.
- E-mail Notice: This is a checkbox which, when checked, will send you an e-mail notification to your Purdue e-mail that your session is ready when the scheduler has found resources to dedicate to your session.
After the interactive job is submitted you will be taken to your list of active interactive app sessions. You can monitor the status of the job from here until it starts, or if you enabled the email notification, watch your Purdue email for the notification the job has started.
Once it is indicated the job has started you can connect to the desktop with the "Connect to Jupyter" button. Once connected, you can create new notebooks, selecting the currently available Anaconda versions available as modules, and any personally created Notebook kernels.
Often times you may want to use one of your existing Anaconda environments within your Jupyter session to use libraries specific to your workflow. In order to do so, you must ensure that the Anaconda environment you want to use contains the Python packages "IPyKernel" and "IPython" which are packages that are required by Jupyter. When you create a Jupyter session, Open OnDemand will check through your existing Anaconda environments and create a Jupyter kernel for any Anaconda environment that contains these two packages, and you will be able to select to use that kernel from within the application.
The session will be terminated after the number of hours you specified have elapsed or you terminate the session early with the "Delete" button from the list of sessions. Deleting the session when you are finished will free up queue resources for your lab mates and other users on the system.
MATLAB
The MATLAB app will launch a MATLAB session on a compute node and allow you to connect directly to it in a web browser.
To launch a MATLAB session on a compute node, select the MATLAB app. From the submit form, select from the available options - the version of MATLAB you are interested in running, the queue to which you wish to submit, and the number of wallclock hours you wish to have job running. There is also a checkbox that enable a notification to your email when the job starts.
After the interactive job is submitted you will be taken to your list of active interactive app sessions. You can monitor the status of the job from here until it starts, or if you enabled the email notification, watch your Purdue email for the notification the job has started.
Once it is indicated the job has started you can connect to the desktop with the "Launch noVNC in New Tab" button. The session will be terminated after the wallclock hours you specified have elapsed or you terminate the session early with the "Delete" button from the list of sessions. Deleting the session when you are finished will free up queue resources for your lab mates and other users on the system.
RStudio Server
The RStudio app will launch a RStudio session on a compute node and allow you to connect directly to it in a web browser.
To launch a RStudio session on a compute node, select the RStudio app. From the submit form, select from the available options - the queue to which you wish to submit, and the number of wallclock hours you wish to have job running. There is also a checkbox that enable a notification to your email when the job starts.
After the interactive job is submitted you will be taken to your list of active interactive app sessions. You can monitor the status of the job from here until it starts, or if you enabled the email notification, watch your Purdue email for the notification the job has started.
Once it is indicated the job has started you can connect to the desktop with the "Connect to RStudio Server" button. The session will be terminated after the wallclock hours you specified have elapsed or you terminate the session early with the "Delete" button from the list of sessions. Deleting the session when you are finished will free up queue resources for your lab mates and other users on the system.
Files
The Files app will let you access your files in your Home Directory, Scratch, and Data Depot spaces. The app lets you manage create, manage, and delete files and directories from your web browser. Navigate by double clicking on folders in the file explorer or by using the file tree on the left.
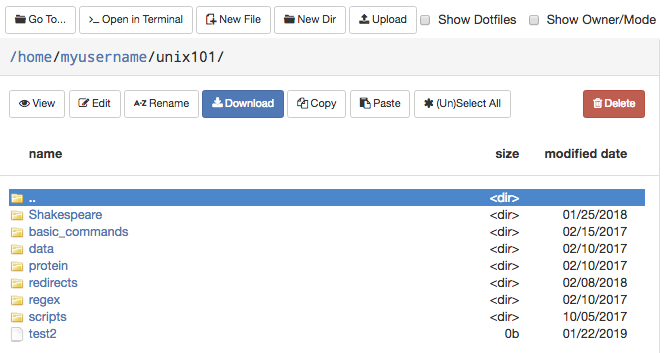
On the top row, there are buttons to:
- Go To: directly input a directory to navigate to
- Open in Terminal: launches the Shell app and navigates you to the current directory in the terminal
- New File: creates a new, empty file
- New Dir: creates a new, empty directory
- Upload: upload a file from your computer
Note: File uploads from your browser are limited to 100 GB per file. Be mindful that uploads over a few gigabytes may be unreliable through your browser, especially from off-campus connections. For very large files or off-campus transfers alternative methods such as Globus are highly recommended.
The second row of buttons lets you perform typical file management operations. The Edit button will open files in a fully fledged browser based text editor - it features syntax highlighting and vim and Emacs key bindings.
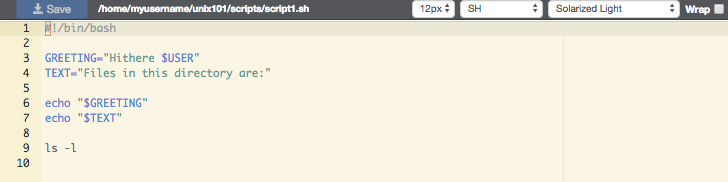
Jobs
There are two apps under the Jobs apps: Active Jobs and Job Composer. These are detailed below.
Link to section 'Active Jobs' of 'Jobs' Active Jobs
This shows you active SLURM jobs currently on the cluster. The default view will show you your current jobs, similar to squeue -u rices
. Using the button labeled "Your Jobs" in the upper right allows you to select different filters by queue (account). All accounts output by slist
will appear for you here. Using the arrow on the left hand side will expand the full job details.
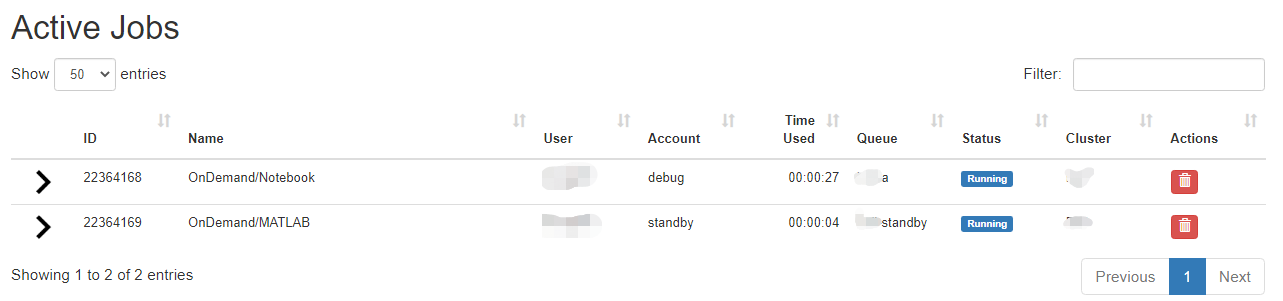
Link to section 'Job Composer' of 'Jobs' Job Composer
The Job Composer app allows you to create and submit jobs to the cluster. You can select from pre-defined templates (most of these are taken from the User Guide examples) or you can create your own templates for frequently used workflows.
Link to section 'Creating Job from Existing Template' of 'Jobs' Creating Job from Existing Template
Click "New Job" menu, then select "From Template":
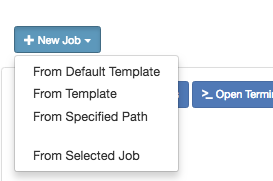
Then select from one of the available templates.
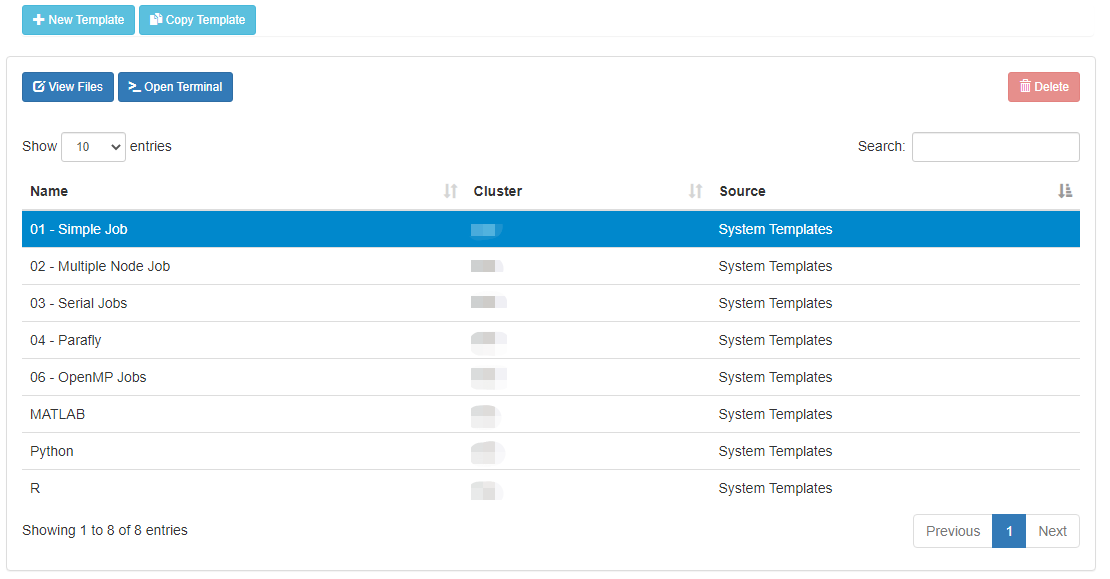
Click 'Create New Job' in second pane.
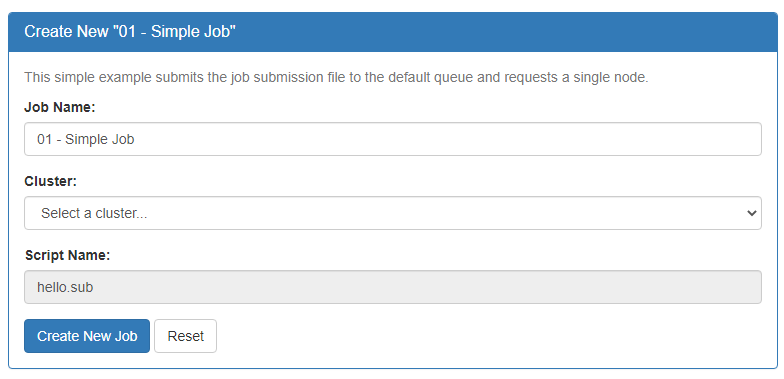
Your new job should be selected in your list of jobs. In the 'Submit Script' pane you can see the job script that was generated with an 'Open Editor' link to open the script in the built-in editor. Open the file in the editor and edit the script as necessary. By default the job will specify standby queue - this should be changed as appropriate, along with the node and walltime requests.
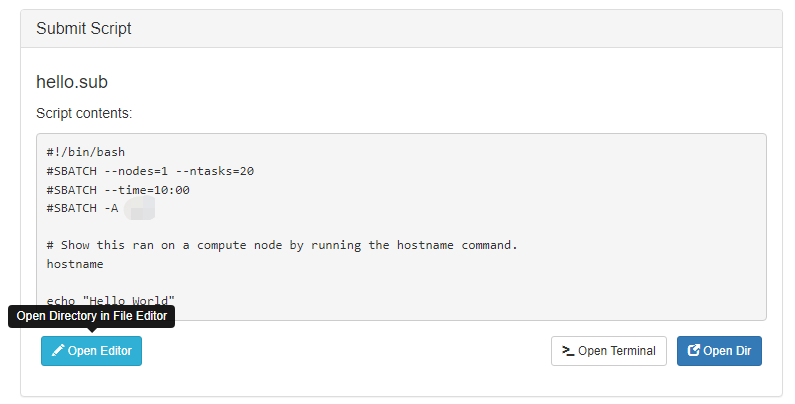
When you are finished with editing the job and are ready to submit, click the green 'Submit' button at the top of the job list. You can monitor progress from here or from the Active Jobs app. Once completed, you should see the output files appear:
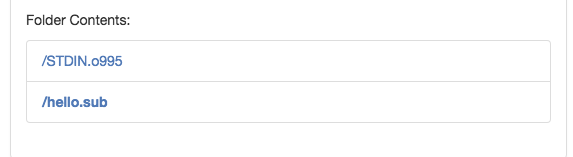
Clicking on one of the output files will open it in the file editor for your viewing.
Link to section 'Creating New Template' of 'Jobs' Creating New Template
First, prepare a template directory containing a template submission script along with any input files. Then, to import the job into the Job Composer app, click the 'Create New Template' button. Fill in the directory containing your template job script and files in the first box. Give it an appropriate name and notes.
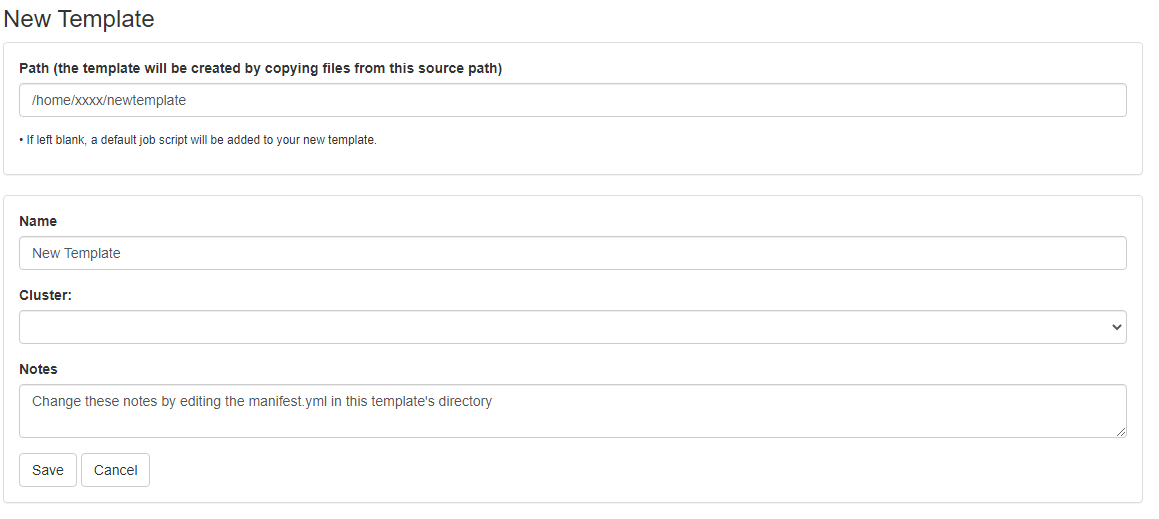
This template will now appear in your list of templates to choose from when composing jobs. You can now go create and submit a job from this new template.
Cluster Tools
The Cluster Tools menu contains cluster utilities. At the moment, only a terminal app is provided. Additional apps may be developed and provided in the future.
Link to section 'Shell Access' of 'Cluster Tools' Shell Access
Launching the shell app will provide you with a web-based terminal session on the cluster front-end. This is equivalent to using a standalone SSH client to connect to scholar.rcac.purdue.edu
where you are connected to one several front-ends. The normal acceptable front-end use policy applies to access through the web-app. X11 Forwarding is not supported. Use of one of the interactive apps is recommended for graphical applications.
Software
Link to section 'Environment module' of 'Software' Environment module
Link to section 'Software catalog' of 'Software' Software catalog
Compiling Source Code
Documentation on compiling source code on Scholar.
Compiling Serial Programs
A serial program is a single process which executes as a sequential stream of instructions on one processor core. Compilers capable of serial programming are available for C, C++, and versions of Fortran.
Here are a few sample serial programs:
- serial_hello.f
- serial_hello.f90
- serial_hello.f95
- serial_hello.c
-
To load a compiler, enter one of the following:
$ module load intel
$ module load gcc
Language | Intel Compiler | GNU Compiler | |
---|---|---|---|
Fortran 77 |
|
|
|
Fortran 90 |
|
|
|
Fortran 95 |
|
|
|
C |
|
|
|
C++ |
|
|
The Intel and GNU compilers will not output anything for a successful compilation. Also, the Intel compiler does not recognize the suffix ".f95".
Compiling MPI Programs
OpenMPI and Intel MPI (IMPI) are implementations of the Message-Passing Interface (MPI) standard. Libraries for these MPI implementations and compilers for C, C++, and Fortran are available on all clusters.
Language | Header Files |
---|---|
Fortran 77 |
|
Fortran 90 |
|
Fortran 95 |
|
C |
|
C++ |
|
Here are a few sample programs using MPI:
To see the available MPI libraries:
$ module avail openmpi
$ module avail impi
Language | Intel MPI | OpenMPI |
---|---|---|
Fortran 77 |
|
|
Fortran 90 |
|
|
Fortran 95 |
|
|
C |
|
|
C++ |
|
|
The Intel and GNU compilers will not output anything for a successful compilation. Also, the Intel compiler does not recognize the suffix ".f95".
Here is some more documentation from other sources on the MPI libraries:
Compiling OpenMP Programs
All compilers installed on Brown include OpenMP functionality for C, C++, and Fortran. An OpenMP program is a single process that takes advantage of a multi-core processor and its shared memory to achieve a form of parallel computing called multithreading. It distributes the work of a process over processor cores in a single compute node without the need for MPI communications.
Language | Header Files |
---|---|
Fortran 77 |
|
Fortran 90 |
|
Fortran 95 |
|
C |
|
C++ |
|
Sample programs illustrate task parallelism of OpenMP:
A sample program illustrates loop-level (data) parallelism of OpenMP:
To load a compiler, enter one of the following:
$ module load intel
$ module load gcc
Language | Intel Compiler | GNU Compiler |
---|---|---|
Fortran 77 |
|
|
Fortran 90 |
|
|
Fortran 95 |
|
|
C |
|
|
C++ |
|
|
The Intel and GNU compilers will not output anything for a successful compilation. Also, the Intel compiler does not recognize the suffix ".f95".
Here is some more documentation from other sources on OpenMP:
Compiling Hybrid Programs
A hybrid program combines both MPI and shared-memory to take advantage of compute clusters with multi-core compute nodes. Libraries for OpenMPI and Intel MPI (IMPI) and compilers which include OpenMP for C, C++, and Fortran are available.
Language | Header Files |
---|---|
Fortran 77 |
|
Fortran 90 |
|
Fortran 95 |
|
C |
|
C++ |
|
A few examples illustrate hybrid programs with task parallelism of OpenMP:
This example illustrates a hybrid program with loop-level (data) parallelism of OpenMP:
To see the available MPI libraries:
$ module avail impi
$ module avail openmpi
The following tables illustrate how to compile your hybrid (MPI/OpenMP) program. Any compiler flags accepted by Intel ifort/icc compilers are compatible with their respective MPI compiler.
Language | Command |
---|---|
Fortran 77 |
|
Fortran 90 |
|
Fortran 95 |
|
C |
|
C++ |
|
Language | Command |
---|---|
Fortran 77 |
|
Fortran 90 |
|
Fortran 95 |
|
C |
|
C++ |
|
The Intel and GNU compilers will not output anything for a successful compilation. Also, the Intel compiler does not recognize the suffix .f95
.
Intel MKL Library
Intel Math Kernel Library (MKL) contains ScaLAPACK, LAPACK, Sparse Solver, BLAS, Sparse BLAS, CBLAS, GMP, FFTs, DFTs, VSL, VML, and Interval Arithmetic routines. MKL resides in the directory stored in the environment variable MKL_HOME, after loading a version of the Intel compiler with module.
By using module load to load an Intel compiler your environment will have several variables set up to help link applications with MKL. Here are some example combinations of simplified linking options:
$ module load intel
$ echo $LINK_LAPACK
-L${MKL_HOME}/lib/intel64 -lmkl_intel_lp64 -lmkl_intel_thread -lmkl_core -liomp5 -lpthread
$ echo $LINK_LAPACK95
-L${MKL_HOME}/lib/intel64 -lmkl_lapack95_lp64 -lmkl_blas95_lp64 -lmkl_intel_lp64 -lmkl_intel_thread -lmkl_core -liomp5 -lpthread
RCAC recommends you use the provided variables to define MKL linking options in your compiling procedures. The Intel compiler modules also provide two other environment variables, LINK_LAPACK_STATIC and LINK_LAPACK95_STATIC that you may use if you need to link MKL statically.
RCAC recommends that you use dynamic linking of libguide. If so, define LD_LIBRARY_PATH such that you are using the correct version of libguide at run time. If you use static linking of libguide, then:
- If you use the Intel compilers, link in the libguide version that comes with the compiler (use the -openmp option).
- If you do not use the Intel compilers, link in the libguide version that comes with the Intel MKL above.
Here are some more documentation from other sources on the Intel MKL:
Compiling GPU Programs
The Scholar cluster nodes contain 1 GPU that support CUDA and OpenCL. See the detailed hardware overview for the specifics on the GPUs in Scholar. This section focuses on using CUDA.
A simple CUDA program has a basic workflow:
- Initialize an array on the host (CPU).
- Copy array from host memory to GPU memory.
- Apply an operation to array on GPU.
- Copy array from GPU memory to host memory.
Here is a sample CUDA program:
Both front-ends and GPU-enabled compute nodes have the CUDA tools and libraries available to compile CUDA programs. To compile a CUDA program, load CUDA, and use nvcc to compile the program:
$ module load gcc/11.4.1 cuda/12.6.0
$ nvcc gpu_hello.cu -o gpu_hello
./gpu_hello
No GPU specified, using first GPUhello, world
The example illustrates only how to copy an array between a CPU and its GPU but does not perform a serious computation.
The following program times three square matrix multiplications on a CPU and on the global and shared memory of a GPU:
$ module load cuda
$ nvcc mm.cu -o mm
$ ./mm 0
speedup
-------
Elapsed time in CPU: 6555.2 milliseconds
Elapsed time in GPU (global memory): 32.9 milliseconds 199.1
Elapsed time in GPU (shared memory): 3.0 milliseconds 2191.8
For best performance, the input array or matrix must be sufficiently large to overcome the overhead in copying the input and output data to and from the GPU.
For more information about NVIDIA, CUDA, and GPUs:
Running Jobs
There is one method for submitting jobs to Scholar. You may use SLURM to submit jobs to a partition on Scholar. SLURM performs job scheduling. Jobs may be any type of program. You may use either the batch or interactive mode to run your jobs. Use the batch mode for finished programs; use the interactive mode only for debugging.
In this section, you'll find a few pages describing the basics of creating and submitting SLURM jobs. As well, a number of example SLURM jobs that you may be able to adapt to your own needs.
Basics of SLURM Jobs
The Simple Linux Utility for Resource Management (SLURM) is a system providing job scheduling and job management on compute clusters. With SLURM, a user requests resources and submits a job to a queue. The system will then take jobs from queues, allocate the necessary nodes, and execute them.
Do NOT run large, long, multi-threaded, parallel, or CPU-intensive jobs on a front-end login host. All users share the front-end hosts, and running anything but the smallest test job will negatively impact everyone's ability to use Scholar. Always use SLURM to submit your work as a job.
Link to section 'Submitting a Job' of 'Basics of SLURM Jobs' Submitting a Job
The main steps to submitting a job are:
Follow the links below for information on these steps, and other basic information about jobs. A number of example SLURM jobs are also available.
Queues
Link to section 'Scholar Queue' of 'Queues' Scholar Queue
This is the default queue for submitting jobs on Scholar. The maximum walltime on scholar queue is 4 hours.
Link to section 'Long Queue' of 'Queues' Long Queue
If your job requires more than 4 hours to complete, you can submit it to the long queue. The maximum walltime is 3 days. There are only 5 nodes in this queue, so you may have to wait for some time to get access to a node.
Link to section 'GPU Queue' of 'Queues' GPU Queue
If your job needs access to an Nvidia GPU accelerator, then use the gpu queue. The maximum walltime is 4 hours.
Link to section 'Debug Queue' of 'Queues' Debug Queue
The debug queue allows you to quickly start small, short, interactive jobs in order to debug code, test programs, or test configurations. You are limited to one running job at a time in the queue, and you may run up to two compute nodes for 30 minutes. The expectation is that debug jobs should start within a couple of minutes, assuming all of its dedicated nodes are not taken by others.
Link to section 'List of Queues' of 'Queues' List of Queues
To see a list of all queues on Scholar that you may submit to, use the slist command
This lists each queue you can submit to, the number of nodes allocated to the queue, how many are available to run jobs, and the maximum walltime you may request. Options to the command will give more detailed information. This command can be used to get a general idea of how busy an individual queue is and how long you may have to wait for your job to start.
Job Submission Script
To submit work to a SLURM queue, you must first create a job submission file. This job submission file is essentially a simple shell script. It will set any required environment variables, load any necessary modules, create or modify files and directories, and run any applications that you need:
#!/bin/bash
# FILENAME: myjobsubmissionfile
# Loads Matlab and sets the application up
module load matlab
# Change to the directory from which you originally submitted this job.
cd $SLURM_SUBMIT_DIR
# Runs a Matlab script named 'myscript'
matlab -nodisplay -singleCompThread -r myscript
Once your script is prepared, you are ready to submit your job.
Link to section 'Job Script Environment Variables' of 'Job Submission Script' Job Script Environment Variables
Name | Description |
---|---|
SLURM_SUBMIT_DIR | Absolute path of the current working directory when you submitted this job |
SLURM_JOBID | Job ID number assigned to this job by the batch system |
SLURM_JOB_NAME | Job name supplied by the user |
SLURM_JOB_NODELIST | Names of nodes assigned to this job |
SLURM_CLUSTER_NAME | Name of the cluster executing the job |
SLURM_SUBMIT_HOST | Hostname of the system where you submitted this job |
SLURM_JOB_PARTITION | Name of the original queue to which you submitted this job |
Submitting a Job
Once you have a job submission file, you may submit this script to SLURM using the sbatch
command. SLURM will find, or wait for, available resources matching your request and run your job there.
To submit your job to one compute node:
$ sbatch --nodes=1 myjobsubmissionfile
Slurm uses the word 'Account' and the option '-A' to specify different batch queues. To submit your job to a specific queue:
$ sbatch --nodes=1 -A scholar myjobsubmissionfile
By default, each job receives 30 minutes of wall time, or clock time. If you know that your job will not need more than a certain amount of time to run, request less than the maximum wall time, as this may allow your job to run sooner. To request the 1 hour and 30 minutes of wall time:
$ sbatch -t 1:30:00 --nodes=1 -A scholar myjobsubmissionfile
The --nodes
value indicates how many compute nodes you would like for your job.
Each compute node in Scholar has 20 processor cores.
In some cases, you may want to request multiple nodes. To utilize multiple nodes, you will need to have a program or code that is specifically programmed to use multiple nodes such as with MPI. Simply requesting more nodes will not make your work go faster. Your code must support this ability.
To request 2 compute nodes:
$ sbatch --nodes=2 myjobsubmissionfile
By default, jobs on Scholar will share nodes with other jobs.
To submit a job using 1 compute node with 4 tasks, each using the default 1 core and 1 GPU per node:
$ sbatch --nodes=1 --ntasks=4 --gpus-per-node=1 myjobsubmissionfile
If more convenient, you may also specify any command line options to sbatch
from within your job submission file, using a special form of comment:
#!/bin/sh -l
# FILENAME: myjobsubmissionfile
#SBATCH -A myqueuename
#SBATCH --nodes=1
#SBATCH --time=1:30:00
#SBATCH --job-name myjobname
# Print the hostname of the compute node on which this job is running.
/bin/hostname
If an option is present in both your job submission file and on the command line, the option on the command line will take precedence.
After you submit your job with SBATCH
, it may wait in queue for minutes, hours, or even weeks. How long it takes for a job to start depends on the specific queue, the resources and time requested, and other jobs already waiting in that queue requested as well. It is impossible to say for sure when any given job will start. For best results, request no more resources than your job requires.
Once your job is submitted, you can monitor the job status, wait for the job to complete, and check the job output.
Job Dependencies
Dependencies are an automated way of holding and releasing jobs. Jobs with a dependency are held until the condition is satisfied. Once the condition is satisfied jobs only then become eligible to run and must still queue as normal.
Job dependencies may be configured to ensure jobs start in a specified order. Jobs can be configured to run after other job state changes, such as when the job starts or the job ends.
These examples illustrate setting dependencies in several ways. Typically dependencies are set by capturing and using the job ID from the last job submitted.
To run a job after job myjobid has started:
sbatch --dependency=after:myjobid myjobsubmissionfile
To run a job after job myjobid ends without error:
sbatch --dependency=afterok:myjobid myjobsubmissionfile
To run a job after job myjobid ends with errors:
sbatch --dependency=afternotok:myjobid myjobsubmissionfile
To run a job after job myjobid ends with or without errors:
sbatch --dependency=afterany:myjobid myjobsubmissionfile
To set more complex dependencies on multiple jobs and conditions:
sbatch --dependency=after:myjobid1:myjobid2:myjobid3,afterok:myjobid4 myjobsubmissionfile
Holding a Job
Sometimes you may want to submit a job but not have it run just yet. You may be wanting to allow lab mates to cut in front of you in the queue - so hold the job until their jobs have started, and then release yours.
To place a hold on a job before it starts running, use the scontrol hold job command:
$ scontrol hold job myjobid
Once a job has started running it can not be placed on hold.
To release a hold on a job, use the scontrol release job command:
$ scontrol release job myjobid
You find the job ID using the squeue command as explained in the SLURM Job Status section.
Checking Job Status
Once a job is submitted there are several commands you can use to monitor the progress of the job.
To see your jobs, use the squeue -u command and specify your username:
(Remember, in our SLURM environment a queue is referred to as an 'Account')
squeue -u myusername
JOBID ACCOUNT NAME USER ST TIME NODES NODELIST(REASON)
182792 scholar job1 myusername R 20:19 1 scholar-a000
185841 scholar job2 myusername R 20:19 1 scholar-a001
185844 scholar job3 myusername R 20:18 1 scholar-a002
185847 scholar job4 myusername R 20:18 1 scholar-a003
To retrieve useful information about your queued or running job, use the scontrol show job command with your job's ID number. The output should look similar to the following:
scontrol show job 3519
JobId=3519 JobName=t.sub
UserId=myusername GroupId=mygroup MCS_label=N/A
Priority=3 Nice=0 Account=(null) QOS=(null)
JobState=PENDING Reason=BeginTime Dependency=(null)
Requeue=1 Restarts=0 BatchFlag=1 Reboot=0 ExitCode=0:0
RunTime=00:00:00 TimeLimit=7-00:00:00 TimeMin=N/A
SubmitTime=2019-08-29T16:56:52 EligibleTime=2019-08-29T23:30:00
AccrueTime=Unknown
StartTime=2019-08-29T23:30:00 EndTime=2019-09-05T23:30:00 Deadline=N/A
PreemptTime=None SuspendTime=None SecsPreSuspend=0
LastSchedEval=2019-08-29T16:56:52
Partition=workq AllocNode:Sid=mack-fe00:54476
ReqNodeList=(null) ExcNodeList=(null)
NodeList=(null)
NumNodes=1 NumCPUs=2 NumTasks=2 CPUs/Task=1 ReqB:S:C:T=0:0:*:*
TRES=cpu=2,node=1,billing=2
Socks/Node=* NtasksPerN:B:S:C=0:0:*:* CoreSpec=*
MinCPUsNode=1 MinMemoryNode=0 MinTmpDiskNode=0
Features=(null) DelayBoot=00:00:00
OverSubscribe=OK Contiguous=0 Licenses=(null) Network=(null)
Command=/home/myusername/jobdir/myjobfile.sub
WorkDir=/home/myusername/jobdir
StdErr=/home/myusername/jobdir/slurm-3519.out
StdIn=/dev/null
StdOut=/home/myusername/jobdir/slurm-3519.out
Power=
There are several useful bits of information in this output.
JobState
lets you know if the job is Pending, Running, Completed, or Held.RunTime and TimeLimit
will show how long the job has run and its maximum time.SubmitTime
is when the job was submitted to the cluster.NumNodes
,NumCPUs
,NumTasks
andCPUs/Task
are the number of Nodes, CPUs, Tasks, and CPUs per Task are shown.WorkDir
is the job's working directory.StdOut
andStderr
are the locations of stdout and stderr of the job, respectively.Reason
will show why aPENDING
job isn't running. The above error says that it has been requested to start at a specific, later time.
Checking Job Output
Once a job is submitted, and has started, it will write its standard output and standard error to files that you can read.
SLURM catches output written to standard output and standard error - what would be printed to your screen if you ran your program interactively. Unless you specfied otherwise, SLURM will put the output in the directory where you submitted the job in a file named slurm-
followed by the job id
, with the extension out
. For example slurm-3509.out
. Note that both stdout and stderr will be written into the same file, unless you specify otherwise.
If your program writes its own output files, those files will be created as defined by the program. This may be in the directory where the program was run, or may be defined in a configuration or input file. You will need to check the documentation for your program for more details.
Link to section 'Redirecting Job Output' of 'Checking Job Output' Redirecting Job Output
It is possible to redirect job output to somewhere other than the default location with the --error
and --output
directives:
#!/bin/bash
#SBATCH --output=/home/myusername/joboutput/myjob.out
#SBATCH --error=/home/myusername/joboutput/myjob.out
# This job prints "Hello World" to output and exits
echo "Hello World"
Canceling a Job
To stop a job before it finishes or remove it from a queue, use the scancel command:
scancel myjobid
You find the job ID using the squeue command as explained in the SLURM Job Status section.
PBS to Slurm
This is a reference for the most common command, environment variables, and job specification options used by the workload management systems and their equivalents.
Quick Guide
This table lists the most common command, environment variables, and job specification options used by the workload management systems and their equivalents (adapted from http://www.schedmd.com/slurmdocs/rosetta.html).
User Commands | PBS/Torque | Slurm |
---|---|---|
Job submission | qsub [script_file] |
sbatch [script_file] |
Interactive Job | qsub -I |
sinteractive |
Job deletion | qdel [job_id] |
scancel [job_id] |
Job status (by job) | qstat [job_id] |
squeue [-j job_id] |
Job status (by user) | qstat -u [user_name] |
squeue [-u user_name] |
Job hold | qhold [job_id] |
scontrol hold [job_id] |
Job release | qrls [job_id] |
scontrol release [job_id] |
Queue info | qstat -Q |
squeue |
Queue access | qlist |
slist |
Node list | pbsnodes -l |
sinfo -N scontrol show nodes |
Cluster status | qstat -a |
sinfo |
GUI | xpbsmon |
sview |
Environment | PBS/Torque | Slurm |
Job ID | $PBS_JOBID |
$SLURM_JOB_ID |
Job Name | $PBS_JOBNAME |
$SLURM_JOB_NAME |
Job Queue/Account | $PBS_QUEUE |
$SLURM_JOB_ACCOUNT |
Submit Directory | $PBS_O_WORKDIR |
$SLURM_SUBMIT_DIR |
Submit Host | $PBS_O_HOST |
$SLURM_SUBMIT_HOST |
Number of nodes | $PBS_NUM_NODES |
$SLURM_JOB_NUM_NODES |
Number of Tasks | $PBS_NP |
$SLURM_NTASKS |
Number of Tasks Per Node | $PBS_NUM_PPN |
$SLURM_NTASKS_PER_NODE |
Node List (Compact) | n/a | $SLURM_JOB_NODELIST |
Node List (One Core Per Line) | LIST=$(cat $PBS_NODEFILE) |
LIST=$(srun hostname) |
Job Array Index | $PBS_ARRAYID |
$SLURM_ARRAY_TASK_ID |
Job Specification | PBS/Torque | Slurm |
Script directive | #PBS |
#SBATCH |
Queue | -q [queue] |
-A [queue] |
Node Count | -l nodes=[count] |
-N [min[-max]] |
CPU Count | -l ppn=[count] |
-n [count] Note: total, not per node |
Wall Clock Limit | -l walltime=[hh:mm:ss] |
-t [min] OR-t [hh:mm:ss] OR-t [days-hh:mm:ss] |
Standard Output FIle | -o [file_name] |
-o [file_name] |
Standard Error File | -e [file_name] |
-e [file_name] |
Combine stdout/err | -j oe (both to stdout) OR-j eo (both to stderr) |
(use -o without -e) |
Copy Environment | -V |
--export=[ALL | NONE | variables] Note: default behavior is ALL |
Copy Specific Environment Variable | -v myvar=somevalue |
--export=NONE,myvar=somevalue OR--export=ALL,myvar=somevalue |
Event Notification | -m abe |
--mail-type=[events] |
Email Address | -M [address] |
--mail-user=[address] |
Job Name | -N [name] |
--job-name=[name] |
Job Restart | -r [y|n] |
--requeue OR--no-requeue |
Working Directory | --workdir=[dir_name] |
|
Resource Sharing | -l naccesspolicy=singlejob |
--exclusive OR--shared |
Memory Size | -l mem=[MB] |
--mem=[mem][M|G|T] OR--mem-per-cpu=[mem][M|G|T] |
Account to charge | -A [account] |
-A [account] |
Tasks Per Node | -l ppn=[count] |
--tasks-per-node=[count] |
CPUs Per Task | --cpus-per-task=[count] |
|
Job Dependency | -W depend=[state:job_id] |
--depend=[state:job_id] |
Job Arrays | -t [array_spec] |
--array=[array_spec] |
Generic Resources | -l other=[resource_spec] |
--gres=[resource_spec] |
Licenses | --licenses=[license_spec] |
|
Begin Time | -A "y-m-d h:m:s" |
--begin=y-m-d[Th:m[:s]] |
See the official Slurm Documentation for further details.
Notable Differences
-
Separate commands for Batch and Interactive jobs
Unlike PBS, in Slurm interactive jobs and batch jobs are launched with completely distinct commands.
Usesbatch [allocation request options] script
to submit a job to the batch scheduler, andsinteractive [allocation request options]
to launch an interactive job. sinteractive accepts most of the same allocation request options as sbatch does. -
No need for
cd $PBS_O_WORKDIR
In Slurm your batch job starts to run in the directory from which you submitted the script whereas in PBS/Torque you need to explicitly move back to that directory with
cd $PBS_O_WORKDIR
. -
No need to manually export environment
The environment variables that are defined in your shell session at the time that you submit the script are exported into your batch job, whereas in PBS/Torque you need to use the
-V
flag to export your environment. -
Location of output files
The output and error files are created in their final location immediately that the job begins or an error is generated, whereas in PBS/Torque temporary files are created that are only moved to the final location at the end of the job. Therefore in Slurm you can examine the output and error files from your job during its execution.
See the official Slurm Documentation for further details.
Example Jobs
A number of example jobs are available for you to look over and adapt to your own needs. The first few are generic examples, and latter ones go into specifics for particular software packages.
Generic SLURM Jobs
The following examples demonstrate the basics of SLURM jobs, and are designed to cover common job request scenarios. These example jobs will need to be modified to run your application or code.
Simple Job
Every SLURM job consists of a job submission file. A job submission file contains a list of commands that run your program and a set of resource (nodes, walltime, queue) requests. The resource requests can appear in the job submission file or can be specified at submit-time as shown below.
This simple example submits the job submission file hello.sub to the scholar queue on Scholar and requests a single node:
#!/bin/bash
# FILENAME: hello.sub
# Show this ran on a compute node by running the hostname command.
hostname
echo "Hello World"
sbatch -A scholar --nodes=1 --ntasks=1 --cpus-per-task=1 --time=00:01:00 hello.sub
Submitted batch job 3521
For a real job you would replace echo "Hello World"
with a command, or sequence of commands, that run your program.
After your job finishes running, the ls command will show a new file in your directory, the .out file:
ls -l
hello.sub
slurm-3521.out
The file slurm-3521.out contains the output and errors your program would have written to the screen if you had typed its commands at a command prompt:
cat slurm-3521.out
scholar-a001.rcac.purdue.edu
Hello World
You should see the hostname of the compute node your job was executed on. Following should be the "Hello World" statement.
Multiple Node
In some cases, you may want to request multiple nodes. To utilize multiple nodes, you will need to have a program or code that is specifically programmed to use multiple nodes such as with MPI. Simply requesting more nodes will not make your work go faster. Your code must support this ability.
This example shows a request for multiple compute nodes. The job submission file contains a single command to show the names of the compute nodes allocated:
# FILENAME: myjobsubmissionfile.sub
#!/bin/bash
echo "$SLURM_JOB_NODELIST"
sbatch --nodes=2 --ntasks=40 --time=00:10:00 -A scholar myjobsubmissionfile.sub
Compute nodes allocated:
scholar-a[014-015]
The above example will allocate the total of 40 CPU cores across 2 nodes. Note that if your multi-node job requests fewer than each node's full 20 cores per node, by default Slurm provides no guarantee with respect to how this total is distributed between assigned nodes (i.e. the cores may not necessarily be split evenly). If you need specific arrangements of your tasks and cores, you can use --cpus-per-task= and/or --ntasks-per-node= flags. See Slurm documentation or man sbatch for more options.
Directives
So far these examples have shown submitting jobs with the resource requests on the sbatch
command line such as:
sbatch -A scholar --nodes=1 --time=00:01:00 hello.sub
The resource requests can also be put into job submission file itself. Documenting the resource requests in the job submission is desirable because the job can be easily reproduced later. Details left in your command history are quickly lost. Arguments are specified with the #SBATCH
syntax:
#!/bin/bash
# FILENAME: hello.sub
#SBATCH -A scholar
#SBATCH --nodes=1 --time=00:01:00
# Show this ran on a compute node by running the hostname command.
hostname
echo "Hello World"
The #SBATCH
directives must appear at the top of your submission file. SLURM will stop parsing directives as soon as it encounters a line that does not start with '#'. If you insert a directive in the middle of your script, it will be ignored.
This job can be then submitted with:
sbatch hello.sub
Specific Types of Nodes
SLURM allows running a job on specific types of compute nodes to accommodate special hardware requirements (e.g. a certain CPU or GPU type, etc.)
Cluster nodes have a set of descriptive features assigned to them, and users can specify which of these features are required by their job by using the constraint option at submission time. Only nodes having features matching the job constraints will be used to satisfy the request.
Example: a job requires a compute node in an "A" sub-cluster:
sbatch --nodes=1 --ntasks=20 --constraint=A myjobsubmissionfile.sub
Compute node allocated:
scholar-a003
Feature constraints can be used for both batch and interactive jobs, as well as for individual job steps inside a job. Multiple constraints can be specified with a predefined syntax to achieve complex request logic (see detailed description of the '--constraint' option in man sbatch
or online Slurm documentation).
Refer to Detailed Hardware Specification section for list of available sub-cluster labels, their respective per-node memory sizes and other hardware details. You could also use sfeatures
command to list available constraint feature names for different node types.
Interactive Jobs
Interactive jobs are run on compute nodes, while giving you a shell to interact with. They give you the ability to type commands or use a graphical interface in the same way as if you were on a front-end login host.
To submit an interactive job, use sinteractive to run a login shell on allocated resources.
sinteractive accepts most of the same resource requests as sbatch, so to request a login shell on the cpu account while allocating 2 nodes and 20 total cores, you might do:
sinteractive -A cpu -N2 -n40
To quit your interactive job:
exit
or Ctrl-D
The above example will allocate the total of 40 CPU cores across 2 nodes. Note that if your multi-node job requests fewer than each node's full 20 cores per node, by default Slurm provides no guarantee with respect to how this total is distributed between assigned nodes (i.e. the cores may not necessarily be split evenly). If you need specific arrangements of your tasks and cores, you can use --cpus-per-task= and/or --ntasks-per-node= flags. See Slurm documentation or man salloc for more options.
Serial Jobs
This shows how to submit one of the serial programs compiled in the section Compiling Serial Programs.
Create a job submission file:
#!/bin/bash
# FILENAME: serial_hello.sub
./serial_hello
Submit the job:
sbatch --nodes=1 --ntasks=1 --time=00:01:00 serial_hello.sub
After the job completes, view results in the output file:
cat slurm-myjobid.out
Runhost:scholar-a009.rcac.purdue.edu
hello, world
If the job failed to run, then view error messages in the file slurm-myjobid.out.
OpenMP
A shared-memory job is a single process that takes advantage of a multi-core processor and its shared memory to achieve parallelization.
This example shows how to submit an OpenMP program compiled in the section Compiling OpenMP Programs.
When running OpenMP programs, all threads must be on the same compute node to take advantage of shared memory. The threads cannot communicate between nodes.
To run an OpenMP program, set the environment variable OMP_NUM_THREADS to the desired number of threads:
In csh:
setenv OMP_NUM_THREADS 20
In bash:
export OMP_NUM_THREADS=20
This should almost always be equal to the number of cores on a compute node. You may want to set to another appropriate value if you are running several processes in parallel in a single job or node.
Create a job submissionfile:
#!/bin/bash
# FILENAME: omp_hello.sub
#SBATCH --nodes=1
#SBATCH --ntasks=20
#SBATCH --time=00:01:00
export OMP_NUM_THREADS=20
./omp_hello
Submit the job:
sbatch omp_hello.sub
View the results from one of the sample OpenMP programs about task parallelism:
cat omp_hello.sub.omyjobid
SERIAL REGION: Runhost:scholar-a003.rcac.purdue.edu Thread:0 of 1 thread hello, world
PARALLEL REGION: Runhost:scholar-a003.rcac.purdue.edu Thread:0 of 20 threads hello, world
PARALLEL REGION: Runhost:scholar-a003.rcac.purdue.edu Thread:1 of 20 threads hello, world
...
If the job failed to run, then view error messages in the file slurm-myjobid.out.
If an OpenMP program uses a lot of memory and 20 threads use all of the memory of the compute node, use fewer processor cores (OpenMP threads) on that compute node.
MPI
An MPI job is a set of processes that take advantage of multiple compute nodes by communicating with each other. OpenMPI and Intel MPI (IMPI) are implementations of the MPI standard.
This section shows how to submit one of the MPI programs compiled in the section Compiling MPI Programs.
Use module load to set up the paths to access these libraries. Use module avail to see all MPI packages installed on Scholar.
Create a job submission file:
#!/bin/bash
# FILENAME: mpi_hello.sub
#SBATCH --nodes=2
#SBATCH --ntasks-per-node=20
#SBATCH --time=00:01:00
#SBATCH -A scholar
srun -n 40 ./mpi_hello
SLURM can run an MPI program with the srun command. The number of processes is requested with the -n option. If you do not specify the -n option, it will default to the total number of processor cores you request from SLURM.
If the code is built with OpenMPI, it can be run with a simple srun -n command. If it is built with Intel IMPI, then you also need to add the --mpi=pmi2 option: srun --mpi=pmi2 -n 40 ./mpi_hello in this example.
Submit the MPI job:
sbatch ./mpi_hello.sub
View results in the output file:
cat slurm-myjobid.out
Runhost:scholar-a010.rcac.purdue.edu Rank:0 of 40 ranks hello, world
Runhost:scholar-a010.rcac.purdue.edu Rank:1 of 40 ranks hello, world
...
Runhost:scholar-a011.rcac.purdue.edu Rank:20 of 40 ranks hello, world
Runhost:scholar-a011.rcac.purdue.edu Rank:21 of 40 ranks hello, world
...
If the job failed to run, then view error messages in the output file.
If an MPI job uses a lot of memory and 20 MPI ranks per compute node use all of the memory of the compute nodes, request more compute nodes, while keeping the total number of MPI ranks unchanged.
Submit the job with double the number of compute nodes and modify the resource request to halve the number of MPI ranks per compute node.
#!/bin/bash
# FILENAME: mpi_hello.sub
#SBATCH --nodes=4
#SBATCH --ntasks-per-node=10
#SBATCH -t 00:01:00
#SBATCH -A scholar
srun -n 40 ./mpi_hello
sbatch ./mpi_hello.sub
View results in the output file:
cat slurm-myjobid.out
Runhost:scholar-a10.rcac.purdue.edu Rank:0 of 40 ranks hello, world
Runhost:scholar-a010.rcac.purdue.edu Rank:1 of 40 ranks hello, world
...
Runhost:scholar-a011.rcac.purdue.edu Rank:10 of 40 ranks hello, world
...
Runhost:scholar-a012.rcac.purdue.edu Rank:20 of 40 ranks hello, world
...
Runhost:scholar-a013.rcac.purdue.edu Rank:30 of 40 ranks hello, world
...
Notes
- Use slist to determine which queues (
--account
or-A
option) are available to you. The name of the queue which is available to everyone on Scholar is "scholar". - Invoking an MPI program on Scholar with ./program is typically wrong, since this will use only one MPI process and defeat the purpose of using MPI. Unless that is what you want (rarely the case), you should use srun or mpiexec to invoke an MPI program.
- In general, the exact order in which MPI ranks output similar write requests to an output file is random.
GPU
The Scholar cluster nodes contain NVIDIA GPUs that support CUDA and OpenCL. See the detailed hardware overview for the specifics on the GPUs in Scholar.
This section illustrates how to use SLURM to submit a simple GPU program.
Suppose that you named your executable file gpu_hello from the sample code gpu_hello.cu
(see the section on compiling NVIDIA GPU codes). Prepare a job submission file with an appropriate name, here named gpu_hello.sub:
#!/bin/bash
# FILENAME: gpu_hello.sub
module load cuda
host=`hostname -s`
echo $CUDA_VISIBLE_DEVICES
# Run on the first available GPU
./gpu_hello 0
Submit the job:
sbatch -A gpu --nodes=1 --gres=gpu:1 -t 00:01:00 gpu_hello.sub
Requesting a GPU from the scheduler is required.
You can specify total number of GPUs, or number of GPUs per node, or even number of GPUs per task:
sbatch -A gpu --nodes=1 --gres=gpu:1 -t 00:01:00 gpu_hello.sub
sbatch -A gpu --nodes=1 --gpus-per-node=1 -t 00:01:00 gpu_hello.sub
sbatch -A gpu --nodes=1 --gpus-per-task=1 -t 00:01:00 gpu_hello.sub
After job completion, view the new output file in your directory:
ls -l
gpu_hello
gpu_hello.cu
gpu_hello.sub
slurm-myjobid.out
View results in the file for all standard output, slurm-myjobid.out
0
hello, world
If the job failed to run, then view error messages in the file slurm-myjobid.out.
To use multiple GPUs in your job, simply specify a larger value to the GPU specification parameter. However, be aware of the number of GPUs installed on the node(s) you may be requesting. The scheduler can not allocate more GPUs than physically exist. See detailed hardware overview and output of sfeatures command for the specifics on the GPUs in Scholar.
Link to section 'Collecting System Resource Utilization Data' of 'Monitoring Resources' Collecting System Resource Utilization Data
Knowing the precise resource utilization an application had during a job, such as CPU load or memory, can be incredibly useful. This is especially the case when the application isn't performing as expected.
One approach is to run a program like htop
during an interactive job and keep an eye on system resources. You can get precise time-series data from nodes associated with your job using XDmod as well, online. But these methods don't gather telemetry in an automated fashion, nor do they give you control over the resolution or format of the data.
As a matter of course, a robust implementation of some HPC workload would include resource utilization data as a diagnostic tool in the event of some failure.
The monitor
utility is a simple command line system resource monitoring tool for gathering such telemetry and is available as a module.
module load monitor
Complete documentation is available online at resource-monitor.readthedocs.io. A full manual page is also available for reference, man monitor
.
In the context of a SLURM job you will need to put this monitoring task in the background to allow the rest of your job script to proceed. Be sure to interrupt these tasks at the end of your job.
#!/bin/bash
# FILENAME: monitored_job.sh
module load monitor
# track per-code CPU load
monitor cpu percent --all-cores >cpu-percent.log &
CPU_PID=$!
# track memory usage
monitor cpu memory >cpu-memory.log &
MEM_PID=$!
# your code here
# shut down the resource monitors
kill -s INT $CPU_PID $MEM_PID
A particularly elegant solution would be to include such tools in your prologue script and have the tear down in your epilogue script.
For large distributed jobs spread across multiple nodes, mpiexec
can be used to gather telemetry from all nodes in the job. The hostname is included in each line of output so that data can be grouped as such. A concise way of constructing the needed list of hostnames in SLURM is to simply use srun hostname | sort -u
.
#!/bin/bash
# FILENAME: monitored_job.sh
module load monitor
# track all CPUs (one monitor per host)
mpiexec -machinefile <(srun hostname | sort -u) \
monitor cpu percent --all-cores >cpu-percent.log &
CPU_PID=$!
# track memory on all hosts (one monitor per host)
mpiexec -machinefile <(srun hostname | sort -u) \
monitor cpu memory >cpu-memory.log &
MEM_PID=$!
# your code here
# shut down the resource monitors
kill -s INT $CPU_PID $MEM_PID
To get resource data in a more readily computable format, the monitor
program can be told to output in CSV format with the --csv
flag.
monitor cpu memory --csv >cpu-memory.csv
For a distributed job you will need to suppress the header lines otherwise one will be created by each host.
monitor cpu memory --csv | head -1 >cpu-memory.csv
mpiexec -machinefile <(srun hostname | sort -u) \
monitor cpu memory --csv --no-header >>cpu-memory.csv
Specific Applications
The following examples demonstrate job submission files for some common real-world applications. See the Generic SLURM Examples section for more examples on job submissions that can be adapted for use.
Gaussian
Gaussian is a computational chemistry software package which works on electronic structure. This section illustrates how to submit a small Gaussian job to a Slurm queue. This Gaussian example runs the Fletcher-Powell multivariable optimization.
Prepare a Gaussian input file with an appropriate filename, here named myjob.com. The final blank line is necessary:
#P TEST OPT=FP STO-3G OPTCYC=2
STO-3G FLETCHER-POWELL OPTIMIZATION OF WATER
0 1
O
H 1 R
H 1 R 2 A
R 0.96
A 104.
To submit this job, load Gaussian then run the provided script, named subg16. This job uses one compute node with 20 processor cores:
module load gaussian16
subg16 myjob -N 1 -n 20
View job status:
squeue -u myusername
View results in the file for Gaussian output, here named myjob.log. Only the first and last few lines appear here:
Entering Gaussian System, Link 0=/apps/cent7/gaussian/g16-A.03/g16-haswell/g16/g16
Initial command:
/apps/cent7/gaussian/g16-A.03/g16-haswell/g16/l1.exe /scratch/scholar/myusername/gaussian/Gau-7781.inp -scrdir=/scratch/scholar/myusername/gaussian/
Entering Link 1 = /apps/cent7/gaussian/g16-A.03/g16-haswell/g16/l1.exe PID= 7782.
Copyright (c) 1988,1990,1992,1993,1995,1998,2003,2009,2016,
Gaussian, Inc. All Rights Reserved.
.
.
.
Job cpu time: 0 days 0 hours 3 minutes 28.2 seconds.
Elapsed time: 0 days 0 hours 0 minutes 12.9 seconds.
File lengths (MBytes): RWF= 17 Int= 0 D2E= 0 Chk= 2 Scr= 2
Normal termination of Gaussian 16 at Tue May 1 17:12:00 2018.
real 13.85
user 202.05
sys 6.12
Machine:
scholar-a012.rcac.purdue.edu
scholar-a012.rcac.purdue.edu
scholar-a012.rcac.purdue.edu
scholar-a012.rcac.purdue.edu
scholar-a012.rcac.purdue.edu
scholar-a012.rcac.purdue.edu
scholar-a012.rcac.purdue.edu
scholar-a012.rcac.purdue.edu
Link to section 'Examples of Gaussian SLURM Job Submissions' of 'Gaussian' Examples of Gaussian SLURM Job Submissions
Submit job using 20 processor cores on a single node:
subg16 myjob -N 1 -n 20 -t 200:00:00 -A myqueuename
Submit job using 20 processor cores on each of 2 nodes:
subg16 myjob -N 2 --ntasks-per-node=20 -t 200:00:00 -A myqueuename
To submit a bash job, a submit script sample looks like:
#!/bin/bash
#SBATCH -A myqueuename # Queue name(use 'slist' command to find queues' name)
#SBATCH --nodes=1 # Total # of nodes
#SBATCH --ntasks=64 # Total # of MPI tasks
#SBATCH --time=1:00:00 # Total run time limit (hh:mm:ss)
#SBATCH -J myjobname # Job name
#SBATCH -o myjob.o%j # Name of stdout output file
#SBATCH -e myjob.e%j # Name of stderr error file
module load gaussian16
g16 < myjob.com
For more information about Gaussian:
Machine Learning
We support several common machine learning (ML) frameworks on the community clusters through pre-installed modules. The collection of these pre-installed ML modules is referred to as ml-toolkit throughout this documentation. Currently, the following libraries are included in ML-Toolkit.
caffe cntk gym keras
mxnet opencv pytorch
tensorflow tflearn theano
Note that managing dependencies with ML applications can be non-trivial, therefore, we recommend users start by using ml-toolkit. If a custom installation is required after trying ml-toolkit, make sure to read documentation carefully.
ML-Toolkit
A set of pre-installed popular machine learning (ML) libraries, called ML-Toolkit is maintained on Scholar. These are Anaconda/Python-based distributions of the respective libraries. Currently, applications are supported for Python 2 and 3. Detailed instructions for searching and using the installed ML applications are presented below.
Link to section 'Instructions for using ML-Toolkit Modules' of 'ML-Toolkit' Instructions for using ML-Toolkit Modules
Link to section 'Find and Use Installed ML Packages' of 'ML-Toolkit' Find and Use Installed ML Packages
To search or load a machine learning application, you must first load one of the learning modules. The learning module loads the prerequisites (such as anaconda and cudnn) and makes ML applications visible to the user.
Step 1. Find and load a preferred learning module. Several learning modules may be available, corresponding to a specific Python version and whether the ML applications have GPU support or not. Running module load learning without specifying a version will load the version with the most recent python version. To see all available modules, run module spider learning then load the desired module.
Step 2. Find and load the desired machine learning libraries
ML packages are installed under the common application name ml-toolkit-X, where X can be cpu or gpu.
You can use the module spider ml-toolkit command to see all options and versions of each library.
Load the desired modules using the module load command. Note that both CPU and GPU options may exist for many libraries, so be sure to load the correct version. For example, if you wanted to load the most recent version of PyTorch for CPU, you would run module load ml-toolkit-cpu/pytorch
caffe cntk gym keras mxnet
opencv pytorch tensorflow tflearn theano
Step 3. You can list which ML applications are loaded in your environment using the command module list
Link to section 'Verify application import' of 'ML-Toolkit' Verify application import
Step 4. The next step is to check that you can actually use the desired ML application. You can do this by running the import command in Python. The example below tests if PyTorch has been loaded correctly.
python -c "import torch; print(torch.__version__)"
If the import operation succeeded, then you can run your own ML code. Some ML applications (such as tensorflow) print diagnostic warnings while loading -- this is the expected behavior.
If the import fails with an error, please see the troubleshooting information below.
Step 5. To load a different set of applications, unload the previously loaded applications and load the new desired applications. The example below loads Tensorflow and Keras instead of PyTorch and OpenCV.
module unload ml-toolkit-cpu/opencv
module unload ml-toolkit-cpu/pytorch
module load ml-toolkit-cpu/tensorflow
module load ml-toolkit-cpu/keras
Link to section 'Troubleshooting' of 'ML-Toolkit' Troubleshooting
ML applications depend on a wide range of Python packages and mixing multiple versions of these packages can lead to error. The following guidelines will assist you in identifying the cause of the problem.
- Check that you are using the correct version of Python with the command python --version. This should match the Python version in the loaded anaconda module.
- Start from a clean environment. Either start a new terminal session or unload all the modules using module purge. Then load the desired modules following Steps 1-2.
- Verify that PYTHONPATH does not point to undesired packages. Run the following command to print PYTHONPATH: echo $PYTHONPATH. Make sure that your Python environment is clean. Watch out for any locally installed packages that might conflict.
- If you don't see GPU devices in your code, make sure that you are using the ml-toolkit-gpu/ modules and not using their cpu versions.
- ML applications often have dependency on specific versions of Cuda and CuDNN libraries. Make sure that you have loaded the required versions using the command: module list
- Note that Caffe has a conflicting version of PyQt5. So, if you want to use Spyder (or any GUI application that uses PyQt), then you should unload the caffe module.
- Use Google search to your advantage. Copy the error message in Google and check probable causes.
More examples showing how to use ml-toolkit modules in a batch job are presented in ML Batch Jobs guide.
Link to section 'Installation of Custom ML Libraries' of 'Custom ML Packages' Installation of Custom ML Libraries
While we try to include as many common ML frameworks and versions as we can in ML-Toolkit, we recognize that there are also situations in which a custom installation may be preferable. We recommend using conda-env-mod to install and manage Python packages. Please follow the steps carefully, otherwise you may end up with a faulty installation. The example below shows how to install TensorFlow in your home directory.
Link to section 'Install' of 'Custom ML Packages' Install
Step 1: Unload all modules and start with a clean environment.
module purge
Step 2: Load the anaconda module with desired Python version.
module load anaconda
Step 2A: If the ML application requires Cuda and CuDNN, load the appropriate modules. Be sure to check that the versions you load are compatible with the desired ML package.
module load cuda
module load cudnn
Many machine-learning packages (including PyTorch and TensorFlow) now provide installation pathways that include the full cudatoolkit within the environment, making it unnecessary to load these modules.
Step 3: Create a custom anaconda environment. Make sure the python version matches the Python version in the anaconda module.
conda-env-mod create -n env_name_here
Step 4: Activate the anaconda environment by loading the modules displayed at the end of step 3.
module load use.own
module load conda-env/env_name_here-py3.6.4
Step 5: Now install the desired ML application. You can install multiple Python packages at this step using either conda or pip.
pip install --ignore-installed tensorflow==2.6
If the installation succeeded, you can now proceed to testing and using the installed application. You must load the environment you created as well as any supporting modules (e.g., anaconda) whenever you want to use this installation. If your installation did not succeed, please refer to the troubleshooting section below as well as documentation for the desired package you are installing.
Note that loading the modules generated by conda-env-mod has different behavior than conda create env_name_here followed by source activate env_name_here. After running source activate, you may not be able to access any Python packages in anaconda or ml-toolkit modules. Therefore, using conda-env-mod is the preferred way of using your custom installations.
Link to section 'Testing the Installation' of 'Custom ML Packages' Testing the Installation
-
Verify the installation by using a simple import statement, like that listed below for TensorFlow:
python -c "import tensorflow as tf; print(tf.__version__);"
Note that a successful import of TensorFlow will print a variety of system and hardware information. This is expected.
If importing the package leads to errors, be sure to verify that all dependencies for the package have been managed, and the correct versions installed. Dependency issues between python packages are the most common cause for errors. For example, in TF, conflicts with the h5py or numpy versions are common, but upgrading those packages typically solves the problem. Managing dependencies for ML libraries can be non-trivial.
-
Next, we can test using our installation of TensorFlow for a GPU run. For this we shall use the matrix multiplication example from Tensorflow documentation.
# filename: matrixmult.py import tensorflow as tf print("Num GPUs Available: ", len(tf.config.list_physical_devices('GPU'))) tf.debugging.set_log_device_placement(True) # Place tensors on the CPU with tf.device('/CPU:0'): a = tf.constant([[1.0, 2.0, 3.0], [4.0, 5.0, 6.0]]) b = tf.constant([[1.0, 2.0], [3.0, 4.0], [5.0, 6.0]]) # Run on the GPU c = tf.matmul(a, b) print(c)
-
Run the example
$ python matrixmult.py
-
This will produce an output like:
Num GPUs Available: 3 2022-07-25 10:33:23.358919: I tensorflow/core/platform/cpu_feature_guard.cc:193] This TensorFlow binary is optimized with oneAPI Deep Neural Network Library (oneDNN) to use the following CPU instructions in performance-critical operations: AVX2 AVX512F FMA To enable them in other operations, rebuild TensorFlow with the appropriate compiler flags. 2022-07-25 10:33:26.223459: I tensorflow/core/common_runtime/gpu/gpu_device.cc:1532] Created device /job:localhost/replica:0/task:0/device:GPU:0 with 22183 MB memory: -> device: 0, name: NVIDIA A30, pci bus id: 0000:3b:00.0, compute capability: 8.0 2022-07-25 10:33:26.225495: I tensorflow/core/common_runtime/gpu/gpu_device.cc:1532] Created device /job:localhost/replica:0/task:0/device:GPU:1 with 22183 MB memory: -> device: 1, name: NVIDIA A30, pci bus id: 0000:af:00.0, compute capability: 8.0 2022-07-25 10:33:26.228514: I tensorflow/core/common_runtime/gpu/gpu_device.cc:1532] Created device /job:localhost/replica:0/task:0/device:GPU:2 with 22183 MB memory: -> device: 2, name: NVIDIA A30, pci bus id: 0000:d8:00.0, compute capability: 8.0 2022-07-25 10:33:26.933709: I tensorflow/core/common_runtime/eager/execute.cc:1323] Executing op MatMul in device /job:localhost/replica:0/task:0/device:GPU:0 2022-07-25 10:33:28.181855: I tensorflow/stream_executor/cuda/cuda_blas.cc:1786] TensorFloat-32 will be used for the matrix multiplication. This will only be logged once. tf.Tensor( [[22. 28.] [49. 64.]], shape=(2, 2), dtype=float32)
-
For more details, please refer to Tensorflow User Guide.
Link to section 'Troubleshooting' of 'Custom ML Packages' Troubleshooting
In most situations, dependencies among Python modules lead to errors. If you cannot use a Python package after installing it, please follow the steps below to find a workaround.
- Unload all the modules.
module purge
- Clean up PYTHONPATH.
unset PYTHONPATH
- Next load the modules, e.g., anaconda and your custom environment.
module load anaconda module load use.own module load conda-env/env_name_here-py3.6.4
- For GPU-enabled applications, you may also need to load the corresponding cuda/ and cudnn/ modules.
- Now try running your code again.
- A few applications only run on specific versions of Python (e.g. Python 3.6). Please check the documentation of your application if that is the case.
- If you have installed a newer version of an ml-toolkit package (e.g., a newer version of PyTorch or Tensorflow), make sure that the ml-toolkit modules are NOT loaded. In general, we recommend that you don't mix ml-toolkit modules with your custom installations.
- GPU-enabled ML applications often have dependencies on specific versions of Cuda and CuDNN. For example, Tensorflow version 1.5.0 and higher needs Cuda 9. Please check the application documentation about such dependencies.
Link to section 'Tensorboard' of 'Custom ML Packages' Tensorboard
- You can visualize data from a Tensorflow session using Tensorboard. For this, you need to save your session summary as described in the Tensorboard User Guide.
- Launch Tensorboard:
$ python -m tensorboard.main --logdir=/path/to/session/logs
- When Tensorboard is launched successfully, it will give you the URL for accessing Tensorboard.
<... build related warnings ...> TensorBoard 0.4.0 at http://scholar-a000.rcac.purdue.edu:6006
- Follow the printed URL to visualize your model.
- Please note that due to firewall rules, the Tensorboard URL may only be accessible from Scholar nodes. If you cannot access the URL directly, you can use Firefox browser in Thinlinc.
- For more details, please refer to the Tensorboard User Guide.
Link to section 'Running ML Code in a Batch Job' of 'ML Batch Jobs' Running ML Code in a Batch Job
Batch jobs allow us to automate model training without human intervention. They are also useful when you need to run a large number of simulations on the clusters. In the example below, we shall run a simple tensor_hello.py script in a batch job. We consider two situations: in the first example, we use the ML-Toolkit modules to run tensorflow, while in the second example, we use a custom installation of tensorflow (See Custom ML Packages page).
Link to section 'Using ML-Toolkit Modules' of 'ML Batch Jobs' Using ML-Toolkit Modules
Save the following code as tensor_hello.sub in the same directory where tensor_hello.py is located.
# filename: tensor_hello.sub
#SBATCH --nodes=1
#SBATCH --ntasks-per-node=1
#SBATCH --gres=gpu:1
#SBATCH --time=00:05:00
#SBATCH -A scholar
#SBATCH -J hello_tensor
module purge
module load learning
module load ml-toolkit-gpu/tensorflow
module list
python tensor_hello.py
Link to section 'Using a Custom Installation' of 'ML Batch Jobs' Using a Custom Installation
Save the following code as tensor_hello.sub in the same directory where tensor_hello.py is located.
# filename: tensor_hello.sub
#SBATCH --nodes=1
#SBATCH --ntasks-per-node=1
#SBATCH --gres=gpu:1
#SBATCH --time=00:05:00
#SBATCH -A scholar
#SBATCH -J hello_tensor
module purge
module load anaconda
module load cuda
module load cudnn
module load use.own
module load conda-env/my_tf_env-py3.8.5
module list
echo $PYTHONPATH
python tensor_hello.py
Link to section 'Running a Job' of 'ML Batch Jobs' Running a Job
Now you can submit the batch job using the sbatch command.
sbatch tensor_hello.sub
Once the job finishes, you will find an output file (slurm-xxxxx.out).
Matlab
MATLAB® (MATrix LABoratory) is a high-level language and interactive environment for numerical computation, visualization, and programming. MATLAB is a product of MathWorks.
MATLAB, Simulink, Compiler, and several of the optional toolboxes are available to faculty, staff, and students. To see the kind and quantity of all MATLAB licenses plus the number that you are currently using you can use the matlab_licenses command:
$ module load matlab
$ matlab_licenses
The MATLAB client can be run in the front-end for application development, however, computationally intensive jobs must be run on compute nodes.
The following sections provide several examples illustrating how to submit MATLAB jobs to a Linux compute cluster.
Matlab Script (.m File)
This section illustrates how to submit a small, serial, MATLAB program as a job to a batch queue. This MATLAB program prints the name of the run host and gets three random numbers.
Prepare a MATLAB script myscript.m, and a MATLAB function file myfunction.m:
% FILENAME: myscript.m
% Display name of compute node which ran this job.
[c name] = system('hostname');
fprintf('\n\nhostname:%s\n', name);
% Display three random numbers.
A = rand(1,3);
fprintf('%f %f %f\n', A);
quit;
% FILENAME: myfunction.m
function result = myfunction ()
% Return name of compute node which ran this job.
[c name] = system('hostname');
result = sprintf('hostname:%s', name);
% Return three random numbers.
A = rand(1,3);
r = sprintf('%f %f %f', A);
result=strvcat(result,r);
end
Also, prepare a job submission file, here named myjob.sub. Run with the name of the script:
#!/bin/bash
# FILENAME: myjob.sub
echo "myjob.sub"
# Load module, and set up environment for Matlab to run
module load matlab
unset DISPLAY
# -nodisplay: run MATLAB in text mode; X11 server not needed
# -singleCompThread: turn off implicit parallelism
# -r: read MATLAB program; use MATLAB JIT Accelerator
# Run Matlab, with the above options and specifying our .m file
matlab -nodisplay -singleCompThread -r myscript
myjob.sub
< M A T L A B (R) >
Copyright 1984-2011 The MathWorks, Inc.
R2011b (7.13.0.564) 64-bit (glnxa64)
August 13, 2011
To get started, type one of these: helpwin, helpdesk, or demo.
For product information, visit www.mathworks.com.
hostname:scholar-a001.rcac.purdue.edu
0.814724 0.905792 0.126987
Output shows that a processor core on one compute node (scholar-a001) processed the job. Output also displays the three random numbers.
For more information about MATLAB:
Implicit Parallelism
MATLAB implements implicit parallelism which is automatic multithreading of many computations, such as matrix multiplication, linear algebra, and performing the same operation on a set of numbers. This is different from the explicit parallelism of the Parallel Computing Toolbox.
MATLAB offers implicit parallelism in the form of thread-parallel enabled functions. Since these processor cores, or threads, share a common memory, many MATLAB functions contain multithreading potential. Vector operations, the particular application or algorithm, and the amount of computation (array size) contribute to the determination of whether a function runs serially or with multithreading.
When your job triggers implicit parallelism, it attempts to allocate its threads on all processor cores of the compute node on which the MATLAB client is running, including processor cores running other jobs. This competition can degrade the performance of all jobs running on the node.
When you know that you are coding a serial job but are unsure whether you are using thread-parallel enabled operations, run MATLAB with implicit parallelism turned off. Beginning with the R2009b, you can turn multithreading off by starting MATLAB with -singleCompThread:
$ matlab -nodisplay -singleCompThread -r mymatlabprogram
When you are using implicit parallelism, make sure you request exclusive access to a compute node, as MATLAB has no facility for sharing nodes.
For more information about MATLAB's implicit parallelism:
Profile Manager
MATLAB offers two kinds of profiles for parallel execution: the 'local' profile and user-defined cluster profiles. The 'local' profile runs a MATLAB job on the processor core(s) of the same compute node, or front-end, that is running the client. To run a MATLAB job on compute node(s) different from the node running the client, you must define a Cluster Profile using the Cluster Profile Manager.
To prepare a user-defined cluster profile, use the Cluster Profile Manager in the Parallel menu. This profile contains the scheduler details (queue, nodes, processors, walltime, etc.) of your job submission. Ultimately, your cluster profile will be an argument to MATLAB functions like batch().
For your convenience, a generic cluster profile is provided that can be downloaded: myslurmprofile.settings
Please note that modifications are very likely to be required to make myslurmprofile.settings work. You may need to change values for number of nodes, number of workers, walltime, and submission queue specified in the file. As well, the generic profile itself depends on the particular job scheduler on the cluster, so you may need to download or create two or more generic profiles under different names. Each time you run a job using a Cluster Profile, make sure the specific profile you are using is appropriate for the job and the cluster.
To import the profile, start a MATLAB session and select Manage Cluster Profiles... from the Parallel menu. In the Cluster Profile Manager, select Import, navigate to the folder containing the profile, select myslurmprofile.settings and click OK. Remember that the profile will need to be customized for your specific needs. If you have any questions, please contact us.
For detailed information about MATLAB's Parallel Computing Toolbox, examples, demos, and tutorials:
Parallel Computing Toolbox (parfor)
The MATLAB Parallel Computing Toolbox (PCT) extends the MATLAB language with high-level, parallel-processing features such as parallel for loops, parallel regions, message passing, distributed arrays, and parallel numerical methods. It offers a shared-memory computing environment running on the local cluster profile in addition to your MATLAB client. Moreover, the MATLAB Distributed Computing Server (DCS) scales PCT applications up to the limit of your DCS licenses.
This section illustrates the fine-grained parallelism of a parallel for loop (parfor) in a pool job.
The following examples illustrate a method for submitting a small, parallel, MATLAB program with a parallel loop (parfor statement) as a job to a queue. This MATLAB program prints the name of the run host and shows the values of variables numlabs and labindex for each iteration of the parfor loop.
This method uses the job submission command to submit a MATLAB client which calls the MATLAB batch() function with a user-defined cluster profile.
Prepare a MATLAB pool program in a MATLAB script with an appropriate filename, here named myscript.m:
% FILENAME: myscript.m
% SERIAL REGION
[c name] = system('hostname');
fprintf('SERIAL REGION: hostname:%s\n', name)
numlabs = parpool('poolsize');
fprintf(' hostname numlabs labindex iteration\n')
fprintf(' ------------------------------- ------- -------- ---------\n')
tic;
% PARALLEL LOOP
parfor i = 1:8
[c name] = system('hostname');
name = name(1:length(name)-1);
fprintf('PARALLEL LOOP: %-31s %7d %8d %9d\n', name,numlabs,labindex,i)
pause(2);
end
% SERIAL REGION
elapsed_time = toc; % get elapsed time in parallel loop
fprintf('\n')
[c name] = system('hostname');
name = name(1:length(name)-1);
fprintf('SERIAL REGION: hostname:%s\n', name)
fprintf('Elapsed time in parallel loop: %f\n', elapsed_time)
The execution of a pool job starts with a worker executing the statements of the first serial region up to the parfor block, when it pauses. A set of workers (the pool) executes the parfor block. When they finish, the first worker resumes by executing the second serial region. The code displays the names of the compute nodes running the batch session and the worker pool.
Prepare a MATLAB script that calls MATLAB function batch() which makes a four-lab pool on which to run the MATLAB code in the file myscript.m. Use an appropriate filename, here named mylclbatch.m:
% FILENAME: mylclbatch.m
!echo "mylclbatch.m"
!hostname
pjob=batch('myscript','Profile','myslurmprofile','Pool',4,'CaptureDiary',true);
wait(pjob);
diary(pjob);
quit;
Prepare a job submission file with an appropriate filename, here named myjob.sub:
#!/bin/bash
# FILENAME: myjob.sub
echo "myjob.sub"
hostname
module load matlab
unset DISPLAY
matlab -nodisplay -r mylclbatch
Submit the job as a single compute node with one processor core.
One processor core runs myjob.sub and mylclbatch.m.
Once this job starts, a second job submission is made.
myjob.sub
< M A T L A B (R) >
Copyright 1984-2013 The MathWorks, Inc.
R2013a (8.1.0.604) 64-bit (glnxa64)
February 15, 2013
To get started, type one of these: helpwin, helpdesk, or demo.
For product information, visit www.mathworks.com.
mylclbatch.mscholar-a000.rcac.purdue.edu
SERIAL REGION: hostname:scholar-a000.rcac.purdue.edu
hostname numlabs labindex iteration
------------------------------- ------- -------- ---------
PARALLEL LOOP: scholar-a001.rcac.purdue.edu 4 1 2
PARALLEL LOOP: scholar-a002.rcac.purdue.edu 4 1 4
PARALLEL LOOP: scholar-a001.rcac.purdue.edu 4 1 5
PARALLEL LOOP: scholar-a002.rcac.purdue.edu 4 1 6
PARALLEL LOOP: scholar-a003.rcac.purdue.edu 4 1 1
PARALLEL LOOP: scholar-a003.rcac.purdue.edu 4 1 3
PARALLEL LOOP: scholar-a004.rcac.purdue.edu 4 1 7
PARALLEL LOOP: scholar-a004.rcac.purdue.edu 4 1 8
SERIAL REGION: hostname:scholar-a001.rcac.purdue.edu
Elapsed time in parallel loop: 5.411486
To scale up this method to handle a real application, increase the wall time in the submission command to accommodate a longer running job. Secondly, increase the wall time of myslurmprofile by using the Cluster Profile Manager in the Parallel menu to enter a new wall time in the property SubmitArguments.
For more information about MATLAB Parallel Computing Toolbox:
Parallel Toolbox (spmd)
The MATLAB Parallel Computing Toolbox (PCT) extends the MATLAB language with high-level, parallel-processing features such as parallel for loops, parallel regions, message passing, distributed arrays, and parallel numerical methods. It offers a shared-memory computing environment with a maximum of eight MATLAB workers (labs, threads; versions R2009a) and 12 workers (labs, threads; version R2011a) running on the local configuration in addition to your MATLAB client. Moreover, the MATLAB Distributed Computing Server (DCS) scales PCT applications up to the limit of your DCS licenses.
This section illustrates how to submit a small, parallel, MATLAB program with a parallel region (spmd statement) as a MATLAB pool job to a batch queue.
This example uses the submission command to submit to compute nodes a MATLAB client which interprets a Matlab .m with a user-defined cluster profile which scatters the MATLAB workers onto different compute nodes. This method uses the MATLAB interpreter, the Parallel Computing Toolbox, and the Distributed Computing Server; so, it requires and checks out six licenses: one MATLAB license for the client running on the compute node, one PCT license, and four DCS licenses. Four DCS licenses run the four copies of the spmd statement. This job is completely off the front end.
Prepare a MATLAB script called myscript.m:
% FILENAME: myscript.m
% SERIAL REGION
[c name] = system('hostname');
fprintf('SERIAL REGION: hostname:%s\n', name)
p = parpool('4');
fprintf(' hostname numlabs labindex\n')
fprintf(' ------------------------------- ------- --------\n')
tic;
% PARALLEL REGION
spmd
[c name] = system('hostname');
name = name(1:length(name)-1);
fprintf('PARALLEL REGION: %-31s %7d %8d\n', name,numlabs,labindex)
pause(2);
end
% SERIAL REGION
elapsed_time = toc; % get elapsed time in parallel region
delete(p);
fprintf('\n')
[c name] = system('hostname');
name = name(1:length(name)-1);
fprintf('SERIAL REGION: hostname:%s\n', name)
fprintf('Elapsed time in parallel region: %f\n', elapsed_time)
quit;
Prepare a job submission file with an appropriate filename, here named myjob.sub. Run with the name of the script:
#!/bin/bash
# FILENAME: myjob.sub
echo "myjob.sub"
module load matlab
unset DISPLAY
matlab -nodisplay -r myscript
Run MATLAB to set the default parallel configuration to your job configuration:
$ matlab -nodisplay
>> parallel.defaultClusterProfile('myslurmprofile');
>> quit;
$
Once this job starts, a second job submission is made.
myjob.sub
< M A T L A B (R) >
Copyright 1984-2011 The MathWorks, Inc.
R2011b (7.13.0.564) 64-bit (glnxa64)
August 13, 2011
To get started, type one of these: helpwin, helpdesk, or demo.
For product information, visit www.mathworks.com.
SERIAL REGION: hostname:scholar-a001.rcac.purdue.edu
Starting matlabpool using the 'myslurmprofile' profile ... connected to 4 labs.
hostname numlabs labindex
------------------------------- ------- --------
Lab 2:
PARALLEL REGION: scholar-a002.rcac.purdue.edu 4 2
Lab 1:
PARALLEL REGION: scholar-a001.rcac.purdue.edu 4 1
Lab 3:
PARALLEL REGION: scholar-a003.rcac.purdue.edu 4 3
Lab 4:
PARALLEL REGION: scholar-a004.rcac.purdue.edu 4 4
Sending a stop signal to all the labs ... stopped.
SERIAL REGION: hostname:scholar-a001.rcac.purdue.edu
Elapsed time in parallel region: 3.382151
Output shows the name of one compute node (a001) that processed the job submission file myjob.sub and the two serial regions. The job submission scattered four processor cores (four MATLAB labs) among four different compute nodes (a001,a002,a003,a004) that processed the four parallel regions. The total elapsed time demonstrates that the jobs ran in parallel.
For more information about MATLAB Parallel Computing Toolbox:
Distributed Computing Server (parallel job)
The MATLAB Parallel Computing Toolbox (PCT) enables a parallel job via the MATLAB Distributed Computing Server (DCS). The tasks of a parallel job are identical, run simultaneously on several MATLAB workers (labs), and communicate with each other. This section illustrates an MPI-like program.
This section illustrates how to submit a small, MATLAB parallel job with four workers running one MPI-like task to a batch queue. The MATLAB program broadcasts an integer to four workers and gathers the names of the compute nodes running the workers and the lab IDs of the workers.
This example uses the job submission command to submit a Matlab script with a user-defined cluster profile which scatters the MATLAB workers onto different compute nodes. This method uses the MATLAB interpreter, the Parallel Computing Toolbox, and the Distributed Computing Server; so, it requires and checks out six licenses: one MATLAB license for the client running on the compute node, one PCT license, and four DCS licenses. Four DCS licenses run the four copies of the parallel job. This job is completely off the front end.
Prepare a MATLAB script named myscript.m :
% FILENAME: myscript.m
% Specify pool size.
% Convert the parallel job to a pool job.
parpool('4');
spmd
if labindex == 1
% Lab (rank) #1 broadcasts an integer value to other labs (ranks).
N = labBroadcast(1,int64(1000));
else
% Each lab (rank) receives the broadcast value from lab (rank) #1.
N = labBroadcast(1);
end
% Form a string with host name, total number of labs, lab ID, and broadcast value.
[c name] =system('hostname');
name = name(1:length(name)-1);
fmt = num2str(floor(log10(numlabs))+1);
str = sprintf(['%s:%d:%' fmt 'd:%d '], name,numlabs,labindex,N);
% Apply global concatenate to all str's.
% Store the concatenation of str's in the first dimension (row) and on lab #1.
result = gcat(str,1,1);
if labindex == 1
disp(result)
end
end % spmd
matlabpool close force;
quit;
Also, prepare a job submission, here named myjob.sub. Run with the name of the script:
# FILENAME: myjob.sub
echo "myjob.sub"
module load matlab
unset DISPLAY
# -nodisplay: run MATLAB in text mode; X11 server not needed
# -r: read MATLAB program; use MATLAB JIT Accelerator
matlab -nodisplay -r myscript
Run MATLAB to set the default parallel configuration to your appropriate Profile:
$ matlab -nodisplay
>> defaultParallelConfig('myslurmprofile');
>> quit;
$
Submit the job as a single compute node with one processor core.
Once this job starts, a second job submission is made.
myjob.sub
< M A T L A B (R) >
Copyright 1984-2011 The MathWorks, Inc.
R2011b (7.13.0.564) 64-bit (glnxa64)
August 13, 2011
To get started, type one of these: helpwin, helpdesk, or demo.
For product information, visit www.mathworks.com.
>Starting matlabpool using the 'myslurmprofile' configuration ... connected to 4 labs.
Lab 1:
scholar-a006.rcac.purdue.edu:4:1:1000
scholar-a007.rcac.purdue.edu:4:2:1000
scholar-a008.rcac.purdue.edu:4:3:1000
scholar-a009.rcac.purdue.edu:4:4:1000
Sending a stop signal to all the labs ... stopped.
Did not find any pre-existing parallel jobs created by matlabpool.
Output shows the name of one compute node (a006) that processed the job submission file myjob.sub. The job submission scattered four processor cores (four MATLAB labs) among four different compute nodes (a006,a007,a008,a009) that processed the four parallel regions.
To scale up this method to handle a real application, increase the wall time in the submission command to accommodate a longer running job. Secondly, increase the wall time of myslurmprofile by using the Cluster Profile Manager in the Parallel menu to enter a new wall time in the property SubmitArguments.
For more information about parallel jobs:
Python
Notice: Python 2.7 has reached end-of-life on Jan 1, 2020 (announcement). Please update your codes and your job scripts to use Python 3.
Python is a high-level, general-purpose, interpreted, dynamic programming language. We suggest using Anaconda which is a Python distribution made for large-scale data processing, predictive analytics, and scientific computing. For example, to use the default Anaconda distribution:
$ module load conda
For a full list of available Anaconda and Python modules enter:
$ module spider conda
Example Python Jobs
This section illustrates how to submit a small Python job to a SLURM queue.
Link to section 'Example 1: Hello world' of 'Example Python Jobs' Example 1: Hello world
Prepare a Python input file with an appropriate filename, here named hello.py
:
# FILENAME: hello.py
import string, sys
print("Hello, world!")
Prepare a job submission file with an appropriate filename, here named myjob.sub:
#!/bin/bash
# FILENAME: myjob.sub
module load conda
python hello.py
Hello, world!
Link to section 'Example 2: Matrix multiply' of 'Example Python Jobs' Example 2: Matrix multiply
Save the following script as matrix.py:
# Matrix multiplication program
x = [[3,1,4],[1,5,9],[2,6,5]]
y = [[3,5,8,9],[7,9,3,2],[3,8,4,6]]
result = [[sum(a*b for a,b in zip(x_row,y_col)) for y_col in zip(*y)] for x_row in x]
for r in result:
print(r)
Change the last line in the job submission file above to read:
python matrix.py
The standard output file from this job will result in the following matrix:
[28, 56, 43, 53]
[65, 122, 59, 73]
[63, 104, 54, 60]
Link to section 'Example 3: Sine wave plot using numpy and matplotlib packages' of 'Example Python Jobs' Example 3: Sine wave plot using numpy and matplotlib packages
Save the following script as sine.py:
import numpy as np
import matplotlib
matplotlib.use('Agg')
import matplotlib.pyplot as plt
x = np.linspace(-np.pi, np.pi, 201)
plt.plot(x, np.sin(x))
plt.xlabel('Angle [rad]')
plt.ylabel('sin(x)')
plt.axis('tight')
plt.savefig('sine.png')
Change your job submission file to submit this script and the job will output a png file and blank standard output and error files.
For more information about Python:
Managing Environments with Conda
Conda is a package manager in Anaconda that allows you to create and manage multiple environments where you can pick and choose which packages you want to use. To use Conda you must load an Anaconda module:
$ module load conda
Many packages are pre-installed in the global environment. To see these packages:
$ conda list
To create your own custom environment:
$ conda create --name MyEnvName python=3.8 FirstPackageName SecondPackageName -y
The --name option specifies that the environment created will be named MyEnvName. You can include as many packages as you require separated by a space. Including the -y option lets you skip the prompt to install the package. By default environments are created and stored in the $HOME/.conda directory.
To create an environment at a custom location:
$ conda create --prefix=$HOME/MyEnvName python=3.8 PackageName -y
To see a list of your environments:
$ conda env list
To remove unwanted environments:
$ conda remove --name MyEnvName --all
To add packages to your environment:
$ conda install --name MyEnvName PackageNames
To remove a package from an environment:
$ conda remove --name MyEnvName PackageName
Installing packages when creating your environment, instead of one at a time, will help you avoid dependency issues.
To activate or deactivate an environment you have created:
$ source activate MyEnvName
$ source deactivate MyEnvName
If you created your conda environment at a custom location using --prefix option, then you can activate or deactivate it using the full path.
$ source activate $HOME/MyEnvName
$ source deactivate $HOME/MyEnvName
To use a custom environment inside a job you must load the module and activate the environment inside your job submission script. Add the following lines to your submission script:
$ module load conda
$ source activate MyEnvName
For more information about Python:
Managing Packages with Pip
Pip is a Python package manager. Many Python package documentation provide pip
instructions that result in permission errors because by default pip
will install in a system-wide location and fail.
Exception:
Traceback (most recent call last):
... ... stack trace ... ...
OSError: [Errno 13] Permission denied: '/apps/cent7/anaconda/2020.07-py38/lib/python3.8/site-packages/mkl_random-1.1.1.dist-info'
If you encounter this error, it means that you cannot modify the global Python installation. We recommend installing Python packages in a conda environment. Detailed instructions for installing packages with pip can be found in our Python package installation page.
Below we list some other useful pip commands.
- Search for a package in PyPI channels:
$ pip search packageName
- Check which packages are installed globally:
$ pip list
- Check which packages you have personally installed:
$ pip list --user
- Snapshot installed packages:
$ pip freeze > requirements.txt
- You can install packages from a snapshot inside a new conda environment. Make sure to load the appropriate conda environment first.
$ pip install -r requirements.txt
For more information about Python:
Installing Packages
Installing Python packages in an Anaconda environment is recommended. One key advantage of Anaconda is that it allows users to install unrelated packages in separate self-contained environments. Individual packages can later be reinstalled or updated without impacting others. If you are unfamiliar with Conda environments, please check our Conda Guide.
To facilitate the process of creating and using Conda environments, we support a script (conda-env-mod) that generates a module file for an environment, as well as an optional Jupyter kernel to use this environment in a JupyterHub notebook.
You must load one of the anaconda modules in order to use this script.
$ module load conda
Step-by-step instructions for installing custom Python packages are presented below.
Link to section 'Step 1: Create a conda environment' of 'Installing Packages' Step 1: Create a conda environment
Users can use the conda-env-mod script to create an empty conda environment. This script needs either a name or a path for the desired environment. After the environment is created, it generates a module file for using it in future. Please note that conda-env-mod is different from the official conda-env script and supports a limited set of subcommands. Detailed instructions for using conda-env-mod can be found with the command conda-env-mod --help.
-
Example 1: Create a conda environment named mypackages in user's
$HOME
directory.$ conda-env-mod create -n mypackages
-
Example 2: Create a conda environment named mypackages at a custom location.
$ conda-env-mod create -p /depot/mylab/apps/mypackages
Please follow the on-screen instructions while the environment is being created. After finishing, the script will print the instructions to use this environment.
... ... ... Preparing transaction: ...working... done Verifying transaction: ...working... done Executing transaction: ...working... done +------------------------------------------------------+ | To use this environment, load the following modules: | | module load use.own | | module load conda-env/mypackages-py3.8.5 | +------------------------------------------------------+ Your environment "mypackages" was created successfully.
Note down the module names, as you will need to load these modules every time you want to use this environment. You may also want to add the module load lines in your jobscript, if it depends on custom Python packages.
By default, module files are generated in your $HOME/privatemodules directory. The location of module files can be customized by specifying the -m /path/to/modules option to conda-env-mod.
Note: The main differences between -p and -m are: 1) -p will change the location of packages to be installed for the env and the module file will still be located at the $HOME/privatemodules directory as defined in use.own. 2) -m will only change the location of the module file. So the method to load modules created with -m and -p are different, see Example 3 for details.
- Example 3: Create a conda environment named labpackages in your group's Data Depot space and place the module file at a shared location for the group to use.
$ conda-env-mod create -p /depot/mylab/apps/labpackages -m /depot/mylab/etc/modules ... ... ... Preparing transaction: ...working... done Verifying transaction: ...working... done Executing transaction: ...working... done +-------------------------------------------------------+ | To use this environment, load the following modules: | | module use /depot/mylab/etc/modules | | module load conda-env/labpackages-py3.8.5 | +-------------------------------------------------------+ Your environment "labpackages" was created successfully.
If you used a custom module file location, you need to run the module use command as printed by the command output above.
By default, only the environment and a module file are created (no Jupyter kernel). If you plan to use your environment in a JupyterHub notebook, you need to append a --jupyter flag to the above commands.
- Example 4: Create a Jupyter-enabled conda environment named labpackages in your group's Data Depot space and place the module file at a shared location for the group to use.
$ conda-env-mod create -p /depot/mylab/apps/labpackages -m /depot/mylab/etc/modules --jupyter ... ... ... Jupyter kernel created: "Python (My labpackages Kernel)" ... ... ... Your environment "labpackages" was created successfully.
Link to section 'Step 2: Load the conda environment' of 'Installing Packages' Step 2: Load the conda environment
-
The following instructions assume that you have used conda-env-mod script to create an environment named mypackages (Examples 1 or 2 above). If you used conda create instead, please use conda activate mypackages.
$ module load use.own $ module load conda-env/mypackages-py3.8.5
Note that the conda-env module name includes the Python version that it supports (Python 3.8.5 in this example). This is same as the Python version in the conda module.
-
If you used a custom module file location (Example 3 above), please use module use to load the conda-env module.
$ module use /depot/mylab/etc/modules $ module load conda-env/labpackages-py3.8.5
Link to section 'Step 3: Install packages' of 'Installing Packages' Step 3: Install packages
Now you can install custom packages in the environment using either conda install or pip install.
Link to section 'Installing with conda' of 'Installing Packages' Installing with conda
-
Example 1: Install OpenCV (open-source computer vision library) using conda.
$ conda install opencv
-
Example 2: Install a specific version of OpenCV using conda.
$ conda install opencv=4.5.5
-
Example 3: Install OpenCV from a specific anaconda channel.
$ conda install -c anaconda opencv
Link to section 'Installing with pip' of 'Installing Packages' Installing with pip
-
Example 4: Install pandas using pip.
$ pip install pandas
-
Example 5: Install a specific version of pandas using pip.
$ pip install pandas==1.4.3
Follow the on-screen instructions while the packages are being installed. If installation is successful, please proceed to the next section to test the packages.
Note: Do NOT run Pip with the --user argument, as that will install packages in a different location and might mess up your account environment.
Link to section 'Step 4: Test the installed packages' of 'Installing Packages' Step 4: Test the installed packages
To use the installed Python packages, you must load the module for your conda environment. If you have not loaded the conda-env module, please do so following the instructions at the end of Step 1.
$ module load use.own
$ module load conda-env/mypackages-py3.8.5
- Example 1: Test that OpenCV is available.
$ python -c "import cv2; print(cv2.__version__)"
- Example 2: Test that pandas is available.
$ python -c "import pandas; print(pandas.__version__)"
If the commands finished without errors, then the installed packages can be used in your program.
Link to section 'Additional capabilities of conda-env-mod script' of 'Installing Packages' Additional capabilities of conda-env-mod script
The conda-env-mod tool is intended to facilitate creation of a minimal Anaconda environment, matching module file and optionally a Jupyter kernel. Once created, the environment can then be accessed via familiar module load command, tuned and expanded as necessary. Additionally, the script provides several auxiliary functions to help manage environments, module files and Jupyter kernels.
General usage for the tool adheres to the following pattern:
$ conda-env-mod help
$ conda-env-mod <subcommand> <required argument> [optional arguments]
where required arguments are one of
- -n|--name ENV_NAME (name of the environment)
- -p|--prefix ENV_PATH (location of the environment)
and optional arguments further modify behavior for specific actions (e.g. -m to specify alternative location for generated module files).
Given a required name or prefix for an environment, the conda-env-mod script supports the following subcommands:
- create - to create a new environment, its corresponding module file and optional Jupyter kernel.
- delete - to delete existing environment along with its module file and Jupyter kernel.
- module - to generate just the module file for a given existing environment.
- kernel - to generate just the Jupyter kernel for a given existing environment (note that the environment has to be created with a --jupyter option).
- help - to display script usage help.
Using these subcommands, you can iteratively fine-tune your environments, module files and Jupyter kernels, as well as delete and re-create them with ease. Below we cover several commonly occurring scenarios.
Note: When you try to use conda-env-mod delete, remember to include the arguments as you create the environment (i.e. -p package_location and/or -m module_location).
Link to section 'Generating module file for an existing environment' of 'Installing Packages' Generating module file for an existing environment
If you already have an existing configured Anaconda environment and want to generate a module file for it, follow appropriate examples from Step 1 above, but use the module subcommand instead of the create one. E.g.
$ conda-env-mod module -n mypackages
and follow printed instructions on how to load this module. With an optional --jupyter flag, a Jupyter kernel will also be generated.
Note that the module name mypackages should be exactly the same with the older conda environment name. Note also that if you intend to proceed with a Jupyter kernel generation (via the --jupyter flag or a kernel subcommand later), you will have to ensure that your environment has ipython and ipykernel packages installed into it. To avoid this and other related complications, we highly recommend making a fresh environment using a suitable conda-env-mod create .... --jupyter command instead.
Link to section 'Generating Jupyter kernel for an existing environment' of 'Installing Packages' Generating Jupyter kernel for an existing environment
If you already have an existing configured Anaconda environment and want to generate a Jupyter kernel file for it, you can use the kernel subcommand. E.g.
$ conda-env-mod kernel -n mypackages
This will add a "Python (My mypackages Kernel)" item to the dropdown list of available kernels upon your next login to the JupyterHub.
Note that generated Jupiter kernels are always personal (i.e. each user has to make their own, even for shared environments). Note also that you (or the creator of the shared environment) will have to ensure that your environment has ipython and ipykernel packages installed into it.
Link to section 'Managing and using shared Python environments' of 'Installing Packages' Managing and using shared Python environments
Here is a suggested workflow for a common group-shared Anaconda environment with Jupyter capabilities:
The PI or lab software manager:
-
Creates the environment and module file (once):
$ module purge $ module load conda $ conda-env-mod create -p /depot/mylab/apps/labpackages -m /depot/mylab/etc/modules --jupyter
-
Installs required Python packages into the environment (as many times as needed):
$ module use /depot/mylab/etc/modules $ module load conda-env/labpackages-py3.8.5 $ conda install ....... # all the necessary packages
Lab members:
-
Lab members can start using the environment in their command line scripts or batch jobs simply by loading the corresponding module:
$ module use /depot/mylab/etc/modules $ module load conda-env/labpackages-py3.8.5 $ python my_data_processing_script.py .....
-
To use the environment in Jupyter notebooks, each lab member will need to create his/her own Jupyter kernel (once). This is because Jupyter kernels are private to individuals, even for shared environments.
$ module use /depot/mylab/etc/modules $ module load conda-env/labpackages-py3.8.5 $ conda-env-mod kernel -p /depot/mylab/apps/labpackages
A similar process can be devised for instructor-provided or individually-managed class software, etc.
Link to section 'Troubleshooting' of 'Installing Packages' Troubleshooting
- Python packages often fail to install or run due to dependency incompatibility with other packages. More specifically, if you previously installed packages in your home directory it is safer to clean those installations.
$ mv ~/.local ~/.local.bak $ mv ~/.cache ~/.cache.bak
- Unload all the modules.
$ module purge
- Clean up PYTHONPATH.
$ unset PYTHONPATH
- Next load the modules (e.g. anaconda) that you need.
$ module load conda/2024.02-py311 $ module load use.own $ module load conda-env/2024.02-py311
- Now try running your code again.
- Few applications only run on specific versions of Python (e.g. Python 3.6). Please check the documentation of your application if that is the case.
Installing Packages from Source
We maintain several Anaconda installations. Anaconda maintains numerous popular scientific Python libraries in a single installation. If you need a Python library not included with normal Python we recommend first checking Anaconda. For a list of modules currently installed in the Anaconda Python distribution:
$ module load conda
$ conda list
# packages in environment at /apps/spack/bell/apps/anaconda/2020.02-py37-gcc-4.8.5-u747gsx:
#
# Name Version Build Channel
_ipyw_jlab_nb_ext_conf 0.1.0 py37_0
_libgcc_mutex 0.1 main
alabaster 0.7.12 py37_0
anaconda 2020.02 py37_0
...
If you see the library in the list, you can simply import it into your Python code after loading the Anaconda module.
If you do not find the package you need, you should be able to install the library in your own Anaconda customization. First try to install it with Conda or Pip. If the package is not available from either Conda or Pip, you may be able to install it from source.
Use the following instructions as a guideline for installing packages from source. Make sure you have a download link to the software (usually it will be a tar.gz
archive file). You will substitute it on the wget line below.
We also assume that you have already created an empty conda environment as described in our Python package installation guide.
$ mkdir ~/src
$ cd ~/src
$ wget http://path/to/source/tarball/app-1.0.tar.gz
$ tar xzvf app-1.0.tar.gz
$ cd app-1.0
$ module load conda
$ module load use.own
$ module load conda-env/mypackages-py3.8.5
$ python setup.py install
$ cd ~
$ python
>>> import app
>>> quit()
The "import app" line should return without any output if installed successfully. You can then import the package in your python scripts.
If you need further help or run into any issues installing a library, contact us or drop by Coffee Hour for in-person help.
For more information about Python:
Example: Create and Use Biopython Environment with Conda
Link to section 'Using conda to create an environment that uses the biopython package' of 'Example: Create and Use Biopython Environment with Conda' Using conda to create an environment that uses the biopython package
To use Conda you must first load the anaconda module:
module load conda
Create an empty conda environment to install biopython:
conda-env-mod create -n biopython
Now activate the biopython environment:
module load use.own
module load conda-env/biopython-py3.12.5
Install the biopython packages in your environment:
conda install --channel anaconda biopython -y
Fetching package metadata ..........
Solving package specifications .........
.......
Linking packages ...
[ COMPLETE ]|################################################################
The --channel option specifies that it searches the anaconda channel for the biopython package. The -y argument is optional and allows you to skip the installation prompt. A list of packages will be displayed as they are installed.
Remember to add the following lines to your job submission script to use the custom environment in your jobs:
module load conda
module load use.own
module load conda-env/biopython-py3.12.5
If you need further help or run into any issues with creating environments, contact us or drop by Coffee Hour for in-person help.
For more information about Python:
Numpy Parallel Behavior
The widely available Numpy package is the best way to handle numerical computation in Python. The numpy
package provided by our anaconda
modules is optimized using Intel's MKL library. It will automatically parallelize many operations to make use of all the cores available on a machine.
In many contexts that would be the ideal behavior. On the cluster however that very likely is not in fact the preferred behavior because often more than one user is present on the system and/or more than one job on a node. Having multiple processes contend for those resources will actually result in lesser performance.
Setting the MKL_NUM_THREADS
or OMP_NUM_THREADS
environment variable(s) allows you to control this behavior. Our anaconda modules automatically set these variables to 1 if and only if you do not currently have that variable defined.
When submitting batch jobs it is always a good idea to be explicit rather than implicit. If you are submitting a job that you want to make use of the full resources available on the node, set one or both of these variables to the number of cores you want to allow numpy to make use of.
#!/bin/bash
module load conda
export MKL_NUM_THREADS=20
...
If you are submitting multiple jobs that you intend to be scheduled together on the same node, it is probably best to restrict numpy to a single core.
#!/bin/bash
module load conda
export MKL_NUM_THREADS=1
R
R, a GNU project, is a language and environment for data manipulation, statistics, and graphics. It is an open source version of the S programming language. R is quickly becoming the language of choice for data science due to the ease with which it can produce high quality plots and data visualizations. It is a versatile platform with a large, growing community and collection of packages.
For more general information on R visit The R Project for Statistical Computing.
Running R jobs
This section illustrates how to submit a small R job to a SLURM queue. The example job computes a Pythagorean triple.
Prepare an R input file with an appropriate filename, here named myjob.R:
# FILENAME: myjob.R
# Compute a Pythagorean triple.
a = 3
b = 4
c = sqrt(a*a + b*b)
c # display result
Prepare a job submission file with an appropriate filename, here named myjob.sub:
#!/bin/bash
# FILENAME: myjob.sub
module load r
# --vanilla:
# --no-save: do not save datasets at the end of an R session
R --vanilla --no-save < myjob.R
For other examples or R jobs:
Installing R packages
Link to section 'Challenges of Managing R Packages in the Cluster Environment' of 'Installing R packages' Challenges of Managing R Packages in the Cluster Environment
- Different clusters have different hardware and softwares. So, if you have access to multiple clusters, you must install your R packages separately for each cluster.
- Each cluster has multiple versions of R and packages installed with one version of R may not work with another version of R. So, libraries for each R version must be installed in a separate directory.
- You can define the directory where your R packages will be installed using the environment variable
R_LIBS_USER
. - For your convenience, a sample ~/.Rprofile example file is provided that can be downloaded to your cluster account and renamed into
~/.Rprofile
(or appended to one) to customize your installation preferences. Detailed instructions.
Link to section 'Installing Packages' of 'Installing R packages' Installing Packages
-
Step 0: Set up installation preferences.
Follow the steps for setting up your~/.Rprofile
preferences. This step needs to be done only once. If you have created a~/.Rprofile
file previously on Scholar, ignore this step. -
Step 1: Check if the package is already installed.
As part of the R installations on community clusters, a lot of R libraries are pre-installed. You can check if your package is already installed by opening an R terminal and entering the commandinstalled.packages()
. For example,module load r/4.4.1 R
installed.packages()["units",c("Package","Version")] Package Version "units" "0.8-1" quit()
If the package you are trying to use is already installed, simply load the library, e.g.,
library('units')
. Otherwise, move to the next step to install the package. -
Step 2: Load required dependencies. (if needed)
For simple packages you may not need this step. However, some R packages depend on other libraries. For example, thesf
package depends ongdal
andgeos
libraries. So, you will need to load the corresponding modules before installingsf
. Read the documentation for the package to identify which modules should be loaded.module load gdal module load geos
-
Step 3: Install the package.
Now install the desired package using the commandinstall.packages('package_name')
. R will automatically download the package and all its dependencies from CRAN and install each one. Your terminal will show the build progress and eventually show whether the package was installed successfully or not.R
install.packages('sf', repos="https://cran.case.edu/") Installing package into ‘/home/myusername/R/x86_64-pc-linux-gnu-library/4.4.1’ (as ‘lib’ is unspecified) trying URL 'https://cran.case.edu/src/contrib/sf_0.9-7.tar.gz' Content type 'application/x-gzip' length 4203095 bytes (4.0 MB) ================================================== downloaded 4.0 MB ... ... more progress messages ... ... ** testing if installed package can be loaded from final location ** testing if installed package keeps a record of temporary installation path * DONE (sf) The downloaded source packages are in ‘/tmp/RtmpSVAGio/downloaded_packages’
- Step 4: Troubleshooting. (if needed)
If Step 3 ended with an error, you need to investigate why the build failed. Most common reason for build failure is not loading the necessary modules.
Link to section 'Loading Libraries' of 'Installing R packages' Loading Libraries
Once you have packages installed you can load them with the library()
function as shown below:
library('packagename')
The package is now installed and loaded and ready to be used in R.
Link to section 'Example: Installing dplyr' of 'Installing R packages' Example: Installing dplyr
The following demonstrates installing the dplyr
package assuming the above-mentioned custom ~/.Rprofile
is in place (note its effect in the "Installing package into" information message):
module load r
R
install.packages('dplyr', repos="http://ftp.ussg.iu.edu/CRAN/")
Installing package into ‘/home/myusername/R/scholar/4.4.1’
(as ‘lib’ is unspecified)
...
also installing the dependencies 'crayon', 'utf8', 'bindr', 'cli', 'pillar', 'assertthat', 'bindrcpp', 'glue', 'pkgconfig', 'rlang', 'Rcpp', 'tibble', 'BH', 'plogr'
...
...
...
The downloaded source packages are in
'/tmp/RtmpHMzm9z/downloaded_packages'
library(dplyr)
Attaching package: 'dplyr'
For more information about installing R packages:
Loading Data into R
R is an environment for manipulating data. In order to manipulate data, it must be brought into the R environment. R has a function to read any file that data is stored in. Some of the most common file types like comma-separated variable(CSV) files have functions that come in the basic R packages. Other less common file types require additional packages to be installed. To read data from a CSV file into the R environment, enter the following command in the R prompt:
> read.csv(file = "path/to/data.csv", header = TRUE)
When R reads the file it creates an object that can then become the target of other functions. By default the read.csv() function will give the object the name of the .csv file. To assign a different name to the object created by read.csv enter the following in the R prompt:
> my_variable <- read.csv(file = "path/to/data.csv", header = FALSE)
To display the properties (structure) of loaded data, enter the following:
> str(my_variable)
For more functions and tutorials:
RStudio
RStudio is a graphical integrated development environment (IDE) for R. RStudio is the most popular environment for developing both R scripts and packages. RStudio is provided on most Research systems.
There are two methods to launch RStudio on the cluster: command-line and application menu icon.
Link to section 'Launch RStudio by the command-line:' of 'RStudio' Launch RStudio by the command-line:
module load gcc
module load r
module load rstudio
rstudio
Note that RStudio is a graphical program and in order to run it you must have a local X11 server running or use Thinlinc Remote Desktop environment. See the ssh X11 forwarding section for more details.
Link to section 'Launch Rstudio by the application menu icon:' of 'RStudio' Launch Rstudio by the application menu icon:
- Log into desktop.scholar.rcac.purdue.edu with web browser or ThinLinc client
- Click on the
Applications
drop down menu on the top left corner - Choose
Cluster Software
and thenRStudio
R and RStudio are free to download and run on your local machine. For more information about RStudio:
Setting Up R Preferences with .Rprofile
For your convenience, a sample ~/.Rprofile example file is provided that can be downloaded to your cluster account and renamed into ~/.Rprofile
(or appended to one). Follow these steps to download our recommended ~/.Rprofile
example and copy it into place:
curl -#LO https://www.rcac.purdue.edu/files/knowledge/run/examples/apps/r/Rprofile_example
mv -ib Rprofile_example ~/.Rprofile
The above installation step needs to be done only once on Scholar. Now load the R module and run R:
module load r/4.4.1
R
.libPaths()
[1] "/home/myusername/R/scholar/4.1.2-gcc-6.3.0-ymdumss"
[2] "/apps/spack/scholar/apps/r/4.1.2-gcc-6.3.0-ymdumss/rlib/R/library"
.libPaths()
should output something similar to above if it is set up correctly.
You are now ready to install R packages into the dedicated directory /home/myusername/R/scholar/4.1.2-gcc-6.3.0-ymdumss
.
Singularity
Note: Singularity was originally a project out of Lawrence Berkeley National Laboratory. It has now been spun off into a distinct offering under a new corporate entity under the name Sylabs Inc. This guide pertains to the open source community edition, SingularityCE.
Link to section 'What is Singularity?' of 'Singularity' What is Singularity?
Singularity is a new feature of the Community Clusters allowing the portability and reproducibility of operating system and application environments through the use of Linux containers. It gives users complete control over their environment.
Singularity is like Docker but tuned explicitly for HPC clusters. More information is available from the project’s website.
Link to section 'Features' of 'Singularity' Features
- Run the latest applications on an Ubuntu or Centos userland
- Gain access to the latest developer tools
- Launch MPI programs easily
- Much more
Singularity’s user guide is available at: sylabs.io/guides/3.8/user-guide
Link to section 'Example' of 'Singularity' Example
Here is an example using an Ubuntu 16.04 image on Scholar:
singularity exec /depot/itap/singularity/ubuntu1604.img cat /etc/lsb-release
DISTRIB_ID=Ubuntu
DISTRIB_RELEASE=16.04
DISTRIB_CODENAME=xenial
DISTRIB_DESCRIPTION="Ubuntu 16.04 LTS"
Here is another example using a Centos 7 image:
singularity exec /depot/itap/singularity/centos7.img cat /etc/redhat-release
CentOS Linux release 7.2.1511 (Core)
Link to section 'Purdue Cluster Specific Notes' of 'Singularity' Purdue Cluster Specific Notes
All service providers will integrate Singularity slightly differently depending on site. The largest customization will be which default files are inserted into your images so that routine services will work.
Services we configure for your images include DNS settings and account information. File systems we overlay into your images are your home directory, scratch, Data Depot, and application file systems.
Here is a list of paths:
- /etc/resolv.conf
- /etc/hosts
- /home/$USER
- /apps
- /scratch
- /depot
This means that within the container environment these paths will be present and the same as outside the container. The /apps
, /scratch
, and /depot
directories will need to exist inside your container to work properly.
Link to section 'Creating Singularity Images' of 'Singularity' Creating Singularity Images
Due to how singularity containers work, you must have root privileges to build an image. Once you have a singularity container image built on your own system, you can copy the image file up to the cluster (you do not need root privileges to run the container).
You can find information and documentation for how to install and use singularity on your system:
We have version 3.8.0-1.el7
on the cluster. You will most likely not be able to run any container built with any singularity past that version. So be sure to follow the installation guide for version 3.8 on your system.
singularity --version
singularity version 3.8.0-1.el7
Everything you need on how to build a container is available from their user-guide. Below are merely some quick tips for getting your own containers built for Scholar.
You can use a Definition File to both build your container and share its specification with collaborators (for the sake of reproducibility). Here is a simplistic example of such a file:
# FILENAME: Buildfile
Bootstrap: docker
From: ubuntu:18.04
%post
apt-get update && apt-get upgrade -y
mkdir /apps /depot /scratch
To build the image itself:
sudo singularity build ubuntu-18.04.sif Buildfile
The challenge with this approach however is that it must start from scratch if you decide to change something. In order to create a container image iteratively and interactively, you can use the --sandbox
option.
sudo singularity build --sandbox ubuntu-18.04 docker://ubuntu:18.04
This will not create a flat image file but a directory tree (i.e., a folder), the contents of which are the container's filesystem. In order to get a shell inside the container that allows you to modify it, user the --writable
option.
sudo singularity shell --writable ubuntu-18.04
Singularity: Invoking an interactive shell within container...
Singularity ubuntu-18.04.sandbox:~>
You can then proceed to install any libraries, software, etc. within the container. Then to create the final image file, exit
the shell and call the build
command once more on the sandbox.
sudo singularity build ubuntu-18.04.sif ubuntu-18.04
Finally, copy the new image to Scholar and run it.
Windows
Windows virtual machines (VMs) are supported as batch jobs on HPC systems. This section illustrates how to submit a job and run a Windows instance in order to run Windows applications on the high-performance computing systems.
The following images are pre-configured and made available by staff:
- Windows 2016 Server Basic (minimal software pre-loaded)
- Windows 2016 Server GIS (GIS Software Stack pre-loaded)
The Windows VMs can be launched in two fashions:
- Menu Launcher - Point and click to start
- Command Line - Advanced and customized usage
Click each of the above links for detailed instructions on using them.
Link to section 'Software Provided in Pre-configured Virtual Machines' of 'Windows' Software Provided in Pre-configured Virtual Machines
The Windows 2016 Base server image available on Scholar has the following software packages preloaded:
- Anaconda Python 2 and Python 3
- JMP 13
- Matlab R2017b
- Microsoft Office 2016
- Notepad++
- NVivo 12
- Rstudio
- Stata SE 15
- VLC Media Player
Command line
If you wish to work with Windows VMs on the command line or work into scripted workflows you can interact directly with the Windows system:
Copy a Windows 2016 Server VM image to your storage. Scratch or Research Data Depot are good locations to save a VM image. If you are using scratch, remember that scratch spaces are temporary, and be sure to safely back up your disk image somewhere permanent, such as Research Data Depot or Fortress. To copy a basic image:
$ cp /apps/external/apps/windows/images/latest.qcow2 $RCAC_SCRATCH/windows.qcow2
To copy a GIS image:
$ cp /depot/itap/windows/gis/2k16.qcow2 $RCAC_SCRATCH/windows.qcow2
To launch a virtual machine in a batch job, use the "windows" script, specifying the path to your Windows virtual machine image. With no other command-line arguments, the windows script will autodetect a number cores and memory for the Windows VM. A Windows network connection will be made to your home directory. To launch:
$ windows -i $RCAC_SCRATCH/windows.qcow2
Link to section 'Command line options:' of 'Command line' Command line options:
-i <path to qcow image file> (For example, $RCAC_SCRATCH/windows-2k16.qcow2)
-m <RAM>G (For example, 32G)
-c <cores> (For example, 20)
-s <smbpath> (UNIX Path to map as a drive, for example, $RCAC_SCRATCH)
-b (If present, launches VM in background. Use VNC to connect to Windows.)
To launch a virtual machine with 32GB of RAM, 20 cores, and a network mapping to your home directory:
$ windows -i /path/to/image.qcow2 -m 32G -c 20 -s $HOME
To launch a virtual machine with 16GB of RAM, 10 cores, and a network mapping to your Data Depot space:
$ windows -i /path/to/image.qcow2 -m 16G -c 10 -s /depot/mylab
The Windows 2016 server desktop will open, and automatically log in as an administrator, so that you can install any software into the Windows virtual machine that your research requires. Changes to the image will be stored in the file specified with the -i option.
Menu Launcher
Windows VMs can be easily launched through the login/thinlinc">Thinlinc remote desktop environment.
- Log in via login/thinlinc">Thinlinc.
- Click on Applications menu in the upper left corner.
- Look under the Cluster Software menu.
- The "Windows 10" launcher will launch a VM directly on the front-end.
- Follow the dialogs to set up your VM.
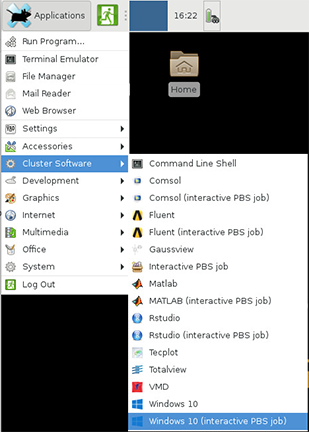
The dialog menus will walk you through setting up and loading your VM.
- You can choose to create a new image or load a saved image.
- New VMs should be saved on Scratch or Research Data Depot as they are too large for Home Directories.
- If you are using scratch, remember that scratch spaces are temporary, and be sure to safely back up your disk image somewhere permanent, such as Research Data Depot or Fortress.
You will also be prompted to select a storage space to mount on your image (Home, Scratch, or Data Depot). You can only choose one to be mounted. It will appear on a shortcut on the desktop once the VM loads.
Link to section 'Notes' of 'Menu Launcher' Notes
Using the menu launcher will launch automatically select reasonable CPU and memory values. If you wish to choose other options or work Windows VMs into scripted workflows see the section on using the command line.
NGC (Nvidia GPU Cloud)
Link to section 'What is NGC?' of 'NGC (Nvidia GPU Cloud)' What is NGC?
Nvidia GPU cloud (NGC) is a GPU-accelerated cloud platform optimized for deep learning and scientific computing. NGC offers a comprehensive catalogue of GPU-accelerated containers, so the application runs quickly and reliably on the high performance computing environment. NGC was deployed to extend the cluster capabilities and to enable powerful software and deliver the fastest results. By utilizing Singularity and NGC, users can focus on building lean models, producing optimal solutions and gathering faster insights. For more information, please visit https://www.nvidia.com/en-us/gpu-cloud and NGC software catalog.
Link to section 'Getting Started' of 'NGC (Nvidia GPU Cloud)' Getting Started
Users can download containers from the NGC software catalog and run them directly using Singularity instructions from the corresponding container’s catalog page.
In addition, a subset of pre-downloaded NGC containers wrapped into convenient software modules are provided. These modules wrap underlying complexity and provide the same commands that are expected from non-containerized versions of each application.
On Scholar, type the command below to see the lists of NGC containers we deployed.
$ module load ngc
$ module avail
Link to section 'Example' of 'NGC (Nvidia GPU Cloud)' Example
This example demonstrates how to run LAMMPS with NGC modules.
First, let's prepare the run folder and download the input file for the example we are going to run.
$ cd $CLUSTER_SCRATCH
$ mkdir -p lammps_ngc
$ cd lammps_ngc
$ wget https://lammps.sandia.gov/inputs/in.lj.txt
Then ssh to gpu and load cuda, ngc and lammps modules
$ ssh gpu.scholar.rcac.purdue.edu
$ module load cuda
$ module load ngc
$ module load lammps/29Oct2020
Finally we can set variables and start running lammps.
$ gpu_count=1
$ input=in.lj.txt
$ mpirun -n ${gpu_count} lmp -k on g ${gpu_count} -sf kk -pk kokkos cuda/aware on neigh full comm device binsize 2.8 -var x 8 -var y 4 -var z 8 -in ${input}
For more information, see each application’s NGC catalog page . For applications deployed as modules, see module help command for direct link to the relevant page (e.g. module help lammps/29Oct2020 in the above example).
BioContainers Collection
Link to section 'What is BioContainers?' of 'BioContainers Collection' What is BioContainers?
The BioContainers project came from the idea of using the containers-based technologies such as Docker or rkt for bioinformatics software. Having a common and controllable environment for running software could help to deal with some of the current problems during software development and distribution. BioContainers is a community-driven project that provides the infrastructure and basic guidelines to create, manage and distribute bioinformatics containers with a special focus on omics fields such as proteomics, genomics, transcriptomics and metabolomics. . For more information, please visit BioContainers project.
Link to section ' Getting Started ' of 'BioContainers Collection' Getting Started
Users can download bioinformatic containers from the BioContainers.pro and run them directly using Singularity instructions from the corresponding container’s catalog page.
Brief Singularity guide and examples are available at the Scholar Singularity user guide page. Detailed Singularity user guide is available at: sylabs.io/guides/3.8/user-guide
In addition, a subset of pre-downloaded biocontainers wrapped into convenient software modules are provided. These modules wrap underlying complexity and provide the same commands that are expected from non-containerized versions of each application.
On Scholar, type the command below to see the lists of biocontainers we deployed.
module load biocontainers
module avail
------------ BioContainers collection modules -------------
bamtools/2.5.1
beast2/2.6.3
bedtools/2.30.0
blast/2.11.0
bowtie2/2.4.2
bwa/0.7.17
cufflinks/2.2.1
deeptools/3.5.1
fastqc/0.11.9
faststructure/1.0
htseq/0.13.5
[....]
Link to section ' Example ' of 'BioContainers Collection' Example
This example demonstrates how to run BLASTP with the blast module. This blast module is a biocontainer wrapper for NCBI BLAST.
module load biocontainers
module load blast
blastp -query query.fasta -db nr -out output.txt -outfmt 6 -evalue 0.01
To run a job in batch mode, first prepare a job script that specifies the BioContainer modules you want to launch and the resources required to run it. Then, use the sbatch
command to submit your job script to Slurm. The following example shows the job script to use Bowtie2 in bioinformatic analysis.
#!/bin/bash
#SBATCH -A myqueuename
#SBATCH -o bowtie2_%j.txt
#SBATCH -e bowtie2_%j.err
#SBATCH --nodes=1
#SBATCH --ntasks-per-node=1
#SBATCH --cpus-per-task=8
#SBATCH --time=1:30:00
#SBATCH --job-name bowtie2
# Load the Bowtie module
module load biocontainers
module load bowtie2
# Indexing a reference genome
bowtie2-build ref.fasta ref
# Aligning paired-end reads
bowtie2 -p 8 -x ref -1 reads_1.fq -2 reads_2.fq -S align.sam
To help users get started, we provided detailed user guides for each containerized bioinformatics module on the ReadTheDocs platform
Ansys Fluent
Ansys is a CAE/multiphysics engineering simulation software that utilizes finite element analysis for numerically solving a wide variety of mechanical problems. The software contains a list of packages and can simulate many structural properties such as strength, toughness, elasticity, thermal expansion, fluid dynamics as well as acoustic and electromagnetic attributes.
Link to section 'Ansys Licensing' of 'Ansys Fluent' Ansys Licensing
The Ansys licensing on our community clusters is maintained by Purdue ECN group. There are two types of licenses: teaching and research. For more information, please refer to ECN Ansys licensing page. If you are interested in purchasing your own research license, please send email to software@ecn.purdue.edu.
Link to section 'Ansys Workflow' of 'Ansys Fluent' Ansys Workflow
Ansys software consists of several sub-packages such as Workbench and Fluent. Most simulations are performed using the Ansys Workbench console, a GUI interface to manage and edit the simulation workflow. It requires X11 forwarding for remote display so a SSH client software with X11 support or a remote desktop portal is required. Please see Logging In section for more details. To ensure preferred performance, ThinLinc remote desktop connection is highly recommended.
Typically users break down larger structures into small components in geometry with each of them modeled and tested individually. A user may start by defining the dimensions of an object, adding weight, pressure, temperature, and other physical properties.
Ansys Fluent is a computational fluid dynamics (CFD) simulation software known for its advanced physics modeling capabilities and accuracy. Fluent offers unparalleled analysis capabilities and provides all the tools needed to design and optimize new equipment and to troubleshoot existing installations.
In the following sections, we provide step-by-step instructions to lead you through the process of using Fluent. We will create a classical elbow pipe model and simulate the fluid dynamics when water flows through the pipe. The project files have been generated and can be downloaded via fluent_tutorial.zip.
Link to section 'Loading Ansys Module' of 'Ansys Fluent' Loading Ansys Module
Different versions of Ansys are installed on the clusters and can be listed with module spider
or module avail
command in the terminal.
$ module avail ansys/
---------------------- Core Applications -----------------------------
ansys/2019R3 ansys/2020R1 ansys/2021R2 ansys/2022R1 (D)
Before launching Ansys Workbench, a specific version of Ansys module needs to be loaded. For example, you can module load ansys/2021R2
to use the latest Ansys 2021R2. If no version is specified, the default module -> (D) (ansys/2022R1
in this case) will be loaded. You can also check the loaded modules with module list
command.
Link to section 'Launching Ansys Workbench' of 'Ansys Fluent' Launching Ansys Workbench
Open a terminal on Scholar, enter rcac-runwb2
to launch Ansys Workbench.
You can also use runwb2
to launch Ansys Workbench. The main difference between runwb2
and rcac-runwb2
is that the latter sets the project folder to be in your scratch space. Ansys has an known bug that it might crash when the project folder is set to $HOME
on our systems.
Preparing Case Files for Fluent
Link to section 'Creating a Fluent fluid analysis system' of 'Preparing Case Files for Fluent' Creating a Fluent fluid analysis system
In the Ansys Workbench, create a new fluid flow analysis by double-clicking the Fluid Flow (Fluent) option under the Analysis Systems in the Toolbox on the left panel. You can also drag-and-drop the analysis system into the Project Schematic. A green dotted outline indicating a potential location for the new system initially appears in the Project Schematic. When you drag the system to one of the outlines, it turns into a red box to indicate the chosen location of the new system.
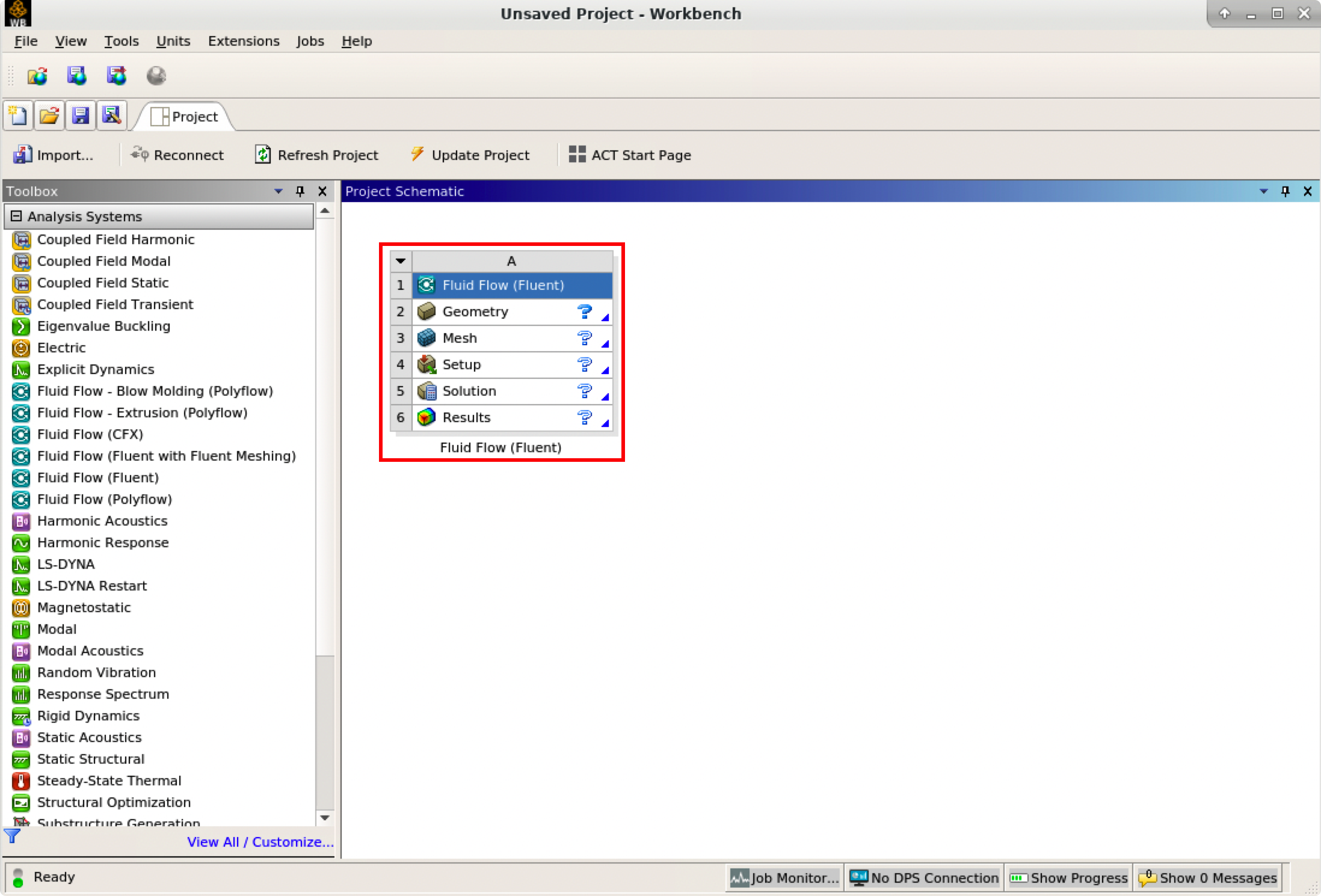
The red rectangle indicates the Fluid Flow system for Fluent, which includes all the essential workflows from “2 Geometry” to “6 Results”. You can rename it and carry out the necessary step-by-step procedures by double-clicking the corresponding cells.
It is important to save the project. Ansys Workbench saves the project with a .wbpj
extension and also all the supporting files into a folder with the same name. In this case, a file named elbow_demo.wbpj
and a folder $Ansys_PROJECT_FOLDER/elbow_demo_files/
are created in the Ansys project folder:
$ ll
total 33
drwxr-xr-x 7 myusername itap 9 Mar 3 17:47 elbow_demo_files
-rw-r--r-- 1 myusername itap 42597 Mar 3 17:47 elbow_demo.wbpj
You should always “Update Project” and save it after finishing a procedure.
Link to section 'Creating Geometry in the Ansys DesignModeler' of 'Preparing Case Files for Fluent' Creating Geometry in the Ansys DesignModeler
Create a geometry in the Ansys DesignModeler (by double-clicking “Geometry” cell in workflow), or import the appropriate geometry file (by right-clicking the Geometry cell and selecting “Import Geometry” option from the context menu).
You can use Ansys DesignModeler to create 2D/3D geometries or even draw the objects yourself. In our example, we created only half of the elbow pipe because the symmetry of the structure is taken into account to reduce the computation intensity.
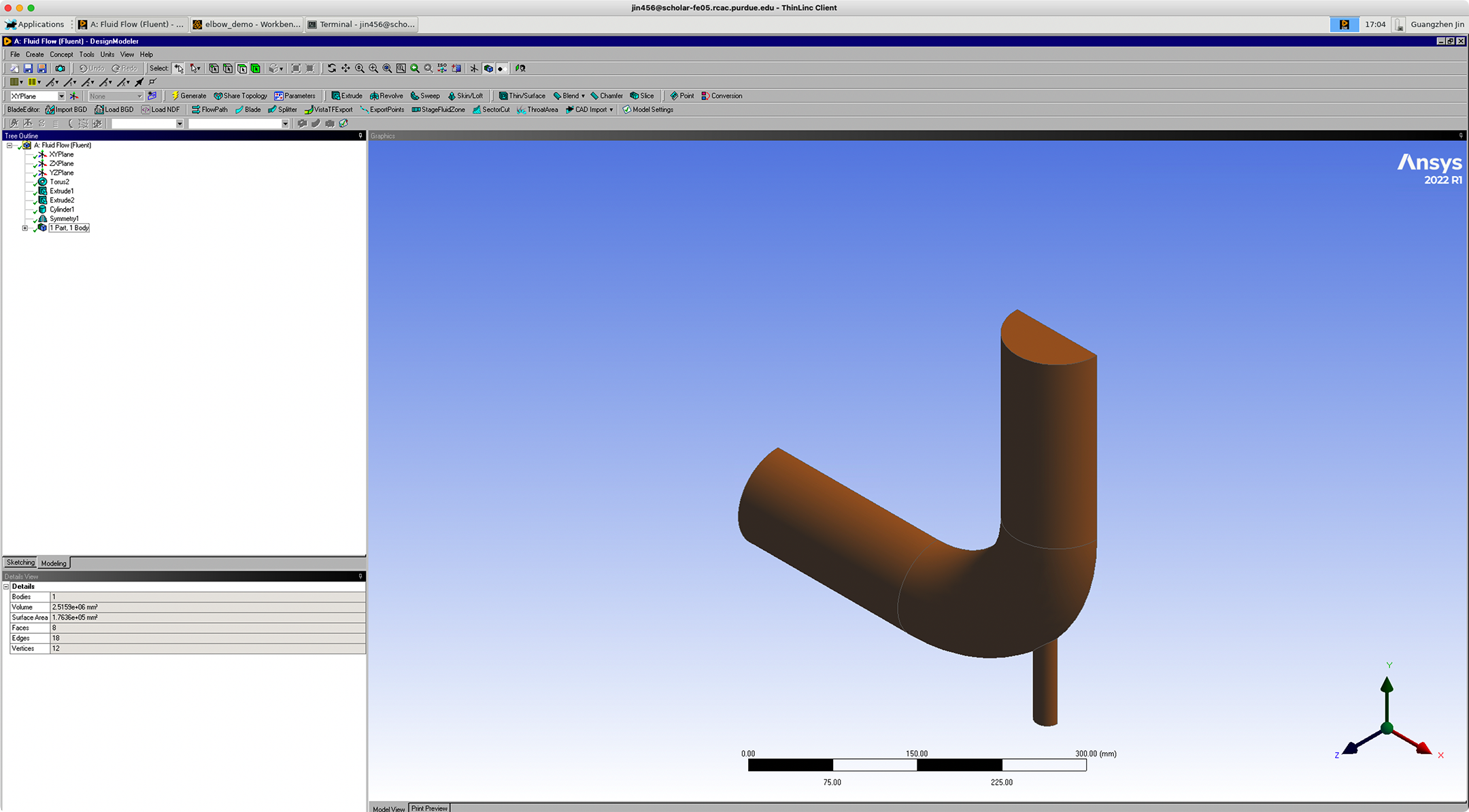
After saving the geometry, a geometry file FFF.agdb
will be created in the folder: $Ansys_PROJECT_FOLDER/elbow_demo_file/dp0/FFF/DM/
. The project in Workbench will be updated automatically.
If you import a pre-existing geometry into Ansys DesignModeler, it will also generate this file with the same filename at this location.
Link to section 'Creating mesh in the Ansys Meshing' of 'Preparing Case Files for Fluent' Creating mesh in the Ansys Meshing
Now that we have created the elbow pipe geometry, a computational mesh can be generated by the Meshing application throughout the flow volume.
With the successful creation of the geometry, there should be a green check showing the completion of “Geometry” in the Ansys Workbench. A Refresh Required icon within the “Mesh” cell indicates the mesh needs to be updated and refreshed for the system.
Then it’s time to open the Ansys Meshing application by double-clicking the “Mesh” cell and editing the mesh for the project. Generally, there are several steps we need to take to define the mesh:
- Create names for all geometry boundaries such as the inlets, outlets and fluid body. Note: You can use the strings “velocity inlet” and “pressure outlet” in the named selections (with or without hyphens or underscore characters) to allow Ansys Fluent to automatically detect and assign the corresponding boundary types accordingly. Use “Fluid” for the body to let Ansys Fluent automatically detect that the volume is a fluid zone and treat it accordingly.
- Set basic meshing parameters for the Ansys Meshing application. Here are several important parameters you may need to assign: Sizing, Quality, Body Sizing Control, Inflation.
- Select “Generate” to generate the mesh and “Update” to update the mesh into the system. Note: Once the mesh is generated, you can view the mesh statistics by opening the Statistics node in the Details of “Mesh” view. This will display information such as the number of nodes and the number of elements, which gives you a general idea for the future computational resources and time.
After generation and updating the mesh, a mesh file FFF.msh
will be generated in folder $Ansys_PROJECT_FOLDER/elbow_demo_file/dp0/FFF/MECH/
and a mesh database file FFF.mshdb
will be generated in folder $Ansys_PROJECT_FOLDER/elbow_demo_file/dp0/global/MECH/
.
Parameters used in demo case (use default if not assigned):
- Length Unit=”mm”
- Names defined for geometry:
- velocity-inlet-large (large inlet on pipe);
- velocity-inlet-small (small inlet on pipe);
- pressure-outlet (outlet on pipe);
- symmetry (symmetry surface);
- Fluid (body);
- Mesh:
- Quality: Smoothing=”high”;
- Inflation: Use Automatic Inflation=“Program Controlled”, Inflation Option=”Smooth Transition”;
- Statistics:
- Nodes=29371;
- Elements=87647.
Link to section 'Calculation with Fluent' of 'Preparing Case Files for Fluent' Calculation with Fluent
Now all the preparations have been ready for the numerical calculation in Ansys Fluent. Both “Geometry” and “Mesh” cells should have green checks on. We can set up the CFD simulation parameters in Ansys Fluent by double-clicking the “Setup” cell.
When Ansys Fluent is first started or by selecting “editing” on the “Setup” cell, the Fluent Launcher is displayed, enabling you to view and/or set certain Ansys Fluent start-up options (e.g. Precision, Parallel, Display). Note that “Dimension” is fixed to “3D” because we are using a 3D model in this project.
After the Fluent is opened, an Ansys Fluent settings file FFF.set
is written under the folder $Ansys_PROJECT_FOLDER/elbow_demo_file/dp0/FFF/Fluent/
.
Then we are going to set up all the necessary parameters for Fluent computation. Here are the key steps for the setup:
- Setting up the domain:
- Change the units for length to be consistent with the Mesh;
- Check the mesh statistics and quality;
- Setting up physics:
- Solver: “Energy”, “Viscous Model”, “Near-Wall Treatment”;
- Materials;
- Zones;
- Boundaries: Inlet, Outlet, Internal, Symmetry, Wall;
- Solving:
- Solution Methods;
- Reports;
- Initialization;
- Iterations and output frequency.
Then the calculation will be carried out and the results will be written out into FFF-1.cas.gz
under folder $Ansys_PROJECT_FOLDER/elbow_demo_file/dp0/FFF/Fluent/
.
This file contains all the settings and simulation results which can be loaded for post analysis and re-computation (more details will be introduced in the following sections). If only configurations and settings within the Fluent are needed, we can open independent Fluent or submit Fluent jobs with bash commands by loading the existing case in order to facilitate the computation process.
Parameters used in demo case (use default if not assigned):
- Domain Setup: Length Units=”mm”;
- Solver: Energy=”on”; Viscous Model=”k-epsilon”; Near-Wall Treatment=”Enhanced Wall Treatment”;
- Materials: water (Density=1000[kg/m^3]; Specific Heat=4216[J/kg-k]; Thermal Conductivity=0.677[w/m-k]; Viscosity=8e-4[kg/m-s]);
- Zones=”fluid (water)”;
- Inlet=”velocity-inlet-large” (Velocity Magnitude=0.4m/s, Specification Method=”Intensity and Hydraulic Diameter”, Turbulent Intensity=5%; Hydraulic Diameter=100mm; Thermal Temperature=293.15k) &”velocity-inlet-small” (Velocity Magnitude=1.2m/s, Specification Method=”Intensity and Hydraulic Diameter”, Turbulent Intensity=5%; Hydraulic Diameter=25mm; Thermal Temperature=313.15k); Internal=”interior-fluid”; Symmetry=”symmetry”; Wall=”wall-fluid”;
- Solution Methods: Gradient=”Green-Gauss Node Based”;
- Report: plot residual and “Facet Maximum” for “pressure-outlet”
- Hybrid Initialization;
- 300 iterations.
Case Calculating with Fluent
Link to section 'Calculation with Fluent' of 'Case Calculating with Fluent' Calculation with Fluent
Now all the files are ready for the Fluent calculations. Both “Geometry” and “Mesh” cells should have green checks. We can set up the CFD simulation parameters in the Ansys Fluent by double-clicking the “Setup” cell.
Ansys Fluent Launcher can be started by selecting “editing” on the “Setup” cell with many startup options (e.g. Precision, Parallel, Display). Note that “Dimension” is fixed to “3D” because we are using a 3D model in this project.
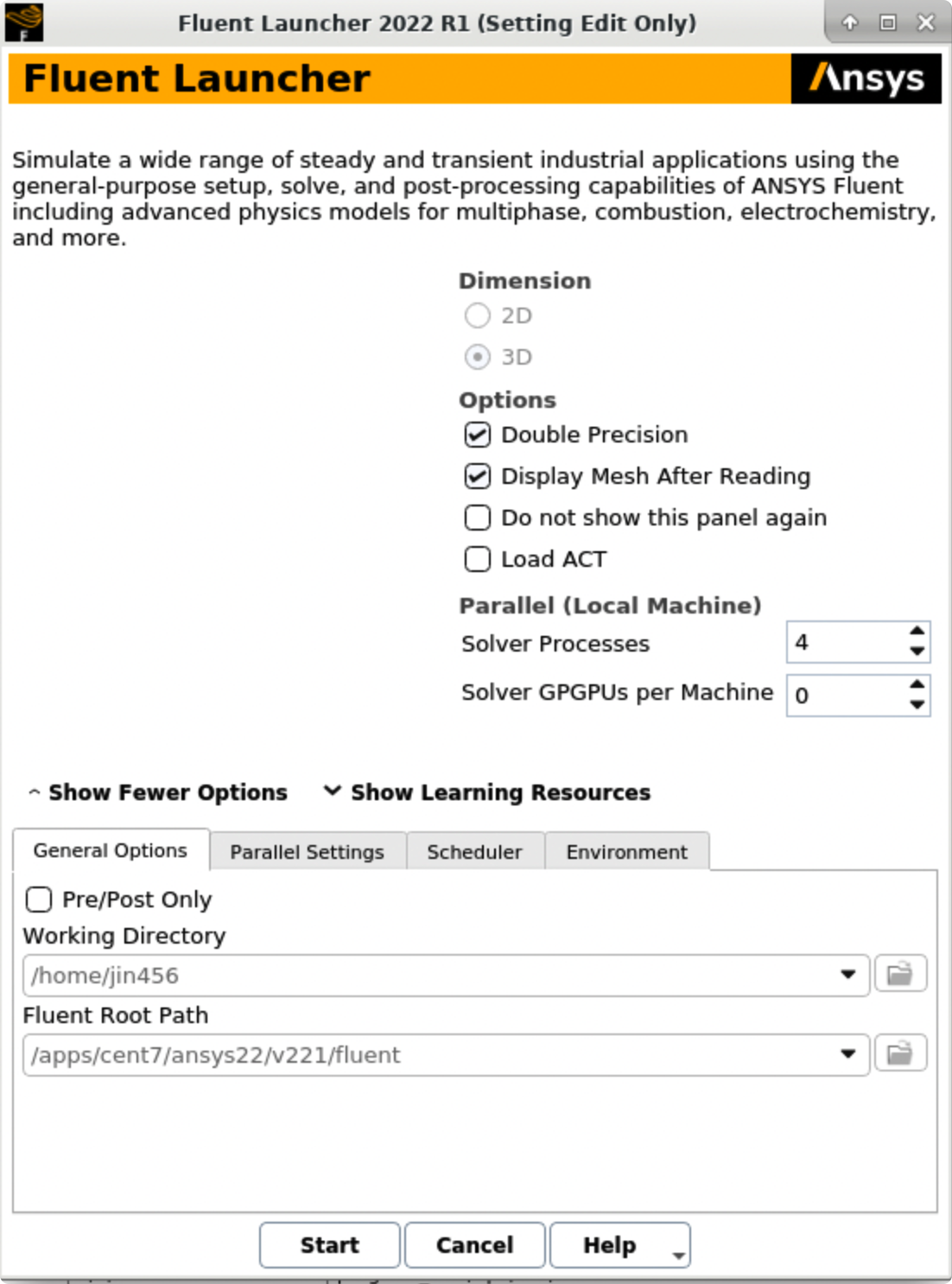
After the Fluent is opened, an Ansys Fluent settings file FFF.set
is written under the folder $Ansys_PROJECT_FOLDER/elbow_demo_file/dp0/FFF/Fluent/
.
Then we are going to set up all the necessary parameters for Fluent computation. Here are the key steps for the setup:
- Setting up the domain:
- Change the units for length to be consistent with the Mesh;
- Check the mesh statistics and quality;
- Setting up physics:
- Solver: “Energy”, “Viscous Model”, “Near-Wall Treatment”;
- Materials;
- Zones;
- Boundaries: Inlet, Outlet, Internal, Symmetry, Wall;
- Solving:
- Solution Methods;
- Reports;
- Initialization;
- Iterations and output frequency.
Then the calculation will be carried out and the results will be written out into FFF-1.cas.gz
under folder $Ansys_PROJECT_FOLDER/elbow_demo_file/dp0/FFF/Fluent/
.
This file contains all the settings and simulation results which can be loaded for post analysis and re-computation (more details will be introduced in the following sections). If only configurations and settings within the Fluent are needed, we can open independent Fluent or submit Fluent jobs with bash commands by loading the existing case in order to facilitate the computation process.
Parameters used in demo case (use default if not assigned):
- Domain Setup: Length Units=”mm”;
- Solver: Energy=”on”; Viscous Model=”k-epsilon”; Near-Wall Treatment=”Enhanced Wall Treatment”;
- Materials: water (Density=1000[kg/m^3]; Specific Heat=4216[J/kg-k]; Thermal Conductivity=0.677[w/m-k]; Viscosity=8e-4[kg/m-s]);
- Zones=”fluid (water)”;
- Inlet=”velocity-inlet-large” (Velocity Magnitude=0.4m/s, Specification Method=”Intensity and Hydraulic Diameter”, Turbulent Intensity=5%; Hydraulic Diameter=100mm; Thermal Temperature=293.15k) &”velocity-inlet-small” (Velocity Magnitude=1.2m/s, Specification Method=”Intensity and Hydraulic Diameter”, Turbulent Intensity=5%; Hydraulic Diameter=25mm; Thermal Temperature=313.15k); Internal=”interior-fluid”; Symmetry=”symmetry”; Wall=”wall-fluid”;
- Solution Methods: Gradient=”Green-Gauss Node Based”;
- Report: plot residual and “Facet Maximum” for “pressure-outlet”
- Hybrid Initialization;
- 300 iterations.
Link to section 'Results analysis' of 'Case Calculating with Fluent' Results analysis
The best methods to view and analyze the simulation should be the Ansys Fluent (directly after computation) or the Ansys CFD-Post (entering “Results” in Ansys Workbench). Both methods are straightforward so we will not cover this part in this tutorial. Here is a final simulation result showing the temperature of the symmetry after 300 iterations for reference:
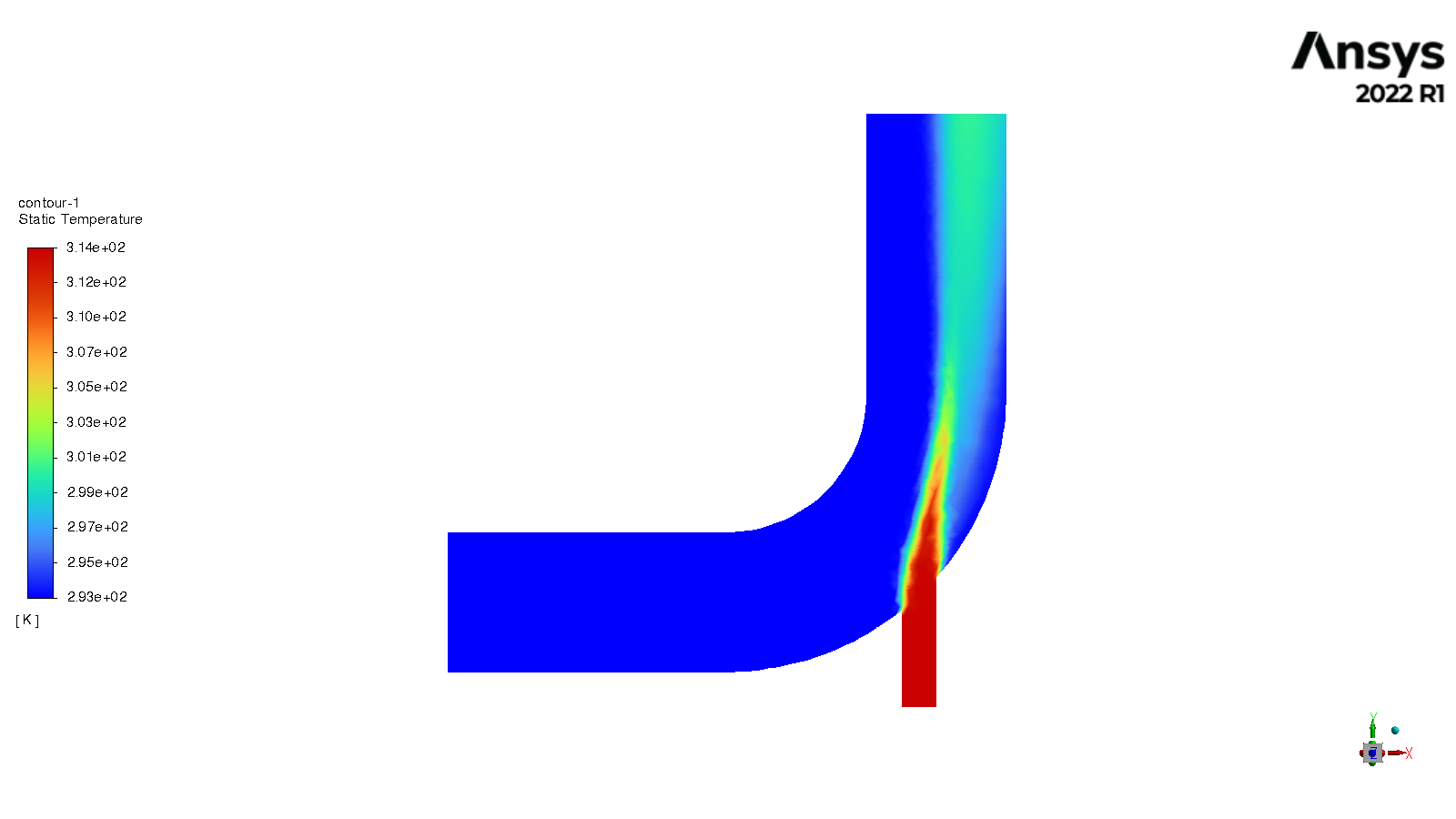
Fluent Text User Interface and Journal File
Link to section 'Fluent Text User Interface (TUI)' of 'Fluent Text User Interface and Journal File' Fluent Text User Interface (TUI)
If you pay attention to the “Console” window in the Fluent window when setting up and carrying out the calculation, corresponding commands can be found and executed one after another. Almost all the setting processes can be accomplished by the command lines, which is called Fluent Text User Interface (TUI). Here are the main commands in Fluent TUI:
adjoint/ parallel/ solve/
define/ plot/ surface/
display/ preferences/ turbo-workflow/
exit print-license-usage views/
file/ report/
mesh/ server/
For example, instead of opening a case by clicking buttons in Ansys Fluent, we can type /file read-case case_file_name.cas.gz
to open the saved case.
Link to section 'Fluent Journal Files' of 'Fluent Text User Interface and Journal File' Fluent Journal Files
A Fluent journal file is a series of TUI commands stored in a text file. The file can be written in a text editor or generated by Fluent as a transcript of the commands given to Fluent during your session.
A journal file generated by Fluent will include any GUI operations (in a TUI form, though). This is quite useful if you have a series of tasks that you need to execute, as it provides a shortcut. To record a journal file, start recording with File -> Write -> Start Journal..., perform whatever tasks you need, and then stop recording with File -> Write -> Stop Journal...
You can also write your own journal file into a text file. The basic rule for a Fluent journal file is to reproduce the TUI commands that controlled the configuration and calculation of Fluent in their order. You can add a comment in a line starting with a ;
(semicolon).
Here are some reasons why you should use a Fluent journal file:
- Using journal files with bash scripting can allow you to automate your jobs.
- Using journal files can allow you to parameterize your models easily and automatically.
- Using a journal file can set parameters you do not have in your case file e.g. autosaving.
- Using a journal file can allow you to safely save, stop and restart your jobs easily.
The order of your journal file commands is highly important. The correct sequences must be followed and some stages have multiple options e.g. different initialization methods.
Here is a sample Fluent journal file for the demo case:
;testJournal.jou
;Set the TUI version for Fluent
/file/set-tui-version "22.1"
;Read the case. The default folder
/file read-case /home/jin456/Fluent_files/tutorial_case1/elbow_files/dp0/FFF/Fluent/FFF-1.cas.gz
;Initialize the case with Hybrid Initialization
/solve/initialize/hyb-initialization
;Set Number of Iterations to 1000, Reporting Interval to 10 iterations and Profile Update Interval to 1 iteration
/solve/iterate 1000 10 1
;Outputting solver performance data upon completion of the simulation
/parallel timer usage
;Write out the simulation results.
/file write-case-data /home/jin456/Fluent_files/tutorial_case1/elbow_files/dp0/FFF/Fluent/result.cas.h5
;After computation, exit Flent
/exit
Before running this Fluent journal file, you need to make sure: 1) the ansys module has been loaded (it’s highly recommended to load the same version of Ansys when you built the case project); 2) the project case file (***.cas.gz
) has been created.
Then we can use Fluent to run this journal file by simply using:fluent 3ddp -t$NTASKS -g -i testJournal.jou
in the terminal. Here, 3d
indicates this is a 3d model, dp
indicates double precision, -t$NTASKS
tells Fluent how many Solver Processes it will take (e.g. -t4
), -g
means to run without the GUI or graphics, -i
testJournal.jou tells Fluent to read the specific journal file.
Here is a table for the available command line Options for Linux/UNIX and Windows Platforms in Ansys Fluent.
Option | Platform | Description |
---|---|---|
-cc |
all | Use the classic color scheme |
-ccp x |
Windows only | Use the Microsoft Job Scheduler where x is the head node name. |
-cnf=x |
all | Specify the hosts or machine list file |
-driver |
all | Sets the graphics driver (available drivers vary by platform - opengl or x11 or null(Linux/UNIX) - opengl or msw or null (Windows)) |
-env |
all | Show environment variables |
-fgw |
all | Disables the embedded graphics |
-g |
all | Run without the GUI or graphics (Linux/UNIX); Run with the GUI minimized (Windows) |
-gr |
all | Run without graphics |
-gu |
all | Run without the GUI but with graphics (Linux/UNIX); Run with the GUI minimized but with graphics (Windows) |
-help |
all | Display command line options |
-hidden |
Windows only | Run in batch mode |
-host_ip=host:ip |
all | Specify the IP interface to be used by the host process |
-i journal |
all | Reads the specified journal file |
-lsf |
Linux/UNIX only | Run FLUENT using LSF |
-mpi= |
all | Specify MPI implementation |
-mpitest |
all | Will launch an MPI program to collect network performance data |
-nm |
all | Do not display mesh after reading |
-pcheck |
Linux/UNIX only | Checks all nodes |
-post |
all | Run the FLUENT post-processing-only executable |
-p |
all | Choose the interconnect |
-r |
all | List all releases installed |
-rx |
all | Specify release number |
-sge |
Linux/UNIX only | Run FLUENT under Sun Grid Engine |
-sge queue |
Linux/UNIX only | Name of the queue for a given computing grid |
-sgeckpt ckpt_obj |
Linux/UNIX only | Set checkpointing object to ckpt_objfor SGE |
-sgepe fluent_pe min_n-max_n |
Linux/UNIX only | Set the parallel environment for SGE to fluent_pe, min_nand max_n are number of min and max nodes requested |
-tx |
all | Specify the number of processors x |
For more information for Fluent text user interface and journal files, please refer to Fluent FAQ.
Submitting Fluent jobs to SLURM
The Fluent simulations can also run in batch. In this section we provide an example script for submitting Fluent jobs to the SLURM scheduler. Please refer to the Running Jobs section of our user guide for detailed tutorials of submitting jobs.
#!/bin/bash
# Job script for submitting a FLUENT job on multiple cores on a single node
# Apply resources via SLURM
#SBATCH --nodes=1
#SBATCH --ntasks=4
#SBATCH --time=01:00:00
#SBATCH --job-name=fluent_test
#SBATCH -o fluent_test_%j.out
#SBATCH -e fluent_test_%j.err
# Loads Ansys and sets the application up
module purge
module load ansys/2022R1
#Initiating Fluent and reading input journal file
fluent 3ddp -t$NTASKS -g -i testJournal.jou
For more information about submitting Fluent jobs, please refer to Fluent FAQ .
Using Jupyter Hub on Scholar
JupyterHub is a multi-user Hub that spawns, manages, and proxies multiple instances of the single-user Jupyter notebook server. JupyterHub can be used to serve notebooks to a class of students, a corporate data science group, or a scientific research group.
Users can use the Open OnDemand (https://gateway.scholar.rcac.purdue.edu/) portal to launch private Jupyter servers on the Scholar compute nodes. OOD provides a browser-based interface to launch and run Jupyter notebooks. The old centralized Jupyter server has been deprecated with Scholar's OS upgrade in Dec 2024.
Frequently Asked Questions
Some common questions, errors, and problems are categorized below. Click the Expand Topics link in the upper right to see all entries at once. You can also use the search box above to search the user guide for any issues you are seeing.
About Scholar
Frequently asked questions about Scholar.
Can you remove me from the Scholar mailing list?
Your subscription in the Scholar mailing list is tied to your account on Scholar. If you are no longer using your account on Scholar, your account can be deleted from the My Accounts page. Hover over the resource you wish to remove yourself from and click the red 'X' button. Your account and mailing list subscription will be removed overnight. Be sure to make a copy of any data you wish to keep first.
How is Scholar different than other Community Clusters?
Scholar differs from other Community Clusters in many significant aspects:
- Scholar is a hybrid cluster for teaching courses that require high-performance computing.
- A subset of Scholar front-ends contain Nvidia Tesla V100 accelerator cards. You can access these front ends by logging in to
gpu.scholar.rcac.purdue.edu
. - A subset of Scholar compute nodes contain Nvidia Tesla V100 accelerator cards which can significantly improve performance of compute-intensive workloads. These can be utilized by submitting jobs to the gpu queue (add -A gpu to your job submission command).
- A selection of GPU-enabled application containers from the Nvidia GPU Cloud (NGC) collection is installed.
Do I need to do anything to my firewall to access Scholar?
No firewall changes are needed to access Scholar. However, to access data through Network Drives (i.e., CIFS, "Z: Drive"), you must be on a Purdue campus network or connected through VPN.
Does Scholar have the same home directory as other clusters?
The Scholar home directory and its contents are exclusive to Scholar cluster front-end hosts and compute nodes. This home directory is not available on other RCAC machines but Scholar. There is no automatic copying or synchronization between home directories.
At your discretion you can manually copy all or parts of your main research computing home to Scholar using one of the suggested methods.
If you plan to use hsi
or htar
commands to access Fortress tape archive from Scholar, please see also the keytab generation question for a temporary workaround to a potential caveat, while a permanent mitigation is being developed.
Logging In & Accounts
Frequently asked questions about logging in & accounts.
Errors
Common errors and solutions/work-arounds for them.
/usr/bin/xauth: error in locking authority file
Link to section 'Problem' of '/usr/bin/xauth: error in locking authority file' Problem
I receive this message when logging in:
/usr/bin/xauth: error in locking authority file
Link to section 'Solution' of '/usr/bin/xauth: error in locking authority file' Solution
Your home directory disk quota is full. You may check your quota with myquota
.
You will need to free up space in your home directory.
ncdu
command is a convenient interactive tool to examine disk usage. Consider running ncdu $HOME
to analyze where the bulk of the usage is. With this knowledge, you could then archive your data elsewhere (e.g. your research group's Data Depot space, or Fortress tape archive), or delete files you no longer need.
There are several common locations that tend to grow large over time and are merely cached downloads. The following are safe to delete if you see them in the output of ncdu $HOME
:
/home/myusername/.local/share/Trash
/home/myusername/.cache/pip
/home/myusername/.conda/pkgs
/home/myusername/.singularity/cache
My SSH connection hangs
Link to section 'Problem' of 'My SSH connection hangs' Problem
Your console hangs while trying to connect to a RCAC Server.
Link to section 'Solution' of 'My SSH connection hangs' Solution
This can happen due to various reasons. Most common reasons for hanging SSH terminals are:
- Network: If you are connected over wifi, make sure that your Internet connection is fine.
- Busy front-end server: When you connect to a cluster, you SSH to one of the front-end login nodes. Due to transient user loads, one or more of the front-ends may become unresponsive for a short while. To avoid this, try reconnecting to the cluster or wait until the login node you have connected to has reduced load.
- File system issue: If a server has issues with one or more of the file systems (
home
,scratch
, ordepot
) it may freeze your terminal. To avoid this you can connect to another front-end.
If neither of the suggestions above work, please contact support specifying the name of the server where your console is hung.
Thinlinc session frozen
Link to section 'Problem' of 'Thinlinc session frozen' Problem
Your Thinlinc session is frozen and you can not launch any commands or close the session.
Link to section 'Solution' of 'Thinlinc session frozen' Solution
This can happen due to various reasons. The most common reason is that you ran something memory-intensive inside that Thinlinc session on a front-end, so parts of the Thinlinc session got killed by Cgroups, and the entire session got stuck.
- If you are using a web-version Thinlinc remote desktop (inside the browser):
The web version does not have the capability to kill the existing session, only the standalone client does. Please install the standalone client and follow the steps below:
- If you are using a Thinlinc client:
Close the ThinLinc client, reopen the client login popup, and select
End existing session
.Select "End existing session" and try "Connect" again.
Thinlinc session unreachable
Link to section 'Problem' of 'Thinlinc session unreachable' Problem
When trying to login to Thinlinc and re-connect to your existing session, you receive an error "Your Thinlinc session is currently unreachable".
Link to section 'Solution' of 'Thinlinc session unreachable' Solution
This can happen if the specific login node your existing remote desktop session was residing on is currently offline or down, so Thinlinc can not reconnect to your existing session. Most often the session is non-recoverable at this point, so the solution is to terminate your existing Thinlinc desktop session and start a new one.
- If you are using a web-version Thinlinc remote desktop (inside the browser):
The web version does not have the capability to kill the existing session, only the standalone client does. Please install the standalone client and follow the steps below:
- If you are using a Thinlinc client:
Close the ThinLinc client, reopen the client login popup, and select
End existing session
.Select "End existing session" and try "Connect" again.
How to disable Thinlinc screensaver
Link to section 'Problem' of 'How to disable Thinlinc screensaver' Problem
Your ThinLinc desktop is locked after being idle for a while, and it asks for a password to refresh it. It means the "screensaver" and "lock screen" functions are turned on, but you want to disable these functions.
Link to section 'Solution' of 'How to disable Thinlinc screensaver' Solution
If your screen is locked, close the ThinLinc client, reopen the client login popup, and select End existing session
.
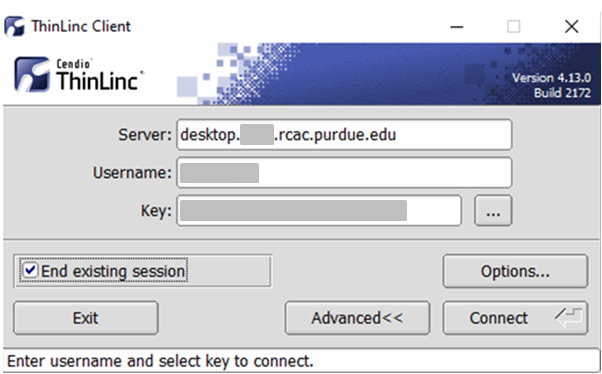
To permanently avoid screen lock issue, right click desktop and select Applications
, then settings
, and select Screensaver
.
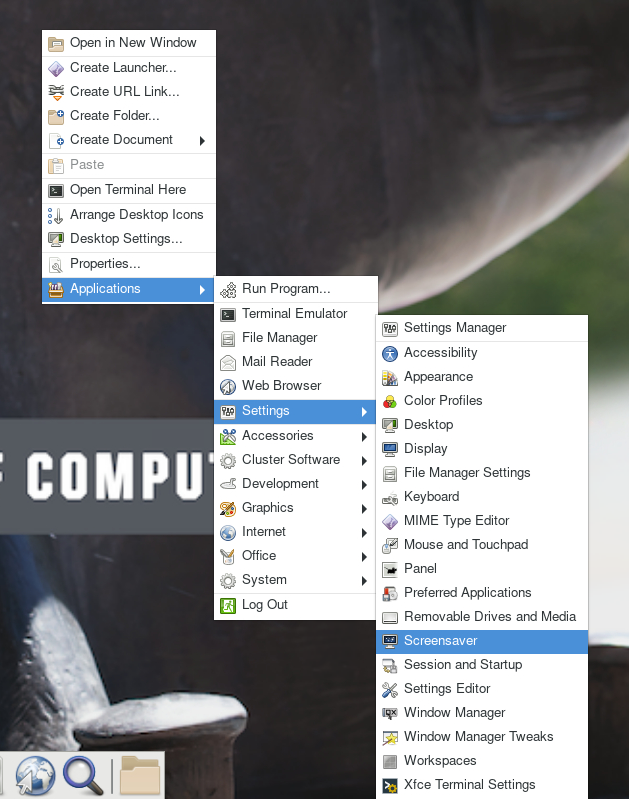
Under Screensaver, turn off the Enable Screensaver
, then under Lock Screen, turn off the Enable Lock Screen
, and close the window.
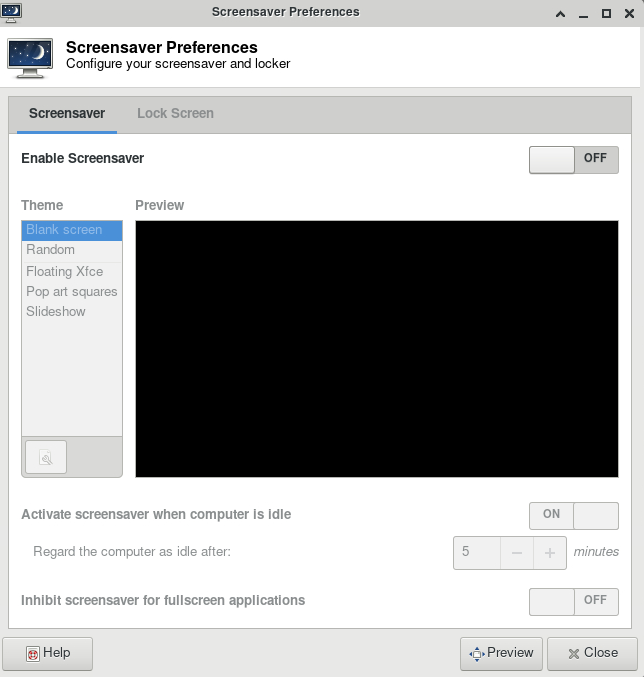
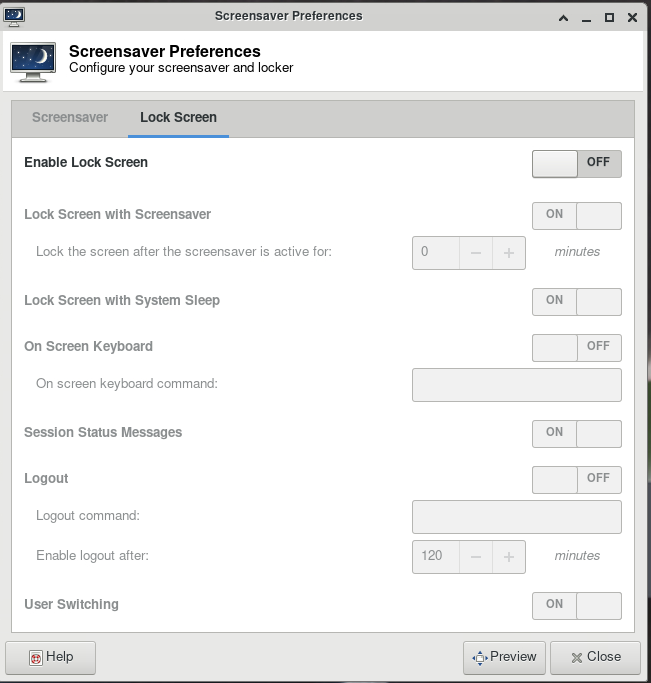
Questions
Frequently asked questions about logging in & accounts.
I worked on Scholar after I graduated/left Purdue, but can not access it anymore
Link to section 'Problem' of 'I worked on Scholar after I graduated/left Purdue, but can not access it anymore' Problem
You have graduated or left Purdue but continue collaboration with your Purdue colleagues. You find that your access to Purdue resources has suddenly stopped and your password is no longer accepted.
Link to section 'Solution' of 'I worked on Scholar after I graduated/left Purdue, but can not access it anymore' Solution
Access to all resources depends on having a valid Purdue Career Account. Expired Career Accounts are removed twice a year, during Spring and October breaks (more details at the official page). If your Career Account was purged due to expiration, you will not be be able to access the resources.
To provide remote collaborators with valid Purdue credentials, the University provides a special procedure called Request for Privileges (R4P). If you need to continue your collaboration with your Purdue PI, the PI will have to submit or renew an R4P request on your behalf.
After your R4P is completed and Career Account is restored, please note two additional necessary steps:
-
Access: Restored Career Accounts by default do not have any RCAC resources enabled for them. Your PI will have to login to the Manage Users tool and explicitly re-enable your access by un-checking and then ticking back checkboxes for desired queues/Unix groups resources.
-
Email: Restored Career Accounts by default do not have their @purdue.edu email service enabled. While this does not preclude you from using RCAC resources, any email messages (be that generated on the clusters, or any service announcements) would not be delivered - which may cause inconvenience or loss of compute jobs. To avoid this, we recommend setting your restored @purdue.edu email service to "Forward" (to an actual address you read). The easiest way to ensure it is to go through the Account Setup process.
Jobs
Frequently asked questions related to running jobs.
Errors
Common errors and potential solutions/workarounds for them.
cannot connect to X server / cannot open display
Link to section 'Problem' of 'cannot connect to X server / cannot open display' Problem
You receive the following message after entering a command to bring up a graphical window
cannot connect to X server
cannot open display
Link to section 'Solution' of 'cannot connect to X server / cannot open display' Solution
This can happen due to multiple reasons:
- Reason: Your SSH client software does not support graphical display by itself (e.g. SecureCRT or PuTTY).
- Solution: Try using a client software like Thinlinc or MobaXterm as described in the SSH X11 Forwarding guide.
-
Reason: You did not enable X11 forwarding in your SSH connection.
-
Solution: If you are in a Windows environment, make sure that X11 forwarding is enabled in your connection settings (e.g. in MobaXterm or PuTTY). If you are in a Linux environment, try
ssh -Y -l username hostname
-
- Reason: If you are trying to open a graphical window within an interactive PBS job, make sure you are using the
-X
option withqsub
after following the previous step(s) for connecting to the front-end. Please see the example in the Interactive Jobs guide. - Reason: If none of the above apply, make sure that you are within quota of your home directory.
bash: command not found
Link to section 'Problem' of 'bash: command not found' Problem
You receive the following message after typing a command
bash: command not found
Link to section 'Solution' of 'bash: command not found' Solution
This means the system doesn't know how to find your command. Typically, you need to load a module to do it.
bash: module command not found
Link to section 'Problem' of 'bash: module command not found' Problem
You receive the following message after typing a command, e.g. module load intel
bash: module command not found
Link to section 'Solution' of 'bash: module command not found' Solution
The system cannot find the module command. You need to source the modules.sh file as below
source /etc/profile.d/modules.sh
or
#!/bin/bash -i
Close Firefox / Firefox is already running but not responding
Link to section 'Problem' of 'Close Firefox / Firefox is already running but not responding' Problem
You receive the following message after trying to launch Firefox browser inside your graphics desktop:
Close Firefox
Firefox is already running, but not responding. To open a new window,
you must first close the existing Firefox process, or restart your system.
Link to section 'Solution' of 'Close Firefox / Firefox is already running but not responding' Solution
When Firefox runs, it creates several lock files in the Firefox profile directory (inside ~/.mozilla/firefox/
folder in your home directory). If a newly-started Firefox instance detects the presence of these lock files, it complains.
This error can happen due to multiple reasons:
- Reason: You had a single Firefox process running, but it terminated abruptly without a chance to clean its lock files (e.g. the job got terminated, session ended, node crashed or rebooted, etc).
- Solution: If you are certain you do not have any other Firefox processes running elsewhere, please use the following command in a terminal window to detect and remove the lock files:
$ unlock-firefox
- Solution: If you are certain you do not have any other Firefox processes running elsewhere, please use the following command in a terminal window to detect and remove the lock files:
- Reason: You may indeed have another Firefox process (in another Thinlinc or Gateway session on this or other cluster, another front-end or compute node). With many clusters sharing common home directory, a running Firefox instance on one can affect another.
- Solution: Try finding and closing running Firefox process(es) on other nodes and clusters.
- Solution: If you must have multiple Firefoxes running simultaneously, you may be able to create separate Firefox profiles and select which one to use for each instance.
Jupyter: database is locked / can not load notebook format
Link to section 'Problem' of 'Jupyter: database is locked / can not load notebook format' Problem
You receive the following message after trying to load existing Jupyter notebooks inside your JupyterHub session:
Error loading notebook
An unknown error occurred while loading this notebook. This version can load notebook formats or earlier. See the server log for details.
Alternatively, the notebook may open but present an error when creating or saving a notebook:
Autosave Failed!
Unexpected error while saving file: MyNotebookName.ipynb database is locked
Link to section 'Solution' of 'Jupyter: database is locked / can not load notebook format' Solution
When Jupyter notebooks are opened, the server keeps track of their state in an internal database (located inside ~/.local/share/jupyter/
folder in your home directory). If a Jupyter process gets terminated abruptly (e.g. due to an out-of-memory error or a host reboot), the database lock is not cleared properly, and future instances of Jupyter detect the lock and complain.
Please follow these steps to resolve:
- Fully exit from your existing Jupyter session (close all notebooks, terminate Jupyter, log out from JupyterHub or JupyterLab, terminate OnDemand gateway's Jupyter app, etc).
- In a terminal window (SSH, Thinlinc or OnDemand gateway's terminal app) use the following command to clean up stale database locks:
$ unlock-jupyter
- Start a new Jupyter session as usual.
Questions
Frequently asked questions about jobs.
How do I know Non-uniform Memory Access (NUMA) layout on Scholar?
- You can learn about processor layout on Scholar nodes using the following command:
scholar-a003:~$ lstopo-no-graphics
- For detailed IO connectivity:
scholar-a003:~$ lstopo-no-graphics --physical --whole-io
- Please note that NUMA information is useful for advanced MPI/OpenMP/GPU optimizations. For most users, using default NUMA settings in MPI or OpenMP would give you the best performance.
Why cannot I use --mem=0 when submitting jobs?
Link to section 'Question' of 'Why cannot I use --mem=0 when submitting jobs?' Question
Why can't I specify --mem=0
for my job?
Link to section 'Answer' of 'Why cannot I use --mem=0 when submitting jobs?' Answer
We no longer support requesting unlimited memory (--mem=0
) as it has an adverse effect on the way scheduler allocates job, and could lead to large amount of nodes being blocked from usage.
Most often we suggest relying on default memory allocation (cluster-specific). But if you have to request custom amounts of memory, you can do it explicitly. For example --mem=20G
.
If you want to use the entire node's memory, you can submit the job with the --exclusive
option.
Can I extend the walltime on a job?
In some circumstances, yes. Walltime extensions must be requested of and completed by staff. Walltime extension requests will be considered on named (your advisor or research lab) queues. Standby or debug queue jobs cannot be extended.
Extension requests are at the discretion of staff based on factors such as any upcoming maintenance or resource availability. Jobs in the the 'scholar' queue on Scholar cannot be extended. 'Long' queue jobs can be extended to the maximum for that queue.
Please be mindful of time remaining on your job when making requests and make requests at least 24 hours before the end of your job AND during business hours. We cannot guarantee jobs will be extended in time with less than 24 hours notice, after-hours, during weekends, or on a holiday.
We ask that you make accurate walltime requests during job submissions. Accurate walltimes will allow the job scheduler to efficiently and quickly schedule jobs on the cluster. Please consider that extensions can impact scheduling efficiency for all users of the cluster.
Requests can be made by contacting support. We ask that you:
- Provide numerical job IDs, cluster name, and your desired extension amount.
- Provide at least 24 hours notice before job will end (more if request is made on a weekend or holiday).
- Consider making requests during business hours. We may not be able to respond in time to requests made after-hours, on a weekend, or on a holiday.
Data
Frequently asked questions about data and data management.
How is my Data Secured on Scholar?
Scholar is operated in line with policies, standards, and best practices as described within Secure Purdue, and specific to RCAC Resources.
Security controls for Scholar are based on ones defined in NIST cybersecurity standards.
Scholar supports research at the L1 fundamental and L2 sensitive levels. Scholar is not approved for storing data at the L3 restricted (covered by HIPAA) or L4 Export Controlled (ITAR), or any Controlled Unclassified Information (CUI).
For resources designed to support research with heightened security requirements, please look for resources within the REED+ Ecosystem.
Link to section 'For additional information' of 'How is my Data Secured on Scholar?' For additional information
Log in with your Purdue Career Account.
Can I share data with outside collaborators?
Yes! Globus allows convenient sharing of data with outside collaborators. Data can be shared with collaborators' personal computers or directly with many other computing resources at other institutions. See the Globus documentation on how to share data:
Does Scholar have the same home directory as other clusters?
The Scholar home directory and its contents are exclusive to Scholar cluster front-end hosts and compute nodes. This home directory is not available on other RCAC machines but Scholar. There is no automatic copying or synchronization between home directories.
At your discretion you can manually copy all or parts of your main research computing home to Scholar using one of the suggested methods.
If you plan to use hsi
or htar
commands to access Fortress tape archive from Scholar, please see also the keytab generation question for a temporary workaround to a potential caveat, while a permanent mitigation is being developed.
HSI/HTAR: Unable to authenticate user with remote gateway (error 2 or 9)
There could be a variety of such errors, with wordings along the lines of
Could not initialize keytab on remote server.
result = -2, errno = 2rver connection
*** hpssex_OpenConnection: Unable to authenticate user with remote gateway at 128.211.138.40.1217result = -2, errno = 9
Unable to setup communication to HPSS...
ERROR (main) unable to open remote gateway server connection
HTAR: HTAR FAILED
and
*** hpssex_OpenConnection: Unable to authenticate user with remote gateway at 128.211.138.40.1217result = -11000, errno = 9
Unable to setup communication to HPSS...
*** HSI: error opening logging
Error - authentication/initialization failed
The root cause for these errors is an expired or non-existent keytab file (a special authentication token stored in your home directory). These keytabs are valid for 90 days and on most RCAC resources they are usually automatically checked and regenerated when you execute hsi
or htar
commands. However, if the keytab is invalid, or fails to generate, Fortress may be unable to authenticate you and you would see the above errors. This is especially common on those RCAC clusters that have their own dedicated home directories (such as Bell), or on standalone installations (such as if you downloaded and installed HSI and HTAR on your non-RCAC computer).
This is a temporary problem and a permanent system-wide solution is being developed. In the interim, the recommended workaround is to generate a new valid keytab file in your main research computing home directory, and then copy it to your home directory on Scholar. The fortresskey
command is used to generate the keytab and can be executed on another cluster or a dedicated data management host data.rcac.purdue.edu
:
$ ssh myusername@data.rcac.purdue.edu fortresskey
$ scp -pr myusername@data.rcac.purdue.edu:~/.private $HOME
With a valid keytab in place, you should then be able to use hsi
and htar
commands to access Fortress from Scholar. Note that only one keytab can be valid at any given time (i.e. if you regenerated it, you may have to copy the new keytab to all systems that you intend to use hsi
or htar
from if they do not share the main research computing home directory).
Can I access Fortress from Scholar?
Yes. While Fortress directories are not directly mounted on Scholar for performance and archival protection reasons, they can be accessed from Scholar front-ends and nodes using any of the recommended methods of HSI, HTAR or Globus.
Software
Frequently asked questions about software.
Cannot use pip after loading ml-toolkit modules
Link to section 'Question' of 'Cannot use pip after loading ml-toolkit modules' Question
Pip throws an error after loading the machine learning modules. How can I fix it?
Link to section 'Answer' of 'Cannot use pip after loading ml-toolkit modules' Answer
Machine learning modules (tensorflow, pytorch, opencv etc.) include a version of pip that is newer than the one installed with Anaconda. As a result it will throw an error when you try to use it.
$ pip --version
Traceback (most recent call last):
File "/apps/cent7/anaconda/5.1.0-py36/bin/pip", line 7, in <module>
from pip import main
ImportError: cannot import name 'main'
The preferred way to use pip with the machine learning modules is to invoke it via Python as shown below.
$ python -m pip --version
How can I get access to Sentaurus software?
Link to section 'Question' of 'How can I get access to Sentaurus software?' Question
How can I get access to Sentaurus tools for micro- and nano-electronics design?
Link to section 'Answer' of 'How can I get access to Sentaurus software?' Answer
Sentaurus software license requires a signed NDA. Please contact Dr. Mark Johnson, Director of ECE Instructional Laboratories to complete the process.
Once the licensing process is complete and you have been added into a cae2
Unix group, you could use Sentaurus on RCAC community clusters by loading the corresponding environment module:
module load sentaurus
Julia package installation
Users do not have write permission to the default julia package installation destination. However, users can install packages into home directory under ~/.julia
.
Users can side step this by explicitly defining where to put julia packages:
$ export JULIA_DEPOT_PATH=$HOME/.julia
$ julia -e 'using Pkg; Pkg.add("PackageName")'
About Research Computing
Frequently asked questions about RCAC.
Can I get a private server from RCAC?
Link to section 'Question' of 'Can I get a private server from RCAC?' Question
Can I get a private (virtual or physical) server from RCAC?
Link to section 'Answer' of 'Can I get a private server from RCAC?' Answer
Often, researchers may want a private server to run databases, web servers, or other software. RCAC currently has Geddes, a Community Composable Platform optimized for composable, cloud-like workflows that are complementary to the batch applications run on Community Clusters. Funded by the National Science Foundation under grant OAC-2018926, Geddes consists of Dell Compute nodes with two 64-core AMD Epyc 'Rome' processors (128 cores per node).
To purchase access to Geddes today, go to the Cluster Access Purchase page. Please subscribe to our Community Cluster Program Mailing List to stay informed on the latest purchasing developments or contact us (rcac-cluster-purchase@lists.purdue.edu) if you have any questions.
Datasets
Please refer to our Federated Datasets Documentation website for up-to-date datasets on Anvil and instructions on how to use them.
Hammer User Guide
Hammer is optimized for Purdue's communities utilizing loosely-coupled, high-throughput computing.
Link to section 'Overview of Hammer' of 'Overview of Hammer' Overview of Hammer
Hammer is optimized for Purdue's communities utilizing loosely-coupled, high-throughput computing. Hammer was initially built through a partnership with HP and Intel in April 2015. Hammer was expanded again in late 2016. Hammer will be expanded annually, with each year's purchase of nodes to remain in production for 5 years from their initial purchase.
To purchase access to Hammer today, go to the Cluster Access Purchase page. Please subscribe to our Community Cluster Program Mailing List to stay informed on the latest purchasing developments or contact us via email at rcac-cluster-purchase@lists.purdue.edu if you have any questions.
Link to section 'Hammer Specifications' of 'Overview of Hammer' Hammer Specifications
Most Hammer nodes consist of identical hardware. All Hammer nodes have variable numbers of processor cores, and 10 Gbps or 25 Gbps Ethernet interconnects.
Front-Ends | Number of Nodes | Processors per Node | Cores per Node | Memory per Node | Retires in |
---|---|---|---|---|---|
2 | Two Haswell CPUs @ 2.60GHz | 20 | 64 GB | 2020 |
Sub-Cluster | Number of Nodes | Processors per Node | Cores per Node | Memory per Node | Retires in |
---|---|---|---|---|---|
A | 198 | Two Haswell CPUs @ 2.60GHz | 20 | 64 GB | 2020 |
B | 40 | Two Haswell CPUs @ 2.60GHz | 40 (Logical) | 128 GB | 2021 |
C | 27 | Two Sky Lake CPUs @ 2.60GHz | 48 (Logical) | 192 GB | 2022 |
D | 18 | Two Sky Lake CPUs @ 2.60GHz | 48 (Logical) | 192 GB | 2023 |
E | 15 | Two Intel Xeon Gold CPUs @ 2.60GHz | 48 (Logical) | 96 GB | 2024 |
Hammer nodes run Rocky 8 and use Slurm (Simple Linux Utility for Resource Management) as the batch scheduler for resource and job management. The application of operating system patches occurs as security needs dictate. All nodes allow for unlimited stack usage, as well as unlimited core dump size (though disk space and server quotas may still be a limiting factor).
This compiler and these libraries are loaded by default. To load the recommended set again:
$ module load rcac
To verify what you loaded:
$ module list
Link to section 'Accounts on Hammer' of 'Accounts' Accounts on Hammer
Link to section 'Obtaining an Account' of 'Accounts' Obtaining an Account
To obtain an account, you must be part of a research group which has purchased access to Hammer. Refer to the Accounts / Access page for more details on how to request access.
Link to section 'Outside Collaborators' of 'Accounts' Outside Collaborators
A valid Purdue Career Account is required for access to any resource. If you do not currently have a valid Purdue Career Account you must have a current Purdue faculty or staff member file a Request for Privileges (R4P) before you can proceed.
Logging In
To submit jobs on Hammer, log in to the submission host hammer.rcac.purdue.edu
via SSH. This submission host is actually 2 front-end hosts: hammer-fe00
and hammer-fe01
. The login process randomly assigns one of these front-ends to each login to hammer.rcac.purdue.edu
.
Purdue Login
Link to section 'SSH' of 'Purdue Login' SSH
- SSH to the cluster as usual.
- When asked for a password, type your password followed by "
,push
". - Your Purdue Duo client will receive a notification to approve the login.
Link to section 'Thinlinc' of 'Purdue Login' Thinlinc
- When asked for a password, type your password followed by "
,push
". - Your Purdue Duo client will receive a notification to approve the login.
- The native Thinlinc client will prompt for Duo approval twice due to the way Thinlinc works.
- The native Thinlinc client also supports key-based authentication.
Passwords
Hammer supports either Purdue two-factor authentication (Purdue Login) or SSH keys.
SSH Client Software
Secure Shell or SSH is a way of establishing a secure connection between two computers. It uses public-key cryptography to authenticate the user with the remote computer and to establish a secure connection. Its usual function involves logging in to a remote machine and executing commands. There are many SSH clients available for all operating systems:
Linux / Solaris / AIX / HP-UX / Unix:
- The
ssh
command is pre-installed. Log in usingssh myusername@hammer.rcac.purdue.edu
from a terminal.
Microsoft Windows:
- MobaXterm is a small, easy to use, full-featured SSH client. It includes X11 support for remote displays, SFTP capabilities, and limited SSH authentication forwarding for keys.
Mac OS X:
- The
ssh
command is pre-installed. You may start a local terminal window from "Applications->Utilities". Log in by typing the commandssh myusername@hammer.rcac.purdue.edu
.
When prompted for password, enter your Purdue career account password followed by ",push
". Your Purdue Duo client will then receive a notification to approve the login.
SSH Keys
Link to section 'General overview' of 'SSH Keys' General overview
To connect to Hammer using SSH keys, you must follow three high-level steps:
- Generate a key pair consisting of a private and a public key on your local machine.
- Copy the public key to the cluster and append it to
$HOME/.ssh/authorized_keys
file in your account. - Test if you can ssh from your local computer to the cluster without using your Purdue password.
Detailed steps for different operating systems and specific SSH client softwares are give below.
Link to section 'Mac and Linux:' of 'SSH Keys' Mac and Linux:
-
Run
ssh-keygen
in a terminal on your local machine. You may supply a filename and a passphrase for protecting your private key, but it is not mandatory. To accept the default settings, press Enter without specifying a filename.
Note: If you do not protect your private key with a passphrase, anyone with access to your computer could SSH to your account on Hammer. -
By default, the key files will be stored in
~/.ssh/id_rsa
and~/.ssh/id_rsa.pub
on your local machine. -
Copy the contents of the public key into
$HOME/.ssh/authorized_keys
on the cluster with the following command. When asked for a password, type your password followed by ",push
". Your Purdue Duo client will receive a notification to approve the login.ssh-copy-id -i ~/.ssh/id_rsa.pub myusername@hammer.rcac.purdue.edu
Note: use your actual Purdue account user name.
If your system does not have the
ssh-copy-id
command, use this instead:cat ~/.ssh/id_rsa.pub | ssh myusername@hammer.rcac.purdue.edu "mkdir -p ~/.ssh && chmod 700 ~/.ssh && cat >> ~/.ssh/authorized_keys"
-
Test the new key by SSH-ing to the server. The login should now complete without asking for a password.
-
If the private key has a non-default name or location, you need to specify the key by
ssh -i my_private_key_name myusername@hammer.rcac.purdue.edu
Link to section 'Windows:' of 'SSH Keys' Windows:
Programs | Instructions |
---|---|
MobaXterm | Open a local terminal and follow Linux steps |
Git Bash | Follow Linux steps |
Windows 10 PowerShell | Follow Linux steps |
Windows 10 Subsystem for Linux | Follow Linux steps |
PuTTY | Follow steps below |
PuTTY:
-
Launch PuTTYgen, keep the default key type (RSA) and length (2048-bits) and click Generate button.
The "Generate" button can be found under the "Actions" section of the PuTTY Key Generator interface. -
Once the key pair is generated:
Use the Save public key button to save the public key, e.g.
Documents\SSH_Keys\mylaptop_public_key.pub
Use the Save private key button to save the private key, e.g.
Documents\SSH_Keys\mylaptop_private_key.ppk
. When saving the private key, you can also choose a reminder comment, as well as an optional passphrase to protect your key, as shown in the image below. Note: If you do not protect your private key with a passphrase, anyone with access to your computer could SSH to your account on Hammer.The PuTTY Key Generator form has inputs for the Key passphrase and optional reminder comment. From the menu of PuTTYgen, use the "Conversion -> Export OpenSSH key" tool to convert the private key into openssh format, e.g.
Documents\SSH_Keys\mylaptop_private_key.openssh
to be used later for Thinlinc. -
Configure PuTTY to use key-based authentication:
Launch PuTTY and navigate to "Connection -> SSH ->Auth" on the left panel, click Browse button under the "Authentication parameters" section and choose your private key, e.g. mylaptop_private_key.ppk
After clicking Connection -> SSH ->Auth panel, the "Browse" option can be found at the bottom of the resulting panel. Navigate back to "Session" on the left panel. Highlight "Default Settings" and click the "Save" button to ensure the change in place.
-
Connect to the cluster. When asked for a password, type your password followed by "
,push
". Your Purdue Duo client will receive a notification to approve the login. Copy the contents of public key from PuTTYgen as shown below and paste it into$HOME/.ssh/authorized_keys
. Please double-check that your text editor did not wrap or fold the pasted value (it should be one very long line).The "Public key" will look like a long string of random letters and numbers in a text box at the top of the window. - Test by connecting to the cluster. If successful, you will not be prompted for a password or receive a Duo notification. If you protected your private key with a passphrase in step 2, you will instead be prompted to enter your chosen passphrase when connecting.
SSH X11 Forwarding
SSH supports tunneling of X11 (X-Windows). If you have an X11 server running on your local machine, you may use X11 applications on remote systems and have their graphical displays appear on your local machine. These X11 connections are tunneled and encrypted automatically by your SSH client.
Link to section 'Installing an X11 Server' of 'SSH X11 Forwarding' Installing an X11 Server
To use X11, you will need to have a local X11 server running on your personal machine. Both free and commercial X11 servers are available for various operating systems.
Linux / Solaris / AIX / HP-UX / Unix:
- An X11 server is at the core of all graphical sessions. If you are logged in to a graphical environment on these operating systems, you are already running an X11 server.
- ThinLinc is an alternative to running an X11 server directly on your Linux computer. ThinLinc is a service that allows you to connect to a persistent remote graphical desktop session.
Microsoft Windows:
- ThinLinc is an alternative to running an X11 server directly on your Windows computer. ThinLinc is a service that allows you to connect to a persistent remote graphical desktop session.
- MobaXterm is a small, easy to use, full-featured SSH client. It includes X11 support for remote displays, SFTP capabilities, and limited SSH authentication forwarding for keys.
Mac OS X:
- X11 is available as an optional install on the Mac OS X install disks prior to 10.7/Lion. Run the installer, select the X11 option, and follow the instructions. For 10.7+ please download XQuartz.
- ThinLinc is an alternative to running an X11 server directly on your Mac computer. ThinLinc is a service that allows you to connect to a persistent remote graphical desktop session.
Link to section 'Enabling X11 Forwarding in your SSH Client' of 'SSH X11 Forwarding' Enabling X11 Forwarding in your SSH Client
Once you are running an X11 server, you will need to enable X11 forwarding/tunneling in your SSH client:
ssh
: X11 tunneling should be enabled by default. To be certain it is enabled, you may usessh -Y
.- MobaXterm: Select "New session" and "SSH." Under "Advanced SSH Settings" check the box for X11 Forwarding.
SSH will set the remote environment variable $DISPLAY
to "localhost:XX.YY"
when this is working correctly. If you had previously set your $DISPLAY
environment variable to your local IP or hostname, you must remove any set
/export
/setenv
of this variable from your login scripts. The environment variable $DISPLAY
must be left as SSH sets it, which is to a random local port address. Setting $DISPLAY
to an IP or hostname will not work.
ThinLinc
RCAC provides Cendio's ThinLinc as an alternative to running an X11 server directly on your computer. It allows you to run graphical applications or graphical interactive jobs directly on Hammer through a persistent remote graphical desktop session.
ThinLinc is a service that allows you to connect to a persistent remote graphical desktop session. This service works very well over a high latency, low bandwidth, or off-campus connection compared to running an X11 server locally. It is also very helpful for Windows users who do not have an easy to use local X11 server, as little to no set up is required on your computer.
There are two ways in which to use ThinLinc: preferably through the native client or through a web browser.
Link to section 'Installing the ThinLinc native client' of 'ThinLinc' Installing the ThinLinc native client
The native ThinLinc client will offer the best experience especially over off-campus connections and is the recommended method for using ThinLinc. It is compatible with Windows, Mac OS X, and Linux.
- Download the ThinLinc client from the ThinLinc website.
- Start the ThinLinc client on your computer.
- In the client's login window, use desktop.hammer.rcac.purdue.edu as the Server. Use your Purdue Career Account username and password, but append "
,push
" to your password. - Click the Connect button.
- Your Purdue Login Duo will receive a notification to approve your login.
- Continue to following section on connecting to Hammer from ThinLinc.
Link to section 'Using ThinLinc through your web browser' of 'ThinLinc' Using ThinLinc through your web browser
The ThinLinc service can be accessed from your web browser as a convenience to installing the native client. This option works with no set up and is a good option for those on computers where you do not have privileges to install software. All that is required is an up-to-date web browser. Older versions of Internet Explorer may not work.
- Open a web browser and navigate to desktop.hammer.rcac.purdue.edu.
- Log in with your Purdue Career Account username and password, but append "
,push
" to your password. - You may safely proceed past any warning messages from your browser.
- Your Purdue Login Duo will receive a notification to approve your login.
- Continue to the following section on connecting to Hammer from ThinLinc.
Link to section 'Connecting to Hammer from ThinLinc' of 'ThinLinc' Connecting to Hammer from ThinLinc
- Once logged in, you will be presented with a remote Linux desktop running directly on a cluster front-end.
- Open the terminal application on the remote desktop.
- Once logged in to the Hammer head node, you may use graphical editors, debuggers, software like Matlab, or run graphical interactive jobs. For example, to test the X forwarding connection issue the following command to launch the graphical editor gedit:
$ gedit
- This session will remain persistent even if you disconnect from the session. Any interactive jobs or applications you left running will continue running even if you are not connected to the session.
Link to section 'Tips for using ThinLinc native client' of 'ThinLinc' Tips for using ThinLinc native client
- To exit a full screen ThinLinc session press the F8 key on your keyboard (fn + F8 key for Mac users) and click to disconnect or exit full screen.
- Full screen mode can be disabled when connecting to a session by clicking the Options button and disabling full screen mode from the Screen tab.
Link to section 'Configure ThinLinc to use SSH Keys' of 'ThinLinc' Configure ThinLinc to use SSH Keys
- The web client does NOT support public-key authentication.
-
ThinLinc native client supports the use of an SSH key pair. For help generating and uploading keys to the cluster, see SSH Keys section in our user guide for details.
To set up SSH key authentication on the ThinLinc client:
-
Open the Options panel, and select Public key as your authentication method on the Security tab.
The "Options..." button in the ThinLinc Client can be found towards the bottom left, above the "Connect" button. -
In the options dialog, switch to the "Security" tab and select the "Public key" radio button:
The "Security" tab found in the options dialog, will be the last of available tabs. The "Public key" option can be found in the "Authentication method" options group. - Click OK to return to the ThinLinc Client login window. You should now see a Key field in place of the Password field.
-
In the Key field, type the path to your locally stored private key or click the ... button to locate and select the key on your local system. Note: If PuTTY is used to generate the SSH Key pairs, please choose the private key in the openssh format.
The ThinLinc Client login window will now display key field instead of a password field.
-
Purchasing Nodes
RCAC operates a significant shared cluster computing infrastructure developed over several years through focused acquisitions using funds from grants, faculty startup packages, and institutional sources. These "community clusters" are now at the foundation of Purdue's research cyberinfrastructure.
We strongly encourage any Purdue faculty or staff with computational needs to join this growing community and enjoy the enormous benefits this shared infrastructure provides:
- Peace of Mind
RCAC system administrators take care of security patches, attempted hacks, operating system upgrades, and hardware repair so faculty and graduate students can concentrate on research.
- Low Overhead
RCAC data centers provide infrastructure such as networking, racks, floor space, cooling, and power.
- Cost Effective
RCAC works with vendors to obtain the best price for computing resources by pooling funds from different disciplines to leverage greater group purchasing power.
Through the Community Cluster Program, Purdue affiliates have invested several million dollars in computational and storage resources from Q4 2006 to the present with great success in both the research accomplished and the money saved on equipment purchases.
For more information or to purchase access to our latest cluster today, see the Purchase page. Have questions? contact us at rcac-cluster-purchase@lists.purdue.edu to discuss.
File Storage and Transfer
Learn more about file storage transfer for Hammer.
Link to section 'Archive and Compression' of 'Archive and Compression' Archive and Compression
There are several options for archiving and compressing groups of files or directories. The mostly commonly used options are:
Link to section 'tar' of 'Archive and Compression' tar
See the official documentation for tar for more information.
Saves many files together into a single archive file, and restores individual files from the archive. Includes automatic archive compression/decompression options and special features for incremental and full backups.
Examples:
(list contents of archive somefile.tar)
$ tar tvf somefile.tar
(extract contents of somefile.tar)
$ tar xvf somefile.tar
(extract contents of gzipped archive somefile.tar.gz)
$ tar xzvf somefile.tar.gz
(extract contents of bzip2 archive somefile.tar.bz2)
$ tar xjvf somefile.tar.bz2
(archive all ".c" files in current directory into one archive file)
$ tar cvf somefile.tar *.c
(archive and gzip-compress all files in a directory into one archive file)
$ tar czvf somefile.tar.gz somedirectory/
(archive and bzip2-compress all files in a directory into one archive file)
$ tar cjvf somefile.tar.bz2 somedirectory/
Other arguments for tar can be explored by using the man tar command.
Link to section 'gzip' of 'Archive and Compression' gzip
The standard compression system for all GNU software.
Examples:
(compress file somefile - also removes uncompressed file)
$ gzip somefile
(uncompress file somefile.gz - also removes compressed file)
$ gunzip somefile.gz
Link to section 'bzip2' of 'Archive and Compression' bzip2
See the official documentation for bzip for more information.
Strong, lossless data compressor based on the Burrows-Wheeler transform. Stronger compression than gzip.
Examples:
(compress file somefile - also removes uncompressed file)
$ bzip2 somefile
(uncompress file somefile.bz2 - also removes compressed file)
$ bunzip2 somefile.bz2
There are several other, less commonly used, options available as well:
- zip
- 7zip
- xz
Link to section 'Storage Environment Variables' of 'Storage Environment Variables' Storage Environment Variables
Several environment variables are automatically defined for you to help you manage your storage. Use environment variables instead of actual paths whenever possible to avoid problems if the specific paths to any of these change.
Name | Description |
---|---|
HOME | /home/myusername |
PWD | path to your current directory |
RCAC_SCRATCH | /scratch/hammer/myusername |
By convention, environment variable names are all uppercase. You may use them on the command line or in any scripts in place of and in combination with hard-coded values:
$ ls $HOME
...
$ ls $RCAC_SCRATCH/myproject
...
To find the value of any environment variable:
$ echo $RCAC_SCRATCH
${resource.scratch}/m/myusername
To list the values of all environment variables:
$ env
USER=myusername
HOME=/home/myusername
RCAC_SCRATCH=${resource.scratch}/m/myusername
...
You may create or overwrite an environment variable. To pass (export) the value of a variable in bash:
$ export MYPROJECT=$RCAC_SCRATCH/myproject
To assign a value to an environment variable in either tcsh or csh:
$ setenv MYPROJECT value
Storage Options
File storage options on RCAC systems include long-term storage (home directories, depot, Fortress) and short-term storage (scratch directories, /tmp directory). Each option has different performance and intended uses, and some options vary from system to system as well. Daily snapshots of home directories are provided for a limited time for accidental deletion recovery. Scratch directories and temporary storage are not backed up and old files are regularly purged from scratch and /tmp directories. More details about each storage option appear below.
Home Directory
Home directories are provided for long-term file storage. Each user has one home directory. You should use your home directory for storing important program files, scripts, input data sets, critical results, and frequently used files. You should store infrequently used files on Fortress. Your home directory becomes your current working directory, by default, when you log in.
Daily snapshots of your home directory are provided for a limited period of time in the event of accidental deletion. For additional security, you should store another copy of your files on more permanent storage, such as the Fortress HPSS Archive.
Your home directory physically resides on a GPFS storage system in the data center. To find the path to your home directory, first log in then immediately enter the following:
$ pwd
/home/myusername
Or from any subdirectory:
$ echo $HOME
/home/myusername
Your home directory has a quota limiting the total size of files you may store within. For more information, refer to the Storage Quotas / Limits Section.
Link to section 'Lost File Recovery' of 'Home Directory' Lost File Recovery
Nightly snapshots for 7 days, weekly snapshots for 4 weeks, and monthly snapshots for 3 months are kept. This means you will find snapshots from the last 7 nights, the last 4 Sundays, and the last 3 first of the months. Files are available going back between two and three months, depending on how long ago the last first of the month was. Snapshots beyond this are not kept. For additional security, you should store another copy of your files on more permanent storage, such as the Fortress HPSS Archive.
Link to section 'Performance' of 'Home Directory' Performance
Your home directory is medium-performance, non-purged space suitable for tasks like sharing data, editing files, developing and building software, and many other uses.
Your home directory is not designed or intended for use as high-performance working space for running data-intensive jobs with heavy I/O demands.
Link to section 'Long-Term Storage' of 'Long-Term Storage' Long-Term Storage
Long-term Storage or Permanent Storage is available to users on the High Performance Storage System (HPSS), an archival storage system, called Fortress. Program files, data files and any other files which are not used often, but which must be saved, can be put in permanent storage. Fortress currently has over 10PB of capacity.
For more information about Fortress, how it works, and user guides, and how to obtain an account:
Scratch Space
Scratch directories are provided for short-term file storage only. The quota of your scratch directory is much greater than the quota of your home directory. You should use your scratch directory for storing temporary input files which your job reads or for writing temporary output files which you may examine after execution of your job. You should use your home directory and Fortress for longer-term storage or for holding critical results. The hsi and htar commands provide easy-to-use interfaces into the archive and can be used to copy files into the archive interactively or even automatically at the end of your regular job submission scripts.
Files in scratch directories are not recoverable. Files in scratch directories are not backed up. If you accidentally delete a file, a disk crashes, or old files are purged, they cannot be restored.
Files are purged from scratch directories not accessed or had content modified in 60 days. Owners of these files receive a notice one week before removal via email. Be sure to regularly check your Purdue email account or set up mail forwarding to an email account you do regularly check. For more information, please refer to our Scratch File Purging Policy.
All users may access scratch directories on Hammer. To find the path to your scratch directory:
$ findscratch
${resource.scratch}/m/myusername
The value of variable $RCAC_SCRATCH is your scratch directory path. Use this variable in any scripts. Your actual scratch directory path may change without warning, but this variable will remain current.
$ echo $RCAC_SCRATCH
${resource.scratch}/m/myusername
Scratch directories are specific per cluster. I.e. only the ${resource.scratch} directory is available on Hammer front-end and compute nodes. No other scratch directories are available on Hammer.
Your scratch directory has a quota capping the total size and number of files you may store in it. For more information, refer to the section Storage Quotas / Limits.
Link to section 'Performance' of 'Scratch Space' Performance
Your scratch directory is located on a high-performance, large-capacity parallel filesystem engineered to provide work-area storage optimized for a wide variety of job types. It is designed to perform well with data-intensive computations, while scaling well to large numbers of simultaneous connections.
/tmp Directory
/tmp directories are provided for short-term file storage only. Each front-end and compute node has a /tmp directory. Your program may write temporary data to the /tmp
directory of the compute node on which it is running. That data is available for as long as your program is active. Once your program terminates, that temporary data is no longer available. When used properly, /tmp
may provide faster local storage to an active process than any other storage option. You should use your home directory and Fortress for longer-term storage or for holding critical results.
Backups are not performed for the /tmp
directory and removes files from /tmp
whenever space is low or whenever the system needs a reboot. In the event of a disk crash or file purge, files in /tmp
are not recoverable. You should copy any important files to more permanent storage.
Storage Quota / Limits
Some limits are imposed on your disk usage on research systems. A quota is implemented on each filesystem. Each filesystem (home directory, scratch directory, etc.) may have a different limit. If you exceed the quota, you will not be able to save new files or new data to the filesystem until you delete or move data to long-term storage.
Link to section 'Checking Quota' of 'Storage Quota / Limits' Checking Quota
To check the current quotas of your home and scratch directories check the My Quota page or use the myquota
command:
$ myquota
Type Filesystem Size Limit Use Files Limit Use
==============================================================================
home myusername 5.0GB 25.0GB 20% - - -
scratch hammer 220.7GB 100.0TB 0.22% 8k 2,000k 0.43%
The columns are as follows:
- Type: indicates home or scratch directory or your depot space.
- Filesystem: name of storage option.
- Size: sum of file sizes in bytes.
- Limit: allowed maximum on sum of file sizes in bytes.
- Use: percentage of file-size limit currently in use.
- Files: number of files and directories (not the size).
- Limit: allowed maximum on number of files and directories. It is possible, though unlikely, to reach this limit and not the file-size limit if you create a large number of very small files.
- Use: percentage of file-number limit currently in use.
If you find that you reached your quota in either your home directory or your scratch file directory, obtain estimates of your disk usage. Find the top-level directories which have a high disk usage, then study the subdirectories to discover where the heaviest usage lies.
To see in a human-readable format an estimate of the disk usage of your top-level directories in your home directory:
$ du -h --max-depth=1 $HOME >myfile
32K /home/myusername/mysubdirectory_1
529M /home/myusername/mysubdirectory_2
608K /home/myusername/mysubdirectory_3
The second directory is the largest of the three, so apply command du to it.
To see in a human-readable format an estimate of the disk usage of your top-level directories in your scratch file directory:
$ du -h --max-depth=1 $RCAC_SCRATCH >myfile
K ${resource.scratch}/m/myusername
This strategy can be very helpful in figuring out the location of your largest usage. Move unneeded files and directories to long-term storage to free space in your home and scratch directories.
Link to section 'Increasing Quota' of 'Storage Quota / Limits' Increasing Quota
Link to section 'Home Directory' of 'Storage Quota / Limits' Home Directory
If you find you need additional disk space in your home directory, please consider archiving and compressing old files and moving them to long-term storage on the Fortress HPSS Archive, or purchase the Depot space for long-term storage. Unfortunately, it is not possible to increase your home directory quota beyond it's current level.
Link to section 'Scratch Space' of 'Storage Quota / Limits' Scratch Space
If you find you need additional disk space in your scratch space, please first consider archiving and compressing old files and moving them to long-term storage on the Fortress HPSS Archive. If you are unable to do so, you may ask for a quota increase by contacting support.
Link to section 'Sharing Files from Hammer' of 'Sharing' Sharing Files from Hammer
Hammer supports several methods for file sharing. Use the links below to learn more about these methods.
Link to section 'Sharing Data with Globus' of 'Globus' Sharing Data with Globus
Data on any RCAC resource can be shared with other users within Purdue or with collaborators at other institutions. Globus allows convenient sharing of data with outside collaborators. Data can be shared with collaborators' personal computers or directly with many other computing resources at other institutions.
To share files, login to https://transfer.rcac.purdue.edu, navigate to the endpoint (collection) of your choice, and follow instructions as described in Globus documentation on how to share data:
See also RCAC Globus presentation.
File Transfer
Hammer supports several methods for file transfer. Use the links below to learn more about these methods.
SCP
SCP (Secure CoPy) is a simple way of transferring files between two machines that use the SSH protocol. SCP is available as a protocol choice in some graphical file transfer programs and also as a command line program on most Linux, Unix, and Mac OS X systems. SCP can copy single files, but will also recursively copy directory contents if given a directory name.
After Aug 17, 2020, the community clusters will not support password-based authentication for login. Methods that can be used include two-factor authentication (Purdue Login) or SSH keys. If you do not have SSH keys installed, you would need to type your Purdue Login response into the SFTP's "Password" prompt.
Link to section 'Command-line usage:' of 'SCP' Command-line usage:
You can transfer files both to and from Hammer while initiating an SCP session on either some other computer or on Hammer (in other words, directionality of connection and directionality of data flow are independent from each other). The scp
command appears somewhat similar to the familiar cp
command, with an extra user@host:file
syntax to denote files and directories on a remote host. Either Hammer or another computer can be a remote.
-
Example: Initiating SCP session on some other computer (i.e. you are on some other computer, connecting to Hammer):
(transfer TO Hammer) (Individual files) $ scp sourcefile myusername@hammer.rcac.purdue.edu:somedir/destinationfile $ scp sourcefile myusername@hammer.rcac.purdue.edu:somedir/ (Recursive directory copy) $ scp -pr sourcedirectory/ myusername@hammer.rcac.purdue.edu:somedir/ (transfer FROM Hammer) (Individual files) $ scp myusername@hammer.rcac.purdue.edu:somedir/sourcefile destinationfile $ scp myusername@hammer.rcac.purdue.edu:somedir/sourcefile somedir/ (Recursive directory copy) $ scp -pr myusername@hammer.rcac.purdue.edu:sourcedirectory somedir/
The -p flag is optional. When used, it will cause the transfer to preserve file attributes and permissions. The -r flag is required for recursive transfers of entire directories.
-
Example: Initiating SCP session on Hammer (i.e. you are on Hammer, connecting to some other computer):
(transfer TO Hammer) (Individual files) $ scp myusername@$another.computer.example.com:sourcefile somedir/destinationfile $ scp myusername@$another.computer.example.com:sourcefile somedir/ (Recursive directory copy) $ scp -pr myusername@$another.computer.example.com:sourcedirectory/ somedir/ (transfer FROM Hammer) (Individual files) $ scp somedir/sourcefile myusername@$another.computer.example.com:destinationfile $ scp somedir/sourcefile myusername@$another.computer.example.com:somedir/ (Recursive directory copy) $ scp -pr sourcedirectory myusername@$another.computer.example.com:somedir/
The -p flag is optional. When used, it will cause the transfer to preserve file attributes and permissions. The -r flag is required for recursive transfers of entire directories.
Link to section 'Software (SCP clients)' of 'SCP' Software (SCP clients)
Linux and other Unix-like systems:
- The
scp
command-line program should already be installed.
Microsoft Windows:
- MobaXterm
Free, full-featured, graphical Windows SSH, SCP, and SFTP client. - Command-line
scp
program can be installed as part of Windows Subsystem for Linux (WSL), or Git-Bash.
Mac OS X:
- The
scp
command-line program should already be installed. You may start a local terminal window from "Applications->Utilities". - Cyberduck is a full-featured and free graphical SFTP and SCP client.
Globus
Link to section 'Globus' of 'Globus' Globus
Globus, previously known as Globus Online, is a powerful and easy to use file transfer service for transferring files virtually anywhere. It works within RCAC's various research storage systems; it connects between RCAC and remote research sites running Globus; and it connects research systems to personal systems. You may use Globus to connect to your home, scratch, and Fortress storage directories. Since Globus is web-based, it works on any operating system that is connected to the internet. The Globus Personal client is available on Windows, Linux, and Mac OS X. It is primarily used as a graphical means of transfer but it can also be used over the command line.
Link to section 'Link to section 'Globus Web:' of 'Globus' Globus Web:' of 'Globus' Link to section 'Globus Web:' of 'Globus' Globus Web:
- Navigate to http://transfer.rcac.purdue.edu
- Click "Proceed" to log in with your Purdue Career Account.
- On your first login it will ask to make a connection to a Globus account. Accept the conditions.
- Now you are at the main screen. Click "File Transfer" which will bring you to a two-panel interface (if you only see one panel, you can use selector in the top-right corner to switch the view).
- You will need to select one collection and file path on one side as the source, and the second collection on the other as the destination. This can be one of several Purdue endpoints, or another University, or even your personal computer (see Personal Client section below).
The RCAC collections are as follows. A search for "Purdue" will give you several suggested results you can choose from, or you can give a more specific search.
- Home Directory storage: "Purdue Research Computing - Home Directories", however, you can start typing "Purdue" and "Home Directories" and it will suggest appropriate matches.
- Weber scratch storage: "Purdue Weber Cluster", however, you can start typing "Purdue" and "Weber and it will suggest appropriate matches. From here you will need to navigate into the first letter of your username, and then into your username.
- Research Data Depot: "Purdue Research Computing - Data Depot", a search for "Depot" should provide appropriate matches to choose from.
- Fortress: "Purdue Fortress HPSS Archive", a search for "Fortress" should provide appropriate matches to choose from.
From here, select a file or folder in either side of the two-pane window, and then use the arrows in the top-middle of the interface to instruct Globus to move files from one side to the other. You can transfer files in either direction. You will receive an email once the transfer is completed.
Link to section 'Link to section 'Globus Personal Client setup:' of 'Globus' Globus Personal Client setup:' of 'Globus' Link to section 'Globus Personal Client setup:' of 'Globus' Globus Personal Client setup:
Globus Connect Personal is a small software tool you can install to make your own computer a Globus endpoint on its own. It is useful if you need to transfer files via Globus to and from your computer directly.
- On the "Collections" page from earlier, click "Get Globus Connect Personal" or download a version for your operating system it from here: Globus Connect Personal
- Name this particular personal system and follow the setup prompts to create your Globus Connect Personal endpoint.
- Your personal system is now available as a collection within the Globus transfer interface.
Link to section 'Link to section 'Globus Command Line:' of 'Globus' Globus Command Line:' of 'Globus' Link to section 'Globus Command Line:' of 'Globus' Globus Command Line:
Globus supports command line interface, allowing advanced automation of your transfers.
To use the recommended standalone Globus CLI application (the globus command):
- First time use: issue the globus login command and follow instructions for initial login.
- Commands for interfacing with the CLI can be found via Using the Command Line Interface, as well as the Globus CLI Examples pages.
Link to section 'Link to section 'Sharing Data with Outside Collaborators' of 'Globus' Sharing Data with Outside Collaborators' of 'Globus' Link to section 'Sharing Data with Outside Collaborators' of 'Globus' Sharing Data with Outside Collaborators
Globus allows convenient sharing of data with outside collaborators. Data can be shared with collaborators' personal computers or directly with many other computing resources at other institutions. See the Globus documentation on how to share data:
For links to more information, please see Globus Support page and RCAC Globus presentation.
Windows Network Drive / SMB
SMB (Server Message Block), also known as CIFS, is an easy to use file transfer protocol that is useful for transferring files between RCAC systems and a desktop or laptop. You may use SMB to connect to your home, scratch, and Fortress storage directories. The SMB protocol is available on Windows, Linux, and Mac OS X. It is primarily used as a graphical means of transfer but it can also be used over the command line.
Note: to access Hammer through SMB file sharing, you must be on a Purdue campus network or connected through VPN.
Link to section 'Windows:' of 'Windows Network Drive / SMB' Windows:
- Windows 7: Click Windows menu > Computer, then click Map Network Drive in the top bar
- Windows 8 & 10: Tap the Windows key, type computer, select This PC, click Computer > Map Network Drive in the top bar
- Windows 11: Tap the Windows key, type File Explorer, select This PC, click Computer > Map Network Drive in the top bar
- In the folder location enter the following information and click Finish:
- To access your Hammer home directory, enter \\home.hammer.rcac.purdue.edu\hammer-home.
- To access your scratch space on Hammer, enter \\scratch.hammer.rcac.purdue.edu\hammer-scratch. Once mapped, you will be able to navigate to your scratch directory.
- Note: Use your career account login name and password when prompted. (You will not need to add "
,push
" nor use your Purdue Duo client.) - Your home or scratch directory should now be mounted as a drive in the Computer window.
Link to section 'Mac OS X:' of 'Windows Network Drive / SMB' Mac OS X:
- In the Finder, click Go > Connect to Server
- In the Server Address enter the following information and click Connect:
- To access your Hammer home directory, enter smb://home.hammer.rcac.purdue.edu/hammer-home.
- To access your scratch space on Hammer, enter smb://scratch.hammer.rcac.purdue.edu/hammer-scratch. Once mapped, you will be able to navigate to your scratch directory.
- Note: Use your career account login name and password when prompted. (You will not need to add "
,push
" nor use your Purdue Duo client.) - Your home or scratch directory should now be mounted as a drive in the Computer window.
Link to section 'Linux:' of 'Windows Network Drive / SMB' Linux:
- There are several graphical methods to connect in Linux depending on your desktop environment. Once you find out how to connect to a network server on your desktop environment, choose the Samba/SMB protocol and adapt the information from the Mac OS X section to connect.
- If you would like access via samba on the command line you may install smbclient which will give you FTP-like access and can be used as shown below. For all the possible ways to connect look at the Mac OS X instructions.
smbclient //home.hammer.rcac.purdue.edu/hammer-home -U myusername smbclient //scratch.hammer.rcac.purdue.edu/hammer-scratch -U myusername
- Note: Use your career account login name and password when prompted. (You will not need to add "
,push
" nor use your Purdue Duo client.)
FTP / SFTP
FTP is not supported on any research systems because it does not allow for secure transmission of data. Use SFTP instead, as described below.
SFTP (Secure File Transfer Protocol) is a reliable way of transferring files between two machines. SFTP is available as a protocol choice in some graphical file transfer programs and also as a command-line program on most Linux, Unix, and Mac OS X systems. SFTP has more features than SCP and allows for other operations on remote files, remote directory listing, and resuming interrupted transfers. Command-line SFTP cannot recursively copy directory contents; to do so, try using SCP or graphical SFTP client.
After Aug 17, 2020, the community clusters will not support password-based authentication for login. Methods that can be used include two-factor authentication (Purdue Login) or SSH keys. If you do not have SSH keys installed, you would need to type your Purdue Login response into the SFTP's "Password" prompt.
Link to section 'Command-line usage' of 'FTP / SFTP' Command-line usage
You can transfer files both to and from Hammer while initiating an SFTP session on either some other computer or on Hammer (in other words, directionality of connection and directionality of data flow are independent from each other). Once the connection is established, you use put
or get
subcommands between "local" and "remote" computers. Either Hammer or another computer can be a remote.
-
Example: Initiating SFTP session on some other computer (i.e. you are on another computer, connecting to Hammer):
$ sftp myusername@hammer.rcac.purdue.edu (transfer TO Hammer) sftp> put sourcefile somedir/destinationfile sftp> put -P sourcefile somedir/ (transfer FROM Hammer) sftp> get sourcefile somedir/destinationfile sftp> get -P sourcefile somedir/ sftp> exit
The -P flag is optional. When used, it will cause the transfer to preserve file attributes and permissions.
-
Example: Initiating SFTP session on Hammer (i.e. you are on Hammer, connecting to some other computer):
$ sftp myusername@$another.computer.example.com (transfer TO Hammer) sftp> get sourcefile somedir/destinationfile sftp> get -P sourcefile somedir/ (transfer FROM Hammer) sftp> put sourcefile somedir/destinationfile sftp> put -P sourcefile somedir/ sftp> exit
The -P flag is optional. When used, it will cause the transfer to preserve file attributes and permissions.
Link to section 'Software (SFTP clients)' of 'FTP / SFTP' Software (SFTP clients)
Linux and other Unix-like systems:
- The
sftp
command-line program should already be installed.
Microsoft Windows:
- MobaXterm
Free, full-featured, graphical Windows SSH, SCP, and SFTP client. - Command-line
sftp
program can be installed as part of Windows Subsystem for Linux (WSL), or Git-Bash.
Mac OS X:
- The
sftp
command-line program should already be installed. You may start a local terminal window from "Applications->Utilities". - Cyberduck is a full-featured and free graphical SFTP and SCP client.
Software
Link to section 'Environment module' of 'Software' Environment module
Link to section 'Software catalog' of 'Software' Software catalog
Our clusters provide a number of software packages to users of the system via the module
command.
Link to section 'Environment Management with the Module Command' of 'Environment Management with the Module Command' Environment Management with the Module Command
The module command is the preferred method to manage your processing environment. With this command, you may load applications and compilers along with their libraries and paths. Modules are packages that you load and unload as needed.
Please use the module command and do not manually configure your environment, as staff may make changes to the specifics of various packages. If you use the module command to manage your environment, these changes will not be noticeable.
Link to section 'Hierarchy' of 'Environment Management with the Module Command' Hierarchy
Many modules have dependencies on other modules. For example, a particular openmpi
module requires a specific version of the Intel compiler to be loaded. Often, these dependencies are not clear to users of the module, and there are many modules which may conflict. Arranging modules in a hierarchical fashion makes this dependency clear. This arrangement also helps make the software stack easy to understand - your view of the modules will not be cluttered with a bunch of conflicting packages.
Your default module view on Hammer will include a set of compilers and a set of basic software that has no dependencies (such as Matlab and Fluent). To make software available that depends on a compiler, you must first load the compiler, and then software which depends on it becomes available to you. In this way, all software you see when doing module avail is completely compatible with each other.
Link to section 'Using the Hierarchy' of 'Environment Management with the Module Command' Using the Hierarchy
Your default module view on Hammer will include a set of compilers and a set of basic software that has no dependencies (such as Matlab and Fluent).
To see what modules are available on this system by default:
$ module avail
To see which versions of a specific compiler are available on this system:
$ module avail gcc
$ module avail intel
To continue further into the hierarchy of modules, you will need to choose a compiler. As an example, if you are planning on using the Intel compiler you will first want to load
the Intel compiler:
$ module load intel
With intel
loaded, you can repeat the avail
command, and at the bottom of the output you will see the section of additional software that the intel module provides:
$ module avail
Several of these new packages also provide additional software packages, such as MPI libraries. You can repeat the last two steps with one of the MPI packages such as openmpi
and you will have a few more software packages available to you.
If you are looking for a specific software package and do not see it in your default view, the module command provides a search function for searching the entire hierarchy tree of modules without the need for you to manually load
and avail
on every module.
To search for a software package:
$ module spider openmpi
-------------------------------------------------------------------------------------------------------------------------------------------------------------------------------------
openmpi:
-------------------------------------------------------------------------------------------------------------------------------------------------------------------------------------
Versions:
openmpi/2.1.6
openmpi/3.1.4
openmpi/3.1.6
openmpi/4.0.5
openmpi/4.1.3
-------------------------------------------------------------------------------------------------------------------------------------------------------------------------------------
For detailed information about a specific "openmpi" package (including how to load the modules) use the module's full name.
Note that names that have a trailing (E) are extensions provided by other modules.
For example:
$ module spider openmpi/4.1.3
This will search for the openmpi
software package. If you do not specify a specific version of the package, you will be given a list of versions available on the system. Select the version you wish to use and spider
that to see how to access the module:
$ module spider openmpi/4.1.3
...
You will need to load all module(s) on any one of the lines below before the "openmpi/4.1.3" module is available to load.
aocc/2.1.0
gcc/10.2.0
gcc/4.8.5
gcc/6.3.0
gcc/9.3.0
intel/17.0.1.132
intel/19.0.5.281
...
The output of this command will instruct you that you can load this module directly, or in the case of the above example, that you will need to first load a module or two. With the information provided with this command, you can now construct a load
command to load a version of OpenMPI into your environment:
$ module load intel/19.0.5.281 openmpi/4.1.3
Some user communities may maintain copies of their domain software for others to use. For example, the Purdue Bioinformatics Core provides a wide set of bioinformatics software for use by any user of RCAC clusters via the bioinfo
module. The spider
command will also search this repository of modules. If it finds a software package available in the bioinfo module repository, the spider
command will instruct you to load the bioinfo module first.
Link to section 'Load / Unload a Module' of 'Environment Management with the Module Command' Load / Unload a Module
All modules consist of both a name and a version number. When loading a module, you may use only the name to load the default version, or you may specify which version you wish to load.
For each cluster, RCAC makes a recommendation regarding the set of compiler, math library, and MPI library for parallel code. To load the recommended set:
$ module load rcac
To verify what you loaded:
$ module list
To load the default version of a specific compiler, choose one of the following commands:
$ module load gcc
$ module load intel
To load a specific version of a compiler, include the version number:
$ module load gcc/11.2.0
When running a job, you must use the job submission file to load on the compute node(s) any relevant modules. Loading modules on the front end before submitting your job makes the software available to your session on the front-end, but not to your job submission script environment. You must load the necessary modules in your job submission script.
To unload a compiler or software package you loaded previously:
$ module unload gcc
$ module unload intel
$ module unload matlab
To unload all currently loaded modules and reset your environment:
$ module purge
Link to section 'Show Module Details' of 'Environment Management with the Module Command' Show Module Details
To learn more about what a module does to your environment, you may use the module show command.
$ module show matlab
Here is an example showing what loading the default Matlab does to the processing environment:
-------------------------------------------------------------------------------------------------------------------------------------------
/opt/spack/modulefiles/Core/matlab/R2022a.lua:
-------------------------------------------------------------------------------------------------------------------------------------------------------------------------------------
whatis("Name : matlab")
whatis("Version : R2022a")
...
setenv("MATLAB_HOME","/apps/spack/hammer/apps/matlab/R2022a-gcc-8.5.0-u54n6sa")
setenv("RCAC_MATLAB_ROOT","/apps/spack/hammer/apps/matlab/R2022a-gcc-8.5.0-u54n6sa")
setenv("RCAC_MATLAB_VERSION","R2022a")
setenv("MATLAB","/apps/spack/hammer/apps/matlab/R2022a-gcc-8.5.0-u54n6sa")
setenv("MLROOT","/apps/spack/hammer/apps/matlab/R2022a-gcc-8.5.0-u54n6sa")
setenv("ARCH","glnxa64")
append_path("PATH","/apps/spack/hammer/apps/matlab/R2022a-gcc-8.5.0-u54n6sa/bin/glnxa64:/apps/spack/hammer/apps/matlab/R2019a-gcc-4.8.5-jg35hvf/bin")
append_path("CMAKE_PREFIX_PATH","/apps/spack/hammer/apps/matlab/R2022a-gcc-8.5.0-u54n6sa/")
append_path("LD_LIBRARY_PATH","/apps/spack/hammer/apps/matlab/R2022a-gcc-8.5.0-u54n6sa/runtime/glnxa64:/apps/spack/hammer/apps/matlab/R2022a-gcc-8.5.0-u54n6sa/bin/glnxa64")
For more information about Lmod:
Compiling Source Code
Documentation on compiling source code on Hammer.
Compiling Serial Programs
A serial program is a single process which executes as a sequential stream of instructions on one processor core. Compilers capable of serial programming are available for C, C++, and versions of Fortran.
Here are a few sample serial programs:
- serial_hello.f
- serial_hello.f90
- serial_hello.f95
- serial_hello.c
-
To load a compiler, enter one of the following:
$ module load intel
$ module load gcc
Language | Intel Compiler | GNU Compiler | |
---|---|---|---|
Fortran 77 |
|
|
|
Fortran 90 |
|
|
|
Fortran 95 |
|
|
|
C |
|
|
|
C++ |
|
|
The Intel and GNU compilers will not output anything for a successful compilation. Also, the Intel compiler does not recognize the suffix ".f95".
Compiling OpenMP Programs
All compilers installed on Brown include OpenMP functionality for C, C++, and Fortran. An OpenMP program is a single process that takes advantage of a multi-core processor and its shared memory to achieve a form of parallel computing called multithreading. It distributes the work of a process over processor cores in a single compute node without the need for MPI communications.
Language | Header Files |
---|---|
Fortran 77 |
|
Fortran 90 |
|
Fortran 95 |
|
C |
|
C++ |
|
Sample programs illustrate task parallelism of OpenMP:
A sample program illustrates loop-level (data) parallelism of OpenMP:
To load a compiler, enter one of the following:
$ module load intel
$ module load gcc
Language | Intel Compiler | GNU Compiler |
---|---|---|
Fortran 77 |
|
|
Fortran 90 |
|
|
Fortran 95 |
|
|
C |
|
|
C++ |
|
|
The Intel and GNU compilers will not output anything for a successful compilation. Also, the Intel compiler does not recognize the suffix ".f95".
Here is some more documentation from other sources on OpenMP:
Intel MKL Library
Intel Math Kernel Library (MKL) contains ScaLAPACK, LAPACK, Sparse Solver, BLAS, Sparse BLAS, CBLAS, GMP, FFTs, DFTs, VSL, VML, and Interval Arithmetic routines. MKL resides in the directory stored in the environment variable MKL_HOME, after loading a version of the Intel compiler with module.
By using module load to load an Intel compiler your environment will have several variables set up to help link applications with MKL. Here are some example combinations of simplified linking options:
$ module load intel
$ echo $LINK_LAPACK
-L${MKL_HOME}/lib/intel64 -lmkl_intel_lp64 -lmkl_intel_thread -lmkl_core -liomp5 -lpthread
$ echo $LINK_LAPACK95
-L${MKL_HOME}/lib/intel64 -lmkl_lapack95_lp64 -lmkl_blas95_lp64 -lmkl_intel_lp64 -lmkl_intel_thread -lmkl_core -liomp5 -lpthread
RCAC recommends you use the provided variables to define MKL linking options in your compiling procedures. The Intel compiler modules also provide two other environment variables, LINK_LAPACK_STATIC and LINK_LAPACK95_STATIC that you may use if you need to link MKL statically.
RCAC recommends that you use dynamic linking of libguide. If so, define LD_LIBRARY_PATH such that you are using the correct version of libguide at run time. If you use static linking of libguide, then:
- If you use the Intel compilers, link in the libguide version that comes with the compiler (use the -openmp option).
- If you do not use the Intel compilers, link in the libguide version that comes with the Intel MKL above.
Here are some more documentation from other sources on the Intel MKL:
Compiling MPI Programs
OpenMPI and Intel MPI (IMPI) are implementations of the Message-Passing Interface (MPI) standard. Libraries for these MPI implementations and compilers for C, C++, and Fortran are available on all clusters.
Language | Header Files |
---|---|
Fortran 77 |
|
Fortran 90 |
|
Fortran 95 |
|
C |
|
C++ |
|
Here are a few sample programs using MPI:
To see the available MPI libraries:
$ module avail openmpi
$ module avail impi
Language | Intel MPI | OpenMPI |
---|---|---|
Fortran 77 |
|
|
Fortran 90 |
|
|
Fortran 95 |
|
|
C |
|
|
C++ |
|
|
The Intel and GNU compilers will not output anything for a successful compilation. Also, the Intel compiler does not recognize the suffix ".f95".
Here is some more documentation from other sources on the MPI libraries:
Running Jobs
There is one method for submitting jobs to Hammer. You may use SLURM to submit jobs to a partition on Hammer. SLURM performs job scheduling. Jobs may be any type of program. You may use either the batch or interactive mode to run your jobs. Use the batch mode for finished programs; use the interactive mode only for debugging.
In this section, you'll find a few pages describing the basics of creating and submitting SLURM jobs. As well, a number of example SLURM jobs that you may be able to adapt to your own needs.
PBS to Slurm
This is a reference for the most common command, environment variables, and job specification options used by the workload management systems and their equivalents.
Quick Guide
This table lists the most common command, environment variables, and job specification options used by the workload management systems and their equivalents (adapted from http://www.schedmd.com/slurmdocs/rosetta.html).
User Commands | PBS/Torque | Slurm |
---|---|---|
Job submission | qsub [script_file] |
sbatch [script_file] |
Interactive Job | qsub -I |
sinteractive |
Job deletion | qdel [job_id] |
scancel [job_id] |
Job status (by job) | qstat [job_id] |
squeue [-j job_id] |
Job status (by user) | qstat -u [user_name] |
squeue [-u user_name] |
Job hold | qhold [job_id] |
scontrol hold [job_id] |
Job release | qrls [job_id] |
scontrol release [job_id] |
Queue info | qstat -Q |
squeue |
Queue access | qlist |
slist |
Node list | pbsnodes -l |
sinfo -N scontrol show nodes |
Cluster status | qstat -a |
sinfo |
GUI | xpbsmon |
sview |
Environment | PBS/Torque | Slurm |
Job ID | $PBS_JOBID |
$SLURM_JOB_ID |
Job Name | $PBS_JOBNAME |
$SLURM_JOB_NAME |
Job Queue/Account | $PBS_QUEUE |
$SLURM_JOB_ACCOUNT |
Submit Directory | $PBS_O_WORKDIR |
$SLURM_SUBMIT_DIR |
Submit Host | $PBS_O_HOST |
$SLURM_SUBMIT_HOST |
Number of nodes | $PBS_NUM_NODES |
$SLURM_JOB_NUM_NODES |
Number of Tasks | $PBS_NP |
$SLURM_NTASKS |
Number of Tasks Per Node | $PBS_NUM_PPN |
$SLURM_NTASKS_PER_NODE |
Node List (Compact) | n/a | $SLURM_JOB_NODELIST |
Node List (One Core Per Line) | LIST=$(cat $PBS_NODEFILE) |
LIST=$(srun hostname) |
Job Array Index | $PBS_ARRAYID |
$SLURM_ARRAY_TASK_ID |
Job Specification | PBS/Torque | Slurm |
Script directive | #PBS |
#SBATCH |
Queue | -q [queue] |
-A [queue] |
Node Count | -l nodes=[count] |
-N [min[-max]] |
CPU Count | -l ppn=[count] |
-n [count] Note: total, not per node |
Wall Clock Limit | -l walltime=[hh:mm:ss] |
-t [min] OR-t [hh:mm:ss] OR-t [days-hh:mm:ss] |
Standard Output FIle | -o [file_name] |
-o [file_name] |
Standard Error File | -e [file_name] |
-e [file_name] |
Combine stdout/err | -j oe (both to stdout) OR-j eo (both to stderr) |
(use -o without -e) |
Copy Environment | -V |
--export=[ALL | NONE | variables] Note: default behavior is ALL |
Copy Specific Environment Variable | -v myvar=somevalue |
--export=NONE,myvar=somevalue OR--export=ALL,myvar=somevalue |
Event Notification | -m abe |
--mail-type=[events] |
Email Address | -M [address] |
--mail-user=[address] |
Job Name | -N [name] |
--job-name=[name] |
Job Restart | -r [y|n] |
--requeue OR--no-requeue |
Working Directory | --workdir=[dir_name] |
|
Resource Sharing | -l naccesspolicy=singlejob |
--exclusive OR--shared |
Memory Size | -l mem=[MB] |
--mem=[mem][M|G|T] OR--mem-per-cpu=[mem][M|G|T] |
Account to charge | -A [account] |
-A [account] |
Tasks Per Node | -l ppn=[count] |
--tasks-per-node=[count] |
CPUs Per Task | --cpus-per-task=[count] |
|
Job Dependency | -W depend=[state:job_id] |
--depend=[state:job_id] |
Job Arrays | -t [array_spec] |
--array=[array_spec] |
Generic Resources | -l other=[resource_spec] |
--gres=[resource_spec] |
Licenses | --licenses=[license_spec] |
|
Begin Time | -A "y-m-d h:m:s" |
--begin=y-m-d[Th:m[:s]] |
See the official Slurm Documentation for further details.
Notable Differences
-
Separate commands for Batch and Interactive jobs
Unlike PBS, in Slurm interactive jobs and batch jobs are launched with completely distinct commands.
Usesbatch [allocation request options] script
to submit a job to the batch scheduler, andsinteractive [allocation request options]
to launch an interactive job. sinteractive accepts most of the same allocation request options as sbatch does. -
No need for
cd $PBS_O_WORKDIR
In Slurm your batch job starts to run in the directory from which you submitted the script whereas in PBS/Torque you need to explicitly move back to that directory with
cd $PBS_O_WORKDIR
. -
No need to manually export environment
The environment variables that are defined in your shell session at the time that you submit the script are exported into your batch job, whereas in PBS/Torque you need to use the
-V
flag to export your environment. -
Location of output files
The output and error files are created in their final location immediately that the job begins or an error is generated, whereas in PBS/Torque temporary files are created that are only moved to the final location at the end of the job. Therefore in Slurm you can examine the output and error files from your job during its execution.
See the official Slurm Documentation for further details.
Basics of SLURM Jobs
The Simple Linux Utility for Resource Management (SLURM) is a system providing job scheduling and job management on compute clusters. With SLURM, a user requests resources and submits a job to a queue. The system will then take jobs from queues, allocate the necessary nodes, and execute them.
Do NOT run large, long, multi-threaded, parallel, or CPU-intensive jobs on a front-end login host. All users share the front-end hosts, and running anything but the smallest test job will negatively impact everyone's ability to use Hammer. Always use SLURM to submit your work as a job.
Link to section 'Submitting a Job' of 'Basics of SLURM Jobs' Submitting a Job
The main steps to submitting a job are:
Follow the links below for information on these steps, and other basic information about jobs. A number of example SLURM jobs are also available.
Job Submission Script
To submit work to a SLURM queue, you must first create a job submission file. This job submission file is essentially a simple shell script. It will set any required environment variables, load any necessary modules, create or modify files and directories, and run any applications that you need:
#!/bin/bash
# FILENAME: myjobsubmissionfile
# Loads Matlab and sets the application up
module load matlab
# Change to the directory from which you originally submitted this job.
cd $SLURM_SUBMIT_DIR
# Runs a Matlab script named 'myscript'
matlab -nodisplay -singleCompThread -r myscript
Once your script is prepared, you are ready to submit your job.
Link to section 'Job Script Environment Variables' of 'Job Submission Script' Job Script Environment Variables
Name | Description |
---|---|
SLURM_SUBMIT_DIR | Absolute path of the current working directory when you submitted this job |
SLURM_JOBID | Job ID number assigned to this job by the batch system |
SLURM_JOB_NAME | Job name supplied by the user |
SLURM_JOB_NODELIST | Names of nodes assigned to this job |
SLURM_CLUSTER_NAME | Name of the cluster executing the job |
SLURM_SUBMIT_HOST | Hostname of the system where you submitted this job |
SLURM_JOB_PARTITION | Name of the original queue to which you submitted this job |
Submitting a Job
Once you have a job submission file, you may submit this script to SLURM using the sbatch
command. SLURM will find, or wait for, available resources matching your request and run your job there.
To submit your job to one compute node:
$ sbatch --nodes=1 myjobsubmissionfile
Slurm uses the word 'Account' and the option '-A' to specify different batch queues. To submit your job to a specific queue:
$ sbatch --nodes=1 -A standby myjobsubmissionfile
By default, each job receives 30 minutes of wall time, or clock time. If you know that your job will not need more than a certain amount of time to run, request less than the maximum wall time, as this may allow your job to run sooner. To request the 1 hour and 30 minutes of wall time:
$ sbatch -t 1:30:00 --nodes=1 -A standby myjobsubmissionfile
The --nodes
value indicates how many compute nodes you would like for your job.
Each compute node in Hammer has 20 processor cores.
In some cases, you may want to request multiple nodes. To utilize multiple nodes, you will need to have a program or code that is specifically programmed to use multiple nodes such as with MPI. Simply requesting more nodes will not make your work go faster. Your code must support this ability.
To request 2 compute nodes:
$ sbatch --nodes=2 myjobsubmissionfile
SLURM jobs will have exclusive access to compute nodes and other jobs will not use the same nodes. SLURM will allow a single job to run multiple tasks
, and those tasks can be allocated resources with the --ntasks
option.
To submit a job using 1 compute node with 4 tasks, each using the default 1 core and 1 GPU per node:
$ sbatch --nodes=1 --ntasks=4 --gpus-per-node=1 myjobsubmissionfile
If more convenient, you may also specify any command line options to sbatch
from within your job submission file, using a special form of comment:
#!/bin/sh -l
# FILENAME: myjobsubmissionfile
#SBATCH -A myqueuename
#SBATCH --nodes=1
#SBATCH --time=1:30:00
#SBATCH --job-name myjobname
# Print the hostname of the compute node on which this job is running.
/bin/hostname
If an option is present in both your job submission file and on the command line, the option on the command line will take precedence.
After you submit your job with SBATCH
, it may wait in queue for minutes, hours, or even weeks. How long it takes for a job to start depends on the specific queue, the resources and time requested, and other jobs already waiting in that queue requested as well. It is impossible to say for sure when any given job will start. For best results, request no more resources than your job requires.
Once your job is submitted, you can monitor the job status, wait for the job to complete, and check the job output.
Checking Job Status
Once a job is submitted there are several commands you can use to monitor the progress of the job.
To see your jobs, use the squeue -u command and specify your username:
(Remember, in our SLURM environment a queue is referred to as an 'Account')
squeue -u myusername
JOBID ACCOUNT NAME USER ST TIME NODES NODELIST(REASON)
182792 standby job1 myusername R 20:19 1 hammer-a000
185841 standby job2 myusername R 20:19 1 hammer-a001
185844 standby job3 myusername R 20:18 1 hammer-a002
185847 standby job4 myusername R 20:18 1 hammer-a003
To retrieve useful information about your queued or running job, use the scontrol show job command with your job's ID number. The output should look similar to the following:
scontrol show job 3519
JobId=3519 JobName=t.sub
UserId=myusername GroupId=mygroup MCS_label=N/A
Priority=3 Nice=0 Account=(null) QOS=(null)
JobState=PENDING Reason=BeginTime Dependency=(null)
Requeue=1 Restarts=0 BatchFlag=1 Reboot=0 ExitCode=0:0
RunTime=00:00:00 TimeLimit=7-00:00:00 TimeMin=N/A
SubmitTime=2019-08-29T16:56:52 EligibleTime=2019-08-29T23:30:00
AccrueTime=Unknown
StartTime=2019-08-29T23:30:00 EndTime=2019-09-05T23:30:00 Deadline=N/A
PreemptTime=None SuspendTime=None SecsPreSuspend=0
LastSchedEval=2019-08-29T16:56:52
Partition=workq AllocNode:Sid=mack-fe00:54476
ReqNodeList=(null) ExcNodeList=(null)
NodeList=(null)
NumNodes=1 NumCPUs=2 NumTasks=2 CPUs/Task=1 ReqB:S:C:T=0:0:*:*
TRES=cpu=2,node=1,billing=2
Socks/Node=* NtasksPerN:B:S:C=0:0:*:* CoreSpec=*
MinCPUsNode=1 MinMemoryNode=0 MinTmpDiskNode=0
Features=(null) DelayBoot=00:00:00
OverSubscribe=OK Contiguous=0 Licenses=(null) Network=(null)
Command=/home/myusername/jobdir/myjobfile.sub
WorkDir=/home/myusername/jobdir
StdErr=/home/myusername/jobdir/slurm-3519.out
StdIn=/dev/null
StdOut=/home/myusername/jobdir/slurm-3519.out
Power=
There are several useful bits of information in this output.
JobState
lets you know if the job is Pending, Running, Completed, or Held.RunTime and TimeLimit
will show how long the job has run and its maximum time.SubmitTime
is when the job was submitted to the cluster.NumNodes
,NumCPUs
,NumTasks
andCPUs/Task
are the number of Nodes, CPUs, Tasks, and CPUs per Task are shown.WorkDir
is the job's working directory.StdOut
andStderr
are the locations of stdout and stderr of the job, respectively.Reason
will show why aPENDING
job isn't running. The above error says that it has been requested to start at a specific, later time.
Checking Job Output
Once a job is submitted, and has started, it will write its standard output and standard error to files that you can read.
SLURM catches output written to standard output and standard error - what would be printed to your screen if you ran your program interactively. Unless you specfied otherwise, SLURM will put the output in the directory where you submitted the job in a file named slurm-
followed by the job id
, with the extension out
. For example slurm-3509.out
. Note that both stdout and stderr will be written into the same file, unless you specify otherwise.
If your program writes its own output files, those files will be created as defined by the program. This may be in the directory where the program was run, or may be defined in a configuration or input file. You will need to check the documentation for your program for more details.
Link to section 'Redirecting Job Output' of 'Checking Job Output' Redirecting Job Output
It is possible to redirect job output to somewhere other than the default location with the --error
and --output
directives:
#!/bin/bash
#SBATCH --output=/home/myusername/joboutput/myjob.out
#SBATCH --error=/home/myusername/joboutput/myjob.out
# This job prints "Hello World" to output and exits
echo "Hello World"
Holding a Job
Sometimes you may want to submit a job but not have it run just yet. You may be wanting to allow lab mates to cut in front of you in the queue - so hold the job until their jobs have started, and then release yours.
To place a hold on a job before it starts running, use the scontrol hold job command:
$ scontrol hold job myjobid
Once a job has started running it can not be placed on hold.
To release a hold on a job, use the scontrol release job command:
$ scontrol release job myjobid
You find the job ID using the squeue command as explained in the SLURM Job Status section.
Job Dependencies
Dependencies are an automated way of holding and releasing jobs. Jobs with a dependency are held until the condition is satisfied. Once the condition is satisfied jobs only then become eligible to run and must still queue as normal.
Job dependencies may be configured to ensure jobs start in a specified order. Jobs can be configured to run after other job state changes, such as when the job starts or the job ends.
These examples illustrate setting dependencies in several ways. Typically dependencies are set by capturing and using the job ID from the last job submitted.
To run a job after job myjobid has started:
sbatch --dependency=after:myjobid myjobsubmissionfile
To run a job after job myjobid ends without error:
sbatch --dependency=afterok:myjobid myjobsubmissionfile
To run a job after job myjobid ends with errors:
sbatch --dependency=afternotok:myjobid myjobsubmissionfile
To run a job after job myjobid ends with or without errors:
sbatch --dependency=afterany:myjobid myjobsubmissionfile
To set more complex dependencies on multiple jobs and conditions:
sbatch --dependency=after:myjobid1:myjobid2:myjobid3,afterok:myjobid4 myjobsubmissionfile
Canceling a Job
To stop a job before it finishes or remove it from a queue, use the scancel command:
scancel myjobid
You find the job ID using the squeue command as explained in the SLURM Job Status section.
Queues
Link to section '"mylab" Queues' of 'Queues' "mylab" Queues
Hammer, as a community cluster, has one or more queues dedicated to and named after each partner who has purchased access to the cluster. These queues provide partners and their researchers with priority access to their portion of the cluster. Jobs in these queues are typically limited to 336 hours. The expectation is that any jobs submitted to your research lab queues will start within 4 hours, assuming the queue currently has enough capacity for the job (that is, your lab mates aren't using all of the cores currently).
Link to section 'Standby Queue' of 'Queues' Standby Queue
Additionally, community clusters provide a "standby" queue which is available to all cluster users. This "standby" queue allows users to utilize portions of the cluster that would otherwise be idle, but at a lower priority than partner-queue jobs, and with a relatively short time limit, to ensure "standby" jobs will not be able to tie up resources and prevent partner-queue jobs from running quickly. Jobs in standby are limited to 4 hours. There is no expectation of job start time. If the cluster is very busy with partner queue jobs, or you are requesting a very large job, jobs in standby may take hours or days to start.
Link to section 'Debug Queue' of 'Queues' Debug Queue
The debug queue allows you to quickly start small, short, interactive jobs in order to debug code, test programs, or test configurations. You are limited to one running job at a time in the queue, and you may run up to two compute nodes for 30 minutes. The expectation is that debug jobs should start within a couple of minutes, assuming all of its dedicated nodes are not taken by others.
Link to section 'List of Queues' of 'Queues' List of Queues
To see a list of all queues on Hammer that you may submit to, use the slist command
This lists each queue you can submit to, the number of nodes allocated to the queue, how many are available to run jobs, and the maximum walltime you may request. Options to the command will give more detailed information. This command can be used to get a general idea of how busy an individual queue is and how long you may have to wait for your job to start.
Example Jobs
A number of example jobs are available for you to look over and adapt to your own needs. The first few are generic examples, and latter ones go into specifics for particular software packages.
Generic SLURM Jobs
The following examples demonstrate the basics of SLURM jobs, and are designed to cover common job request scenarios. These example jobs will need to be modified to run your application or code.
Simple Job
Every SLURM job consists of a job submission file. A job submission file contains a list of commands that run your program and a set of resource (nodes, walltime, queue) requests. The resource requests can appear in the job submission file or can be specified at submit-time as shown below.
This simple example submits the job submission file hello.sub to the standby queue on Hammer and requests a single node:
#!/bin/bash
# FILENAME: hello.sub
# Show this ran on a compute node by running the hostname command.
hostname
echo "Hello World"
sbatch -A standby --nodes=1 --ntasks=1 --cpus-per-task=1 --time=00:01:00 hello.sub
Submitted batch job 3521
For a real job you would replace echo "Hello World"
with a command, or sequence of commands, that run your program.
After your job finishes running, the ls command will show a new file in your directory, the .out file:
ls -l
hello.sub
slurm-3521.out
The file slurm-3521.out contains the output and errors your program would have written to the screen if you had typed its commands at a command prompt:
cat slurm-3521.out
hammer-a001.rcac.purdue.edu
Hello World
You should see the hostname of the compute node your job was executed on. Following should be the "Hello World" statement.
Multiple Node
In some cases, you may want to request multiple nodes. To utilize multiple nodes, you will need to have a program or code that is specifically programmed to use multiple nodes such as with MPI. Simply requesting more nodes will not make your work go faster. Your code must support this ability.
This example shows a request for multiple compute nodes. The job submission file contains a single command to show the names of the compute nodes allocated:
# FILENAME: myjobsubmissionfile.sub
#!/bin/bash
echo "$SLURM_JOB_NODELIST"
sbatch --nodes=2 --ntasks=40 --time=00:10:00 -A standby myjobsubmissionfile.sub
Compute nodes allocated:
hammer-a[014-015]
The above example will allocate the total of 40 CPU cores across 2 nodes. Note that if your multi-node job requests fewer than each node's full 20 cores per node, by default Slurm provides no guarantee with respect to how this total is distributed between assigned nodes (i.e. the cores may not necessarily be split evenly). If you need specific arrangements of your tasks and cores, you can use --cpus-per-task= and/or --ntasks-per-node= flags. See Slurm documentation or man sbatch for more options.
Directives
So far these examples have shown submitting jobs with the resource requests on the sbatch
command line such as:
sbatch -A standby --nodes=1 --time=00:01:00 hello.sub
The resource requests can also be put into job submission file itself. Documenting the resource requests in the job submission is desirable because the job can be easily reproduced later. Details left in your command history are quickly lost. Arguments are specified with the #SBATCH
syntax:
#!/bin/bash
# FILENAME: hello.sub
#SBATCH -A standby
#SBATCH --nodes=1 --time=00:01:00
# Show this ran on a compute node by running the hostname command.
hostname
echo "Hello World"
The #SBATCH
directives must appear at the top of your submission file. SLURM will stop parsing directives as soon as it encounters a line that does not start with '#'. If you insert a directive in the middle of your script, it will be ignored.
This job can be then submitted with:
sbatch hello.sub
Specific Types of Nodes
SLURM allows running a job on specific types of compute nodes to accommodate special hardware requirements (e.g. a certain CPU or GPU type, etc.)
Cluster nodes have a set of descriptive features assigned to them, and users can specify which of these features are required by their job by using the constraint option at submission time. Only nodes having features matching the job constraints will be used to satisfy the request.
Example: a job requires a compute node in an "A" sub-cluster:
sbatch --nodes=1 --ntasks=20 --constraint=A myjobsubmissionfile.sub
Compute node allocated:
hammer-a003
Feature constraints can be used for both batch and interactive jobs, as well as for individual job steps inside a job. Multiple constraints can be specified with a predefined syntax to achieve complex request logic (see detailed description of the '--constraint' option in man sbatch
or online Slurm documentation).
Refer to Detailed Hardware Specification section for list of available sub-cluster labels, their respective per-node memory sizes and other hardware details. You could also use sfeatures
command to list available constraint feature names for different node types.
Interactive Jobs
Interactive jobs are run on compute nodes, while giving you a shell to interact with. They give you the ability to type commands or use a graphical interface in the same way as if you were on a front-end login host.
To submit an interactive job, use sinteractive to run a login shell on allocated resources.
sinteractive accepts most of the same resource requests as sbatch, so to request a login shell on the cpu account while allocating 2 nodes and 20 total cores, you might do:
sinteractive -A cpu -N2 -n40
To quit your interactive job:
exit
or Ctrl-D
The above example will allocate the total of 40 CPU cores across 2 nodes. Note that if your multi-node job requests fewer than each node's full 20 cores per node, by default Slurm provides no guarantee with respect to how this total is distributed between assigned nodes (i.e. the cores may not necessarily be split evenly). If you need specific arrangements of your tasks and cores, you can use --cpus-per-task= and/or --ntasks-per-node= flags. See Slurm documentation or man salloc for more options.
Serial Jobs
This shows how to submit one of the serial programs compiled in the section Compiling Serial Programs.
Create a job submission file:
#!/bin/bash
# FILENAME: serial_hello.sub
./serial_hello
Submit the job:
sbatch --nodes=1 --ntasks=1 --time=00:01:00 serial_hello.sub
After the job completes, view results in the output file:
cat slurm-myjobid.out
Runhost:hammer-a009.rcac.purdue.edu
hello, world
If the job failed to run, then view error messages in the file slurm-myjobid.out.
OpenMP
A shared-memory job is a single process that takes advantage of a multi-core processor and its shared memory to achieve parallelization.
This example shows how to submit an OpenMP program compiled in the section Compiling OpenMP Programs.
When running OpenMP programs, all threads must be on the same compute node to take advantage of shared memory. The threads cannot communicate between nodes.
To run an OpenMP program, set the environment variable OMP_NUM_THREADS to the desired number of threads:
In csh:
setenv OMP_NUM_THREADS 20
In bash:
export OMP_NUM_THREADS=20
This should almost always be equal to the number of cores on a compute node. You may want to set to another appropriate value if you are running several processes in parallel in a single job or node.
Create a job submissionfile:
#!/bin/bash
# FILENAME: omp_hello.sub
#SBATCH --nodes=1
#SBATCH --ntasks=20
#SBATCH --time=00:01:00
export OMP_NUM_THREADS=20
./omp_hello
Submit the job:
sbatch omp_hello.sub
View the results from one of the sample OpenMP programs about task parallelism:
cat omp_hello.sub.omyjobid
SERIAL REGION: Runhost:hammer-a003.rcac.purdue.edu Thread:0 of 1 thread hello, world
PARALLEL REGION: Runhost:hammer-a003.rcac.purdue.edu Thread:0 of 20 threads hello, world
PARALLEL REGION: Runhost:hammer-a003.rcac.purdue.edu Thread:1 of 20 threads hello, world
...
If the job failed to run, then view error messages in the file slurm-myjobid.out.
If an OpenMP program uses a lot of memory and 20 threads use all of the memory of the compute node, use fewer processor cores (OpenMP threads) on that compute node.
MPI
An MPI job is a set of processes that take advantage of multiple compute nodes by communicating with each other. OpenMPI and Intel MPI (IMPI) are implementations of the MPI standard.
This section shows how to submit one of the MPI programs compiled in the section Compiling MPI Programs.
Use module load to set up the paths to access these libraries. Use module avail to see all MPI packages installed on Hammer.
Create a job submission file:
#!/bin/bash
# FILENAME: mpi_hello.sub
#SBATCH --nodes=2
#SBATCH --ntasks-per-node=20
#SBATCH --time=00:01:00
#SBATCH -A standby
srun -n 40 ./mpi_hello
SLURM can run an MPI program with the srun command. The number of processes is requested with the -n option. If you do not specify the -n option, it will default to the total number of processor cores you request from SLURM.
If the code is built with OpenMPI, it can be run with a simple srun -n command. If it is built with Intel IMPI, then you also need to add the --mpi=pmi2 option: srun --mpi=pmi2 -n 40 ./mpi_hello in this example.
Submit the MPI job:
sbatch ./mpi_hello.sub
View results in the output file:
cat slurm-myjobid.out
Runhost:hammer-a010.rcac.purdue.edu Rank:0 of 40 ranks hello, world
Runhost:hammer-a010.rcac.purdue.edu Rank:1 of 40 ranks hello, world
...
Runhost:hammer-a011.rcac.purdue.edu Rank:20 of 40 ranks hello, world
Runhost:hammer-a011.rcac.purdue.edu Rank:21 of 40 ranks hello, world
...
If the job failed to run, then view error messages in the output file.
If an MPI job uses a lot of memory and 20 MPI ranks per compute node use all of the memory of the compute nodes, request more compute nodes, while keeping the total number of MPI ranks unchanged.
Submit the job with double the number of compute nodes and modify the resource request to halve the number of MPI ranks per compute node.
#!/bin/bash
# FILENAME: mpi_hello.sub
#SBATCH --nodes=4
#SBATCH --ntasks-per-node=10
#SBATCH -t 00:01:00
#SBATCH -A standby
srun -n 40 ./mpi_hello
sbatch ./mpi_hello.sub
View results in the output file:
cat slurm-myjobid.out
Runhost:hammer-a10.rcac.purdue.edu Rank:0 of 40 ranks hello, world
Runhost:hammer-a010.rcac.purdue.edu Rank:1 of 40 ranks hello, world
...
Runhost:hammer-a011.rcac.purdue.edu Rank:10 of 40 ranks hello, world
...
Runhost:hammer-a012.rcac.purdue.edu Rank:20 of 40 ranks hello, world
...
Runhost:hammer-a013.rcac.purdue.edu Rank:30 of 40 ranks hello, world
...
Notes
- Use slist to determine which queues (
--account
or-A
option) are available to you. The name of the queue which is available to everyone on Hammer is "standby". - Invoking an MPI program on Hammer with ./program is typically wrong, since this will use only one MPI process and defeat the purpose of using MPI. Unless that is what you want (rarely the case), you should use srun or mpiexec to invoke an MPI program.
- In general, the exact order in which MPI ranks output similar write requests to an output file is random.
Link to section 'Collecting System Resource Utilization Data' of 'Monitoring Resources' Collecting System Resource Utilization Data
Knowing the precise resource utilization an application had during a job, such as CPU load or memory, can be incredibly useful. This is especially the case when the application isn't performing as expected.
One approach is to run a program like htop
during an interactive job and keep an eye on system resources. You can get precise time-series data from nodes associated with your job using XDmod as well, online. But these methods don't gather telemetry in an automated fashion, nor do they give you control over the resolution or format of the data.
As a matter of course, a robust implementation of some HPC workload would include resource utilization data as a diagnostic tool in the event of some failure.
The monitor
utility is a simple command line system resource monitoring tool for gathering such telemetry and is available as a module.
module load monitor
Complete documentation is available online at resource-monitor.readthedocs.io. A full manual page is also available for reference, man monitor
.
In the context of a SLURM job you will need to put this monitoring task in the background to allow the rest of your job script to proceed. Be sure to interrupt these tasks at the end of your job.
#!/bin/bash
# FILENAME: monitored_job.sh
module load monitor
# track per-code CPU load
monitor cpu percent --all-cores >cpu-percent.log &
CPU_PID=$!
# track memory usage
monitor cpu memory >cpu-memory.log &
MEM_PID=$!
# your code here
# shut down the resource monitors
kill -s INT $CPU_PID $MEM_PID
A particularly elegant solution would be to include such tools in your prologue script and have the tear down in your epilogue script.
For large distributed jobs spread across multiple nodes, mpiexec
can be used to gather telemetry from all nodes in the job. The hostname is included in each line of output so that data can be grouped as such. A concise way of constructing the needed list of hostnames in SLURM is to simply use srun hostname | sort -u
.
#!/bin/bash
# FILENAME: monitored_job.sh
module load monitor
# track all CPUs (one monitor per host)
mpiexec -machinefile <(srun hostname | sort -u) \
monitor cpu percent --all-cores >cpu-percent.log &
CPU_PID=$!
# track memory on all hosts (one monitor per host)
mpiexec -machinefile <(srun hostname | sort -u) \
monitor cpu memory >cpu-memory.log &
MEM_PID=$!
# your code here
# shut down the resource monitors
kill -s INT $CPU_PID $MEM_PID
To get resource data in a more readily computable format, the monitor
program can be told to output in CSV format with the --csv
flag.
monitor cpu memory --csv >cpu-memory.csv
For a distributed job you will need to suppress the header lines otherwise one will be created by each host.
monitor cpu memory --csv | head -1 >cpu-memory.csv
mpiexec -machinefile <(srun hostname | sort -u) \
monitor cpu memory --csv --no-header >>cpu-memory.csv
Specific Applications
The following examples demonstrate job submission files for some common real-world applications. See the Generic SLURM Examples section for more examples on job submissions that can be adapted for use.
Gaussian
Gaussian is a computational chemistry software package which works on electronic structure. This section illustrates how to submit a small Gaussian job to a Slurm queue. This Gaussian example runs the Fletcher-Powell multivariable optimization.
Prepare a Gaussian input file with an appropriate filename, here named myjob.com. The final blank line is necessary:
#P TEST OPT=FP STO-3G OPTCYC=2
STO-3G FLETCHER-POWELL OPTIMIZATION OF WATER
0 1
O
H 1 R
H 1 R 2 A
R 0.96
A 104.
To submit this job, load Gaussian then run the provided script, named subg16. This job uses one compute node with 20 processor cores:
module load gaussian16
subg16 myjob -N 1 -n 20
View job status:
squeue -u myusername
View results in the file for Gaussian output, here named myjob.log. Only the first and last few lines appear here:
Entering Gaussian System, Link 0=/apps/cent7/gaussian/g16-A.03/g16-haswell/g16/g16
Initial command:
/apps/cent7/gaussian/g16-A.03/g16-haswell/g16/l1.exe ${resource.scratch}/m/myusername/gaussian/Gau-7781.inp -scrdir=${resource.scratch}/m/myusername/gaussian/
Entering Link 1 = /apps/cent7/gaussian/g16-A.03/g16-haswell/g16/l1.exe PID= 7782.
Copyright (c) 1988,1990,1992,1993,1995,1998,2003,2009,2016,
Gaussian, Inc. All Rights Reserved.
.
.
.
Job cpu time: 0 days 0 hours 3 minutes 28.2 seconds.
Elapsed time: 0 days 0 hours 0 minutes 12.9 seconds.
File lengths (MBytes): RWF= 17 Int= 0 D2E= 0 Chk= 2 Scr= 2
Normal termination of Gaussian 16 at Tue May 1 17:12:00 2018.
real 13.85
user 202.05
sys 6.12
Machine:
hammer-a012.rcac.purdue.edu
hammer-a012.rcac.purdue.edu
hammer-a012.rcac.purdue.edu
hammer-a012.rcac.purdue.edu
hammer-a012.rcac.purdue.edu
hammer-a012.rcac.purdue.edu
hammer-a012.rcac.purdue.edu
hammer-a012.rcac.purdue.edu
Link to section 'Examples of Gaussian SLURM Job Submissions' of 'Gaussian' Examples of Gaussian SLURM Job Submissions
Submit job using 20 processor cores on a single node:
subg16 myjob -N 1 -n 20 -t 200:00:00 -A myqueuename
Submit job using 20 processor cores on each of 2 nodes:
subg16 myjob -N 2 --ntasks-per-node=20 -t 200:00:00 -A myqueuename
To submit a bash job, a submit script sample looks like:
#!/bin/bash
#SBATCH -A myqueuename # Queue name(use 'slist' command to find queues' name)
#SBATCH --nodes=1 # Total # of nodes
#SBATCH --ntasks=64 # Total # of MPI tasks
#SBATCH --time=1:00:00 # Total run time limit (hh:mm:ss)
#SBATCH -J myjobname # Job name
#SBATCH -o myjob.o%j # Name of stdout output file
#SBATCH -e myjob.e%j # Name of stderr error file
module load gaussian16
g16 < myjob.com
For more information about Gaussian:
Machine Learning
We support several common machine learning (ML) frameworks on the community clusters through pre-installed modules. The collection of these pre-installed ML modules is referred to as ml-toolkit throughout this documentation. Currently, the following libraries are included in ML-Toolkit.
caffe cntk gym keras
mxnet opencv pytorch
tensorflow tflearn theano
Note that managing dependencies with ML applications can be non-trivial, therefore, we recommend users start by using ml-toolkit. If a custom installation is required after trying ml-toolkit, make sure to read documentation carefully.
ML-Toolkit
A set of pre-installed popular machine learning (ML) libraries, called ML-Toolkit is maintained on Hammer. These are Anaconda/Python-based distributions of the respective libraries. Currently, applications are supported for Python 2 and 3. Detailed instructions for searching and using the installed ML applications are presented below.
Link to section 'Instructions for using ML-Toolkit Modules' of 'ML-Toolkit' Instructions for using ML-Toolkit Modules
Link to section 'Find and Use Installed ML Packages' of 'ML-Toolkit' Find and Use Installed ML Packages
To search or load a machine learning application, you must first load one of the learning modules. The learning module loads the prerequisites (such as anaconda) and makes ML applications visible to the user.
Step 1. Find and load a preferred learning module. Several learning modules may be available, corresponding to a specific Python version and whether the ML applications have GPU support or not. Running module load learning without specifying a version will load the version with the most recent python version. To see all available modules, run module spider learning then load the desired module.
Step 2. Find and load the desired machine learning libraries
ML packages are installed under the common application name ml-toolkit-cpu
You can use the module spider ml-toolkit command to see all options and versions of each library.
Load the desired modules using the module load command. Note that both CPU and GPU options may exist for many libraries, so be sure to load the correct version. For example, if you wanted to load the most recent version of PyTorch for CPU, you would run module load ml-toolkit-cpu/pytorch
caffe cntk gym keras mxnet
opencv pytorch tensorflow tflearn theano
Step 3. You can list which ML applications are loaded in your environment using the command module list
Link to section 'Verify application import' of 'ML-Toolkit' Verify application import
Step 4. The next step is to check that you can actually use the desired ML application. You can do this by running the import command in Python. The example below tests if PyTorch has been loaded correctly.
python -c "import torch; print(torch.__version__)"
If the import operation succeeded, then you can run your own ML code. Some ML applications (such as tensorflow) print diagnostic warnings while loading -- this is the expected behavior.
If the import fails with an error, please see the troubleshooting information below.
Step 5. To load a different set of applications, unload the previously loaded applications and load the new desired applications. The example below loads Tensorflow and Keras instead of PyTorch and OpenCV.
module unload ml-toolkit-cpu/opencv
module unload ml-toolkit-cpu/pytorch
module load ml-toolkit-cpu/tensorflow
module load ml-toolkit-cpu/keras
Link to section 'Troubleshooting' of 'ML-Toolkit' Troubleshooting
ML applications depend on a wide range of Python packages and mixing multiple versions of these packages can lead to error. The following guidelines will assist you in identifying the cause of the problem.
- Check that you are using the correct version of Python with the command python --version. This should match the Python version in the loaded anaconda module.
- Start from a clean environment. Either start a new terminal session or unload all the modules using module purge. Then load the desired modules following Steps 1-2.
- Verify that PYTHONPATH does not point to undesired packages. Run the following command to print PYTHONPATH: echo $PYTHONPATH. Make sure that your Python environment is clean. Watch out for any locally installed packages that might conflict.
- Note that Caffe has a conflicting version of PyQt5. So, if you want to use Spyder (or any GUI application that uses PyQt), then you should unload the caffe module.
- Use Google search to your advantage. Copy the error message in Google and check probable causes.
More examples showing how to use ml-toolkit modules in a batch job are presented in ML Batch Jobs guide.
Link to section 'Running ML Code in a Batch Job' of 'ML Batch Jobs' Running ML Code in a Batch Job
Batch jobs allow us to automate model training without human intervention. They are also useful when you need to run a large number of simulations on the clusters. In the example below, we shall run a simple tensor_hello.py script in a batch job. We consider two situations: in the first example, we use the ML-Toolkit modules to run tensorflow, while in the second example, we use a custom installation of tensorflow (See Custom ML Packages page).
Link to section 'Using ML-Toolkit Modules' of 'ML Batch Jobs' Using ML-Toolkit Modules
Save the following code as tensor_hello.sub in the same directory where tensor_hello.py is located.
# filename: tensor_hello.sub
#SBATCH --nodes=1
#SBATCH --ntasks-per-node=20
#SBATCH --time=00:05:00
#SBATCH -A standby
#SBATCH -J hello_tensor
module purge
module load learning
module load ml-toolkit-cpu/tensorflow
module list
python tensor_hello.py
Link to section 'Using a Custom Installation' of 'ML Batch Jobs' Using a Custom Installation
Save the following code as tensor_hello.sub in the same directory where tensor_hello.py is located.
# filename: tensor_hello.sub
#SBATCH --nodes=1
#SBATCH --ntasks-per-node=20
#SBATCH --time=00:05:00
#SBATCH -A standby
#SBATCH -J hello_tensor
module purge
module load anaconda
module load use.own
module load conda-env/my_tf_env-py3.6.4
module list
echo $PYTHONPATH
python tensor_hello.py
Link to section 'Running a Job' of 'ML Batch Jobs' Running a Job
Now you can submit the batch job using the sbatch command.
sbatch tensor_hello.sub
Once the job finishes, you will find an output file (slurm-xxxxx.out).
Link to section 'Installation of Custom ML Libraries' of 'Custom ML Packages' Installation of Custom ML Libraries
While we try to include as many common ML frameworks and versions as we can in ML-Toolkit, we recognize that there are also situations in which a custom installation may be preferable. We recommend using conda-env-mod to install and manage Python packages. Please follow the steps carefully, otherwise you may end up with a faulty installation. The example below shows how to install TensorFlow in your home directory.
Link to section 'Install' of 'Custom ML Packages' Install
Step 1: Unload all modules and start with a clean environment.
module purge
Step 2: Load the anaconda module with desired Python version.
module load anaconda
Step 3: Create a custom anaconda environment. Make sure the python version matches the Python version in the anaconda module.
conda-env-mod create -n env_name_here
Step 4: Activate the anaconda environment by loading the modules displayed at the end of step 3.
module load use.own
module load conda-env/env_name_here-py3.6.4
Step 5: Now install the desired ML application. You can install multiple Python packages at this step using either conda or pip.
pip install --ignore-installed tensorflow==2.6
If the installation succeeded, you can now proceed to testing and using the installed application. You must load the environment you created as well as any supporting modules (e.g., anaconda) whenever you want to use this installation. If your installation did not succeed, please refer to the troubleshooting section below as well as documentation for the desired package you are installing.
Note that loading the modules generated by conda-env-mod has different behavior than conda create env_name_here followed by source activate env_name_here. After running source activate, you may not be able to access any Python packages in anaconda or ml-toolkit modules. Therefore, using conda-env-mod is the preferred way of using your custom installations.
Link to section 'Testing the Installation' of 'Custom ML Packages' Testing the Installation
-
Verify the installation by using a simple import statement, like that listed below for TensorFlow:
python -c "import tensorflow as tf; print(tf.__version__);"
Note that a successful import of TensorFlow will print a variety of system and hardware information. This is expected.
If importing the package leads to errors, be sure to verify that all dependencies for the package have been managed, and the correct versions installed. Dependency issues between python packages are the most common cause for errors. For example, in TF, conflicts with the h5py or numpy versions are common, but upgrading those packages typically solves the problem. Managing dependencies for ML libraries can be non-trivial.
-
Link to section 'Troubleshooting' of 'Custom ML Packages' Troubleshooting
In most situations, dependencies among Python modules lead to errors. If you cannot use a Python package after installing it, please follow the steps below to find a workaround.
- Unload all the modules.
module purge
- Clean up PYTHONPATH.
unset PYTHONPATH
- Next load the modules, e.g., anaconda and your custom environment.
module load anaconda module load use.own module load conda-env/env_name_here-py3.6.4
- Now try running your code again.
- A few applications only run on specific versions of Python (e.g. Python 3.6). Please check the documentation of your application if that is the case.
- If you have installed a newer version of an ml-toolkit package (e.g., a newer version of PyTorch or Tensorflow), make sure that the ml-toolkit modules are NOT loaded. In general, we recommend that you don't mix ml-toolkit modules with your custom installations.
Link to section 'Tensorboard' of 'Custom ML Packages' Tensorboard
- You can visualize data from a Tensorflow session using Tensorboard. For this, you need to save your session summary as described in the Tensorboard User Guide.
- Launch Tensorboard:
$ python -m tensorboard.main --logdir=/path/to/session/logs
- When Tensorboard is launched successfully, it will give you the URL for accessing Tensorboard.
<... build related warnings ...> TensorBoard 0.4.0 at http://hammer-a000.rcac.purdue.edu:6006
- Follow the printed URL to visualize your model.
- Please note that due to firewall rules, the Tensorboard URL may only be accessible from Hammer nodes. If you cannot access the URL directly, you can use Firefox browser in Thinlinc.
- For more details, please refer to the Tensorboard User Guide.
- Unload all the modules.
Matlab
MATLAB® (MATrix LABoratory) is a high-level language and interactive environment for numerical computation, visualization, and programming. MATLAB is a product of MathWorks.
MATLAB, Simulink, Compiler, and several of the optional toolboxes are available to faculty, staff, and students. To see the kind and quantity of all MATLAB licenses plus the number that you are currently using you can use the matlab_licenses command:
$ module load matlab
$ matlab_licenses
The MATLAB client can be run in the front-end for application development, however, computationally intensive jobs must be run on compute nodes.
The following sections provide several examples illustrating how to submit MATLAB jobs to a Linux compute cluster.
Matlab Script (.m File)
This section illustrates how to submit a small, serial, MATLAB program as a job to a batch queue. This MATLAB program prints the name of the run host and gets three random numbers.
Prepare a MATLAB script myscript.m, and a MATLAB function file myfunction.m:
% FILENAME: myscript.m
% Display name of compute node which ran this job.
[c name] = system('hostname');
fprintf('\n\nhostname:%s\n', name);
% Display three random numbers.
A = rand(1,3);
fprintf('%f %f %f\n', A);
quit;
% FILENAME: myfunction.m
function result = myfunction ()
% Return name of compute node which ran this job.
[c name] = system('hostname');
result = sprintf('hostname:%s', name);
% Return three random numbers.
A = rand(1,3);
r = sprintf('%f %f %f', A);
result=strvcat(result,r);
end
Also, prepare a job submission file, here named myjob.sub. Run with the name of the script:
#!/bin/bash
# FILENAME: myjob.sub
echo "myjob.sub"
# Load module, and set up environment for Matlab to run
module load matlab
unset DISPLAY
# -nodisplay: run MATLAB in text mode; X11 server not needed
# -singleCompThread: turn off implicit parallelism
# -r: read MATLAB program; use MATLAB JIT Accelerator
# Run Matlab, with the above options and specifying our .m file
matlab -nodisplay -singleCompThread -r myscript
myjob.sub
< M A T L A B (R) >
Copyright 1984-2011 The MathWorks, Inc.
R2011b (7.13.0.564) 64-bit (glnxa64)
August 13, 2011
To get started, type one of these: helpwin, helpdesk, or demo.
For product information, visit www.mathworks.com.
hostname:hammer-a001.rcac.purdue.edu
0.814724 0.905792 0.126987
Output shows that a processor core on one compute node (hammer-a001) processed the job. Output also displays the three random numbers.
For more information about MATLAB:
Implicit Parallelism
MATLAB implements implicit parallelism which is automatic multithreading of many computations, such as matrix multiplication, linear algebra, and performing the same operation on a set of numbers. This is different from the explicit parallelism of the Parallel Computing Toolbox.
MATLAB offers implicit parallelism in the form of thread-parallel enabled functions. Since these processor cores, or threads, share a common memory, many MATLAB functions contain multithreading potential. Vector operations, the particular application or algorithm, and the amount of computation (array size) contribute to the determination of whether a function runs serially or with multithreading.
When your job triggers implicit parallelism, it attempts to allocate its threads on all processor cores of the compute node on which the MATLAB client is running, including processor cores running other jobs. This competition can degrade the performance of all jobs running on the node.
When you know that you are coding a serial job but are unsure whether you are using thread-parallel enabled operations, run MATLAB with implicit parallelism turned off. Beginning with the R2009b, you can turn multithreading off by starting MATLAB with -singleCompThread:
$ matlab -nodisplay -singleCompThread -r mymatlabprogram
When you are using implicit parallelism, make sure you request exclusive access to a compute node, as MATLAB has no facility for sharing nodes.
For more information about MATLAB's implicit parallelism:
Profile Manager
MATLAB offers two kinds of profiles for parallel execution: the 'local' profile and user-defined cluster profiles. The 'local' profile runs a MATLAB job on the processor core(s) of the same compute node, or front-end, that is running the client. To run a MATLAB job on compute node(s) different from the node running the client, you must define a Cluster Profile using the Cluster Profile Manager.
To prepare a user-defined cluster profile, use the Cluster Profile Manager in the Parallel menu. This profile contains the scheduler details (queue, nodes, processors, walltime, etc.) of your job submission. Ultimately, your cluster profile will be an argument to MATLAB functions like batch().
For your convenience, a generic cluster profile is provided that can be downloaded: myslurmprofile.settings
Please note that modifications are very likely to be required to make myslurmprofile.settings work. You may need to change values for number of nodes, number of workers, walltime, and submission queue specified in the file. As well, the generic profile itself depends on the particular job scheduler on the cluster, so you may need to download or create two or more generic profiles under different names. Each time you run a job using a Cluster Profile, make sure the specific profile you are using is appropriate for the job and the cluster.
To import the profile, start a MATLAB session and select Manage Cluster Profiles... from the Parallel menu. In the Cluster Profile Manager, select Import, navigate to the folder containing the profile, select myslurmprofile.settings and click OK. Remember that the profile will need to be customized for your specific needs. If you have any questions, please contact us.
For detailed information about MATLAB's Parallel Computing Toolbox, examples, demos, and tutorials:
Parallel Computing Toolbox (parfor)
The MATLAB Parallel Computing Toolbox (PCT) extends the MATLAB language with high-level, parallel-processing features such as parallel for loops, parallel regions, message passing, distributed arrays, and parallel numerical methods. It offers a shared-memory computing environment running on the local cluster profile in addition to your MATLAB client. Moreover, the MATLAB Distributed Computing Server (DCS) scales PCT applications up to the limit of your DCS licenses.
This section illustrates the fine-grained parallelism of a parallel for loop (parfor) in a pool job.
The following examples illustrate a method for submitting a small, parallel, MATLAB program with a parallel loop (parfor statement) as a job to a queue. This MATLAB program prints the name of the run host and shows the values of variables numlabs and labindex for each iteration of the parfor loop.
This method uses the job submission command to submit a MATLAB client which calls the MATLAB batch() function with a user-defined cluster profile.
Prepare a MATLAB pool program in a MATLAB script with an appropriate filename, here named myscript.m:
% FILENAME: myscript.m
% SERIAL REGION
[c name] = system('hostname');
fprintf('SERIAL REGION: hostname:%s\n', name)
numlabs = parpool('poolsize');
fprintf(' hostname numlabs labindex iteration\n')
fprintf(' ------------------------------- ------- -------- ---------\n')
tic;
% PARALLEL LOOP
parfor i = 1:8
[c name] = system('hostname');
name = name(1:length(name)-1);
fprintf('PARALLEL LOOP: %-31s %7d %8d %9d\n', name,numlabs,labindex,i)
pause(2);
end
% SERIAL REGION
elapsed_time = toc; % get elapsed time in parallel loop
fprintf('\n')
[c name] = system('hostname');
name = name(1:length(name)-1);
fprintf('SERIAL REGION: hostname:%s\n', name)
fprintf('Elapsed time in parallel loop: %f\n', elapsed_time)
The execution of a pool job starts with a worker executing the statements of the first serial region up to the parfor block, when it pauses. A set of workers (the pool) executes the parfor block. When they finish, the first worker resumes by executing the second serial region. The code displays the names of the compute nodes running the batch session and the worker pool.
Prepare a MATLAB script that calls MATLAB function batch() which makes a four-lab pool on which to run the MATLAB code in the file myscript.m. Use an appropriate filename, here named mylclbatch.m:
% FILENAME: mylclbatch.m
!echo "mylclbatch.m"
!hostname
pjob=batch('myscript','Profile','myslurmprofile','Pool',4,'CaptureDiary',true);
wait(pjob);
diary(pjob);
quit;
Prepare a job submission file with an appropriate filename, here named myjob.sub:
#!/bin/bash
# FILENAME: myjob.sub
echo "myjob.sub"
hostname
module load matlab
unset DISPLAY
matlab -nodisplay -r mylclbatch
Submit the job as a single compute node with one processor core.
One processor core runs myjob.sub and mylclbatch.m.
Once this job starts, a second job submission is made.
myjob.sub
< M A T L A B (R) >
Copyright 1984-2013 The MathWorks, Inc.
R2013a (8.1.0.604) 64-bit (glnxa64)
February 15, 2013
To get started, type one of these: helpwin, helpdesk, or demo.
For product information, visit www.mathworks.com.
mylclbatch.mhammer-a000.rcac.purdue.edu
SERIAL REGION: hostname:hammer-a000.rcac.purdue.edu
hostname numlabs labindex iteration
------------------------------- ------- -------- ---------
PARALLEL LOOP: hammer-a001.rcac.purdue.edu 4 1 2
PARALLEL LOOP: hammer-a002.rcac.purdue.edu 4 1 4
PARALLEL LOOP: hammer-a001.rcac.purdue.edu 4 1 5
PARALLEL LOOP: hammer-a002.rcac.purdue.edu 4 1 6
PARALLEL LOOP: hammer-a003.rcac.purdue.edu 4 1 1
PARALLEL LOOP: hammer-a003.rcac.purdue.edu 4 1 3
PARALLEL LOOP: hammer-a004.rcac.purdue.edu 4 1 7
PARALLEL LOOP: hammer-a004.rcac.purdue.edu 4 1 8
SERIAL REGION: hostname:hammer-a001.rcac.purdue.edu
Elapsed time in parallel loop: 5.411486
To scale up this method to handle a real application, increase the wall time in the submission command to accommodate a longer running job. Secondly, increase the wall time of myslurmprofile by using the Cluster Profile Manager in the Parallel menu to enter a new wall time in the property SubmitArguments.
For more information about MATLAB Parallel Computing Toolbox:
Parallel Toolbox (spmd)
The MATLAB Parallel Computing Toolbox (PCT) extends the MATLAB language with high-level, parallel-processing features such as parallel for loops, parallel regions, message passing, distributed arrays, and parallel numerical methods. It offers a shared-memory computing environment with a maximum of eight MATLAB workers (labs, threads; versions R2009a) and 12 workers (labs, threads; version R2011a) running on the local configuration in addition to your MATLAB client. Moreover, the MATLAB Distributed Computing Server (DCS) scales PCT applications up to the limit of your DCS licenses.
This section illustrates how to submit a small, parallel, MATLAB program with a parallel region (spmd statement) as a MATLAB pool job to a batch queue.
This example uses the submission command to submit to compute nodes a MATLAB client which interprets a Matlab .m with a user-defined cluster profile which scatters the MATLAB workers onto different compute nodes. This method uses the MATLAB interpreter, the Parallel Computing Toolbox, and the Distributed Computing Server; so, it requires and checks out six licenses: one MATLAB license for the client running on the compute node, one PCT license, and four DCS licenses. Four DCS licenses run the four copies of the spmd statement. This job is completely off the front end.
Prepare a MATLAB script called myscript.m:
% FILENAME: myscript.m
% SERIAL REGION
[c name] = system('hostname');
fprintf('SERIAL REGION: hostname:%s\n', name)
p = parpool('4');
fprintf(' hostname numlabs labindex\n')
fprintf(' ------------------------------- ------- --------\n')
tic;
% PARALLEL REGION
spmd
[c name] = system('hostname');
name = name(1:length(name)-1);
fprintf('PARALLEL REGION: %-31s %7d %8d\n', name,numlabs,labindex)
pause(2);
end
% SERIAL REGION
elapsed_time = toc; % get elapsed time in parallel region
delete(p);
fprintf('\n')
[c name] = system('hostname');
name = name(1:length(name)-1);
fprintf('SERIAL REGION: hostname:%s\n', name)
fprintf('Elapsed time in parallel region: %f\n', elapsed_time)
quit;
Prepare a job submission file with an appropriate filename, here named myjob.sub. Run with the name of the script:
#!/bin/bash
# FILENAME: myjob.sub
echo "myjob.sub"
module load matlab
unset DISPLAY
matlab -nodisplay -r myscript
Run MATLAB to set the default parallel configuration to your job configuration:
$ matlab -nodisplay
>> parallel.defaultClusterProfile('myslurmprofile');
>> quit;
$
Once this job starts, a second job submission is made.
myjob.sub
< M A T L A B (R) >
Copyright 1984-2011 The MathWorks, Inc.
R2011b (7.13.0.564) 64-bit (glnxa64)
August 13, 2011
To get started, type one of these: helpwin, helpdesk, or demo.
For product information, visit www.mathworks.com.
SERIAL REGION: hostname:hammer-a001.rcac.purdue.edu
Starting matlabpool using the 'myslurmprofile' profile ... connected to 4 labs.
hostname numlabs labindex
------------------------------- ------- --------
Lab 2:
PARALLEL REGION: hammer-a002.rcac.purdue.edu 4 2
Lab 1:
PARALLEL REGION: hammer-a001.rcac.purdue.edu 4 1
Lab 3:
PARALLEL REGION: hammer-a003.rcac.purdue.edu 4 3
Lab 4:
PARALLEL REGION: hammer-a004.rcac.purdue.edu 4 4
Sending a stop signal to all the labs ... stopped.
SERIAL REGION: hostname:hammer-a001.rcac.purdue.edu
Elapsed time in parallel region: 3.382151
Output shows the name of one compute node (a001) that processed the job submission file myjob.sub and the two serial regions. The job submission scattered four processor cores (four MATLAB labs) among four different compute nodes (a001,a002,a003,a004) that processed the four parallel regions. The total elapsed time demonstrates that the jobs ran in parallel.
For more information about MATLAB Parallel Computing Toolbox:
Distributed Computing Server (parallel job)
The MATLAB Parallel Computing Toolbox (PCT) enables a parallel job via the MATLAB Distributed Computing Server (DCS). The tasks of a parallel job are identical, run simultaneously on several MATLAB workers (labs), and communicate with each other. This section illustrates an MPI-like program.
This section illustrates how to submit a small, MATLAB parallel job with four workers running one MPI-like task to a batch queue. The MATLAB program broadcasts an integer to four workers and gathers the names of the compute nodes running the workers and the lab IDs of the workers.
This example uses the job submission command to submit a Matlab script with a user-defined cluster profile which scatters the MATLAB workers onto different compute nodes. This method uses the MATLAB interpreter, the Parallel Computing Toolbox, and the Distributed Computing Server; so, it requires and checks out six licenses: one MATLAB license for the client running on the compute node, one PCT license, and four DCS licenses. Four DCS licenses run the four copies of the parallel job. This job is completely off the front end.
Prepare a MATLAB script named myscript.m :
% FILENAME: myscript.m
% Specify pool size.
% Convert the parallel job to a pool job.
parpool('4');
spmd
if labindex == 1
% Lab (rank) #1 broadcasts an integer value to other labs (ranks).
N = labBroadcast(1,int64(1000));
else
% Each lab (rank) receives the broadcast value from lab (rank) #1.
N = labBroadcast(1);
end
% Form a string with host name, total number of labs, lab ID, and broadcast value.
[c name] =system('hostname');
name = name(1:length(name)-1);
fmt = num2str(floor(log10(numlabs))+1);
str = sprintf(['%s:%d:%' fmt 'd:%d '], name,numlabs,labindex,N);
% Apply global concatenate to all str's.
% Store the concatenation of str's in the first dimension (row) and on lab #1.
result = gcat(str,1,1);
if labindex == 1
disp(result)
end
end % spmd
matlabpool close force;
quit;
Also, prepare a job submission, here named myjob.sub. Run with the name of the script:
# FILENAME: myjob.sub
echo "myjob.sub"
module load matlab
unset DISPLAY
# -nodisplay: run MATLAB in text mode; X11 server not needed
# -r: read MATLAB program; use MATLAB JIT Accelerator
matlab -nodisplay -r myscript
Run MATLAB to set the default parallel configuration to your appropriate Profile:
$ matlab -nodisplay
>> defaultParallelConfig('myslurmprofile');
>> quit;
$
Submit the job as a single compute node with one processor core.
Once this job starts, a second job submission is made.
myjob.sub
< M A T L A B (R) >
Copyright 1984-2011 The MathWorks, Inc.
R2011b (7.13.0.564) 64-bit (glnxa64)
August 13, 2011
To get started, type one of these: helpwin, helpdesk, or demo.
For product information, visit www.mathworks.com.
>Starting matlabpool using the 'myslurmprofile' configuration ... connected to 4 labs.
Lab 1:
hammer-a006.rcac.purdue.edu:4:1:1000
hammer-a007.rcac.purdue.edu:4:2:1000
hammer-a008.rcac.purdue.edu:4:3:1000
hammer-a009.rcac.purdue.edu:4:4:1000
Sending a stop signal to all the labs ... stopped.
Did not find any pre-existing parallel jobs created by matlabpool.
Output shows the name of one compute node (a006) that processed the job submission file myjob.sub. The job submission scattered four processor cores (four MATLAB labs) among four different compute nodes (a006,a007,a008,a009) that processed the four parallel regions.
To scale up this method to handle a real application, increase the wall time in the submission command to accommodate a longer running job. Secondly, increase the wall time of myslurmprofile by using the Cluster Profile Manager in the Parallel menu to enter a new wall time in the property SubmitArguments.
For more information about parallel jobs:
Python
Notice: Python 2.7 has reached end-of-life on Jan 1, 2020 (announcement). Please update your codes and your job scripts to use Python 3.
Python is a high-level, general-purpose, interpreted, dynamic programming language. We suggest using Anaconda which is a Python distribution made for large-scale data processing, predictive analytics, and scientific computing. For example, to use the default Anaconda distribution:
$ module load conda
For a full list of available Anaconda and Python modules enter:
$ module spider conda
Example Python Jobs
This section illustrates how to submit a small Python job to a SLURM queue.
Link to section 'Example 1: Hello world' of 'Example Python Jobs' Example 1: Hello world
Prepare a Python input file with an appropriate filename, here named hello.py
:
# FILENAME: hello.py
import string, sys
print("Hello, world!")
Prepare a job submission file with an appropriate filename, here named myjob.sub:
#!/bin/bash
# FILENAME: myjob.sub
module load conda
python hello.py
Hello, world!
Link to section 'Example 2: Matrix multiply' of 'Example Python Jobs' Example 2: Matrix multiply
Save the following script as matrix.py:
# Matrix multiplication program
x = [[3,1,4],[1,5,9],[2,6,5]]
y = [[3,5,8,9],[7,9,3,2],[3,8,4,6]]
result = [[sum(a*b for a,b in zip(x_row,y_col)) for y_col in zip(*y)] for x_row in x]
for r in result:
print(r)
Change the last line in the job submission file above to read:
python matrix.py
The standard output file from this job will result in the following matrix:
[28, 56, 43, 53]
[65, 122, 59, 73]
[63, 104, 54, 60]
Link to section 'Example 3: Sine wave plot using numpy and matplotlib packages' of 'Example Python Jobs' Example 3: Sine wave plot using numpy and matplotlib packages
Save the following script as sine.py:
import numpy as np
import matplotlib
matplotlib.use('Agg')
import matplotlib.pyplot as plt
x = np.linspace(-np.pi, np.pi, 201)
plt.plot(x, np.sin(x))
plt.xlabel('Angle [rad]')
plt.ylabel('sin(x)')
plt.axis('tight')
plt.savefig('sine.png')
Change your job submission file to submit this script and the job will output a png file and blank standard output and error files.
For more information about Python:
Managing Environments with Conda
Conda is a package manager in Anaconda that allows you to create and manage multiple environments where you can pick and choose which packages you want to use. To use Conda you must load an Anaconda module:
$ module load conda
Many packages are pre-installed in the global environment. To see these packages:
$ conda list
To create your own custom environment:
$ conda create --name MyEnvName python=3.8 FirstPackageName SecondPackageName -y
The --name option specifies that the environment created will be named MyEnvName. You can include as many packages as you require separated by a space. Including the -y option lets you skip the prompt to install the package. By default environments are created and stored in the $HOME/.conda directory.
To create an environment at a custom location:
$ conda create --prefix=$HOME/MyEnvName python=3.8 PackageName -y
To see a list of your environments:
$ conda env list
To remove unwanted environments:
$ conda remove --name MyEnvName --all
To add packages to your environment:
$ conda install --name MyEnvName PackageNames
To remove a package from an environment:
$ conda remove --name MyEnvName PackageName
Installing packages when creating your environment, instead of one at a time, will help you avoid dependency issues.
To activate or deactivate an environment you have created:
$ source activate MyEnvName
$ source deactivate MyEnvName
If you created your conda environment at a custom location using --prefix option, then you can activate or deactivate it using the full path.
$ source activate $HOME/MyEnvName
$ source deactivate $HOME/MyEnvName
To use a custom environment inside a job you must load the module and activate the environment inside your job submission script. Add the following lines to your submission script:
$ module load conda
$ source activate MyEnvName
For more information about Python:
Managing Packages with Pip
Pip is a Python package manager. Many Python package documentation provide pip
instructions that result in permission errors because by default pip
will install in a system-wide location and fail.
Exception:
Traceback (most recent call last):
... ... stack trace ... ...
OSError: [Errno 13] Permission denied: '/apps/cent7/anaconda/2020.07-py38/lib/python3.8/site-packages/mkl_random-1.1.1.dist-info'
If you encounter this error, it means that you cannot modify the global Python installation. We recommend installing Python packages in a conda environment. Detailed instructions for installing packages with pip can be found in our Python package installation page.
Below we list some other useful pip commands.
- Search for a package in PyPI channels:
$ pip search packageName
- Check which packages are installed globally:
$ pip list
- Check which packages you have personally installed:
$ pip list --user
- Snapshot installed packages:
$ pip freeze > requirements.txt
- You can install packages from a snapshot inside a new conda environment. Make sure to load the appropriate conda environment first.
$ pip install -r requirements.txt
For more information about Python:
Installing Packages
Installing Python packages in an Anaconda environment is recommended. One key advantage of Anaconda is that it allows users to install unrelated packages in separate self-contained environments. Individual packages can later be reinstalled or updated without impacting others. If you are unfamiliar with Conda environments, please check our Conda Guide.
To facilitate the process of creating and using Conda environments, we support a script (conda-env-mod) that generates a module file for an environment, as well as an optional Jupyter kernel to use this environment in a JupyterHub notebook.
You must load one of the anaconda modules in order to use this script.
$ module load conda
Step-by-step instructions for installing custom Python packages are presented below.
Link to section 'Step 1: Create a conda environment' of 'Installing Packages' Step 1: Create a conda environment
Users can use the conda-env-mod script to create an empty conda environment. This script needs either a name or a path for the desired environment. After the environment is created, it generates a module file for using it in future. Please note that conda-env-mod is different from the official conda-env script and supports a limited set of subcommands. Detailed instructions for using conda-env-mod can be found with the command conda-env-mod --help.
-
Example 1: Create a conda environment named mypackages in user's
$HOME
directory.$ conda-env-mod create -n mypackages
-
Example 2: Create a conda environment named mypackages at a custom location.
$ conda-env-mod create -p /depot/mylab/apps/mypackages
Please follow the on-screen instructions while the environment is being created. After finishing, the script will print the instructions to use this environment.
... ... ... Preparing transaction: ...working... done Verifying transaction: ...working... done Executing transaction: ...working... done +------------------------------------------------------+ | To use this environment, load the following modules: | | module load use.own | | module load conda-env/mypackages-py3.8.5 | +------------------------------------------------------+ Your environment "mypackages" was created successfully.
Note down the module names, as you will need to load these modules every time you want to use this environment. You may also want to add the module load lines in your jobscript, if it depends on custom Python packages.
By default, module files are generated in your $HOME/privatemodules directory. The location of module files can be customized by specifying the -m /path/to/modules option to conda-env-mod.
Note: The main differences between -p and -m are: 1) -p will change the location of packages to be installed for the env and the module file will still be located at the $HOME/privatemodules directory as defined in use.own. 2) -m will only change the location of the module file. So the method to load modules created with -m and -p are different, see Example 3 for details.
- Example 3: Create a conda environment named labpackages in your group's Data Depot space and place the module file at a shared location for the group to use.
$ conda-env-mod create -p /depot/mylab/apps/labpackages -m /depot/mylab/etc/modules ... ... ... Preparing transaction: ...working... done Verifying transaction: ...working... done Executing transaction: ...working... done +-------------------------------------------------------+ | To use this environment, load the following modules: | | module use /depot/mylab/etc/modules | | module load conda-env/labpackages-py3.8.5 | +-------------------------------------------------------+ Your environment "labpackages" was created successfully.
If you used a custom module file location, you need to run the module use command as printed by the command output above.
By default, only the environment and a module file are created (no Jupyter kernel). If you plan to use your environment in a JupyterHub notebook, you need to append a --jupyter flag to the above commands.
- Example 4: Create a Jupyter-enabled conda environment named labpackages in your group's Data Depot space and place the module file at a shared location for the group to use.
$ conda-env-mod create -p /depot/mylab/apps/labpackages -m /depot/mylab/etc/modules --jupyter ... ... ... Jupyter kernel created: "Python (My labpackages Kernel)" ... ... ... Your environment "labpackages" was created successfully.
Link to section 'Step 2: Load the conda environment' of 'Installing Packages' Step 2: Load the conda environment
-
The following instructions assume that you have used conda-env-mod script to create an environment named mypackages (Examples 1 or 2 above). If you used conda create instead, please use conda activate mypackages.
$ module load use.own $ module load conda-env/mypackages-py3.8.5
Note that the conda-env module name includes the Python version that it supports (Python 3.8.5 in this example). This is same as the Python version in the conda module.
-
If you used a custom module file location (Example 3 above), please use module use to load the conda-env module.
$ module use /depot/mylab/etc/modules $ module load conda-env/labpackages-py3.8.5
Link to section 'Step 3: Install packages' of 'Installing Packages' Step 3: Install packages
Now you can install custom packages in the environment using either conda install or pip install.
Link to section 'Installing with conda' of 'Installing Packages' Installing with conda
-
Example 1: Install OpenCV (open-source computer vision library) using conda.
$ conda install opencv
-
Example 2: Install a specific version of OpenCV using conda.
$ conda install opencv=4.5.5
-
Example 3: Install OpenCV from a specific anaconda channel.
$ conda install -c anaconda opencv
Link to section 'Installing with pip' of 'Installing Packages' Installing with pip
-
Example 4: Install pandas using pip.
$ pip install pandas
-
Example 5: Install a specific version of pandas using pip.
$ pip install pandas==1.4.3
Follow the on-screen instructions while the packages are being installed. If installation is successful, please proceed to the next section to test the packages.
Note: Do NOT run Pip with the --user argument, as that will install packages in a different location and might mess up your account environment.
Link to section 'Step 4: Test the installed packages' of 'Installing Packages' Step 4: Test the installed packages
To use the installed Python packages, you must load the module for your conda environment. If you have not loaded the conda-env module, please do so following the instructions at the end of Step 1.
$ module load use.own
$ module load conda-env/mypackages-py3.8.5
- Example 1: Test that OpenCV is available.
$ python -c "import cv2; print(cv2.__version__)"
- Example 2: Test that pandas is available.
$ python -c "import pandas; print(pandas.__version__)"
If the commands finished without errors, then the installed packages can be used in your program.
Link to section 'Additional capabilities of conda-env-mod script' of 'Installing Packages' Additional capabilities of conda-env-mod script
The conda-env-mod tool is intended to facilitate creation of a minimal Anaconda environment, matching module file and optionally a Jupyter kernel. Once created, the environment can then be accessed via familiar module load command, tuned and expanded as necessary. Additionally, the script provides several auxiliary functions to help manage environments, module files and Jupyter kernels.
General usage for the tool adheres to the following pattern:
$ conda-env-mod help
$ conda-env-mod <subcommand> <required argument> [optional arguments]
where required arguments are one of
- -n|--name ENV_NAME (name of the environment)
- -p|--prefix ENV_PATH (location of the environment)
and optional arguments further modify behavior for specific actions (e.g. -m to specify alternative location for generated module files).
Given a required name or prefix for an environment, the conda-env-mod script supports the following subcommands:
- create - to create a new environment, its corresponding module file and optional Jupyter kernel.
- delete - to delete existing environment along with its module file and Jupyter kernel.
- module - to generate just the module file for a given existing environment.
- kernel - to generate just the Jupyter kernel for a given existing environment (note that the environment has to be created with a --jupyter option).
- help - to display script usage help.
Using these subcommands, you can iteratively fine-tune your environments, module files and Jupyter kernels, as well as delete and re-create them with ease. Below we cover several commonly occurring scenarios.
Note: When you try to use conda-env-mod delete, remember to include the arguments as you create the environment (i.e. -p package_location and/or -m module_location).
Link to section 'Generating module file for an existing environment' of 'Installing Packages' Generating module file for an existing environment
If you already have an existing configured Anaconda environment and want to generate a module file for it, follow appropriate examples from Step 1 above, but use the module subcommand instead of the create one. E.g.
$ conda-env-mod module -n mypackages
and follow printed instructions on how to load this module. With an optional --jupyter flag, a Jupyter kernel will also be generated.
Note that the module name mypackages should be exactly the same with the older conda environment name. Note also that if you intend to proceed with a Jupyter kernel generation (via the --jupyter flag or a kernel subcommand later), you will have to ensure that your environment has ipython and ipykernel packages installed into it. To avoid this and other related complications, we highly recommend making a fresh environment using a suitable conda-env-mod create .... --jupyter command instead.
Link to section 'Generating Jupyter kernel for an existing environment' of 'Installing Packages' Generating Jupyter kernel for an existing environment
If you already have an existing configured Anaconda environment and want to generate a Jupyter kernel file for it, you can use the kernel subcommand. E.g.
$ conda-env-mod kernel -n mypackages
This will add a "Python (My mypackages Kernel)" item to the dropdown list of available kernels upon your next login to the JupyterHub.
Note that generated Jupiter kernels are always personal (i.e. each user has to make their own, even for shared environments). Note also that you (or the creator of the shared environment) will have to ensure that your environment has ipython and ipykernel packages installed into it.
Link to section 'Managing and using shared Python environments' of 'Installing Packages' Managing and using shared Python environments
Here is a suggested workflow for a common group-shared Anaconda environment with Jupyter capabilities:
The PI or lab software manager:
-
Creates the environment and module file (once):
$ module purge $ module load conda $ conda-env-mod create -p /depot/mylab/apps/labpackages -m /depot/mylab/etc/modules --jupyter
-
Installs required Python packages into the environment (as many times as needed):
$ module use /depot/mylab/etc/modules $ module load conda-env/labpackages-py3.8.5 $ conda install ....... # all the necessary packages
Lab members:
-
Lab members can start using the environment in their command line scripts or batch jobs simply by loading the corresponding module:
$ module use /depot/mylab/etc/modules $ module load conda-env/labpackages-py3.8.5 $ python my_data_processing_script.py .....
-
To use the environment in Jupyter notebooks, each lab member will need to create his/her own Jupyter kernel (once). This is because Jupyter kernels are private to individuals, even for shared environments.
$ module use /depot/mylab/etc/modules $ module load conda-env/labpackages-py3.8.5 $ conda-env-mod kernel -p /depot/mylab/apps/labpackages
A similar process can be devised for instructor-provided or individually-managed class software, etc.
Link to section 'Troubleshooting' of 'Installing Packages' Troubleshooting
- Python packages often fail to install or run due to dependency incompatibility with other packages. More specifically, if you previously installed packages in your home directory it is safer to clean those installations.
$ mv ~/.local ~/.local.bak $ mv ~/.cache ~/.cache.bak
- Unload all the modules.
$ module purge
- Clean up PYTHONPATH.
$ unset PYTHONPATH
- Next load the modules (e.g. anaconda) that you need.
$ module load conda/2024.02-py311 $ module load use.own $ module load conda-env/2024.02-py311
- Now try running your code again.
- Few applications only run on specific versions of Python (e.g. Python 3.6). Please check the documentation of your application if that is the case.
Installing Packages from Source
We maintain several Anaconda installations. Anaconda maintains numerous popular scientific Python libraries in a single installation. If you need a Python library not included with normal Python we recommend first checking Anaconda. For a list of modules currently installed in the Anaconda Python distribution:
$ module load conda
$ conda list
# packages in environment at /apps/spack/bell/apps/anaconda/2020.02-py37-gcc-4.8.5-u747gsx:
#
# Name Version Build Channel
_ipyw_jlab_nb_ext_conf 0.1.0 py37_0
_libgcc_mutex 0.1 main
alabaster 0.7.12 py37_0
anaconda 2020.02 py37_0
...
If you see the library in the list, you can simply import it into your Python code after loading the Anaconda module.
If you do not find the package you need, you should be able to install the library in your own Anaconda customization. First try to install it with Conda or Pip. If the package is not available from either Conda or Pip, you may be able to install it from source.
Use the following instructions as a guideline for installing packages from source. Make sure you have a download link to the software (usually it will be a tar.gz
archive file). You will substitute it on the wget line below.
We also assume that you have already created an empty conda environment as described in our Python package installation guide.
$ mkdir ~/src
$ cd ~/src
$ wget http://path/to/source/tarball/app-1.0.tar.gz
$ tar xzvf app-1.0.tar.gz
$ cd app-1.0
$ module load conda
$ module load use.own
$ module load conda-env/mypackages-py3.8.5
$ python setup.py install
$ cd ~
$ python
>>> import app
>>> quit()
The "import app" line should return without any output if installed successfully. You can then import the package in your python scripts.
If you need further help or run into any issues installing a library, contact us or drop by Coffee Hour for in-person help.
For more information about Python:
Example: Create and Use Biopython Environment with Conda
Link to section 'Using conda to create an environment that uses the biopython package' of 'Example: Create and Use Biopython Environment with Conda' Using conda to create an environment that uses the biopython package
To use Conda you must first load the anaconda module:
module load conda
Create an empty conda environment to install biopython:
conda-env-mod create -n biopython
Now activate the biopython environment:
module load use.own
module load conda-env/biopython-py3.12.5
Install the biopython packages in your environment:
conda install --channel anaconda biopython -y
Fetching package metadata ..........
Solving package specifications .........
.......
Linking packages ...
[ COMPLETE ]|################################################################
The --channel option specifies that it searches the anaconda channel for the biopython package. The -y argument is optional and allows you to skip the installation prompt. A list of packages will be displayed as they are installed.
Remember to add the following lines to your job submission script to use the custom environment in your jobs:
module load conda
module load use.own
module load conda-env/biopython-py3.12.5
If you need further help or run into any issues with creating environments, contact us or drop by Coffee Hour for in-person help.
For more information about Python:
Numpy Parallel Behavior
The widely available Numpy package is the best way to handle numerical computation in Python. The numpy
package provided by our anaconda
modules is optimized using Intel's MKL library. It will automatically parallelize many operations to make use of all the cores available on a machine.
In many contexts that would be the ideal behavior. On the cluster however that very likely is not in fact the preferred behavior because often more than one user is present on the system and/or more than one job on a node. Having multiple processes contend for those resources will actually result in lesser performance.
Setting the MKL_NUM_THREADS
or OMP_NUM_THREADS
environment variable(s) allows you to control this behavior. Our anaconda modules automatically set these variables to 1 if and only if you do not currently have that variable defined.
When submitting batch jobs it is always a good idea to be explicit rather than implicit. If you are submitting a job that you want to make use of the full resources available on the node, set one or both of these variables to the number of cores you want to allow numpy to make use of.
#!/bin/bash
module load conda
export MKL_NUM_THREADS=20
...
If you are submitting multiple jobs that you intend to be scheduled together on the same node, it is probably best to restrict numpy to a single core.
#!/bin/bash
module load conda
export MKL_NUM_THREADS=1
R
R, a GNU project, is a language and environment for data manipulation, statistics, and graphics. It is an open source version of the S programming language. R is quickly becoming the language of choice for data science due to the ease with which it can produce high quality plots and data visualizations. It is a versatile platform with a large, growing community and collection of packages.
For more general information on R visit The R Project for Statistical Computing.
Loading Data into R
R is an environment for manipulating data. In order to manipulate data, it must be brought into the R environment. R has a function to read any file that data is stored in. Some of the most common file types like comma-separated variable(CSV) files have functions that come in the basic R packages. Other less common file types require additional packages to be installed. To read data from a CSV file into the R environment, enter the following command in the R prompt:
> read.csv(file = "path/to/data.csv", header = TRUE)
When R reads the file it creates an object that can then become the target of other functions. By default the read.csv() function will give the object the name of the .csv file. To assign a different name to the object created by read.csv enter the following in the R prompt:
> my_variable <- read.csv(file = "path/to/data.csv", header = FALSE)
To display the properties (structure) of loaded data, enter the following:
> str(my_variable)
For more functions and tutorials:
Running R jobs
This section illustrates how to submit a small R job to a SLURM queue. The example job computes a Pythagorean triple.
Prepare an R input file with an appropriate filename, here named myjob.R:
# FILENAME: myjob.R
# Compute a Pythagorean triple.
a = 3
b = 4
c = sqrt(a*a + b*b)
c # display result
Prepare a job submission file with an appropriate filename, here named myjob.sub:
#!/bin/bash
# FILENAME: myjob.sub
module load r
# --vanilla:
# --no-save: do not save datasets at the end of an R session
R --vanilla --no-save < myjob.R
For other examples or R jobs:
Installing R packages
Link to section 'Challenges of Managing R Packages in the Cluster Environment' of 'Installing R packages' Challenges of Managing R Packages in the Cluster Environment
- Different clusters have different hardware and softwares. So, if you have access to multiple clusters, you must install your R packages separately for each cluster.
- Each cluster has multiple versions of R and packages installed with one version of R may not work with another version of R. So, libraries for each R version must be installed in a separate directory.
- You can define the directory where your R packages will be installed using the environment variable
R_LIBS_USER
. - For your convenience, a sample ~/.Rprofile example file is provided that can be downloaded to your cluster account and renamed into
~/.Rprofile
(or appended to one) to customize your installation preferences. Detailed instructions.
Link to section 'Installing Packages' of 'Installing R packages' Installing Packages
-
Step 0: Set up installation preferences.
Follow the steps for setting up your~/.Rprofile
preferences. This step needs to be done only once. If you have created a~/.Rprofile
file previously on Hammer, ignore this step. -
Step 1: Check if the package is already installed.
As part of the R installations on community clusters, a lot of R libraries are pre-installed. You can check if your package is already installed by opening an R terminal and entering the commandinstalled.packages()
. For example,module load r/4.4.1 R
installed.packages()["units",c("Package","Version")] Package Version "units" "0.8-1" quit()
If the package you are trying to use is already installed, simply load the library, e.g.,
library('units')
. Otherwise, move to the next step to install the package. -
Step 2: Load required dependencies. (if needed)
For simple packages you may not need this step. However, some R packages depend on other libraries. For example, thesf
package depends ongdal
andgeos
libraries. So, you will need to load the corresponding modules before installingsf
. Read the documentation for the package to identify which modules should be loaded.module load gdal module load geos
-
Step 3: Install the package.
Now install the desired package using the commandinstall.packages('package_name')
. R will automatically download the package and all its dependencies from CRAN and install each one. Your terminal will show the build progress and eventually show whether the package was installed successfully or not.R
install.packages('sf', repos="https://cran.case.edu/") Installing package into ‘/home/myusername/R/x86_64-pc-linux-gnu-library/4.4.1’ (as ‘lib’ is unspecified) trying URL 'https://cran.case.edu/src/contrib/sf_0.9-7.tar.gz' Content type 'application/x-gzip' length 4203095 bytes (4.0 MB) ================================================== downloaded 4.0 MB ... ... more progress messages ... ... ** testing if installed package can be loaded from final location ** testing if installed package keeps a record of temporary installation path * DONE (sf) The downloaded source packages are in ‘/tmp/RtmpSVAGio/downloaded_packages’
- Step 4: Troubleshooting. (if needed)
If Step 3 ended with an error, you need to investigate why the build failed. Most common reason for build failure is not loading the necessary modules.
Link to section 'Loading Libraries' of 'Installing R packages' Loading Libraries
Once you have packages installed you can load them with the library()
function as shown below:
library('packagename')
The package is now installed and loaded and ready to be used in R.
Link to section 'Example: Installing dplyr' of 'Installing R packages' Example: Installing dplyr
The following demonstrates installing the dplyr
package assuming the above-mentioned custom ~/.Rprofile
is in place (note its effect in the "Installing package into" information message):
module load r
R
install.packages('dplyr', repos="http://ftp.ussg.iu.edu/CRAN/")
Installing package into ‘/home/myusername/R/hammer/4.4.1’
(as ‘lib’ is unspecified)
...
also installing the dependencies 'crayon', 'utf8', 'bindr', 'cli', 'pillar', 'assertthat', 'bindrcpp', 'glue', 'pkgconfig', 'rlang', 'Rcpp', 'tibble', 'BH', 'plogr'
...
...
...
The downloaded source packages are in
'/tmp/RtmpHMzm9z/downloaded_packages'
library(dplyr)
Attaching package: 'dplyr'
For more information about installing R packages:
RStudio
RStudio is a graphical integrated development environment (IDE) for R. RStudio is the most popular environment for developing both R scripts and packages. RStudio is provided on most Research systems.
There are two methods to launch RStudio on the cluster: command-line and application menu icon.
Link to section 'Launch RStudio by the command-line:' of 'RStudio' Launch RStudio by the command-line:
module load gcc
module load r
module load rstudio
rstudio
Note that RStudio is a graphical program and in order to run it you must have a local X11 server running or use Thinlinc Remote Desktop environment. See the ssh X11 forwarding section for more details.
Link to section 'Launch Rstudio by the application menu icon:' of 'RStudio' Launch Rstudio by the application menu icon:
- Log into desktop.hammer.rcac.purdue.edu with web browser or ThinLinc client
- Click on the
Applications
drop down menu on the top left corner - Choose
Cluster Software
and thenRStudio
R and RStudio are free to download and run on your local machine. For more information about RStudio:
Link to section 'RStudio Server on Hammer' of 'Running RStudio Server on Hammer' RStudio Server on Hammer
A different version of RStudio is also installed on Hammer. RStudio Server allows you to run RStudio through your web browser.
Link to section 'Projects' of 'Running RStudio Server on Hammer' Projects
One benefit of RStudio is that your work can be separated into projects. You can give each project a working directory, workspace, history and source documents. When you are creating a new project, you can start it in a new empty directory, one with code and data already present or by cloning a repository.
RStudio Server allows easy collaboration and sharing of R projects. Just click on the project drop down menu in the top right corner and add the career account user names of those you wish to share with.
Link to section 'Sessions' of 'Running RStudio Server on Hammer' Sessions
Another feature is the ability to run multiple sessions at once. You can do multiple instances of the same project in parallel or work on different projects simultaneously. The sessions dropdown menu is in the upper right corner right above the project menu. Here you can kill or open sessions. Note that closing a window does not end a session, so please kill sessions when you are not using them.
You can view an overview of all your projects and active sessions by clicking on the blue RStudio Server Home logo in the top left corner of the window next to the file menu.
Link to section 'Packages' of 'Running RStudio Server on Hammer' Packages
You can install new packages with the install.packages() function in the console. You can also graphically select any packages you have previously installed on any cluster. Simply select packages from the tabs on the bottom right side of the window and select the package you wish to load.
For more information about RStudio:
Setting Up R Preferences with .Rprofile
For your convenience, a sample ~/.Rprofile example file is provided that can be downloaded to your cluster account and renamed into ~/.Rprofile
(or appended to one). Follow these steps to download our recommended ~/.Rprofile
example and copy it into place:
curl -#LO https://www.rcac.purdue.edu/files/knowledge/run/examples/apps/r/Rprofile_example
mv -ib Rprofile_example ~/.Rprofile
The above installation step needs to be done only once on Hammer. Now load the R module and run R:
module load r/4.4.1
R
.libPaths()
[1] "/home/myusername/R/hammer/4.1.2-gcc-6.3.0-ymdumss"
[2] "/apps/spack/hammer/apps/r/4.1.2-gcc-6.3.0-ymdumss/rlib/R/library"
.libPaths()
should output something similar to above if it is set up correctly.
You are now ready to install R packages into the dedicated directory /home/myusername/R/hammer/4.1.2-gcc-6.3.0-ymdumss
.
Spark
Apache Spark is an open-source data analytics cluster computing framework.
Hadoop
Spark is not tied to the two-stage MapReduce paradigm, and promises performance up to 100 times faster than Hadoop MapReduce for certain applications. Spark provides primitives for in-memory cluster computing that allows user programs to load data into a cluster's memory and query it repeatedly, making it well suited to machine learning algorithms.
Before to submit a Spark application to a YARN cluster, export environment variables:
$ source /etc/default/hadoop
To submit a Spark application to a YARN cluster:
$ cd /apps/hathi/spark
$ ./bin/spark-submit --master yarn --deploy-mode cluster examples/src/main/python/pi.py 100
Please note that there are two ways to specify the master: yarn-cluster and yarn-client. In cluster mode, your driver program will run on the worker nodes; while in client mode, your driver program will run within the spark-submit process which runs on the hathi front end. We recommand that you always use the cluster mode on hathi to avoid overloading the front end nodes.
To write your own spark jobs, use the Spark Pi as a baseline to start.
Spark can work with input files from both HDFS and local file system. The default after exporting the environment variables is from HDFS. To use input files that are on the cluster storage (e.g., data depot), specify: file:///path/to/file.
Note: when reading input files from cluster storage, the files must be accessible from any node in the cluster.
To run an interactive analysis or to learn the API with Spark Shell:
$ cd /apps/hathi/spark
$ ./bin/pyspark
Create a Resilient Distributed Dataset (RDD) from Hadoop InputFormats (such as HDFS files):
>>> textFile = sc.textFile("derby.log")
15/09/22 09:31:58 INFO storage.MemoryStore: ensureFreeSpace(67728) called with curMem=122343, maxMem=278302556
15/09/22 09:31:58 INFO storage.MemoryStore: Block broadcast_1 stored as values in memory (estimated size 66.1 KB, free 265.2 MB)
15/09/22 09:31:58 INFO storage.MemoryStore: ensureFreeSpace(14729) called with curMem=190071, maxMem=278302556
15/09/22 09:31:58 INFO storage.MemoryStore: Block broadcast_1_piece0 stored as bytes in memory (estimated size 14.4 KB, free 265.2 MB)
15/09/22 09:31:58 INFO storage.BlockManagerInfo: Added broadcast_1_piece0 in memory on localhost:57813 (size: 14.4 KB, free: 265.4 MB)
15/09/22 09:31:58 INFO spark.SparkContext: Created broadcast 1 from textFile at NativeMethodAccessorImpl.java:-2
Note: derby.log is a file on hdfs://hathi-adm.rcac.purdue.edu:8020/user/myusername/derby.log
Call the count() action on the RDD:
>>> textFile.count()
15/09/22 09:32:01 INFO mapred.FileInputFormat: Total input paths to process : 1
15/09/22 09:32:01 INFO spark.SparkContext: Starting job: count at :1
15/09/22 09:32:01 INFO scheduler.DAGScheduler: Got job 0 (count at :1) with 2 output partitions (allowLocal=false)
15/09/22 09:32:01 INFO scheduler.DAGScheduler: Final stage: ResultStage 0(count at :1)
......
15/09/22 09:32:03 INFO executor.Executor: Finished task 1.0 in stage 0.0 (TID 1). 1870 bytes result sent to driver
15/09/22 09:32:04 INFO scheduler.TaskSetManager: Finished task 0.0 in stage 0.0 (TID 0) in 2254 ms on localhost (1/2)
15/09/22 09:32:04 INFO scheduler.TaskSetManager: Finished task 1.0 in stage 0.0 (TID 1) in 2220 ms on localhost (2/2)
15/09/22 09:32:04 INFO scheduler.TaskSchedulerImpl: Removed TaskSet 0.0, whose tasks have all completed, from pool
15/09/22 09:32:04 INFO scheduler.DAGScheduler: ResultStage 0 (count at :1) finished in 2.317 s
15/09/22 09:32:04 INFO scheduler.DAGScheduler: Job 0 finished: count at :1, took 2.548350 s
93
To learn programming in Spark, refer to Spark Programming Guide
To learn submitting Spark applications, refer to Submitting Applications
PBS
This section walks through how to submit and run a Spark job using PBS on the compute nodes of Hammer.
pbs-spark-submit launches an Apache Spark program within a PBS job, including starting the Spark master and worker processes in standalone mode, running a user supplied Spark job, and stopping the Spark master and worker processes. The Spark program and its associated services will be constrained by the resource limits of the job and will be killed off when the job ends. This effectively allows PBS to act as a Spark cluster manager.
The following steps assume that you have a Spark program that can run without errors.
To use Spark and pbs-spark-submit, you need to load the following two modules to setup SPARK_HOME and PBS_SPARK_HOME environment variables.
module load spark
module load pbs-spark-submit
The following example submission script serves as a template to build your customized, more complex Spark job submission. This job requests 2 whole compute nodes for 10 minutes, and submits to the default queue.
#PBS -N spark-pi
#PBS -l nodes=2:ppn=20
#PBS -l walltime=00:10:00
#PBS -q standby
#PBS -o spark-pi.out
#PBS -e spark-pi.err
cd $PBS_O_WORKDIR
module load spark
module load pbs-spark-submit
pbs-spark-submit $SPARK_HOME/examples/src/main/python/pi.py 1000
In the submission script above, this command submits the pi.py program to the nodes that are allocated to your job.
pbs-spark-submit $SPARK_HOME/examples/src/main/python/pi.py 1000
You can set various environment variables in your submission script to change the setting of Spark program. For example, the following line sets the SPARK_LOG_DIR to $HOME/log. The default value is current working directory.
export SPARK_LOG_DIR=$HOME/log
The same environment variables can be set via the pbs-spark-submit command line argument. For example, the following line sets the SPARK_LOG_DIR to $HOME/log2.
pbs-spark-submit --log-dir $HOME/log2
Environment Variable | Default | Shell Export | Command Line Args |
---|---|---|---|
SPAKR_CONF_DIR | $SPARK_HOME/conf | export SPARK_CONF_DIR=$HOME/conf | --conf-dir |
SPAKR_LOG_DIR | Current Working Directory | export SPARK_LOG_DIR=$HOME/log | --log-dir |
SPAKR_LOCAL_DIR | /tmp | export SPARK_LOCAL_DIR=$RCAC_SCRATCH/local | NA |
SCRATCHDIR | Current Working Directory | export SCRATCHDIR=$RCAC_SCRATCH/scratch | --work-dir |
SPARK_MASTER_PORT | 7077 | export SPARK_MASTER_PORT=7078 | NA |
SPARK_DAEMON_JAVA_OPTS | None | export SPARK_DAEMON_JAVA_OPTS="-Dkey=value" | -D key=value |
Note that SCRATCHDIR must be a shared scratch directory across all nodes of a job.
In addition, pbs-spark-submit supports command line arguments to change the properties of the Spark daemons and the Spark jobs. For example, the --no-stop argument tells Spark to not stop the master and worker daemons after the Spark application is finished, and the --no-init argument tells Spark to not initialize the Spark master and worker processes. This is intended for use in a sequence of invocations of Spark programs within the same job.
pbs-spark-submit --no-stop $SPARK_HOME/examples/src/main/python/pi.py 800
pbs-spark-submit --no-init $SPARK_HOME/examples/src/main/python/pi.py 1000
Use the following command to see the complete list of command line arguments.
pbs-spark-submit -h
To learn programming in Spark, refer to Spark Programming Guide
To learn submitting Spark applications, refer to Submitting Applications
Singularity
Note: Singularity was originally a project out of Lawrence Berkeley National Laboratory. It has now been spun off into a distinct offering under a new corporate entity under the name Sylabs Inc. This guide pertains to the open source community edition, SingularityCE.
Link to section 'What is Singularity?' of 'Singularity' What is Singularity?
Singularity is a new feature of the Community Clusters allowing the portability and reproducibility of operating system and application environments through the use of Linux containers. It gives users complete control over their environment.
Singularity is like Docker but tuned explicitly for HPC clusters. More information is available from the project’s website.
Link to section 'Features' of 'Singularity' Features
- Run the latest applications on an Ubuntu or Centos userland
- Gain access to the latest developer tools
- Launch MPI programs easily
- Much more
Singularity’s user guide is available at: sylabs.io/guides/3.8/user-guide
Link to section 'Example' of 'Singularity' Example
Here is an example using an Ubuntu 16.04 image on Hammer:
singularity exec /depot/itap/singularity/ubuntu1604.img cat /etc/lsb-release
DISTRIB_ID=Ubuntu
DISTRIB_RELEASE=16.04
DISTRIB_CODENAME=xenial
DISTRIB_DESCRIPTION="Ubuntu 16.04 LTS"
Here is another example using a Centos 7 image:
singularity exec /depot/itap/singularity/centos7.img cat /etc/redhat-release
CentOS Linux release 7.2.1511 (Core)
Link to section 'Purdue Cluster Specific Notes' of 'Singularity' Purdue Cluster Specific Notes
All service providers will integrate Singularity slightly differently depending on site. The largest customization will be which default files are inserted into your images so that routine services will work.
Services we configure for your images include DNS settings and account information. File systems we overlay into your images are your home directory, scratch, Data Depot, and application file systems.
Here is a list of paths:
- /etc/resolv.conf
- /etc/hosts
- /home/$USER
- /apps
- /scratch
- /depot
This means that within the container environment these paths will be present and the same as outside the container. The /apps
, /scratch
, and /depot
directories will need to exist inside your container to work properly.
Link to section 'Creating Singularity Images' of 'Singularity' Creating Singularity Images
Due to how singularity containers work, you must have root privileges to build an image. Once you have a singularity container image built on your own system, you can copy the image file up to the cluster (you do not need root privileges to run the container).
You can find information and documentation for how to install and use singularity on your system:
We have version 3.8.0-1.el7
on the cluster. You will most likely not be able to run any container built with any singularity past that version. So be sure to follow the installation guide for version 3.8 on your system.
singularity --version
singularity version 3.8.0-1.el7
Everything you need on how to build a container is available from their user-guide. Below are merely some quick tips for getting your own containers built for Hammer.
You can use a Definition File to both build your container and share its specification with collaborators (for the sake of reproducibility). Here is a simplistic example of such a file:
# FILENAME: Buildfile
Bootstrap: docker
From: ubuntu:18.04
%post
apt-get update && apt-get upgrade -y
mkdir /apps /depot /scratch
To build the image itself:
sudo singularity build ubuntu-18.04.sif Buildfile
The challenge with this approach however is that it must start from scratch if you decide to change something. In order to create a container image iteratively and interactively, you can use the --sandbox
option.
sudo singularity build --sandbox ubuntu-18.04 docker://ubuntu:18.04
This will not create a flat image file but a directory tree (i.e., a folder), the contents of which are the container's filesystem. In order to get a shell inside the container that allows you to modify it, user the --writable
option.
sudo singularity shell --writable ubuntu-18.04
Singularity: Invoking an interactive shell within container...
Singularity ubuntu-18.04.sandbox:~>
You can then proceed to install any libraries, software, etc. within the container. Then to create the final image file, exit
the shell and call the build
command once more on the sandbox.
sudo singularity build ubuntu-18.04.sif ubuntu-18.04
Finally, copy the new image to Hammer and run it.
Windows
Windows virtual machines (VMs) are supported as batch jobs on HPC systems. This section illustrates how to submit a job and run a Windows instance in order to run Windows applications on the high-performance computing systems.
The following images are pre-configured and made available by staff:
- Windows 2016 Server Basic (minimal software pre-loaded)
- Windows 2016 Server GIS (GIS Software Stack pre-loaded)
The Windows VMs can be launched in two fashions:
- Menu Launcher - Point and click to start
- Command Line - Advanced and customized usage
Click each of the above links for detailed instructions on using them.
Link to section 'Software Provided in Pre-configured Virtual Machines' of 'Windows' Software Provided in Pre-configured Virtual Machines
The Windows 2016 Base server image available on Hammer has the following software packages preloaded:
- Anaconda Python 2 and Python 3
- JMP 13
- Matlab R2017b
- Microsoft Office 2016
- Notepad++
- NVivo 12
- Rstudio
- Stata SE 15
- VLC Media Player
Command line
If you wish to work with Windows VMs on the command line or work into scripted workflows you can interact directly with the Windows system:
Copy a Windows 2016 Server VM image to your storage. Scratch or Research Data Depot are good locations to save a VM image. If you are using scratch, remember that scratch spaces are temporary, and be sure to safely back up your disk image somewhere permanent, such as Research Data Depot or Fortress. To copy a basic image:
$ cp /apps/external/apps/windows/images/latest.qcow2 $RCAC_SCRATCH/windows.qcow2
To copy a GIS image:
$ cp /depot/itap/windows/gis/2k16.qcow2 $RCAC_SCRATCH/windows.qcow2
To launch a virtual machine in a batch job, use the "windows" script, specifying the path to your Windows virtual machine image. With no other command-line arguments, the windows script will autodetect a number cores and memory for the Windows VM. A Windows network connection will be made to your home directory. To launch:
$ windows -i $RCAC_SCRATCH/windows.qcow2
Link to section 'Command line options:' of 'Command line' Command line options:
-i <path to qcow image file> (For example, $RCAC_SCRATCH/windows-2k16.qcow2)
-m <RAM>G (For example, 32G)
-c <cores> (For example, 20)
-s <smbpath> (UNIX Path to map as a drive, for example, $RCAC_SCRATCH)
-b (If present, launches VM in background. Use VNC to connect to Windows.)
To launch a virtual machine with 32GB of RAM, 20 cores, and a network mapping to your home directory:
$ windows -i /path/to/image.qcow2 -m 32G -c 20 -s $HOME
To launch a virtual machine with 16GB of RAM, 10 cores, and a network mapping to your Data Depot space:
$ windows -i /path/to/image.qcow2 -m 16G -c 10 -s /depot/mylab
The Windows 2016 server desktop will open, and automatically log in as an administrator, so that you can install any software into the Windows virtual machine that your research requires. Changes to the image will be stored in the file specified with the -i option.
Menu Launcher
Windows VMs can be easily launched through the login/thinlinc">Thinlinc remote desktop environment.
- Log in via login/thinlinc">Thinlinc.
- Click on Applications menu in the upper left corner.
- Look under the Cluster Software menu.
- The "Windows 10" launcher will launch a VM directly on the front-end.
- Follow the dialogs to set up your VM.
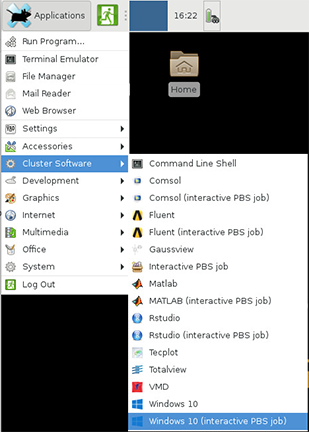
The dialog menus will walk you through setting up and loading your VM.
- You can choose to create a new image or load a saved image.
- New VMs should be saved on Scratch or Research Data Depot as they are too large for Home Directories.
- If you are using scratch, remember that scratch spaces are temporary, and be sure to safely back up your disk image somewhere permanent, such as Research Data Depot or Fortress.
You will also be prompted to select a storage space to mount on your image (Home, Scratch, or Data Depot). You can only choose one to be mounted. It will appear on a shortcut on the desktop once the VM loads.
Link to section 'Notes' of 'Menu Launcher' Notes
Using the menu launcher will launch automatically select reasonable CPU and memory values. If you wish to choose other options or work Windows VMs into scripted workflows see the section on using the command line.
Mathematica
Mathematica implements numeric and symbolic mathematics. This section illustrates how to submit a small Mathematica job to a PBS queue. This Mathematica example finds the three roots of a third-degree polynomial.
Prepare a Mathematica input file with an appropriate filename, here named myjob.in:
(* FILENAME: myjob.in *)
(* Find roots of a polynomial. *)
p=x^3+3*x^2+3*x+1
Solve[p==0]
Quit
Prepare a job submission file with an appropriate filename, here named myjob.sub:
#!/bin/sh -l # FILENAME: myjob.sub module load mathematica cd $PBS_O_WORKDIR math < myjob.in
Submit the job:
$ qsub -l nodes=1:ppn=20 myjob.sub
View job status:
$ qstat -u myusername
View results in the file for all standard output, here named myjob.sub.omyjobid:
Mathematica 5.2 for Linux x86 (64 bit)
Copyright 1988-2005 Wolfram Research, Inc.
-- Terminal graphics initialized --
In[1]:=
In[2]:=
In[2]:=
In[3]:=
2 3
Out[3]= 1 + 3 x + 3 x + x
In[4]:=
Out[4]= {{x -> -1}, {x -> -1}, {x -> -1}}
In[5]:=
View the standard error file, myjob.sub.emyjobid:
rmdir: ./ligo/rengel/tasks: Directory not empty
rmdir: ./ligo/rengel: Directory not empty
rmdir: ./ligo: Directory not empty
For more information about Mathematica:
Octave
GNU Octave is a high-level, interpreted, programming language for numerical computations. Octave is a structured language (similar to C) and mostly compatible with MATLAB. You may use Octave to avoid the need for a MATLAB license, both during development and as a deployed application. By doing so, you may be able to run your application on more systems or more easily distribute it to others.
This section illustrates how to submit a small Octave job to a PBS queue. This Octave example computes the inverse of a matrix.
Prepare an Octave script file with an appropriate filename, here named myjob.m:
% FILENAME: myjob.m
% Invert matrix A.
A = [1 2 3; 4 5 6; 7 8 0]
inv(A)
quit
Prepare a job submission file with an appropriate filename, here named myjob.sub:
#!/bin/sh -l
# FILENAME: myjob.sub
module load octave
cd $PBS_O_WORKDIR
unset DISPLAY
# Use the -q option to suppress startup messages.
# octave -q < myjob.m
octave < myjob.m
The command octave myjob.m (without the redirection) also works in the preceding script.
Submit the job:
$ qsub -l nodes=1:ppn=20 myjob.sub
View job status:
$ qstat -u myusername
View results in the file for all standard output, myjob.sub.omyjobid:
A =
1 2 3
4 5 6
7 8 0
ans =
-1.77778 0.88889 -0.11111
1.55556 -0.77778 0.22222
-0.11111 0.22222 -0.11111
Any output written to standard error will appear in myjob.sub.emyjobid.
For more information about Octave:
Using Jupyter Hub
Link to section 'What is Jupyter Hub' of 'Using Jupyter Hub' What is Jupyter Hub
Jupyter is an acronym meaning Julia, Python and R. The application was originally developed for use with these languages but now supports many more. Jupyter stores your project in a notebook. It is called a notebook because it is not just a block of code but rather a collection of information that relate to a project. The way you organize your notebook can explain processes and steps taken as well as highlight results. Notebooks provide a variety of formatting options while downloading so you can share the project appropriately for the situation. In addition, Jupyter can compile and run code, as well as save its output, making it an ideal workspace for many types of projects.
Jupyter Hub is currently available here or under the url https://notebook.hammer.rcac.purdue.edu.
Link to section 'Getting Started' of 'Using Jupyter Hub' Getting Started
When you are logging to Jupyter Hub on one of the clusters you need to use your career account credentials. After, you will see the contents of your home directory in a file explorer. To start a new notebook click the "New" dropdown menu at the right-top and select one of the kernels available. Bash, R or Python.
Link to section 'Create your own environment' of 'Using Jupyter Hub' Create your own environment
You can create your own environment in a kernel using a conda environment. Whatever environment you have created using conda can become in a Kernel ready to use in Jupyter Hub, just following some steps in the terminal or from the conda tab in the Jupyter Hub dashboard.
Below are listed the steps needed to create the environment for Jupyter from the terminal.
-
Load the anaconda module or use your own local installation.
$ module load anaconda/5.1.0-py36
-
Create your own Conda environment with the following packages.
$ conda create -n MyEnvName ipython ipykernel [...more-needed-packages...]
(and if you need a specific Python version in your environment, you can also add a
python=x.y
specification to the above command). -
Activate your environment.
$ source activate MyEnvName
-
Install the new Kernel.
$ ipython kernel install --user --name MyEnvName --display-name "Python (My Own MyEnvName Kernel)"
The
--name
value is used by Jupyter internally. These commands will overwrite any existing kernel with the same name.--display-name
is what you see in the notebook menus. - Go to your Jupyter dashboard and reload the page, you will see your own Kernel when you create a new Notebook. If you want to change the Kernel in the current Notebook, just go to the Kernel tab and select it from the "Change Kernel" option.
If you want to create the environment from the Dashboard, just go to the conda tab and create a new one with one of the available kernels, it will take some minutes while all base packages are being installed, after the new environment shows up in the list you can just select the libraries you want from the box under the list.
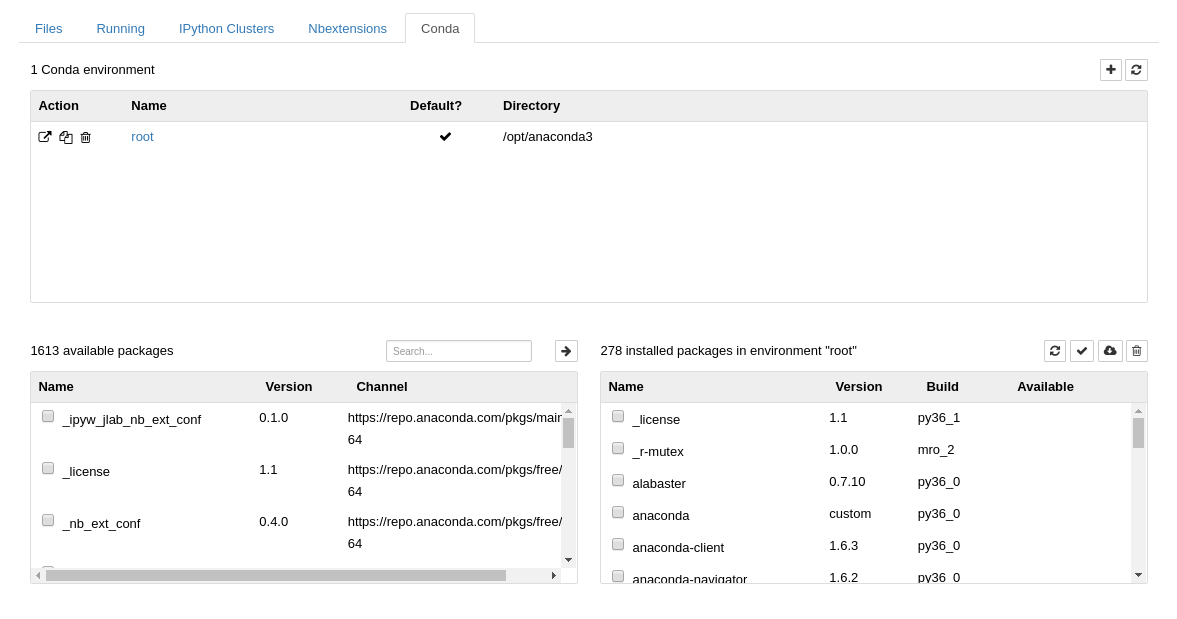
Additionally, You can change the environment you are using at any time by clicking the "Kernel" dropdown menu and selecting "Change kernel".
If you want to install a new kernel different from Python (e.g. R or Bash), please refer to the links at the end.
To run code in a cell, select the cell and click the "run cell" icon on the toolbar.
To add descriptions or other plain text change the cell to markdown format. Any standard markdown tags will apply after you click the "run cell" tool.
Below is a simple example of a notebook created following the steps outlined above.
For more information about Jupyter Hub, kernels and example notebooks:
Frequently Asked Questions
Some common questions, errors, and problems are categorized below. Click the Expand Topics link in the upper right to see all entries at once. You can also use the search box above to search the user guide for any issues you are seeing.
About Hammer
Frequently asked questions about Hammer.
Can you remove me from the Hammer mailing list?
Your subscription in the Hammer mailing list is tied to your account on Hammer. If you are no longer using your account on Hammer, your account can be deleted from the My Accounts page. Hover over the resource you wish to remove yourself from and click the red 'X' button. Your account and mailing list subscription will be removed overnight. Be sure to make a copy of any data you wish to keep first.
How is Hammer different than other Community Clusters?
- Hammer is optimized for loosely-coupled, high-throughput computation. The scheduler is configured to favor starting jobs quickly and ensure maximum utilization.
- The maximum job size is 8 processor cores. If you require resources with a greater degree of parallelism, please consider an alternate community cluster system optimized for high-performance, parallel computing.
- Jobs are scheduled on a whole-node basis and will not share nodes with other jobs by default. You may submit jobs that use less than one node, however, you will be allocated a whole node from your queue unless node sharing is enabled. Node sharing is enabled by adding ‑l naccesspolicy=singleuser to your job's requirements.
Do I need to do anything to my firewall to access Hammer?
No firewall changes are needed to access Hammer. However, to access data through Network Drives (i.e., CIFS, "Z: Drive"), you must be on a Purdue campus network or connected through VPN.
Logging In & Accounts
Frequently asked questions about logging in & accounts.
Errors
Common errors and solutions/work-arounds for them.
/usr/bin/xauth: error in locking authority file
Link to section 'Problem' of '/usr/bin/xauth: error in locking authority file' Problem
I receive this message when logging in:
/usr/bin/xauth: error in locking authority file
Link to section 'Solution' of '/usr/bin/xauth: error in locking authority file' Solution
Your home directory disk quota is full. You may check your quota with myquota
.
You will need to free up space in your home directory.
ncdu
command is a convenient interactive tool to examine disk usage. Consider running ncdu $HOME
to analyze where the bulk of the usage is. With this knowledge, you could then archive your data elsewhere (e.g. your research group's Data Depot space, or Fortress tape archive), or delete files you no longer need.
There are several common locations that tend to grow large over time and are merely cached downloads. The following are safe to delete if you see them in the output of ncdu $HOME
:
/home/myusername/.local/share/Trash
/home/myusername/.cache/pip
/home/myusername/.conda/pkgs
/home/myusername/.singularity/cache
My SSH connection hangs
Link to section 'Problem' of 'My SSH connection hangs' Problem
Your console hangs while trying to connect to a RCAC Server.
Link to section 'Solution' of 'My SSH connection hangs' Solution
This can happen due to various reasons. Most common reasons for hanging SSH terminals are:
- Network: If you are connected over wifi, make sure that your Internet connection is fine.
- Busy front-end server: When you connect to a cluster, you SSH to one of the front-end login nodes. Due to transient user loads, one or more of the front-ends may become unresponsive for a short while. To avoid this, try reconnecting to the cluster or wait until the login node you have connected to has reduced load.
- File system issue: If a server has issues with one or more of the file systems (
home
,scratch
, ordepot
) it may freeze your terminal. To avoid this you can connect to another front-end.
If neither of the suggestions above work, please contact support specifying the name of the server where your console is hung.
Thinlinc session frozen
Link to section 'Problem' of 'Thinlinc session frozen' Problem
Your Thinlinc session is frozen and you can not launch any commands or close the session.
Link to section 'Solution' of 'Thinlinc session frozen' Solution
This can happen due to various reasons. The most common reason is that you ran something memory-intensive inside that Thinlinc session on a front-end, so parts of the Thinlinc session got killed by Cgroups, and the entire session got stuck.
- If you are using a web-version Thinlinc remote desktop (inside the browser):
The web version does not have the capability to kill the existing session, only the standalone client does. Please install the standalone client and follow the steps below:
- If you are using a Thinlinc client:
Close the ThinLinc client, reopen the client login popup, and select
End existing session
.Select "End existing session" and try "Connect" again.
Thinlinc session unreachable
Link to section 'Problem' of 'Thinlinc session unreachable' Problem
When trying to login to Thinlinc and re-connect to your existing session, you receive an error "Your Thinlinc session is currently unreachable".
Link to section 'Solution' of 'Thinlinc session unreachable' Solution
This can happen if the specific login node your existing remote desktop session was residing on is currently offline or down, so Thinlinc can not reconnect to your existing session. Most often the session is non-recoverable at this point, so the solution is to terminate your existing Thinlinc desktop session and start a new one.
- If you are using a web-version Thinlinc remote desktop (inside the browser):
The web version does not have the capability to kill the existing session, only the standalone client does. Please install the standalone client and follow the steps below:
- If you are using a Thinlinc client:
Close the ThinLinc client, reopen the client login popup, and select
End existing session
.Select "End existing session" and try "Connect" again.
Questions
Frequently asked questions about logging in & accounts.
I worked on Hammer after I graduated/left Purdue, but can not access it anymore
Link to section 'Problem' of 'I worked on Hammer after I graduated/left Purdue, but can not access it anymore' Problem
You have graduated or left Purdue but continue collaboration with your Purdue colleagues. You find that your access to Purdue resources has suddenly stopped and your password is no longer accepted.
Link to section 'Solution' of 'I worked on Hammer after I graduated/left Purdue, but can not access it anymore' Solution
Access to all resources depends on having a valid Purdue Career Account. Expired Career Accounts are removed twice a year, during Spring and October breaks (more details at the official page). If your Career Account was purged due to expiration, you will not be be able to access the resources.
To provide remote collaborators with valid Purdue credentials, the University provides a special procedure called Request for Privileges (R4P). If you need to continue your collaboration with your Purdue PI, the PI will have to submit or renew an R4P request on your behalf.
After your R4P is completed and Career Account is restored, please note two additional necessary steps:
-
Access: Restored Career Accounts by default do not have any RCAC resources enabled for them. Your PI will have to login to the Manage Users tool and explicitly re-enable your access by un-checking and then ticking back checkboxes for desired queues/Unix groups resources.
-
Email: Restored Career Accounts by default do not have their @purdue.edu email service enabled. While this does not preclude you from using RCAC resources, any email messages (be that generated on the clusters, or any service announcements) would not be delivered - which may cause inconvenience or loss of compute jobs. To avoid this, we recommend setting your restored @purdue.edu email service to "Forward" (to an actual address you read). The easiest way to ensure it is to go through the Account Setup process.
Jobs
Frequently asked questions related to running jobs.
Errors
Common errors and potential solutions/workarounds for them.
cannot connect to X server / cannot open display
Link to section 'Problem' of 'cannot connect to X server / cannot open display' Problem
You receive the following message after entering a command to bring up a graphical window
cannot connect to X server
cannot open display
Link to section 'Solution' of 'cannot connect to X server / cannot open display' Solution
This can happen due to multiple reasons:
- Reason: Your SSH client software does not support graphical display by itself (e.g. SecureCRT or PuTTY).
- Solution: Try using a client software like Thinlinc or MobaXterm as described in the SSH X11 Forwarding guide.
-
Reason: You did not enable X11 forwarding in your SSH connection.
-
Solution: If you are in a Windows environment, make sure that X11 forwarding is enabled in your connection settings (e.g. in MobaXterm or PuTTY). If you are in a Linux environment, try
ssh -Y -l username hostname
-
- Reason: If you are trying to open a graphical window within an interactive PBS job, make sure you are using the
-X
option withqsub
after following the previous step(s) for connecting to the front-end. Please see the example in the Interactive Jobs guide. - Reason: If none of the above apply, make sure that you are within quota of your home directory.
bash: command not found
Link to section 'Problem' of 'bash: command not found' Problem
You receive the following message after typing a command
bash: command not found
Link to section 'Solution' of 'bash: command not found' Solution
This means the system doesn't know how to find your command. Typically, you need to load a module to do it.
qdel: Server could not connect to MOM 12345.hammer-adm.rcac.purdue.edu
Link to section 'Problem' of 'qdel: Server could not connect to MOM 12345.hammer-adm.rcac.purdue.edu' Problem
You receive the following message after attempting to delete a job with the qdel
command
qdel: Server could not connect to MOM 12345.hammer-adm.rcac.purdue.edu
Link to section 'Solution' of 'qdel: Server could not connect to MOM 12345.hammer-adm.rcac.purdue.edu' Solution
This error usually indicates that at least one node running your job has stopped responding or crashed. Please forward the job ID to support, and staff can help remove the job from the queue.
bash: module command not found
Link to section 'Problem' of 'bash: module command not found' Problem
You receive the following message after typing a command, e.g. module load intel
bash: module command not found
Link to section 'Solution' of 'bash: module command not found' Solution
The system cannot find the module command. You need to source the modules.sh file as below
source /etc/profile.d/modules.sh
or
#!/bin/bash -i
1234.hammer-adm.rcac.purdue.edu.SC: line 12: 12345 Killed
Link to section 'Problem' of '1234.hammer-adm.rcac.purdue.edu.SC: line 12: 12345 Killed' Problem
Your PBS job stopped running and you received an email with the following:
/var/spool/torque/mom_priv/jobs/1234.hammer-adm.rcac.purdue.edu.SC: line 12: 12345 Killed <command name>
Link to section 'Solution' of '1234.hammer-adm.rcac.purdue.edu.SC: line 12: 12345 Killed' Solution
This means that the node your job was running on ran out of memory to support your program or code. This may be due to your job or other jobs sharing your node(s) consuming more memory in total than is available on the node. Your program was killed by the node to preserve the operating system. There are two possible causes:
- You requested your job share node(s) with other jobs. You should request all cores of the node or request exclusive access. Either your job or one of the other jobs running on the node consumed too much memory. Requesting exclusive access will give you full control over all the memory on the node.
- Your job requires more memory than is available on the node. You should use more nodes if your job supports MPI or run a smaller dataset.
Questions
Frequently asked questions about jobs.
How do I check my job output while it is running?
Link to section 'Problem' of 'How do I check my job output while it is running?' Problem
After submitting your job to the cluster, you want to see the output that it generates.
Link to section 'Solution' of 'How do I check my job output while it is running?' Solution
There are two simple ways to do this:
- qpeek: Use the tool qpeek to check the job's output. Syntax of the command is:
qpeek <jobid>
- Redirect your output to a file: To do this you need to edit the main command in your jobscript as shown below. Please note the redirection command starting with the greater than (>) sign.
myapplication ...other arguments... > "${PBS_JOBID}.output"
tail "<jobid>.output"
What is the "debug" queue?
The debug queue allows you to quickly start small, short, interactive jobs in order to debug code, test programs, or test configurations. You are limited to one running job at a time in the queue, and you may run up to two compute nodes for 30 minutes.
How can I get email alerts about my PBS job status?
Link to section 'Question' of 'How can I get email alerts about my PBS job status?' Question
How can I be notified when my PBS job was executed and if it completed successfully?
Link to section 'Answer' of 'How can I get email alerts about my PBS job status?' Answer
Submit your job with the following command line arguments
qsub -M email_address -m bea myjobsubmissionfile
Or, include the following in your job submission file.
#PBS -M email_address
#PBS -m bae
The -m option can have the following letters; "a", "b", and "e":
a - mail is sent when the job is aborted by the batch system.
b - mail is sent when the job begins execution.
e - mail is sent when the job terminates.
Can I extend the walltime on a job?
In some circumstances, yes. Walltime extensions must be requested of and completed by staff. Walltime extension requests will be considered on named (your advisor or research lab) queues. Standby or debug queue jobs cannot be extended.
Extension requests are at the discretion of staff based on factors such as any upcoming maintenance or resource availability. Extensions can be made past the normal maximum walltime on named queues but these jobs are subject to early termination should a conflicting maintenance downtime be scheduled.
Please be mindful of time remaining on your job when making requests and make requests at least 24 hours before the end of your job AND during business hours. We cannot guarantee jobs will be extended in time with less than 24 hours notice, after-hours, during weekends, or on a holiday.
We ask that you make accurate walltime requests during job submissions. Accurate walltimes will allow the job scheduler to efficiently and quickly schedule jobs on the cluster. Please consider that extensions can impact scheduling efficiency for all users of the cluster.
Requests can be made by contacting support. We ask that you:
- Provide numerical job IDs, cluster name, and your desired extension amount.
- Provide at least 24 hours notice before job will end (more if request is made on a weekend or holiday).
- Consider making requests during business hours. We may not be able to respond in time to requests made after-hours, on a weekend, or on a holiday.
How do I know Non-uniform Memory Access (NUMA) layout on Hammer?
- You can learn about processor layout on Hammer nodes using the following command:
hammer-a003:~$ lstopo-no-graphics
- For detailed IO connectivity:
hammer-a003:~$ lstopo-no-graphics --physical --whole-io
- Please note that NUMA information is useful for advanced MPI/OpenMP/GPU optimizations. For most users, using default NUMA settings in MPI or OpenMP would give you the best performance.
Why cannot I use --mem=0 when submitting jobs?
Link to section 'Question' of 'Why cannot I use --mem=0 when submitting jobs?' Question
Why can't I specify --mem=0
for my job?
Link to section 'Answer' of 'Why cannot I use --mem=0 when submitting jobs?' Answer
We no longer support requesting unlimited memory (--mem=0
) as it has an adverse effect on the way scheduler allocates job, and could lead to large amount of nodes being blocked from usage.
Most often we suggest relying on default memory allocation (cluster-specific). But if you have to request custom amounts of memory, you can do it explicitly. For example --mem=20G
.
If you want to use the entire node's memory, you can submit the job with the --exclusive
option.
Data
Frequently asked questions about data and data management.
My scratch files were purged. Can I retrieve them?
Unfortunately, once files are purged, they are purged permanently and cannot be retrieved. Notices of pending purges are sent one week in advance to your Purdue email address. Be sure to regularly check your Purdue email or set up forwarding to an account you do frequently check.
Link to section 'Can you tell me what files were purged?' of 'My scratch files were purged. Can I retrieve them?' Can you tell me what files were purged?
You can see a list of files removed with the command lastpurge
. The command accepts a -n
option to specify how many weeks/purges ago you want to look back at.
How is my Data Secured on Hammer?
Hammer is operated in line with policies, standards, and best practices as described within Secure Purdue, and specific to RCAC Resources.
Security controls for Hammer are based on ones defined in NIST cybersecurity standards.
Hammer supports research at the L1 fundamental and L2 sensitive levels. Hammer is not approved for storing data at the L3 restricted (covered by HIPAA) or L4 Export Controlled (ITAR), or any Controlled Unclassified Information (CUI).
For resources designed to support research with heightened security requirements, please look for resources within the REED+ Ecosystem.
Link to section 'For additional information' of 'How is my Data Secured on Hammer?' For additional information
Log in with your Purdue Career Account.
Can I share data with outside collaborators?
Yes! Globus allows convenient sharing of data with outside collaborators. Data can be shared with collaborators' personal computers or directly with many other computing resources at other institutions. See the Globus documentation on how to share data:
Can I access Fortress from Hammer?
Yes. While Fortress directories are not directly mounted on Hammer for performance and archival protection reasons, they can be accessed from Hammer front-ends and nodes using any of the recommended methods of HSI, HTAR or Globus.
Software
Frequently asked questions about software.
Cannot use pip after loading ml-toolkit modules
Link to section 'Question' of 'Cannot use pip after loading ml-toolkit modules' Question
Pip throws an error after loading the machine learning modules. How can I fix it?
Link to section 'Answer' of 'Cannot use pip after loading ml-toolkit modules' Answer
Machine learning modules (tensorflow, pytorch, opencv etc.) include a version of pip that is newer than the one installed with Anaconda. As a result it will throw an error when you try to use it.
$ pip --version
Traceback (most recent call last):
File "/apps/cent7/anaconda/5.1.0-py36/bin/pip", line 7, in <module>
from pip import main
ImportError: cannot import name 'main'
The preferred way to use pip with the machine learning modules is to invoke it via Python as shown below.
$ python -m pip --version
How can I get access to Sentaurus software?
Link to section 'Question' of 'How can I get access to Sentaurus software?' Question
How can I get access to Sentaurus tools for micro- and nano-electronics design?
Link to section 'Answer' of 'How can I get access to Sentaurus software?' Answer
Sentaurus software license requires a signed NDA. Please contact Dr. Mark Johnson, Director of ECE Instructional Laboratories to complete the process.
Once the licensing process is complete and you have been added into a cae2
Unix group, you could use Sentaurus on RCAC community clusters by loading the corresponding environment module:
module load sentaurus
About Research Computing
Frequently asked questions about RCAC.
Can I get a private server from RCAC?
Link to section 'Question' of 'Can I get a private server from RCAC?' Question
Can I get a private (virtual or physical) server from RCAC?
Link to section 'Answer' of 'Can I get a private server from RCAC?' Answer
Often, researchers may want a private server to run databases, web servers, or other software. RCAC currently has Geddes, a Community Composable Platform optimized for composable, cloud-like workflows that are complementary to the batch applications run on Community Clusters. Funded by the National Science Foundation under grant OAC-2018926, Geddes consists of Dell Compute nodes with two 64-core AMD Epyc 'Rome' processors (128 cores per node).
To purchase access to Geddes today, go to the Cluster Access Purchase page. Please subscribe to our Community Cluster Program Mailing List to stay informed on the latest purchasing developments or contact us (rcac-cluster-purchase@lists.purdue.edu) if you have any questions.
Negishi User Guide
Negishi is a Community Cluster optimized for communities running traditional, tightly-coupled science and engineering applications.
Link to section 'Overview of Negishi' of 'Overview of Negishi' Overview of Negishi
Negishi is a Community Cluster optimized for communities running traditional, tightly-coupled science and engineering applications. Negishi is being built through a partnership with Dell and AMD over the summer of 2022. Negishi consists of Dell compute nodes with two 64-core AMD Epyc "Milan" processors (128 cores per node) and 256 GB of memory. All nodes have 100 Gbps HDR Infiniband interconnect and a 6-year warranty.
New with Negishi is that access is being offered on the basis of each 64-core Rome processor, or a half-node share. To purchase access to Negishi today, go to the Cluster Access Purchase page. Please subscribe to our Community Cluster Program Mailing List to stay informed on the latest purchasing developments or contact us via email at rcac-cluster-purchase@lists.purdue.edu if you have any questions.
Link to section 'Negishi Interactive' of 'Overview of Negishi' Negishi Interactive
The interactive tier on our Negishi cluster provides entry-level access to high performance computing. This includes login to the system, data storage on our high-performance scratch filesystem, and a small allocation that allows jobs submitted to an "interactive" account limited to a few cores. This subscription is useful for getting workloads off your personal machine, integrated with more robust research computing and data systems, and a platform for smaller workloads. Transitioning to a larger allocation with priority scheduling is easy and simple.
Link to section 'Negishi Namesake' of 'Overview of Negishi' Negishi Namesake
Negishi is named in honor of Dr. Ei-ichi Negishi, the Herbert C. Brown Distinguished Professor in the Department of Chemistry at Purdue. More information about his life and impact on Purdue is available in a Biography of Negishi.
Link to section 'Negishi Specifications' of 'Overview of Negishi' Negishi Specifications
All Negishi compute nodes have 128 processor cores, 256 GB memory and 100 Gbps HDR100 Infiniband interconnects.
Front-Ends | Number of Nodes | Processors per Node | Cores per Node | Memory per Node | Retires in |
---|---|---|---|---|---|
8 | Two AMD EPYC 7763 64-Core Processors @ 2.2GHz | 128 | 512 GB | 2028 |
Sub-Cluster | Number of Nodes | Processors per Node | Cores per Node | Memory per Node | Retires in |
---|---|---|---|---|---|
A | 450 | Two AMD Epyc 7763 “Milan” CPUs @ 2.2GHz | 128 | 256 GB | 2028 |
B | 6 | Two AMD Epyc 7763 “Milan” CPUs @ 2.2GHz | 128 | 1 TB | 2028 |
C | 16 | Two AMD Epyc 7763 “Milan” CPUs @ 2.2GHz | 128 | 512 GB | 2028 |
G | 5 | Two AMD Epyc 7313 “Milan” CPUs @ 3.0GHz, Three AMD MI210 GPUs (64GB) |
32 | 512 GB | 2028 |
Negishi nodes run Rocky Linux 8 and use Slurm (Simple Linux Utility for Resource Management) as the batch scheduler for resource and job management. The application of operating system patches occurs as security needs dictate. All nodes allow for unlimited stack usage, as well as unlimited core dump size (though disk space and server quotas may still be a limiting factor).
On Negishi, the following set of compiler and message-passing libraries for parallel code are recommended:
- GCC 12.2.0
- OpenMPI or MVAPICH2
Link to section 'Software catalog' of 'Overview of Negishi' Software catalog
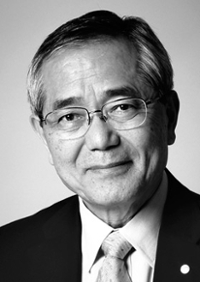
Link to section 'Ei-ichi Negishi' of 'Biography of Ei-ichi Negishi' Ei-ichi Negishi
Ei-ichi Negishi (1935-2021) was the Herbert C. Brown Distinguished Professor in the Department of Chemistry at Purdue. He came to Purdue in 1966 as a postdoctoral researcher in the lab of the Late Herbert C. Brown, and published 33 papers with Prof. Brown up through the time that Prof. Brown was awarded the Nobel Prize in Chemistry in 1979. With the award of the Nobel to Ei-ichi Negishi in 2010, Purdue has the rare distinction of a pair of Nobel Prize awards in two closely related areas. Professor Negishi’s Nobel Prize was awarded in recognition of his work on palladium-catalyzed cross-coupling chemistry (known world-– wide as the Negishi coupling). That work was described by the Nobel Foundation as "great art in a test tube". This is certainly appropriate as great scientists regard themselves as artists and explorers. The impact of that work was widespread, as it had been used in synthetic organic chemistry research worldwide, as well as in the commercial production of an array of pharmaceuticals and molecules used in the electronics industry. In recognition of and consistent with this idea, Ei-ichi and co-recipient Akira Suzuki were recently awarded Japan's highest cultural award, the "Order of Culture", bestowed in Nov. 2010 by the Emperor.
Professor Negishi was a prolific researcher, with ~400 publications on an array of problems in synthetic organic chemistry, leading to numerous awards. To name just a few, the list includes the Chemical Society of Japan Award (1997), the American Chemical Society Award in Organometallic Chemistry (1998), the McCoy Award (1998), the Sigma Xi Award at Purdue (2003), the Nobel Prize in Chemistry (2010), the Order of Culture in Japan (2010), the American Chemical Society Award for Creative Work in Synthetic Organic Chemistry (2010), the Indiana Sagamore of the Wabash (2011) and the Purdue Order of the Griffin (2011). He was elected to the American Academy of Arts and Sciences in 2011. Professor Negishi was leading the Negishi-Brown Institute, which had continued his work on catalytic organic synthesis. Dr. Negishi was passionate about the prospects for catalytic approaches to the reduction of carbon dioxide to enable large scale production of useful products from this environmental waste product. It is very fitting that Purdue bestow an honorary doctorate degree on Professor Negishi, whose accomplishments and contributions will have a permanent impact on Purdue’s stature and global recognition.
Link to section 'Accounts on Negishi' of 'Accounts' Accounts on Negishi
Link to section 'Obtaining an Account' of 'Accounts' Obtaining an Account
To obtain an account, you must be part of a research group which has purchased access to Negishi. Refer to the Accounts / Access page for more details on how to request access.
Link to section 'Outside Collaborators' of 'Accounts' Outside Collaborators
A valid Purdue Career Account is required for access to any resource. If you do not currently have a valid Purdue Career Account you must have a current Purdue faculty or staff member file a Request for Privileges (R4P) before you can proceed.
Logging In
To submit jobs on Negishi, log in to the submission host negishi.rcac.purdue.edu
via SSH. This submission host is actually 8 front-end hosts: login00.negishi
through login07.negishi
. The login process randomly assigns one of these front-ends to each login to negishi.rcac.purdue.edu
.
Passwords
Negishi supports either Purdue two-factor authentication (Purdue Login) or SSH keys.
Purdue Login
Link to section 'SSH' of 'Purdue Login' SSH
- SSH to the cluster as usual.
- When asked for a password, type your password followed by "
,push
". - Your Purdue Duo client will receive a notification to approve the login.
Link to section 'Thinlinc' of 'Purdue Login' Thinlinc
- When asked for a password, type your password followed by "
,push
". - Your Purdue Duo client will receive a notification to approve the login.
- The native Thinlinc client will prompt for Duo approval twice due to the way Thinlinc works.
- The native Thinlinc client also supports key-based authentication.
SSH Client Software
Secure Shell or SSH is a way of establishing a secure connection between two computers. It uses public-key cryptography to authenticate the user with the remote computer and to establish a secure connection. Its usual function involves logging in to a remote machine and executing commands. There are many SSH clients available for all operating systems:
Linux / Solaris / AIX / HP-UX / Unix:
- The
ssh
command is pre-installed. Log in usingssh myusername@negishi.rcac.purdue.edu
from a terminal.
Microsoft Windows:
- MobaXterm is a small, easy to use, full-featured SSH client. It includes X11 support for remote displays, SFTP capabilities, and limited SSH authentication forwarding for keys.
Mac OS X:
- The
ssh
command is pre-installed. You may start a local terminal window from "Applications->Utilities". Log in by typing the commandssh myusername@negishi.rcac.purdue.edu
.
When prompted for password, enter your Purdue career account password followed by ",push
". Your Purdue Duo client will then receive a notification to approve the login.
SSH Keys
Link to section 'General overview' of 'SSH Keys' General overview
To connect to Negishi using SSH keys, you must follow three high-level steps:
- Generate a key pair consisting of a private and a public key on your local machine.
- Copy the public key to the cluster and append it to
$HOME/.ssh/authorized_keys
file in your account. - Test if you can ssh from your local computer to the cluster without using your Purdue password.
Detailed steps for different operating systems and specific SSH client softwares are give below.
Link to section 'Mac and Linux:' of 'SSH Keys' Mac and Linux:
-
Run
ssh-keygen
in a terminal on your local machine. You may supply a filename and a passphrase for protecting your private key, but it is not mandatory. To accept the default settings, press Enter without specifying a filename.
Note: If you do not protect your private key with a passphrase, anyone with access to your computer could SSH to your account on Negishi. -
By default, the key files will be stored in
~/.ssh/id_rsa
and~/.ssh/id_rsa.pub
on your local machine. -
Copy the contents of the public key into
$HOME/.ssh/authorized_keys
on the cluster with the following command. When asked for a password, type your password followed by ",push
". Your Purdue Duo client will receive a notification to approve the login.ssh-copy-id -i ~/.ssh/id_rsa.pub myusername@negishi.rcac.purdue.edu
Note: use your actual Purdue account user name.
If your system does not have the
ssh-copy-id
command, use this instead:cat ~/.ssh/id_rsa.pub | ssh myusername@negishi.rcac.purdue.edu "mkdir -p ~/.ssh && chmod 700 ~/.ssh && cat >> ~/.ssh/authorized_keys"
-
Test the new key by SSH-ing to the server. The login should now complete without asking for a password.
-
If the private key has a non-default name or location, you need to specify the key by
ssh -i my_private_key_name myusername@negishi.rcac.purdue.edu
Link to section 'Windows:' of 'SSH Keys' Windows:
Programs | Instructions |
---|---|
MobaXterm | Open a local terminal and follow Linux steps |
Git Bash | Follow Linux steps |
Windows 10 PowerShell | Follow Linux steps |
Windows 10 Subsystem for Linux | Follow Linux steps |
PuTTY | Follow steps below |
PuTTY:
-
Launch PuTTYgen, keep the default key type (RSA) and length (2048-bits) and click Generate button.
The "Generate" button can be found under the "Actions" section of the PuTTY Key Generator interface. -
Once the key pair is generated:
Use the Save public key button to save the public key, e.g.
Documents\SSH_Keys\mylaptop_public_key.pub
Use the Save private key button to save the private key, e.g.
Documents\SSH_Keys\mylaptop_private_key.ppk
. When saving the private key, you can also choose a reminder comment, as well as an optional passphrase to protect your key, as shown in the image below. Note: If you do not protect your private key with a passphrase, anyone with access to your computer could SSH to your account on Negishi.The PuTTY Key Generator form has inputs for the Key passphrase and optional reminder comment. From the menu of PuTTYgen, use the "Conversion -> Export OpenSSH key" tool to convert the private key into openssh format, e.g.
Documents\SSH_Keys\mylaptop_private_key.openssh
to be used later for Thinlinc. -
Configure PuTTY to use key-based authentication:
Launch PuTTY and navigate to "Connection -> SSH ->Auth" on the left panel, click Browse button under the "Authentication parameters" section and choose your private key, e.g. mylaptop_private_key.ppk
After clicking Connection -> SSH ->Auth panel, the "Browse" option can be found at the bottom of the resulting panel. Navigate back to "Session" on the left panel. Highlight "Default Settings" and click the "Save" button to ensure the change in place.
-
Connect to the cluster. When asked for a password, type your password followed by "
,push
". Your Purdue Duo client will receive a notification to approve the login. Copy the contents of public key from PuTTYgen as shown below and paste it into$HOME/.ssh/authorized_keys
. Please double-check that your text editor did not wrap or fold the pasted value (it should be one very long line).The "Public key" will look like a long string of random letters and numbers in a text box at the top of the window. - Test by connecting to the cluster. If successful, you will not be prompted for a password or receive a Duo notification. If you protected your private key with a passphrase in step 2, you will instead be prompted to enter your chosen passphrase when connecting.
SSH X11 Forwarding
SSH supports tunneling of X11 (X-Windows). If you have an X11 server running on your local machine, you may use X11 applications on remote systems and have their graphical displays appear on your local machine. These X11 connections are tunneled and encrypted automatically by your SSH client.
Link to section 'Installing an X11 Server' of 'SSH X11 Forwarding' Installing an X11 Server
To use X11, you will need to have a local X11 server running on your personal machine. Both free and commercial X11 servers are available for various operating systems.
Linux / Solaris / AIX / HP-UX / Unix:
- An X11 server is at the core of all graphical sessions. If you are logged in to a graphical environment on these operating systems, you are already running an X11 server.
- ThinLinc is an alternative to running an X11 server directly on your Linux computer. ThinLinc is a service that allows you to connect to a persistent remote graphical desktop session.
Microsoft Windows:
- ThinLinc is an alternative to running an X11 server directly on your Windows computer. ThinLinc is a service that allows you to connect to a persistent remote graphical desktop session.
- MobaXterm is a small, easy to use, full-featured SSH client. It includes X11 support for remote displays, SFTP capabilities, and limited SSH authentication forwarding for keys.
Mac OS X:
- X11 is available as an optional install on the Mac OS X install disks prior to 10.7/Lion. Run the installer, select the X11 option, and follow the instructions. For 10.7+ please download XQuartz.
- ThinLinc is an alternative to running an X11 server directly on your Mac computer. ThinLinc is a service that allows you to connect to a persistent remote graphical desktop session.
Link to section 'Enabling X11 Forwarding in your SSH Client' of 'SSH X11 Forwarding' Enabling X11 Forwarding in your SSH Client
Once you are running an X11 server, you will need to enable X11 forwarding/tunneling in your SSH client:
ssh
: X11 tunneling should be enabled by default. To be certain it is enabled, you may usessh -Y
.- MobaXterm: Select "New session" and "SSH." Under "Advanced SSH Settings" check the box for X11 Forwarding.
SSH will set the remote environment variable $DISPLAY
to "localhost:XX.YY"
when this is working correctly. If you had previously set your $DISPLAY
environment variable to your local IP or hostname, you must remove any set
/export
/setenv
of this variable from your login scripts. The environment variable $DISPLAY
must be left as SSH sets it, which is to a random local port address. Setting $DISPLAY
to an IP or hostname will not work.
ThinLinc
RCAC provides Cendio's ThinLinc as an alternative to running an X11 server directly on your computer. It allows you to run graphical applications or graphical interactive jobs directly on Negishi through a persistent remote graphical desktop session.
ThinLinc is a service that allows you to connect to a persistent remote graphical desktop session. This service works very well over a high latency, low bandwidth, or off-campus connection compared to running an X11 server locally. It is also very helpful for Windows users who do not have an easy to use local X11 server, as little to no set up is required on your computer.
There are two ways in which to use ThinLinc: preferably through the native client or through a web browser.
Link to section 'Installing the ThinLinc native client' of 'ThinLinc' Installing the ThinLinc native client
The native ThinLinc client will offer the best experience especially over off-campus connections and is the recommended method for using ThinLinc. It is compatible with Windows, Mac OS X, and Linux.
- Download the ThinLinc client from the ThinLinc website.
- Start the ThinLinc client on your computer.
- In the client's login window, use desktop.negishi.rcac.purdue.edu as the Server. Use your Purdue Career Account username and password, but append "
,push
" to your password. - Click the Connect button.
- Your Purdue Login Duo will receive a notification to approve your login.
- Continue to following section on connecting to Negishi from ThinLinc.
Link to section 'Using ThinLinc through your web browser' of 'ThinLinc' Using ThinLinc through your web browser
The ThinLinc service can be accessed from your web browser as a convenience to installing the native client. This option works with no set up and is a good option for those on computers where you do not have privileges to install software. All that is required is an up-to-date web browser. Older versions of Internet Explorer may not work.
- Open a web browser and navigate to desktop.negishi.rcac.purdue.edu.
- Log in with your Purdue Career Account username and password, but append "
,push
" to your password. - You may safely proceed past any warning messages from your browser.
- Your Purdue Login Duo will receive a notification to approve your login.
- Continue to the following section on connecting to Negishi from ThinLinc.
Link to section 'Connecting to Negishi from ThinLinc' of 'ThinLinc' Connecting to Negishi from ThinLinc
- Once logged in, you will be presented with a remote Linux desktop running directly on a cluster front-end.
- Open the terminal application on the remote desktop.
- Once logged in to the Negishi head node, you may use graphical editors, debuggers, software like Matlab, or run graphical interactive jobs. For example, to test the X forwarding connection issue the following command to launch the graphical editor gedit:
$ gedit
- This session will remain persistent even if you disconnect from the session. Any interactive jobs or applications you left running will continue running even if you are not connected to the session.
Link to section 'Tips for using ThinLinc native client' of 'ThinLinc' Tips for using ThinLinc native client
- To exit a full screen ThinLinc session press the F8 key on your keyboard (fn + F8 key for Mac users) and click to disconnect or exit full screen.
- Full screen mode can be disabled when connecting to a session by clicking the Options button and disabling full screen mode from the Screen tab.
Link to section 'Configure ThinLinc to use SSH Keys' of 'ThinLinc' Configure ThinLinc to use SSH Keys
- The web client does NOT support public-key authentication.
-
ThinLinc native client supports the use of an SSH key pair. For help generating and uploading keys to the cluster, see SSH Keys section in our user guide for details.
To set up SSH key authentication on the ThinLinc client:
-
Open the Options panel, and select Public key as your authentication method on the Security tab.
The "Options..." button in the ThinLinc Client can be found towards the bottom left, above the "Connect" button. -
In the options dialog, switch to the "Security" tab and select the "Public key" radio button:
The "Security" tab found in the options dialog, will be the last of available tabs. The "Public key" option can be found in the "Authentication method" options group. - Click OK to return to the ThinLinc Client login window. You should now see a Key field in place of the Password field.
-
In the Key field, type the path to your locally stored private key or click the ... button to locate and select the key on your local system. Note: If PuTTY is used to generate the SSH Key pairs, please choose the private key in the openssh format.
The ThinLinc Client login window will now display key field instead of a password field.
-
Purchasing Nodes
RCAC operates a significant shared cluster computing infrastructure developed over several years through focused acquisitions using funds from grants, faculty startup packages, and institutional sources. These "community clusters" are now at the foundation of Purdue's research cyberinfrastructure.
We strongly encourage any Purdue faculty or staff with computational needs to join this growing community and enjoy the enormous benefits this shared infrastructure provides:
- Peace of Mind
RCAC system administrators take care of security patches, attempted hacks, operating system upgrades, and hardware repair so faculty and graduate students can concentrate on research.
- Low Overhead
RCAC data centers provide infrastructure such as networking, racks, floor space, cooling, and power.
- Cost Effective
RCAC works with vendors to obtain the best price for computing resources by pooling funds from different disciplines to leverage greater group purchasing power.
Through the Community Cluster Program, Purdue affiliates have invested several million dollars in computational and storage resources from Q4 2006 to the present with great success in both the research accomplished and the money saved on equipment purchases.
For more information or to purchase access to our latest cluster today, see the Purchase page. Have questions? contact us at rcac-cluster-purchase@lists.purdue.edu to discuss.
File Storage and Transfer
Learn more about file storage transfer for Negishi.
Link to section 'Archive and Compression' of 'Archive and Compression' Archive and Compression
There are several options for archiving and compressing groups of files or directories. The mostly commonly used options are:
Link to section 'tar' of 'Archive and Compression' tar
See the official documentation for tar for more information.
Saves many files together into a single archive file, and restores individual files from the archive. Includes automatic archive compression/decompression options and special features for incremental and full backups.
Examples:
(list contents of archive somefile.tar)
$ tar tvf somefile.tar
(extract contents of somefile.tar)
$ tar xvf somefile.tar
(extract contents of gzipped archive somefile.tar.gz)
$ tar xzvf somefile.tar.gz
(extract contents of bzip2 archive somefile.tar.bz2)
$ tar xjvf somefile.tar.bz2
(archive all ".c" files in current directory into one archive file)
$ tar cvf somefile.tar *.c
(archive and gzip-compress all files in a directory into one archive file)
$ tar czvf somefile.tar.gz somedirectory/
(archive and bzip2-compress all files in a directory into one archive file)
$ tar cjvf somefile.tar.bz2 somedirectory/
Other arguments for tar can be explored by using the man tar command.
Link to section 'gzip' of 'Archive and Compression' gzip
The standard compression system for all GNU software.
Examples:
(compress file somefile - also removes uncompressed file)
$ gzip somefile
(uncompress file somefile.gz - also removes compressed file)
$ gunzip somefile.gz
Link to section 'bzip2' of 'Archive and Compression' bzip2
See the official documentation for bzip for more information.
Strong, lossless data compressor based on the Burrows-Wheeler transform. Stronger compression than gzip.
Examples:
(compress file somefile - also removes uncompressed file)
$ bzip2 somefile
(uncompress file somefile.bz2 - also removes compressed file)
$ bunzip2 somefile.bz2
There are several other, less commonly used, options available as well:
- zip
- 7zip
- xz
Link to section 'Storage Environment Variables' of 'Storage Environment Variables' Storage Environment Variables
Several environment variables are automatically defined for you to help you manage your storage. Use environment variables instead of actual paths whenever possible to avoid problems if the specific paths to any of these change.
Name | Description |
---|---|
HOME | /home/myusername |
PWD | path to your current directory |
RCAC_SCRATCH | /scratch/negishi/myusername |
By convention, environment variable names are all uppercase. You may use them on the command line or in any scripts in place of and in combination with hard-coded values:
$ ls $HOME
...
$ ls $RCAC_SCRATCH/myproject
...
To find the value of any environment variable:
$ echo $RCAC_SCRATCH
/scratch/negishi/myusername
To list the values of all environment variables:
$ env
USER=myusername
HOME=/home/myusername
RCAC_SCRATCH=/scratch/negishi/myusername
...
You may create or overwrite an environment variable. To pass (export) the value of a variable in bash:
$ export MYPROJECT=$RCAC_SCRATCH/myproject
To assign a value to an environment variable in either tcsh or csh:
$ setenv MYPROJECT value
Storage Options
File storage options on RCAC systems include long-term storage (home directories, depot, Fortress) and short-term storage (scratch directories, /tmp directory). Each option has different performance and intended uses, and some options vary from system to system as well. Daily snapshots of home directories are provided for a limited time for accidental deletion recovery. Scratch directories and temporary storage are not backed up and old files are regularly purged from scratch and /tmp directories. More details about each storage option appear below.
Home Directory
Home directories are provided for long-term file storage. Each user has one home directory. You should use your home directory for storing important program files, scripts, input data sets, critical results, and frequently used files. You should store infrequently used files on Fortress. Your home directory becomes your current working directory, by default, when you log in.
Your home directory physically resides on a dedicated storage system only accessible for Negishi. To find the path to your home directory, first log in then immediately enter the following:
$ pwd
/home/myusername
Or from any subdirectory:
$ echo $HOME
/home/myusername
Please note that your Negishi home directory and its contents are exclusive to Negishi cluster, including front-end hosts and compute nodes. This home directory is not available on other RCAC machines but Negishi. There is no automatic copying or synchronization between home directories, but at your discretion you can manually copy all or parts of your main home to Negishi using one of the suggested methods.
Your home directory has a quota limiting the total size of files you may store within. For more information, refer to the Storage Quotas / Limits Section.
Link to section 'Lost File Recovery' of 'Home Directory' Lost File Recovery
Nightly snapshots for 7 days, weekly snapshots for 4 weeks, and monthly snapshots for 3 months are kept. This means you will find snapshots from the last 7 nights, the last 4 Sundays, and the last 3 first of the months. Files are available going back between two and three months, depending on how long ago the last first of the month was. Snapshots beyond this are not kept. For additional security, you should store another copy of your files on more permanent storage, such as the Fortress HPSS Archive.
Link to section 'Performance' of 'Home Directory' Performance
Your home directory is medium-performance, non-purged space suitable for tasks like sharing data, editing files, developing and building software, and many other uses.
Your home directory is not designed or intended for use as high-performance working space for running data-intensive jobs with heavy I/O demands.
Link to section 'Long-Term Storage' of 'Long-Term Storage' Long-Term Storage
Long-term Storage or Permanent Storage is available to users on the High Performance Storage System (HPSS), an archival storage system, called Fortress. Program files, data files and any other files which are not used often, but which must be saved, can be put in permanent storage. Fortress currently has over 10PB of capacity.
For more information about Fortress, how it works, and user guides, and how to obtain an account:
/tmp Directory
/tmp directories are provided for short-term file storage only. Each front-end and compute node has a /tmp directory. Your program may write temporary data to the /tmp
directory of the compute node on which it is running. That data is available for as long as your program is active. Once your program terminates, that temporary data is no longer available. When used properly, /tmp
may provide faster local storage to an active process than any other storage option. You should use your home directory and Fortress for longer-term storage or for holding critical results.
Backups are not performed for the /tmp
directory and removes files from /tmp
whenever space is low or whenever the system needs a reboot. In the event of a disk crash or file purge, files in /tmp
are not recoverable. You should copy any important files to more permanent storage.
Scratch Space
Scratch directories are provided for short-term file storage only. The quota of your scratch directory is much greater than the quota of your home directory. You should use your scratch directory for storing temporary input files which your job reads or for writing temporary output files which you may examine after execution of your job. You should use your home directory and Fortress for longer-term storage or for holding critical results. The hsi and htar commands provide easy-to-use interfaces into the archive and can be used to copy files into the archive interactively or even automatically at the end of your regular job submission scripts.
Files in scratch directories are not recoverable. Files in scratch directories are not backed up. If you accidentally delete a file, a disk crashes, or old files are purged, they cannot be restored.
Files are purged from scratch directories not accessed or had content modified in 60 days. Owners of these files receive a notice one week before removal via email. Be sure to regularly check your Purdue email account or set up mail forwarding to an email account you do regularly check. For more information, please refer to our Scratch File Purging Policy.
All users may access scratch directories on Negishi. To find the path to your scratch directory:
$ findscratch
/scratch/negishi/myusername
The value of variable $RCAC_SCRATCH is your scratch directory path. Use this variable in any scripts. Your actual scratch directory path may change without warning, but this variable will remain current.
$ echo $RCAC_SCRATCH
/scratch/negishi/myusername
Scratch directories are specific per cluster. I.e. only the /scratch/negishi directory is available on Negishi front-end and compute nodes. No other scratch directories are available on Negishi.
Your scratch directory has a quota capping the total size and number of files you may store in it. For more information, refer to the section Storage Quotas / Limits.
Link to section 'Performance' of 'Scratch Space' Performance
Your scratch directory is located on a high-performance, large-capacity parallel filesystem engineered to provide work-area storage optimized for a wide variety of job types. It is designed to perform well with data-intensive computations, while scaling well to large numbers of simultaneous connections.
File Transfer
Negishi supports several methods for file transfer. Use the links below to learn more about these methods.
SCP
SCP (Secure CoPy) is a simple way of transferring files between two machines that use the SSH protocol. SCP is available as a protocol choice in some graphical file transfer programs and also as a command line program on most Linux, Unix, and Mac OS X systems. SCP can copy single files, but will also recursively copy directory contents if given a directory name.
After Aug 17, 2020, the community clusters will not support password-based authentication for login. Methods that can be used include two-factor authentication (Purdue Login) or SSH keys. If you do not have SSH keys installed, you would need to type your Purdue Login response into the SFTP's "Password" prompt.
Link to section 'Command-line usage:' of 'SCP' Command-line usage:
You can transfer files both to and from Negishi while initiating an SCP session on either some other computer or on Negishi (in other words, directionality of connection and directionality of data flow are independent from each other). The scp
command appears somewhat similar to the familiar cp
command, with an extra user@host:file
syntax to denote files and directories on a remote host. Either Negishi or another computer can be a remote.
-
Example: Initiating SCP session on some other computer (i.e. you are on some other computer, connecting to Negishi):
(transfer TO Negishi) (Individual files) $ scp sourcefile myusername@negishi.rcac.purdue.edu:somedir/destinationfile $ scp sourcefile myusername@negishi.rcac.purdue.edu:somedir/ (Recursive directory copy) $ scp -pr sourcedirectory/ myusername@negishi.rcac.purdue.edu:somedir/ (transfer FROM Negishi) (Individual files) $ scp myusername@negishi.rcac.purdue.edu:somedir/sourcefile destinationfile $ scp myusername@negishi.rcac.purdue.edu:somedir/sourcefile somedir/ (Recursive directory copy) $ scp -pr myusername@negishi.rcac.purdue.edu:sourcedirectory somedir/
The -p flag is optional. When used, it will cause the transfer to preserve file attributes and permissions. The -r flag is required for recursive transfers of entire directories.
-
Example: Initiating SCP session on Negishi (i.e. you are on Negishi, connecting to some other computer):
(transfer TO Negishi) (Individual files) $ scp myusername@$another.computer.example.com:sourcefile somedir/destinationfile $ scp myusername@$another.computer.example.com:sourcefile somedir/ (Recursive directory copy) $ scp -pr myusername@$another.computer.example.com:sourcedirectory/ somedir/ (transfer FROM Negishi) (Individual files) $ scp somedir/sourcefile myusername@$another.computer.example.com:destinationfile $ scp somedir/sourcefile myusername@$another.computer.example.com:somedir/ (Recursive directory copy) $ scp -pr sourcedirectory myusername@$another.computer.example.com:somedir/
The -p flag is optional. When used, it will cause the transfer to preserve file attributes and permissions. The -r flag is required for recursive transfers of entire directories.
Link to section 'Software (SCP clients)' of 'SCP' Software (SCP clients)
Linux and other Unix-like systems:
- The
scp
command-line program should already be installed.
Microsoft Windows:
- MobaXterm
Free, full-featured, graphical Windows SSH, SCP, and SFTP client. - Command-line
scp
program can be installed as part of Windows Subsystem for Linux (WSL), or Git-Bash.
Mac OS X:
- The
scp
command-line program should already be installed. You may start a local terminal window from "Applications->Utilities". - Cyberduck is a full-featured and free graphical SFTP and SCP client.
Globus
Link to section 'Globus' of 'Globus' Globus
Globus, previously known as Globus Online, is a powerful and easy to use file transfer service for transferring files virtually anywhere. It works within RCAC's various research storage systems; it connects between RCAC and remote research sites running Globus; and it connects research systems to personal systems. You may use Globus to connect to your home, scratch, and Fortress storage directories. Since Globus is web-based, it works on any operating system that is connected to the internet. The Globus Personal client is available on Windows, Linux, and Mac OS X. It is primarily used as a graphical means of transfer but it can also be used over the command line.
Link to section 'Link to section 'Globus Web:' of 'Globus' Globus Web:' of 'Globus' Link to section 'Globus Web:' of 'Globus' Globus Web:
- Navigate to http://transfer.rcac.purdue.edu
- Click "Proceed" to log in with your Purdue Career Account.
- On your first login it will ask to make a connection to a Globus account. Accept the conditions.
- Now you are at the main screen. Click "File Transfer" which will bring you to a two-panel interface (if you only see one panel, you can use selector in the top-right corner to switch the view).
- You will need to select one collection and file path on one side as the source, and the second collection on the other as the destination. This can be one of several Purdue endpoints, or another University, or even your personal computer (see Personal Client section below).
The RCAC collections are as follows. A search for "Purdue" will give you several suggested results you can choose from, or you can give a more specific search.
- Home Directory storage: "Purdue Research Computing - Home Directories", however, you can start typing "Purdue" and "Home Directories" and it will suggest appropriate matches.
- Weber scratch storage: "Purdue Weber Cluster", however, you can start typing "Purdue" and "Weber and it will suggest appropriate matches. From here you will need to navigate into the first letter of your username, and then into your username.
- Research Data Depot: "Purdue Research Computing - Data Depot", a search for "Depot" should provide appropriate matches to choose from.
- Fortress: "Purdue Fortress HPSS Archive", a search for "Fortress" should provide appropriate matches to choose from.
From here, select a file or folder in either side of the two-pane window, and then use the arrows in the top-middle of the interface to instruct Globus to move files from one side to the other. You can transfer files in either direction. You will receive an email once the transfer is completed.
Link to section 'Link to section 'Globus Personal Client setup:' of 'Globus' Globus Personal Client setup:' of 'Globus' Link to section 'Globus Personal Client setup:' of 'Globus' Globus Personal Client setup:
Globus Connect Personal is a small software tool you can install to make your own computer a Globus endpoint on its own. It is useful if you need to transfer files via Globus to and from your computer directly.
- On the "Collections" page from earlier, click "Get Globus Connect Personal" or download a version for your operating system it from here: Globus Connect Personal
- Name this particular personal system and follow the setup prompts to create your Globus Connect Personal endpoint.
- Your personal system is now available as a collection within the Globus transfer interface.
Link to section 'Link to section 'Globus Command Line:' of 'Globus' Globus Command Line:' of 'Globus' Link to section 'Globus Command Line:' of 'Globus' Globus Command Line:
Globus supports command line interface, allowing advanced automation of your transfers.
To use the recommended standalone Globus CLI application (the globus command):
- First time use: issue the globus login command and follow instructions for initial login.
- Commands for interfacing with the CLI can be found via Using the Command Line Interface, as well as the Globus CLI Examples pages.
Link to section 'Link to section 'Sharing Data with Outside Collaborators' of 'Globus' Sharing Data with Outside Collaborators' of 'Globus' Link to section 'Sharing Data with Outside Collaborators' of 'Globus' Sharing Data with Outside Collaborators
Globus allows convenient sharing of data with outside collaborators. Data can be shared with collaborators' personal computers or directly with many other computing resources at other institutions. See the Globus documentation on how to share data:
For links to more information, please see Globus Support page and RCAC Globus presentation.
Windows Network Drive / SMB
SMB (Server Message Block), also known as CIFS, is an easy to use file transfer protocol that is useful for transferring files between RCAC systems and a desktop or laptop. You may use SMB to connect to your home, scratch, and Fortress storage directories. The SMB protocol is available on Windows, Linux, and Mac OS X. It is primarily used as a graphical means of transfer but it can also be used over the command line.
Note: to access Negishi through SMB file sharing, you must be on a Purdue campus network or connected through VPN.
Link to section 'Windows:' of 'Windows Network Drive / SMB' Windows:
- Windows 7: Click Windows menu > Computer, then click Map Network Drive in the top bar
- Windows 8 & 10: Tap the Windows key, type computer, select This PC, click Computer > Map Network Drive in the top bar
- Windows 11: Tap the Windows key, type File Explorer, select This PC, click Computer > Map Network Drive in the top bar
- In the folder location enter the following information and click Finish:
- To access your Negishi home directory, enter \\home.negishi.rcac.purdue.edu\negishi-home.
- To access your scratch space on Negishi, enter \\scratch.negishi.rcac.purdue.edu\negishi-scratch. Once mapped, you will be able to navigate to your scratch directory.
- Note: Use your career account login name and password when prompted. (You will not need to add "
,push
" nor use your Purdue Duo client.) - Your home or scratch directory should now be mounted as a drive in the Computer window.
Link to section 'Mac OS X:' of 'Windows Network Drive / SMB' Mac OS X:
- In the Finder, click Go > Connect to Server
- In the Server Address enter the following information and click Connect:
- To access your Negishi home directory, enter smb://home.negishi.rcac.purdue.edu/negishi-home.
- To access your scratch space on Negishi, enter smb://scratch.negishi.rcac.purdue.edu/negishi-scratch. Once mapped, you will be able to navigate to your scratch directory.
- Note: Use your career account login name and password when prompted. (You will not need to add "
,push
" nor use your Purdue Duo client.) - Your home or scratch directory should now be mounted as a drive in the Computer window.
Link to section 'Linux:' of 'Windows Network Drive / SMB' Linux:
- There are several graphical methods to connect in Linux depending on your desktop environment. Once you find out how to connect to a network server on your desktop environment, choose the Samba/SMB protocol and adapt the information from the Mac OS X section to connect.
- If you would like access via samba on the command line you may install smbclient which will give you FTP-like access and can be used as shown below. For all the possible ways to connect look at the Mac OS X instructions.
smbclient //home.negishi.rcac.purdue.edu/negishi-home -U myusername smbclient //scratch.negishi.rcac.purdue.edu/negishi-scratch -U myusername
- Note: Use your career account login name and password when prompted. (You will not need to add "
,push
" nor use your Purdue Duo client.)
FTP / SFTP
FTP is not supported on any research systems because it does not allow for secure transmission of data. Use SFTP instead, as described below.
SFTP (Secure File Transfer Protocol) is a reliable way of transferring files between two machines. SFTP is available as a protocol choice in some graphical file transfer programs and also as a command-line program on most Linux, Unix, and Mac OS X systems. SFTP has more features than SCP and allows for other operations on remote files, remote directory listing, and resuming interrupted transfers. Command-line SFTP cannot recursively copy directory contents; to do so, try using SCP or graphical SFTP client.
After Aug 17, 2020, the community clusters will not support password-based authentication for login. Methods that can be used include two-factor authentication (Purdue Login) or SSH keys. If you do not have SSH keys installed, you would need to type your Purdue Login response into the SFTP's "Password" prompt.
Link to section 'Command-line usage' of 'FTP / SFTP' Command-line usage
You can transfer files both to and from Negishi while initiating an SFTP session on either some other computer or on Negishi (in other words, directionality of connection and directionality of data flow are independent from each other). Once the connection is established, you use put
or get
subcommands between "local" and "remote" computers. Either Negishi or another computer can be a remote.
-
Example: Initiating SFTP session on some other computer (i.e. you are on another computer, connecting to Negishi):
$ sftp myusername@negishi.rcac.purdue.edu (transfer TO Negishi) sftp> put sourcefile somedir/destinationfile sftp> put -P sourcefile somedir/ (transfer FROM Negishi) sftp> get sourcefile somedir/destinationfile sftp> get -P sourcefile somedir/ sftp> exit
The -P flag is optional. When used, it will cause the transfer to preserve file attributes and permissions.
-
Example: Initiating SFTP session on Negishi (i.e. you are on Negishi, connecting to some other computer):
$ sftp myusername@$another.computer.example.com (transfer TO Negishi) sftp> get sourcefile somedir/destinationfile sftp> get -P sourcefile somedir/ (transfer FROM Negishi) sftp> put sourcefile somedir/destinationfile sftp> put -P sourcefile somedir/ sftp> exit
The -P flag is optional. When used, it will cause the transfer to preserve file attributes and permissions.
Link to section 'Software (SFTP clients)' of 'FTP / SFTP' Software (SFTP clients)
Linux and other Unix-like systems:
- The
sftp
command-line program should already be installed.
Microsoft Windows:
- MobaXterm
Free, full-featured, graphical Windows SSH, SCP, and SFTP client. - Command-line
sftp
program can be installed as part of Windows Subsystem for Linux (WSL), or Git-Bash.
Mac OS X:
- The
sftp
command-line program should already be installed. You may start a local terminal window from "Applications->Utilities". - Cyberduck is a full-featured and free graphical SFTP and SCP client.
Copying files from Purdue IT research computing home directory to Negishi
The Negishi home directory and its contents are specific to the Negishi cluster, and are not available on other RCAC machines. For people having access to other Community Clusters and Negishi, there is no automatic copying or synchronization between main and Negishi home directories. At your discretion, you can manually copy all or parts of your main research computing home to Negishi using one of the methods described below.
Please note that copying may fail if the size of your research computing home directory is larger than the Negishi one's quota. Please check usage and limits before proceeding!
Link to section 'Complete copy' of 'Copying files from Purdue IT research computing home directory to Negishi' Complete copy
For your convenience, a custom tool copy-rcac-home
is provided to simplify at-will duplication of your main research computing home directory into Negishi. The tool performs a complete 1-to-1 copy using rsync -auH
(with exception of a narrow subset of system-specific service files).
To use the tool, simply type copy-rcac-home
in a terminal window on a Negishi front-end or compute node:
$ copy-rcac-home
This script will copy entire contents of your main RCAC
home directory into your Negishi cluster's $HOME.
Note: copying may fail if the size of your RCAC home directory
is larger than your quota on the Negishi one (25GB).
BEFORE PROCEEDING, please run 'myquota' command on another
cluster to see your usage there and judge whether it would fit!
Would you like to proceed? [Y/n]:
At this stage answering yes
will proceed with copying, or you can respond with a no
(or Ctrl-C
) to cancel. See copy-rcac-home --help
for more details on the tool.
Link to section 'Partial copy' of 'Copying files from Purdue IT research computing home directory to Negishi' Partial copy
Desired parts (or whole) of your research computing home directories can be copied to Negishi via any of the home directories' supported transfer methods, such as SCP, SFTP, rsync, or Globus.
-
Example: recursive copying of a subdirectory from RCAC home directory into Negishi home using
scp
.(if you are on Negishi, use other cluster name for the remote part) $ scp -pr myothercluster.rcac.purdue.edu:somedirectory/ ~/ (if you are on another cluster, use Negishi for the remote part) $ scp -pr somedirectory/ myusername@negishi.rcac.purdue.edu:~/
-
Example: copying using Globus.
Search collections for "Purdue Research Computing - Home Directories" and "Purdue Negishi Cluster" endpoints, respectively, then transfer desired files and/or directories as usual.
Storage Quota / Limits
Some limits are imposed on your disk usage on research systems. A quota is implemented on each filesystem. Each filesystem (home directory, scratch directory, etc.) may have a different limit. If you exceed the quota, you will not be able to save new files or new data to the filesystem until you delete or move data to long-term storage.
Link to section 'Checking Quota' of 'Storage Quota / Limits' Checking Quota
To check the current quotas of your home and scratch directories check the My Quota page or use the myquota
command:
$ myquota
Type Filesystem Size Limit Use Files Limit Use
==============================================================================
home myusername 5.0GB 25.0GB 20% - - -
scratch negishi 220.7GB 100.0TB 0.22% 8k 2,000k 0.43%
The columns are as follows:
- Type: indicates home or scratch directory or your depot space.
- Filesystem: name of storage option.
- Size: sum of file sizes in bytes.
- Limit: allowed maximum on sum of file sizes in bytes.
- Use: percentage of file-size limit currently in use.
- Files: number of files and directories (not the size).
- Limit: allowed maximum on number of files and directories. It is possible, though unlikely, to reach this limit and not the file-size limit if you create a large number of very small files.
- Use: percentage of file-number limit currently in use.
If you find that you reached your quota in either your home directory or your scratch file directory, obtain estimates of your disk usage. Find the top-level directories which have a high disk usage, then study the subdirectories to discover where the heaviest usage lies.
To see in a human-readable format an estimate of the disk usage of your top-level directories in your home directory:
$ du -h --max-depth=1 $HOME >myfile
32K /home/myusername/mysubdirectory_1
529M /home/myusername/mysubdirectory_2
608K /home/myusername/mysubdirectory_3
The second directory is the largest of the three, so apply command du to it.
To see in a human-readable format an estimate of the disk usage of your top-level directories in your scratch file directory:
$ du -h --max-depth=1 $RCAC_SCRATCH >myfile
160K /scratch/negishi/myusername
This strategy can be very helpful in figuring out the location of your largest usage. Move unneeded files and directories to long-term storage to free space in your home and scratch directories.
Link to section 'Increasing Quota' of 'Storage Quota / Limits' Increasing Quota
Link to section 'Home Directory' of 'Storage Quota / Limits' Home Directory
If you find you need additional disk space in your home directory, please consider archiving and compressing old files and moving them to long-term storage on the Fortress HPSS Archive, or purchase the Depot space for long-term storage. Unfortunately, it is not possible to increase your home directory quota beyond it's current level.
Link to section 'Scratch Space' of 'Storage Quota / Limits' Scratch Space
If you find you need additional disk space in your scratch space, please first consider archiving and compressing old files and moving them to long-term storage on the Fortress HPSS Archive. If you are unable to do so, you may ask for a quota increase by contacting support.
Link to section 'Sharing Files from Negishi' of 'Sharing' Sharing Files from Negishi
Negishi supports several methods for file sharing. Use the links below to learn more about these methods.
Link to section 'Sharing Data with Globus' of 'Globus' Sharing Data with Globus
Data on any RCAC resource can be shared with other users within Purdue or with collaborators at other institutions. Globus allows convenient sharing of data with outside collaborators. Data can be shared with collaborators' personal computers or directly with many other computing resources at other institutions.
To share files, login to https://transfer.rcac.purdue.edu, navigate to the endpoint (collection) of your choice, and follow instructions as described in Globus documentation on how to share data:
See also RCAC Globus presentation.
Lost File Recovery
Negishi is protected against accidental file deletion through a series of snapshots taken every night just after midnight. Each snapshot provides the state of your files at the time the snapshot was taken. It does so by storing only the files which have changed between snapshots. A file that has not changed between snapshots is only stored once but will appear in every snapshot. This is an efficient method of providing snapshots because the snapshot system does not have to store multiple copies of every file.
These snapshots are kept for a limited time at various intervals. RCAC keeps nightly snapshots for 7 days, weekly snapshots for 4 weeks, and monthly snapshots for 3 months. This means you will find snapshots from the last 7 nights, the last 4 Sundays, and the last 3 first of the months. Files are available going back between two and three months, depending on how long ago the last first of the month was. Snapshots beyond this are not kept.
Only files which have been saved during an overnight snapshot are recoverable. If you lose a file the same day you created it, the file is not recoverable because the snapshot system has not had a chance to save the file.
Snapshots are not a substitute for regular backups. It is the responsibility of the researchers to back up any important data to the Fortress Archive. Negishi does protect against hardware failures or physical disasters through other means however these other means are also not substitutes for backups.
Files in scratch directories are not recoverable. Files in scratch directories are not backed up. If you accidentally delete a file, a disk crashes, or old files are purged, they cannot be restored.
Negishi offers several ways for researchers to access snapshots of their files.
flost
If you know when you lost the file, the easiest way is to use the flost command. This tool is available from any RCAC resource. If you do not have access to a compute cluster, any Data Depot user may use an SSH client to connect to negishi.rcac.purdue.edu and run this command.
To run the tool you will need to specify the location where the lost file was with the -w argument:
$ flost -w /depot/mylab
Replace mylab with the name of your lab's Negishi directory. If you know more specifically where the lost file was you may provide the full path to that directory.
This tool will prompt you for the date on which you lost the file or would like to recover the file from. If the tool finds an appropriate snapshot it will provide instructions on how to search for and recover the file.
If you are not sure what date you lost the file you may try entering different dates into the flost to try to find the file or you may also manually browse the snapshots as described below.
Manual Browsing
You may also search through the snapshots by hand on the Negishi filesystem if you are not sure what date you lost the file or would like to browse by hand. Snapshots can be browsed from any RCAC resource. If you do not have access to a compute cluster, any Negishi user may use an SSH client to connect to negishi.rcac.purdue.edu and browse from there. The snapshots are located at /depot/.snapshots
on these resources.
You can also mount the snapshot directory over Samba (or SMB, CIFS) on Windows or Mac OS X. Mount (or map) the snapshot directory in the same way as you did for your main Negishi space substituting the server name and path for \\datadepot.rcac.purdue.edu\depot\.winsnaps
(Windows) or smb://datadepot.rcac.purdue.edu/depot/.winsnaps
(Mac OS X).
Once connected to the snapshot directory through SSH or Samba, you will see something similar to this:
SSH to negishi.rcac.purdue.edu | Samba mount on datadepot.rcac.purdue.edu |
---|---|
|
![]() |
Each of these directories is a snapshot of the entire Negishi filesystem at the timestamp encoded into the directory name. The format for this timestamp is year, two digits for month, two digits for day, followed by the time of the day.
You may cd into any of these directories where you will find the entire Negishi filesystem. Use cd to continue into your lab's Negishi space and then you may browse the snapshot as normal.
If you are browsing these directories over a Samba network drive you can simply drag and drop the files over into your live Data Depot folder.
Once you find the file you are looking for, use cp to copy the file back into your lab's live Negishi space. Do not attempt to modify files directly in the snapshot directories.
Windows
If you use Negishi through "network drives" on Windows you may recover lost files directly from within Windows:
- Open the folder that contained the lost file.
- Right click inside the window and select "Properties".
- Click on the "Previous Versions" tab.
- A list of snapshots will be displayed.
- Select the snapshot from which you wish to restore.
- In the new window, locate the file you wish to restore.
- Simply drag the file or folder to their correct locations.
In the "Previous Versions" window the list contains two columns. The first column is the timestamp on which the snapshot was taken. The second column is the date on which the selected file or folder was last modified in that snapshot. This may give you some extra clues to which snapshot contains the version of the file you are looking for.
Mac OS X
Mac OS X does not provide any way to access the Negishi snapshots directly. To access the snapshots there are two options: browse the snapshots by hand through a network drive mount or use an automated command-line based tool.
To browse the snapshots by hand, follow the directions outlined in the Manual Browsing section.
To use the automated command-line tool, log into a compute cluster or into the host negishi.rcac.purdue.edu (which is available to all Negishi users) and use the flost tool. On Mac OS X you can use the built-in SSH terminal application to connect.
- Open the Applications folder from Finder.
- Navigate to the Utilities folder.
- Double click the Terminal application to open it.
- Type the following command when the terminal opens.
Replace myusername with your Purdue career account username and provide your password when prompted.$ ssh myusername@negishi.rcac.purdue.edu
Once logged in use the flost tool as described above. The tool will guide you through the process and show you the commands necessary to retrieve your lost file.
Gateway (Open OnDemand)
Negishi's Gateway is an open-source HPC portal developed by the Ohio Supercomputing Center. Open OnDemand allows one to interact with HPC resources through a web browser and easily manage files, submit jobs, and interact with graphical applications directly in a browser, all with no software to install. Negishi has an instance of OnDemand available that can be accessed via gateway.negishi.rcac.purdue.edu.
Link to section 'Logging In' of 'Gateway (Open OnDemand)' Logging In
To log into Gateway:
- Navigate to gateway.negishi.rcac.purdue.edu
- Log in using your Career account username and Purdue Login Duo client.
On the splash page you will see a quota usage report. If you are over 90% on any of your quotas a warning will be displayed. This information will update every 10-15 minutes while you are active on Gateway.
Link to section 'Apps' of 'Gateway (Open OnDemand)' Apps
There are a number of built-in apps in Gateway that can be accessed from the top menu bar. Below are links to documentation on each app.
Interactive Apps
There are several interactive apps available through Gateway that can be accessed through the Interactive Apps dropdown menu. These apps are provided with a basic node and software configuration as a 'quick-launch' option to get your work up and running quickly. For simplicity, minimal options are provided - these apps are not intended for complex configuration/customization scenarios.
After you a submit an interactive app to the queue, Gateway will track and manage the session. Once it starts, you may connect and disconnect from the session in your browser, leaving the job running while you log out of your browser.
Each of the available apps are documented through the following links.
Compute Node Desktop
The Compute Node Desktop app will launch a graphical desktop session on a compute node. This is similar to using Thinlinc, however, this gives you a desktop directly on a compute node instead on a front-end. This app is useful if you have a custom application or application not directly available as an interactive app you would like to run inside Gateway.
To launch a desktop session on a compute node, select the Negishi Compute Desktop app. From the submit form, select from the available options - the queue to which you wish to submit and the number of wallclock hours you wish to have job running. There is also a checkbox that enable a notification to your email when the job starts.
After the interactive job is submitted you will be taken to your list of active interactive app sessions. You can monitor the status of the job from here until it starts, or if you enabled the email notification, watch your Purdue email for the notification the job has started.
Once it is indicated the job has started you can connect to the desktop with the "Launch noVNC in New Tab" button. The session will be terminated after the wallclock hours you specified have elapsed or you terminate the session early with the "Delete" button from the list of sessions. Deleting the session when you are finished will free up queue resources for your lab mates and other users on the system.
MATLAB
The MATLAB app will launch a MATLAB session on a compute node and allow you to connect directly to it in a web browser.
To launch a MATLAB session on a compute node, select the MATLAB app. From the submit form, select from the available options - the version of MATLAB you are interested in running, the queue to which you wish to submit, and the number of wallclock hours you wish to have job running. There is also a checkbox that enable a notification to your email when the job starts.
After the interactive job is submitted you will be taken to your list of active interactive app sessions. You can monitor the status of the job from here until it starts, or if you enabled the email notification, watch your Purdue email for the notification the job has started.
Once it is indicated the job has started you can connect to the desktop with the "Launch noVNC in New Tab" button. The session will be terminated after the wallclock hours you specified have elapsed or you terminate the session early with the "Delete" button from the list of sessions. Deleting the session when you are finished will free up queue resources for your lab mates and other users on the system.
Jupyter Notebook
The Notebook app will launch a Notebook session on a compute node and allow you to connect directly to it in a web browser.
To launch a Notebook session on a compute node, select the Notebook app. From the submit form, select from the available options:
- Queue: This is a dropdown menu from which you can select a queue from all of the queues to which you have permission to submit.
- Walltime: This is a field which expects a number and represents how many hours you want to keep the session running. Note that this value should not exceed the maximum value given next to the selected queue name from the queue dropdown menu.
- Number of Cores/GPUs: This is a field which expects a number and represents the number of your resources your session is requesting. Note that the amount of memory allocated for your session is proportional to the number of cores or GPUs that you request for your job, so if your session is running out of memory, consider increasing this value.
- Use Jupyter Lab: This is a checkbox which, when checked, will run Jupyter Lab instead of Jupyter Notebook. Both of these applications are interfaces to Jupyter, and you can launch Jupyter notebooks from within Jupyter Lab. Jupyter Notebook is more "barebones" while Jupyter Lab has additional features such as the ability to interact with additional file types.
- E-mail Notice: This is a checkbox which, when checked, will send you an e-mail notification to your Purdue e-mail that your session is ready when the scheduler has found resources to dedicate to your session.
After the interactive job is submitted you will be taken to your list of active interactive app sessions. You can monitor the status of the job from here until it starts, or if you enabled the email notification, watch your Purdue email for the notification the job has started.
Once it is indicated the job has started you can connect to the desktop with the "Connect to Jupyter" button. Once connected, you can create new notebooks, selecting the currently available Anaconda versions available as modules, and any personally created Notebook kernels.
Often times you may want to use one of your existing Anaconda environments within your Jupyter session to use libraries specific to your workflow. In order to do so, you must ensure that the Anaconda environment you want to use contains the Python packages "IPyKernel" and "IPython" which are packages that are required by Jupyter. When you create a Jupyter session, Open OnDemand will check through your existing Anaconda environments and create a Jupyter kernel for any Anaconda environment that contains these two packages, and you will be able to select to use that kernel from within the application.
The session will be terminated after the number of hours you specified have elapsed or you terminate the session early with the "Delete" button from the list of sessions. Deleting the session when you are finished will free up queue resources for your lab mates and other users on the system.
RStudio Server
The RStudio app will launch a RStudio session on a compute node and allow you to connect directly to it in a web browser.
To launch a RStudio session on a compute node, select the RStudio app. From the submit form, select from the available options - the queue to which you wish to submit, and the number of wallclock hours you wish to have job running. There is also a checkbox that enable a notification to your email when the job starts.
After the interactive job is submitted you will be taken to your list of active interactive app sessions. You can monitor the status of the job from here until it starts, or if you enabled the email notification, watch your Purdue email for the notification the job has started.
Once it is indicated the job has started you can connect to the desktop with the "Connect to RStudio Server" button. The session will be terminated after the wallclock hours you specified have elapsed or you terminate the session early with the "Delete" button from the list of sessions. Deleting the session when you are finished will free up queue resources for your lab mates and other users on the system.
Files
The Files app will let you access your files in your Home Directory, Scratch, and Data Depot spaces. The app lets you manage create, manage, and delete files and directories from your web browser. Navigate by double clicking on folders in the file explorer or by using the file tree on the left.
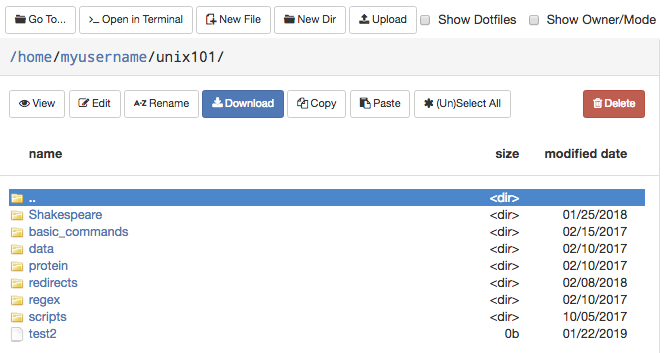
On the top row, there are buttons to:
- Go To: directly input a directory to navigate to
- Open in Terminal: launches the Shell app and navigates you to the current directory in the terminal
- New File: creates a new, empty file
- New Dir: creates a new, empty directory
- Upload: upload a file from your computer
Note: File uploads from your browser are limited to 100 GB per file. Be mindful that uploads over a few gigabytes may be unreliable through your browser, especially from off-campus connections. For very large files or off-campus transfers alternative methods such as Globus are highly recommended.
The second row of buttons lets you perform typical file management operations. The Edit button will open files in a fully fledged browser based text editor - it features syntax highlighting and vim and Emacs key bindings.
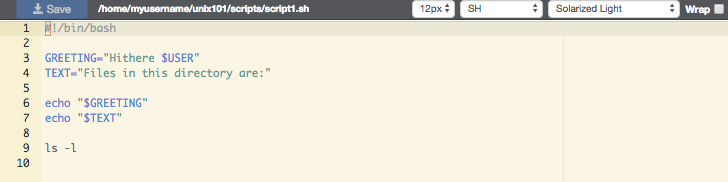
Jobs
There are two apps under the Jobs apps: Active Jobs and Job Composer. These are detailed below.
Link to section 'Active Jobs' of 'Jobs' Active Jobs
This shows you active SLURM jobs currently on the cluster. The default view will show you your current jobs, similar to squeue -u rices
. Using the button labeled "Your Jobs" in the upper right allows you to select different filters by queue (account). All accounts output by slist
will appear for you here. Using the arrow on the left hand side will expand the full job details.
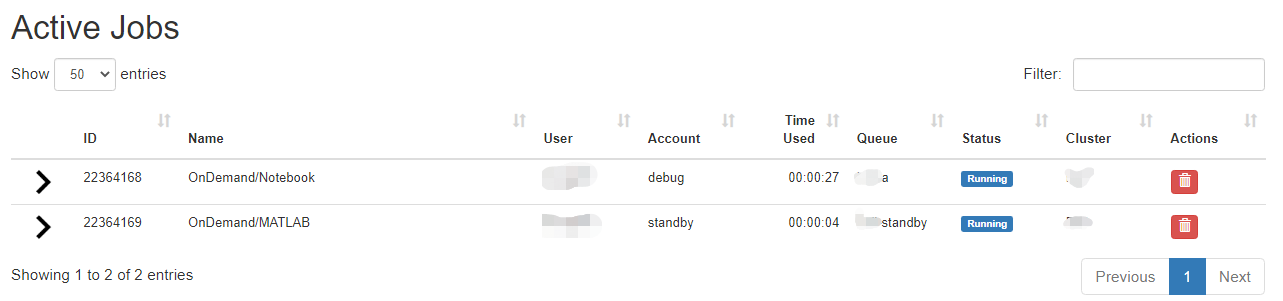
Link to section 'Job Composer' of 'Jobs' Job Composer
The Job Composer app allows you to create and submit jobs to the cluster. You can select from pre-defined templates (most of these are taken from the User Guide examples) or you can create your own templates for frequently used workflows.
Link to section 'Creating Job from Existing Template' of 'Jobs' Creating Job from Existing Template
Click "New Job" menu, then select "From Template":
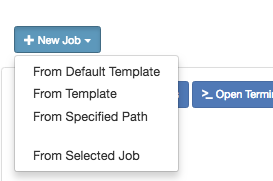
Then select from one of the available templates.
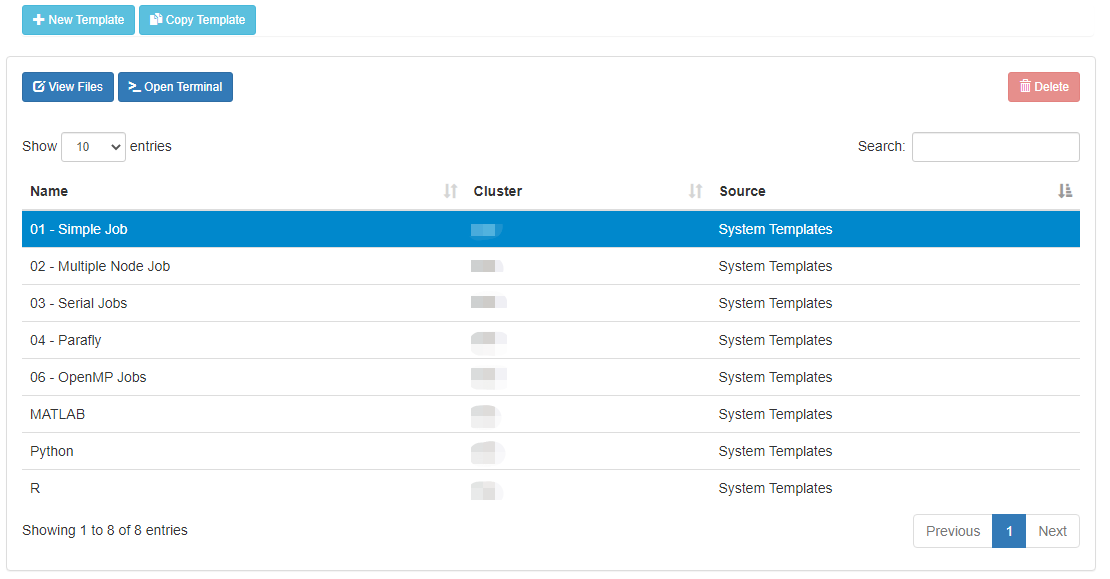
Click 'Create New Job' in second pane.
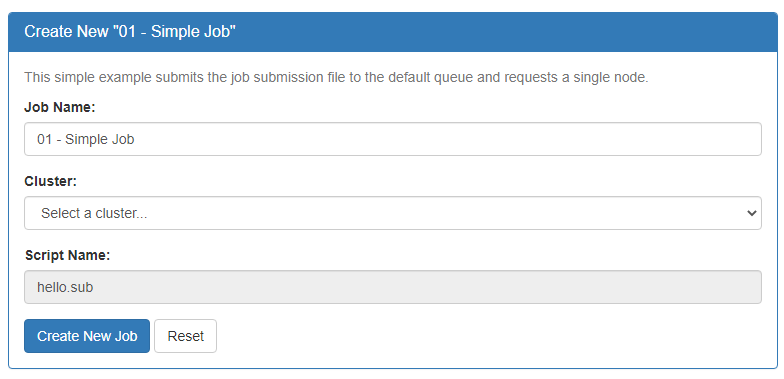
Your new job should be selected in your list of jobs. In the 'Submit Script' pane you can see the job script that was generated with an 'Open Editor' link to open the script in the built-in editor. Open the file in the editor and edit the script as necessary. By default the job will specify standby queue - this should be changed as appropriate, along with the node and walltime requests.
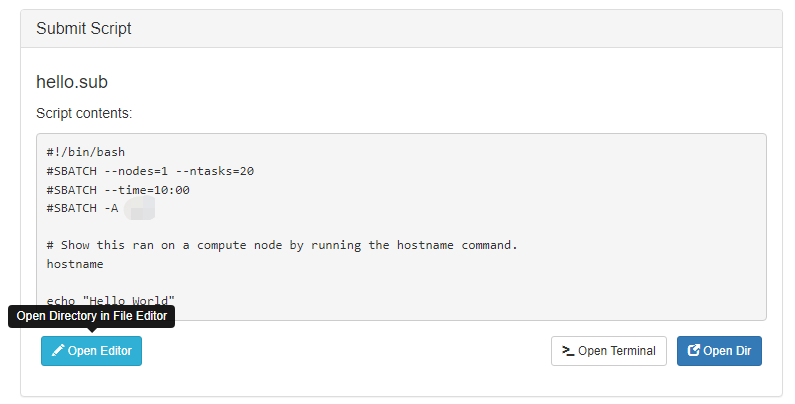
When you are finished with editing the job and are ready to submit, click the green 'Submit' button at the top of the job list. You can monitor progress from here or from the Active Jobs app. Once completed, you should see the output files appear:
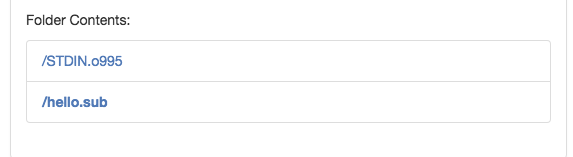
Clicking on one of the output files will open it in the file editor for your viewing.
Link to section 'Creating New Template' of 'Jobs' Creating New Template
First, prepare a template directory containing a template submission script along with any input files. Then, to import the job into the Job Composer app, click the 'Create New Template' button. Fill in the directory containing your template job script and files in the first box. Give it an appropriate name and notes.
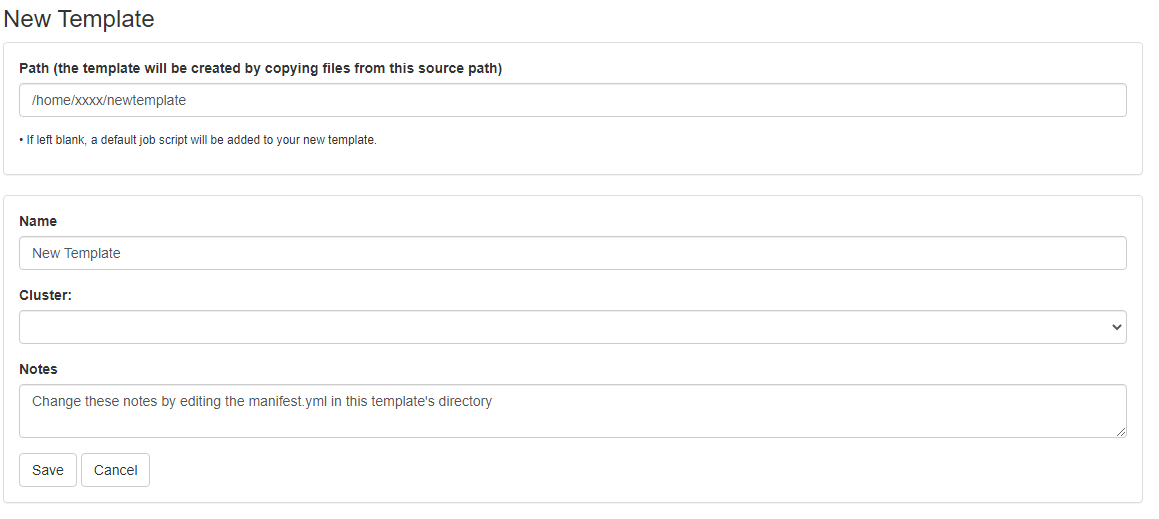
This template will now appear in your list of templates to choose from when composing jobs. You can now go create and submit a job from this new template.
Cluster Tools
The Cluster Tools menu contains cluster utilities. At the moment, only a terminal app is provided. Additional apps may be developed and provided in the future.
Link to section 'Shell Access' of 'Cluster Tools' Shell Access
Launching the shell app will provide you with a web-based terminal session on the cluster front-end. This is equivalent to using a standalone SSH client to connect to negishi.rcac.purdue.edu
where you are connected to one several front-ends. The normal acceptable front-end use policy applies to access through the web-app. X11 Forwarding is not supported. Use of one of the interactive apps is recommended for graphical applications.
Software
Link to section 'Environment module' of 'Software' Environment module
Link to section 'Software catalog' of 'Software' Software catalog
Compiling Source Code
Documentation on compiling source code on Negishi.
Compiling Serial Programs
A serial program is a single process which executes as a sequential stream of instructions on one processor core. Compilers capable of serial programming are available for C, C++, and versions of Fortran.
Here are a few sample serial programs:
- serial_hello.f
- serial_hello.f90
- serial_hello.f95
- serial_hello.c
-
To load a compiler, enter one of the following:
$ module load intel
$ module load gcc
Language | Intel Compiler | GNU Compiler | |
---|---|---|---|
Fortran 77 |
|
|
|
Fortran 90 |
|
|
|
Fortran 95 |
|
|
|
C |
|
|
|
C++ |
|
|
The Intel and GNU compilers will not output anything for a successful compilation. Also, the Intel compiler does not recognize the suffix ".f95".
Compiling MPI Programs
OpenMPI and Intel MPI (IMPI) are implementations of the Message-Passing Interface (MPI) standard. Libraries for these MPI implementations and compilers for C, C++, and Fortran are available on all clusters.
Language | Header Files |
---|---|
Fortran 77 |
|
Fortran 90 |
|
Fortran 95 |
|
C |
|
C++ |
|
Here are a few sample programs using MPI:
To see the available MPI libraries:
$ module avail openmpi
$ module avail impi
Language | Intel MPI | OpenMPI |
---|---|---|
Fortran 77 |
|
|
Fortran 90 |
|
|
Fortran 95 |
|
|
C |
|
|
C++ |
|
|
The Intel and GNU compilers will not output anything for a successful compilation. Also, the Intel compiler does not recognize the suffix ".f95".
Here is some more documentation from other sources on the MPI libraries:
Compiling OpenMP Programs
All compilers installed on Brown include OpenMP functionality for C, C++, and Fortran. An OpenMP program is a single process that takes advantage of a multi-core processor and its shared memory to achieve a form of parallel computing called multithreading. It distributes the work of a process over processor cores in a single compute node without the need for MPI communications.
Language | Header Files |
---|---|
Fortran 77 |
|
Fortran 90 |
|
Fortran 95 |
|
C |
|
C++ |
|
Sample programs illustrate task parallelism of OpenMP:
A sample program illustrates loop-level (data) parallelism of OpenMP:
To load a compiler, enter one of the following:
$ module load intel
$ module load gcc
Language | Intel Compiler | GNU Compiler |
---|---|---|
Fortran 77 |
|
|
Fortran 90 |
|
|
Fortran 95 |
|
|
C |
|
|
C++ |
|
|
The Intel and GNU compilers will not output anything for a successful compilation. Also, the Intel compiler does not recognize the suffix ".f95".
Here is some more documentation from other sources on OpenMP:
Compiling Hybrid Programs
A hybrid program combines both MPI and shared-memory to take advantage of compute clusters with multi-core compute nodes. Libraries for OpenMPI and Intel MPI (IMPI) and compilers which include OpenMP for C, C++, and Fortran are available.
Language | Header Files |
---|---|
Fortran 77 |
|
Fortran 90 |
|
Fortran 95 |
|
C |
|
C++ |
|
A few examples illustrate hybrid programs with task parallelism of OpenMP:
This example illustrates a hybrid program with loop-level (data) parallelism of OpenMP:
To see the available MPI libraries:
$ module avail impi
$ module avail openmpi
The following tables illustrate how to compile your hybrid (MPI/OpenMP) program. Any compiler flags accepted by Intel ifort/icc compilers are compatible with their respective MPI compiler.
Language | Command |
---|---|
Fortran 77 |
|
Fortran 90 |
|
Fortran 95 |
|
C |
|
C++ |
|
Language | Command |
---|---|
Fortran 77 |
|
Fortran 90 |
|
Fortran 95 |
|
C |
|
C++ |
|
The Intel and GNU compilers will not output anything for a successful compilation. Also, the Intel compiler does not recognize the suffix .f95
.
Intel MKL Library
Intel Math Kernel Library (MKL) contains ScaLAPACK, LAPACK, Sparse Solver, BLAS, Sparse BLAS, CBLAS, GMP, FFTs, DFTs, VSL, VML, and Interval Arithmetic routines. MKL resides in the directory stored in the environment variable MKL_HOME, after loading a version of the Intel compiler with module.
By using module load to load an Intel compiler your environment will have several variables set up to help link applications with MKL. Here are some example combinations of simplified linking options:
$ module load intel
$ echo $LINK_LAPACK
-L${MKL_HOME}/lib/intel64 -lmkl_intel_lp64 -lmkl_intel_thread -lmkl_core -liomp5 -lpthread
$ echo $LINK_LAPACK95
-L${MKL_HOME}/lib/intel64 -lmkl_lapack95_lp64 -lmkl_blas95_lp64 -lmkl_intel_lp64 -lmkl_intel_thread -lmkl_core -liomp5 -lpthread
RCAC recommends you use the provided variables to define MKL linking options in your compiling procedures. The Intel compiler modules also provide two other environment variables, LINK_LAPACK_STATIC and LINK_LAPACK95_STATIC that you may use if you need to link MKL statically.
RCAC recommends that you use dynamic linking of libguide. If so, define LD_LIBRARY_PATH such that you are using the correct version of libguide at run time. If you use static linking of libguide, then:
- If you use the Intel compilers, link in the libguide version that comes with the compiler (use the -openmp option).
- If you do not use the Intel compilers, link in the libguide version that comes with the Intel MKL above.
Here are some more documentation from other sources on the Intel MKL:
Running Jobs
There is one method for submitting jobs to Negishi. You may use SLURM to submit jobs to a partition on Negishi. SLURM performs job scheduling. Jobs may be any type of program. You may use either the batch or interactive mode to run your jobs. Use the batch mode for finished programs; use the interactive mode only for debugging.
In this section, you'll find a few pages describing the basics of creating and submitting SLURM jobs. As well, a number of example SLURM jobs that you may be able to adapt to your own needs.
Basics of SLURM Jobs
The Simple Linux Utility for Resource Management (SLURM) is a system providing job scheduling and job management on compute clusters. With SLURM, a user requests resources and submits a job to a queue. The system will then take jobs from queues, allocate the necessary nodes, and execute them.
Do NOT run large, long, multi-threaded, parallel, or CPU-intensive jobs on a front-end login host. All users share the front-end hosts, and running anything but the smallest test job will negatively impact everyone's ability to use Negishi. Always use SLURM to submit your work as a job.
Link to section 'Submitting a Job' of 'Basics of SLURM Jobs' Submitting a Job
The main steps to submitting a job are:
Follow the links below for information on these steps, and other basic information about jobs. A number of example SLURM jobs are also available.
Queues
Link to section '"mylab" Queues' of 'Queues' "mylab" Queues
Negishi, as a community cluster, has one or more queues dedicated to and named after each partner who has purchased access to the cluster. These queues provide partners and their researchers with priority access to their portion of the cluster. Jobs in these queues are typically limited to 336 hours. The expectation is that any jobs submitted to your research lab queues will start within 4 hours, assuming the queue currently has enough capacity for the job (that is, your lab mates aren't using all of the cores currently).
Link to section 'Standby Queue' of 'Queues' Standby Queue
Additionally, community clusters provide a "standby" queue which is available to all cluster users. This "standby" queue allows users to utilize portions of the cluster that would otherwise be idle, but at a lower priority than partner-queue jobs, and with a relatively short time limit, to ensure "standby" jobs will not be able to tie up resources and prevent partner-queue jobs from running quickly. Jobs in standby are limited to 4 hours. There is no expectation of job start time. If the cluster is very busy with partner queue jobs, or you are requesting a very large job, jobs in standby may take hours or days to start.
Link to section 'Debug Queue' of 'Queues' Debug Queue
The debug queue allows you to quickly start small, short, interactive jobs in order to debug code, test programs, or test configurations. You are limited to one running job at a time in the queue, and you may run up to two compute nodes for 30 minutes. The expectation is that debug jobs should start within a couple of minutes, assuming all of its dedicated nodes are not taken by others.
Link to section 'List of Queues' of 'Queues' List of Queues
To see a list of all queues on Negishi that you may submit to, use the slist command
This lists each queue you can submit to, the number of nodes allocated to the queue, how many are available to run jobs, and the maximum walltime you may request. Options to the command will give more detailed information. This command can be used to get a general idea of how busy an individual queue is and how long you may have to wait for your job to start.
Job Submission Script
To submit work to a SLURM queue, you must first create a job submission file. This job submission file is essentially a simple shell script. It will set any required environment variables, load any necessary modules, create or modify files and directories, and run any applications that you need:
#!/bin/bash
# FILENAME: myjobsubmissionfile
# Loads Matlab and sets the application up
module load matlab
# Change to the directory from which you originally submitted this job.
cd $SLURM_SUBMIT_DIR
# Runs a Matlab script named 'myscript'
matlab -nodisplay -singleCompThread -r myscript
Once your script is prepared, you are ready to submit your job.
Link to section 'Job Script Environment Variables' of 'Job Submission Script' Job Script Environment Variables
Name | Description |
---|---|
SLURM_SUBMIT_DIR | Absolute path of the current working directory when you submitted this job |
SLURM_JOBID | Job ID number assigned to this job by the batch system |
SLURM_JOB_NAME | Job name supplied by the user |
SLURM_JOB_NODELIST | Names of nodes assigned to this job |
SLURM_CLUSTER_NAME | Name of the cluster executing the job |
SLURM_SUBMIT_HOST | Hostname of the system where you submitted this job |
SLURM_JOB_PARTITION | Name of the original queue to which you submitted this job |
Submitting a Job
Once you have a job submission file, you may submit this script to SLURM using the sbatch
command. SLURM will find, or wait for, available resources matching your request and run your job there.
To submit your job to one compute node:
$ sbatch --nodes=1 myjobsubmissionfile
Slurm uses the word 'Account' and the option '-A' to specify different batch queues. To submit your job to a specific queue:
$ sbatch --nodes=1 -A standby myjobsubmissionfile
By default, each job receives 30 minutes of wall time, or clock time. If you know that your job will not need more than a certain amount of time to run, request less than the maximum wall time, as this may allow your job to run sooner. To request the 1 hour and 30 minutes of wall time:
$ sbatch -t 1:30:00 --nodes=1 -A standby myjobsubmissionfile
The --nodes
value indicates how many compute nodes you would like for your job.
Each compute node in Negishi has 128 processor cores.
In some cases, you may want to request multiple nodes. To utilize multiple nodes, you will need to have a program or code that is specifically programmed to use multiple nodes such as with MPI. Simply requesting more nodes will not make your work go faster. Your code must support this ability.
To request 2 compute nodes:
$ sbatch --nodes=2 myjobsubmissionfile
By default, jobs on Negishi will share nodes with other jobs.
To submit a job using 1 compute node with 4 tasks, each using the default 1 core and 1 GPU per node:
$ sbatch --nodes=1 --ntasks=4 --gpus-per-node=1 myjobsubmissionfile
If more convenient, you may also specify any command line options to sbatch
from within your job submission file, using a special form of comment:
#!/bin/sh -l
# FILENAME: myjobsubmissionfile
#SBATCH -A myqueuename
#SBATCH --nodes=1
#SBATCH --time=1:30:00
#SBATCH --job-name myjobname
# Print the hostname of the compute node on which this job is running.
/bin/hostname
If an option is present in both your job submission file and on the command line, the option on the command line will take precedence.
After you submit your job with SBATCH
, it may wait in queue for minutes, hours, or even weeks. How long it takes for a job to start depends on the specific queue, the resources and time requested, and other jobs already waiting in that queue requested as well. It is impossible to say for sure when any given job will start. For best results, request no more resources than your job requires.
Once your job is submitted, you can monitor the job status, wait for the job to complete, and check the job output.
Checking Job Status
Once a job is submitted there are several commands you can use to monitor the progress of the job.
To see your jobs, use the squeue -u command and specify your username:
(Remember, in our SLURM environment a queue is referred to as an 'Account')
squeue -u myusername
JOBID ACCOUNT NAME USER ST TIME NODES NODELIST(REASON)
182792 standby job1 myusername R 20:19 1 a000
185841 standby job2 myusername R 20:19 1 a001
185844 standby job3 myusername R 20:18 1 a002
185847 standby job4 myusername R 20:18 1 a003
To retrieve useful information about your queued or running job, use the scontrol show job command with your job's ID number. The output should look similar to the following:
scontrol show job 3519
JobId=3519 JobName=t.sub
UserId=myusername GroupId=mygroup MCS_label=N/A
Priority=3 Nice=0 Account=(null) QOS=(null)
JobState=PENDING Reason=BeginTime Dependency=(null)
Requeue=1 Restarts=0 BatchFlag=1 Reboot=0 ExitCode=0:0
RunTime=00:00:00 TimeLimit=7-00:00:00 TimeMin=N/A
SubmitTime=2019-08-29T16:56:52 EligibleTime=2019-08-29T23:30:00
AccrueTime=Unknown
StartTime=2019-08-29T23:30:00 EndTime=2019-09-05T23:30:00 Deadline=N/A
PreemptTime=None SuspendTime=None SecsPreSuspend=0
LastSchedEval=2019-08-29T16:56:52
Partition=workq AllocNode:Sid=mack-fe00:54476
ReqNodeList=(null) ExcNodeList=(null)
NodeList=(null)
NumNodes=1 NumCPUs=2 NumTasks=2 CPUs/Task=1 ReqB:S:C:T=0:0:*:*
TRES=cpu=2,node=1,billing=2
Socks/Node=* NtasksPerN:B:S:C=0:0:*:* CoreSpec=*
MinCPUsNode=1 MinMemoryNode=0 MinTmpDiskNode=0
Features=(null) DelayBoot=00:00:00
OverSubscribe=OK Contiguous=0 Licenses=(null) Network=(null)
Command=/home/myusername/jobdir/myjobfile.sub
WorkDir=/home/myusername/jobdir
StdErr=/home/myusername/jobdir/slurm-3519.out
StdIn=/dev/null
StdOut=/home/myusername/jobdir/slurm-3519.out
Power=
There are several useful bits of information in this output.
JobState
lets you know if the job is Pending, Running, Completed, or Held.RunTime and TimeLimit
will show how long the job has run and its maximum time.SubmitTime
is when the job was submitted to the cluster.NumNodes
,NumCPUs
,NumTasks
andCPUs/Task
are the number of Nodes, CPUs, Tasks, and CPUs per Task are shown.WorkDir
is the job's working directory.StdOut
andStderr
are the locations of stdout and stderr of the job, respectively.Reason
will show why aPENDING
job isn't running. The above error says that it has been requested to start at a specific, later time.
Checking Job Output
Once a job is submitted, and has started, it will write its standard output and standard error to files that you can read.
SLURM catches output written to standard output and standard error - what would be printed to your screen if you ran your program interactively. Unless you specfied otherwise, SLURM will put the output in the directory where you submitted the job in a file named slurm-
followed by the job id
, with the extension out
. For example slurm-3509.out
. Note that both stdout and stderr will be written into the same file, unless you specify otherwise.
If your program writes its own output files, those files will be created as defined by the program. This may be in the directory where the program was run, or may be defined in a configuration or input file. You will need to check the documentation for your program for more details.
Link to section 'Redirecting Job Output' of 'Checking Job Output' Redirecting Job Output
It is possible to redirect job output to somewhere other than the default location with the --error
and --output
directives:
#!/bin/bash
#SBATCH --output=/home/myusername/joboutput/myjob.out
#SBATCH --error=/home/myusername/joboutput/myjob.out
# This job prints "Hello World" to output and exits
echo "Hello World"
Job Dependencies
Dependencies are an automated way of holding and releasing jobs. Jobs with a dependency are held until the condition is satisfied. Once the condition is satisfied jobs only then become eligible to run and must still queue as normal.
Job dependencies may be configured to ensure jobs start in a specified order. Jobs can be configured to run after other job state changes, such as when the job starts or the job ends.
These examples illustrate setting dependencies in several ways. Typically dependencies are set by capturing and using the job ID from the last job submitted.
To run a job after job myjobid has started:
sbatch --dependency=after:myjobid myjobsubmissionfile
To run a job after job myjobid ends without error:
sbatch --dependency=afterok:myjobid myjobsubmissionfile
To run a job after job myjobid ends with errors:
sbatch --dependency=afternotok:myjobid myjobsubmissionfile
To run a job after job myjobid ends with or without errors:
sbatch --dependency=afterany:myjobid myjobsubmissionfile
To set more complex dependencies on multiple jobs and conditions:
sbatch --dependency=after:myjobid1:myjobid2:myjobid3,afterok:myjobid4 myjobsubmissionfile
Holding a Job
Sometimes you may want to submit a job but not have it run just yet. You may be wanting to allow lab mates to cut in front of you in the queue - so hold the job until their jobs have started, and then release yours.
To place a hold on a job before it starts running, use the scontrol hold job command:
$ scontrol hold job myjobid
Once a job has started running it can not be placed on hold.
To release a hold on a job, use the scontrol release job command:
$ scontrol release job myjobid
You find the job ID using the squeue command as explained in the SLURM Job Status section.
Canceling a Job
To stop a job before it finishes or remove it from a queue, use the scancel command:
scancel myjobid
You find the job ID using the squeue command as explained in the SLURM Job Status section.
PBS to Slurm
This is a reference for the most common command, environment variables, and job specification options used by the workload management systems and their equivalents.
Quick Guide
This table lists the most common command, environment variables, and job specification options used by the workload management systems and their equivalents (adapted from http://www.schedmd.com/slurmdocs/rosetta.html).
User Commands | PBS/Torque | Slurm |
---|---|---|
Job submission | qsub [script_file] |
sbatch [script_file] |
Interactive Job | qsub -I |
sinteractive |
Job deletion | qdel [job_id] |
scancel [job_id] |
Job status (by job) | qstat [job_id] |
squeue [-j job_id] |
Job status (by user) | qstat -u [user_name] |
squeue [-u user_name] |
Job hold | qhold [job_id] |
scontrol hold [job_id] |
Job release | qrls [job_id] |
scontrol release [job_id] |
Queue info | qstat -Q |
squeue |
Queue access | qlist |
slist |
Node list | pbsnodes -l |
sinfo -N scontrol show nodes |
Cluster status | qstat -a |
sinfo |
GUI | xpbsmon |
sview |
Environment | PBS/Torque | Slurm |
Job ID | $PBS_JOBID |
$SLURM_JOB_ID |
Job Name | $PBS_JOBNAME |
$SLURM_JOB_NAME |
Job Queue/Account | $PBS_QUEUE |
$SLURM_JOB_ACCOUNT |
Submit Directory | $PBS_O_WORKDIR |
$SLURM_SUBMIT_DIR |
Submit Host | $PBS_O_HOST |
$SLURM_SUBMIT_HOST |
Number of nodes | $PBS_NUM_NODES |
$SLURM_JOB_NUM_NODES |
Number of Tasks | $PBS_NP |
$SLURM_NTASKS |
Number of Tasks Per Node | $PBS_NUM_PPN |
$SLURM_NTASKS_PER_NODE |
Node List (Compact) | n/a | $SLURM_JOB_NODELIST |
Node List (One Core Per Line) | LIST=$(cat $PBS_NODEFILE) |
LIST=$(srun hostname) |
Job Array Index | $PBS_ARRAYID |
$SLURM_ARRAY_TASK_ID |
Job Specification | PBS/Torque | Slurm |
Script directive | #PBS |
#SBATCH |
Queue | -q [queue] |
-A [queue] |
Node Count | -l nodes=[count] |
-N [min[-max]] |
CPU Count | -l ppn=[count] |
-n [count] Note: total, not per node |
Wall Clock Limit | -l walltime=[hh:mm:ss] |
-t [min] OR-t [hh:mm:ss] OR-t [days-hh:mm:ss] |
Standard Output FIle | -o [file_name] |
-o [file_name] |
Standard Error File | -e [file_name] |
-e [file_name] |
Combine stdout/err | -j oe (both to stdout) OR-j eo (both to stderr) |
(use -o without -e) |
Copy Environment | -V |
--export=[ALL | NONE | variables] Note: default behavior is ALL |
Copy Specific Environment Variable | -v myvar=somevalue |
--export=NONE,myvar=somevalue OR--export=ALL,myvar=somevalue |
Event Notification | -m abe |
--mail-type=[events] |
Email Address | -M [address] |
--mail-user=[address] |
Job Name | -N [name] |
--job-name=[name] |
Job Restart | -r [y|n] |
--requeue OR--no-requeue |
Working Directory | --workdir=[dir_name] |
|
Resource Sharing | -l naccesspolicy=singlejob |
--exclusive OR--shared |
Memory Size | -l mem=[MB] |
--mem=[mem][M|G|T] OR--mem-per-cpu=[mem][M|G|T] |
Account to charge | -A [account] |
-A [account] |
Tasks Per Node | -l ppn=[count] |
--tasks-per-node=[count] |
CPUs Per Task | --cpus-per-task=[count] |
|
Job Dependency | -W depend=[state:job_id] |
--depend=[state:job_id] |
Job Arrays | -t [array_spec] |
--array=[array_spec] |
Generic Resources | -l other=[resource_spec] |
--gres=[resource_spec] |
Licenses | --licenses=[license_spec] |
|
Begin Time | -A "y-m-d h:m:s" |
--begin=y-m-d[Th:m[:s]] |
See the official Slurm Documentation for further details.
Notable Differences
-
Separate commands for Batch and Interactive jobs
Unlike PBS, in Slurm interactive jobs and batch jobs are launched with completely distinct commands.
Usesbatch [allocation request options] script
to submit a job to the batch scheduler, andsinteractive [allocation request options]
to launch an interactive job. sinteractive accepts most of the same allocation request options as sbatch does. -
No need for
cd $PBS_O_WORKDIR
In Slurm your batch job starts to run in the directory from which you submitted the script whereas in PBS/Torque you need to explicitly move back to that directory with
cd $PBS_O_WORKDIR
. -
No need to manually export environment
The environment variables that are defined in your shell session at the time that you submit the script are exported into your batch job, whereas in PBS/Torque you need to use the
-V
flag to export your environment. -
Location of output files
The output and error files are created in their final location immediately that the job begins or an error is generated, whereas in PBS/Torque temporary files are created that are only moved to the final location at the end of the job. Therefore in Slurm you can examine the output and error files from your job during its execution.
See the official Slurm Documentation for further details.
Example Jobs
A number of example jobs are available for you to look over and adapt to your own needs. The first few are generic examples, and latter ones go into specifics for particular software packages.
Generic SLURM Jobs
The following examples demonstrate the basics of SLURM jobs, and are designed to cover common job request scenarios. These example jobs will need to be modified to run your application or code.
Simple Job
Every SLURM job consists of a job submission file. A job submission file contains a list of commands that run your program and a set of resource (nodes, walltime, queue) requests. The resource requests can appear in the job submission file or can be specified at submit-time as shown below.
This simple example submits the job submission file hello.sub to the standby queue on Negishi and requests a single node:
#!/bin/bash
# FILENAME: hello.sub
# Show this ran on a compute node by running the hostname command.
hostname
echo "Hello World"
sbatch -A standby --nodes=1 --ntasks=1 --cpus-per-task=1 --time=00:01:00 hello.sub
Submitted batch job 3521
For a real job you would replace echo "Hello World"
with a command, or sequence of commands, that run your program.
After your job finishes running, the ls command will show a new file in your directory, the .out file:
ls -l
hello.sub
slurm-3521.out
The file slurm-3521.out contains the output and errors your program would have written to the screen if you had typed its commands at a command prompt:
cat slurm-3521.out
a001.negishi.rcac.purdue.edu
Hello World
You should see the hostname of the compute node your job was executed on. Following should be the "Hello World" statement.
Multiple Node
In some cases, you may want to request multiple nodes. To utilize multiple nodes, you will need to have a program or code that is specifically programmed to use multiple nodes such as with MPI. Simply requesting more nodes will not make your work go faster. Your code must support this ability.
This example shows a request for multiple compute nodes. The job submission file contains a single command to show the names of the compute nodes allocated:
# FILENAME: myjobsubmissionfile.sub
#!/bin/bash
echo "$SLURM_JOB_NODELIST"
sbatch --nodes=2 --ntasks=256 --time=00:10:00 -A standby myjobsubmissionfile.sub
Compute nodes allocated:
a[014-015].negishi
The above example will allocate the total of 256 CPU cores across 2 nodes. Note that if your multi-node job requests fewer than each node's full 128 cores per node, by default Slurm provides no guarantee with respect to how this total is distributed between assigned nodes (i.e. the cores may not necessarily be split evenly). If you need specific arrangements of your tasks and cores, you can use --cpus-per-task= and/or --ntasks-per-node= flags. See Slurm documentation or man sbatch for more options.
Directives
So far these examples have shown submitting jobs with the resource requests on the sbatch
command line such as:
sbatch -A standby --nodes=1 --time=00:01:00 hello.sub
The resource requests can also be put into job submission file itself. Documenting the resource requests in the job submission is desirable because the job can be easily reproduced later. Details left in your command history are quickly lost. Arguments are specified with the #SBATCH
syntax:
#!/bin/bash
# FILENAME: hello.sub
#SBATCH -A standby
#SBATCH --nodes=1 --time=00:01:00
# Show this ran on a compute node by running the hostname command.
hostname
echo "Hello World"
The #SBATCH
directives must appear at the top of your submission file. SLURM will stop parsing directives as soon as it encounters a line that does not start with '#'. If you insert a directive in the middle of your script, it will be ignored.
This job can be then submitted with:
sbatch hello.sub
Specific Types of Nodes
SLURM allows running a job on specific types of compute nodes to accommodate special hardware requirements (e.g. a certain CPU or GPU type, etc.)
Cluster nodes have a set of descriptive features assigned to them, and users can specify which of these features are required by their job by using the constraint option at submission time. Only nodes having features matching the job constraints will be used to satisfy the request.
Example: a job requires a compute node in an "A" sub-cluster:
sbatch --nodes=1 --ntasks=128 --constraint=A myjobsubmissionfile.sub
Compute node allocated:
a003.negishi
Feature constraints can be used for both batch and interactive jobs, as well as for individual job steps inside a job. Multiple constraints can be specified with a predefined syntax to achieve complex request logic (see detailed description of the '--constraint' option in man sbatch
or online Slurm documentation).
Refer to Detailed Hardware Specification section for list of available sub-cluster labels, their respective per-node memory sizes and other hardware details. You could also use sfeatures
command to list available constraint feature names for different node types.
Interactive Jobs
Interactive jobs are run on compute nodes, while giving you a shell to interact with. They give you the ability to type commands or use a graphical interface in the same way as if you were on a front-end login host.
To submit an interactive job, use sinteractive to run a login shell on allocated resources.
sinteractive accepts most of the same resource requests as sbatch, so to request a login shell on the cpu account while allocating 2 nodes and 128 total cores, you might do:
sinteractive -A cpu -N2 -n256
To quit your interactive job:
exit
or Ctrl-D
The above example will allocate the total of 256 CPU cores across 2 nodes. Note that if your multi-node job requests fewer than each node's full 128 cores per node, by default Slurm provides no guarantee with respect to how this total is distributed between assigned nodes (i.e. the cores may not necessarily be split evenly). If you need specific arrangements of your tasks and cores, you can use --cpus-per-task= and/or --ntasks-per-node= flags. See Slurm documentation or man salloc for more options.
Serial Jobs
This shows how to submit one of the serial programs compiled in the section Compiling Serial Programs.
Create a job submission file:
#!/bin/bash
# FILENAME: serial_hello.sub
./serial_hello
Submit the job:
sbatch --nodes=1 --ntasks=1 --time=00:01:00 serial_hello.sub
After the job completes, view results in the output file:
cat slurm-myjobid.out
Runhost:a009.negishi.rcac.purdue.edu
hello, world
If the job failed to run, then view error messages in the file slurm-myjobid.out.
OpenMP
A shared-memory job is a single process that takes advantage of a multi-core processor and its shared memory to achieve parallelization.
This example shows how to submit an OpenMP program compiled in the section Compiling OpenMP Programs.
When running OpenMP programs, all threads must be on the same compute node to take advantage of shared memory. The threads cannot communicate between nodes.
To run an OpenMP program, set the environment variable OMP_NUM_THREADS to the desired number of threads:
In csh:
setenv OMP_NUM_THREADS 128
In bash:
export OMP_NUM_THREADS=128
This should almost always be equal to the number of cores on a compute node. You may want to set to another appropriate value if you are running several processes in parallel in a single job or node.
Create a job submissionfile:
#!/bin/bash
# FILENAME: omp_hello.sub
#SBATCH --nodes=1
#SBATCH --ntasks=128
#SBATCH --time=00:01:00
export OMP_NUM_THREADS=128
./omp_hello
Submit the job:
sbatch omp_hello.sub
View the results from one of the sample OpenMP programs about task parallelism:
cat omp_hello.sub.omyjobid
SERIAL REGION: Runhost:a003.negishi.rcac.purdue.edu Thread:0 of 1 thread hello, world
PARALLEL REGION: Runhost:a003.negishi.rcac.purdue.edu Thread:0 of 128 threads hello, world
PARALLEL REGION: Runhost:a003.negishi.rcac.purdue.edu Thread:1 of 128 threads hello, world
...
If the job failed to run, then view error messages in the file slurm-myjobid.out.
If an OpenMP program uses a lot of memory and 128 threads use all of the memory of the compute node, use fewer processor cores (OpenMP threads) on that compute node.
MPI
An MPI job is a set of processes that take advantage of multiple compute nodes by communicating with each other. OpenMPI and Intel MPI (IMPI) are implementations of the MPI standard.
This section shows how to submit one of the MPI programs compiled in the section Compiling MPI Programs.
Use module load to set up the paths to access these libraries. Use module avail to see all MPI packages installed on Negishi.
Create a job submission file:
#!/bin/bash
# FILENAME: mpi_hello.sub
#SBATCH --nodes=2
#SBATCH --ntasks-per-node=128
#SBATCH --time=00:01:00
#SBATCH -A standby
srun -n 256 ./mpi_hello
SLURM can run an MPI program with the srun command. The number of processes is requested with the -n option. If you do not specify the -n option, it will default to the total number of processor cores you request from SLURM.
If the code is built with OpenMPI, it can be run with a simple srun -n command. If it is built with Intel IMPI, then you also need to add the --mpi=pmi2 option: srun --mpi=pmi2 -n 256 ./mpi_hello in this example.
Submit the MPI job:
sbatch ./mpi_hello.sub
View results in the output file:
cat slurm-myjobid.out
Runhost:a010.negishi.rcac.purdue.edu Rank:0 of 256 ranks hello, world
Runhost:a010.negishi.rcac.purdue.edu Rank:1 of 256 ranks hello, world
...
Runhost:a011.negishi.rcac.purdue.edu Rank:128 of 256 ranks hello, world
Runhost:a011.negishi.rcac.purdue.edu Rank:129 of 256 ranks hello, world
...
If the job failed to run, then view error messages in the output file.
If an MPI job uses a lot of memory and 128 MPI ranks per compute node use all of the memory of the compute nodes, request more compute nodes, while keeping the total number of MPI ranks unchanged.
Submit the job with double the number of compute nodes and modify the resource request to halve the number of MPI ranks per compute node.
#!/bin/bash
# FILENAME: mpi_hello.sub
#SBATCH --nodes=4
#SBATCH --ntasks-per-node=64
#SBATCH -t 00:01:00
#SBATCH -A standby
srun -n 256 ./mpi_hello
sbatch ./mpi_hello.sub
View results in the output file:
cat slurm-myjobid.out
Runhost:a010.negishi.rcac.purdue.edu Rank:0 of 256 ranks hello, world
Runhost:a010.negishi.rcac.purdue.edu Rank:1 of 256 ranks hello, world
...
Runhost:a011.negishi.rcac.purdue.edu Rank:64 of 256 ranks hello, world
...
Runhost:a012.negishi.rcac.purdue.edu Rank:128 of 256 ranks hello, world
...
Runhost:a013.negishi.rcac.purdue.edu Rank:192 of 256 ranks hello, world
...
Notes
- Use slist to determine which queues (
--account
or-A
option) are available to you. The name of the queue which is available to everyone on Negishi is "standby". - Invoking an MPI program on Negishi with ./program is typically wrong, since this will use only one MPI process and defeat the purpose of using MPI. Unless that is what you want (rarely the case), you should use srun or mpiexec to invoke an MPI program.
- In general, the exact order in which MPI ranks output similar write requests to an output file is random.
Link to section 'Collecting System Resource Utilization Data' of 'Monitoring Resources' Collecting System Resource Utilization Data
Knowing the precise resource utilization an application had during a job, such as CPU load or memory, can be incredibly useful. This is especially the case when the application isn't performing as expected.
One approach is to run a program like htop
during an interactive job and keep an eye on system resources. You can get precise time-series data from nodes associated with your job using XDmod as well, online. But these methods don't gather telemetry in an automated fashion, nor do they give you control over the resolution or format of the data.
As a matter of course, a robust implementation of some HPC workload would include resource utilization data as a diagnostic tool in the event of some failure.
The monitor
utility is a simple command line system resource monitoring tool for gathering such telemetry and is available as a module.
module load monitor
Complete documentation is available online at resource-monitor.readthedocs.io. A full manual page is also available for reference, man monitor
.
In the context of a SLURM job you will need to put this monitoring task in the background to allow the rest of your job script to proceed. Be sure to interrupt these tasks at the end of your job.
#!/bin/bash
# FILENAME: monitored_job.sh
module load monitor
# track per-code CPU load
monitor cpu percent --all-cores >cpu-percent.log &
CPU_PID=$!
# track memory usage
monitor cpu memory >cpu-memory.log &
MEM_PID=$!
# your code here
# shut down the resource monitors
kill -s INT $CPU_PID $MEM_PID
A particularly elegant solution would be to include such tools in your prologue script and have the tear down in your epilogue script.
For large distributed jobs spread across multiple nodes, mpiexec
can be used to gather telemetry from all nodes in the job. The hostname is included in each line of output so that data can be grouped as such. A concise way of constructing the needed list of hostnames in SLURM is to simply use srun hostname | sort -u
.
#!/bin/bash
# FILENAME: monitored_job.sh
module load monitor
# track all CPUs (one monitor per host)
mpiexec -machinefile <(srun hostname | sort -u) \
monitor cpu percent --all-cores >cpu-percent.log &
CPU_PID=$!
# track memory on all hosts (one monitor per host)
mpiexec -machinefile <(srun hostname | sort -u) \
monitor cpu memory >cpu-memory.log &
MEM_PID=$!
# your code here
# shut down the resource monitors
kill -s INT $CPU_PID $MEM_PID
To get resource data in a more readily computable format, the monitor
program can be told to output in CSV format with the --csv
flag.
monitor cpu memory --csv >cpu-memory.csv
For a distributed job you will need to suppress the header lines otherwise one will be created by each host.
monitor cpu memory --csv | head -1 >cpu-memory.csv
mpiexec -machinefile <(srun hostname | sort -u) \
monitor cpu memory --csv --no-header >>cpu-memory.csv
Specific Applications
The following examples demonstrate job submission files for some common real-world applications. See the Generic SLURM Examples section for more examples on job submissions that can be adapted for use.
Gaussian
Gaussian is a computational chemistry software package which works on electronic structure. This section illustrates how to submit a small Gaussian job to a Slurm queue. This Gaussian example runs the Fletcher-Powell multivariable optimization.
Prepare a Gaussian input file with an appropriate filename, here named myjob.com. The final blank line is necessary:
#P TEST OPT=FP STO-3G OPTCYC=2
STO-3G FLETCHER-POWELL OPTIMIZATION OF WATER
0 1
O
H 1 R
H 1 R 2 A
R 0.96
A 104.
To submit this job, load Gaussian then run the provided script, named subg16. This job uses one compute node with 128 processor cores:
module load gaussian16
subg16 myjob -N 1 -n 128
View job status:
squeue -u myusername
View results in the file for Gaussian output, here named myjob.log. Only the first and last few lines appear here:
Entering Gaussian System, Link 0=/apps/cent7/gaussian/g16-A.03/g16-haswell/g16/g16
Initial command:
/apps/cent7/gaussian/g16-A.03/g16-haswell/g16/l1.exe /scratch/negishi/myusername/gaussian/Gau-7781.inp -scrdir=/scratch/negishi/myusername/gaussian/
Entering Link 1 = /apps/cent7/gaussian/g16-A.03/g16-haswell/g16/l1.exe PID= 7782.
Copyright (c) 1988,1990,1992,1993,1995,1998,2003,2009,2016,
Gaussian, Inc. All Rights Reserved.
.
.
.
Job cpu time: 0 days 0 hours 3 minutes 28.2 seconds.
Elapsed time: 0 days 0 hours 0 minutes 12.9 seconds.
File lengths (MBytes): RWF= 17 Int= 0 D2E= 0 Chk= 2 Scr= 2
Normal termination of Gaussian 16 at Tue May 1 17:12:00 2018.
real 13.85
user 202.05
sys 6.12
Machine:
a012.negishi.rcac.purdue.edu
a012.negishi.rcac.purdue.edu
a012.negishi.rcac.purdue.edu
a012.negishi.rcac.purdue.edu
a012.negishi.rcac.purdue.edu
a012.negishi.rcac.purdue.edu
a012.negishi.rcac.purdue.edu
a012.negishi.rcac.purdue.edu
Link to section 'Examples of Gaussian SLURM Job Submissions' of 'Gaussian' Examples of Gaussian SLURM Job Submissions
Submit job using 128 processor cores on a single node:
subg16 myjob -N 1 -n 128 -t 200:00:00 -A myqueuename
Submit job using 128 processor cores on each of 2 nodes:
subg16 myjob -N 2 --ntasks-per-node=128 -t 200:00:00 -A myqueuename
To submit a bash job, a submit script sample looks like:
#!/bin/bash
#SBATCH -A myqueuename # Queue name(use 'slist' command to find queues' name)
#SBATCH --nodes=1 # Total # of nodes
#SBATCH --ntasks=64 # Total # of MPI tasks
#SBATCH --time=1:00:00 # Total run time limit (hh:mm:ss)
#SBATCH -J myjobname # Job name
#SBATCH -o myjob.o%j # Name of stdout output file
#SBATCH -e myjob.e%j # Name of stderr error file
module load gaussian16
g16 < myjob.com
For more information about Gaussian:
Matlab
MATLAB® (MATrix LABoratory) is a high-level language and interactive environment for numerical computation, visualization, and programming. MATLAB is a product of MathWorks.
MATLAB, Simulink, Compiler, and several of the optional toolboxes are available to faculty, staff, and students. To see the kind and quantity of all MATLAB licenses plus the number that you are currently using you can use the matlab_licenses command:
$ module load matlab
$ matlab_licenses
The MATLAB client can be run in the front-end for application development, however, computationally intensive jobs must be run on compute nodes.
The following sections provide several examples illustrating how to submit MATLAB jobs to a Linux compute cluster.
Matlab Script (.m File)
This section illustrates how to submit a small, serial, MATLAB program as a job to a batch queue. This MATLAB program prints the name of the run host and gets three random numbers.
Prepare a MATLAB script myscript.m, and a MATLAB function file myfunction.m:
% FILENAME: myscript.m
% Display name of compute node which ran this job.
[c name] = system('hostname');
fprintf('\n\nhostname:%s\n', name);
% Display three random numbers.
A = rand(1,3);
fprintf('%f %f %f\n', A);
quit;
% FILENAME: myfunction.m
function result = myfunction ()
% Return name of compute node which ran this job.
[c name] = system('hostname');
result = sprintf('hostname:%s', name);
% Return three random numbers.
A = rand(1,3);
r = sprintf('%f %f %f', A);
result=strvcat(result,r);
end
Also, prepare a job submission file, here named myjob.sub. Run with the name of the script:
#!/bin/bash
# FILENAME: myjob.sub
echo "myjob.sub"
# Load module, and set up environment for Matlab to run
module load matlab
unset DISPLAY
# -nodisplay: run MATLAB in text mode; X11 server not needed
# -singleCompThread: turn off implicit parallelism
# -r: read MATLAB program; use MATLAB JIT Accelerator
# Run Matlab, with the above options and specifying our .m file
matlab -nodisplay -singleCompThread -r myscript
myjob.sub
< M A T L A B (R) >
Copyright 1984-2011 The MathWorks, Inc.
R2011b (7.13.0.564) 64-bit (glnxa64)
August 13, 2011
To get started, type one of these: helpwin, helpdesk, or demo.
For product information, visit www.mathworks.com.
hostname:a001.negishi.rcac.purdue.edu
0.814724 0.905792 0.126987
Output shows that a processor core on one compute node (a001) processed the job. Output also displays the three random numbers.
For more information about MATLAB:
Implicit Parallelism
MATLAB implements implicit parallelism which is automatic multithreading of many computations, such as matrix multiplication, linear algebra, and performing the same operation on a set of numbers. This is different from the explicit parallelism of the Parallel Computing Toolbox.
MATLAB offers implicit parallelism in the form of thread-parallel enabled functions. Since these processor cores, or threads, share a common memory, many MATLAB functions contain multithreading potential. Vector operations, the particular application or algorithm, and the amount of computation (array size) contribute to the determination of whether a function runs serially or with multithreading.
When your job triggers implicit parallelism, it attempts to allocate its threads on all processor cores of the compute node on which the MATLAB client is running, including processor cores running other jobs. This competition can degrade the performance of all jobs running on the node.
When you know that you are coding a serial job but are unsure whether you are using thread-parallel enabled operations, run MATLAB with implicit parallelism turned off. Beginning with the R2009b, you can turn multithreading off by starting MATLAB with -singleCompThread:
$ matlab -nodisplay -singleCompThread -r mymatlabprogram
When you are using implicit parallelism, make sure you request exclusive access to a compute node, as MATLAB has no facility for sharing nodes.
For more information about MATLAB's implicit parallelism:
Profile Manager
MATLAB offers two kinds of profiles for parallel execution: the 'local' profile and user-defined cluster profiles. The 'local' profile runs a MATLAB job on the processor core(s) of the same compute node, or front-end, that is running the client. To run a MATLAB job on compute node(s) different from the node running the client, you must define a Cluster Profile using the Cluster Profile Manager.
To prepare a user-defined cluster profile, use the Cluster Profile Manager in the Parallel menu. This profile contains the scheduler details (queue, nodes, processors, walltime, etc.) of your job submission. Ultimately, your cluster profile will be an argument to MATLAB functions like batch().
For your convenience, a generic cluster profile is provided that can be downloaded: myslurmprofile.settings
Please note that modifications are very likely to be required to make myslurmprofile.settings work. You may need to change values for number of nodes, number of workers, walltime, and submission queue specified in the file. As well, the generic profile itself depends on the particular job scheduler on the cluster, so you may need to download or create two or more generic profiles under different names. Each time you run a job using a Cluster Profile, make sure the specific profile you are using is appropriate for the job and the cluster.
To import the profile, start a MATLAB session and select Manage Cluster Profiles... from the Parallel menu. In the Cluster Profile Manager, select Import, navigate to the folder containing the profile, select myslurmprofile.settings and click OK. Remember that the profile will need to be customized for your specific needs. If you have any questions, please contact us.
For detailed information about MATLAB's Parallel Computing Toolbox, examples, demos, and tutorials:
Parallel Computing Toolbox (parfor)
The MATLAB Parallel Computing Toolbox (PCT) extends the MATLAB language with high-level, parallel-processing features such as parallel for loops, parallel regions, message passing, distributed arrays, and parallel numerical methods. It offers a shared-memory computing environment running on the local cluster profile in addition to your MATLAB client. Moreover, the MATLAB Distributed Computing Server (DCS) scales PCT applications up to the limit of your DCS licenses.
This section illustrates the fine-grained parallelism of a parallel for loop (parfor) in a pool job.
The following examples illustrate a method for submitting a small, parallel, MATLAB program with a parallel loop (parfor statement) as a job to a queue. This MATLAB program prints the name of the run host and shows the values of variables numlabs and labindex for each iteration of the parfor loop.
This method uses the job submission command to submit a MATLAB client which calls the MATLAB batch() function with a user-defined cluster profile.
Prepare a MATLAB pool program in a MATLAB script with an appropriate filename, here named myscript.m:
% FILENAME: myscript.m
% SERIAL REGION
[c name] = system('hostname');
fprintf('SERIAL REGION: hostname:%s\n', name)
numlabs = parpool('poolsize');
fprintf(' hostname numlabs labindex iteration\n')
fprintf(' ------------------------------- ------- -------- ---------\n')
tic;
% PARALLEL LOOP
parfor i = 1:8
[c name] = system('hostname');
name = name(1:length(name)-1);
fprintf('PARALLEL LOOP: %-31s %7d %8d %9d\n', name,numlabs,labindex,i)
pause(2);
end
% SERIAL REGION
elapsed_time = toc; % get elapsed time in parallel loop
fprintf('\n')
[c name] = system('hostname');
name = name(1:length(name)-1);
fprintf('SERIAL REGION: hostname:%s\n', name)
fprintf('Elapsed time in parallel loop: %f\n', elapsed_time)
The execution of a pool job starts with a worker executing the statements of the first serial region up to the parfor block, when it pauses. A set of workers (the pool) executes the parfor block. When they finish, the first worker resumes by executing the second serial region. The code displays the names of the compute nodes running the batch session and the worker pool.
Prepare a MATLAB script that calls MATLAB function batch() which makes a four-lab pool on which to run the MATLAB code in the file myscript.m. Use an appropriate filename, here named mylclbatch.m:
% FILENAME: mylclbatch.m
!echo "mylclbatch.m"
!hostname
pjob=batch('myscript','Profile','myslurmprofile','Pool',4,'CaptureDiary',true);
wait(pjob);
diary(pjob);
quit;
Prepare a job submission file with an appropriate filename, here named myjob.sub:
#!/bin/bash
# FILENAME: myjob.sub
echo "myjob.sub"
hostname
module load matlab
unset DISPLAY
matlab -nodisplay -r mylclbatch
Submit the job as a single compute node with one processor core.
One processor core runs myjob.sub and mylclbatch.m.
Once this job starts, a second job submission is made.
myjob.sub
< M A T L A B (R) >
Copyright 1984-2013 The MathWorks, Inc.
R2013a (8.1.0.604) 64-bit (glnxa64)
February 15, 2013
To get started, type one of these: helpwin, helpdesk, or demo.
For product information, visit www.mathworks.com.
mylclbatch.ma000.negishi.rcac.purdue.edu
SERIAL REGION: hostname:a000.negishi.rcac.purdue.edu
hostname numlabs labindex iteration
------------------------------- ------- -------- ---------
PARALLEL LOOP: a001.negishi.rcac.purdue.edu 4 1 2
PARALLEL LOOP: a002.negishi.rcac.purdue.edu 4 1 4
PARALLEL LOOP: a001.negishi.rcac.purdue.edu 4 1 5
PARALLEL LOOP: a002.negishi.rcac.purdue.edu 4 1 6
PARALLEL LOOP: a003.negishi.rcac.purdue.edu 4 1 1
PARALLEL LOOP: a003.negishi.rcac.purdue.edu 4 1 3
PARALLEL LOOP: a004.negishi.rcac.purdue.edu 4 1 7
PARALLEL LOOP: a004.negishi.rcac.purdue.edu 4 1 8
SERIAL REGION: hostname:a001.negishi.rcac.purdue.edu
Elapsed time in parallel loop: 5.411486
To scale up this method to handle a real application, increase the wall time in the submission command to accommodate a longer running job. Secondly, increase the wall time of myslurmprofile by using the Cluster Profile Manager in the Parallel menu to enter a new wall time in the property SubmitArguments.
For more information about MATLAB Parallel Computing Toolbox:
Parallel Toolbox (spmd)
The MATLAB Parallel Computing Toolbox (PCT) extends the MATLAB language with high-level, parallel-processing features such as parallel for loops, parallel regions, message passing, distributed arrays, and parallel numerical methods. It offers a shared-memory computing environment with a maximum of eight MATLAB workers (labs, threads; versions R2009a) and 12 workers (labs, threads; version R2011a) running on the local configuration in addition to your MATLAB client. Moreover, the MATLAB Distributed Computing Server (DCS) scales PCT applications up to the limit of your DCS licenses.
This section illustrates how to submit a small, parallel, MATLAB program with a parallel region (spmd statement) as a MATLAB pool job to a batch queue.
This example uses the submission command to submit to compute nodes a MATLAB client which interprets a Matlab .m with a user-defined cluster profile which scatters the MATLAB workers onto different compute nodes. This method uses the MATLAB interpreter, the Parallel Computing Toolbox, and the Distributed Computing Server; so, it requires and checks out six licenses: one MATLAB license for the client running on the compute node, one PCT license, and four DCS licenses. Four DCS licenses run the four copies of the spmd statement. This job is completely off the front end.
Prepare a MATLAB script called myscript.m:
% FILENAME: myscript.m
% SERIAL REGION
[c name] = system('hostname');
fprintf('SERIAL REGION: hostname:%s\n', name)
p = parpool('4');
fprintf(' hostname numlabs labindex\n')
fprintf(' ------------------------------- ------- --------\n')
tic;
% PARALLEL REGION
spmd
[c name] = system('hostname');
name = name(1:length(name)-1);
fprintf('PARALLEL REGION: %-31s %7d %8d\n', name,numlabs,labindex)
pause(2);
end
% SERIAL REGION
elapsed_time = toc; % get elapsed time in parallel region
delete(p);
fprintf('\n')
[c name] = system('hostname');
name = name(1:length(name)-1);
fprintf('SERIAL REGION: hostname:%s\n', name)
fprintf('Elapsed time in parallel region: %f\n', elapsed_time)
quit;
Prepare a job submission file with an appropriate filename, here named myjob.sub. Run with the name of the script:
#!/bin/bash
# FILENAME: myjob.sub
echo "myjob.sub"
module load matlab
unset DISPLAY
matlab -nodisplay -r myscript
Run MATLAB to set the default parallel configuration to your job configuration:
$ matlab -nodisplay
>> parallel.defaultClusterProfile('myslurmprofile');
>> quit;
$
Once this job starts, a second job submission is made.
myjob.sub
< M A T L A B (R) >
Copyright 1984-2011 The MathWorks, Inc.
R2011b (7.13.0.564) 64-bit (glnxa64)
August 13, 2011
To get started, type one of these: helpwin, helpdesk, or demo.
For product information, visit www.mathworks.com.
SERIAL REGION: hostname:a001.negishi.rcac.purdue.edu
Starting matlabpool using the 'myslurmprofile' profile ... connected to 4 labs.
hostname numlabs labindex
------------------------------- ------- --------
Lab 2:
PARALLEL REGION: a002.negishi.rcac.purdue.edu 4 2
Lab 1:
PARALLEL REGION: a001.negishi.rcac.purdue.edu 4 1
Lab 3:
PARALLEL REGION: a003.negishi.rcac.purdue.edu 4 3
Lab 4:
PARALLEL REGION: a004.negishi.rcac.purdue.edu 4 4
Sending a stop signal to all the labs ... stopped.
SERIAL REGION: hostname:a001.negishi.rcac.purdue.edu
Elapsed time in parallel region: 3.382151
Output shows the name of one compute node (a001) that processed the job submission file myjob.sub and the two serial regions. The job submission scattered four processor cores (four MATLAB labs) among four different compute nodes (a001,a002,a003,a004) that processed the four parallel regions. The total elapsed time demonstrates that the jobs ran in parallel.
For more information about MATLAB Parallel Computing Toolbox:
Distributed Computing Server (parallel job)
The MATLAB Parallel Computing Toolbox (PCT) enables a parallel job via the MATLAB Distributed Computing Server (DCS). The tasks of a parallel job are identical, run simultaneously on several MATLAB workers (labs), and communicate with each other. This section illustrates an MPI-like program.
This section illustrates how to submit a small, MATLAB parallel job with four workers running one MPI-like task to a batch queue. The MATLAB program broadcasts an integer to four workers and gathers the names of the compute nodes running the workers and the lab IDs of the workers.
This example uses the job submission command to submit a Matlab script with a user-defined cluster profile which scatters the MATLAB workers onto different compute nodes. This method uses the MATLAB interpreter, the Parallel Computing Toolbox, and the Distributed Computing Server; so, it requires and checks out six licenses: one MATLAB license for the client running on the compute node, one PCT license, and four DCS licenses. Four DCS licenses run the four copies of the parallel job. This job is completely off the front end.
Prepare a MATLAB script named myscript.m :
% FILENAME: myscript.m
% Specify pool size.
% Convert the parallel job to a pool job.
parpool('4');
spmd
if labindex == 1
% Lab (rank) #1 broadcasts an integer value to other labs (ranks).
N = labBroadcast(1,int64(1000));
else
% Each lab (rank) receives the broadcast value from lab (rank) #1.
N = labBroadcast(1);
end
% Form a string with host name, total number of labs, lab ID, and broadcast value.
[c name] =system('hostname');
name = name(1:length(name)-1);
fmt = num2str(floor(log10(numlabs))+1);
str = sprintf(['%s:%d:%' fmt 'd:%d '], name,numlabs,labindex,N);
% Apply global concatenate to all str's.
% Store the concatenation of str's in the first dimension (row) and on lab #1.
result = gcat(str,1,1);
if labindex == 1
disp(result)
end
end % spmd
matlabpool close force;
quit;
Also, prepare a job submission, here named myjob.sub. Run with the name of the script:
# FILENAME: myjob.sub
echo "myjob.sub"
module load matlab
unset DISPLAY
# -nodisplay: run MATLAB in text mode; X11 server not needed
# -r: read MATLAB program; use MATLAB JIT Accelerator
matlab -nodisplay -r myscript
Run MATLAB to set the default parallel configuration to your appropriate Profile:
$ matlab -nodisplay
>> defaultParallelConfig('myslurmprofile');
>> quit;
$
Submit the job as a single compute node with one processor core.
Once this job starts, a second job submission is made.
myjob.sub
< M A T L A B (R) >
Copyright 1984-2011 The MathWorks, Inc.
R2011b (7.13.0.564) 64-bit (glnxa64)
August 13, 2011
To get started, type one of these: helpwin, helpdesk, or demo.
For product information, visit www.mathworks.com.
>Starting matlabpool using the 'myslurmprofile' configuration ... connected to 4 labs.
Lab 1:
a006.negishi.rcac.purdue.edu:4:1:1000
a007.negishi.rcac.purdue.edu:4:2:1000
a008.negishi.rcac.purdue.edu:4:3:1000
a009.negishi.rcac.purdue.edu:4:4:1000
Sending a stop signal to all the labs ... stopped.
Did not find any pre-existing parallel jobs created by matlabpool.
Output shows the name of one compute node (a006) that processed the job submission file myjob.sub. The job submission scattered four processor cores (four MATLAB labs) among four different compute nodes (a006,a007,a008,a009) that processed the four parallel regions.
To scale up this method to handle a real application, increase the wall time in the submission command to accommodate a longer running job. Secondly, increase the wall time of myslurmprofile by using the Cluster Profile Manager in the Parallel menu to enter a new wall time in the property SubmitArguments.
For more information about parallel jobs:
Python
Notice: Python 2.7 has reached end-of-life on Jan 1, 2020 (announcement). Please update your codes and your job scripts to use Python 3.
Python is a high-level, general-purpose, interpreted, dynamic programming language. We suggest using Anaconda which is a Python distribution made for large-scale data processing, predictive analytics, and scientific computing. For example, to use the default Anaconda distribution:
$ module load conda
For a full list of available Anaconda and Python modules enter:
$ module spider conda
Example Python Jobs
This section illustrates how to submit a small Python job to a SLURM queue.
Link to section 'Example 1: Hello world' of 'Example Python Jobs' Example 1: Hello world
Prepare a Python input file with an appropriate filename, here named hello.py
:
# FILENAME: hello.py
import string, sys
print("Hello, world!")
Prepare a job submission file with an appropriate filename, here named myjob.sub:
#!/bin/bash
# FILENAME: myjob.sub
module load conda
python hello.py
Hello, world!
Link to section 'Example 2: Matrix multiply' of 'Example Python Jobs' Example 2: Matrix multiply
Save the following script as matrix.py:
# Matrix multiplication program
x = [[3,1,4],[1,5,9],[2,6,5]]
y = [[3,5,8,9],[7,9,3,2],[3,8,4,6]]
result = [[sum(a*b for a,b in zip(x_row,y_col)) for y_col in zip(*y)] for x_row in x]
for r in result:
print(r)
Change the last line in the job submission file above to read:
python matrix.py
The standard output file from this job will result in the following matrix:
[28, 56, 43, 53]
[65, 122, 59, 73]
[63, 104, 54, 60]
Link to section 'Example 3: Sine wave plot using numpy and matplotlib packages' of 'Example Python Jobs' Example 3: Sine wave plot using numpy and matplotlib packages
Save the following script as sine.py:
import numpy as np
import matplotlib
matplotlib.use('Agg')
import matplotlib.pyplot as plt
x = np.linspace(-np.pi, np.pi, 201)
plt.plot(x, np.sin(x))
plt.xlabel('Angle [rad]')
plt.ylabel('sin(x)')
plt.axis('tight')
plt.savefig('sine.png')
Change your job submission file to submit this script and the job will output a png file and blank standard output and error files.
For more information about Python:
Managing Environments with Conda
Conda is a package manager in Anaconda that allows you to create and manage multiple environments where you can pick and choose which packages you want to use. To use Conda you must load an Anaconda module:
$ module load conda
Many packages are pre-installed in the global environment. To see these packages:
$ conda list
To create your own custom environment:
$ conda create --name MyEnvName python=3.8 FirstPackageName SecondPackageName -y
The --name option specifies that the environment created will be named MyEnvName. You can include as many packages as you require separated by a space. Including the -y option lets you skip the prompt to install the package. By default environments are created and stored in the $HOME/.conda directory.
To create an environment at a custom location:
$ conda create --prefix=$HOME/MyEnvName python=3.8 PackageName -y
To see a list of your environments:
$ conda env list
To remove unwanted environments:
$ conda remove --name MyEnvName --all
To add packages to your environment:
$ conda install --name MyEnvName PackageNames
To remove a package from an environment:
$ conda remove --name MyEnvName PackageName
Installing packages when creating your environment, instead of one at a time, will help you avoid dependency issues.
To activate or deactivate an environment you have created:
$ source activate MyEnvName
$ source deactivate MyEnvName
If you created your conda environment at a custom location using --prefix option, then you can activate or deactivate it using the full path.
$ source activate $HOME/MyEnvName
$ source deactivate $HOME/MyEnvName
To use a custom environment inside a job you must load the module and activate the environment inside your job submission script. Add the following lines to your submission script:
$ module load conda
$ source activate MyEnvName
For more information about Python:
Managing Packages with Pip
Pip is a Python package manager. Many Python package documentation provide pip
instructions that result in permission errors because by default pip
will install in a system-wide location and fail.
Exception:
Traceback (most recent call last):
... ... stack trace ... ...
OSError: [Errno 13] Permission denied: '/apps/cent7/anaconda/2020.07-py38/lib/python3.8/site-packages/mkl_random-1.1.1.dist-info'
If you encounter this error, it means that you cannot modify the global Python installation. We recommend installing Python packages in a conda environment. Detailed instructions for installing packages with pip can be found in our Python package installation page.
Below we list some other useful pip commands.
- Search for a package in PyPI channels:
$ pip search packageName
- Check which packages are installed globally:
$ pip list
- Check which packages you have personally installed:
$ pip list --user
- Snapshot installed packages:
$ pip freeze > requirements.txt
- You can install packages from a snapshot inside a new conda environment. Make sure to load the appropriate conda environment first.
$ pip install -r requirements.txt
For more information about Python:
Installing Packages
Installing Python packages in an Anaconda environment is recommended. One key advantage of Anaconda is that it allows users to install unrelated packages in separate self-contained environments. Individual packages can later be reinstalled or updated without impacting others. If you are unfamiliar with Conda environments, please check our Conda Guide.
To facilitate the process of creating and using Conda environments, we support a script (conda-env-mod) that generates a module file for an environment, as well as an optional Jupyter kernel to use this environment in a JupyterHub notebook.
You must load one of the anaconda modules in order to use this script.
$ module load conda
Step-by-step instructions for installing custom Python packages are presented below.
Link to section 'Step 1: Create a conda environment' of 'Installing Packages' Step 1: Create a conda environment
Users can use the conda-env-mod script to create an empty conda environment. This script needs either a name or a path for the desired environment. After the environment is created, it generates a module file for using it in future. Please note that conda-env-mod is different from the official conda-env script and supports a limited set of subcommands. Detailed instructions for using conda-env-mod can be found with the command conda-env-mod --help.
-
Example 1: Create a conda environment named mypackages in user's
$HOME
directory.$ conda-env-mod create -n mypackages
-
Example 2: Create a conda environment named mypackages at a custom location.
$ conda-env-mod create -p /depot/mylab/apps/mypackages
Please follow the on-screen instructions while the environment is being created. After finishing, the script will print the instructions to use this environment.
... ... ... Preparing transaction: ...working... done Verifying transaction: ...working... done Executing transaction: ...working... done +------------------------------------------------------+ | To use this environment, load the following modules: | | module load use.own | | module load conda-env/mypackages-py3.8.5 | +------------------------------------------------------+ Your environment "mypackages" was created successfully.
Note down the module names, as you will need to load these modules every time you want to use this environment. You may also want to add the module load lines in your jobscript, if it depends on custom Python packages.
By default, module files are generated in your $HOME/privatemodules directory. The location of module files can be customized by specifying the -m /path/to/modules option to conda-env-mod.
Note: The main differences between -p and -m are: 1) -p will change the location of packages to be installed for the env and the module file will still be located at the $HOME/privatemodules directory as defined in use.own. 2) -m will only change the location of the module file. So the method to load modules created with -m and -p are different, see Example 3 for details.
- Example 3: Create a conda environment named labpackages in your group's Data Depot space and place the module file at a shared location for the group to use.
$ conda-env-mod create -p /depot/mylab/apps/labpackages -m /depot/mylab/etc/modules ... ... ... Preparing transaction: ...working... done Verifying transaction: ...working... done Executing transaction: ...working... done +-------------------------------------------------------+ | To use this environment, load the following modules: | | module use /depot/mylab/etc/modules | | module load conda-env/labpackages-py3.8.5 | +-------------------------------------------------------+ Your environment "labpackages" was created successfully.
If you used a custom module file location, you need to run the module use command as printed by the command output above.
By default, only the environment and a module file are created (no Jupyter kernel). If you plan to use your environment in a JupyterHub notebook, you need to append a --jupyter flag to the above commands.
- Example 4: Create a Jupyter-enabled conda environment named labpackages in your group's Data Depot space and place the module file at a shared location for the group to use.
$ conda-env-mod create -p /depot/mylab/apps/labpackages -m /depot/mylab/etc/modules --jupyter ... ... ... Jupyter kernel created: "Python (My labpackages Kernel)" ... ... ... Your environment "labpackages" was created successfully.
Link to section 'Step 2: Load the conda environment' of 'Installing Packages' Step 2: Load the conda environment
-
The following instructions assume that you have used conda-env-mod script to create an environment named mypackages (Examples 1 or 2 above). If you used conda create instead, please use conda activate mypackages.
$ module load use.own $ module load conda-env/mypackages-py3.8.5
Note that the conda-env module name includes the Python version that it supports (Python 3.8.5 in this example). This is same as the Python version in the conda module.
-
If you used a custom module file location (Example 3 above), please use module use to load the conda-env module.
$ module use /depot/mylab/etc/modules $ module load conda-env/labpackages-py3.8.5
Link to section 'Step 3: Install packages' of 'Installing Packages' Step 3: Install packages
Now you can install custom packages in the environment using either conda install or pip install.
Link to section 'Installing with conda' of 'Installing Packages' Installing with conda
-
Example 1: Install OpenCV (open-source computer vision library) using conda.
$ conda install opencv
-
Example 2: Install a specific version of OpenCV using conda.
$ conda install opencv=4.5.5
-
Example 3: Install OpenCV from a specific anaconda channel.
$ conda install -c anaconda opencv
Link to section 'Installing with pip' of 'Installing Packages' Installing with pip
-
Example 4: Install pandas using pip.
$ pip install pandas
-
Example 5: Install a specific version of pandas using pip.
$ pip install pandas==1.4.3
Follow the on-screen instructions while the packages are being installed. If installation is successful, please proceed to the next section to test the packages.
Note: Do NOT run Pip with the --user argument, as that will install packages in a different location and might mess up your account environment.
Link to section 'Step 4: Test the installed packages' of 'Installing Packages' Step 4: Test the installed packages
To use the installed Python packages, you must load the module for your conda environment. If you have not loaded the conda-env module, please do so following the instructions at the end of Step 1.
$ module load use.own
$ module load conda-env/mypackages-py3.8.5
- Example 1: Test that OpenCV is available.
$ python -c "import cv2; print(cv2.__version__)"
- Example 2: Test that pandas is available.
$ python -c "import pandas; print(pandas.__version__)"
If the commands finished without errors, then the installed packages can be used in your program.
Link to section 'Additional capabilities of conda-env-mod script' of 'Installing Packages' Additional capabilities of conda-env-mod script
The conda-env-mod tool is intended to facilitate creation of a minimal Anaconda environment, matching module file and optionally a Jupyter kernel. Once created, the environment can then be accessed via familiar module load command, tuned and expanded as necessary. Additionally, the script provides several auxiliary functions to help manage environments, module files and Jupyter kernels.
General usage for the tool adheres to the following pattern:
$ conda-env-mod help
$ conda-env-mod <subcommand> <required argument> [optional arguments]
where required arguments are one of
- -n|--name ENV_NAME (name of the environment)
- -p|--prefix ENV_PATH (location of the environment)
and optional arguments further modify behavior for specific actions (e.g. -m to specify alternative location for generated module files).
Given a required name or prefix for an environment, the conda-env-mod script supports the following subcommands:
- create - to create a new environment, its corresponding module file and optional Jupyter kernel.
- delete - to delete existing environment along with its module file and Jupyter kernel.
- module - to generate just the module file for a given existing environment.
- kernel - to generate just the Jupyter kernel for a given existing environment (note that the environment has to be created with a --jupyter option).
- help - to display script usage help.
Using these subcommands, you can iteratively fine-tune your environments, module files and Jupyter kernels, as well as delete and re-create them with ease. Below we cover several commonly occurring scenarios.
Note: When you try to use conda-env-mod delete, remember to include the arguments as you create the environment (i.e. -p package_location and/or -m module_location).
Link to section 'Generating module file for an existing environment' of 'Installing Packages' Generating module file for an existing environment
If you already have an existing configured Anaconda environment and want to generate a module file for it, follow appropriate examples from Step 1 above, but use the module subcommand instead of the create one. E.g.
$ conda-env-mod module -n mypackages
and follow printed instructions on how to load this module. With an optional --jupyter flag, a Jupyter kernel will also be generated.
Note that the module name mypackages should be exactly the same with the older conda environment name. Note also that if you intend to proceed with a Jupyter kernel generation (via the --jupyter flag or a kernel subcommand later), you will have to ensure that your environment has ipython and ipykernel packages installed into it. To avoid this and other related complications, we highly recommend making a fresh environment using a suitable conda-env-mod create .... --jupyter command instead.
Link to section 'Generating Jupyter kernel for an existing environment' of 'Installing Packages' Generating Jupyter kernel for an existing environment
If you already have an existing configured Anaconda environment and want to generate a Jupyter kernel file for it, you can use the kernel subcommand. E.g.
$ conda-env-mod kernel -n mypackages
This will add a "Python (My mypackages Kernel)" item to the dropdown list of available kernels upon your next login to the JupyterHub.
Note that generated Jupiter kernels are always personal (i.e. each user has to make their own, even for shared environments). Note also that you (or the creator of the shared environment) will have to ensure that your environment has ipython and ipykernel packages installed into it.
Link to section 'Managing and using shared Python environments' of 'Installing Packages' Managing and using shared Python environments
Here is a suggested workflow for a common group-shared Anaconda environment with Jupyter capabilities:
The PI or lab software manager:
-
Creates the environment and module file (once):
$ module purge $ module load conda $ conda-env-mod create -p /depot/mylab/apps/labpackages -m /depot/mylab/etc/modules --jupyter
-
Installs required Python packages into the environment (as many times as needed):
$ module use /depot/mylab/etc/modules $ module load conda-env/labpackages-py3.8.5 $ conda install ....... # all the necessary packages
Lab members:
-
Lab members can start using the environment in their command line scripts or batch jobs simply by loading the corresponding module:
$ module use /depot/mylab/etc/modules $ module load conda-env/labpackages-py3.8.5 $ python my_data_processing_script.py .....
-
To use the environment in Jupyter notebooks, each lab member will need to create his/her own Jupyter kernel (once). This is because Jupyter kernels are private to individuals, even for shared environments.
$ module use /depot/mylab/etc/modules $ module load conda-env/labpackages-py3.8.5 $ conda-env-mod kernel -p /depot/mylab/apps/labpackages
A similar process can be devised for instructor-provided or individually-managed class software, etc.
Link to section 'Troubleshooting' of 'Installing Packages' Troubleshooting
- Python packages often fail to install or run due to dependency incompatibility with other packages. More specifically, if you previously installed packages in your home directory it is safer to clean those installations.
$ mv ~/.local ~/.local.bak $ mv ~/.cache ~/.cache.bak
- Unload all the modules.
$ module purge
- Clean up PYTHONPATH.
$ unset PYTHONPATH
- Next load the modules (e.g. anaconda) that you need.
$ module load conda/2024.02-py311 $ module load use.own $ module load conda-env/2024.02-py311
- Now try running your code again.
- Few applications only run on specific versions of Python (e.g. Python 3.6). Please check the documentation of your application if that is the case.
Installing Packages from Source
We maintain several Anaconda installations. Anaconda maintains numerous popular scientific Python libraries in a single installation. If you need a Python library not included with normal Python we recommend first checking Anaconda. For a list of modules currently installed in the Anaconda Python distribution:
$ module load conda
$ conda list
# packages in environment at /apps/spack/bell/apps/anaconda/2020.02-py37-gcc-4.8.5-u747gsx:
#
# Name Version Build Channel
_ipyw_jlab_nb_ext_conf 0.1.0 py37_0
_libgcc_mutex 0.1 main
alabaster 0.7.12 py37_0
anaconda 2020.02 py37_0
...
If you see the library in the list, you can simply import it into your Python code after loading the Anaconda module.
If you do not find the package you need, you should be able to install the library in your own Anaconda customization. First try to install it with Conda or Pip. If the package is not available from either Conda or Pip, you may be able to install it from source.
Use the following instructions as a guideline for installing packages from source. Make sure you have a download link to the software (usually it will be a tar.gz
archive file). You will substitute it on the wget line below.
We also assume that you have already created an empty conda environment as described in our Python package installation guide.
$ mkdir ~/src
$ cd ~/src
$ wget http://path/to/source/tarball/app-1.0.tar.gz
$ tar xzvf app-1.0.tar.gz
$ cd app-1.0
$ module load conda
$ module load use.own
$ module load conda-env/mypackages-py3.8.5
$ python setup.py install
$ cd ~
$ python
>>> import app
>>> quit()
The "import app" line should return without any output if installed successfully. You can then import the package in your python scripts.
If you need further help or run into any issues installing a library, contact us or drop by Coffee Hour for in-person help.
For more information about Python:
Example: Create and Use Biopython Environment with Conda
Link to section 'Using conda to create an environment that uses the biopython package' of 'Example: Create and Use Biopython Environment with Conda' Using conda to create an environment that uses the biopython package
To use Conda you must first load the anaconda module:
module load conda
Create an empty conda environment to install biopython:
conda-env-mod create -n biopython
Now activate the biopython environment:
module load use.own
module load conda-env/biopython-py3.12.5
Install the biopython packages in your environment:
conda install --channel anaconda biopython -y
Fetching package metadata ..........
Solving package specifications .........
.......
Linking packages ...
[ COMPLETE ]|################################################################
The --channel option specifies that it searches the anaconda channel for the biopython package. The -y argument is optional and allows you to skip the installation prompt. A list of packages will be displayed as they are installed.
Remember to add the following lines to your job submission script to use the custom environment in your jobs:
module load conda
module load use.own
module load conda-env/biopython-py3.12.5
If you need further help or run into any issues with creating environments, contact us or drop by Coffee Hour for in-person help.
For more information about Python:
Numpy Parallel Behavior
The widely available Numpy package is the best way to handle numerical computation in Python. The numpy
package provided by our anaconda
modules is optimized using Intel's MKL library. It will automatically parallelize many operations to make use of all the cores available on a machine.
In many contexts that would be the ideal behavior. On the cluster however that very likely is not in fact the preferred behavior because often more than one user is present on the system and/or more than one job on a node. Having multiple processes contend for those resources will actually result in lesser performance.
Setting the MKL_NUM_THREADS
or OMP_NUM_THREADS
environment variable(s) allows you to control this behavior. Our anaconda modules automatically set these variables to 1 if and only if you do not currently have that variable defined.
When submitting batch jobs it is always a good idea to be explicit rather than implicit. If you are submitting a job that you want to make use of the full resources available on the node, set one or both of these variables to the number of cores you want to allow numpy to make use of.
#!/bin/bash
module load conda
export MKL_NUM_THREADS=128
...
If you are submitting multiple jobs that you intend to be scheduled together on the same node, it is probably best to restrict numpy to a single core.
#!/bin/bash
module load conda
export MKL_NUM_THREADS=1
R
R, a GNU project, is a language and environment for data manipulation, statistics, and graphics. It is an open source version of the S programming language. R is quickly becoming the language of choice for data science due to the ease with which it can produce high quality plots and data visualizations. It is a versatile platform with a large, growing community and collection of packages.
For more general information on R visit The R Project for Statistical Computing.
Running R jobs
This section illustrates how to submit a small R job to a SLURM queue. The example job computes a Pythagorean triple.
Prepare an R input file with an appropriate filename, here named myjob.R:
# FILENAME: myjob.R
# Compute a Pythagorean triple.
a = 3
b = 4
c = sqrt(a*a + b*b)
c # display result
Prepare a job submission file with an appropriate filename, here named myjob.sub:
#!/bin/bash
# FILENAME: myjob.sub
module load r
# --vanilla:
# --no-save: do not save datasets at the end of an R session
R --vanilla --no-save < myjob.R
For other examples or R jobs:
Installing R packages
Link to section 'Challenges of Managing R Packages in the Cluster Environment' of 'Installing R packages' Challenges of Managing R Packages in the Cluster Environment
- Different clusters have different hardware and softwares. So, if you have access to multiple clusters, you must install your R packages separately for each cluster.
- Each cluster has multiple versions of R and packages installed with one version of R may not work with another version of R. So, libraries for each R version must be installed in a separate directory.
- You can define the directory where your R packages will be installed using the environment variable
R_LIBS_USER
. - For your convenience, a sample ~/.Rprofile example file is provided that can be downloaded to your cluster account and renamed into
~/.Rprofile
(or appended to one) to customize your installation preferences. Detailed instructions.
Link to section 'Installing Packages' of 'Installing R packages' Installing Packages
-
Step 0: Set up installation preferences.
Follow the steps for setting up your~/.Rprofile
preferences. This step needs to be done only once. If you have created a~/.Rprofile
file previously on Negishi, ignore this step. -
Step 1: Check if the package is already installed.
As part of the R installations on community clusters, a lot of R libraries are pre-installed. You can check if your package is already installed by opening an R terminal and entering the commandinstalled.packages()
. For example,module load r/4.4.1 R
installed.packages()["units",c("Package","Version")] Package Version "units" "0.8-1" quit()
If the package you are trying to use is already installed, simply load the library, e.g.,
library('units')
. Otherwise, move to the next step to install the package. -
Step 2: Load required dependencies. (if needed)
For simple packages you may not need this step. However, some R packages depend on other libraries. For example, thesf
package depends ongdal
andgeos
libraries. So, you will need to load the corresponding modules before installingsf
. Read the documentation for the package to identify which modules should be loaded.module load gdal module load geos
-
Step 3: Install the package.
Now install the desired package using the commandinstall.packages('package_name')
. R will automatically download the package and all its dependencies from CRAN and install each one. Your terminal will show the build progress and eventually show whether the package was installed successfully or not.R
install.packages('sf', repos="https://cran.case.edu/") Installing package into ‘/home/myusername/R/x86_64-pc-linux-gnu-library/4.4.1’ (as ‘lib’ is unspecified) trying URL 'https://cran.case.edu/src/contrib/sf_0.9-7.tar.gz' Content type 'application/x-gzip' length 4203095 bytes (4.0 MB) ================================================== downloaded 4.0 MB ... ... more progress messages ... ... ** testing if installed package can be loaded from final location ** testing if installed package keeps a record of temporary installation path * DONE (sf) The downloaded source packages are in ‘/tmp/RtmpSVAGio/downloaded_packages’
- Step 4: Troubleshooting. (if needed)
If Step 3 ended with an error, you need to investigate why the build failed. Most common reason for build failure is not loading the necessary modules.
Link to section 'Loading Libraries' of 'Installing R packages' Loading Libraries
Once you have packages installed you can load them with the library()
function as shown below:
library('packagename')
The package is now installed and loaded and ready to be used in R.
Link to section 'Example: Installing dplyr' of 'Installing R packages' Example: Installing dplyr
The following demonstrates installing the dplyr
package assuming the above-mentioned custom ~/.Rprofile
is in place (note its effect in the "Installing package into" information message):
module load r
R
install.packages('dplyr', repos="http://ftp.ussg.iu.edu/CRAN/")
Installing package into ‘/home/myusername/R/negishi/4.4.1’
(as ‘lib’ is unspecified)
...
also installing the dependencies 'crayon', 'utf8', 'bindr', 'cli', 'pillar', 'assertthat', 'bindrcpp', 'glue', 'pkgconfig', 'rlang', 'Rcpp', 'tibble', 'BH', 'plogr'
...
...
...
The downloaded source packages are in
'/tmp/RtmpHMzm9z/downloaded_packages'
library(dplyr)
Attaching package: 'dplyr'
For more information about installing R packages:
Loading Data into R
R is an environment for manipulating data. In order to manipulate data, it must be brought into the R environment. R has a function to read any file that data is stored in. Some of the most common file types like comma-separated variable(CSV) files have functions that come in the basic R packages. Other less common file types require additional packages to be installed. To read data from a CSV file into the R environment, enter the following command in the R prompt:
> read.csv(file = "path/to/data.csv", header = TRUE)
When R reads the file it creates an object that can then become the target of other functions. By default the read.csv() function will give the object the name of the .csv file. To assign a different name to the object created by read.csv enter the following in the R prompt:
> my_variable <- read.csv(file = "path/to/data.csv", header = FALSE)
To display the properties (structure) of loaded data, enter the following:
> str(my_variable)
For more functions and tutorials:
RStudio
RStudio is a graphical integrated development environment (IDE) for R. RStudio is the most popular environment for developing both R scripts and packages. RStudio is provided on most Research systems.
There are two methods to launch RStudio on the cluster: command-line and application menu icon.
Link to section 'Launch RStudio by the command-line:' of 'RStudio' Launch RStudio by the command-line:
module load gcc
module load r
module load rstudio
rstudio
Note that RStudio is a graphical program and in order to run it you must have a local X11 server running or use Thinlinc Remote Desktop environment. See the ssh X11 forwarding section for more details.
Link to section 'Launch Rstudio by the application menu icon:' of 'RStudio' Launch Rstudio by the application menu icon:
- Log into desktop.negishi.rcac.purdue.edu with web browser or ThinLinc client
- Click on the
Applications
drop down menu on the top left corner - Choose
Cluster Software
and thenRStudio
R and RStudio are free to download and run on your local machine. For more information about RStudio:
Setting Up R Preferences with .Rprofile
For your convenience, a sample ~/.Rprofile example file is provided that can be downloaded to your cluster account and renamed into ~/.Rprofile
(or appended to one). Follow these steps to download our recommended ~/.Rprofile
example and copy it into place:
curl -#LO https://www.rcac.purdue.edu/files/knowledge/run/examples/apps/r/Rprofile_example
mv -ib Rprofile_example ~/.Rprofile
The above installation step needs to be done only once on Negishi. Now load the R module and run R:
module load r/4.4.1
R
.libPaths()
[1] "/home/myusername/R/negishi/4.1.2-gcc-6.3.0-ymdumss"
[2] "/apps/spack/negishi/apps/r/4.1.2-gcc-6.3.0-ymdumss/rlib/R/library"
.libPaths()
should output something similar to above if it is set up correctly.
You are now ready to install R packages into the dedicated directory /home/myusername/R/negishi/4.1.2-gcc-6.3.0-ymdumss
.
Singularity
On Negishi, Singularity functionality is provided by Apptainer - see Apptainer section for details.
Windows
Windows virtual machines (VMs) are supported as batch jobs on HPC systems. This section illustrates how to submit a job and run a Windows instance in order to run Windows applications on the high-performance computing systems.
The following images are pre-configured and made available by staff:
- Windows 2016 Server Basic (minimal software pre-loaded)
- Windows 2016 Server GIS (GIS Software Stack pre-loaded)
The Windows VMs can be launched in two fashions:
- Menu Launcher - Point and click to start
- Command Line - Advanced and customized usage
Click each of the above links for detailed instructions on using them.
Link to section 'Software Provided in Pre-configured Virtual Machines' of 'Windows' Software Provided in Pre-configured Virtual Machines
The Windows 2016 Base server image available on Negishi has the following software packages preloaded:
- Anaconda Python 2 and Python 3
- JMP 13
- Matlab R2017b
- Microsoft Office 2016
- Notepad++
- NVivo 12
- Rstudio
- Stata SE 15
- VLC Media Player
Command line
If you wish to work with Windows VMs on the command line or work into scripted workflows you can interact directly with the Windows system:
Copy a Windows 2016 Server VM image to your storage. Scratch or Research Data Depot are good locations to save a VM image. If you are using scratch, remember that scratch spaces are temporary, and be sure to safely back up your disk image somewhere permanent, such as Research Data Depot or Fortress. To copy a basic image:
$ cp /apps/external/apps/windows/images/latest.qcow2 $RCAC_SCRATCH/windows.qcow2
To copy a GIS image:
$ cp /depot/itap/windows/gis/2k16.qcow2 $RCAC_SCRATCH/windows.qcow2
To launch a virtual machine in a batch job, use the "windows" script, specifying the path to your Windows virtual machine image. With no other command-line arguments, the windows script will autodetect a number cores and memory for the Windows VM. A Windows network connection will be made to your home directory. To launch:
$ windows -i $RCAC_SCRATCH/windows.qcow2
Link to section 'Command line options:' of 'Command line' Command line options:
-i <path to qcow image file> (For example, $RCAC_SCRATCH/windows-2k16.qcow2)
-m <RAM>G (For example, 32G)
-c <cores> (For example, 20)
-s <smbpath> (UNIX Path to map as a drive, for example, $RCAC_SCRATCH)
-b (If present, launches VM in background. Use VNC to connect to Windows.)
To launch a virtual machine with 32GB of RAM, 20 cores, and a network mapping to your home directory:
$ windows -i /path/to/image.qcow2 -m 32G -c 20 -s $HOME
To launch a virtual machine with 16GB of RAM, 10 cores, and a network mapping to your Data Depot space:
$ windows -i /path/to/image.qcow2 -m 16G -c 10 -s /depot/mylab
The Windows 2016 server desktop will open, and automatically log in as an administrator, so that you can install any software into the Windows virtual machine that your research requires. Changes to the image will be stored in the file specified with the -i option.
Menu Launcher
Windows VMs can be easily launched through the login/thinlinc">Thinlinc remote desktop environment.
- Log in via login/thinlinc">Thinlinc.
- Click on Applications menu in the upper left corner.
- Look under the Cluster Software menu.
- The "Windows 10" launcher will launch a VM directly on the front-end.
- Follow the dialogs to set up your VM.
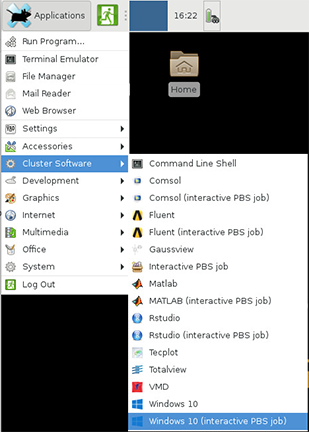
The dialog menus will walk you through setting up and loading your VM.
- You can choose to create a new image or load a saved image.
- New VMs should be saved on Scratch or Research Data Depot as they are too large for Home Directories.
- If you are using scratch, remember that scratch spaces are temporary, and be sure to safely back up your disk image somewhere permanent, such as Research Data Depot or Fortress.
You will also be prompted to select a storage space to mount on your image (Home, Scratch, or Data Depot). You can only choose one to be mounted. It will appear on a shortcut on the desktop once the VM loads.
Link to section 'Notes' of 'Menu Launcher' Notes
Using the menu launcher will launch automatically select reasonable CPU and memory values. If you wish to choose other options or work Windows VMs into scripted workflows see the section on using the command line.
BioContainers Collection
Link to section 'What is BioContainers?' of 'BioContainers Collection' What is BioContainers?
The BioContainers project came from the idea of using the containers-based technologies such as Docker or rkt for bioinformatics software. Having a common and controllable environment for running software could help to deal with some of the current problems during software development and distribution. BioContainers is a community-driven project that provides the infrastructure and basic guidelines to create, manage and distribute bioinformatics containers with a special focus on omics fields such as proteomics, genomics, transcriptomics and metabolomics. . For more information, please visit BioContainers project.
Link to section ' Getting Started ' of 'BioContainers Collection' Getting Started
Users can download bioinformatic containers from the BioContainers.pro and run them directly using Singularity instructions from the corresponding container’s catalog page.
Brief Singularity guide and examples are available at the Negishi Singularity user guide page. Detailed Singularity user guide is available at: sylabs.io/guides/3.8/user-guide
In addition, a subset of pre-downloaded biocontainers wrapped into convenient software modules are provided. These modules wrap underlying complexity and provide the same commands that are expected from non-containerized versions of each application.
On Negishi, type the command below to see the lists of biocontainers we deployed.
module load biocontainers
module avail
------------ BioContainers collection modules -------------
bamtools/2.5.1
beast2/2.6.3
bedtools/2.30.0
blast/2.11.0
bowtie2/2.4.2
bwa/0.7.17
cufflinks/2.2.1
deeptools/3.5.1
fastqc/0.11.9
faststructure/1.0
htseq/0.13.5
[....]
Link to section ' Example ' of 'BioContainers Collection' Example
This example demonstrates how to run BLASTP with the blast module. This blast module is a biocontainer wrapper for NCBI BLAST.
module load biocontainers
module load blast
blastp -query query.fasta -db nr -out output.txt -outfmt 6 -evalue 0.01
To run a job in batch mode, first prepare a job script that specifies the BioContainer modules you want to launch and the resources required to run it. Then, use the sbatch
command to submit your job script to Slurm. The following example shows the job script to use Bowtie2 in bioinformatic analysis.
#!/bin/bash
#SBATCH -A myqueuename
#SBATCH -o bowtie2_%j.txt
#SBATCH -e bowtie2_%j.err
#SBATCH --nodes=1
#SBATCH --ntasks-per-node=1
#SBATCH --cpus-per-task=8
#SBATCH --time=1:30:00
#SBATCH --job-name bowtie2
# Load the Bowtie module
module load biocontainers
module load bowtie2
# Indexing a reference genome
bowtie2-build ref.fasta ref
# Aligning paired-end reads
bowtie2 -p 8 -x ref -1 reads_1.fq -2 reads_2.fq -S align.sam
To help users get started, we provided detailed user guides for each containerized bioinformatics module on the ReadTheDocs platform
Ansys Fluent
Ansys is a CAE/multiphysics engineering simulation software that utilizes finite element analysis for numerically solving a wide variety of mechanical problems. The software contains a list of packages and can simulate many structural properties such as strength, toughness, elasticity, thermal expansion, fluid dynamics as well as acoustic and electromagnetic attributes.
Link to section 'Ansys Licensing' of 'Ansys Fluent' Ansys Licensing
The Ansys licensing on our community clusters is maintained by Purdue ECN group. There are two types of licenses: teaching and research. For more information, please refer to ECN Ansys licensing page. If you are interested in purchasing your own research license, please send email to software@ecn.purdue.edu.
Link to section 'Ansys Workflow' of 'Ansys Fluent' Ansys Workflow
Ansys software consists of several sub-packages such as Workbench and Fluent. Most simulations are performed using the Ansys Workbench console, a GUI interface to manage and edit the simulation workflow. It requires X11 forwarding for remote display so a SSH client software with X11 support or a remote desktop portal is required. Please see Logging In section for more details. To ensure preferred performance, ThinLinc remote desktop connection is highly recommended.
Typically users break down larger structures into small components in geometry with each of them modeled and tested individually. A user may start by defining the dimensions of an object, adding weight, pressure, temperature, and other physical properties.
Ansys Fluent is a computational fluid dynamics (CFD) simulation software known for its advanced physics modeling capabilities and accuracy. Fluent offers unparalleled analysis capabilities and provides all the tools needed to design and optimize new equipment and to troubleshoot existing installations.
In the following sections, we provide step-by-step instructions to lead you through the process of using Fluent. We will create a classical elbow pipe model and simulate the fluid dynamics when water flows through the pipe. The project files have been generated and can be downloaded via fluent_tutorial.zip.
Link to section 'Loading Ansys Module' of 'Ansys Fluent' Loading Ansys Module
Different versions of Ansys are installed on the clusters and can be listed with module spider
or module avail
command in the terminal.
$ module avail ansys/
---------------------- Core Applications -----------------------------
ansys/2019R3 ansys/2020R1 ansys/2021R2 ansys/2022R1 (D)
Before launching Ansys Workbench, a specific version of Ansys module needs to be loaded. For example, you can module load ansys/2021R2
to use the latest Ansys 2021R2. If no version is specified, the default module -> (D) (ansys/2022R1
in this case) will be loaded. You can also check the loaded modules with module list
command.
Link to section 'Launching Ansys Workbench' of 'Ansys Fluent' Launching Ansys Workbench
Open a terminal on Negishi, enter rcac-runwb2
to launch Ansys Workbench.
You can also use runwb2
to launch Ansys Workbench. The main difference between runwb2
and rcac-runwb2
is that the latter sets the project folder to be in your scratch space. Ansys has an known bug that it might crash when the project folder is set to $HOME
on our systems.
Preparing Case Files for Fluent
Link to section 'Creating a Fluent fluid analysis system' of 'Preparing Case Files for Fluent' Creating a Fluent fluid analysis system
In the Ansys Workbench, create a new fluid flow analysis by double-clicking the Fluid Flow (Fluent) option under the Analysis Systems in the Toolbox on the left panel. You can also drag-and-drop the analysis system into the Project Schematic. A green dotted outline indicating a potential location for the new system initially appears in the Project Schematic. When you drag the system to one of the outlines, it turns into a red box to indicate the chosen location of the new system.
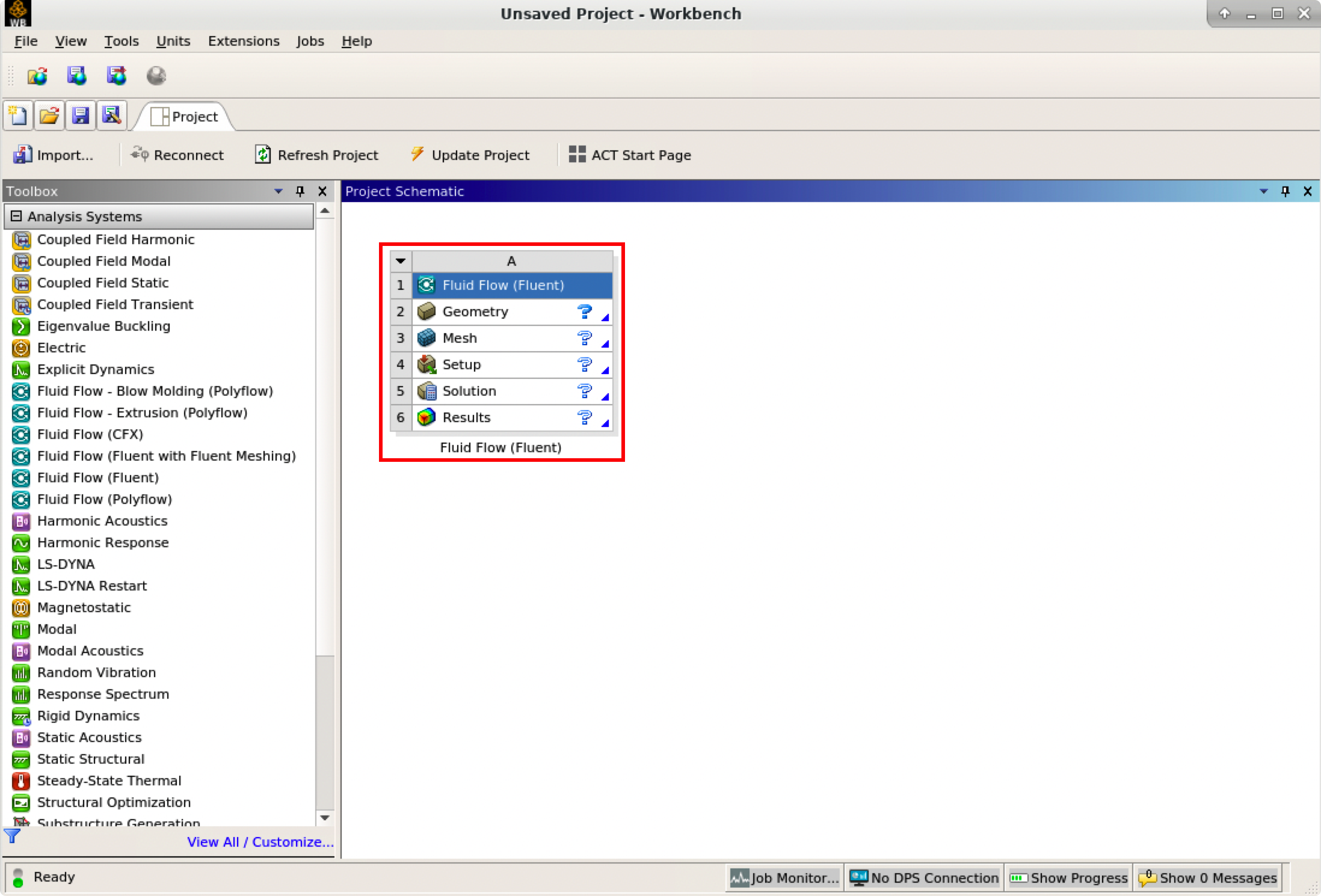
The red rectangle indicates the Fluid Flow system for Fluent, which includes all the essential workflows from “2 Geometry” to “6 Results”. You can rename it and carry out the necessary step-by-step procedures by double-clicking the corresponding cells.
It is important to save the project. Ansys Workbench saves the project with a .wbpj
extension and also all the supporting files into a folder with the same name. In this case, a file named elbow_demo.wbpj
and a folder $Ansys_PROJECT_FOLDER/elbow_demo_files/
are created in the Ansys project folder:
$ ll
total 33
drwxr-xr-x 7 myusername itap 9 Mar 3 17:47 elbow_demo_files
-rw-r--r-- 1 myusername itap 42597 Mar 3 17:47 elbow_demo.wbpj
You should always “Update Project” and save it after finishing a procedure.
Link to section 'Creating Geometry in the Ansys DesignModeler' of 'Preparing Case Files for Fluent' Creating Geometry in the Ansys DesignModeler
Create a geometry in the Ansys DesignModeler (by double-clicking “Geometry” cell in workflow), or import the appropriate geometry file (by right-clicking the Geometry cell and selecting “Import Geometry” option from the context menu).
You can use Ansys DesignModeler to create 2D/3D geometries or even draw the objects yourself. In our example, we created only half of the elbow pipe because the symmetry of the structure is taken into account to reduce the computation intensity.
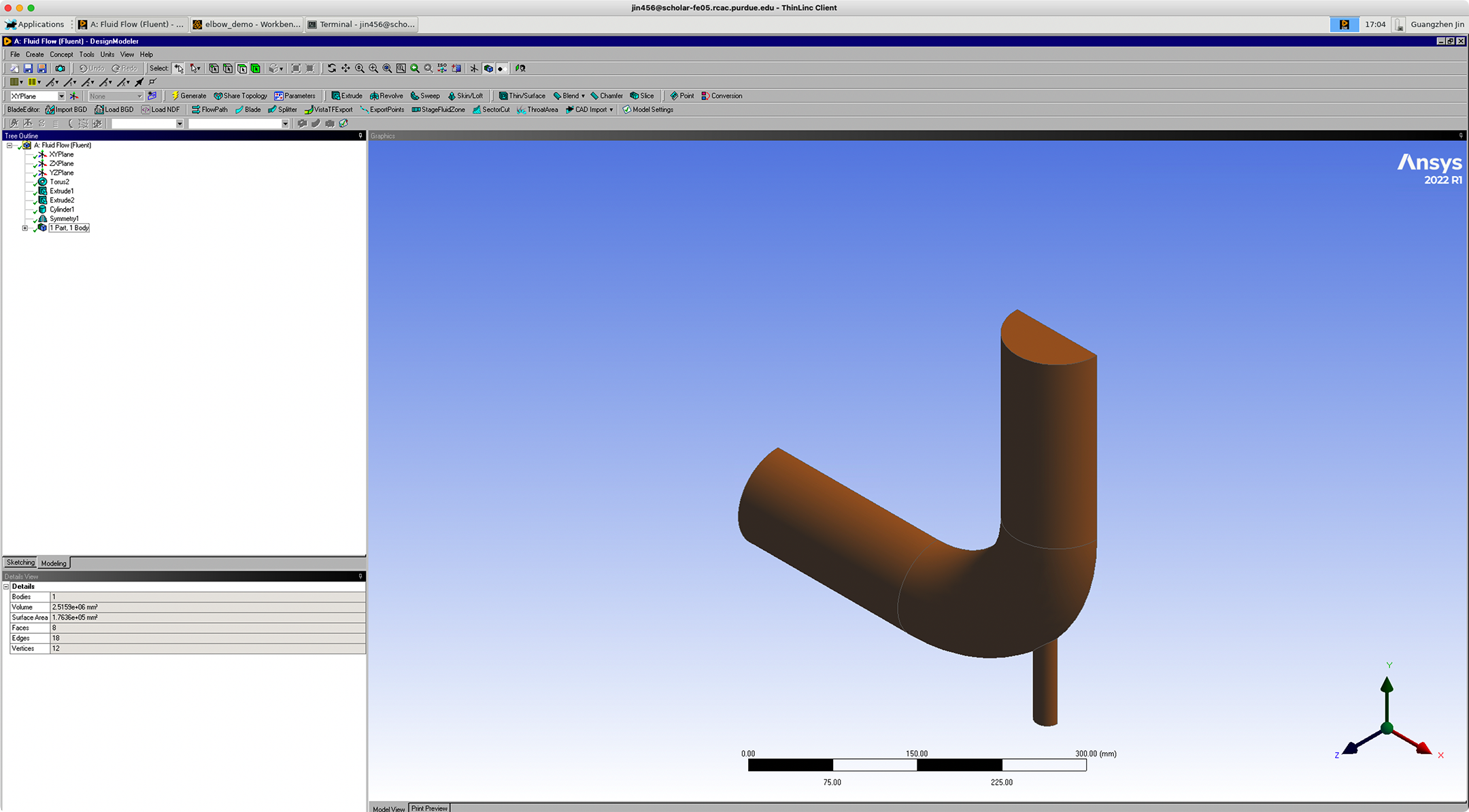
After saving the geometry, a geometry file FFF.agdb
will be created in the folder: $Ansys_PROJECT_FOLDER/elbow_demo_file/dp0/FFF/DM/
. The project in Workbench will be updated automatically.
If you import a pre-existing geometry into Ansys DesignModeler, it will also generate this file with the same filename at this location.
Link to section 'Creating mesh in the Ansys Meshing' of 'Preparing Case Files for Fluent' Creating mesh in the Ansys Meshing
Now that we have created the elbow pipe geometry, a computational mesh can be generated by the Meshing application throughout the flow volume.
With the successful creation of the geometry, there should be a green check showing the completion of “Geometry” in the Ansys Workbench. A Refresh Required icon within the “Mesh” cell indicates the mesh needs to be updated and refreshed for the system.
Then it’s time to open the Ansys Meshing application by double-clicking the “Mesh” cell and editing the mesh for the project. Generally, there are several steps we need to take to define the mesh:
- Create names for all geometry boundaries such as the inlets, outlets and fluid body. Note: You can use the strings “velocity inlet” and “pressure outlet” in the named selections (with or without hyphens or underscore characters) to allow Ansys Fluent to automatically detect and assign the corresponding boundary types accordingly. Use “Fluid” for the body to let Ansys Fluent automatically detect that the volume is a fluid zone and treat it accordingly.
- Set basic meshing parameters for the Ansys Meshing application. Here are several important parameters you may need to assign: Sizing, Quality, Body Sizing Control, Inflation.
- Select “Generate” to generate the mesh and “Update” to update the mesh into the system. Note: Once the mesh is generated, you can view the mesh statistics by opening the Statistics node in the Details of “Mesh” view. This will display information such as the number of nodes and the number of elements, which gives you a general idea for the future computational resources and time.
After generation and updating the mesh, a mesh file FFF.msh
will be generated in folder $Ansys_PROJECT_FOLDER/elbow_demo_file/dp0/FFF/MECH/
and a mesh database file FFF.mshdb
will be generated in folder $Ansys_PROJECT_FOLDER/elbow_demo_file/dp0/global/MECH/
.
Parameters used in demo case (use default if not assigned):
- Length Unit=”mm”
- Names defined for geometry:
- velocity-inlet-large (large inlet on pipe);
- velocity-inlet-small (small inlet on pipe);
- pressure-outlet (outlet on pipe);
- symmetry (symmetry surface);
- Fluid (body);
- Mesh:
- Quality: Smoothing=”high”;
- Inflation: Use Automatic Inflation=“Program Controlled”, Inflation Option=”Smooth Transition”;
- Statistics:
- Nodes=29371;
- Elements=87647.
Link to section 'Calculation with Fluent' of 'Preparing Case Files for Fluent' Calculation with Fluent
Now all the preparations have been ready for the numerical calculation in Ansys Fluent. Both “Geometry” and “Mesh” cells should have green checks on. We can set up the CFD simulation parameters in Ansys Fluent by double-clicking the “Setup” cell.
When Ansys Fluent is first started or by selecting “editing” on the “Setup” cell, the Fluent Launcher is displayed, enabling you to view and/or set certain Ansys Fluent start-up options (e.g. Precision, Parallel, Display). Note that “Dimension” is fixed to “3D” because we are using a 3D model in this project.
After the Fluent is opened, an Ansys Fluent settings file FFF.set
is written under the folder $Ansys_PROJECT_FOLDER/elbow_demo_file/dp0/FFF/Fluent/
.
Then we are going to set up all the necessary parameters for Fluent computation. Here are the key steps for the setup:
- Setting up the domain:
- Change the units for length to be consistent with the Mesh;
- Check the mesh statistics and quality;
- Setting up physics:
- Solver: “Energy”, “Viscous Model”, “Near-Wall Treatment”;
- Materials;
- Zones;
- Boundaries: Inlet, Outlet, Internal, Symmetry, Wall;
- Solving:
- Solution Methods;
- Reports;
- Initialization;
- Iterations and output frequency.
Then the calculation will be carried out and the results will be written out into FFF-1.cas.gz
under folder $Ansys_PROJECT_FOLDER/elbow_demo_file/dp0/FFF/Fluent/
.
This file contains all the settings and simulation results which can be loaded for post analysis and re-computation (more details will be introduced in the following sections). If only configurations and settings within the Fluent are needed, we can open independent Fluent or submit Fluent jobs with bash commands by loading the existing case in order to facilitate the computation process.
Parameters used in demo case (use default if not assigned):
- Domain Setup: Length Units=”mm”;
- Solver: Energy=”on”; Viscous Model=”k-epsilon”; Near-Wall Treatment=”Enhanced Wall Treatment”;
- Materials: water (Density=1000[kg/m^3]; Specific Heat=4216[J/kg-k]; Thermal Conductivity=0.677[w/m-k]; Viscosity=8e-4[kg/m-s]);
- Zones=”fluid (water)”;
- Inlet=”velocity-inlet-large” (Velocity Magnitude=0.4m/s, Specification Method=”Intensity and Hydraulic Diameter”, Turbulent Intensity=5%; Hydraulic Diameter=100mm; Thermal Temperature=293.15k) &”velocity-inlet-small” (Velocity Magnitude=1.2m/s, Specification Method=”Intensity and Hydraulic Diameter”, Turbulent Intensity=5%; Hydraulic Diameter=25mm; Thermal Temperature=313.15k); Internal=”interior-fluid”; Symmetry=”symmetry”; Wall=”wall-fluid”;
- Solution Methods: Gradient=”Green-Gauss Node Based”;
- Report: plot residual and “Facet Maximum” for “pressure-outlet”
- Hybrid Initialization;
- 300 iterations.
Case Calculating with Fluent
Link to section 'Calculation with Fluent' of 'Case Calculating with Fluent' Calculation with Fluent
Now all the files are ready for the Fluent calculations. Both “Geometry” and “Mesh” cells should have green checks. We can set up the CFD simulation parameters in the Ansys Fluent by double-clicking the “Setup” cell.
Ansys Fluent Launcher can be started by selecting “editing” on the “Setup” cell with many startup options (e.g. Precision, Parallel, Display). Note that “Dimension” is fixed to “3D” because we are using a 3D model in this project.
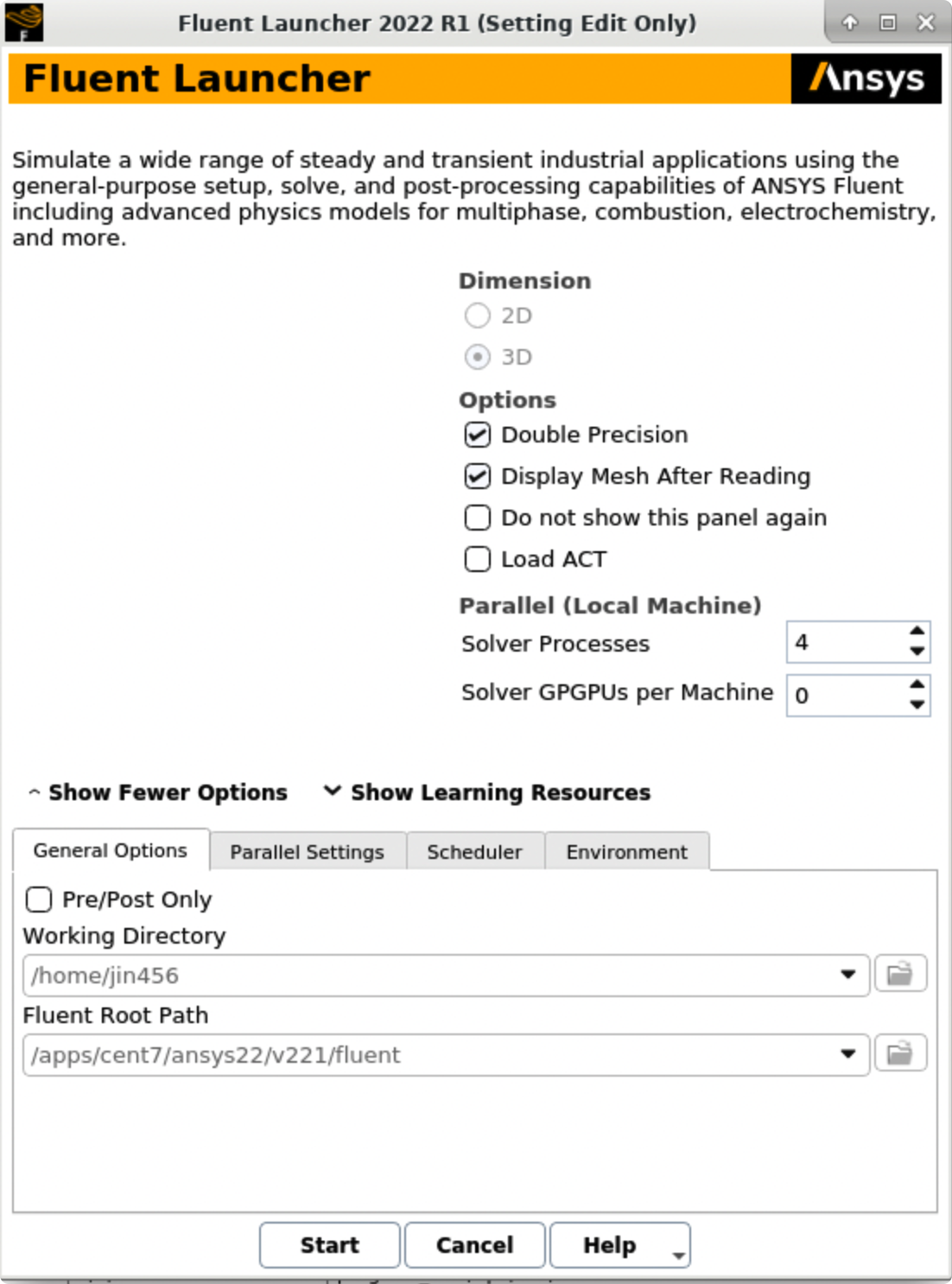
After the Fluent is opened, an Ansys Fluent settings file FFF.set
is written under the folder $Ansys_PROJECT_FOLDER/elbow_demo_file/dp0/FFF/Fluent/
.
Then we are going to set up all the necessary parameters for Fluent computation. Here are the key steps for the setup:
- Setting up the domain:
- Change the units for length to be consistent with the Mesh;
- Check the mesh statistics and quality;
- Setting up physics:
- Solver: “Energy”, “Viscous Model”, “Near-Wall Treatment”;
- Materials;
- Zones;
- Boundaries: Inlet, Outlet, Internal, Symmetry, Wall;
- Solving:
- Solution Methods;
- Reports;
- Initialization;
- Iterations and output frequency.
Then the calculation will be carried out and the results will be written out into FFF-1.cas.gz
under folder $Ansys_PROJECT_FOLDER/elbow_demo_file/dp0/FFF/Fluent/
.
This file contains all the settings and simulation results which can be loaded for post analysis and re-computation (more details will be introduced in the following sections). If only configurations and settings within the Fluent are needed, we can open independent Fluent or submit Fluent jobs with bash commands by loading the existing case in order to facilitate the computation process.
Parameters used in demo case (use default if not assigned):
- Domain Setup: Length Units=”mm”;
- Solver: Energy=”on”; Viscous Model=”k-epsilon”; Near-Wall Treatment=”Enhanced Wall Treatment”;
- Materials: water (Density=1000[kg/m^3]; Specific Heat=4216[J/kg-k]; Thermal Conductivity=0.677[w/m-k]; Viscosity=8e-4[kg/m-s]);
- Zones=”fluid (water)”;
- Inlet=”velocity-inlet-large” (Velocity Magnitude=0.4m/s, Specification Method=”Intensity and Hydraulic Diameter”, Turbulent Intensity=5%; Hydraulic Diameter=100mm; Thermal Temperature=293.15k) &”velocity-inlet-small” (Velocity Magnitude=1.2m/s, Specification Method=”Intensity and Hydraulic Diameter”, Turbulent Intensity=5%; Hydraulic Diameter=25mm; Thermal Temperature=313.15k); Internal=”interior-fluid”; Symmetry=”symmetry”; Wall=”wall-fluid”;
- Solution Methods: Gradient=”Green-Gauss Node Based”;
- Report: plot residual and “Facet Maximum” for “pressure-outlet”
- Hybrid Initialization;
- 300 iterations.
Link to section 'Results analysis' of 'Case Calculating with Fluent' Results analysis
The best methods to view and analyze the simulation should be the Ansys Fluent (directly after computation) or the Ansys CFD-Post (entering “Results” in Ansys Workbench). Both methods are straightforward so we will not cover this part in this tutorial. Here is a final simulation result showing the temperature of the symmetry after 300 iterations for reference:
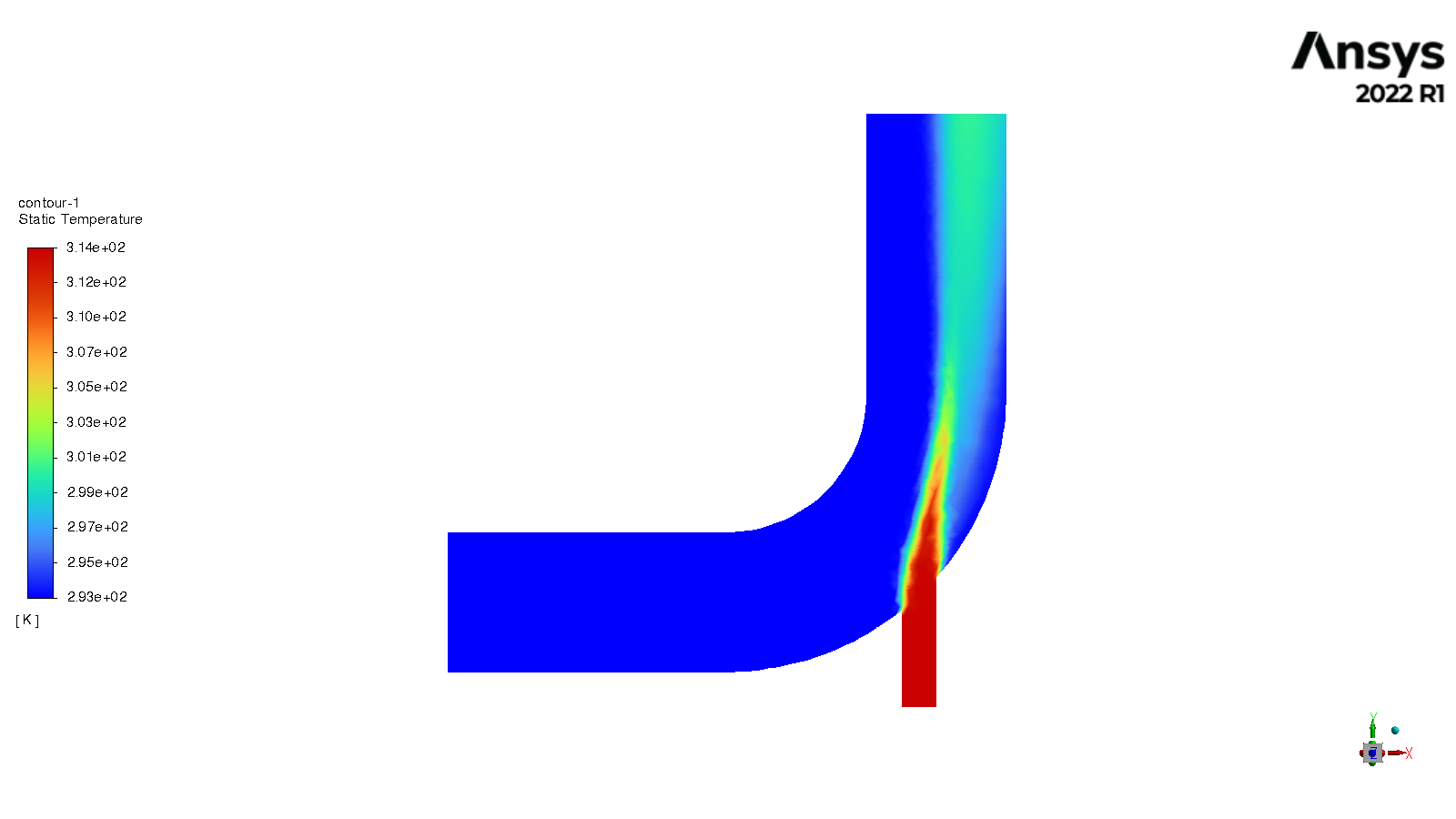
Fluent Text User Interface and Journal File
Link to section 'Fluent Text User Interface (TUI)' of 'Fluent Text User Interface and Journal File' Fluent Text User Interface (TUI)
If you pay attention to the “Console” window in the Fluent window when setting up and carrying out the calculation, corresponding commands can be found and executed one after another. Almost all the setting processes can be accomplished by the command lines, which is called Fluent Text User Interface (TUI). Here are the main commands in Fluent TUI:
adjoint/ parallel/ solve/
define/ plot/ surface/
display/ preferences/ turbo-workflow/
exit print-license-usage views/
file/ report/
mesh/ server/
For example, instead of opening a case by clicking buttons in Ansys Fluent, we can type /file read-case case_file_name.cas.gz
to open the saved case.
Link to section 'Fluent Journal Files' of 'Fluent Text User Interface and Journal File' Fluent Journal Files
A Fluent journal file is a series of TUI commands stored in a text file. The file can be written in a text editor or generated by Fluent as a transcript of the commands given to Fluent during your session.
A journal file generated by Fluent will include any GUI operations (in a TUI form, though). This is quite useful if you have a series of tasks that you need to execute, as it provides a shortcut. To record a journal file, start recording with File -> Write -> Start Journal..., perform whatever tasks you need, and then stop recording with File -> Write -> Stop Journal...
You can also write your own journal file into a text file. The basic rule for a Fluent journal file is to reproduce the TUI commands that controlled the configuration and calculation of Fluent in their order. You can add a comment in a line starting with a ;
(semicolon).
Here are some reasons why you should use a Fluent journal file:
- Using journal files with bash scripting can allow you to automate your jobs.
- Using journal files can allow you to parameterize your models easily and automatically.
- Using a journal file can set parameters you do not have in your case file e.g. autosaving.
- Using a journal file can allow you to safely save, stop and restart your jobs easily.
The order of your journal file commands is highly important. The correct sequences must be followed and some stages have multiple options e.g. different initialization methods.
Here is a sample Fluent journal file for the demo case:
;testJournal.jou
;Set the TUI version for Fluent
/file/set-tui-version "22.1"
;Read the case. The default folder
/file read-case /home/jin456/Fluent_files/tutorial_case1/elbow_files/dp0/FFF/Fluent/FFF-1.cas.gz
;Initialize the case with Hybrid Initialization
/solve/initialize/hyb-initialization
;Set Number of Iterations to 1000, Reporting Interval to 10 iterations and Profile Update Interval to 1 iteration
/solve/iterate 1000 10 1
;Outputting solver performance data upon completion of the simulation
/parallel timer usage
;Write out the simulation results.
/file write-case-data /home/jin456/Fluent_files/tutorial_case1/elbow_files/dp0/FFF/Fluent/result.cas.h5
;After computation, exit Flent
/exit
Before running this Fluent journal file, you need to make sure: 1) the ansys module has been loaded (it’s highly recommended to load the same version of Ansys when you built the case project); 2) the project case file (***.cas.gz
) has been created.
Then we can use Fluent to run this journal file by simply using:fluent 3ddp -t$NTASKS -g -i testJournal.jou
in the terminal. Here, 3d
indicates this is a 3d model, dp
indicates double precision, -t$NTASKS
tells Fluent how many Solver Processes it will take (e.g. -t4
), -g
means to run without the GUI or graphics, -i
testJournal.jou tells Fluent to read the specific journal file.
Here is a table for the available command line Options for Linux/UNIX and Windows Platforms in Ansys Fluent.
Option | Platform | Description |
---|---|---|
-cc |
all | Use the classic color scheme |
-ccp x |
Windows only | Use the Microsoft Job Scheduler where x is the head node name. |
-cnf=x |
all | Specify the hosts or machine list file |
-driver |
all | Sets the graphics driver (available drivers vary by platform - opengl or x11 or null(Linux/UNIX) - opengl or msw or null (Windows)) |
-env |
all | Show environment variables |
-fgw |
all | Disables the embedded graphics |
-g |
all | Run without the GUI or graphics (Linux/UNIX); Run with the GUI minimized (Windows) |
-gr |
all | Run without graphics |
-gu |
all | Run without the GUI but with graphics (Linux/UNIX); Run with the GUI minimized but with graphics (Windows) |
-help |
all | Display command line options |
-hidden |
Windows only | Run in batch mode |
-host_ip=host:ip |
all | Specify the IP interface to be used by the host process |
-i journal |
all | Reads the specified journal file |
-lsf |
Linux/UNIX only | Run FLUENT using LSF |
-mpi= |
all | Specify MPI implementation |
-mpitest |
all | Will launch an MPI program to collect network performance data |
-nm |
all | Do not display mesh after reading |
-pcheck |
Linux/UNIX only | Checks all nodes |
-post |
all | Run the FLUENT post-processing-only executable |
-p |
all | Choose the interconnect |
-r |
all | List all releases installed |
-rx |
all | Specify release number |
-sge |
Linux/UNIX only | Run FLUENT under Sun Grid Engine |
-sge queue |
Linux/UNIX only | Name of the queue for a given computing grid |
-sgeckpt ckpt_obj |
Linux/UNIX only | Set checkpointing object to ckpt_objfor SGE |
-sgepe fluent_pe min_n-max_n |
Linux/UNIX only | Set the parallel environment for SGE to fluent_pe, min_nand max_n are number of min and max nodes requested |
-tx |
all | Specify the number of processors x |
For more information for Fluent text user interface and journal files, please refer to Fluent FAQ.
Submitting Fluent jobs to SLURM
The Fluent simulations can also run in batch. In this section we provide an example script for submitting Fluent jobs to the SLURM scheduler. Please refer to the Running Jobs section of our user guide for detailed tutorials of submitting jobs.
#!/bin/bash
# Job script for submitting a FLUENT job on multiple cores on a single node
# Apply resources via SLURM
#SBATCH --nodes=1
#SBATCH --ntasks=4
#SBATCH --time=01:00:00
#SBATCH --job-name=fluent_test
#SBATCH -o fluent_test_%j.out
#SBATCH -e fluent_test_%j.err
# Loads Ansys and sets the application up
module purge
module load ansys/2022R1
#Initiating Fluent and reading input journal file
fluent 3ddp -t$NTASKS -g -i testJournal.jou
For more information about submitting Fluent jobs, please refer to Fluent FAQ .
Apptainer
Note: Apptainer was formerly known as Singularity and is now a part of the Linux Foundation. When migrating from Singularity see the user compatibility documentation.
Link to section 'What is Apptainer?' of 'Apptainer' What is Apptainer?
Apptainer is an open-source container platform designed to be simple, fast, and secure. It allows the portability and reproducibility of operating systems and application environments through the use of Linux containers. It gives users complete control over their environment.
Apptainer is like Docker but tuned explicitly for HPC clusters. More information is available on the project’s website.
Link to section 'Features' of 'Apptainer' Features
- Run the latest applications on an Ubuntu or Centos userland
- Gain access to the latest developer tools
- Launch MPI programs easily
- Much more
Apptainer’s user guide is available at: apptainer.org/docs/user/main/introduction.html
Link to section 'Example' of 'Apptainer' Example
Here is an example using an Ubuntu 16.04 image on Negishi:
apptainer exec /depot/itap/singularity/ubuntu1604.img cat /etc/lsb-release
DISTRIB_ID=Ubuntu
DISTRIB_RELEASE=16.04
DISTRIB_CODENAME=xenial
DISTRIB_DESCRIPTION="Ubuntu 16.04 LTS"
Here is another example using a Centos 7 image:
apptainer exec /depot/itap/singularity/centos7.img cat /etc/redhat-release
CentOS Linux release 7.3.1611 (Core)
Link to section 'Purdue Cluster Specific Notes' of 'Apptainer' Purdue Cluster Specific Notes
All service providers will integrate Apptainer slightly differently depending on site. The largest customization will be which default files are inserted into your images so that routine services will work.
Services we configure for your images include DNS settings and account information. File systems we overlay into your images are your home directory, scratch, Data Depot, and application file systems.
Here is a list of paths:
- /etc/resolv.conf
- /etc/hosts
- /home/$USER
- /apps
- /scratch
- /depot
This means that within the container environment these paths will be present and the same as outside the container. The /apps
, /scratch
, and /depot
directories will need to exist inside your container to work properly.
Link to section 'Creating Apptainer Images' of 'Apptainer' Creating Apptainer Images
You can build on your system or straight on the cluster (you do not need root privileges to build or run the container).
You can find information and documentation for how to install and use Apptainer on your system:
We have version 1.1.6
(or newer) on the cluster. Please note that installed versions may change throughout cluster life time, so when in doubt, please check exact version with a --version
command line flag:
apptainer --version
apptainer version 1.1.6-1
Everything you need on how to build a container is available from their user guide. Below are merely some quick tips for getting your own containers built for Negishi.
You can use a Definition File to both build your container and share its specification with collaborators (for the sake of reproducibility). Here is a simplistic example of such a file:
# FILENAME: Buildfile
Bootstrap: docker
From: ubuntu:18.04
%post
apt-get update && apt-get upgrade -y
mkdir /apps /depot /scratch
To build the image itself:
apptainer build ubuntu-18.04.sif Buildfile
The challenge with this approach however is that it must start from scratch if you decide to change something. In order to create a container image iteratively and interactively, you can use the --sandbox
option.
apptainer build --sandbox ubuntu-18.04 docker://ubuntu:18.04
This will not create a flat image file but a directory tree (i.e., a folder), the contents of which are the container's filesystem. In order to get a shell inside the container that allows you to modify it, user the --writable
option.
apptainer shell --writable ubuntu-18.04
Apptainer>
You can then proceed to install any libraries, software, etc. within the container. Then to create the final image file, exit
the shell and call the build
command once more on the sandbox.
apptainer build ubuntu-18.04.sif ubuntu-18.04
Finally, copy the new image to Negishi and run it.
VASP
The Vienna Ab initio Simulation Package (VASP) is a computer program for atomic scale materials modelling, e.g. electronic structure calculations and quantum-mechanical molecular dynamics, from first principles.
Link to section 'VASP License' of 'VASP' VASP License
The VASP team allows only registered users who have purchased their own license to use the software and access is only given to the VASP release which is covered by the license of the respective research group. For those who are interested to use VASP on Negishi , please contact support to request access and provide your email address associated with your license for our verification. Once confirmed, the approved users will be given access to the vasp5
or/and vasp6
unix groups.
Prospective users can use the command below to check their unix groups on the system.
$ id $USER
If you are interested to purchase and get a VASP license, please visit VASP website for more information.
Link to section 'VASP 5 and VASP 6 Installations' of 'VASP' VASP 5 and VASP 6 Installations
Negishi provides VASP 5.4.4.pl2 and VASP 6.4.1 installations and modulefiles with our default environment compiler gcc/12.2.0
and mpi library openmpi/4.1.4
. Note that only license-approved users can load the VASP modulefile as below.
You can use the VASP 5.4.4.pl2 module by:
$ module load vasp/5.4.4.pl2
You can use the VASP 6.4.1 module by:
$ module load vasp/6.4.1
Once a VASP module is loaded, you can choose one of the VASP executables to run your code: vasp_std
, vasp_gam
, and vasp_ncl
.
The VASP pseudopotential files are not provided on Negishi, you may need to bring your own POTCAR files.
Link to section 'Build your own VASP 5 and VASP 6' of 'VASP' Build your own VASP 5 and VASP 6
If you would like to use your own VASP on Negishi, please follow the instructions for Installing VASP.6.X.X and Installing VASP.5.X.X.
In the following sections, we provide some instructions about how to install VASP 5 and VASP 6 as well as bash job submit script on Negishi:
VASP Job Submit Script
This shows an example of a job submission file for running VASP pre-built on Negishi:
#!/bin/bash
#SBATCH -A myqueuename # Queue name(use 'slist' command to find queues' name)
#SBATCH --nodes=1 # Total # of nodes
#SBATCH --ntasks=64 # Total # of MPI tasks
#SBATCH --time=1:00:00 # Total run time limit (hh:mm:ss)
#SBATCH -J myjobname # Job name
#SBATCH -o myjob.o%j # Name of stdout output file
#SBATCH -e myjob.e%j # Name of stderr error file
# Manage processing environment, load compilers and applications.
module load vasp/5.4.4.pl2 # or module load vasp/6.4.1
module list
# Launch MPI code
mpirun -np $SLURM_NTASKS vasp_std
Build your own VASP 5
For VASP 5.X.X version, VASP provide several templates of makefile.include
in the /arch
folder, which contain information such as precompiler options, compiler options, and how to link libraries. You can pick up one based on your system and preferred features. Here we provide some examples about how to install the vasp.5.4.4.pl2.tgz
version on Negishi with different module environments.
Link to section 'Step 1: Download' of 'Build your own VASP 5' Step 1: Download
As a license holder, you can download the source code of VASP from the VASP Portal, we will not check your license in this case.
Copy the VASP resource file vasp.5.4.4.pl2.tgz
to the desired location, and unzip the file tar zxvf vasp.5.4.4.pl2.tgz
to obtain the folder /path/to/vasp-build-folder/vasp.5.4.4.pl2
and reveal its content.
Link to section 'Step 2: Prepare makefile.include' of 'Build your own VASP 5' Step 2: Prepare makefile.include
We recommend to use GNU compilers parallelized using OpenMPI, combined with MKL for VASP compilation on Negishi.
We are using MKL library, which include BLAS, LAPACK, ScaLAPACK, and FFTW as suggested at VASP wiki, and we can modify makefile.include.linux_gnu
from /arch
folder:
$ cd /path/to/vasp-build-folder/vasp.5.4.4.pl2
cp arch/makefile.include.linux_gnu makefile.include
Here are the suggested changes for makefile.include
, replace the lines between DEBUG=-O0
and OBJECTS= fftmpiw.o fftmpi_map.o fftw3d.o fft3dlib.o
with
# Intel MKL (FFTW, BLAS, LAPACK, and scaLAPACK)
LLIBS += -L${MKLROOT}/lib/intel64 -Wl,--no-as-needed -lmkl_gf_lp64 -lmkl_gnu_thread -lmkl_core -lmkl_scalapack_lp64 -lmkl_blacs_openmpi_lp64 -lgomp -lpthread -lm -ldl
INCS = -I$(MKLROOT)/include/fftw
FFLAGS += -march=znver3
# For gcc-10 and higher (comment out for older versions)
FFLAGS += -fallow-argument-mismatch
Remove all the GPU stuff
at the end of makefile.include
file
Load the required modules:
module --force purge
module load gcc/12.2.0 openmpi/4.1.4
module load intel-mkl/2019.9.304
Link to section 'Step 3: Make' of 'Build your own VASP 5' Step 3: Make
Build VASP with command make all
to install all three executables vasp_std
, vasp_gam
, and vasp_ncl
or use make std
to install only the vasp_std
executable. Use make veryclean
to remove the build folder if you would like to start over the installation process.
Link to section 'Step 4: Test' of 'Build your own VASP 5' Step 4: Test
You can open an Interactive session to test the installed VASP with GNU/openMPI compilation, you may bring your own VASP test files:
$ cd /path/to/vasp-test-folder/
module --force purge
module load gcc/12.2.0 openmpi/4.1.4 intel-mkl/2019.9.304
module list
mpirun /path/to/vasp-build-folder/vasp.5.4.4.pl2/bin/vasp_std
Link to section 'Step 5: submit a bash job' of 'Build your own VASP 5' Step 5: submit a bash job
To submit a bash job with your own compiled VASP on Negishi, here is an example about how to set up your environment and launch MPI code.
#!/bin/bash
#SBATCH -A myqueuename # Queue name(use 'slist' command to find queues' name)
#SBATCH --nodes=1 # Total # of nodes
#SBATCH --ntasks=64 # Total # of MPI tasks
#SBATCH --time=1:00:00 # Total run time limit (hh:mm:ss)
#SBATCH -J myjobname # Job name
#SBATCH -o myjob.o%j # Name of stdout output file
#SBATCH -e myjob.e%j # Name of stderr error file
# Manage processing environment,load compilers and applications.
module purge
module load gcc/12.2.0 openmpi/4.1.4 intel-mkl/2019.9.304
module list
export PATH=/path/to/vasp-build-folder/vasp.x.x.x/bin:$PATH
# Launch MPI code
mpirun -np $SLURM_NTASKS vasp_std
Build your own VASP 6
For VASP 6.X.X version, VASP provide several templates of makefile.include
, which contain information such as precompiler options, compiler options, and how to link libraries. You can pick up one based on your system and preferred features . Here we provide some examples about how to install vasp 6.4.1
on Negishi with different module environments.
Link to section 'Step 1: Download' of 'Build your own VASP 6' Step 1: Download
As a license holder, you can download the source code of VASP from the VASP Portal, we will not check your license in this case.
Copy the VASP resource file vasp.6.4.1.tgz
to the desired location, and unzip the file tar zxvf vasp.6.4.1.tgz
to obtain the folder /path/to/vasp-build-folder/vasp.6.4.1
and reveal its content.
Link to section 'Step 2: Prepare makefile.include' of 'Build your own VASP 6' Step 2: Prepare makefile.include
We recommend to use GNU compilers parallelized using OpenMPI + OpenMP, combined with MKL for VASP build on Negishi.
We are using MKL library, which include BLAS, LAPACK, ScaLAPACK, and FFTW as suggested at VASP wiki. We can modify makefile.include.gnu_ompi_mkl_omp
from /arch
folder to fit for system setup:
$ cd /path/to/vasp-build-folder/vasp.6.4.1
$ cp makefile.include.gnu_ompi_mkl_omp makefile.include
Here are the suggested changes for makefile.include
:
-
change
VASP_TARGET_CPU ?= -march=native
toVASP_TARGET_CPU ?= -march=znver3
-
remove
MKLROOT ?= /path/to/your/mkl/installation
-
change
LLIBS_MKL =
toLLIBS +=
-
comment out or remove all the lines after
INCS = -I$(MKLROOT)/include/fftw
Then, load the required modules:
$ module purge
$ module load gcc/12.2.0 openmpi/4.1.4
$ module load intel-mkl/2019.9.304
Link to section 'Step 3: Make' of 'Build your own VASP 6' Step 3: Make
Open makefile
, make sure the first line is VERSIONS = std gam ncl
.
Build VASP with command make all
to install all three executables vasp_std
, vasp_gam
, and vasp_ncl
or use make std
to install only the vasp_std
executable. Use make veryclean
to remove the build folder if you would like to start over the installation process.
Link to section 'Step 4: Test' of 'Build your own VASP 6' Step 4: Test
You can open an Interactive session to test the installed VASP 6. Here is an example of testing above installed VASP 6.4.1 with GNU compilers and OpenMPI:
$ cd /path/to/vasp-build-folder/vasp.6.4.1/testsuite
$ module purge
$ module load gcc/12.2.0 openmpi/4.1.4 intel-mkl/2019.9.304
$ ./runtest
Link to section 'Step 5: Submit a bash job' of 'Build your own VASP 6' Step 5: Submit a bash job
To submit a bash job with your own compiled VASP on Negishi, here is an example about how to set up your environment and launch MPI code.
#!/bin/bash
#SBATCH -A myqueuename # Queue name(use 'slist' command to find queues' name)
#SBATCH --nodes=1 # Total # of nodes
#SBATCH --ntasks=64 # Total # of MPI tasks
#SBATCH --time=1:00:00 # Total run time limit (hh:mm:ss)
#SBATCH -J myjobname # Job name
#SBATCH -o myjob.o%j # Name of stdout output file
#SBATCH -e myjob.e%j # Name of stderr error file
# Manage processing environment,load compilers and applications.
module purge
module load gcc/12.2.0 openmpi/4.1.4 intel-mkl/2019.9.304
module list
export PATH=/path/to/vasp-build-folder/vasp.x.x.x/bin:$PATH
# Launch MPI code
mpirun -np $SLURM_NTASKS vasp_std
Frequently Asked Questions
Some common questions, errors, and problems are categorized below. Click the Expand Topics link in the upper right to see all entries at once. You can also use the search box above to search the user guide for any issues you are seeing.
About Negishi
Frequently asked questions about Negishi.
Can you remove me from the Negishi mailing list?
Your subscription in the Negishi mailing list is tied to your account on Negishi. If you are no longer using your account on Negishi, your account can be deleted from the My Accounts page. Hover over the resource you wish to remove yourself from and click the red 'X' button. Your account and mailing list subscription will be removed overnight. Be sure to make a copy of any data you wish to keep first.
How is Negishi different than other Community Clusters?
Negishi differs from the previous Community Clusters in several significant aspects:
- Host naming convention in the Negishi cluster is different from earlier Community Clusters. Everything Negishi-related is contained within a
negishi.rcac.purdue.edu
subdomain. Front-end login nodes are now namedloginNN
(as opposed to earlier<cluster>-feNN
), and compute nodes of each typeX
are namedxNNN
(as opposed to<cluster>-xNNN
). - Negishi OnDemand Gateway is at the gateway.negishi.rcac.purdue.edu (as opposed to earlier
gateway.negishi.rcac.purdue.edu
convention). - Negishi home directories are entirely separate from other Community Clusters home directories. There is no automatic copying or synchronization between the two. At their discretion, users can copy parts or all of the Community Clusters home directory into Negishi - instructions are provided.
- Negishi contains the 3rd generation of AMD EPYC processors, codenamed "Milan". These CPUs support AVX2 vector instructions set. When compiling your code, use of
-march=znver3
flag (for latest GCC, Clang and AOCC compilers) or-march=core-avx2
(for Intel compilers and GCC prior to 11.0) is recommended. - GCC compiler with OpenMPI or MVAPICH2 MPI libraries are recommended for software development on Negishi. You can enable this software with
module load gcc openmpi
(default) ormodule load gcc mvapich2
. - If you use Jupyter notebooks, JupyterHub on Negishi will be available only via the OnDemand Gateway rather than the freestanding version as on some previous systems. Other RCAC systems will transition to OnDemand as well, following Negishi.
Link to section 'Upcoming 2023' of 'How is Negishi different than other Community Clusters?' Upcoming 2023
- A subset of Negishi compute nodes contain AMD Radeon Instinct MI210 accelerator cards which can significantly improve performance of compute-intensive workloads. These can be utilized by submitting jobs to the gpu queue (add -A gpu to your job submission command).
- A selection of GPU-enabled ROCm application containers from the AMD InfinityHub collection is installed.
Do I need to do anything to my firewall to access Negishi?
No firewall changes are needed to access Negishi. However, to access data through Network Drives (i.e., CIFS, "Z: Drive"), you must be on a Purdue campus network or connected through VPN.
Does Negishi have the same home directory as other clusters?
The Negishi home directory and its contents are exclusive to Negishi cluster front-end hosts and compute nodes. This home directory is not available on other RCAC machines but Negishi. There is no automatic copying or synchronization between home directories.
At your discretion you can manually copy all or parts of your main research computing home to Negishi using one of the suggested methods.
If you plan to use hsi
or htar
commands to access Fortress tape archive from Negishi, please see also the keytab generation question for a temporary workaround to a potential caveat, while a permanent mitigation is being developed.
Logging In & Accounts
Frequently asked questions about logging in & accounts.
Errors
Common errors and solutions/work-arounds for them.
/usr/bin/xauth: error in locking authority file
Link to section 'Problem' of '/usr/bin/xauth: error in locking authority file' Problem
I receive this message when logging in:
/usr/bin/xauth: error in locking authority file
Link to section 'Solution' of '/usr/bin/xauth: error in locking authority file' Solution
Your home directory disk quota is full. You may check your quota with myquota
.
You will need to free up space in your home directory.
ncdu
command is a convenient interactive tool to examine disk usage. Consider running ncdu $HOME
to analyze where the bulk of the usage is. With this knowledge, you could then archive your data elsewhere (e.g. your research group's Data Depot space, or Fortress tape archive), or delete files you no longer need.
There are several common locations that tend to grow large over time and are merely cached downloads. The following are safe to delete if you see them in the output of ncdu $HOME
:
/home/myusername/.local/share/Trash
/home/myusername/.cache/pip
/home/myusername/.conda/pkgs
/home/myusername/.singularity/cache
My SSH connection hangs
Link to section 'Problem' of 'My SSH connection hangs' Problem
Your console hangs while trying to connect to a RCAC Server.
Link to section 'Solution' of 'My SSH connection hangs' Solution
This can happen due to various reasons. Most common reasons for hanging SSH terminals are:
- Network: If you are connected over wifi, make sure that your Internet connection is fine.
- Busy front-end server: When you connect to a cluster, you SSH to one of the front-end login nodes. Due to transient user loads, one or more of the front-ends may become unresponsive for a short while. To avoid this, try reconnecting to the cluster or wait until the login node you have connected to has reduced load.
- File system issue: If a server has issues with one or more of the file systems (
home
,scratch
, ordepot
) it may freeze your terminal. To avoid this you can connect to another front-end.
If neither of the suggestions above work, please contact support specifying the name of the server where your console is hung.
Thinlinc session frozen
Link to section 'Problem' of 'Thinlinc session frozen' Problem
Your Thinlinc session is frozen and you can not launch any commands or close the session.
Link to section 'Solution' of 'Thinlinc session frozen' Solution
This can happen due to various reasons. The most common reason is that you ran something memory-intensive inside that Thinlinc session on a front-end, so parts of the Thinlinc session got killed by Cgroups, and the entire session got stuck.
- If you are using a web-version Thinlinc remote desktop (inside the browser):
The web version does not have the capability to kill the existing session, only the standalone client does. Please install the standalone client and follow the steps below:
- If you are using a Thinlinc client:
Close the ThinLinc client, reopen the client login popup, and select
End existing session
.Select "End existing session" and try "Connect" again.
Thinlinc session unreachable
Link to section 'Problem' of 'Thinlinc session unreachable' Problem
When trying to login to Thinlinc and re-connect to your existing session, you receive an error "Your Thinlinc session is currently unreachable".
Link to section 'Solution' of 'Thinlinc session unreachable' Solution
This can happen if the specific login node your existing remote desktop session was residing on is currently offline or down, so Thinlinc can not reconnect to your existing session. Most often the session is non-recoverable at this point, so the solution is to terminate your existing Thinlinc desktop session and start a new one.
- If you are using a web-version Thinlinc remote desktop (inside the browser):
The web version does not have the capability to kill the existing session, only the standalone client does. Please install the standalone client and follow the steps below:
- If you are using a Thinlinc client:
Close the ThinLinc client, reopen the client login popup, and select
End existing session
.Select "End existing session" and try "Connect" again.
How to disable Thinlinc screensaver
Link to section 'Problem' of 'How to disable Thinlinc screensaver' Problem
Your ThinLinc desktop is locked after being idle for a while, and it asks for a password to refresh it. It means the "screensaver" and "lock screen" functions are turned on, but you want to disable these functions.
Link to section 'Solution' of 'How to disable Thinlinc screensaver' Solution
If your screen is locked, close the ThinLinc client, reopen the client login popup, and select End existing session
.
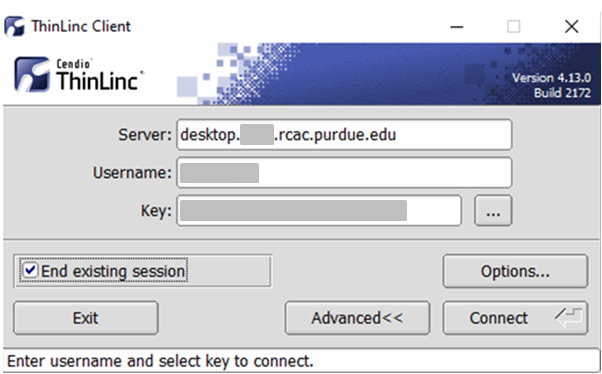
To permanently avoid screen lock issue, right click desktop and select Applications
, then settings
, and select Screensaver
.
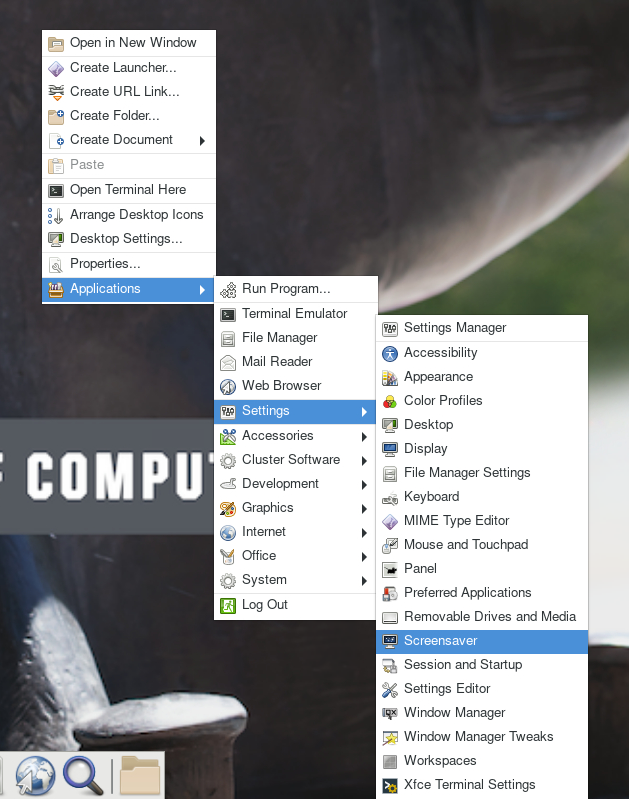
Under Screensaver, turn off the Enable Screensaver
, then under Lock Screen, turn off the Enable Lock Screen
, and close the window.
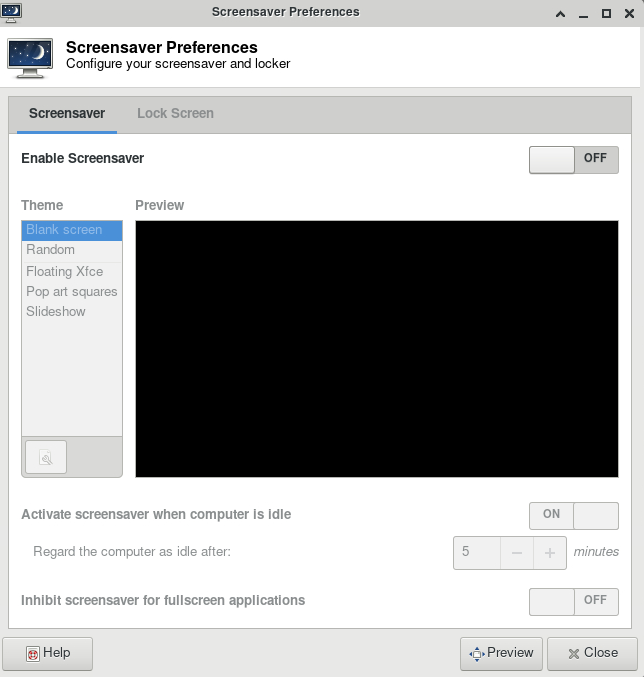
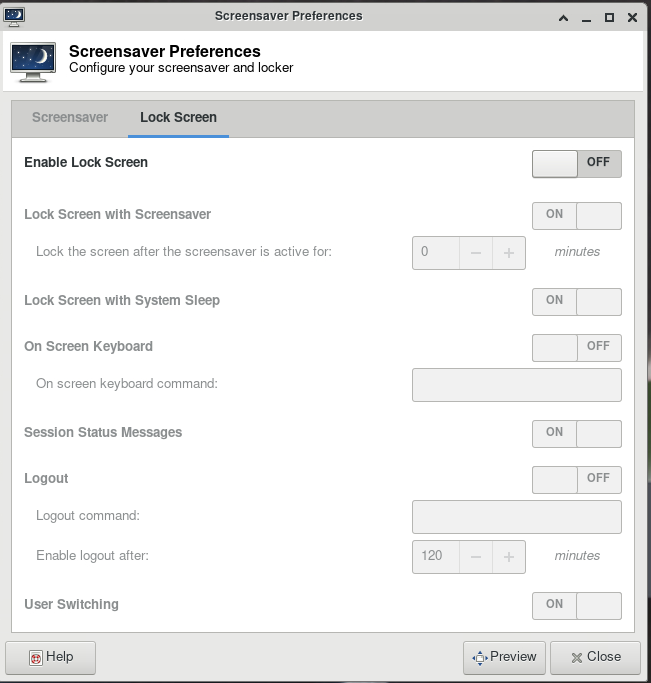
Questions
Frequently asked questions about logging in & accounts.
I worked on Negishi after I graduated/left Purdue, but can not access it anymore
Link to section 'Problem' of 'I worked on Negishi after I graduated/left Purdue, but can not access it anymore' Problem
You have graduated or left Purdue but continue collaboration with your Purdue colleagues. You find that your access to Purdue resources has suddenly stopped and your password is no longer accepted.
Link to section 'Solution' of 'I worked on Negishi after I graduated/left Purdue, but can not access it anymore' Solution
Access to all resources depends on having a valid Purdue Career Account. Expired Career Accounts are removed twice a year, during Spring and October breaks (more details at the official page). If your Career Account was purged due to expiration, you will not be be able to access the resources.
To provide remote collaborators with valid Purdue credentials, the University provides a special procedure called Request for Privileges (R4P). If you need to continue your collaboration with your Purdue PI, the PI will have to submit or renew an R4P request on your behalf.
After your R4P is completed and Career Account is restored, please note two additional necessary steps:
-
Access: Restored Career Accounts by default do not have any RCAC resources enabled for them. Your PI will have to login to the Manage Users tool and explicitly re-enable your access by un-checking and then ticking back checkboxes for desired queues/Unix groups resources.
-
Email: Restored Career Accounts by default do not have their @purdue.edu email service enabled. While this does not preclude you from using RCAC resources, any email messages (be that generated on the clusters, or any service announcements) would not be delivered - which may cause inconvenience or loss of compute jobs. To avoid this, we recommend setting your restored @purdue.edu email service to "Forward" (to an actual address you read). The easiest way to ensure it is to go through the Account Setup process.
Jobs
Frequently asked questions related to running jobs.
Errors
Common errors and potential solutions/workarounds for them.
cannot connect to X server / cannot open display
Link to section 'Problem' of 'cannot connect to X server / cannot open display' Problem
You receive the following message after entering a command to bring up a graphical window
cannot connect to X server
cannot open display
Link to section 'Solution' of 'cannot connect to X server / cannot open display' Solution
This can happen due to multiple reasons:
- Reason: Your SSH client software does not support graphical display by itself (e.g. SecureCRT or PuTTY).
- Solution: Try using a client software like Thinlinc or MobaXterm as described in the SSH X11 Forwarding guide.
-
Reason: You did not enable X11 forwarding in your SSH connection.
-
Solution: If you are in a Windows environment, make sure that X11 forwarding is enabled in your connection settings (e.g. in MobaXterm or PuTTY). If you are in a Linux environment, try
ssh -Y -l username hostname
-
- Reason: If you are trying to open a graphical window within an interactive PBS job, make sure you are using the
-X
option withqsub
after following the previous step(s) for connecting to the front-end. Please see the example in the Interactive Jobs guide. - Reason: If none of the above apply, make sure that you are within quota of your home directory.
bash: command not found
Link to section 'Problem' of 'bash: command not found' Problem
You receive the following message after typing a command
bash: command not found
Link to section 'Solution' of 'bash: command not found' Solution
This means the system doesn't know how to find your command. Typically, you need to load a module to do it.
bash: module command not found
Link to section 'Problem' of 'bash: module command not found' Problem
You receive the following message after typing a command, e.g. module load intel
bash: module command not found
Link to section 'Solution' of 'bash: module command not found' Solution
The system cannot find the module command. You need to source the modules.sh file as below
source /etc/profile.d/modules.sh
or
#!/bin/bash -i
Close Firefox / Firefox is already running but not responding
Link to section 'Problem' of 'Close Firefox / Firefox is already running but not responding' Problem
You receive the following message after trying to launch Firefox browser inside your graphics desktop:
Close Firefox
Firefox is already running, but not responding. To open a new window,
you must first close the existing Firefox process, or restart your system.
Link to section 'Solution' of 'Close Firefox / Firefox is already running but not responding' Solution
When Firefox runs, it creates several lock files in the Firefox profile directory (inside ~/.mozilla/firefox/
folder in your home directory). If a newly-started Firefox instance detects the presence of these lock files, it complains.
This error can happen due to multiple reasons:
- Reason: You had a single Firefox process running, but it terminated abruptly without a chance to clean its lock files (e.g. the job got terminated, session ended, node crashed or rebooted, etc).
- Solution: If you are certain you do not have any other Firefox processes running elsewhere, please use the following command in a terminal window to detect and remove the lock files:
$ unlock-firefox
- Solution: If you are certain you do not have any other Firefox processes running elsewhere, please use the following command in a terminal window to detect and remove the lock files:
- Reason: You may indeed have another Firefox process (in another Thinlinc or Gateway session on this or other cluster, another front-end or compute node). With many clusters sharing common home directory, a running Firefox instance on one can affect another.
- Solution: Try finding and closing running Firefox process(es) on other nodes and clusters.
- Solution: If you must have multiple Firefoxes running simultaneously, you may be able to create separate Firefox profiles and select which one to use for each instance.
Jupyter: database is locked / can not load notebook format
Link to section 'Problem' of 'Jupyter: database is locked / can not load notebook format' Problem
You receive the following message after trying to load existing Jupyter notebooks inside your JupyterHub session:
Error loading notebook
An unknown error occurred while loading this notebook. This version can load notebook formats or earlier. See the server log for details.
Alternatively, the notebook may open but present an error when creating or saving a notebook:
Autosave Failed!
Unexpected error while saving file: MyNotebookName.ipynb database is locked
Link to section 'Solution' of 'Jupyter: database is locked / can not load notebook format' Solution
When Jupyter notebooks are opened, the server keeps track of their state in an internal database (located inside ~/.local/share/jupyter/
folder in your home directory). If a Jupyter process gets terminated abruptly (e.g. due to an out-of-memory error or a host reboot), the database lock is not cleared properly, and future instances of Jupyter detect the lock and complain.
Please follow these steps to resolve:
- Fully exit from your existing Jupyter session (close all notebooks, terminate Jupyter, log out from JupyterHub or JupyterLab, terminate OnDemand gateway's Jupyter app, etc).
- In a terminal window (SSH, Thinlinc or OnDemand gateway's terminal app) use the following command to clean up stale database locks:
$ unlock-jupyter
- Start a new Jupyter session as usual.
Questions
Frequently asked questions about jobs.
How do I know Non-uniform Memory Access (NUMA) layout on Negishi?
- You can learn about processor layout on Negishi nodes using the following command:
a003.negishi:~$ lstopo-no-graphics
- For detailed IO connectivity:
a003.negishi:~$ lstopo-no-graphics --physical --whole-io
- Please note that NUMA information is useful for advanced MPI/OpenMP/GPU optimizations. For most users, using default NUMA settings in MPI or OpenMP would give you the best performance.
Why cannot I use --mem=0 when submitting jobs?
Link to section 'Question' of 'Why cannot I use --mem=0 when submitting jobs?' Question
Why can't I specify --mem=0
for my job?
Link to section 'Answer' of 'Why cannot I use --mem=0 when submitting jobs?' Answer
We no longer support requesting unlimited memory (--mem=0
) as it has an adverse effect on the way scheduler allocates job, and could lead to large amount of nodes being blocked from usage.
Most often we suggest relying on default memory allocation (cluster-specific). But if you have to request custom amounts of memory, you can do it explicitly. For example --mem=20G
.
If you want to use the entire node's memory, you can submit the job with the --exclusive
option.
Can I extend the walltime on a job?
In some circumstances, yes. Walltime extensions must be requested of and completed by staff. Walltime extension requests will be considered on named (your advisor or research lab) queues. Standby or debug queue jobs cannot be extended.
Extension requests are at the discretion of staff based on factors such as any upcoming maintenance or resource availability. Extensions can be made past the normal maximum walltime on named queues but these jobs are subject to early termination should a conflicting maintenance downtime be scheduled.
Please be mindful of time remaining on your job when making requests and make requests at least 24 hours before the end of your job AND during business hours. We cannot guarantee jobs will be extended in time with less than 24 hours notice, after-hours, during weekends, or on a holiday.
We ask that you make accurate walltime requests during job submissions. Accurate walltimes will allow the job scheduler to efficiently and quickly schedule jobs on the cluster. Please consider that extensions can impact scheduling efficiency for all users of the cluster.
Requests can be made by contacting support. We ask that you:
- Provide numerical job IDs, cluster name, and your desired extension amount.
- Provide at least 24 hours notice before job will end (more if request is made on a weekend or holiday).
- Consider making requests during business hours. We may not be able to respond in time to requests made after-hours, on a weekend, or on a holiday.
Data
Frequently asked questions about data and data management.
How is my Data Secured on Negishi?
Negishi is operated in line with policies, standards, and best practices as described within Secure Purdue, and specific to RCAC Resources.
Security controls for Negishi are based on ones defined in NIST cybersecurity standards.
Negishi supports research at the L1 fundamental and L2 sensitive levels. Negishi is not approved for storing data at the L3 restricted (covered by HIPAA) or L4 Export Controlled (ITAR), or any Controlled Unclassified Information (CUI).
For resources designed to support research with heightened security requirements, please look for resources within the REED+ Ecosystem.
Link to section 'For additional information' of 'How is my Data Secured on Negishi?' For additional information
Log in with your Purdue Career Account.
Can I share data with outside collaborators?
Yes! Globus allows convenient sharing of data with outside collaborators. Data can be shared with collaborators' personal computers or directly with many other computing resources at other institutions. See the Globus documentation on how to share data:
Does Negishi have the same home directory as other clusters?
The Negishi home directory and its contents are exclusive to Negishi cluster front-end hosts and compute nodes. This home directory is not available on other RCAC machines but Negishi. There is no automatic copying or synchronization between home directories.
At your discretion you can manually copy all or parts of your main research computing home to Negishi using one of the suggested methods.
If you plan to use hsi
or htar
commands to access Fortress tape archive from Negishi, please see also the keytab generation question for a temporary workaround to a potential caveat, while a permanent mitigation is being developed.
HSI/HTAR: Unable to authenticate user with remote gateway (error 2 or 9)
There could be a variety of such errors, with wordings along the lines of
Could not initialize keytab on remote server.
result = -2, errno = 2rver connection
*** hpssex_OpenConnection: Unable to authenticate user with remote gateway at 128.211.138.40.1217result = -2, errno = 9
Unable to setup communication to HPSS...
ERROR (main) unable to open remote gateway server connection
HTAR: HTAR FAILED
and
*** hpssex_OpenConnection: Unable to authenticate user with remote gateway at 128.211.138.40.1217result = -11000, errno = 9
Unable to setup communication to HPSS...
*** HSI: error opening logging
Error - authentication/initialization failed
The root cause for these errors is an expired or non-existent keytab file (a special authentication token stored in your home directory). These keytabs are valid for 90 days and on most RCAC resources they are usually automatically checked and regenerated when you execute hsi
or htar
commands. However, if the keytab is invalid, or fails to generate, Fortress may be unable to authenticate you and you would see the above errors. This is especially common on those RCAC clusters that have their own dedicated home directories (such as Bell), or on standalone installations (such as if you downloaded and installed HSI and HTAR on your non-RCAC computer).
This is a temporary problem and a permanent system-wide solution is being developed. In the interim, the recommended workaround is to generate a new valid keytab file in your main research computing home directory, and then copy it to your home directory on Negishi. The fortresskey
command is used to generate the keytab and can be executed on another cluster or a dedicated data management host data.rcac.purdue.edu
:
$ ssh myusername@data.rcac.purdue.edu fortresskey
$ scp -pr myusername@data.rcac.purdue.edu:~/.private $HOME
With a valid keytab in place, you should then be able to use hsi
and htar
commands to access Fortress from Negishi. Note that only one keytab can be valid at any given time (i.e. if you regenerated it, you may have to copy the new keytab to all systems that you intend to use hsi
or htar
from if they do not share the main research computing home directory).
Can I access Fortress from Negishi?
Yes. While Fortress directories are not directly mounted on Negishi for performance and archival protection reasons, they can be accessed from Negishi front-ends and nodes using any of the recommended methods of HSI, HTAR or Globus.
Software
Frequently asked questions about software.
Cannot use pip after loading ml-toolkit modules
Link to section 'Question' of 'Cannot use pip after loading ml-toolkit modules' Question
Pip throws an error after loading the machine learning modules. How can I fix it?
Link to section 'Answer' of 'Cannot use pip after loading ml-toolkit modules' Answer
Machine learning modules (tensorflow, pytorch, opencv etc.) include a version of pip that is newer than the one installed with Anaconda. As a result it will throw an error when you try to use it.
$ pip --version
Traceback (most recent call last):
File "/apps/cent7/anaconda/5.1.0-py36/bin/pip", line 7, in <module>
from pip import main
ImportError: cannot import name 'main'
The preferred way to use pip with the machine learning modules is to invoke it via Python as shown below.
$ python -m pip --version
How can I get access to Sentaurus software?
Link to section 'Question' of 'How can I get access to Sentaurus software?' Question
How can I get access to Sentaurus tools for micro- and nano-electronics design?
Link to section 'Answer' of 'How can I get access to Sentaurus software?' Answer
Sentaurus software license requires a signed NDA. Please contact Dr. Mark Johnson, Director of ECE Instructional Laboratories to complete the process.
Once the licensing process is complete and you have been added into a cae2
Unix group, you could use Sentaurus on RCAC community clusters by loading the corresponding environment module:
module load sentaurus
Julia package installation
Users do not have write permission to the default julia package installation destination. However, users can install packages into home directory under ~/.julia
.
Users can side step this by explicitly defining where to put julia packages:
$ export JULIA_DEPOT_PATH=$HOME/.julia
$ julia -e 'using Pkg; Pkg.add("PackageName")'
About Research Computing
Frequently asked questions about RCAC.
Can I get a private server from RCAC?
Link to section 'Question' of 'Can I get a private server from RCAC?' Question
Can I get a private (virtual or physical) server from RCAC?
Link to section 'Answer' of 'Can I get a private server from RCAC?' Answer
Often, researchers may want a private server to run databases, web servers, or other software. RCAC currently has Geddes, a Community Composable Platform optimized for composable, cloud-like workflows that are complementary to the batch applications run on Community Clusters. Funded by the National Science Foundation under grant OAC-2018926, Geddes consists of Dell Compute nodes with two 64-core AMD Epyc 'Rome' processors (128 cores per node).
To purchase access to Geddes today, go to the Cluster Access Purchase page. Please subscribe to our Community Cluster Program Mailing List to stay informed on the latest purchasing developments or contact us (rcac-cluster-purchase@lists.purdue.edu) if you have any questions.
Datasets
Please refer to our Federated Datasets Documentation website for up-to-date datasets on Anvil and instructions on how to use them.
Geddes User Guide
New usage patterns have emerged in research computing that depend on the availability of custom services such as notebooks, databases, elastic software stacks, and science gateways alongside traditional batch HPC. The Geddes Composable Platform is a Kubernetes based private cloud managed with Rancher that provides a platform for creating composable infrastructure on demand. This cloud-style flexibility provides researchers the ability to self-deploy and manage persistent services to complement HPC workflows and run container-based data analysis tools and applications. Funded by the National Science Foundation under grant OAC-2018926, Geddes consists of Dell compute nodes with two 64-core AMD Epyc 'Rome' processors (128 cores per node).
Link to section 'Overview of Geddes' of 'Overview of Geddes' Overview of Geddes
Geddes is a Community Composable Platform optimized for composable, cloud-like workflows that are complementary to the batch applications run on Community Clusters. Funded by the National Science Foundation under grant OAC-2018926, Geddes consists of Dell Compute nodes with two 64-core AMD Epyc 'Rome' processors (128 cores per node).
To purchase access to Geddes today, go to the Cluster Access Purchase page. Please subscribe to our Community Cluster Program Mailing List to stay informed on the latest purchasing developments or contact us if you have any questions.
Link to section 'Geddes Namesake' of 'Overview of Geddes' Geddes Namesake
Geddes is named in honor of Lanelle Geddes, Professor of Nursing. More information about her life and impact on Purdue is available in a Biography of Geddes.
Link to section 'Geddes Specifications' of 'Overview of Geddes' Geddes Specifications
All Geddes compute nodes have 128 processor cores and 100 Gbps Infiniband interconnects.
Worker Type | Number of Nodes | Processors per Node | Cores per Node | Storage per Node | Memory per Node | Retires in |
---|---|---|---|---|---|---|
A | 8 | Two AMD Epyc CPUs @ 2.0GHz | 128 | 24 TB SATA SSD | 1 TB | 2026 |
B | 16 | Two AMD Epyc CPUs @ 2.0GHz | 128 | 24 TB SATA SSD | 512 GB | 2026 |
Number of Nodes | Processors per Node | Cores per Node | GPUs per Node | Storage per Node | Memory per Node | Retires in |
---|---|---|---|---|---|---|
4 | Two AMD Epyc CPUs @ 2.0GHz | 128 | 2 Nvidia A100 | 24 TB SATA SSD | 512 GB | 2026 |
Number of Nodes | Processors per Node | Cores per Node | Storage per Node | Memory per Node | Retires in |
---|---|---|---|---|---|
8 | Two Intel Xeon Gold 6126 | 24 | 24 TB NVMe | 192 GB | 2026 |
Geddes nodes run Rocky 8 and use Rancher and Kubernetes as the resource manager for resource and workload orchestration.
Biography of Lanelle Geddes
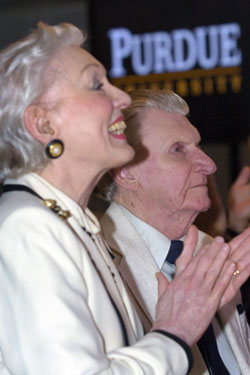
LaNelle E. (Nerger) Geddes was born on September 15, 1935 in Houston, Texas to Carl O. and Evelyn Nerger. She received a B.S. in Nursing from the University of Houston in 1957, and earned a PhD in Biophysics there in 1970. After receiving her PhD, Geddes taught at the Texas Women's University and in the Department of Physiology at Baylor College of Medicine in Houston.
Geddes joined the faculty at Purdue in 1975 where her husband, Leslie A. Geddes, was the head of the Department of Biomedical Engineering. Lanelle started in the School of Nursing as the Assistant Head of the Department. In 1980, she was promoted to the Head of the Department and served as Head until 1991. While at Purdue, Geddes challenged traditional perceptions of nurses as merely doctors' assistants who were wrongly believed to have no expertise or skill for diagnosis and treatment. In addition, Geddes was also instrumental in instituting a four-year nursing baccalaureate program and starting the Freshman Scholars, a program that provided scholarships to outstanding incoming freshman.
Geddes' research was focused on cardiovascular physiology. Her teaching emphasized the impact of human pathophysiologic alterations and their influence on nursing and medical care. Her inclusion of pathophysiology encouraged her students to make better clinical judgments and to be stronger patient advocates. From 1996-2003, Geddes also taught pathophysiology to IU School of Medicine students at Purdue University. Geddes retired as a professor emeritus in 2003.
Geddes' research and teaching had far-reaching impacts, and she received many awards over the course of her career. She was an AMOCO Foundation (Murphy) Award winner, a fellow of the University Teaching Academy, and a Helen B Schleman Gold Medallion Awardee. She also received the Lafayette YWCA Salute to Women Award and the Westminster Village Lifetime Service Award for her work within the community. Geddes passed away on January 25, 2016.
Link to section 'Citations' of 'Biography of Lanelle Geddes' Citations
Archives and Special Collections. (2021, May 19). Geddes, Lanelle E., September 15, 1935 - January 25, 2016. Purdue University. Retrieved from: https://archives.lib.purdue.edu/agents/people/802
https://earchives.lib.purdue.edu/digital/collection/oralhist/id/31
Concepts
Link to section 'Containers & Images' of 'Concepts' Containers & Images
Image - An image is a simple text file that defines the source code of an application you want to run as well as the libraries, dependencies, and tools required for the successful execution of the application. Images are immutable meaning they do not hold state or application data. Images represent a software environment at a specific point of time and provide an easy way to share applications across various environments. Images can be built from scratch or downloaded from various repositories on the internet, additionally many software vendors are now providing containers alongside traditional installation packages like Windows .exe and Linux rpm/deb.
Container - A container is the run-time environment constructed from an image when it is executed or run in a container runtime. Containers allow the user to attach various resources such as network and volumes in order to move and store data. Containers are similar to virtual machines in that they can be attached to when a process is running and have arbitrary commands executed that affect the running instance. However, unlike virtual machines, containers are more lightweight and portable allowing for easy sharing and collaboration as they run identically in all environments.
Tags - Tags are a way of organizing similar image files together for ease of use. You might see several versions of an image represented using various tags. For example, we might be building a new container to serve web pages using our favorite web server: nginx. If we search for the nginx container on Docker Hub image repository we see many options or tags are available for the official nginx container.
The most common you will see are typically :latest
and :number
where
number refers to the most recent few versions of the software releases. In
this example we can see several tags refer to the same image:
1.21.1, mainline, 1, 1.21,
and latest
all reference the same image while the
1.20.1, stable, 1.20
tags all reference a common but different image.
In this case we likely want the nginx image with either the latest
or 1.21.1
tag represented as nginx:latest
and nginx:1.21.1
respectively.
Container Security - Containers enable fast developer velocity and ease compatibility through great portability, but the speed and ease of use come at some costs. In particular it is important that folks utilizing container driver development practices have a well established plan on how to approach container and environment security. Best Practices
Container Registries - Container registries act as large repositories of images, containers, tools and surrounding software to enable easy use of pre-made containers software bundles. Container registries can be public or private and several can be used together for projects. Docker Hub is one of the largest public repositories available, and you will find many official software images present on it. You need a user account to avoid being rate limited by Docker Hub. A private container registry based on Harbor that is available to use. TODO: link to harbor instructions
Docker Hub - Docker Hub is one of the largest container image registries that exists and is well known and widely used in the container community, it serves as an official location of many popular software container images. Container image repositories serve as a way to facilitate sharing of pre-made container images that are “ready for use.” Be careful to always pay attention to who is publishing particular images and verify that you are utilizing containers built only from reliable sources.
Harbor - Harbor is an open source registry for Kubernetes artifacts, it provides private image storage and enforces container security by vulnerability scanning as well as providing RBAC or role based access control to assist with user permissions. Harbor is a registry similar to Docker Hub, however it gives users the ability to create private repositories. You can use this to store your private images as well as keeping copies of common resources like base OS images from Docker Hub and ensure your containers are reasonably secure from common known vulnerabilities.
Link to section 'Container Runtime Concepts' of 'Concepts' Container Runtime Concepts
Docker Desktop - Docker Desktop is an application for your Mac / Windows machine that will allow you to build and run containers on your local computer. Docker desktop serves as a container environment and enables much of the functionality of containers on whatever machine you are currently using. This allows for great flexibility, you can develop and test containers directly on your laptop and deploy them directly with little to no modifications.
Volumes - Volumes provide us with a method to create persistent data that is generated and consumed by one or more containers. For docker this might be a folder on your laptop while on a large Kubernetes cluster this might be many SSD drives and spinning disk trays. Any data that is collected and manipulated by a container that we want to keep between container restarts needs to be written to a volume in order to remain around and be available for later use.
Link to section 'Container Orchestration Concepts' of 'Concepts' Container Orchestration Concepts
Container Orchestration - Container orchestration broadly means the automation of much of the lifecycle management procedures surrounding the usage of containers. Specifically it refers to the software being used to manage those procedures. As containers have seen mass adoption and development in the last decade, they are now being used to power massive environments and several options have emerged to manage the lifecycle of containers. One of the industry leading options is Kubernetes, a software project that has descended from a container orchestrator at Google that was open sourced in 2015.
Kubernetes (K8s) - Kubernetes (often abbreviated as "K8s") is a platform providing container orchestration functionality. It was open sourced by Google around a decade ago and has seen widespread adoption and development in the ensuing years. K8s is the software that provides the core functionality of the Anvil Composable Subsystem by managing the complete lifecycle of containers. Additionally it provides the following functions: service discovery and load balancing, storage orchestration, secret and configuration management. The Kubernetes cluster can be accessed via the Rancher UI or the kubectl command line tool.
Rancher - Rancher is a “is a complete software stack for teams adopting containers.” as described by its website. It can be thought of as a wrapper around Kubernetes, providing an additional set of tools to help operate the K8 cluster efficiently and additional functionality that does not exist in Kubernetes itself. Two examples of the added functionality is the Rancher UI that provides an easy to use GUI interface in a browser and Rancher projects, a concept that allows for multi-tenancy within the cluster. Users can interact directly with Rancher using either the Rancher UI or Rancher CLI to deploy and manage workloads on the Anvil Composable Subsystem.
Rancher UI - The Rancher UI is a web based graphical interface to use the Anvil Composable Subsystem from anywhere.
Rancher CLI - The Rancher CLI provides a convenient text based toolkit to interact with the cluster. The binary can be downloaded from the link on the right hand side of the footer in the Rancher UI. After you download the Rancher CLI, you need to make a few configurations Rancher CLI requires:
-
Your Rancher Server URL, which is used to connect to Rancher Server.
-
An API Bearer Token, which is used to authenticate with Rancher. see Creating an API Key.
After setting up the Rancher CLI you can issue rancher --help
to view the full
range of options available.
Kubectl - Kubectl is a text based tool for working with the underlying Anvil Kubernetes cluster. In order to take advantage of kubectl you will either need to set up a Kubeconfig File or use the built in kubectl shell in the Rancher UI. You can learn more about kubectl and how to download the kubectl file here.
Storage - Storage is utilized to provide persistent data storage between container deployments. The Ceph filesystem provides access to Block, Object and shared file systems. File storage provides an interface to access data in a file and folder hierarchy similar to NTFS or NFS. Block storage is a flexible type of storage that allows for snapshotting and is good for database workloads and generic container storage. Object storage is also provided by Ceph, this features a REST based bucket file system providing S3 and Swift compatibility.
Access
Access to the Geddes Composable Platform is handled via the RCAC web portal. When access is purchased, a Rancher project with your research group's name will be created and managers will be able to click to give users access, similar to how access is managed for community clusters. Links to access Geddes via the Rancher UI and the command line (kubectl) are below.
Rancher
Link to section 'Logging in to Rancher' of 'Rancher' Logging in to Rancher
To access the Geddes user interface, you must be on a Purdue campus network or connected through VPN.
Once connected to a Purdue network, the Geddes Rancher interface can be accessed via a web browser at https://geddes.rcac.purdue.edu. Log in by choosing "log in with shibboleth" and using Purdue Login at the login screen.
Kubectl
Link to section 'Configuring local kubectl access with Kubeconfig file' of 'Kubectl' Configuring local kubectl access with Kubeconfig file
kubectl can be installed and ran on your local machine to perform various actions against the Kubernetes cluster using the API server.
These tools authenticate to Kubernetes using information stored in a kubeconfig file.
To begin accessing Geddes via Kubectl you must first gather your rancher generated Kubeconfig file and set up your local .kube
directory
- From anywhere in the Rancher UI navigate to the top right and click on either Download KubeConfig or Copy KubeConfig to Clipboard
- Create a directory in your home directory (
$HOME
) called.kube
- Change into the newly created directory and copy the file or contents of KubeConfig from earlier into a file called config
- Create a directory in your home directory (
- Test connections to the Geddes cluster
- To look at the current config settings we just set use
$ kubectl config view
- Now let’s list the available resource types present in the API with
$ kubectl api-resources
- To look at the current config settings we just set use
To see more options of kubectl, review the Kubernetes' kubectl cheatsheet.
Link to section 'Accessing kubectl in the rancher web UI' of 'Kubectl' Accessing kubectl in the rancher web UI
You can launch a kubectl command window from within the Rancher UI by selecting
the Kubectl Shell button at the top right or using the hotkey (CTL + `
).
This will deploy a container in the cluster with kubectl installed and give you
an interactive window to use the command from.
Registry
Link to section 'Accessing the Geddes Harbor Registry' of 'Registry' Accessing the Geddes Harbor Registry
The Geddes Harbor registry is only accessible via campus networks and the Purdue VPN. Use a web browser navigate to geddes-registry.rcac.purdue.edu and log in with your Purdue career account username and password. (Do not add ",push
" to your password nor use the Purdue Duo client.)
Link to section 'Using the Geddes Registry Docker Hub Cache' of 'Registry' Using the Geddes Registry Docker Hub Cache
It's advised that you use the Docker Hub cache within Geddes to pull images for your deployments. There's a limit to how many images Docker hub will allow to be pulled within a 24 hour period which Geddes does reach depending on user activity. This means if you're trying to deploy a workload without the cache, or have a currently deployed workload that needs migrated, restarted, or upgraded without the cache, there's a chance it will fail.
To bypass this, use the geddes cache url geddes-registry.rcac.purdue.edu/docker-hub-cache/
in your image names.
For example if you're wanting to pull a notebook from jupyterhub's Docker Hub repo e.g jupyter/tensorflow-notebook:latest
Pulling it from the Geddes cache would look like this geddes-registry.rcac.purdue.edu/docker-hub-cache/jupyter/tensorflow-notebook:latest
.
If the image you are using is an "Official" docker image (like httpd or mongo) the URL will use the "library" in the path instead of the Docker repository name: geddes-registry.rcac.purdue.edu/docker-hub-cache/library/mongo:latest
.
Link to section 'Creating a registry' of 'Registry' Creating a registry
- Using a browser login to geddes-registry.rcac.purdue.edu with your Purdue account username and password
- From the main page click create project, this will act as your registry
- Fill in a name and select whether you want the project to be public or private
- Click ok to create and finalize
Link to section 'Tagging and Pushing Images to Your Harbor Registry' of 'Registry' Tagging and Pushing Images to Your Harbor Registry
- Tag your image
$ docker tag my-image:tag geddes-registry.rcac.purdue.edu/project-registry/my-image:tag
- login to the Geddes registry via command line
$ docker login geddes-registry.rcac.purdue.edu
- Push your image to your project registry
$ docker push geddes-registry.rcac.purdue.edu/project-registry/my-image:tag
Link to section 'Creating a Robot Account for a Private Registry' of 'Registry' Creating a Robot Account for a Private Registry
A robot account and token can be used to authenticate to your registry in place of having to supply or store your private credentials on multi-tenant cloud environments like rancher/Geddes.
- Navigate to your project by logging into geddes-registry.rcac.purdue.edu
- Navigate to the Robot Accounts tab and click New Robot Account
- Fill out the form
- Name your robot account
- Select account expiration if any, select never to make permanent
- Customize what permissions you wish the account to have
- Click Add
- Copy your information
- Your robot’s account name will be something longer than what you specified, since this is a multi-tenant registry, harbor does this to avoid unrelated project owners creating a similarly named robot account
- Export your token as JSON or copy it to a clipboard
NOTE: Harbor does not store account tokens, once you exit this page your token will be unrecoverable
Link to section 'Adding Your Private Registry to Rancher' of 'Registry' Adding Your Private Registry to Rancher
- Select your Project from the top right dropdown
- Using the far left dropdown menu navigate to Storage > Secrets
- Click Create
- Click Registry
- Fill out the form
- Select namespace that will have access to the registry
- Give a name to the Registry secret (this is an arbitrary name)
- Under the Data tab ensure custom is selected
- Enter "geddes-registry.rcac.purdue.edu" under Registry Domain Name
- Enter your robot account's long name eg. robot$my-registry+robot as the Username
- Enter your robot account's token as the password
- Click Create
Link to section 'External Harbor Documentation' of 'Registry' External Harbor Documentation
Workloads
Link to section 'Deploy a Workload' of 'Workloads' Deploy a Workload
- Using the top right dropdown select the Project or Namespace you wish to deploy to.
- Using the far left menu navigate to Workload
- Click Create at the top right
- Select the appropriate Deployment Type for your use case
- Select Namespace if not already done from step 1
- Set a unique Name for your deployment, i.e. “myapp"
- Set Container Image. Ensure you're using the Geddes registry for personal images or the Geddes registry docker-hub cache when
pulling public docker-hub specific images. e.g:
geddes-registry.rcac.purdue.edu/my-registry/myimage:tag
orgeddes- registry.rcac.purdue.edu/docker-hub-cache/library/image:tag
- Click Create
Services
A Service is an abstract way to expose an application running on Pods as a network service. This allows the networking and application to be logically decoupled so state changes in either the application itself or the network connecting application components do not need to be tracked individually by all portions of an application.
Link to section 'Service resources' of 'Services' Service resources
In Kubernetes, a Service is an abstraction which defines a logical set of Pods and a policy by which to access them (sometimes this pattern is called a micro-service). The set of Pods targeted by a Service is usually determined by a Pod selector, but can also be defined other ways.
Link to section 'Publishing Services (ServiceTypes)' of 'Services' Publishing Services (ServiceTypes)
For some parts of your deployment you may need to expose an application externally from the cluster using Services
Kubernetes ServiceTypes allow you to specify what kind of Service you want. The default is ClusterIP.
-
ClusterIP: Exposes the Service on a cluster-internal IP. Choosing this value makes the Service only reachable from within the cluster. This is the default ServiceType.
-
NodePort: Exposes the Service on each Node’s IP at a static port (the NodePort). A ClusterIP Service, to which the NodePort Service routes, is automatically created. You’ll be able to contact the NodePort Service, from outside the cluster, by requesting
<NodeIP>:<NodePort>
. -
LoadBalancer: Exposes the Service externally using a cloud provider’s load balancer. NodePort and ClusterIP Services, to which the external load balancer routes, are automatically created.
You can see an example of exposing a workload using the LoadBalancer type in the examples section.
Link to section 'Ingress' of 'Services' Ingress
An Ingress is an API object that manages external access to the services in a cluster, typically HTTP/HTTPS. An Ingress is not a ServiceType, but rather brings external traffic into the cluster and then passes it to an Ingress Controller to be routed to the correct location. Ingress may provide load balancing, SSL termination and name-based virtual hosting. Traffic routing is controlled by rules defined on the Ingress resource.
Link to section 'Ingress Controller' of 'Services' Ingress Controller
Geddes provides the nginx ingress controller configured to facilitate SSL termination and automatic DNS name generation under the geddes.rcac.purdue.edu
subdomain.
In the Examples section, there are detailed instructions about how to expose a service with an Ingress. Here are the outline of major steps:
- Create a new Deployment under Workload
- Set Container Image to the Docker image you want to use
- Create a Cluster IP service to point our external accessible ingress for later
- Setup Pod Label
- Create a new Ingress page
- Give the URL you would like to use for your web application under Request Host
- Put the Cluster IP you created in Step 1 to Target Service and Port
- The default Ingress is private, which is only accessible within Purdue network. To make a public one, add an Annotation:
kubernetes.io/ingress.class: "public"
Kubernetes provides additional information about Ingress Controllers in the official documentation.
Storage
Geddes has a software defined storage system that provides user-provisioned persistent data storage for container deployments.
Ceph is used to provide block, filesystem and object storage on the Geddes Composable Platform. File storage provides an interface to access data in a file and folder hierarchy similar to Data Depot. Block storage is a flexible type of storage that is good for database workloads and generic container storage. Object storage is ideal for large unstructured data and features a REST based API providing an S3 compatible endpoint that can be utilized by the preexisting ecosystem of S3 client tools.
Link to section 'Storage Classes' of 'Storage' Storage Classes
Geddes provides four different storage classes based on access characteristics and performance needs a workload. Performance classes should be used for workloads with high I/O requirements (databases, AI/ML).
geddes-standard-singlenode
- Block storage based on SSDs that can be accessed by a single node (Single-Node Read/Write).geddes-standard-multinode
- File storage based on SSDs that can be accessed by multiple nodes (Many-Node Read/Write or Many-Node Read-Only)geddes-performance-singlenode
- Block storage based on NVMe drives that can be accessed by a single node (Single-Node Read/Write).geddes-performance-multinode
- File storage based on NVMe drives that can be accessed by multiple nodes (Many-Node Read/Write or Many-Node Read-Only)
Link to section 'Block and Filesystem Storage Provisioning in Deployments' of 'Storage' Block and Filesystem Storage Provisioning in Deployments
Block and Filesystem storage can both be provisioned in a similar way.
-
While deploying a Workload, click the Storage tab and click Add Volume…
-
Select “Create Persistent Volume Claim”
-
Set a unique Persistent Volume Claim Name, i.e. “<username>-volume”
-
Select a Storage Class. The default storage class is "geddes-standard-singlenode".
-
Select an Access Mode. The "geddes-standard-singlenode" class only supports Single-Node Read/Write.
-
Request an amount of storage in Gigabytes
-
Provide a Mount Point for the persistent volume: i.e /data
Link to section 'Backup Strategies' of 'Storage' Backup Strategies
Developers using the Geddes platform should have a backup strategy in place to ensure that your data is safe and can be recovered in case of a disaster. Below is a list of methods that can be used to backup data on Persistent Volume Claims.
Link to section 'Copying Files to and from a Container' of 'Storage' Copying Files to and from a Container
The kubectl cp
command can be used to copy files into or out of a running container.
# get pod id you want to copy to/form
kubectl -n <namespace> get pods
# copy a file from local filesystem to remote pod
kubectl cp /tmp/myfile <namespace>/<pod>:/tmp/myfile
# copy a file from remote pod to local filesystem
kubectl cp <namespace>/<pod>:/tmp/myfile /tmp/myfile
This method requires the tar
executable to be present in your container, which is usually the case with Linux image. More info can be found in the kubectl docs.
Link to section 'Copying Directories from a Container' of 'Storage' Copying Directories from a Container
The kubectl cp
command can also be used to recusively copy entire directories to local storage or places like Data Depot.
# get pod id you want to copy to/form
kubectl -n <namespace> get pods
# copy a directory from remote pod to local filesystem
kubectl cp <namespace>/<pod>:/pvcdirectory /localstorage
Link to section 'Backing up a Database from a Container' of 'Storage' Backing up a Database from a Container
The kubectl exec
command can be used to create a backup or dump of a database and save it to a local directory. For instance, to backup a MySQL database with kubectl, run the following commands from a local workstation or cluster frontend.
# get pod id of your database pod
kubectl -n <namespace> get pods
# run mysqldump in the remote pod and redirect the output to local storage
kubectl -n <namespace> exec <pod> -- mysqldump --user=<username> --password=<password> my_database > my_database_dump.sql
Link to section 'Backups using common Linux tools' of 'Storage' Backups using common Linux tools
If your container has the OpenSSH client or rsync packages installed, one can use the kubectl exec
command to copy or synchonize another storage location.
# get pod id of your pod
kubectl -n <namespace> get pods
# run scp to transfer data from the pod to a remote storage location
kubectl -n <namespace> <pod> exec -- scp -r /data username@negishi.rcac.purdue.edu:~/backup
Link to section 'Automating Backups' of 'Storage' Automating Backups
Kubernetes CronJob resources can be used with the commands above to create an automated backup solution. For more information, refer to the Kubernetes documentation.
Link to section 'Object Storage' of 'Storage' Object Storage
Geddes provides S3 compatible object storage from the endpoint https://s3-prod.geddes.rcac.purdue.edu.
S3 access can be requested by contacting support. Access keys will be provided via Filelocker.
Link to section 'Accessing Object Storage' of 'Storage' Accessing Object Storage
The S3 endpoint provided by Geddes can be accessed in multiple ways. Two popular options for interacting with S3 storage via the command line and GUI are listed below.
S3cmd is a free command line tool for managing data in S3 compatible storage resources that works on Linux and Mac.
- Download: https://s3tools.org/download
- How-To Documentation: https://s3tools.org/s3cmd-howto
Cyberduck is a free server and cloud storage browser that can be used on Windows and Mac.
-
Launch Cyberduck
-
Click + Open Connection at the top of the UI.
-
Select S3 from the dropdown menu
-
Fill in Server, Access Key ID and Secret Access Key fields
-
Click Connect
-
You can now right click to bring up a menu of actions that can be performed against the storage endpoint
Further information about using Cyberduck can be found on the Cyberduck documentation site.
Link to section 'Accessing and Mounting Depot' of 'Storage' Accessing and Mounting Depot
Contact support to request access. Make sure to provide the Geddes namespace that will be accessing depot and the $PATH
to your user/lab depot space. Once Access has been approved and an admin has created the needed Persistent Volumes for depot you can move on to the steps below.
The overall process is:
-
Submit request.
- An admin will create the needed Persistent Volume needed to access your depot space and will provide you with the name pv-depot-<your-pv-name>
-
Create Kubernetes secrets for Depot username/password authentication.
-
Create a Persistent Volume Claim via Rancher UI or Kubectl .
-
Use that claim for your workloads/pods to mount depot.
-
From the rancher UI, use the left navigation bar to select Storage > Secrets
-
Click Create at the top right
-
Select Oqaque and fill out the form.
-
Make sure select the namespace that will be accessing depot
-
Name should be depot-credentials-<myusername>
-
Under the data tab click add to create a second secret key field
-
Provide key/values
-
Key: username value: <yourUsername>
-
Key: password value: <yourPassword>
-
-
Click Create at the bottom right
-
-
From the Rancher UI, use the left navigation bar to select Storage > PersistentVolumeCLaims
-
Click Create at the top right and fill out the form
-
Make sure select the namespace that will be accessing depot
-
Name should be pvc-depot-<yourUsername>
-
Select Use an existing Persistent Volume
-
Use the dropdown to the immediate right to select pv-depot-<your pv name>
-
Click Customize in the form tab on the left
-
Select Many Nodes Read-Write
-
Click Create at the bottom right.
-
-
Create a yaml file i.e depot-pvc.yaml with the code below
apiVersion: v1 kind: PersistentVolumeClaim metadata: name: pvc-depot-<yourUsername> namespace: <namespace> spec: accessModes: - ReadWriteMany resources: requests: storage: 1Mi volumeName: pv-depot-<your pv name> storageClassName: ""
-
Replace all the <yourUsername>
and <namespace> with the appropriate values. -
Do not include the example angle brackets < > in your code
-
-
Apply the yaml with the command $ kubectl apply -f depot-pvc.yaml
Examples
Examples of deploying a database with persistent storage and making it available on the network and deploying a webserver using a self-assigned URL.
Database
Link to section 'Deploy a postgis Database' of 'Database' Deploy a postgis Database
- Select your Project from the top right dropdown
- Using the far left menu, select Workload
- Click Create at the top right
- Select the appropriate Deployment Type for your use case, here we will select and use Deployment
- Fill out the form
- Select Namespace
- Give arbitrary Name
- Set Container Image to the postgis Docker image:
geddes-registry.rcac.purdue.edu/docker-hub-cache/postgis/postgis:latest
- Set the postgres user password
- Select the Add Variable button under the Environment Variables section
- Fill in the fields Variable Name and Value so that we have a variable
POSTGRES_PASSWORD = <some password>
- Create a persistent volume for your database
- Select the Storage tab from within the current form on the left hand side
- Select Add Volume and choose Create Persistent Volume Claim
- Give arbitrary Name
- Select Single-Node Read/Write
- Select appropriate Storage Class from the dropdown and give Capacity in GiB e.g 5
- Provide the default postgres data directory as a Mount Point for the persistent volume
/var/lib/postgresql/data
- Set Sub Path to
data
- Set resource CPU limitations
- Select Resources tab on the left within the current form
- Under the CPU Reservation box fill in
2000
This ensures that Kubernetes will only schedule your workload to nodes that have that resource amount available, guaranteeing your application has 2CPU cores to utilize - Under the CPU Limit box also will in 2000 This ensures that your workload cannot exceed or utilize more than 2CPU cores. This helps resource quota management on the project level.
- Setup Pod Label
- Select Labels & Annotations on the left side of the current form
- Select Add Label under the Pod Labels section
- Give arbitrary unique key and value you can remember later when creating Services and other resources e.g Key:
my-db
Value:postgis
- Select Create to launch the postgis database
Wait a couple minutes while your persistent volume is created and the postgis container is deployed. The “does not have minimum availability” message is expected. But, waiting more than 5 minutes for your workload to deploy typically indicates a problem. You can check for errors by clicking your workload name (i.e. "mydb"), then the lower button on the right side of your deployed pod and selecting View Logs If all goes well, you will see an Active status for your deployment
Link to section 'Expose the Database to external clients' of 'Database' Expose the Database to external clients
Use a LoadBalancer service to automatically assign an IP address on a private Purdue network and open the postgres port (5432). A DNS name will automatically be configured for your service as <servicename>.<namespace>.geddes.rcac.purdue.edu
.
- Using the far left menu and navigate to Service Discovery > Services
- Select Create at the top right
- Select Load Balancer
- Fill out the form
- Ensure to select the namespace where you deployed the postgis database
- Give a Name to your Service. Remember that your final DNS name when the service creates will be in the format of
<servicename>.<namespace>.geddes.rcac.purdue.edu
- Fill in Listening Port and Target Port with the postgis default port 5432
- Select the Selectors tab within the current form
- Fill in Key and Value with the label values you created during the Setup Pod Label step from earlier e.g Key:
my-db
Value:postgis
- IMPORTANT: The yellow bar will turn green if your key-value pair matches the pod label you set during the "Setup Pod Label" deployment step above. If you don't see a green bar with a matching Pod, your LoadBalancer will not work.
- Fill in Key and Value with the label values you created during the Setup Pod Label step from earlier e.g Key:
- Select the Labels & Annotations tab within the current form
- Select Add Annotation
- To deploy to a Purdue Private Address Range fill in Key:
metallb.universe.tf/address-pool
Value:geddes-private-pool
- To deploy to a Public Address Range fill in Key:
metallb.universe.tf/address-pool
Value:geddes-public-pool
Kubernetes will now automatically assign you an IP address from the Geddes private IP pool. You can check the IP address by hovering over the “5432/tcp” link on the Service Discovery page or by viewing your service via kubectl on a terminal.
$ kubectl -n <namespace> get services
Verify your DNS record was created:
$ host <servicename>.<namespace>.geddes.rcac.purdue.edu
Web Server
Link to section 'Nginx Deployment' of 'Web Server' Nginx Deployment
- Select your Project from the top right dropdown
- Using the far left menu so select Workload
- Click Create at the top right
- Select the appropriate Deployment Type for your use case, here we will select and use Deployment
- Fill out the form
- Select Namespace
- Give arbitrary Name
- Set Container Image to the nginx Docker image:
geddes-registry.rcac.purdue.edu/docker-hub-cache/library/nginx
- Create a Cluster IP service to point our external accessible ingress to later
- Click Add Port
- Click Service Type and with the drop select Cluster IP
- In the Private Container Port box type 80
- Setup Pod Label
- Select Labels & Annotations on the left side of the current form
- Select Add Label under the Pod Labels section
- Give arbitrary unique key and value you can remember later when creating Services and other resources e.g Key:
my-web
Value:nginx
- Click Create
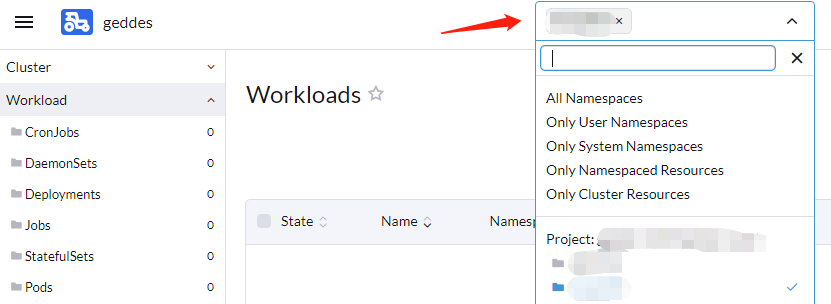
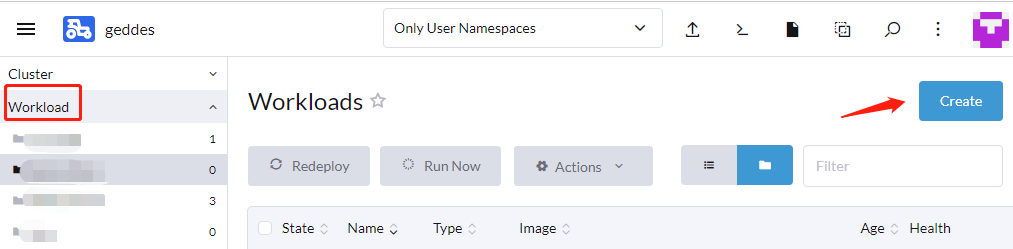
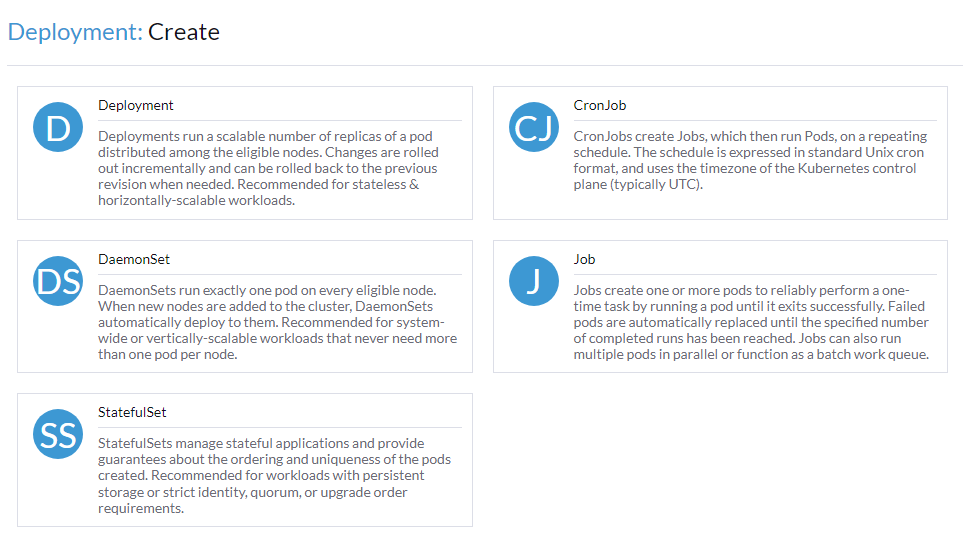
Wait a couple minutes while your application is deployed. The “does not have minimum availability” message is expected. But, waiting more than 5 minutes for your workload to deploy typically indicates a problem. You can check for errors by clicking your workload name (i.e. "mywebserver"), then using the vertical ellipsis on the right hand side of your deployed pod and selecting View Logs
If all goes well, you will see an Active status for your deployment.
Link to section 'Expose the web server to external clients via an Ingress' of 'Web Server' Expose the web server to external clients via an Ingress
- Using the far left menu and navigate to Service Discovery > Ingresses and select Create at the top right
- Fill out the form
- Ensure to select the namespace where you deployed the nginx
- Give an arbitrary Name
- Under Request Host give the url you want for your web application e.g
my-nginx.geddes.rcac.purdue.edu
- Fill in the value Path > Prefix as
/
- Use the Target Service and ;Port dropdowns to select the service you created during the Nginx Deployment section
- The default Ingress is private, which is only accessible within Purdue network. To make a public one, change the Ingress Class to
public
:
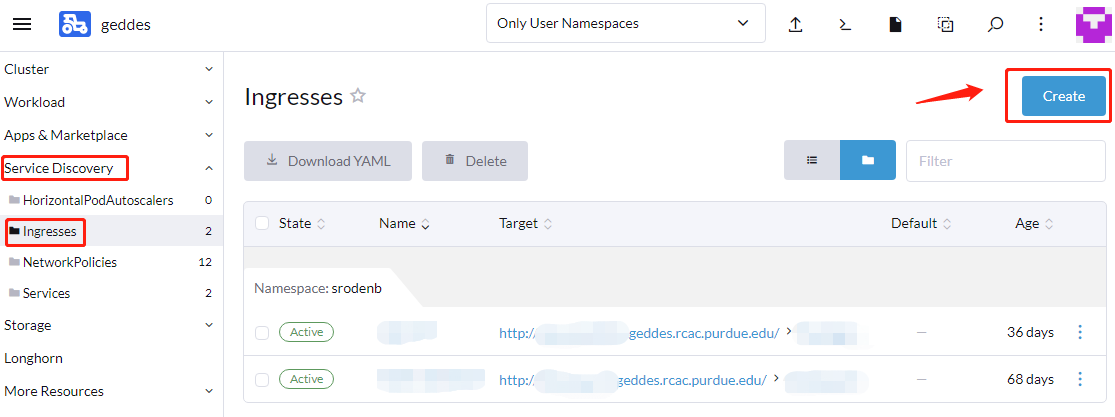
R Shiny
This guide provides instructions on how to build a Docker image for an R Shiny application, push it to the Geddes Registry and deploy it on Geddes.
Link to section 'Create an RShiny Docker Image' of 'R Shiny' Create an RShiny Docker Image
Create a local Dockerfile by saving the following Dockerfile to your computer and editing the contents for your R Shiny App.
FROM rocker/shiny
# install R package dependencies
RUN apt-get update && apt-get install -y \
libssl-dev \
git \
## clean up
&& apt-get clean \
&& rm -rf /var/lib/apt/lists/ \
&& rm -rf /tmp/downloaded_packages/ /tmp/*.rds
## Install any R packages you need
RUN install2.r --error \
<package 1> \
<package 2> \
<package 3> \
## clean up
&& rm -rf /tmp/downloaded_packages/ /tmp/*.rds
## copy shiny app to shiny server location
COPY ./<app directory> /srv/shiny-server/
Link to section 'Docker Build and Testing Process' of 'R Shiny' Docker Build and Testing Process
Build the Docker image locally based on the Dockerfile above. The Dockerfile must be in your current working directory. This command tags the image with the name "myshinyapp" and version 1.0.
docker build -t myshinyapp:1.0 .
Test your application locally. This command will run your container locally and expose the R Shiny port (3838) so it can be accessed via http://localhost:3838 in your web browser.
On Linux or Mac: docker run --network=host myshinyapp:1.1
On Windows: docker run -p 3838:3838 myshinyapp:1.1
Iterate on code changes locally until you want to deploy on Geddes.
Link to section 'Tag and Upload to the Geddes Registry' of 'R Shiny' Tag and Upload to the Geddes Registry
Tag the image for upload to the Geddes Registry
docker tag myshiny:1.0 geddes-registry.rcac.purdue.edu/<repo>/myshinyapp:1.0
Push the image to the Geddes Registry. Run the login command using your Purdue career account username and password if you currently are not authenticated to the registry.
docker login geddes-registry.rcac.purdue.edu
docker push geddes-registry.rcac.purdue.edu/<repo>/myshinyapp:1.0
Link to section 'Deploy the Application on Geddes' of 'R Shiny' Deploy the Application on Geddes
To deploy the application, one can follow the instructions for deploying a web server and replace the image name with the Geddes registry image tag from above: geddes-registry.rcac.purdue.edu/<repo>/myshinyapp:1.0
Troubleshooting
Link to section 'Useful Links' of 'Troubleshooting' Useful Links
There are many valuable Kubernetes troubleshooting guides readily accessible on the Internet. Instead of duplicating them here, we provide links to external documentation that has been useful for users of the Geddes platform.
- Debugging Pods
- Debugging Services
- Pod Failures
- Get a Shell on a Running Container
- Though the Geddes UI, the equivalent method by clicking the vertical elipse and selecting "Execute Shell".
Link to section 'Create a Pod for Debugging' of 'Troubleshooting' Create a Pod for Debugging
Many times, users want to start a simple persistent Pod from a container image like Alpine or Ubuntu to do troubleshooting. The following YAML will deploy an Alpine Linux pod in a namespace that sleeps for 24 hours.
apiVersion: v1
kind: Pod
metadata:
name: debug
namespace: <namespace>
labels:
app: debug
spec:
containers:
- image: geddes-registry.rcac.purdue.edu/docker-hub-cache/library/alpine
command:
- "sleep"
- "86400"
name: debug
One can also launch a persistent Pod by specifying the sleep command via the Geddes UI.
Link to section 'Permission Denied on PVC for non-root User' of 'Troubleshooting' Permission Denied on PVC for non-root User
If your container or process runs as a non-root user and you see a "Permission denied" error, you may need to set the fsGroup SecurityContext on your Pod so permissions are configured correctly on the PVC. This can be done in the Pod's spec.
securityContext:
fsGroup: <gid>
Where gid is the group id your container is running as, or the group id of the process that is trying to write to the PVC.
This setting can also be applied under the Pod menu when deploying a workfload via the Geddes UI.
Link to section 'Pull Rate Limit Error' of 'Troubleshooting' Pull Rate Limit Error
Symptom:
ImagePullBackoff Error with message Failed to pull image "<image>:<tag>
Solution:
Use the Geddes Registry Docker Hub Cache to pull your image from Docker Hub.
Link to section 'Ingress 413 Content Too Large Errors' of 'Troubleshooting' Ingress 413 Content Too Large Errors
By default, the Ingress controller on Geddes can handle requests up to 1 MB in size. If you need to send requests larger than 1 MB you can increase the size with the following Ingress annotation. Using "0" will allow unlimited size.
metadata:
annotations:
nginx.ingress.kubernetes.io/proxy-body-size: "0"
Anvil User Guide
Purdue University is the home of Anvil, a powerful new supercomputer that provides advanced computing capabilities to support a wide range of computational and data-intensive research spanning from traditional high-performance computing to modern artificial intelligence applications.
Overview of Anvil
Purdue University is the home of Anvil, a powerful new supercomputer that provides advanced computing capabilities to support a wide range of computational and data-intensive research spanning from traditional high-performance computing to modern artificial intelligence applications.
Anvil, which is funded by a $10 million award from the National Science Foundation, significantly increases the capacity available to the NSF's Advanced Cyberinfrastructure Coordination Ecosystem: Services & Support (ACCESS) program, which serves tens of thousands of researchers across the U.S.. Anvil enters production in 2021 and serves researchers for five years. Additional funding from the NSF supports Anvil's operations and user support.
The name "Anvil" reflects the Purdue Boilermakers' strength and workmanlike focus on producing results, and the Anvil supercomputer enables important discoveries across many different areas of science and engineering. Anvil also serves as an experiential learning laboratory for students to gain real-world experience using computing for their science, and for student interns to work with the Anvil team for construction and operation. We will be training the research computing practitioners of the future.
Anvil is built in partnership with Dell and AMD and consists of 1,000 nodes with two 64-core AMD Epyc "Milan" processors each and will deliver over 1 billion CPU core hours to ACCESS each year, with a peak performance of 5.3 petaflops. Anvil's nodes are interconnected with 100 Gbps Mellanox HDR InfiniBand. The supercomputer ecosystem also includes 32 large memory nodes, each with 1 TB of RAM, and 16 nodes each with four NVIDIA A100 Tensor Core GPUs providing 1.5 PF of single-precision performance to support machine learning and artificial intelligence applications.
Anvil is funded under NSF award number 2005632. Carol Song is the principal investigator and project director. Preston Smith, executive director of the Rosen Center for Advanced Computing, Xiao Zhu, computational scientist and senior research scientist, and Rajesh Kalyanam, data scientist, software engineer, and research scientist, are all co-PIs on the project.
Link to section 'Anvil Specifications' of 'Overview of Anvil' Anvil Specifications
All Anvil nodes have 128 processor cores, 256 GB to 1 TB of RAM, and 100 Gbps Infiniband interconnects.
Login | Number of Nodes | Processors per Node | Cores per Node | Memory per Node |
---|---|---|---|---|
8 | Two Milan CPUs @ 2.45GHz | 32 | 512 GB |
Sub-Cluster | Number of Nodes | Processors per Node | Cores per Node | Memory per Node |
---|---|---|---|---|
A | 1,000 | Two Milan CPUs @ 2.45GHz | 128 | 256 GB |
B | 32 | Two Milan CPUs @ 2.45GHz | 128 | 1 TB |
G | 16 | Two Milan CPUs @ 2.45GHz + Four NVIDIA A100 GPUs | 128 | 512 GB |
Anvil nodes run CentOS 8 and use Slurm (Simple Linux Utility for Resource Management) as the batch scheduler for resource and job management. The application of operating system patches will occur as security needs dictate. All nodes allow for unlimited stack usage, as well as unlimited core dump size (though disk space and server quotas may still be a limiting factor).
Link to section 'Software catalog' of 'Overview of Anvil' Software catalog
Accessing the System
Link to section 'Accounts on Anvil' of 'Accessing the System' Accounts on Anvil
Obtaining an Account
As an ACCESS computing resource, Anvil is accessible to ACCESS users who receive an allocation on the system. To obtain an account, users may submit a proposal through the ACCESS Allocation Request System.
For details on how to go about requesting an allocation, refer to How do I get onto Anvil through ACCESS.
Interested parties may contact the ACCESS Help Desk for help with an Anvil proposal.
How do I get onto Anvil through ACCESS
Link to section 'What is ACCESS?' of 'How do I get onto Anvil through ACCESS' What is ACCESS?
Advanced Cyberinfrastructure Coordination Ecosystem: Services & Support (ACCESS) is an NSF-funded program that manages access to the national research cyberinfrastructure (CI) resources. Any researcher who seeks to use one of these CI resources must follow ACCESS processes to get onto these resources.
Link to section 'What resources are available via ACCESS?' of 'How do I get onto Anvil through ACCESS' What resources are available via ACCESS?
ACCESS coordinates a diverse set of resources including Anvil and other traditional HPC resources suited for resource-intensive CPU workloads, modern accelerator-based systems (e.g., GPU), as well as cloud resources. Anvil provides both CPU and GPU resources as part of ACCESS. A comprehensive list of all the ACCESS-managed resources can be found here along with their descriptions and ideal workloads: https://allocations.access-ci.org/resources
Link to section 'How do I request access to a resource?' of 'How do I get onto Anvil through ACCESS' How do I request access to a resource?
The process of getting onto these resources is broadly:
- Sign up for an ACCESS account (if you don’t have one already) at https://allocations.access-ci.org.
- Prepare an allocation request with details of your proposed computational workflows (science, software needs), resource requirements, and a short CV. See the individual “Preparing Your … Request” pages for details on what documents are required: https://allocations.access-ci.org/prepare-requests.
- Decide on which allocation tier you want to apply to (more on that below) and submit the request.
Link to section 'Which ACCESS tier should I choose?' of 'How do I get onto Anvil through ACCESS' Which ACCESS tier should I choose?
As you can gather from https://allocations.access-ci.org/project-types, there are four different tiers in ACCESS. Broadly, these tiers provide increasing computational resources with corresponding stringent documentation and resource justification requirements. Furthermore, while Explore and Discover tier requests are reviewed on a rolling basis as they are submitted, Accelerate requests will be reviewed monthly and Maximize will be reviewed twice a year. The review period reflects the level of resources provided, and Explore and Discover applications are generally reviewed within a week. An important point to note is that ACCESS does not award you time on a specific computational resource (except for the Maximize tier). Users are awarded a certain number of ACCESS credits which they then exchange for time on a particular resource. Here are some guidelines on how to choose between the tiers:- If you are a graduate student, you may apply for the Explore tier with a letter from your advisor on institutional letterhead stating that the proposed work is being performed primarily by the graduate student and is separate from other funded grants or the advisor's own research.
- If you would just like to test out a resource and gather some performance data before making a large request, Explore or Discover is again the appropriate option.
- If you would like to run simulations across multiple resources to identify the one best suited for you, Discover will provide you with sufficient credits to exchange across multiple systems.
- One way of determining the appropriate tier is to determine what the credits would translate to in terms of computational resources. The exchange calculator (https://allocations.access-ci.org/exchange_calculator) can be used to calculate what a certain number of ACCESS credits translates to in terms of “core-hours” or “GPU-hours” or “node-hours” on an ACCESS resource. For example: the maximum 400,000 ACCESS credits that you may be awarded in the Explore tier translates to ~334,000 CPU core hours or ~6000 GPU hours on Anvil. Based on the scale of simulations you would like to run, you may need to choose one tier or the other.
Link to section 'What else should I know?' of 'How do I get onto Anvil through ACCESS' What else should I know?
- You may request a separate allocation for each of your research grants and the allocation can last the duration of the grant (except for the Maximize tier which only lasts for 12 months). Allocations that do not cite a grant will last for 12 months.
- Supplements are not allowed (for Explore, Discover, and Accelerate tiers), instead you will need to move to a different tier if you require more resources.
- As noted above, the exchange rates for Anvil CPU and Anvil GPU are different so be sure to check the exchange calculator.
- Be sure to include details of the simulations you would like to run and what software you would like to use. This avoids back and forth with the reviewers and also helps Anvil staff determine if your workloads are well suited to Anvil.
- When your request is approved, you only get ACCESS credits awarded. You still need to go through the step of exchanging these credits for time on Anvil. You need not use up all your credits and may also use part of your credits for time on other ACCESS resources.
- You will also need to go to the allocations page and add any users you would like to have access to these resources. Note that they will need to sign up for ACCESS accounts as well before you can add them.
- For other questions you may have, take a look at ACCESS policies here: (https://allocations.access-ci.org/allocations-policy)
Logging In
Anvil supports the SSH (Secure Shell), ThinLinc, and Open OnDemand mechanisms for logging in. The first two of these use SSH keys. If you need help creating or uploading your SSH keys, please see the Managing SSH Public Keys page for that information.
ACCESS requires that you use the ACCESS Duo service for additional authentication, you will be prompted to authenticate yourself further using Duo and your Duo client app, token, or other contact methods. Consult Manage Multi-Factor Authentication with Duo for account setup instructions.
Link to section 'With SSH' of 'Logging In' With SSH
Anvil accepts standard SSH connections with public keys-based authentication to anvil.rcac.purdue.edu
using your Anvil username:
localhost$ ssh -l my-x-anvil-username anvil.rcac.purdue.edu
Please note:
- Your Anvil username is not the same as your ACCESS username (although it is derived from it). Anvil usernames look like
x-ACCESSusername
or similar, starting with anx-
. - Password-based authentication is not supported on Anvil (in favor of SSH keys). There is no "Anvil password", and your ACCESS password will not be accepted by Anvil's SSH either. SSH keys can be set up from the Open OnDemand interface on Anvil
ondemand.anvil.rcac.purdue.edu
. Please follow the steps in Setting up SSH keys to add your SSH key on Anvil.
When reporting SSH problems to the help desk, please execute the ssh
command with the -vvv
option and include the verbose output in your problem description.
Link to section 'Additional Services and Instructions' of 'Logging In' Additional Services and Instructions
Open OnDemand
Open OnDemand is an open-source HPC portal developed by the Ohio Supercomputing Center. Open OnDemand allows one to interact with HPC resources through a web browser and easily manage files, submit jobs, and interact with graphical applications directly in a browser, all with no software to install. Anvil has an instance of OnDemand available that can be accessed via ondemand.anvil.rcac.purdue.edu.
Link to section 'Logging In' of 'Open OnDemand' Logging In
To log into the Anvil OnDemand portal:
- Navigate to ondemand.anvil.rcac.purdue.edu
- Log in using your ACCESS username and password
- ACCESS Duo authentication is required to login to OnDemand. Consult Manage Multi-Factor Authentication with Duo for account setup instructions
The Anvil team continues to refine the user interface, please reach out to us in case of any queries regarding the use of OnDemand.
SSH Keys
Link to section 'General overview' of 'SSH Keys' General overview
To connect to Anvil using SSH keys, you must follow three high-level steps:
- Generate a key pair consisting of a private and a public key on your local machine.
- Copy the public key to the cluster and append it to
$HOME/.ssh/authorized_keys
file in your account. - Test if you can ssh from your local computer to the cluster directly.
Detailed steps for different operating systems and specific SSH client software are given below.
Link to section 'Mac and Linux:' of 'SSH Keys' Mac and Linux:
-
Run
ssh-keygen
in a terminal on your local machine.localhost >$ ssh-keygen Generating public/private rsa key pair. Enter file in which to save the key (localhost/.ssh/id_rsa):
You may supply a filename and a passphrase for protecting your private key, but it is not mandatory. To accept the default settings, press Enter without specifying a filename.
Note: If you do not protect your private key with a passphrase, anyone with access to your computer could SSH to your account on Anvil.Created directory 'localhost/.ssh'. Enter passphrase (empty for no passphrase): Enter same passphrase again: Your identification has been saved in localhost/.ssh/id_rsa. Your public key has been saved in localhost/.ssh/id_rsa.pub. The key fingerprint is: ... The key's randomart image is: ...
By default, the key files will be stored in
~/.ssh/id_rsa
and~/.ssh/id_rsa.pub
on your local machine. -
Go to the
~/.ssh
folder in your local machine andcat
the key information in theid_rsa.pub file
.localhost/.ssh>$ cat id_rsa.pub ssh-rsa XXXXXXXXXXXXXXXXXXXXXXXXXXXXXXXXXXXXXXXXXXXXXXXXXXXXXXXXXXXXXXXXXXXXXXXXXXXXXXXXXXXXXXXXXXXXXXXXXXXXXXXXXXXXXXXX XXXXXXXXXXXXXXXXXXXXXXXXXXXXXXXXXXXXXXXXXXXXXXXXXXXXXXXXXXXXXXXXXXXXXX= localhost-username@localhost
-
For your first time login to Anvil, please log in to Open OnDemand ondemand.anvil.rcac.purdue.edu using your ACCESS username and password.
-
Once logged on to OnDemand, go to the
Clusters
on the top toolbar. ClickAnvil Shell Access
and you will be able to see the terminal.============================================================================= == Welcome to the Anvil Cluster == …… ============================================================================= ** DID YOU KNOW? ** …… ***************************************************************************** x-anvilusername@login04.anvil:[~] $ pwd /home/x-anvilusername
-
Under the home directory on Anvil, make a
.ssh
directory usingmkdir -p ~/.ssh
if it does not exist.
Create a file~/.ssh/authorized_keys
on the Anvil cluster and copy the contents of the public keyid_rsa.pub
in your local machine into~/.ssh/authorized_keys
.x-anvilusername@login04.anvil:[~] $ pwd /home/x-anvilusername x-anvilusername@login04.anvil:[~] $ cd ~/.ssh x-anvilusername@login04.anvil:[.ssh] $ vi authorized_keys # copy-paste the contents of the public key id_rsa.pub in your local machine (as shown in step 2) to authorized_keys here and save the change of authorized_keys file. Then it is all set! #
-
Test the new key by SSH-ing to the server. The login should now complete without asking for a password.
localhost>$ ssh x-anvilusername@anvil.rcac.purdue.edu ============================================================================= == Welcome to the Anvil Cluster == ... ============================================================================= x-anvilusername@login06.anvil:[~] $
-
If the private key has a non-default name or location, you need to specify the key by
ssh -i my_private_key_name x-anvilusername@anvil.rcac.purdue.edu
.
Link to section 'Windows:' of 'SSH Keys' Windows:
Programs | Instructions |
---|---|
MobaXterm | Open a local terminal and follow Linux steps |
Git Bash | Follow Linux steps |
Windows 10 PowerShell | Follow Linux steps |
Windows 10 Subsystem for Linux | Follow Linux steps |
PuTTY | Follow steps below |
PuTTY:
-
Launch PuTTYgen, keep the default key type (RSA) and length (2048-bits) and click Generate button.
The "Generate" button can be found under the "Actions" section of the PuTTY Key Generator interface. -
Once the key pair is generated:
Use the Save public key button to save the public key, e.g.
Documents\SSH_Keys\mylaptop_public_key.pub
Use the Save private key button to save the private key, e.g.
Documents\SSH_Keys\mylaptop_private_key.ppk
. When saving the private key, you can also choose a reminder comment, as well as an optional passphrase to protect your key, as shown in the image below. Note: If you do not protect your private key with a passphrase, anyone with access to your computer could SSH to your account on Anvil.The PuTTY Key Generator form has inputs for the Key passphrase and optional reminder comment. From the menu of PuTTYgen, use the "Conversion -> Export OpenSSH key" tool to convert the private key into openssh format, e.g.
Documents\SSH_Keys\mylaptop_private_key.openssh
to be used later for Thinlinc. -
Configure PuTTY to use key-based authentication:
Launch PuTTY and navigate to "Connection -> SSH ->Auth" on the left panel, click Browse button under the "Authentication parameters" section and choose your private key, e.g. mylaptop_private_key.ppk
After clicking Connection -> SSH ->Auth panel, the "Browse" option can be found at the bottom of the resulting panel. Navigate back to "Session" on the left panel. Highlight "Default Settings" and click the "Save" button to ensure the change is in place.
-
For your first time login to Anvil, please log in to Open OnDemand ondemand.anvil.rcac.purdue.edu using your ACCESS username and password.
-
Once logged on to OnDemand, go to the
Clusters
on the top toolbar. ClickAnvil Shell Access
and you will be able to see the terminal.============================================================================= == Welcome to the Anvil Cluster == …… ============================================================================= ** DID YOU KNOW? ** …… ***************************************************************************** x-anvilusername@login04.anvil:[~] $ pwd /home/x-anvilusername
-
Under the home directory on Anvil, make a
.ssh
directory usingmkdir -p ~/.ssh
if it does not exist.
Create a file~/.ssh/authorized_keys
on the Anvil cluster and copy the contents of the public keyid_rsa.pub
in your local machine into~/.ssh/authorized_keys
.x-anvilusername@login04.anvil:[~] $ pwd /home/x-anvilusername x-anvilusername@login04.anvil:[~] $ cd ~/.ssh x-anvilusername@login04.anvil:[.ssh] $ vi authorized_keys # copy-paste the contents of the public key id_rsa.pub in your local machine (as shown in step 2) to authorized_keys here and save the change of authorized_keys file. Then it is all set! #
~/.ssh/authorized_keys
. Please double-check that your text editor did not wrap or fold the pasted value (it should be one very long line).The "Public key" will look like a long string of random letters and numbers in a text box at the top of the window. - Test by connecting to the cluster and the login should now complete without asking for a password. If you chose to protect your private key with a passphrase in step 2, you will be prompted to enter the passphrase when connecting.
ThinLinc
For your first time accessing Anvil using ThinLinc client, your desktop might be locked after it has been idle for more than 5 minutes. It is because in the default settings, the "screensaver" and "lock screen" are turned on. To solve this issue, please refer to the FAQs Page.
Anvil provides Cendio's ThinLinc as an alternative to running an X11 server directly on your computer. It allows you to run graphical applications or graphical interactive jobs directly on Anvil through a persistent remote graphical desktop session.
ThinLinc is a service that allows you to connect to a persistent remote graphical desktop session. This service works very well over a high latency, low bandwidth, or off-campus connection compared to running an X11 server locally. It is also very helpful for Windows users who do not have an easy to use local X11 server, as little to no setup is required on your computer.
There are two ways in which to use ThinLinc: preferably through the native client or through a web browser.
Browser-based Thinlinc access is not supported on Anvil at this moment. Please use native Thinlinc client with SSH keys.
Link to section 'Installing the ThinLinc native client' of 'ThinLinc' Installing the ThinLinc native client
The native ThinLinc client will offer the best experience especially over off-campus connections and is the recommended method for using ThinLinc. It is compatible with Windows, Mac OS X, and Linux.
- Download the ThinLinc client from the ThinLinc website.
- Start the ThinLinc client on your computer.
- In the client's login window, use desktop.anvil.rcac.purdue.edu as the Server and use your Anvil username
x-anvilusername
. - At this moment, an SSH key is required to login to ThinLinc client. For help generating and uploading keys to the cluster, see SSH Keys section in our user guide for details.
Link to section 'Configure ThinLinc to use SSH Keys' of 'ThinLinc' Configure ThinLinc to use SSH Keys
-
To set up SSH key authentication on the ThinLinc client:
-
Open the Options panel, and select Public key as your authentication method on the Security tab.
The "Options..." button in the ThinLinc Client can be found towards the bottom left, above the "Connect" button. -
In the options dialog, switch to the "Security" tab and select the "Public key" radio button:
The "Security" tab found in the options dialog, will be the last of available tabs. The "Public key" option can be found in the "Authentication method" options group. - Click OK to return to the ThinLinc Client login window. You should now see a Key field in place of the Password field.
-
In the Key field, type the path to your locally stored private key or click the ... button to locate and select the key on your local system. Note: If PuTTY is used to generate the SSH Key pairs, please choose the private key in the openssh format.
The ThinLinc Client login window will now display key field instead of a password field.
-
- Click the Connect button.
- Continue to following section on connecting to Anvil from ThinLinc.
Link to section 'Connecting to Anvil from ThinLinc' of 'ThinLinc' Connecting to Anvil from ThinLinc
- Once logged in, you will be presented with a remote Linux desktop running directly on a cluster login node.
- Open the terminal application on the remote desktop.
- Once logged in to the Anvil login node, you may use graphical editors, debuggers, software like Matlab, or run graphical interactive jobs. For example, to test the X forwarding connection issue the following command to launch the graphical editor geany:
$ geany
- This session will remain persistent even if you disconnect from the session. Any interactive jobs or applications you left running will continue running even if you are not connected to the session.
Link to section 'Tips for using ThinLinc native client' of 'ThinLinc' Tips for using ThinLinc native client
- To exit a full-screen ThinLinc session press the F8 key on your keyboard (fn + F8 key for Mac users) and click to disconnect or exit full screen.
- Full-screen mode can be disabled when connecting to a session by clicking the Options button and disabling full-screen mode from the Screen tab.
Check Allocation Usage
To keep track of the usage of the allocation by your project team, you can use mybalance
:
x-anvilusername@login01:~ $ mybalance
Allocation Type SU Limit SU Usage SU Usage SU Balance
Account (account) (user)
=============== ======= ======== ========= ========= ==========
xxxxxxxxx CPU 1000.0 95.7 0.0 904.3
You can also check the allocation usage through ACCESS allocations page.
See SU accounting section for detailed description of the way SUs are charged on Anvil.
System Architecture
Link to section 'Compute Nodes' of 'System Architecture' Compute Nodes
Model: | 3rd Gen AMD EPYC™ CPUs (AMD EPYC 7763) |
---|---|
Number of nodes: | 1000 |
Sockets per node: | 2 |
Cores per socket: | 64 |
Cores per node: | 128 |
Hardware threads per core: | 1 |
Hardware threads per node: | 128 |
Clock rate: | 2.45GHz (3.5GHz max boost) |
RAM: | Regular compute node: 256 GB DDR4-3200 Large memory node: (32 nodes with 1TB DDR4-3200) |
Cache: | L1d cache: 32K/core L1i cache: 32K/core L2 cache: 512K/core L3 cache: 32768K |
Local storage: | 480GB local disk |
Link to section 'Login Nodes' of 'System Architecture' Login Nodes
Number of Nodes | Processors per Node | Cores per Node | Memory per Node |
---|---|---|---|
8 | 3rd Gen AMD EPYC™ 7543 CPU | 32 | 512 GB |
Link to section 'Specialized Nodes' of 'System Architecture' Specialized Nodes
Sub-Cluster | Number of Nodes | Processors per Node | Cores per Node | Memory per Node |
---|---|---|---|---|
B | 32 | Two 3rd Gen AMD EPYC™ 7763 CPUs | 128 | 1 TB |
G | 16 | Two 3rd Gen AMD EPYC™ 7763 CPUs + Four NVIDIA A100 GPUs | 128 | 512 GB |
Link to section 'Network' of 'System Architecture' Network
All nodes, as well as the scratch storage system are interconnected by an oversubscribed (3:1 fat tree) HDR InfiniBand interconnect. The nominal per-node bandwidth is 100 Gbps, with message latency as low as 0.90 microseconds. The fabric is implemented as a two-stage fat tree. Nodes are directly connected to Mellanox QM8790 switches with 60 HDR100 links down to nodes and 10 links to spine switches.
Running Jobs
Users familiar with the Linux command line may use standard job submission utilities to manage and run jobs on the Anvil compute nodes.
For GPU jobs, make sure to use --gpus-per-node
argument, otherwise, your job may not run properly.
Accessing the Compute Nodes
Anvil uses the Slurm Workload Manager for job scheduling and management. With Slurm, a user requests resources and submits a job to a queue. The system takes jobs from queues, allocates the necessary compute nodes, and executes them. While users will typically SSH to an Anvil login node to access the Slurm job scheduler, they should note that Slurm should always be used to submit their work as a job rather than run computationally intensive jobs directly on a login node. All users share the login nodes, and running anything but the smallest test job will negatively impact everyone's ability to use Anvil.
Anvil is designed to serve the moderate-scale computation and data needs of the majority of ACCESS users. Users with allocations can submit to a variety of queues with varying job size and walltime limits. Separate sets of queues are utilized for the CPU, GPU, and large memory nodes. Typically, queues with shorter walltime and smaller job size limits will feature faster turnarounds. Some additional points to be aware of regarding the Anvil queues are:
- Anvil provides a debug queue for testing and debugging codes.
- Anvil supports shared-node jobs (more than one job on a single node). Many applications are serial or can only scale to a few cores. Allowing shared nodes improves job throughput, provides higher overall system utilization and allows more users to run on Anvil.
- Anvil supports long-running jobs - run times can be extended to four days for jobs using up to 16 full nodes.
- The maximum allowable job size on Anvil is 7,168 cores. To run larger jobs, submit a consulting ticket to discuss with Anvil support.
- Shared-node queues will be utilized for managing jobs on the GPU and large memory nodes.
Job Accounting
On Anvil, the CPU nodes and GPU nodes are charged separately.Link to section 'For CPU nodes' of 'Job Accounting' For CPU nodes
The charge unit for Anvil is the Service Unit (SU). This corresponds to the equivalent use of one compute core utilizing less than approximately 2G of data in memory for one hour.
Keep in mind that your charges are based on the resources that are tied up by your job and do not necessarily reflect how the resources are used.
If you explicitly request --mem-per-cpu=2G
, SLURM may allocate more resources than expected, since the default memory per core on Anvil is slightly less than 2GB (approximately 1896 MB). By requesting exactly 2GB per core, SLURM may allocate additional cores to meet the memory requirement, which can lead to higher SU charges.
Charges on jobs submitted to the shared queues are based on the number of cores and the fraction of the memory requested, whichever is larger. Jobs submitted as node-exclusive will be charged for all 128 cores, whether the resources are used or not.
Jobs submitted to the large memory nodes will be charged 4 SU per compute core (4x wholenode node charge).
Link to section 'For GPU nodes' of 'Job Accounting' For GPU nodes
1 SU corresponds to the equivalent use of one GPU utilizing less than or equal to approximately 120G of data in memory for one hour.
Each GPU nodes on Anvil have 4 GPUs and all GPU nodes are shared.
Link to section 'For file system' of 'Job Accounting' For file system
Filesystem storage is not charged.
You can use mybalance
command to check your current allocation usage.
Slurm Partitions (Queues)
Anvil provides different queues with varying job sizes and walltimes. There are also limits on the number of jobs queued and running on a per-user and queue basis. Queues and limits are subject to change based on the evaluation from the Early User Program.
Queue Name | Node Type | Max Nodes per Job | Max Cores per Job | Max Duration | Max running Jobs in Queue | Max running + submitted Jobs in Queue | Charging factor |
---|---|---|---|---|---|---|---|
debug | regular | 2 nodes | 256 cores | 2 hrs | 1 | 2 | 1 |
gpu-debug | gpu | 1 node | 2 gpus | 0.5 hrs | 1 | 2 | 1 |
wholenode | regular | 16 nodes | 2,048 cores | 96 hrs | 64 | 2500 | 1 (node-exclusive) |
wide | regular | 56 nodes | 7,168 cores | 12 hrs | 5 | 10 | 1 (node-exclusive) |
shared | regular | 1 node | 128 cores | 96 hrs | 1280 cores | - | 1 |
highmem | large-memory | 1 node | 128 cores | 48 hrs | 2 | 4 | 4 |
gpu | gpu | - | - | 48 hrs | - | - | 1 |
ai | ai | - | - | 48 hrs | - | - | 1 |
For gpu and ai queue: max of 12 GPU in use per user and max of 32 GPU in use per allocation.
Make sure to specify the desired partition when submitting your jobs (e.g. -p wholenode
). If you do not specify one, the job will be directed into the default partition (shared
).
If the partition is node-exclusive (e.g. the wholenode
and wide
queues), even if you ask for 1 core in your job submission script, your job will get allocated an entire node and would not share this node with any other jobs. Hence, it will be charged for 128 cores' worth and squeue
command would show it as 128 cores, too. See SU accounting for more details.
Link to section 'Useful tools' of 'Slurm Partitions (Queues)' Useful tools
- To display all Slurm partitions and their current usage, type
showpartitions
at the command line.x-anvilusername@login03.anvil:[~] $ showpartitions Partition statistics for cluster anvil at CURRENTTIME Partition #Nodes #CPU_cores Cores_pending Job_Nodes MaxJobTime Cores Mem/Node Name State Total Idle Total Idle Resorc Other Min Max Day-hr:mn /node (GB) wholenode up 750 0 96000 512 0 92280 1 infin infinite 128 257 standard up 750 0 96000 512 0 6753 1 infin infinite 128 257 shared:* up 250 97 32000 16395 0 4997 1 infin infinite 128 257 wide up 746 0 95488 512 0 24576 1 infin infinite 128 257 highmem up 32 19 4096 2504 0 0 1 infin infinite 128 1031 debug up 17 0 2176 113 0 128 1 infin infinite 128 257 gpu up 16 0 2048 1371 0 228 1 infin infinite 128 515 ai up 21 18 2016 1814 0 0 1 infin infinite 96 1031 gpu-debug up 16 0 2048 1371 0 0 1 infin infinite 128 515 benchmarking up 1048 116 134144 20782 0 0 1 infin infinite 128 257+ profiling up 4 0 512 0 0 0 1 infin infinite 128 257 azure up 8 0 16 0 0 0 1 infin infinite 2 7
- To show the list of available constraint feature names for different node types, type
sfeatures
at the command line.x-anvilusername@login03.anvil:[~] $ sfeatures NODELIST CPUS MEMORY AVAIL_FEATURES GRES a[000-999] 128 257400 A,a (null) b[000-031] 128 1031400 B,b,testing (null) g[000-015] 128 515400 G,g,A100 gpu:4 h[000-020] 96 1031000 H,h,H100 gpu:4 login[00-07] 32 500000 login (null) ondemand 32 90000 (null) (null) z[000-007] 2 7684 Z,z,power_azure (null)
Batch Jobs
Link to section 'Job Submission Script' of 'Batch Jobs' Job Submission Script
To submit work to a Slurm queue, you must first create a job submission file. This job submission file is essentially a simple shell script. It will set any required environment variables, load any necessary modules, create or modify files and directories, and run any applications that you need:
#!/bin/sh -l
# FILENAME: myjobsubmissionfile
# Loads Matlab and sets the application up
module load matlab
# Change to the directory from which you originally submitted this job.
cd $SLURM_SUBMIT_DIR
# Runs a Matlab script named 'myscript'
matlab -nodisplay -singleCompThread -r myscript
The standard Slurm environment variables that can be used in the job submission file are listed in the table below:
Name | Description |
---|---|
SLURM_SUBMIT_DIR | Absolute path of the current working directory when you submitted this job |
SLURM_JOBID | Job ID number assigned to this job by the batch system |
SLURM_JOB_NAME | Job name supplied by the user |
SLURM_JOB_NODELIST | Names of nodes assigned to this job |
SLURM_SUBMIT_HOST | Hostname of the system where you submitted this job |
SLURM_JOB_PARTITION | Name of the original queue to which you submitted this job |
Once your script is prepared, you are ready to submit your job.
Link to section 'Submitting a Job' of 'Batch Jobs' Submitting a Job
Once you have a job submission file, you may submit this script to SLURM using the sbatch command. Slurm will find, or wait for, available resources matching your request and run your job there.
To submit your job to one compute node with one task:
$ sbatch --nodes=1 --ntasks=1 myjobsubmissionfile
By default, each job receives 30 minutes of wall time, or clock time. If you know that your job will not need more than a certain amount of time to run, request less than the maximum wall time, as this may allow your job to run sooner. To request the 1 hour and 30 minutes of wall time:
$ sbatch -t 1:30:00 --nodes=1 --ntasks=1 myjobsubmissionfile
Each compute node in Anvil has 128 processor cores. In some cases, you may want to request multiple nodes. To utilize multiple nodes, you will need to have a program or code that is specifically programmed to use multiple nodes such as with MPI. Simply requesting more nodes will not make your work go faster. Your code must utilize all the cores to support this ability. To request 2 compute nodes with 256 tasks:
$ sbatch --nodes=2 --ntasks=256 myjobsubmissionfile
If more convenient, you may also specify any command line options to sbatch from within your job submission file, using a special form of comment:
#!/bin/sh -l
# FILENAME: myjobsubmissionfile
#SBATCH -A myallocation
#SBATCH -p queue-name # the default queue is "shared" queue
#SBATCH --nodes=1
#SBATCH --ntasks=1
#SBATCH --time=1:30:00
#SBATCH --job-name myjobname
module purge # Unload all loaded modules and reset everything to original state.
module load ...
...
module list # List currently loaded modules.
# Print the hostname of the compute node on which this job is running.
hostname
If an option is present in both your job submission file and on the command line, the option on the command line will take precedence.
After you submit your job with sbatch
, it may wait in the queue for minutes, hours, or even days. How long it takes for a job to start depends on the specific queue, the available resources, and time requested, and other jobs that are already waiting in that queue. It is impossible to say for sure when any given job will start. For best results, request no more resources than your job requires.
Once your job is submitted, you can monitor the job status, wait for the job to complete, and check the job output.
Link to section 'Checking Job Status' of 'Batch Jobs' Checking Job Status
Once a job is submitted there are several commands you can use to monitor the progress of the job. To see your jobs, use the squeue -u
command and specify your username.
$ squeue -u myusername
JOBID PARTITION NAME USER ST TIME NODES NODELIST(REASON)
188 wholenode job1 myusername R 0:14 2 a[010-011]
189 wholenode job2 myusername R 0:15 1 a012
To retrieve useful information about your queued or running job, use the scontrol show job
command with your job's ID number.
$ scontrol show job 189
JobId=189 JobName=myjobname
UserId=myusername GroupId=mygroup MCS_label=N/A
Priority=103076 Nice=0 Account=myacct QOS=normal
JobState=RUNNING Reason=None Dependency=(null)
Requeue=1 Restarts=0 BatchFlag=0 Reboot=0 ExitCode=0:0
RunTime=00:01:28 TimeLimit=00:30:00 TimeMin=N/A
SubmitTime=2021-10-04T14:59:52 EligibleTime=2021-10-04T14:59:52
AccrueTime=Unknown
StartTime=2021-10-04T14:59:52 EndTime=2021-10-04T15:29:52 Deadline=N/A
SuspendTime=None SecsPreSuspend=0 LastSchedEval=2021-10-04T14:59:52 Scheduler=Main
Partition=wholenode AllocNode:Sid=login05:1202865
ReqNodeList=(null) ExcNodeList=(null)
NodeList=a010
BatchHost=a010
NumNodes=1 NumCPUs=1 NumTasks=1 CPUs/Task=1 ReqB:S:C:T=0:0:*:*
TRES=cpu=1,mem=257526M,node=1,billing=1
Socks/Node=* NtasksPerN:B:S:C=0:0:*:* CoreSpec=*
MinCPUsNode=1 MinMemoryNode=257526M MinTmpDiskNode=0
Features=(null) DelayBoot=00:00:00
OverSubscribe=OK Contiguous=0 Licenses=(null) Network=(null)
Command=(null)
WorkDir=/home/myusername/jobdir
Power=
JobState
lets you know if the job is Pending, Running, Completed, or Held.RunTime and TimeLimit
will show how long the job has run and its maximum time.SubmitTime
is when the job was submitted to the cluster.- The job's number of Nodes, Tasks, Cores (CPUs) and CPUs per Task are shown.
WorkDir
is the job's working directory.StdOut
andStderr
are the locations of stdout and stderr of the job, respectively.Reason
will show why aPENDING
job isn't running.
You can check the predicted wait time for a queued job by running wait_time -j {your_job_id}
For historic (completed) jobs, you can use the jobinfo
command. While not as detailed as scontrol output, it can also report information on jobs that are no longer active.
Link to section 'Checking Job Output' of 'Batch Jobs' Checking Job Output
Once a job is submitted, and has started, it will write its standard output and standard error to files that you can read.
SLURM catches output written to standard output and standard error - what would be printed to your screen if you ran your program interactively. Unless you specified otherwise, SLURM will put the output in the directory where you submitted the job in a file named slurm- followed by the job id, with the extension out. For example slurm-3509.out
. Note that both stdout and stderr will be written into the same file, unless you specify otherwise.
If your program writes its own output files, those files will be created as defined by the program. This may be in the directory where the program was run, or may be defined in a configuration or input file. You will need to check the documentation for your program for more details.
Link to section 'Redirecting Job Output' of 'Batch Jobs' Redirecting Job Output
It is possible to redirect job output to somewhere other than the default location with the --error
and --output
directives:
#! /bin/sh -l
#SBATCH --output=/path/myjob.out
#SBATCH --error=/path/myjob.out
# This job prints "Hello World" to output and exits
echo "Hello World"
Link to section 'Holding a Job' of 'Batch Jobs' Holding a Job
Sometimes you may want to submit a job but not have it run just yet. You may be wanting to allow lab mates to cut in front of you in the queue - so hold the job until their jobs have started, and then release yours.
To place a hold on a job before it starts running, use the scontrol hold job command:
$ scontrol hold job myjobid
Once a job has started running it can not be placed on hold.
To release a hold on a job, use the scontrol release job command:
$ scontrol release job myjobid
Link to section 'Job Dependencies' of 'Batch Jobs' Job Dependencies
Dependencies are an automated way of holding and releasing jobs. Jobs with a dependency are held until the condition is satisfied. Once the condition is satisfied jobs only then become eligible to run and must still queue as normal.
Job dependencies may be configured to ensure jobs start in a specified order. Jobs can be configured to run after other job state changes, such as when the job starts or the job ends.
These examples illustrate setting dependencies in several ways. Typically dependencies are set by capturing and using the job ID from the last job submitted.
To run a job after job myjobid has started:
$ sbatch --dependency=after:myjobid myjobsubmissionfile
To run a job after job myjobid ends without error:
$ sbatch --dependency=afterok:myjobid myjobsubmissionfile
To run a job after job myjobid ends with errors:
$ sbatch --dependency=afternotok:myjobid myjobsubmissionfile
To run a job after job myjobid ends with or without errors:
$ sbatch --dependency=afterany:myjobid myjobsubmissionfile
To set more complex dependencies on multiple jobs and conditions:
$ sbatch --dependency=after:myjobid1:myjobid2:myjobid3,afterok:myjobid4 myjobsubmissionfile
Link to section 'Canceling a Job' of 'Batch Jobs' Canceling a Job
To stop a job before it finishes or remove it from a queue, use the scancel command:
$ scancel myjobid
Interactive Jobs
In addition to the ThinLinc and OnDemand interfaces, users can also choose to run interactive jobs on compute nodes to obtain a shell that they can interact with. This gives users the ability to type commands or use a graphical interface as if they were on a login node.
To submit an interactive job, use sinteractive
to run a login shell on allocated resources.
sinteractive
accepts most of the same resource requests as sbatch, so to request a login shell in the compute queue while allocating 2 nodes and 256 total cores, you might do:
$ sinteractive -p wholenode -N 2 -n 256 -A oneofyourallocations
You can check the predicted wait time for a queued job by running wait_time -j {your_job_id}
To quit your interactive job:
exit
or Ctrl-D
Example Jobs
A number of example jobs are available for you to look over and adapt to your own needs. The first few are generic examples, and latter ones go into specifics for particular software packages.
Generic SLURM Jobs
The following examples demonstrate the basics of SLURM jobs, and are designed to cover common job request scenarios. These example jobs will need to be modified to run your application or code.
Serial job in shared queue
This shows an example of a job submission file of the serial programs:
#!/bin/bash
# FILENAME: myjobsubmissionfile
#SBATCH -A myallocation # Allocation name
#SBATCH --nodes=1 # Total # of nodes (must be 1 for serial job)
#SBATCH --ntasks=1 # Total # of MPI tasks (should be 1 for serial job)
#SBATCH --time=1:30:00 # Total run time limit (hh:mm:ss)
#SBATCH -J myjobname # Job name
#SBATCH -o myjob.o%j # Name of stdout output file
#SBATCH -e myjob.e%j # Name of stderr error file
#SBATCH -p shared # Queue (partition) name
#SBATCH --mail-user=useremailaddress
#SBATCH --mail-type=all # Send email to above address at begin and end of job
# Manage processing environment, load compilers and applications.
module purge
module load compilername
module load applicationname
module list
# Launch serial code
./myexecutablefiles
If you would like to submit one serial job at a time, using shared
queue will only charge 1 core, instead of charging 128 cores for wholenode
queue.
MPI job in wholenode queue
An MPI job is a set of processes that take advantage of multiple compute nodes by communicating with each other. OpenMPI, Intel MPI (IMPI), and MVAPICH2 are implementations of the MPI standard.
This shows an example of a job submission file of the MPI programs:
#!/bin/bash
# FILENAME: myjobsubmissionfile
#SBATCH -A myallocation # Allocation name
#SBATCH --nodes=2 # Total # of nodes
#SBATCH --ntasks=256 # Total # of MPI tasks
#SBATCH --time=1:30:00 # Total run time limit (hh:mm:ss)
#SBATCH -J myjobname # Job name
#SBATCH -o myjob.o%j # Name of stdout output file
#SBATCH -e myjob.e%j # Name of stderr error file
#SBATCH -p wholenode # Queue (partition) name
#SBATCH --mail-user=useremailaddress
#SBATCH--mail-type=all # Send email to above address at begin and end of job
# Manage processing environment, load compilers and applications.
module purge
module load compilername
module load mpilibrary
module load applicationname
module list
# Launch MPI code
mpirun -np $SLURM_NTASKS ./myexecutablefiles
SLURM can run an MPI program with the srun command. The number of processes is requested with the -n option. If you do not specify the -n option, it will default to the total number of processor cores you request from SLURM.
If the code is built with OpenMPI, it can be run with a simple srun -n command. If it is built with Intel IMPI, then you also need to add the --mpi=pmi2 option: srun --mpi=pmi2 -n 256 ./mycode.exe in this example.
Invoking an MPI program on Anvil with ./myexecutablefiles is typically wrong, since this will use only one MPI process and defeat the purpose of using MPI. Unless that is what you want (rarely the case), you should use srun which is the Slurm analog of mpirun or mpiexec, or use mpirun or mpiexec to invoke an MPI program.
OpenMP job in wholenode queue
A shared-memory job is a single process that takes advantage of a multi-core processor and its shared memory to achieve parallelization.
When running OpenMP programs, all threads must be on the same compute node to take advantage of shared memory. The threads cannot communicate between nodes.
To run an OpenMP program, set the environment variable OMP_NUM_THREADS to the desired number of threads. This should almost always be equal to the number of cores on a compute node. You may want to set to another appropriate value if you are running several processes in parallel in a single job or node.
This example shows how to submit an OpenMP program, this job asked for 2 MPI tasks, each with 64 OpenMP threads for a total of 128 CPU-cores:
#!/bin/bash
# FILENAME: myjobsubmissionfile
#SBATCH -A myallocation # Allocation name
#SBATCH --nodes=1 # Total # of nodes (must be 1 for OpenMP job)
#SBATCH --ntasks-per-node=2 # Total # of MPI tasks per node
#SBATCH --cpus-per-task=64 # cpu-cores per task (default value is 1, >1 for multi-threaded tasks)
#SBATCH --time=1:30:00 # Total run time limit (hh:mm:ss)
#SBATCH -J myjobname # Job name
#SBATCH -o myjob.o%j # Name of stdout output file
#SBATCH -e myjob.e%j # Name of stderr error file
#SBATCH -p wholenode # Queue (partition) name
#SBATCH --mail-user=useremailaddress
#SBATCH --mail-type=all # Send email to above address at begin and end of job
# Manage processing environment, load compilers and applications.
module purge
module load compilername
module load applicationname
module list
# Set thread count (default value is 1).
export OMP_NUM_THREADS=$SLURM_CPUS_PER_TASK
# Launch OpenMP code
./myexecutablefiles
The ntasks x cpus-per-task should equal to or less than the total number of CPU cores on a node.
If an OpenMP program uses a lot of memory and 128 threads use all of the memory of the compute node, use fewer processor cores (OpenMP threads) on that compute node.
Hybrid job in wholenode queue
A hybrid program combines both MPI and shared-memory to take advantage of compute clusters with multi-core compute nodes. Libraries for OpenMPI, Intel MPI (IMPI), and MVAPICH2 and compilers which include OpenMP for C, C++, and Fortran are available.
This example shows how to submit a hybrid program, this job asked for 4 MPI tasks (with 2 MPI tasks per node), each with 64 OpenMP threads for a total of 256 CPU-cores:
#!/bin/bash
# FILENAME: myjobsubmissionfile
#SBATCH -A myallocation # Allocation name
#SBATCH --nodes=2 # Total # of nodes
#SBATCH --ntasks-per-node=2 # Total # of MPI tasks per node
#SBATCH --cpus-per-task=64 # cpu-cores per task (default value is 1, >1 for multi-threaded tasks)
#SBATCH --time=1:30:00 # Total run time limit (hh:mm:ss)
#SBATCH -J myjobname # Job name
#SBATCH -o myjob.o%j # Name of stdout output file
#SBATCH -e myjob.e%j # Name of stderr error file
#SBATCH -p wholenode # Queue (partition) name
#SBATCH --mail-user=useremailaddress
#SBATCH --mail-type=all # Send email at begin and end of job
# Manage processing environment, load compilers and applications.
module purge
module load compilername
module load mpilibrary
module load applicationname
module list
# Set thread count (default value is 1).
export OMP_NUM_THREADS=$SLURM_CPUS_PER_TASK
# Launch MPI code
mpirun -np $SLURM_NTASKS ./myexecutablefiles
The ntasks x cpus-per-task should equal to or less than the total number of CPU cores on a node.
GPU job in GPU queue
The Anvil cluster nodes contain GPUs that support CUDA and OpenCL. See the detailed hardware overview for the specifics on the GPUs in Anvil or use sfeatures
command to see the detailed hardware overview..
Link to section 'How to use Slurm to submit a SINGLE-node GPU program:' of 'GPU job in GPU queue' How to use Slurm to submit a SINGLE-node GPU program:
#!/bin/bash
# FILENAME: myjobsubmissionfile
#SBATCH -A myGPUallocation # allocation name
#SBATCH --nodes=1 # Total # of nodes
#SBATCH --ntasks-per-node=1 # Number of MPI ranks per node (one rank per GPU)
#SBATCH --gpus-per-node=1 # Number of GPUs per node
#SBATCH --time=1:30:00 # Total run time limit (hh:mm:ss)
#SBATCH -J myjobname # Job name
#SBATCH -o myjob.o%j # Name of stdout output file
#SBATCH -e myjob.e%j # Name of stderr error file
#SBATCH -p gpu # Queue (partition) name
#SBATCH --mail-user=useremailaddress
#SBATCH --mail-type=all # Send email to above address at begin and end of job
# Manage processing environment, load compilers, and applications.
module purge
module load modtree/gpu
module load applicationname
module list
# Launch GPU code
./myexecutablefiles
Link to section 'How to use Slurm to submit a MULTI-node GPU program:' of 'GPU job in GPU queue' How to use Slurm to submit a MULTI-node GPU program:
#!/bin/bash
# FILENAME: myjobsubmissionfile
#SBATCH -A myGPUallocation # allocation name
#SBATCH --nodes=2 # Total # of nodes
#SBATCH --ntasks-per-node=4 # Number of MPI ranks per node (one rank per GPU)
#SBATCH --gpus-per-node=4 # Number of GPUs per node
#SBATCH --time=1:30:00 # Total run time limit (hh:mm:ss)
#SBATCH -J myjobname # Job name
#SBATCH -o myjob.o%j # Name of stdout output file
#SBATCH -e myjob.e%j # Name of stderr error file
#SBATCH -p gpu # Queue (partition) name
#SBATCH --mail-user=useremailaddress
#SBATCH --mail-type=all # Send email to above address at begin and end of job
# Manage processing environment, load compilers, and applications.
module purge
module load modtree/gpu
module load applicationname
module list
# Launch GPU code
mpirun -np $SLURM_NTASKS ./myexecutablefiles
Make sure to use --gpus-per-node
command, otherwise, your job may not run properly.
NGC GPU container job in GPU queue
Link to section 'What is NGC?' of 'NGC GPU container job in GPU queue' What is NGC?
Nvidia GPU Cloud (NGC) is a GPU-accelerated cloud platform optimized for deep learning and scientific computing. NGC offers a comprehensive catalogue of GPU-accelerated containers, so the application runs quickly and reliably in the high-performance computing environment. Anvil team deployed NGC to extend the cluster capabilities and to enable powerful software and deliver the fastest results. By utilizing Singularity and NGC, users can focus on building lean models, producing optimal solutions, and gathering faster insights. For more information, please visit https://www.nvidia.com/en-us/gpu-cloud and NGC software catalog.
Link to section ' Getting Started ' of 'NGC GPU container job in GPU queue' Getting Started
Users can download containers from the NGC software catalog and run them directly using Singularity instructions from the corresponding container’s catalog page.
In addition, a subset of pre-downloaded NGC containers wrapped into convenient software modules are provided. These modules wrap underlying complexity and provide the same commands that are expected from non-containerized versions of each application.
On Anvil, type the command below to see the lists of NGC containers we deployed.
$ module load modtree/gpu
$ module load ngc
$ module avail
Once module loaded ngc, you can run your code as with normal non-containerized applications. This section illustrates how to use SLURM to submit a job with a containerized NGC program.
#!/bin/bash
# FILENAME: myjobsubmissionfile
#SBATCH -A myallocation # allocation name
#SBATCH --nodes=1 # Total # of nodes
#SBATCH --ntasks-per-node=1 # Number of MPI ranks per node (one rank per GPU)
#SBATCH --gres=gpu:1 # Number of GPUs per node
#SBATCH --time=1:30:00 # Total run time limit (hh:mm:ss)
#SBATCH -J myjobname # Job name
#SBATCH -o myjob.o%j # Name of stdout output file
#SBATCH -e myjob.e%j # Name of stderr error file
#SBATCH -p gpu # Queue (partition) name
#SBATCH --mail-user=useremailaddress
#SBATCH --mail-type=all # Send email to above address at begin and end of job
# Manage processing environment, load compilers, container, and applications.
module purge
module load modtree/gpu
module load ngc
module load applicationname
module list
# Launch GPU code
myexecutablefiles
BioContainers Collection
Link to section 'What is BioContainers?' of 'BioContainers Collection' What is BioContainers?
The BioContainers project came from the idea of using the containers-based technologies such as Docker or rkt for bioinformatics software. Having a common and controllable environment for running software could help to deal with some of the current problems during software development and distribution. BioContainers is a community-driven project that provides the infrastructure and basic guidelines to create, manage and distribute bioinformatics containers with a special focus on omics fields such as proteomics, genomics, transcriptomics, and metabolomics. For more information, please visit BioContainers project.
Link to section ' Getting Started ' of 'BioContainers Collection' Getting Started
Users can download bioinformatic containers from the BioContainers.pro and run them directly using Singularity instructions from the corresponding container’s catalog page.
Detailed Singularity user guide is available at: sylabs.io/guides/3.8/user-guideIn addition, Anvil team provides a subset of pre-downloaded biocontainers wrapped into convenient software modules. These modules wrap underlying complexity and provide the same commands that are expected from non-containerized versions of each application.
On Anvil, type the command below to see the lists of biocontainers we deployed.
$ module purge
$ module load modtree/cpu
$ module load biocontainers
$ module avail
Once module loaded biocontainers, you can run your code as with normal non-containerized applications. This section illustrates how to use SLURM to submit a job with a biocontainers program.
#!/bin/bash
# FILENAME: myjobsubmissionfile
#SBATCH -A myallocation # allocation name
#SBATCH --nodes=1 # Total # of nodes
#SBATCH --ntasks-per-node=1 # Number of MPI ranks per node
#SBATCH --time=1:30:00 # Total run time limit (hh:mm:ss)
#SBATCH -J myjobname # Job name
#SBATCH -o myjob.o%j # Name of stdout output file
#SBATCH -e myjob.e%j # Name of stderr error file
#SBATCH -p wholenode # Queue (partition) name
#SBATCH --mail-user=useremailaddress
#SBATCH --mail-type=all # Send email to above address at begin and end of job
# Manage processing environment, load compilers, container, and applications.
module purge
module load modtree/cpu
module load biocontainers
module load applicationname
module list
# Launch code
./myexecutablefiles
Monitoring Resources
Knowing the precise resource utilization an application had during a job, such as CPU load or memory, can be incredibly useful. This is especially the case when the application isn't performing as expected.
One approach is to run a program like htop
during an interactive job and keep an eye on system resources. You can get precise time-series data from nodes associated with your job using XDmod as well, online. But these methods don't gather telemetry in an automated fashion, nor do they give you control over the resolution or format of the data.
As a matter of course, a robust implementation of some HPC workload would include resource utilization data as a diagnostic tool in the event of some failure.
The monitor
utility is a simple command line system resource monitoring tool for gathering such telemetry and is available as a module.
module load monitor
Complete documentation is available online at resource-monitor.readthedocs.io. A full manual page is also available for reference, man monitor
.
In the context of a SLURM job you will need to put this monitoring task in the background to allow the rest of your job script to proceed. Be sure to interrupt these tasks at the end of your job.
#!/bin/bash
# FILENAME: monitored_job.sh
module load monitor
# track CPU load
monitor cpu percent >cpu-percent.log &
CPU_PID=$!
# track GPU load if any
monitor gpu percent >gpu-percent.log &
GPU_PID=$!
# your code here
# shut down the resource monitors
kill -s INT $CPU_PID $GPU_PID
A particularly elegant solution would be to include such tools in your prologue script and have the tear down in your epilogue script.
For large distributed jobs spread across multiple nodes, mpiexec
can be used to gather telemetry from all nodes in the job. The hostname is included in each line of output so that data can be grouped as such. A concise way of constructing the needed list of hostnames in SLURM is to simply use srun hostname | sort -u
.
#!/bin/bash
# FILENAME: monitored_job.sh
module load monitor
# track all CPUs (one monitor per host)
mpiexec -machinefile <(srun hostname | sort -u) \
monitor cpu percent --all-cores >cpu-percent.log &
CPU_PID=$!
# track all GPUs if any (one monitor per host)
mpiexec -machinefile <(srun hostname | sort -u) \
monitor gpu percent >gpu-percent.log &
GPU_PID=$!
# your code here
# shut down the resource monitors
kill -s INT $CPU_PID $GPU_PID
To get resource data in a more readily computable format, the monitor
program can be told to output in CSV format with the --csv
flag.
monitor cpu memory --csv >cpu-memory.csv
Or for GPU
monitor gpu memory --csv >gpu-memory.csv
For a distributed job you will need to suppress the header lines otherwise one will be created by each host.
monitor cpu memory --csv | head -1 >cpu-memory.csv
mpiexec -machinefile <(srun hostname | sort -u) \
monitor cpu memory --csv --no-header >>cpu-memory.csv
Or for GPU
monitor gpu memory --csv | head -1 >gpu-memory.csv
mpiexec -machinefile <(srun hostname | sort -u) \
monitor gpu memory --csv --no-header >>gpu-memory.csv
Specific Applications
The following examples demonstrate job submission files for some common real-world applications.
See the Generic SLURM Examples section for more examples on job submissions that can be adapted for use.
Python
Python is a high-level, general-purpose, interpreted, dynamic programming language. We suggest using Anaconda which is a Python distribution made for large-scale data processing, predictive analytics, and scientific computing. For example, to use the default Anaconda distribution:
$ module load anaconda
For a full list of available Anaconda and Python modules enter:
$ module spider anaconda
Example Python Jobs
This section illustrates how to submit a small Python job to a PBS queue.
Link to section 'Example 1: Hello world' of 'Example Python Jobs' Example 1: Hello world
Prepare a Python input file with an appropriate filename, here named myjob.in:
# FILENAME: hello.py
import string, sys
print "Hello, world!"
Prepare a job submission file with an appropriate filename, here named myjob.sub:
#!/bin/bash
# FILENAME: myjob.sub
module load anaconda
python hello.py
Basic knowledge about Batch Jobs.
Hello, world!
Link to section 'Example 2: Matrix multiply' of 'Example Python Jobs' Example 2: Matrix multiply
Save the following script as matrix.py:
# Matrix multiplication program
x = [[3,1,4],[1,5,9],[2,6,5]]
y = [[3,5,8,9],[7,9,3,2],[3,8,4,6]]
result = [[sum(a*b for a,b in zip(x_row,y_col)) for y_col in zip(*y)] for x_row in x]
for r in result:
print(r)
Change the last line in the job submission file above to read:
python matrix.py
The standard output file from this job will result in the following matrix:
[28, 56, 43, 53]
[65, 122, 59, 73]
[63, 104, 54, 60]
Link to section 'Example 3: Sine wave plot using numpy and matplotlib packages' of 'Example Python Jobs' Example 3: Sine wave plot using numpy and matplotlib packages
Save the following script as sine.py:
import numpy as np
import matplotlib
matplotlib.use('Agg')
import matplotlib.pylab as plt
x = np.linspace(-np.pi, np.pi, 201)
plt.plot(x, np.sin(x))
plt.xlabel('Angle [rad]')
plt.ylabel('sin(x)')
plt.axis('tight')
plt.savefig('sine.png')
Change your job submission file to submit this script and the job will output a png file and blank standard output and error files.
For more information about Python:
Installing Packages
We recommend installing Python packages in an Anaconda environment. One key advantage of Anaconda is that it allows users to install unrelated packages in separate self-contained environments. Individual packages can later be reinstalled or updated without impacting others.
To facilitate the process of creating and using Conda environments, we support a script (conda-env-mod) that generates a module file for an environment, as well as an optional Jupyter kernel to use this environment in a Jupyter.
You must load one of the conda modules in order to use this script.
$ module load conda
Step-by-step instructions for installing custom Python packages are presented below.
Link to section 'Step 1: Create a conda environment' of 'Installing Packages' Step 1: Create a conda environment
Users can use the conda-env-mod script to create an empty conda environment. This script needs either a name or a path for the desired environment. After the environment is created, it generates a module file for using it in future. Please note that conda-env-mod is different from the official conda-env script and supports a limited set of subcommands. Detailed instructions for using conda-env-mod can be found with the command conda-env-mod --help.
-
Example 1: Create a conda environment named mypackages in user's home directory.
$ conda-env-mod create -n mypackages -y
Including the -y option lets you skip the prompt to install the package.
-
Example 2: Create a conda environment named mypackages at a custom location.
$ conda-env-mod create -p $PROJECT/apps/mypackages -y
Please follow the on-screen instructions while the environment is being created. After finishing, the script will print the instructions to use this environment.
... ... ... Preparing transaction: ...working... done Verifying transaction: ...working... done Executing transaction: ...working... done +---------------------------------------------------------------+ | To use this environment, load the following modules: | | module use $HOME/privatemodules | | module load conda-env/mypackages-py3.8.8 | | (then standard 'conda install' / 'pip install' / run scripts) | +---------------------------------------------------------------+ Your environment "mypackages" was created successfully.
Note down the module names, as you will need to load these modules every time you want to use this environment. You may also want to add the module load lines in your jobscript, if it depends on custom Python packages.
By default, module files are generated in your $HOME/privatemodules directory. The location of module files can be customized by specifying the -m /path/to/modules option.
- Example 3: Create a conda environment named labpackages in your group's $PROJECT folder and place the module file at a shared location for the group to use.
$ conda-env-mod create -p $PROJECT/apps/mypackages -m $PROJECT/etc/modules ... ... ... Preparing transaction: ...working... done Verifying transaction: ...working... done Executing transaction: ...working... done +----------------------------------------------------------------+ | To use this environment, load the following modules: | | module use /anvil/projects/x-mylab/etc/modules | | module load conda-env/mypackages-py3.8.8 | | (then standard 'conda install' / 'pip install' / run scripts) | +----------------------------------------------------------------+ Your environment "labpackages" was created successfully.
If you used a custom module file location, you need to run the module use command as printed by the script.
By default, only the environment and a module file are created (no Jupyter kernel). If you plan to use your environment in a Jupyter, you need to append a --jupyter flag to the above commands.
- Example 4: Create a Jupyter-enabled conda environment named labpackages in your group's $PROJECT folder and place the module file at a shared location for the group to use.
$ conda-env-mod create -p $PROJECT/apps/mypackages/labpackages -m $PROJECT/etc/modules --jupyter ... ... ... Jupyter kernel created: "Python (My labpackages Kernel)" ... ... ... Your environment "labpackages" was created successfully.
Link to section 'Step 2: Load the conda environment' of 'Installing Packages' Step 2: Load the conda environment
-
The following instructions assume that you have used conda-env-mod to create an environment named mypackages (Examples 1 or 2 above). If you used conda create instead, please use conda activate mypackages.
$ module use $HOME/privatemodules $ module load conda-env/mypackages-py3.8.8
Note that the conda-env module name includes the Python version that it supports (Python 3.8.8 in this example). This is same as the Python version in the anaconda module.
-
If you used a custom module file location (Example 3 above), please use module use to load the conda-env module.
$ module use /anvil/projects/x-mylab/etc/modules $ module load conda-env/mypackages-py3.8.8
Link to section 'Step 3: Install packages' of 'Installing Packages' Step 3: Install packages
Now you can install custom packages in the environment using either conda install or pip install.
Link to section 'Installing with conda' of 'Installing Packages' Installing with conda
-
Example 1: Install OpenCV (open-source computer vision library) using conda.
$ conda install opencv
-
Example 2: Install a specific version of OpenCV using conda.
$ conda install opencv=3.1.0
Link to section 'Installing with pip' of 'Installing Packages' Installing with pip
-
Example 4: Install mpi4py using pip.
$ pip install mpi4py
-
Example 5: Install a specific version of mpi4py using pip.
$ pip install mpi4py==3.0.3
Follow the on-screen instructions while the packages are being installed. If installation is successful, please proceed to the next section to test the packages.
Note: Do NOT run Pip with the --user argument, as that will install packages in a different location.
Link to section 'Step 4: Test the installed packages' of 'Installing Packages' Step 4: Test the installed packages
To use the installed Python packages, you must load the module for your conda environment. If you have not loaded the conda-env module, please do so following the instructions at the end of Step 1.
$ module use $HOME/privatemodules
$ module load conda-env/mypackages-py3.8.8
- Example 1: Test that OpenCV is available.
$ python -c "import cv2; print(cv2.__version__)"
- Example 2: Test that mpi4py is available.
$ python -c "import mpi4py; print(mpi4py.__version__)"
If the commands are finished without errors, then the installed packages can be used in your program.
Link to section 'Additional capabilities of conda-env-mod' of 'Installing Packages' Additional capabilities of conda-env-mod
The conda-env-mod tool is intended to facilitate the creation of a minimal Anaconda environment, matching module file, and optionally a Jupyter kernel. Once created, the environment can then be accessed via familiar module load command, tuned and expanded as necessary. Additionally, the script provides several auxiliary functions to help manage environments, module files, and Jupyter kernels.
General usage for the tool adheres to the following pattern:
$ conda-env-mod help
$ conda-env-mod [optional arguments]
where required arguments are one of
- -n|--name ENV_NAME (name of the environment)
- -p|--prefix ENV_PATH (location of the environment)
and optional arguments further modify behavior for specific actions (e.g. -m to specify alternative location for generated module file).
Given a required name or prefix for an environment, the conda-env-mod script supports the following subcommands:
- create - to create a new environment, its corresponding module file and optional Jupyter kernel.
- delete - to delete existing environment along with its module file and Jupyter kernel.
- module - to generate just the module file for a given existing environment.
- kernel - to generate just the Jupyter kernel for a given existing environment (note that the environment has to be created with a --jupyter option).
- help - to display script usage help.
Using these subcommands, you can iteratively fine-tune your environments, module files and Jupyter kernels, as well as delete and re-create them with ease. Below we cover several commonly occurring scenarios.
Link to section 'Generating module file for an existing environment' of 'Installing Packages' Generating module file for an existing environment
If you already have an existing configured Anaconda environment and want to generate a module file for it, follow appropriate examples from Step 1 above, but use the module subcommand instead of the create one. E.g.
$ conda-env-mod module -n mypackages
and follow printed instructions on how to load this module. With an optional --jupyter flag, a Jupyter kernel will also be generated.
Note that if you intend to proceed with a Jupyter kernel generation (via the --jupyter flag or a kernel subcommand later), you will have to ensure that your environment has ipython and ipykernel packages installed into it. To avoid this and other related complications, we highly recommend making a fresh environment using a suitable conda-env-mod create .... --jupyter command instead.
Link to section 'Generating Jupyter kernel for an existing environment' of 'Installing Packages' Generating Jupyter kernel for an existing environment
If you already have an existing configured Anaconda environment and want to generate a Jupyter kernel file for it, you can use the kernel subcommand. E.g.
$ conda-env-mod kernel -n mypackages
This will add a "Python (My mypackages Kernel)" item to the dropdown list of available kernels upon your next time use Jupyter.
Note that generated Jupiter kernels are always personal (i.e. each user has to make their own, even for shared environments). Note also that you (or the creator of the shared environment) will have to ensure that your environment has ipython and ipykernel packages installed into it.
Link to section 'Managing and using shared Python environments' of 'Installing Packages' Managing and using shared Python environments
Here is a suggested workflow for a common group-shared Anaconda environment with Jupyter capabilities:
The PI or lab software manager:
-
Creates the environment and module file (once):
$ module purge $ module load conda $ conda-env-mod create -p $PROJECT/apps/labpackages -m $PROJECT/etc/modules --jupyter
-
Installs required Python packages into the environment (as many times as needed):
$ module use /anvil/projects/x-mylab/etc/modules $ module load conda-env/labpackages-py3.8.8 $ conda install ....... # all the necessary packages
Lab members:
-
Lab members can start using the environment in their command line scripts or batch jobs simply by loading the corresponding module:
$ module use /anvil/projects/x-mylab/etc/modules $ module load conda-env/labpackages-py3.8.8 $ python my_data_processing_script.py .....
-
To use the environment in Jupyter, each lab member will need to create his/her own Jupyter kernel (once). This is because Jupyter kernels are private to individuals, even for shared environments.
$ module use /anvil/projects/x-mylab/etc/modules $ module load conda-env/labpackages-py3.8.8 $ conda-env-mod kernel -p $PROJECT/apps/labpackages
A similar process can be devised for instructor-provided or individually-managed class software, etc.
Link to section 'Troubleshooting' of 'Installing Packages' Troubleshooting
- Python packages often fail to install or run due to dependency with other packages. More specifically, if you previously installed packages in your home directory it is safer to clean those installations.
$ mv ~/.local ~/.local.bak $ mv ~/.cache ~/.cache.bak
- Unload all the modules.
$ module purge
- Clean up PYTHONPATH.
$ unset PYTHONPATH
- Next load the modules (e.g. anaconda) that you need.
$ module load conda $ module module use $HOME/privatemodules $ module load conda-env/mypackages-py3.8.8
- Now try running your code again.
- Few applications only run on specific versions of Python (e.g. Python 3.6). Please check the documentation of your application if that is the case.
Singularity
Note: Singularity was originally a project out of Lawrence Berkeley National Laboratory. It has now been spun off into a distinct offering under a new corporate entity under the name Sylabs Inc. This guide pertains to the open source community edition, SingularityCE.
Link to section 'What is Singularity?' of 'Singularity' What is Singularity?
Singularity is a powerful tool allowing the portability and reproducibility of operating system and application environments through the use of Linux containers. It gives users complete control over their environment.
Singularity is like Docker but tuned explicitly for HPC clusters. More information is available from the project’s website.
Link to section 'Features' of 'Singularity' Features
- Run the latest applications on an Ubuntu or Centos userland
- Gain access to the latest developer tools
- Launch MPI programs easily
- Much more
Singularity’s user guide is available at: sylabs.io/guides/3.8/user-guide
Link to section 'Example' of 'Singularity' Example
Here is an example of downloading a pre-built Docker container image, converting it into Singularity format and running it on Anvil:
$ singularity pull docker://sylabsio/lolcow:latest
INFO: Converting OCI blobs to SIF format
INFO: Starting build...
[....]
INFO: Creating SIF file...
$ singularity exec lolcow_latest.sif cowsay "Hello, world"
______________
< Hello, world >
--------------
\ ^__^
\ (oo)\_______
(__)\ )\/\
||----w |
|| ||
Link to section 'Anvil Cluster Specific Notes' of 'Singularity' Anvil Cluster Specific Notes
All service providers will integrate Singularity slightly differently depending on site. The largest customization will be which default files are inserted into your images so that routine services will work.
Services we configure for your images include DNS settings and account information. File systems we overlay into your images are your home directory, scratch, project space, datasets, and application file systems.
Here is a list of paths:
- /etc/resolv.conf
- /etc/hosts
- /home/$USER
- /apps
- /anvil (including /anvil/scratch, /anvil/projects, and /anvil/datasets)
This means that within the container environment these paths will be present and the same as outside the container. The /apps
and /anvil
directories will need to exist inside your container to work properly.
Link to section 'Creating Singularity Images' of 'Singularity' Creating Singularity Images
Due to how singularity containers work, you must have root privileges to build an image. Once you have a singularity container image built on your own system, you can copy the image file up to the cluster (you do not need root privileges to run the container).
You can find information and documentation for how to install and use singularity on your system:
We have version 3.8.0
on the cluster. You will most likely not be able to run any container built with any singularity past that version. So be sure to follow the installation guide for version 3.8 on your system.
$ singularity --version
singularity version 3.8.0-1.el8
Everything you need on how to build a container is available from their user-guide. Below are merely some quick tips for getting your own containers built for Anvil.
You can use a Container Recipe to both build your container and share its specification with collaborators (for the sake of reproducibility). Here is a simplistic example of such a file:
# FILENAME: Buildfile
Bootstrap: docker
From: ubuntu:18.04
%post
apt-get update && apt-get upgrade -y
mkdir /apps /anvil
To build the image itself:
$ sudo singularity build ubuntu-18.04.sif Buildfile
The challenge with this approach however is that it must start from scratch if you decide to change something. In order to create a container image iteratively and interactively, you can use the --sandbox
option.
$ sudo singularity build --sandbox ubuntu-18.04 docker://ubuntu:18.04
This will not create a flat image file but a directory tree (i.e., a folder), the contents of which are the container's filesystem. In order to get a shell inside the container that allows you to modify it, user the --writable
option.
$ sudo singularity shell --writable ubuntu-18.04
Singularity: Invoking an interactive shell within container...
Singularity ubuntu-18.04.sandbox:~>
You can then proceed to install any libraries, software, etc. within the container. Then to create the final image file, exit
the shell and call the build
command once more on the sandbox.
$ sudo singularity build ubuntu-18.04.sif ubuntu-18.04
Finally, copy the new image to Anvil and run it.
Distributed Deep Learning with Horovod
Link to section 'What is Horovod?' of 'Distributed Deep Learning with Horovod' What is Horovod?
Horovod is a framework originally developed by Uber for distributed deep learning. While a traditionally laborious process, Horovod makes it easy to scale up training scripts from single GPU to multi-GPU processes with minimal code changes. Horovod enables quick experimentation while also ensuring efficient scaling, making it an attractive choice for multi-GPU work.
Link to section 'Installing Horovod' of 'Distributed Deep Learning with Horovod' Installing Horovod
Before continuing, ensure you have loaded the following modules by running:
ml modtree/gpu
ml learning
Next, load the module for the machine learning framework you are using. Examples for tensorflow and pytorch are below:
ml ml-toolkit-gpu/tensorflow
ml ml-toolkit-gpu/pytorch
Create or activate the environment you want Horovod to be installed in then install the following dependencies:
pip install pyparsing
pip install filelock
Finally, install Horovod. The following command will install Horovod with support for both Tensorflow and Pytorch, but if you do not need both simply remove the HOROVOD_WITH_...=1
part of the command.
HOROVOD_WITH_TENSORFLOW=1 HOROVOD_WITH_TORCH=1 pip install horovod[all-frameworks]
Link to section 'Submitting Jobs' of 'Distributed Deep Learning with Horovod' Submitting Jobs
It is highly recommended that you run Horovod within batch jobs instead of interactive jobs. For information about how to format a submission file and submit a batch job, please reference Batch Jobs. Ensure you load the modules listed above as well as your environment in the submission script.
Finally, this line will actually launch your Horovod script inside your job. You will need to limit the number of processes to the number of GPUs you requested.
horovodrun -np {number_of_gpus} python {path/to/training/script.py}
An example usage of this is as follows for 4 GPUs and a file called horovod_mnist.py:
horovodrun -np 4 python horovod_mnist.py
Link to section 'Writing Horovod Code' of 'Distributed Deep Learning with Horovod' Writing Horovod Code
It is relatively easy to incorporate Horovod into existing training scripts. The main additional elements you need to incorporate are listed below (syntax for use with pytorch), but much more information, including syntax for other frameworks, can be found on the Horovod website.
#import required horovod framework -- e.g. for pytorch:
import horovod.torch as hvd
# Initialize Horovod
hvd.init()
# Pin to a GPU
if torch.cuda.is_available():
torch.cuda.set_device(hvd.local_rank())
#Split dataset among workers
train_sampler = torch.utils.data.distributed.DistributedSampler(
train_dataset, num_replicas=hvd.size(), rank=hvd.rank())
#Build Model
#Wrap optimizer with Horovod DistributedOptimizer
optimizer = hvd.DistributedOptimizer(optimizer, named_parameters=model.named_parameters())
#Broadcast initial variable states from first worker to all others
hvd.broadcast_parameters(model.state_dict(), root_rank=0)
#Train model
Gromacs
This shows an example job submission file for running Gromacs on Anvil. The Gromacs versions can be changed depends on the available modules on Anvil.
#!/bin/bash
# FILENAME: myjobsubmissionfile
#SBATCH -A myallocation # Allocation name (run 'mybalance' command to find)
#SBATCH -p shared #Queue (partition) name
#SBATCH --nodes=1 # Total # of nodes
#SBATCH --ntasks=16 # Total # of MPI tasks
#SBATCH --time=96:00:00 # Total run time limit (hh:mm:ss)
#SBATCH --job-name myjob # Job name
#SBATCH -o myjob.o%j # Name of stdout output file
#SBATCH -e myjob.e%j # Name of stderr error file
# Manage processing environment, load compilers and applications.
module --force purge
module load gcc/11.2.0
module load openmpi/4.0.6
module load gromacs/2021.2
module list
# Launch md jobs
#energy minimizations
mpirun -np 1 gmx_mpi grompp -f minim.mdp -c myjob.gro -p topol.top -o em.tpr
mpirun gmx_mpi mdrun -v -deffnm em
#nvt run
mpirun -np 1 gmx_mpi grompp -f nvt.mdp -c em.gro -r em.gro -p topol.top -o nvt.tpr
mpirun gmx_mpi mdrun -deffnm nvt
#npt run
mpirun -np 1 gmx_mpi grompp -f npt.mdp -c nvt.gro -r nvt.gro -t nvt.cpt -p topol.top -o npt.tpr
mpirun gmx_mpi mdrun -deffnm npt
#md run
mpirun -np 1 gmx_mpi grompp -f md.mdp -c npt.gro -t npt.cpt -p topol.top -o md.tpr
mpirun gmx_mpi mdrun -deffnm md
The GPU version of Gromacs was available within ngc container on Anvil. Here is an example job script.
#!/bin/bash
# FILENAME: myjobsubmissionfile
#SBATCH -A myallocation-gpu # Allocation name (run 'mybalance' command to find)
#SBATCH -p gpu #Queue (partition) name
#SBATCH --nodes=1 # Total # of nodes
#SBATCH --ntasks=16 # Total # of MPI tasks
#SBATCH --gpus-per-node=1 #Total # of GPUs
#SBATCH --time=96:00:00 # Total run time limit (hh:mm:ss)
#SBATCH --job-name myjob # Job name
#SBATCH -o myjob.o%j # Name of stdout output file
#SBATCH -e myjob.e%j # Name of stderr error file
# Manage processing environment, load compilers and applications.
module --force purge
module load modtree/gpu
module load ngc
module load gromacs
module list
# Launch md jobs
#energy minimizations
gmx grompp -f minim.mdp -c myjob.gro -p topol.top -o em.tpr
gmx mdrun -v -deffnm em -ntmpi 4 -ntomp 4
#nvt run
gmx grompp -f nvt.mdp -c em.gro -r em.gro -p topol.top -o nvt.tpr
gmx mdrun -deffnm nvt -ntmpi 4 -ntomp 4 -nb gpu -bonded gpu
#npt run
gmx grompp -f npt.mdp -c nvt.gro -r nvt.gro -t nvt.cpt -p topol.top -o npt.tpr
gmx mdrun -deffnm npt -ntmpi 4 -ntomp 4 -nb gpu -bonded gpu
#md run
gmx grompp -f md.mdp -c npt.gro -t npt.cpt -p topol.top -o md.tpr
gmx mdrun -deffnm md -ntmpi 4 -ntomp 4 -nb gpu -bonded gpu
VASP
This shows an example of a job submission file for running Anvil-built VASP with MPI jobs (CPU version):
#!/bin/sh
# FILENAME: myjobsubmissionfile
#SBATCH -A myallocation # Allocation name
#SBATCH --nodes=1 # Total # of nodes
#SBATCH --ntasks=64 # Total # of MPI tasks
#SBATCH --time=1:30:00 # Total run time limit (hh:mm:ss)
#SBATCH -J myjobname # Job name
#SBATCH -o myjob.o%j # Name of stdout output file
#SBATCH -e myjob.e%j # Name of stderr error file
#SBATCH -p shared # Queue (partition) name
# Manage processing environment, load compilers and applications.
module --force purge
module load gcc/11.2.0 openmpi/4.1.6
module load vasp/5.4.4.pl2 # or module load vasp/6.3.0
module list
# Launch MPI code
srun -n $SLURM_NTASKS --kill-on-bad-exit vasp_std # or mpirun -np $SLURM_NTASKS vasp_std
Here is an example job script to run a GPU-VASP job on Anvil.
#!/bin/sh
# FILENAME: myjobsubmissionfile
#SBATCH -A myallocation # Allocation name
#SBATCH --nodes=1 # Total # of nodes
#SBATCH --ntasks=1 # Total # of MPI tasks
#SBATCH --gpus-per-node=1 # Total # of GPUs
#SBATCH --time=1:30:00 # Total run time limit (hh:mm:ss)
#SBATCH -J vasp_gpu # Job name
#SBATCH -o myjob.o%j # Name of stdout output file
#SBATCH -e myjob.e%j # Name of stderr error file
#SBATCH -p gpu # Queue (partition) name
# Manage processing environment, load compilers and applications.
module --force purge
module load modtree/gpu
module purge
module load vasp/6.3.0-gpu
module list
# Launch MPI code
mpirun -np $SLURM_NTASKS vasp_std
Windows Virtual Machine
Few scientific applications (such as ArcGIS, Tableau Desktop, etc.) can only be run in the Windows operating system. In order to facilitate research that uses these applications, Anvil provides an Open OnDemand application to launch a Windows virtual machine (VM) on Anvil compute nodes. The virtual machine is created using the QEMU/KVM emulator and it currently runs the Windows 11 professional operating system.
Link to section 'Important notes' of 'Windows Virtual Machine' Important notes
- The base Windows VM does not have any pre-installed applications and users must install their desired applications inside the VM.
- If the application requires a license, the researchers must purchase their own license and acquire a copy of the software.
- When you launch the Windows VM, it creates a copy of the VM in your scratch space. Any modifications you make to the VM (e.g. installing additional software) will be saved on your private copy and will persist across jobs.
- All Anvil filesystems (
$HOME
,$PROJECT
, and$CLUSTER_SCRATCH
) are available inside the VM as network drives. You can directly operate on files in your$CLUSTER_SCRATCH
.
Link to section 'How to launch Windows VM on Anvil' of 'Windows Virtual Machine' How to launch Windows VM on Anvil
- First login to the Anvil OnDemand portal using your ACCESS credentials.
- From the top menu go to Interactive Applications -> Windows11 Professional.
- In the next page, specify your allocation, queue, walltime, and number of cores. Currently, you must select all 128 cores on a node to run Windows VM. This is to avoid resource conflict among shared jobs.
- Click Launch.
- At this point, Open OnDemand will submit a job to the Anvil scheduler and wait for allocation.
- Once the job starts, you will be presented with a button to connect to the VNC server.
- Click on Launch Windows11 Professional to connect to the VNC display. You may initially see a Linux desktop which will eventually be replaced by the Windows desktop.
- A popup notification will show you the default username and password for the Windows VM. Please note this down. When you login to Windows for the first time, you can change the username and password to your desired username and password.
- Note that it may take upto 5 minutes for the Windows VM to launch properly. This is partly due to the large amount of memory allocated to the VM (216GB). Please wait patiently.
- Once you see the Windows desktop ready, you can proceed with your simulation or workflow.
Link to section 'Advanced use-cases' of 'Windows Virtual Machine' Advanced use-cases
If your workfow requires a different version of Windows, or if you need to launch a personal copy of Windows from a non-standard location, please send a support request from the ACCESS Support portal.
Managing and Transferring Files
File Systems
Anvil provides users with separate home, scratch, and project areas for managing files. These will be accessible via the $HOME
, $SCRATCH
, $PROJECT
and $WORK
environment variables. Each file system is available from all Anvil nodes but has different purge policies and ideal use cases (see table below). Users in the same allocation will share read and write access to the data in the $PROJECT
space. The project space will be created for each allocation. $PROJECT
and $WORK
variables refer to the same location and can be used interchangeably.
$SCRATCH
is a high-performance, internally resilient GPFS parallel file system with 10 PB of usable capacity, configured to deliver up to 150 GB/s bandwidth. The scratch filesystems are for limited-duration, high-performance storage of data for running jobs or workflows and are explicitly not intended to be used as a long-term storage. Doing so, or engaging in measures to circumvent purging, is adversely affecting all users of the system and is prohibited.
Files in scratch directories are not recoverable. Files in scratch directories are not backed up. If you accidentally delete a file, a disk crashes, or old files are purged, they cannot be restored.
File System | Mount Point | Quota | Snapshots | Purpose | Purge policy |
---|---|---|---|---|---|
Anvil ZFS | /home | 25 GB | Full schedule* | Home directories: area for storing personal software, scripts, compiling, editing, etc. | Not purged |
Anvil ZFS | /apps | N/A | Weekly* | Applications | |
Anvil GPFS | /anvil | N/A | No | ||
Anvil GPFS | /anvil/scratch | 100 TB | No | User scratch: area for job I/O activity, temporary storage | Files older than 30-day (access time) will be purged |
Anvil GPFS | /anvil/projects | 5 TB | Full schedule* | Per allocation: area for shared data in a project, common datasets and software installation | Not purged while allocation is active. Removed 90 days after allocation expiration |
Anvil GPFS | /anvil/datasets | N/A | Weekly* | Common data sets (not allocated to users) |
* Full schedule keeps nightly snapshots for 7 days, weekly snapshots for 3 weeks, and monthly snapshots for 2 months.
Link to section 'Useful tool' of 'File Systems' Useful tool
To check the quota of different file systems, type myquota
at the command line.
x-anvilusername@login03.anvil:[~] $myquota
Type Location Size Limit Use Files Limit Use
==============================================================================
home x-anvilusername 261.5MB 25.0GB 1% - - -
scratch anvil 6.3GB 100.0TB 0.01% 3k 1,048k 0.36%
projects accountname1 37.2GB 5.0TB 0.73% 403k 1,048k 39%
projects accountname2 135.8GB 5.0TB 3% 20k 1,048k 2%
Transferring Files
Anvil supports several methods for file transfer to and from the system. Users can transfer files between Anvil and Linux-based systems or Mac using either scp
or rsync
. Windows SSH clients typically include scp-based file transfer capabilities.
SCP
SCP (Secure CoPy) is a simple way of transferring files between two machines that use the SSH protocol. SCP is available as a protocol choice in some graphical file transfer programs and also as a command line program on most Linux, Unix, and Mac OS X systems. SCP can copy single files, but will also recursively copy directory contents if given a directory name. SSH Keys is required for SCP. Following is an example of transferring test.txt
file from Anvil home directory to your local machine, make sure to use your anvil username x-anvilusername
:
localhost> scp x-anvilusername@anvil.rcac.purdue.edu:/home/x-anvilusername/test.txt .
Warning: Permanently added the xxxxxxx host key for IP address 'xxx.xxx.xxx.xxx' to the list of known hosts.
test.txt 100% 0 0.0KB/s 00:00
Rsync
Rsync, or Remote Sync, is a free and efficient command-line tool that lets you transfer files and directories to local and remote destinations. It allows to copy only the changes from the source and offers customization, use for mirroring, performing backups, or migrating data between different filesystems. SSH Keys is required for Rsync. Similar to the above SCP example, make sure to use your anvil username x-anvilusername
here.
SFTP
SFTP (Secure File Transfer Protocol) is a reliable way of transferring files between two machines. SFTP is available as a protocol choice in some graphical file transfer programs and also as a command-line program on most Linux, Unix, and Mac OS X systems. SFTP has more features than SCP and allows for other operations on remote files, remote directory listing, and resuming interrupted transfers. Command-line SFTP cannot recursively copy directory contents; to do so, try using SCP or graphical SFTP client.
Command-line usage:
$ sftp -B buffersize x-anvilusername@anvil.rcac.purdue.edu
(to a remote system from local)
sftp> put sourcefile somedir/destinationfile
sftp> put -P sourcefile somedir/
(from a remote system to local)
sftp> get sourcefile somedir/destinationfile
sftp> get -P sourcefile somedir/
sftp> exit
- -B: optional, specify buffer size for transfer; larger may increase speed, but costs memory
- -P: optional, preserve file attributes and permissions
Linux / Solaris / AIX / HP-UX / Unix:
- The "sftp" command-line program should already be installed.
Microsoft Windows:
- MobaXterm
Free, full-featured, graphical Windows SSH, SCP, and SFTP client.
Mac OS X:
- The "sftp" command-line program should already be installed. You may start a local terminal window from "Applications->Utilities".
- Cyberduck is a full-featured and free graphical SFTP and SCP client.
Globus
Globus is a powerful and easy to use file transfer and sharing service for transferring files virtually anywhere. It works between any ACCESS and non-ACCESS sites running Globus, and it connects any of these research systems to personal systems. You may use Globus to connect to your home, scratch, and project storage directories on Anvil. Since Globus is web-based, it works on any operating system that is connected to the internet. The Globus Personal client is available on Windows, Linux, and Mac OS X. It is primarily used as a graphical means of transfer but it can also be used over the command line. More details can be found at ACCESS Using Globus.
Lost File Recovery
Your HOME and PROJECTS directories on Anvil are protected against accidental file deletion through a series of snapshots taken every night just after midnight. Each snapshot provides the state of your files at the time the snapshot was taken. It does so by storing only the files which have changed between snapshots. A file that has not changed between snapshots is only stored once but will appear in every snapshot. This is an efficient method of providing snapshots because the snapshot system does not have to store multiple copies of every file.
These snapshots are kept for a limited time at various intervals. Please refer to Anvil File Systems to see the frequency of generating snapshots on different mount points. Anvil keeps nightly snapshots for 7 days, weekly snapshots for 3 weeks, and monthly snapshots for 2 months. This means you will find snapshots from the last 7 nights, the last 3 Sundays, and the last 2 first of the months. Files are available going back between two and three months, depending on how long ago the last first of the month was. Snapshots beyond this are not kept.
Only files which have been saved during an overnight snapshot are recoverable. If you lose a file the same day you created it, the file is not recoverable because the snapshot system has not had a chance to save the file.
Snapshots are not a substitute for regular backups. It is the responsibility of the researchers to back up any important data to long-term storage space. Anvil does protect against hardware failures or physical disasters through other means however these other means are also not substitutes for backups.
Anvil offers several ways for researchers to access snapshots of their files.
flost
If you know when you lost the file, the easiest way is to use the flost command.
To run the tool you will need to specify the location where the lost file was with the -w argument:
$ flost -w /home
This script will help you try to recover lost home or group directory contents.
NB: Scratch directories are not backed up and cannot be recovered.
Currently anchoring the search under: /home
If your lost files were on a different filesystem, exit now with Ctrl-C and
rerun flost with a suitable '-w WHERE' argument (or see 'flost -h' for help).
Please enter the date that you lost your files: MM/DD/YYYY
The closest recovery snapshot to your date of loss currently available is from
MM/DD/YYYY 12:00am. First, you will need to SSH to a dedicated
service host zfs.anvil.rcac.purdue.edu, then change your directory
to the snapshot location:
$ ssh zfs.anvil.rcac.purdue.edu
$ cd /home/.zfs/snapshot/zfs-auto-snap_daily-YYYY-MM-DD-0000
$ ls
Then copy files or directories from there back to where they belong:
$ cp mylostfile /home
$ cp -r mylostdirectory /home
Here is an example of /home
directory. If you know more specifically where the lost file was you may provide the full path to that directory.
This tool will prompt you for the date on which you lost the file or would like to recover the file from. If the tool finds an appropriate snapshot it will provide instructions on how to search for and recover the file.
If you are not sure what date you lost the file you may try entering different dates into the flost to try to find the file or you may also manually browse the snapshots in /home/.zfs/snapshot
folder for Home directory and /anvil/projects/.snapshots
folder for Projects directory.
Software
Anvil provides a number of software packages to users of the system via the module
command. To check the list of applications installed as modules on Anvil and their user guides, please go to the Scientific Applications on ACCESS Anvil page. For some common applications such as Python, Singularity, Horovod and R, we also provide detailed instructions and examples on the Specific Applications page.
Module System
The Anvil cluster uses Lmod to manage the user environment, so users have access to the necessary software packages and versions to conduct their research activities. The associated module
command can be used to load applications and compilers, making the corresponding libraries and environment variables automatically available in the user environment.
Lmod is a hierarchical module system, meaning a module can only be loaded after loading the necessary compilers and MPI libraries that it depends on. This helps avoid conflicting libraries and dependencies being loaded at the same time. A list of all available modules on the system can be found with the module spider
command:
$ module spider # list all modules, even those not available due to incompatible with currently loaded modules
-----------------------------------------------------------------------------------
The following is a list of the modules and extensions currently available:
-----------------------------------------------------------------------------------
amdblis: amdblis/3.0
amdfftw: amdfftw/3.0
amdlibflame: amdlibflame/3.0
amdlibm: amdlibm/3.0
amdscalapack: amdscalapack/3.0
anaconda: anaconda/2021.05-py38
aocc: aocc/3.0
Lines 1-45
The module spider
command can also be used to search for specific module names.
$ module spider intel # all modules with names containing 'intel'
-----------------------------------------------------------------------------------
intel:
-----------------------------------------------------------------------------------
Versions:
intel/19.0.5.281
intel/19.1.3.304
Other possible modules matches:
intel-mkl
-----------------------------------------------------------------------------------
$ module spider intel/19.1.3.304 # additional details on a specific module
-----------------------------------------------------------------------------------
intel: intel/19.1.3.304
-----------------------------------------------------------------------------------
This module can be loaded directly: module load intel/19.1.3.304
Help:
Intel Parallel Studio.
When users log into Anvil, a default compiler (GCC), MPI libraries (OpenMPI), and runtime environments (e.g., Cuda on GPU-nodes) are automatically loaded into the user environment. It is recommended that users explicitly specify which modules and which versions are needed to run their codes in their job scripts via the module load
command. Users are advised not to insert module load
commands in their bash profiles, as this can cause issues during initialization of certain software (e.g. Thinlinc).
When users load a module, the module system will automatically replace or deactivate modules to ensure the packages you have loaded are compatible with each other. Following example shows that the module system automatically unload the default Intel compiler version to a user-specified version:
$ module load intel # load default version of Intel compiler
$ module list # see currently loaded modules
Currently Loaded Modules:
1) intel/19.0.5.281
$ module load intel/19.1.3.304 # load a specific version of Intel compiler
$ module list # see currently loaded modules
The following have been reloaded with a version change:
1) intel/19.0.5.281 => intel/19.1.3.304
Most modules on Anvil include extensive help messages, so users can take advantage of the module help APPNAME
command to find information about a particular application or module. Every module also contains two environment variables named $RCAC_APPNAME_ROOT
and $RCAC_APPNAME_VERSION
identifying its installation prefix and its version. This information can be found by module show APPNAME
. Users are encouraged to use generic environment variables such as CC, CXX, FC, MPICC, MPICXX etc. available through the compiler and MPI modules while compiling their code.
Link to section 'Some other common module commands:' of 'Module System' Some other common module commands:
To unload a module
$ module unload mymodulename
To unload all loaded modules and reset everything to original state.
$ module purge
To see all available modules that are compatible with current loaded modules
$ module avail
To display information about a specified module, including environment changes, dependencies, software version and path.
$ module show mymodulename
Compiling, performance, and optimization on Anvil
Anvil CPU nodes have GNU, Intel, and AOCC (AMD) compilers available along with multiple MPI implementations (OpenMPI, Intel MPI (IMPI) and MVAPICH2). Anvil GPU nodes also provide the PGI compiler. Users may want to note the following AMD Milan specific optimization options that can help improve the performance of your code on Anvil:
- The majority of the applications on Anvil are built using GCC 11.2.0 which features an AMD Milan specific optimization flag (
-march=znver3
). - AMD Milan CPUs support the Advanced Vector Extensions 2 (AVX2) vector instructions set. GNU, Intel, and AOCC compilers all have flags to support AVX2. Using AVX2, up to eight floating point operations can be executed per cycle per core, potentially doubling the performance relative to non-AVX2 processors running at the same clock speed.
- In order to enable AVX2 support, when compiling your code, use the
-march=znver3
flag (for GCC 11.2 and newer, Clang and AOCC compilers),-march=znver2
flag (for GCC 10.2), or-march=core-avx2
(for Intel compilers and GCC prior to 9.3).
Other Software Usage Notes:
- Use the same environment that you compile the code to run your executables. When switching between compilers for different applications, make sure that you load the appropriate modules before running your executables.
- Explicitly set the optimization level in your makefiles or compilation scripts. Most well written codes can safely use the highest optimization level (
-O3
), but many compilers set lower default levels (e.g. GNU compilers use the default-O0
, which turns off all optimizations). - Turn off debugging, profiling, and bounds checking when building executables intended for production runs as these can seriously impact performance. These options are all disabled by default. The flag used for bounds checking is compiler dependent, but the debugging (
-g
) and profiling (-pg
) flags tend to be the same for all major compilers. - Some compiler options are the same for all available compilers on Anvil (e.g.
-o
), while others are different. Many options are available in one compiler suite but not the other. For example, Intel, PGI, and GNU compilers use the-qopenmp
,-mp
, and-fopenmp
flags, respectively, for building OpenMP applications. - MPI compiler wrappers (e.g. mpicc, mpif90) all call the appropriate compilers and load the correct MPI libraries depending on the loaded modules. While the same names may be used for different compilers, keep in mind that these are completely independent scripts.
For Python users, Anvil provides two Python distributions: 1) a natively compiled Python module with a small subset of essential numerical libraries which are optimized for the AMD Milan architecture and 2) binaries distributed through Anaconda. Users are recommended to use virtual environments for installing and using additional Python packages.
A broad range of application modules from various science and engineering domains are installed on Anvil, including mathematics and statistical modeling tools, visualization software, computational fluid dynamics codes, molecular modeling packages, and debugging tools.
In addition, Singularity is supported on Anvil and Nvidia GPU Cloud containers are available on Anvil GPU nodes.
Compiling Source code
This section provides some examples of compiling source code on Anvil.
Compiling Serial Programs
A serial program is a single process which executes as a sequential stream of instructions on one processor core. Compilers capable of serial programming are available for C, C++, and versions of Fortran.
Here are a few sample serial programs:
To load a compiler, enter one of the following:
$ module load intel
$ module load gcc
$ module load aocc
Language | Intel Compiler | GNU Compiler | AOCC Compiler | |
---|---|---|---|---|
Fortran 77 |
|
|
|
|
Fortran 90 |
|
|
|
|
Fortran 95 |
|
|
|
|
C |
|
|
|
|
C++ |
|
|
|
The Intel, GNU and AOCC compilers will not output anything for a successful compilation. Also, the Intel compiler does not recognize the suffix ".f95". You may use ".f90" to stand for any Fortran code regardless of version as it is a free-formatted form.
Compiling MPI Programs
OpenMPI, Intel MPI (IMPI) and MVAPICH2 are implementations of the Message-Passing Interface (MPI) standard. Libraries for these MPI implementations and compilers for C, C++, and Fortran are available on Anvil.
Language | Header Files |
---|---|
Fortran 77 |
|
Fortran 90 |
|
Fortran 95 |
|
C |
|
C++ |
|
Here are a few sample programs using MPI:
To see the available MPI libraries:
$ module avail openmpi
$ module avail impi
$ module avail mvapich2
Language | Intel Compiler with Intel MPI (IMPI) | Intel/GNU/AOCC Compiler with OpenMPI/MVAPICH2 |
---|---|---|
Fortran 77 |
|
|
Fortran 90 |
|
|
Fortran 95 |
|
|
C |
|
|
C++ |
|
|
The Intel, GNU and AOCC compilers will not output anything for a successful compilation. Also, the Intel compiler does not recognize the suffix ".f95". You may use ".f90" to stand for any Fortran code regardless of version as it is a free-formatted form.
Here is some more documentation from other sources on the MPI libraries:
Compiling OpenMP Programs
All compilers installed on Anvil include OpenMP functionality for C, C++, and Fortran. An OpenMP program is a single process that takes advantage of a multi-core processor and its shared memory to achieve a form of parallel computing called multithreading. It distributes the work of a process over processor cores in a single compute node without the need for MPI communications.
Language | Header Files |
---|---|
Fortran 77 |
|
Fortran 90 |
|
Fortran 95 |
|
C |
|
C++ |
|
Sample programs illustrate task parallelism of OpenMP:
A sample program illustrates loop-level (data) parallelism of OpenMP:
To load a compiler, enter one of the following:
$ module load intel
$ module load gcc
$ module load aocc
Language | Intel Compiler | GNU Compiler | AOCC Compiler |
---|---|---|---|
Fortran 77 |
|
|
|
Fortran 90 |
|
|
|
Fortran 95 |
|
|
|
C |
|
|
|
C++ |
|
|
|
The Intel, GNU and AOCC compilers will not output anything for a successful compilation. Also, the Intel compiler does not recognize the suffix ".f95". You may use ".f90" to stand for any Fortran code regardless of version as it is a free-formatted form.
Here is some more documentation from other sources on OpenMP:
Compiling Hybrid Programs
A hybrid program combines both MPI and shared-memory to take advantage of compute clusters with multi-core compute nodes. Libraries for OpenMPI, Intel MPI (IMPI) and MVAPICH2 and compilers which include OpenMP for C, C++, and Fortran are available.
Language | Header Files |
---|---|
Fortran 77 |
|
Fortran 90 |
|
Fortran 95 |
|
C |
|
C++ |
|
A few examples illustrate hybrid programs with task parallelism of OpenMP:
This example illustrates a hybrid program with loop-level (data) parallelism of OpenMP:
To see the available MPI libraries:
$ module avail impi
$ module avail openmpi
$ module avail mvapich2
Language | Intel Compiler with Intel MPI (IMPI) | Intel/GNU/AOCC Compiler with OpenMPI/MVAPICH2 |
---|---|---|
Fortran 77 |
|
|
Fortran 90 |
|
|
Fortran 95 |
|
|
C |
|
|
C++ |
|
|
The Intel, GNU and AOCC compilers will not output anything for a successful compilation. Also, the Intel compiler does not recognize the suffix ".f95". You may use ".f90" to stand for any Fortran code regardless of version as it is a free-formatted form.
Compiling NVIDIA GPU Programs
The Anvil cluster contains GPU nodes that support CUDA and OpenCL. See the detailed hardware overview for the specifics on the GPUs in Anvil. This section focuses on using CUDA.
A simple CUDA program has a basic workflow:
- Initialize an array on the host (CPU).
- Copy array from host memory to GPU memory.
- Apply an operation to array on GPU.
- Copy array from GPU memory to host memory.
Here is a sample CUDA program:
Link to section '"modtree/gpu" Recommended Environment' of 'Compiling NVIDIA GPU Programs' "modtree/gpu" Recommended Environment
ModuleTree or modtree helps users to navigate between CPU stack and GPU stack and sets up a default compiler and MPI environment. For Anvil cluster, our team makes a recommendation regarding the cuda version, compiler, and MPI library. This is a proven stable cuda, compiler, and MPI library combination that is recommended if you have no specific requirements. By load the recommended set:
$ module load modtree/gpu
$ module list
# you will have all following modules
Currently Loaded Modules:
1) gcc/8.4.1 2) numactl/2.0.14 3) zlib/1.2.11 4) openmpi/4.0.6 5) cuda/11.2.2 6) modtree/gpu
Both login and GPU-enabled compute nodes have the CUDA tools and libraries available to compile CUDA programs. For complex compilations, submit an interactive job to get to the GPU-enabled compute nodes. The gpu-debug
queue is ideal for this case. To compile a CUDA program, load modtree/gpu
, and use nvcc to compile the program:
$ module load modtree/gpu
$ nvcc gpu_hello.cu -o gpu_hello
./gpu_hello
No GPU specified, using first GPUhello, world
The example illustrates only how to copy an array between a CPU and its GPU but does not perform a serious computation.
The following program times three square matrix multiplications on a CPU and on the global and shared memory of a GPU:
$ module load modtree/gpu
$ nvcc mm.cu -o mm
$ ./mm 0
speedup
-------
Elapsed time in CPU: 7810.1 milliseconds
Elapsed time in GPU (global memory): 19.8 milliseconds 393.9
Elapsed time in GPU (shared memory): 9.2 milliseconds 846.8
For best performance, the input array or matrix must be sufficiently large to overcome the overhead in copying the input and output data to and from the GPU.
For more information about NVIDIA, CUDA, and GPUs:
Provided Software
The Anvil team provides a suite of broadly useful software for users of research computing resources. This suite of software includes compilers, debuggers, visualization libraries, development environments, and other commonly used software libraries. Additionally, some widely-used application software is provided.
Link to section '"modtree/cpu" or "modtree/gpu" Recommended Environment' of 'Provided Software' "modtree/cpu" or "modtree/gpu" Recommended Environment
ModuleTree or modtree helps users to navigate between CPU stack and GPU stack and sets up a default compiler and MPI environment. For Anvil cluster, our team makes recommendations for both CPU and GPU stack regarding the CUDA version, compiler, math library, and MPI library. This is a proven stable CUDA version, compiler, math, and MPI library combinations that are recommended if you have no specific requirements. To load the recommended set:
$ module load modtree/cpu # for CPU
$ module load modtree/gpu # for GPU
Link to section 'GCC Compiler' of 'Provided Software' GCC Compiler
The GNU Compiler (GCC) is provided via the module command on Anvil clusters and will be maintained at a common version compatible across all clusters. Third-party software built with GCC will use this GCC version, rather than the GCC provided by the operating system vendor. To see available GCC compiler versions available from the module command:
$ module avail gcc
Link to section 'Toolchain' of 'Provided Software' Toolchain
The Anvil team will build and maintain an integrated, tested, and supported toolchain of compilers, MPI libraries, data format libraries, and other common libraries. This toolchain will consist of:
- Compiler suite (C, C++, Fortran) (Intel, GCC and AOCC)
- BLAS and LAPACK
- MPI libraries (OpenMPI, MVAPICH, Intel MPI)
- FFTW
- HDF5
- NetCDF
Each of these software packages will be combined with the stable "modtree/cpu" compiler, the latest available Intel compiler, and the common GCC compiler. The goal of these toolchains is to provide a range of compatible compiler and library suites that can be selected to build a wide variety of applications. At the same time, the number of compiler and library combinations is limited to keep the selection easy to navigate and understand. Generally, the toolchain built with the latest Intel compiler will be updated at major releases of the compiler.
Link to section 'Commonly Used Applications' of 'Provided Software' Commonly Used Applications
The Anvil team will go to every effort to provide a broadly useful set of popular software packages for research cluster users. Software packages such as Matlab, Python (Anaconda), NAMD, GROMACS, R, VASP, LAMMPS, and others that are useful to a wide range of cluster users are provided via the module command.
Link to section 'Changes to Provided Software' of 'Provided Software' Changes to Provided Software
Changes to available software, such as the introduction of new compilers and libraries or the retirement of older toolchains, will be scheduled in advance and coordinated with system maintenances. This is done to minimize impact and provide a predictable time for changes. Advance notice of changes will be given with regular maintenance announcements and through notices printed through “module load”s. Be sure to check maintenance announcements and job output for any upcoming changes.
Link to section 'Long Term Support' of 'Provided Software' Long Term Support
The Anvil team understands the need for a stable and unchanging suite of compilers and libraries. Research projects are often tied to specific compiler versions throughout their lifetime. The Anvil team will go to every effort to provide the "modtree/cpu" or "modtree/gpu" environment and the common GCC compiler as a long-term supported environment. These suites will stay unchanged for longer periods than the toolchain built with the latest available Intel compiler.
Installing applications
This section provides some instructions for installing and compiling some common applications on Anvil.VASP
The Vienna Ab initio Simulation Package (VASP) is a computer program for atomic scale materials modelling, e.g. electronic structure calculations and quantum-mechanical molecular dynamics, from first principles.
Link to section 'VASP License' of 'VASP' VASP License
The VASP team allows only registered users who have purchased their own license to use the software and access is only given to the VASP release which is covered by the license of the respective research group. For those who are interested to use VASP on Anvil, please send a ticket to ACCESS Help Desk to request access and provide your license for our verification. Once confirmed, the approved users will be given access to the vasp5
or vasp6
unix groups.
Please note, the license from Materials Design Inc is not accepted at this moment since we cannot verify the license information. If you hold a VASP license from Materials Design Inc, please check our user guide about build your own VASP below.
Prospective users can use the command below to check their unix groups on the system.
$ id $USER
If you are interested to purchase and get a VASP license, please visit VASP website for more information.
Link to section 'VASP 5 and VASP 6 Installations' of 'VASP' VASP 5 and VASP 6 Installations
The Anvil team provides VASP 5.4.4 and VASP 6.3.0 installations and modulefiles with our default environment compiler gcc/11.2.0
and mpi library openmpi/4.1.6
. Note that only license-approved users can load the VASP modulefile as below.
You can use the VASP 5.4.4 module by:
$ module load gcc/11.2.0 openmpi/4.1.6
$ module load vasp/5.4.4.pl2
You can use the VASP 6.3.0 module by:
$ module load gcc/11.2.0 openmpi/4.1.6
$ module load vasp/6.3.0
Once a VASP module is loaded, you can choose one of the VASP executables to run your code: vasp_std
, vasp_gam
, and vasp_ncl
.
The VASP pseudopotential files are not provided on Anvil, you may need to bring your own POTCAR files.
Here is an example of submit script for VASP simulations on Anvil.Link to section 'Build your own VASP 5 and VASP 6' of 'VASP' Build your own VASP 5 and VASP 6
If you would like to use your own VASP on Anvil, please follow the instructions for Installing VASP.6.X.X and Installing VASP.5.X.X.
In the following sections, we provide some instructions about how to install VASP 5 and VASP 6 on Anvil and also the installation scripts:
Build your own VASP 5
For VASP 5.X.X version, VASP provide several templates of makefile.include
in the /arch
folder, which contain information such as precompiler options, compiler options, and how to link libraries. You can pick up one based on your system and preferred features . Here we provide some examples about how to install the vasp.5.4.4.pl2.tgz
version on Anvil with different module environments. We also prepared two versions of VASP5 installation scripts at the end of this page.
Link to section 'Step 1: Download' of 'Build your own VASP 5' Step 1: Download
As a license holder, you can download the source code of VASP from the VASP Portal, we will not check your license in this case.
Copy the VASP resource file vasp.5.4.4.pl2.tgz
to the desired location, and unzip the file tar zxvf vasp.5.4.4.pl2.tgz
to obtain the folder /path/to/vasp-build-folder/vasp.5.4.4.pl2
and reveal its content.
Link to section 'Step 2: Prepare makefile.include' of 'Build your own VASP 5' Step 2: Prepare makefile.include
-
For GNU compilers parallelized using OpenMPI, combined with MKL
We modified the
makefile.include.linux_gnu
file to adapt the Anvil system. Download it to your VASP build folder/path/to/vasp-build-folder/vasp.5.4.4.pl2
:$ cd /path/to/vasp-build-folder/vasp.5.4.4.pl2 $ wget https://www.rcac.purdue.edu/files/knowledge/compile/src/makefile.include.linux_gnu $ cp makefile.include.linux_gnu makefile.include
If you would like to include the Wannier90 interface, you may also need to include the following lines to the end of your
makefile.include
file:# For the interface to Wannier90 (optional) LLIBS += $(WANNIER90_HOME)/libwannier.a
Load the required modules:
$ module purge $ module load gcc/11.2.0 openmpi/4.1.6 $ module load intel-mkl # If you would like to include the Wannier90 interface, also load the following module: # $ module load wannier90/3.1.0
-
For Intel compilers parallelized using IMPI, combined with MKL
Copy the
makefile.include.linux_intel
templet from the/arch
folder to your VASP build folder/path/to/vasp-build-folder/vasp.5.4.4.pl2
:$ cd /path/to/vasp-build-folder/vasp.5.4.4.pl2 $ cp arch/makefile.include.linux_intel makefile.include
For better performance, you may add the following line to the end of your
makefile.include
file (above the GPU section):FFLAGS += -march=core-avx2
If you would like to include the Wannier90 interface, you may also need to include the following lines to the end of your
makefile.include
file (above the GPU section):# For the interface to Wannier90 (optional) LLIBS += $(WANNIER90_HOME)/libwannier.a
Load the required modules:
$ module purge $ module load intel/19.0.5.281 impi/2019.5.281 $ module load intel-mkl # If you would like to include the Wannier90 interface, also load this module: # $ module load wannier90/3.1.0
Link to section 'Step 3: Make' of 'Build your own VASP 5' Step 3: Make
Build VASP with command make all
to install all three executables vasp_std
, vasp_gam
, and vasp_ncl
or use make std
to install only the vasp_std
executable. Use make veryclean
to remove the build folder if you would like to start over the installation process.
Link to section 'Step 4: Test' of 'Build your own VASP 5' Step 4: Test
You can open an Interactive session to test the installed VASP, you may bring your own VASP test files:
$ cd /path/to/vasp-test-folder/
$ module purge
$ module load gcc/11.2.0 openmpi/4.1.6 intel-mkl
# If you included the Wannier90 interface, also load this module:
# $ module load wannier90/3.1.0
$ mpirun /path/to/vasp-build-folder/vasp.5.4.4.pl2/bin/vasp_std
Link to section ' ' of 'Build your own VASP 5'
Build your own VASP 6
For VASP 6.X.X version, VASP provide several templates of makefile.include
, which contain information such as precompiler options, compiler options, and how to link libraries. You can pick up one based on your system and preferred features . Here we provide some examples about how to install vasp 6.3.0
on Anvil with different module environments. We also prepared two versions of VASP6 installation scripts at the end of this page.
Link to section 'Step 1: Download' of 'Build your own VASP 6' Step 1: Download
As a license holder, you can download the source code of VASP from the VASP Portal, we will not check your license in this case.
Copy the VASP resource file vasp.6.3.0.tgz
to the desired location, and unzip the file tar zxvf vasp.6.3.0.tgz
to obtain the folder /path/to/vasp-build-folder/vasp.6.3.0
and reveal its content.
Link to section 'Step 2: Prepare makefile.include' of 'Build your own VASP 6' Step 2: Prepare makefile.include
-
For GNU compilers parallelized using OpenMPI + OpenMP, combined with MKL
We modified the
makefile.include.gnu_ompi_mkl_omp
file to adapt the Anvil system. Download it to your VASP build folder/path/to/vasp-build-folder/vasp.6.3.0
:$ cd /path/to/vasp-build-folder/vasp.6.3.0 $ wget https://www.rcac.purdue.edu/files/knowledge/compile/src/makefile.include.gnu_ompi_mkl_omp $ cp makefile.include.gnu_ompi_mkl_omp makefile.include
If you would like to include the Wannier90 interface, you may also need to include the following lines to the end of your
makefile.include
file:# For the VASP-2-Wannier90 interface (optional) CPP_OPTIONS += -DVASP2WANNIER90 WANNIER90_ROOT ?=$(WANNIER90_HOME) LLIBS += -L$(WANNIER90_ROOT) -lwannier
Then, load the required modules:
$ module purge $ module load gcc/11.2.0 openmpi/4.1.6 $ module load intel-mkl hdf5 # If you would like to include the Wannier90 interface, also load the following module: # $ module load wannier90/3.1.0
-
For Intel compilers parallelized using IMPI + OpenMP, combined with MKL
We modified the
makefile.include.intel_omp
file to adapt the Anvil system. Download it to your VASP build folder/path/to/vasp-build-folder/vasp.6.3.0
:$ cd /path/to/vasp-build-folder/vasp.6.3.0 $ wget https://www.rcac.purdue.edu/files/knowledge/compile/src/makefile.include.intel_omp $ cp makefile.include.intel_omp makefile.include
If you would like to include the Wannier90 interface, you may also need to include the following lines to the end of your
makefile.include
file:# For the VASP-2-Wannier90 interface (optional) CPP_OPTIONS += -DVASP2WANNIER90 WANNIER90_ROOT ?=$(WANNIER90_HOME) LLIBS += -L$(WANNIER90_ROOT) -lwannier
Then, load the required modules:
$ module purge $ module load intel/19.0.5.281 impi/2019.5.281 $ module load intel-mkl hdf5 # If you would like to include the Wannier90 interface, also load the following module: # $ module load wannier90/3.1.0
Link to section 'Step 3: Make' of 'Build your own VASP 6' Step 3: Make
Open makefile
, make sure the first line is VERSIONS = std gam ncl
.
Build VASP with command make all
to install all three executables vasp_std
, vasp_gam
, and vasp_ncl
or use make std
to install only the vasp_std
executable. Use make veryclean
to remove the build folder if you would like to start over the installation process.
Link to section 'Step 4: Test' of 'Build your own VASP 6' Step 4: Test
You can open an Interactive session to test the installed VASP 6. Here is an example of testing above installed VASP 6.3.0 with GNU compilers and OpenMPI:
$ cd /path/to/vasp-build-folder/vasp.6.3.0/testsuite
$ module purge
$ module load gcc/11.2.0 openmpi/4.1.6 intel-mkl hdf5
# If you included the Wannier90 interface, also load the following module:
# $ module load wannier90/3.1.0
$ ./runtest
Link to section ' ' of 'Build your own VASP 6'
LAMMPS
Large-scale Atomic/Molecular Massively Parallel Simulator (LAMMPS) is a molecular dynamics program from Sandia National Laboratories. LAMMPS makes use of Message Passing Interface for parallel communication and is a free and open-source software, distributed under the terms of the GNU General Public License.
Provided LAMMPS module
Link to section 'LAMMPS modules' of 'Provided LAMMPS module' LAMMPS modules
The Anvil team provides LAMMPS module with our default module environment gcc/11.2.0
and openmpi/4.0.6
to all users. It can be accessed by:
$ module load gcc/11.2.0 openmpi/4.0.6
$ module load lammps/20210310
The LAMMPS executable is lmp
and the LAMMPS potential files are installed at $LAMMPS_HOME/share/lammps/potentials
, where the value of $LAMMPS_HOME
is the path to LAMMPS build folder. Use this variable in any scripts. Your actual LAMMPS folder path may change without warning, but this variable will remain current. The current path is:
$ echo $LAMMPS_HOME
$ /apps/spack/anvil/apps/lammps/20210310-gcc-11.2.0-jzfe7x3
LAMMPS Job Submit Script
This is an example of a job submission file for running parallel LAMMPS jobs using the LAMMPS module installed on Anvil.
#!/bin/bash
# FILENAME: myjobsubmissionfile
#SBATCH -A myallocation # Allocation name
#SBATCH --nodes=1 # Total # of nodes
#SBATCH --ntasks=128 # Total # of MPI tasks
#SBATCH --time=1:30:00 # Total run time limit (hh:mm:ss)
#SBATCH -J lammps_tt # Job name
#SBATCH -o myjob.o%j # Name of stdout output file
#SBATCH -e myjob.e%j # Name of stderr error file
#SBATCH -p wholenode # Queue (partition) name
# Manage processing environment, load compilers and applications.
module --force purge
module load gcc/11.2.0 openmpi/4.0.6
module load lammps/20210310
module list
# Launch MPI code
srun -n $SLURM_NTASKS lmp
Build your own LAMMPS
Link to section 'Build your own LAMMPS' of 'Build your own LAMMPS' Build your own LAMMPS
LAMMPS provides a very detailed instruction of Build LAMMPS with a lot of customization options. In the following sections, we provide basic installation instructions of how to install LAMMPS on Anvil, as well as a LAMMPS Installation Script for users who would like to build their own LAMMPS on Anvil:
Link to section 'Step 1: Download' of 'Build your own LAMMPS' Step 1: Download
LAMMPS is an open-source code, you can download LAMMPS as a tarball from LAMMPS download page. There are several versions available on the LAMMPS webpage, we strongly recommend downloading the latest released stable
version and unzip and untar it. It will create a LAMMPS directory:
$ wget https://download.lammps.org/tars/lammps-stable.tar.gz
$ tar -xzvf lammps-stable.tar.gz
$ ls
lammps-23Jun2022 lammps-stable.tar.gz
Link to section 'Step 2: Build source code' of 'Build your own LAMMPS' Step 2: Build source code
LAMMPS provides two ways to build the source code: traditional configure && make
method and the cmake
method. These are two independent approaches and users should not mix them together. You can choose the one you are more familiar with.
Build LAMMPS with Make
Traditional make
method requires a Makefile
file appropriate for your system in either the src/MAKE
, src/MAKE/MACHINES
, src/MAKE/OPTIONS
, or src/MAKE/MINE directory
. It provides various options to customize your LAMMPS. If you would like to build your own LAMMPS on Anvil with make
, please follow the instructions for Build LAMMPS with make. In the following sections, we will provide some instructions on how to install LAMMPS on Anvil with make
.
Link to section 'Include LAMMPS Packages' of 'Build LAMMPS with Make' Include LAMMPS Packages
In LAMMPS, a package is a group of files that enable a specific set of features. For example, force fields for molecular systems or rigid-body constraints are in packages. Usually, you can include only the packages you plan to use, but it doesn't hurt to run LAMMPS with additional packages.
To use make
command to see the make options and package status, you need to first jump to src
subdirectory. Here we will continue use lammps-23Jun2022
as an example:
$ cd lammps-23Jun2022/src # change to main LAMMPS source folder
$ make # see a variety of make options
$ make ps # check which packages are currently installed
For most LAMMPS packages, you can include them by:
$ make yes-PGK_NAME # install a package with its name, default value is "no", which means exclude the package
# For example:
$ make yes-MOLECULE
A few packages require additional steps to include libraries or set variables, as explained on Packages with extra build options. If a package requires external libraries, you must configure and build those libraries before building LAMMPS and especially before enabling such a package.
If you have issues with installing external libraries, please contact us at Help Desk.
Instead of specifying all the package options via the command line, LAMMPS provides some Make shortcuts for installing many packages, such as make yes-most
, which will install most LAMMPS packages w/o libs. You can pick up one of the shortcuts based on your needs.
Link to section 'Compilation' of 'Build LAMMPS with Make' Compilation
Once the desired packages are included, you can compile lammps with our default environment: compiler gcc/11.2.0
and MPI library openmpi/4.0.6
, you can load them all at once by module load modtree/cpu
. Then corresponding make
option will be make g++_openmpi
for OpenMPI with compiler set to GNU g++.
Then the LAMMPS executable lmp_g++_openmpi
will be generated in the build
folder.
LAMMPS support parallel compiling, so you may submit an Interactive job to do parallel compiling.
If you get some error messages and would like to start over the installation process, you can delete compiled objects, libraries and executables with make clean-all
.
Link to section 'Examples' of 'Build LAMMPS with Make' Examples
Here is an example of how to install the lammps-23Jun2022
version on Anvil with most packages enabled:
# Setup module environments $ module purge $ module load modtree/cpu
$ module load hdf5 fftw gsl netlib-lapack
$ module list $ cd lammps-23Jun2022/src # change to main LAMMPS source folder $ make yes-most # install most LAMMPS packages w/o libs $ make ps # check which packages are currently installed # compilation $ make g++_openmpi # or "make -j 12 g++_openmpi" to do parallel compiling if you open an interactive session with 12 cores.
Link to section 'Tips' of 'Build LAMMPS with Make' Tips
When you run LAMMPS and get an error like "command or style is unknown", it is likely due to the fact you did not include the required packages for that command or style. If the command or style is available in a package included in the LAMMPS distribution, the error message will indicate which package would be needed.
For more information about LAMMPS build options, please refer to these sections of LAMMPS documentation:
Build LAMMPS with Cmake
CMake is an alternative to compiling LAMMPS in addition to the traditional Make method. CMake has several advantages, and might be helpful for people with limited experience in compiling software or for those who want to modify or extend LAMMPS. If you prefer using cmake
, please follow the instructions for Build LAMMPS with CMake. In the following sections, we will provide some instructions on how to install LAMMPS on Anvil with cmake
and the LAMMPS Installation Script:
Link to section 'Use CMake to generate a build environment' of 'Build LAMMPS with Cmake' Use CMake to generate a build environment
-
First go to your LAMMPS directory and generate a new folder
build
for build environment. Here we will continue uselammps-23Jun2022
as an example:$ cd lammps-23Jun2022 $ mkdir build; cd build # create and change to a build directory
-
To use
cmake
features, you need tomodule load cmake
first. -
For basic LAMMPS installation with no add-on packages enabled and no customization, you can generate a build environment by:
$ cmake ../cmake # configuration reading CMake scripts from ../cmake
-
You can also choose to include or exclude packages to or from build.
In LAMMPS, a package is a group of files that enable a specific set of features. For example, force fields for molecular systems or rigid-body constraints are in packages. Usually, you can include only the packages you plan to use, but it doesn't hurt to run LAMMPS with additional packages.
For most LAMMPS packages, you can include it by adding the following flag to
cmake
command:-D PKG_NAME=yes # degualt value is "no", which means exclude the package
For example:
$ cmake -D PKG_MOLECULE=yes -D PKG_RIGID=yes -D PKG_MISC=yes ../cmake
A few packages require additional steps to include libraries or set variables, as explained on Packages with extra build options. If you have issue with installing external libraries, please contact us at Help Desk.
-
Instead of specifying all the package options via the command line, LAMMPS provides some CMake setting scripts in
/cmake/presets
folder. You can pick up one of them or customize it based on your needs. -
If you get some error messages after the
cmake ../cmake
step and would like to start over, you can delete the wholebuild
folder and create new one:$ cd lammps-23Jun2022 $ rm -rf build $ mkdir build && cd build
Link to section 'Compilation' of 'Build LAMMPS with Cmake' Compilation
-
Once the build files are generated by
cmake
command, you can compile lammps with our default environments: compilergcc/11.2.0
and MPI libraryopenmpi/4.0.6
, you can load them all at once bymodule load modtree/cpu
. -
Then, the next step is to compile LAMMPS with
make
orcmake --build
, upon completion, the LAMMPS executablelmp
will be generated in thebuild
folder. -
LAMMPS supports parallel compiling, so you may submit an Interactive job to do parallel compilation.
-
If you get some error with compiling, you can delete compiled objects, libraries and executables with
make clean
orcmake --build . --target clean
.
Link to section 'Examples' of 'Build LAMMPS with Cmake' Examples
Here is an example of how to install the lammps-23Jun2022
version on Anvil with most packages enabled:
# Setup module environments
$ module purge
$ module load modtree/cpu
$ module load hdf5 fftw gsl netlib-lapack
$ module load cmake anaconda
$ module list
$ cd lammps-23Jun2022 # change to the LAMMPS distribution directory
$ mkdir build; cd build; # create and change to a build directory
# enable most packages and setup Python package library path
$ cmake -C ../cmake/presets/most.cmake -D PYTHON_EXECUTABLE=$CONDA_PYTHON_EXE ../cmake
# If everything works well, you will see
# -- Build files have been written to: /path-to-lammps/lammps-23Jun2022/build
# compilation
$ make # or "make -j 12" to do parallel compiling if you open an interactive session with 12 cores.
# If everything works well, you will see
# [100%] Built target lmp
The CMake setting script /cmake/presets/most.cmake
we used in the example here will includes 57 most common packages:
$ ASPHERE BOCS BODY BROWNIAN CG-DNA CG-SDK CLASS2 COLLOID COLVARS COMPRESS CORESHELL DIELECTRIC DIFFRACTION DIPOLE DPD-BASIC DPD-MESO DPD-REACT DPD-SMOOTH DRUDE EFF EXTRA-COMPUTE EXTRA-DUMP EXTRA-FIX EXTRA-MOLECULE EXTRA-PAIR FEP GRANULAR INTERLAYER KSPACE MACHDYN MANYBODY MC MEAM MISC ML-IAP ML-SNAP MOFFF MOLECULE OPENMP OPT ORIENT PERI PLUGIN POEMS QEQ REACTION REAXFF REPLICA RIGID SHOCK SPH SPIN SRD TALLY UEF VORONOI YAFF
Link to section 'Tips' of 'Build LAMMPS with Cmake' Tips
When you run LAMMPS and get an error like "command or style is unknown", it is likely due to you did not include the required packages for that command or style. If the command or style is available in a package included in the LAMMPS distribution, the error message will indicate which package would be needed.
After the initial build, whenever you edit LAMMPS source files, enable or disable packages, change compiler flags or build options, you must recompile LAMMPS with make
.
For more information about LAMMPS build options, please following these links from LAMMPS website:
LAMMPS Installation Script
Here we provide a lammps-23Jun2022
installation script with cmake
. It contains the procedures from downloading the source code to what we mentioned in Build LAMMPS with Cmake Example
section. You will start with making an empty folder. Then, download the installation scriptinstall-lammps.sh
to this folder. Since parallel compiling with 12 cores is used in the script, you may submit an Interactive job to ask for 12 cores:
$ mkdir lammps; cd lammps; # create and change to a lammps directory
$ wget https://www.rcac.purdue.edu/files/knowledge/compile/src/install-lammps.sh
$ ls
install-lammps.sh
$ sinteractive -N 1 -n 12 -A oneofyourallocations -p shared -t 1:00:00
$ bash install-lammps.sh
Policies, Helpful Tips and FAQs
Here are details on some policies for research users and systems.
Software Installation Request Policy
The Anvil team will go to every reasonable effort to provide a broadly useful set of popular software packages for research cluster users. However, many domain-specific packages that may only be of use to single users or small groups of users are beyond the capacity of staff to fully maintain and support. Please consider the following if you require software that is not available via the module command:
- If your lab is the only user of a software package, Anvil staff may recommend that you install your software privately, either in your home directory or in your allocation project space. If you need help installing software, the Anvil support team may be able to provide limited help.
- As more users request a particular piece of software, Anvil may decide to provide the software centrally. Matlab, Python (Anaconda), NAMD, GROMACS, and R are all examples of frequently requested and used centrally-installed software.
- Python modules that are available through the Anaconda distribution will be installed through it. Anvil staff may recommend you install other Python modules privately.
If you're not sure how your software request should be handled or need help installing software please contact us at Help Desk.
Helpful Tips
We will strive to ensure that Anvil serves as a valuable resource to the national research community. We hope that you the user will assist us by making note of the following:
- You share Anvil with thousands of other users, and what you do on the system affects others. Exercise good citizenship to ensure that your activity does not adversely impact the system and the research community with whom you share it. For instance: do not run jobs on the login nodes and do not stress the filesystem.
- Help us serve you better by filing informative help desk tickets. Before submitting a help desk ticket do check what the user guide and other documentation say. Search the internet for key phrases in your error logs; that's probably what the consultants answering your ticket are going to do. What have you changed since the last time your job succeeded?
- Describe your issue as precisely and completely as you can: what you did, what happened, verbatim error messages, other meaningful output. When appropriate, include the information a consultant would need to find your artifacts and understand your workflow: e.g. the directory containing your build and/or job script; the modules you were using; relevant job numbers; and recent changes in your workflow that could affect or explain the behavior you're observing.
- Have realistic expectations. Consultants can address system issues and answer questions about Anvil. But they can't teach parallel programming in a ticket and may know nothing about the package you downloaded. They may offer general advice that will help you build, debug, optimize, or modify your code, but you shouldn't expect them to do these things for you.
- Be patient. It may take a business day for a consultant to get back to you, especially if your issue is complex. It might take an exchange or two before you and the consultant are on the same page. If the admins disable your account, it's not punitive. When the file system is in danger of crashing, or a login node hangs, they don't have time to notify you before taking action.
For GPU jobs, make sure to use --gpus-per-node command, otherwise, your job may not run properly.
Link to section 'Helpful Tools' of 'Helpful Tips' Helpful Tools
The Anvil cluster provides a list of useful auxiliary tools:
Tool | Use | |
---|---|---|
myquota |
Check the quota of different file systems. | |
flost |
A utility to recover files from snapshots. | |
showpartitions |
Display all Slurm partitions and their current usage. | |
myscratch |
Show the path to your scratch directory. | |
jobinfo |
Collates job information from the sstat , sacct and squeue SLURM commands to give a uniform interface for both current and historical jobs. |
|
sfeatures |
Show the list of available constraint feature names for different node types. | |
myproject |
Print the location of my project directory. | |
mybalance |
Check the allocation usage of your project team. | |
wait_time |
Show the predicted start time of a given job. To use, enter wait_time [-j | –job_id] job_id for the desired job. |
Frequently Asked Questions
Some common questions, errors, and problems are categorized below. Click the Expand Topics link in the upper right to see all entries at once. You can also use the search box above to search the user guide for any issues you are seeing.
About Anvil
Frequently asked questions about Anvil.
Can you remove me from the Anvil mailing list?
Your subscription in the Anvil mailing list is tied to your account on Anvil which was granted to you through an ACCESS allocation. If you are no longer using your account on Anvil, you can contact your PI or allocation manager to remove you from their Anvil allocation.
How is Anvil different than Purdue Community Clusters?
Anvil is part of the national Advanced Cyberinfrastructure Coordination Ecosystem: Services & Support (ACCESS) ecosystem and is not part of Purdue Community Clusters program. There are a lot of similarities between the systems, yet there are also a few differences in hardware, software and overall governance. For Purdue users accustomed to the way Purdue supercomputing clusters operate, the following summarizes key differences between RCAC clusters and Anvil.
Link to section 'Support' of 'How is Anvil different than Purdue Community Clusters?' Support
-
While Anvil is operated by Purdue RCAC, it is an ACCESS resource, and all support requests have to go through ACCESS channels rather than RCAC ones. Please direct your Anvil questions to the ACCESS Help Desk (https://support.access-ci.org/open-a-ticket) and they will be dispatched to us.
Link to section 'Resource Allocations' of 'How is Anvil different than Purdue Community Clusters?' Resource Allocations
Two key things to remember on Anvil and other ACCESS resources:
- In contrast with Community Clusters, you do not buy nodes on Anvil. To access Anvil, PIs must request an allocation through ACCESS.
- Users don't get access to a dedicated “owner” queue with X-number of cores. Instead, they get an allocation for Y-number of core-hours. Jobs can be submitted to any of the predefined partitions.
More details on these differences are presented below.
-
Access to Anvil is free (no need to purchase nodes), and is governed by ACCESS allocation policies. All allocation requests must be submitted via ACCESS Resource Allocation System. These allocations other than the Maximize ACCESS Request can be requested at any time.
Explore ACCESS allocations are intended for purposes that require small resource amounts. Researchers can try out resources or run benchmarks, instructors can provide access for small-scale classroom activities, research software engineers can develop or port codes, and so on. Graduate students can conduct thesis or dissertation work.
Discover ACCESS allocations are intended to fill the needs of many small-scale research activities or other resource needs. The goal of this opportunity is to allow many researchers, Campus Champions, and Gateways to request allocations with a minimum amount of effort so they can complete their work.
Accelerate ACCESS allocations support activities that require more substantial, mid-scale resource amounts to pursue their research objectives. These include activities such as consolidating multi-grant programs, collaborative projects, preparing for Maximize ACCESS requests, and supporting gateways with growing communities.
Maximize ACCESS allocations are for projects with resource needs beyond those provided by an Accelerate ACCESS project, a Maximize ACCESS request is required. ACCESS does not place an upper limit on the size of allocations that can be requested or awarded at this level, but resource providers may have limits on allocation amounts for specific resources.
-
Unlike the Community Clusters model (where you “own” a certain amount of nodes and can run on them for the lifetime of the cluster), under ACCESS model, you apply for resource allocations on one or more ACCESS systems, and your project is granted certain amounts of Service Units (SUs) on each system. Different ACCESS centers compute SUs differently, but in general SUs are always some measure of CPU-hours or similar resource usage by your jobs. Anvil job accounting page provides more details on how we compute SU consumption on Anvil. Once granted, you can use your allocation’s SUs until they are consumed or expired, after which the allocation must be renewed via established ACCESS process (note: no automatic refills, but there are options to extend the time to use up your SUs and request additional SUs as supplements). You can check your allocation balances on ACCESS website, or use a local
mybalance
command in Anvil terminal window.
Link to section 'Accounts and Passwords' of 'How is Anvil different than Purdue Community Clusters?' Accounts and Passwords
-
Your Anvil account is not the same as your Purdue Career Account. Following ACCESS procedures, you will need to create an ACCESS account (it is these ACCESS user names that your PI or project manager adds to their allocation to grant you access to Anvil). Your Anvil user name will be automatically derived from ACCESS account name, and it will look something similar to
x-ACCESSname
, starting with anx-
. -
Anvil does not support password authentication, and there is no “Anvil password”. The recommended authentication method for SSH is public key-based authentication (“SSH keys”). Please see the user guide for detailed descriptions and steps to configure and use your SSH keys.
Link to section 'Storage and Filesystems' of 'How is Anvil different than Purdue Community Clusters?' Storage and Filesystems
-
Anvil scratch purging policies (see the filesystems section) are significantly more stringent than on Purdue RCAC systems. Files not accessed for 30 days are deleted instantly and automatically (on the filesystem's internal policy engine level). Note: there are no warning emails!
-
Purdue Data Depot is not available on Anvil, but every allocation receives a dedicated project space (
$PROJECT
) shared among allocation members in a way very similar to Data Depot. See the filesystems section in the user guide for more details. You can transfer files between Anvil and Data Depot or Purdue clusters using any of the mutually supported methods (e.g. SCP, SFTP, rsync, Globus). -
Purdue Fortress is available on Anvil, but direct HSI and HTAR are currently not supported. You can transfer files between Anvil and Fortress using any of the mutually supported methods (e.g. SFTP, Globus).
-
Anvil features Globus Connect Server v5 which enables direct HTTPS access to data on Anvil Globus collections right from your browser (both uploads and downloads).
Link to section 'Partitions and Node Types' of 'How is Anvil different than Purdue Community Clusters?' Partitions and Node Types
-
Anvil consists of several types of compute nodes (regular, large memory, GPU-equipped, etc), arranged into multiple partitions according to various hardware properties and scheduling policies. You are free to direct your jobs and use your SUs in any partition that suits your jobs’ specific computational needs and matches your allocation type (CPU vs. GPU). Note that different partitions may “burn” your SUs at a different rate - see Anvil job accounting page for detailed description.
Corollary: On Anvil, you need to specify both allocation account and partition for your jobs (
-A allocation
and-p partition
options), otherwise your job will end up in the defaultshared
partition, which may or may not be optimal. See partitions page for details. -
There are no
standby
,partner
orowner
-type queues on Anvil. All jobs in all partitions are prioritized equally.
Link to section 'Software Stack' of 'How is Anvil different than Purdue Community Clusters?' Software Stack
-
Two completely separate software stacks and corresponding Lmod module files are provided for CPU- and GPU-based applications. Use
module load modtree/cpu
andmodule load modtree/gpu
to switch between them. The CPU stack is loaded by default when you login to the system. See example jobs section for specific instructions and submission scripts templates.
Link to section 'Composable Subsystem' of 'How is Anvil different than Purdue Community Clusters?' Composable Subsystem
-
A composable subsystem alongside of the main HPC cluster is a uniquely empowering feature of Anvil. Composable subsystem is a Kubernetes-based private cloud that enables researchers to define and stand up custom services, such as notebooks, databases, elastic software stacks, and science gateways.
Link to section 'Everything Else' of 'How is Anvil different than Purdue Community Clusters?' Everything Else
-
The above list provides highlights of major differences an RCAC user would find on Anvil, but it is by no means exhaustive. Please refer to Anvil user guide for detailed descriptions, or reach out to us through ACCESS Help Desk (https://support.access-ci.org/open-a-ticket)
Logging In & Accounts
Frequently asked questions related to Logging In & Accounts.
Questions
Common login-related questions.
Can I use browser-based Thinlinc to access Anvil?
Link to section 'Problem' of 'Can I use browser-based Thinlinc to access Anvil?' Problem
You would like to use browser-based Thinlinc to access Anvil, but do not know what username and password to use.
Link to section 'Solution' of 'Can I use browser-based Thinlinc to access Anvil?' Solution
Password based access is not supported at this moment. Please use Thinlinc Client instead.
For your first time login to Anvil, you will have to login to Open OnDemand with your ACCESS username and password to start an anvil terminal and then set up SSH keys. Then you are able to use your native Thinlic client to access Anvil with SSH keys.
What is my username and password to access Anvil?
Link to section 'Problem' of 'What is my username and password to access Anvil?' Problem
You would like to login to Anvil, but do not know what username and password to use.
Link to section 'Solution' of 'What is my username and password to access Anvil?' Solution
Currently, you can access Anvil through:
-
SSH client:
You can login with standard SSH connections with SSH keys-based authentication to
anvil.rcac.purdue.edu
using your Anvil username. -
Native Thinlinc Client:
You can access native Thinlic client with SSH keys.
-
Open OnDemand:
You can access Open OnDemand with your ACCESS username and password.
What if my ThinLinc screen is locked?
Link to section 'Problem' of 'What if my ThinLinc screen is locked?' Problem
Your ThinLinc desktop is locked after being idle for a while, and it asks for a password to refresh it, but you do not know the password.
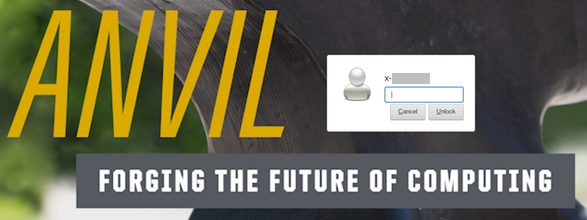
Link to section 'Solution' of 'What if my ThinLinc screen is locked?' Solution
If your screen is locked, close the ThinLinc client, reopen the client login popup, and select End existing session
.
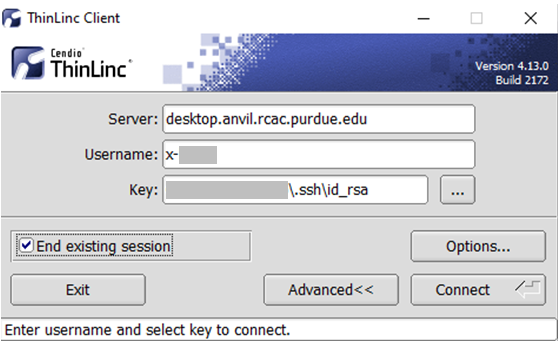
To permanently avoid screen lock issue, right click desktop and select Applications
, then settings
, and select Screensaver
.
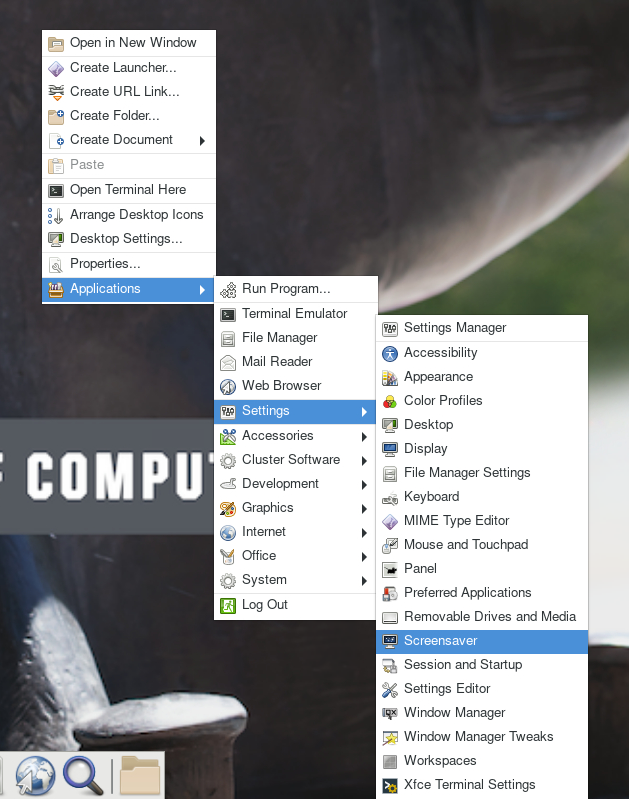
Under Screensaver, turn off the Enable Screensaver
, then under Lock Screen, turn off the Enable Lock Screen
, and close the window.
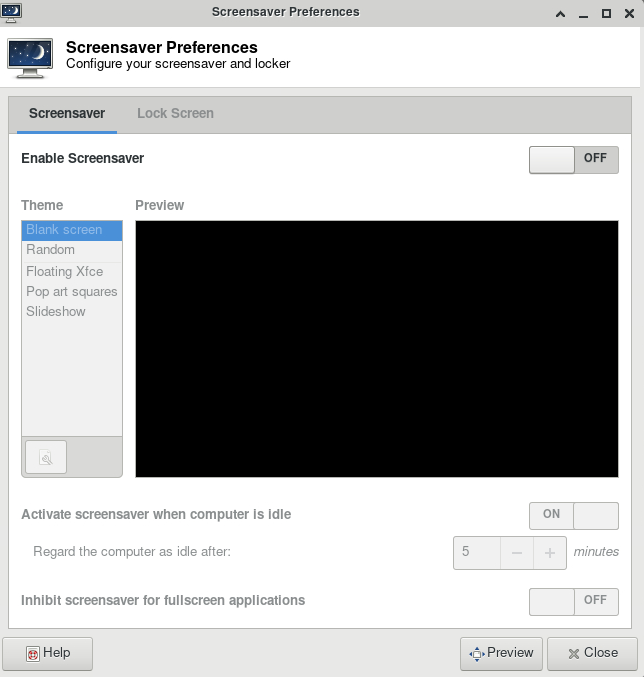
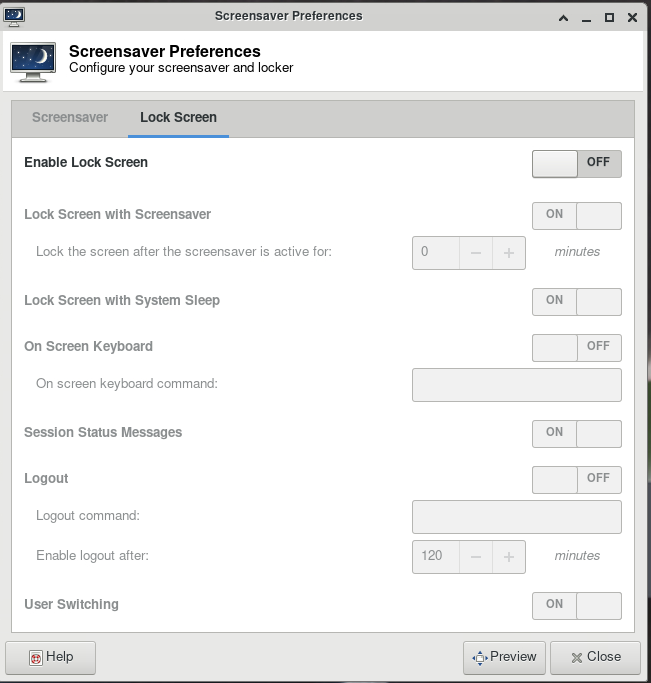
Jobs
Frequently asked questions related to running jobs.
Errors
Common errors and potential solutions/workarounds for them.
Close Firefox / Firefox is already running but not responding
Link to section 'Problem' of 'Close Firefox / Firefox is already running but not responding' Problem
You receive the following message after trying to launch Firefox browser inside your graphics desktop:
Close Firefox
Firefox is already running, but not responding. To open a new window,
you must first close the existing Firefox process, or restart your system.
Link to section 'Solution' of 'Close Firefox / Firefox is already running but not responding' Solution
When Firefox runs, it creates several lock files in the Firefox profile directory (inside ~/.mozilla/firefox/
folder in your home directory). If a newly-started Firefox instance detects the presence of these lock files, it complains.
This error can happen due to multiple reasons:
- Reason: You had a single Firefox process running, but it terminated abruptly without a chance to clean its lock files (e.g. the job got terminated, session ended, node crashed or rebooted, etc).
- Solution: If you are certain you do not have any other Firefox processes running elsewhere, please use the following command in a terminal window to detect and remove the lock files:
$ unlock-firefox
- Solution: If you are certain you do not have any other Firefox processes running elsewhere, please use the following command in a terminal window to detect and remove the lock files:
- Reason: You may indeed have another Firefox process (in another Thinlinc or Gateway session on this or other cluster, another front-end or compute node). With many clusters sharing common home directory, a running Firefox instance on one can affect another.
- Solution: Try finding and closing running Firefox process(es) on other nodes and clusters.
- Solution: If you must have multiple Firefoxes running simultaneously, you may be able to create separate Firefox profiles and select which one to use for each instance.
Jupyter: database is locked / can not load notebook format
Link to section 'Problem' of 'Jupyter: database is locked / can not load notebook format' Problem
You receive the following message after trying to load existing Jupyter notebooks inside your JupyterHub session:
Error loading notebook
An unknown error occurred while loading this notebook. This version can load notebook formats or earlier. See the server log for details.
Alternatively, the notebook may open but present an error when creating or saving a notebook:
Autosave Failed!
Unexpected error while saving file: MyNotebookName.ipynb database is locked
Link to section 'Solution' of 'Jupyter: database is locked / can not load notebook format' Solution
When Jupyter notebooks are opened, the server keeps track of their state in an internal database (located inside ~/.local/share/jupyter/
folder in your home directory). If a Jupyter process gets terminated abruptly (e.g. due to an out-of-memory error or a host reboot), the database lock is not cleared properly, and future instances of Jupyter detect the lock and complain.
Please follow these steps to resolve:
- Fully exit from your existing Jupyter session (close all notebooks, terminate Jupyter, log out from JupyterHub or JupyterLab, terminate OnDemand gateway's Jupyter app, etc).
- In a terminal window (SSH, Thinlinc or OnDemand gateway's terminal app) use the following command to clean up stale database locks:
$ unlock-jupyter
- Start a new Jupyter session as usual.
Anvil Composable Subsystem
New usage patterns have emerged in research computing that depend on the availability of custom services such as notebooks, databases, elastic software stacks, and science gateways alongside traditional batch HPC. The Anvil Composable Subsystem is a Kubernetes based private cloud managed with Rancher that provides a platform for creating composable infrastructure on demand. This cloud-style flexibility provides researchers the ability to self-deploy and manage persistent services to complement HPC workflows and run container-based data analysis tools and applications.
Concepts
Link to section 'Containers & Images' of 'Concepts' Containers & Images
Image - An image is a simple text file that defines the source code of an application you want to run as well as the libraries, dependencies, and tools required for the successful execution of the application. Images are immutable meaning they do not hold state or application data. Images represent a software environment at a specific point of time and provide an easy way to share applications across various environments. Images can be built from scratch or downloaded from various repositories on the internet, additionally many software vendors are now providing containers alongside traditional installation packages like Windows .exe and Linux rpm/deb.
Container - A container is the run-time environment constructed from an image when it is executed or run in a container runtime. Containers allow the user to attach various resources such as network and volumes in order to move and store data. Containers are similar to virtual machines in that they can be attached to when a process is running and have arbitrary commands executed that affect the running instance. However, unlike virtual machines, containers are more lightweight and portable allowing for easy sharing and collaboration as they run identically in all environments.
Tags - Tags are a way of organizing similar image files together for ease of use. You might see several versions of an image represented using various tags. For example, we might be building a new container to serve web pages using our favorite web server: nginx. If we search for the nginx container on Docker Hub image repository we see many options or tags are available for the official nginx container.
The most common you will see are typically :latest
and :number
where
number refers to the most recent few versions of the software releases. In
this example we can see several tags refer to the same image:
1.21.1, mainline, 1, 1.21,
and latest
all reference the same image while the
1.20.1, stable, 1.20
tags all reference a common but different image.
In this case we likely want the nginx image with either the latest
or 1.21.1
tag represented as nginx:latest
and nginx:1.21.1
respectively.
Container Security - Containers enable fast developer velocity and ease compatibility through great portability, but the speed and ease of use come at some costs. In particular it is important that folks utilizing container driver development practices have a well established plan on how to approach container and environment security. Best Practices
Container Registries - Container registries act as large repositories of images, containers, tools and surrounding software to enable easy use of pre-made containers software bundles. Container registries can be public or private and several can be used together for projects. Docker Hub is one of the largest public repositories available, and you will find many official software images present on it. You need a user account to avoid being rate limited by Docker Hub. A private container registry based on Harbor that is available to use. TODO: link to harbor instructions
Docker Hub - Docker Hub is one of the largest container image registries that exists and is well known and widely used in the container community, it serves as an official location of many popular software container images. Container image repositories serve as a way to facilitate sharing of pre-made container images that are “ready for use.” Be careful to always pay attention to who is publishing particular images and verify that you are utilizing containers built only from reliable sources.
Harbor - Harbor is an open source registry for Kubernetes artifacts, it provides private image storage and enforces container security by vulnerability scanning as well as providing RBAC or role based access control to assist with user permissions. Harbor is a registry similar to Docker Hub, however it gives users the ability to create private repositories. You can use this to store your private images as well as keeping copies of common resources like base OS images from Docker Hub and ensure your containers are reasonably secure from common known vulnerabilities.
Link to section 'Container Runtime Concepts' of 'Concepts' Container Runtime Concepts
Docker Desktop - Docker Desktop is an application for your Mac / Windows machine that will allow you to build and run containers on your local computer. Docker desktop serves as a container environment and enables much of the functionality of containers on whatever machine you are currently using. This allows for great flexibility, you can develop and test containers directly on your laptop and deploy them directly with little to no modifications.
Volumes - Volumes provide us with a method to create persistent data that is generated and consumed by one or more containers. For docker this might be a folder on your laptop while on a large Kubernetes cluster this might be many SSD drives and spinning disk trays. Any data that is collected and manipulated by a container that we want to keep between container restarts needs to be written to a volume in order to remain around and be available for later use.
Link to section 'Container Orchestration Concepts' of 'Concepts' Container Orchestration Concepts
Container Orchestration - Container orchestration broadly means the automation of much of the lifecycle management procedures surrounding the usage of containers. Specifically it refers to the software being used to manage those procedures. As containers have seen mass adoption and development in the last decade, they are now being used to power massive environments and several options have emerged to manage the lifecycle of containers. One of the industry leading options is Kubernetes, a software project that has descended from a container orchestrator at Google that was open sourced in 2015.
Kubernetes (K8s) - Kubernetes (often abbreviated as "K8s") is a platform providing container orchestration functionality. It was open sourced by Google around a decade ago and has seen widespread adoption and development in the ensuing years. K8s is the software that provides the core functionality of the Anvil Composable Subsystem by managing the complete lifecycle of containers. Additionally it provides the following functions: service discovery and load balancing, storage orchestration, secret and configuration management. The Kubernetes cluster can be accessed via the Rancher UI or the kubectl command line tool.
Rancher - Rancher is a “is a complete software stack for teams adopting containers.” as described by its website. It can be thought of as a wrapper around Kubernetes, providing an additional set of tools to help operate the K8 cluster efficiently and additional functionality that does not exist in Kubernetes itself. Two examples of the added functionality is the Rancher UI that provides an easy to use GUI interface in a browser and Rancher projects, a concept that allows for multi-tenancy within the cluster. Users can interact directly with Rancher using either the Rancher UI or Rancher CLI to deploy and manage workloads on the Anvil Composable Subsystem.
Rancher UI - The Rancher UI is a web based graphical interface to use the Anvil Composable Subsystem from anywhere.
Rancher CLI - The Rancher CLI provides a convenient text based toolkit to interact with the cluster. The binary can be downloaded from the link on the right hand side of the footer in the Rancher UI. After you download the Rancher CLI, you need to make a few configurations Rancher CLI requires:
-
Your Rancher Server URL, which is used to connect to Rancher Server.
-
An API Bearer Token, which is used to authenticate with Rancher. see Creating an API Key.
After setting up the Rancher CLI you can issue rancher --help
to view the full
range of options available.
Kubectl - Kubectl is a text based tool for working with the underlying Anvil Kubernetes cluster. In order to take advantage of kubectl you will either need to set up a Kubeconfig File or use the built in kubectl shell in the Rancher UI. You can learn more about kubectl and how to download the kubectl file here.
Storage - Storage is utilized to provide persistent data storage between container deployments. The Ceph filesystem provides access to Block, Object and shared file systems. File storage provides an interface to access data in a file and folder hierarchy similar to NTFS or NFS. Block storage is a flexible type of storage that allows for snapshotting and is good for database workloads and generic container storage. Object storage is also provided by Ceph, this features a REST based bucket file system providing S3 and Swift compatibility.
Access
How to Access the Anvil Composable Subsystem via the Rancher UI, the command line (kubectl) and the Anvil Harbor registry.
Rancher
Logging in to Rancher
The Anvil Composable Subsystem Rancher interface can be accessed via a web browser at https://composable.anvil.rcac.purdue.edu. Log in by choosing "log in with shibboleth" and using your ACCESS credentials at the ACCESS login screen.
kubectl
Link to section 'Configuring local kubectl access with Kubeconfig file' of 'kubectl' Configuring local kubectl access with Kubeconfig file
kubectl can be installed and run on your local machine to perform various actions against the Kubernetes cluster using the API server.
These tools authenticate to Kubernetes using information stored in a kubeconfig file.
To authenticate to the Anvil cluster you can download a kubeconfig file that is generated by Rancher as well as the kubectl tool binary.
-
From anywhere in the rancher UI navigate to the cluster dashboard by hovering over the box to the right of the cattle and selecting anvil under the "Clusters" banner.
-
Click on kubeconfig file at the top right
-
Click copy to clipboard
-
Create a hidden folder called .kube in your home directory
-
Copy the contents of your kubeconfig file from step 2 to a file called config in the newly create .kube directory
-
-
You can now issue commands using kubectl against the Anvil Rancher cluster
-
to look at the current config settings we just set use
kubectl config view
-
now let’s list the available resource types present in the API with
kubectl api-resources
-
To see more options of kubectl review the cheatsheet found on Kubernetes' kubectl cheatsheet.
Link to section 'Accessing kubectl in the rancher web UI' of 'kubectl' Accessing kubectl in the rancher web UI
You can launch a kubectl command window from within the Rancher UI by selecting the Launch kubectl button to the left of the Kubeconfig File button. This will deploy a container in the cluster with kubectl installed and give you an interactive window to use the command from.
Harbor
Link to section 'Logging into the Anvil Registry UI with ACCESS credentials' of 'Harbor' Logging into the Anvil Registry UI with ACCESS credentials
Harbor is configured to use ACCESS as an OpenID Connect (OIDC) authentication provider. This allows you to login using your ACCESS credentials.
To login to the harbor registry using your ACCESS credentials:
Navigate to https://registry.anvil.rcac.purdue.edu in your favorite web browser.
-
Click the Login via OIDC Provider button.
-
This redirects you to the ACCESS account for authentication.
-
-
If this is the first time that you are logging in to Harbor with OIDC, specify a user name for Harbor to associate with your OIDC username.
-
This is the user name by which you are identified in Harbor, which is used when adding you to projects, assigning roles, and so on. If the username is already taken, you are prompted to choose another one.
-
-
After the OIDC provider has authenticated you, you are redirected back to the Anvil Harbor Registry.
Workloads
Link to section 'Deploy a Workload' of 'Workloads' Deploy a Workload
- Using the top right dropdown select the Project or Namespace you wish to deploy to.
- Using the far left menu navigate to Workload
- Click Create at the top right
- Select the appropriate Deployment Type for your use case
- Select Namespace if not already done from step 1
- Set a unique Name for your deployment, i.e. “myapp"
- Set Container Image. Ensure you're using the Anvil registry for personal images or the Anvil registry docker-hub cache when pulling public docker-hub specific images. e.g:
registry.anvil.rcac.purdue.edu/my-registry/myimage:tag
orregistry.anvil.rcac.purdue.edu/docker-hub-cache/library/image:tag
- Click Create
Wait a couple minutes while your application is deployed. The “does not have minimum availability” message is expected. But, waiting more than 5 minutes for your workload to deploy typically indicates a problem. You can check for errors by clicking your workload name (i.e. "myapp"), then the lower button on the right side of your deployed pod and selecting View Logs
If all goes well, you will see an Active status for your deployment
You can then interact with your deployed container on the command line by clicking the button with three dots on the right side of the screen and choosing "Execute Shell"
Services
Link to section 'Service' of 'Services' Service
A Service is an abstract way to expose an application running on Pods as a network service. This allows the networking and application to be logically decoupled so state changes in either the application itself or the network connecting application components do not need to be tracked individually by all portions of an application.
Link to section 'Service resources' of 'Services' Service resources
In Kubernetes, a Service is an abstraction which defines a logical set of Pods and a policy by which to access them (sometimes this pattern is called a micro-service). The set of Pods targeted by a Service is usually determined by a Pod selector, but can also be defined other ways.
Link to section 'Publishing Services (ServiceTypes)' of 'Services' Publishing Services (ServiceTypes)
For some parts of your application you may want to expose a Service onto an external IP address, that’s outside of your cluster.
Kubernetes ServiceTypes allow you to specify what kind of Service you want. The default is ClusterIP.
-
ClusterIP: Exposes the Service on a cluster-internal IP. Choosing this value makes the Service only reachable from within the cluster. This is the default ServiceType.
-
NodePort: Exposes the Service on each Node’s IP at a static port (the NodePort). A ClusterIP Service, to which the NodePort Service routes, is automatically created. You’ll be able to contact the NodePort Service, from outside the cluster, by requesting
<NodeIP>:<NodePort>
. -
LoadBalancer: Exposes the Service externally using Anvil's load balancer. NodePort and ClusterIP Services, to which the external load balancer routes, are automatically created. Users can create LoadBalancer resources using a private IP pool on Purdue's network with the annotation
metallb.universe.tf/address-pool: anvil-private-pool
. Public LoadBalancer IPs are avaiable via request only.
You can see an example of exposing a workload using the LoadBalancer type on Anvil in the examples section.
-
ExternalName: Maps the Service to the contents of the externalName field (e.g. foo.bar.example.com), by returning a CNAME record with its value. No proxying of any kind is set up.
Link to section 'Ingress' of 'Services' Ingress
An Ingress is an API object that manages external access to the services in a cluster, typically HTTP/HTTPS. An Ingress is not a ServiceType, but rather brings external traffic into the cluster and then passes it to an Ingress Controller to be routed to the correct location. Ingress may provide load balancing, SSL termination and name-based virtual hosting. Traffic routing is controlled by rules defined on the Ingress resource.
You can see an example of a service being exposed with an Ingress on Anvil in the examples section.
Link to section 'Ingress Controller' of 'Services' Ingress Controller
Anvil provides the nginx ingress controller configured to facilitate SSL termination and automatic DNS name generation under the anvilcloud.rcac.purdue.edu
subdomain.
Kubernetes provides additional information about Ingress Controllers in the official documentation.
Registry
Link to section 'Accessing the Anvil Composable Registry' of 'Registry' Accessing the Anvil Composable Registry
The Anvil registry uses Harbor, an open source registry to manage containers and artifacts, it can be accessed at the following URL: https://registry.anvil.rcac.purdue.edu
Link to section 'Using the Anvil Registry Docker Hub Cache' of 'Registry' Using the Anvil Registry Docker Hub Cache
It’s advised that you use the Docker Hub cache within Anvil to pull images for deployments. There’s a limit to how many images Docker hub will allow to be pulled in a 24 hour period which Anvil reaches depending on user activity. This means if you’re trying to deploy a workload, or have a currently deployed workload that needs migrated, restarted, or upgraded, there’s a chance it will fail.
To bypass this, use the Anvil cache url registry.anvil.rcac.purdue.edu/docker-hub-cache/ in your image names
For example if you’re wanting to pull a notebook from jupyterhub’s Docker Hub repo e.g jupyter/tensorflow-notebook:latest
Pulling it from the Anvil cache would look like this registry.anvil.rcac.purdue.edu/docker-hub-cache/jupyter/tensorflow-notebook:latest
Link to section 'Using OIDC from the Docker or Helm CLI' of 'Registry' Using OIDC from the Docker or Helm CLI
After you have authenticated via OIDC and logged into the Harbor interface for the first time, you can use the Docker or Helm CLI to access Harbor.
The Docker and Helm CLIs cannot handle redirection for OIDC, so Harbor provides a CLI secret for use when logging in from Docker or Helm.
-
Log in to Harbor with an OIDC user account.
-
Click your username at the top of the screen and select User Profile.
-
Click the clipboard icon to copy the CLI secret associated with your account.
-
Optionally click the … icon in your user profile to display buttons for automatically generating or manually creating a new CLI secret.
-
A user can only have one CLI secret, so when a new secret is generated or create, the old one becomes invalid.
-
-
If you generated a new CLI secret, click the clipboard icon to copy it.
You can now use your CLI secret as the password when logging in to Harbor from the Docker or Helm CLI.
docker login -u <username> -p <cli secret> registry.anvil.rcac.purdue.edu
Link to section 'Creating a harbor Registry' of 'Registry' Creating a harbor Registry
-
Using a browser login to https://registry.anvil.rcac.purdue.edu with your ACCESS account username and password
-
From the main page click create project, this will act as your registry
-
Fill in a name and select whether you want the project to be public or private
-
Click ok to create and finalize
Link to section 'Tagging and Pushing Images to Your Harbor Registry' of 'Registry' Tagging and Pushing Images to Your Harbor Registry
-
Tag your image
$ docker tag my-image:tag registry.anvil.rcac.purdue.edu/project-registry/my-image:tag
-
login to the Anvil registry via command line
$ docker login registry.anvil.rcac.purdue.edu
-
Push your image to your project registry
$ docker push registry.anvil.rcac.purdue.edu/project-registry/my-image:tag
Link to section 'Creating a Robot Account for a Private Registry' of 'Registry' Creating a Robot Account for a Private Registry
A robot account and token can be used to authenticate to your registry in place of having to supply or store your private credentials on multi-tenant cloud environments like Rancher/Anvil.
-
Navigate to your project after logging into https://registry.anvil.rcac.purdue.edu
-
Navigate to the Robot Accounts tab and click New Robot Account
-
Fill out the form
-
Name your robot account
-
Select account expiration if any, select never to make permanent
-
Customize what permissions you wish the account to have
-
Click Add
-
-
Copy your information
-
Your robot’s account name will be something longer than what you specified, since this is a multi-tenant registry, harbor does this to avoid unrelated project owners creating a similarly named robot account
-
Export your token as JSON or copy it to a clipboard
-
Link to section 'Adding Your Private Registry to Rancher' of 'Registry' Adding Your Private Registry to Rancher
-
From your project navigate to
Resources > secrets
-
Navigate to the Registry Credentials tab and click Add Registry
-
Fill out the form
-
Give a name to the Registry secret (this is an arbitrary name)
-
Select whether or not the registry will be available to all or a single namespace
-
Select address as “custom” and provide “registry.anvil.rcac.purdue.edu”
-
Enter your robot account’s long name eg.
robot$my-registry+robot
as the Username -
Enter your robot account’s token as the password
-
Click Save
-
Link to section 'External Harbor Documentation' of 'Registry' External Harbor Documentation
-
You can learn more about the Harbor project on the official website: https://goharbor.io/
Storage
Storage is utilized to provide persistent data storage between container deployments and comes in a few options on Anvil.
The Ceph software is used to provide block, filesystem and object storage on the Anvil composable cluster. File storage provides an interface to access data in a file and folder hierarchy similar to NTFS or NFS. Block storage is a flexible type of storage that allows for snapshotting and is good for database workloads and generic container storage. Object storage is ideal for large unstructured data and features a REST based API providing an S3 compatible endpoint that can be utilized by the preexisting ecosystem of S3 client tools.
Link to section 'Storage Classes' of 'Storage' Storage Classes
Anvil Composable provides two different storage classes based on access characteristics needs of workloads.
- anvil-block - Block storage based on SSDs that can be accessed by a single node (Single-Node Read/Write).
anvil-filesystem
- File storage based on SSDs that can be accessed by multiple nodes (Many-Node Read/Write or Many-Node Read-Only)
Link to section 'Provisioning Block and Filesystem Storage for use in deployments' of 'Storage' Provisioning Block and Filesystem Storage for use in deployments
Block and Filesystem storage can both be provisioned in a similar way.
-
While deploying a Workload, select the Volumes drop down and click Add Volume…
-
Select “Add a new persistent volume (claim)”
-
Set a unique volume name, i.e. “<username>-volume”
-
Select a Storage Class. The default storage class is Ceph for this Kubernetes cluster
-
Request an amount of storage in Gigabytes
-
Click Define
-
Provide a Mount Point for the persistent volume: i.e /data
Link to section 'Backup Strategies' of 'Storage' Backup Strategies
Developers using the Anvil Composable Platform should have a backup strategy in place to ensure that your data is safe and can be recovered in case of a disaster. Below is a list of methods that can be used to backup data on Persistent Volume Claims.
Link to section 'Copying Files to and from a Container' of 'Storage' Copying Files to and from a Container
The kubectl cp
command can be used to copy files into or out of a running container.
# get pod id you want to copy to/form
kubectl -n <namespace> get pods
# copy a file from local filesystem to remote pod
kubectl cp /tmp/myfile <namespace>/<pod>:/tmp/myfile
# copy a file from remote pod to local filesystem
kubectl cp <namespace>/<pod>:/tmp/myfile /tmp/myfile
This method requires the tar
executable to be present in your container, which is usually the case with Linux image. More info can be found in the kubectl docs.
Link to section 'Copying Directories from a Container' of 'Storage' Copying Directories from a Container
The kubectl cp
command can also be used to recusively copy entire directories to local storage or places like Data Depot.
# get pod id you want to copy to/form
kubectl -n <namespace> get pods
# copy a directory from remote pod to local filesystem
kubectl cp <namespace>/<pod>:/pvcdirectory /localstorage
Link to section 'Backing up a Database from a Container' of 'Storage' Backing up a Database from a Container
The kubectl exec
command can be used to create a backup or dump of a database and save it to a local directory. For instance, to backup a MySQL database with kubectl, run the following commands from a local workstation or cluster frontend.
# get pod id of your database pod
kubectl -n <namespace> get pods
# run mysqldump in the remote pod and redirect the output to local storage
kubectl -n <namespace> exec <pod> -- mysqldump --user=<username> --password=<password> my_database > my_database_dump.sql
Link to section 'Backups using common Linux tools' of 'Storage' Backups using common Linux tools
If your container has the OpenSSH client or rsync packages installed, one can use the kubectl exec
command to copy or synchonize another storage location.
# get pod id of your pod
kubectl -n <namespace> get pods
# run scp to transfer data from the pod to a remote storage location
kubectl -n <namespace> <pod> exec -- scp -r /data username@anvil.rcac.purdue.edu:~/backup
Link to section 'Automating Backups' of 'Storage' Automating Backups
Kubernetes CronJob resources can be used with the commands above to create an automated backup solution. For more information, refer to the Kubernetes documentation.
Link to section 'Accessing object storage externally from local machine using Cyberduck' of 'Storage' Accessing object storage externally from local machine using Cyberduck
Cyberduck is a free server and cloud storage browser that can be used to access the public S3 endpoint provided by Anvil.
-
Launch Cyberduck
-
Click + Open Connection at the top of the UI.
-
Select S3 from the dropdown menu
-
Fill in Server, Access Key ID and Secret Access Key fields
-
Click Connect
-
You can now right click to bring up a menu of actions that can be performed against the storage endpoint
Further information about using Cyberduck can be found on the Cyberduck documentation site.
Examples
Examples of deploying a database with persistent storage and making it available on the network and deploying a webserver using a self-assigned URL.
Database
Link to section 'Deploy a postgis Database' of 'Database' Deploy a postgis Database
- Select your Project from the top right dropdown
- Using the far left menu, select Workload
- Click Create at the top right
- Select the appropriate Deployment Type for your use case, here we will select and use Deployment
- Fill out the form
- Select Namespace
- Give arbitrary Name
- Set Container Image to the postgis Docker image:
registry.anvil.rcac.purdue.edu/docker-hub-cache/postgis/postgis:latest
- Set the postgres user password
- Select the Add Variable button under the Environment Variables section
- Fill in the fields Variable Name and Value so that we have a variable
POSTGRES_PASSWORD = <some password>
- Create a persistent volume for your database
- Select the Storage tab from within the current form on the left hand side
- Select Add Volume and choose Create Persistent Volume Claim
- Give arbitrary Name
- Select Single-Node Read/Write
- Select appropriate Storage Class from the dropdown and give Capacity in GiB e.g 5
- Provide the default postgres data directory as a Mount Point for the persistent volume
/var/lib/postgresql/data
- Set Sub Path to
data
- Set resource CPU limitations
- Select Resources tab on the left within the current form
- Under the CPU Reservation box fill in
2000
This ensures that Kubernetes will only schedule your workload to nodes that have that resource amount available, guaranteeing your application has 2CPU cores to utilize - Under the CPU Limit box also will in 2000 This ensures that your workload cannot exceed or utilize more than 2CPU cores. This helps resource quota management on the project level.
- Setup Pod Label
- Select Labels & Annotations on the left side of the current form
- Select Add Label under the Pod Labels section
- Give arbitrary unique key and value you can remember later when creating Services and other resources e.g Key:
my-db
Value:postgis
- Select Create to launch the postgis database
Wait a couple minutes while your persistent volume is created and the postgis container is deployed. The “does not have minimum availability” message is expected. But, waiting more than 5 minutes for your workload to deploy typically indicates a problem. You can check for errors by clicking your workload name (i.e. "mydb"), then the lower button on the right side of your deployed pod and selecting View Logs If all goes well, you will see an Active status for your deployment
Link to section 'Expose the Database to external clients' of 'Database' Expose the Database to external clients
Use a LoadBalancer service to automatically assign an IP address on a private Purdue network and open the postgres port (5432). A DNS name will automatically be configured for your service as <servicename>.<namespace>.anvilcloud.rcac.purdue.edu
.
- Using the far left menu and navigate to Service Discovery > Services
- Select Create at the top right
- Select Load Balancer
- Fill out the form
- Ensure to select the namespace where you deployed the postgis database
- Give a Name to your Service. Remember that your final DNS name when the service creates will be in the format of
<servicename>.<namespace>.anvilcloud.rcac.purdue.edu
- Fill in Listening Port and Target Port with the postgis default port 5432
- Select the Selectors tab within the current form
- Fill in Key and Value with the label values you created during the Setup Pod Label step from earlier e.g Key:
my-db
Value:postgis
- IMPORTANT: The yellow bar will turn green if your key-value pair matches the pod label you set during the "Setup Pod Label" deployment step above. If you don't see a green bar with a matching Pod, your LoadBalancer will not work.
- Fill in Key and Value with the label values you created during the Setup Pod Label step from earlier e.g Key:
- Select the Labels & Annotations tab within the current form
- Select Add Annotation
- To deploy to a Purdue Private Address Range fill in Key:
metallb.universe.tf/address-pool
Value:anvil-private-pool
- To deploy to a Public Address Range fill in Key:
metallb.universe.tf/address-pool
Value:anvil-public-pool
Kubernetes will now automatically assign you an IP address from the Anvil Cloud private IP pool. You can check the IP address by hovering over the “5432/tcp” link on the Service Discovery page or by viewing your service via kubectl on a terminal.
$ kubectl -n <namespace> get services
Verify your DNS record was created:
$ host <servicename>.<namespace>.anvilcloud.rcac.purdue.edu
Web Server
Link to section 'Nginx Deployment' of 'Web Server' Nginx Deployment
- Select your Project from the top right dropdown
- Using the far left menu so select Workload
- Click Create at the top right
- Select the appropriate Deployment Type for your use case, here we will select and use Deployment
- Fill out the form
- Select Namespace
- Give arbitrary Name
- Set Container Image to the postgis Docker image:
registry.anvil.rcac.purdue.edu/docker-hub-cache/library/nginx
- Create a Cluster IP service to point our external accessible ingress to later
- Click Add Port
- Click Service Type and with the drop select Cluster IP
- In the Private Container Port box type 80
- Setup Pod Label
- Select Labels & Annotations on the left side of the current form
- Select Add Label under the Pod Labels section
- Give arbitrary unique key and value you can remember later when creating Services and other resources e.g Key:
my-web
Value:nginx
- Click Create
Wait a couple minutes while your application is deployed. The “does not have minimum availability” message is expected. But, waiting more than 5 minutes for your workload to deploy typically indicates a problem. You can check for errors by clicking your workload name (i.e. "mywebserver"), then using the vertical ellipsis on the right hand side of your deployed pod and selecting View Logs
If all goes well, you will see an Active status for your deployment.
Link to section 'Expose the web server to external clients via an Ingress' of 'Web Server' Expose the web server to external clients via an Ingress
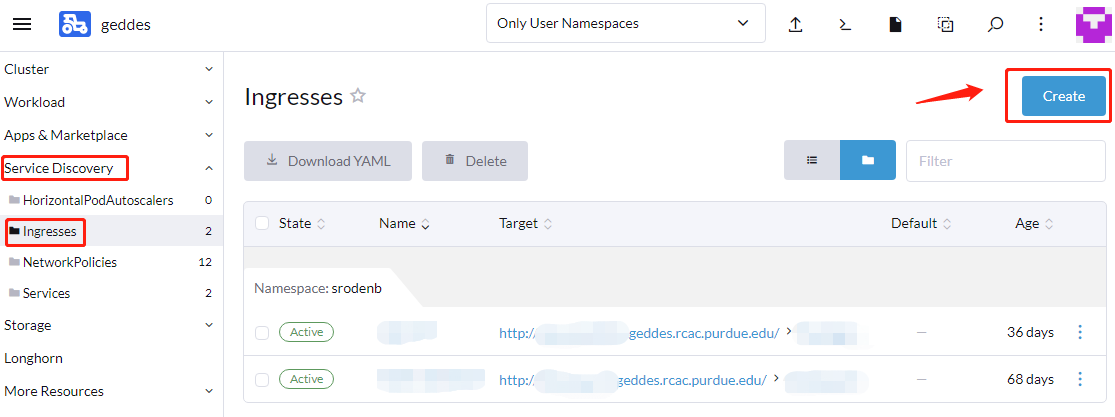
- Using the far left menu and navigate to Service Discovery > Ingresses and select Create at the top right
- Fill out the form
- Ensure to select the namespace where you deployed the nginx
- Give an arbitrary Name
- Under Request Host give the url you want for your web application e.g
my-nginx.anvilcloud.rcac.purdue.edu
- Fill in the value Path > Prefix as
/
- Use the Target Service and ;Port dropdowns to select the service you created during the Nginx Deployment section
- Click Create
Help
Link to section 'Looking for help?' of 'Help' Looking for help?
You can find varieties of help topics from the new ACCESS Support Portal, including helpful user guides, communities to connect with other researchers, submit a ticket for expert help, and longer-term MATCH Research Support, etc.
Link to section 'Send a ticket to Anvil support team' of 'Help' Send a ticket to Anvil support team
Specifically, if you would like to ask questions to our Anvil support team, you can send a ticket to ACCESS Help Desk:
-
Login to submit a ticket:
You should find the "login" button in the top right of the page. -
If you already have an XSEDE account, use your XSEDE portal username and password to login to ACCESS site. Make sure to choose
ACCESS-CI
as your identity provider:Follow "Log on with CILogon". Make sure to choose ACCESS-CI
as your identity provider.If you already have an XSEDE account, use your XSEDE portal username and password. -
ACCESS login requires Duo service for additional authentication. If you already set up XSEDE Duo service, you will continue to receive Duo pushes from ACCESS. If you have not set up Duo service, please refer to the Manage Multi-Factor Authentication page for account setup instructions.
-
Then, select
Anvil
from the resource list to send ticket to Anvil support team:Please follow the template in
Problem description
section when submitting a ticket to Anvil support:
Datasets
Please refer to our Federated Datasets Documentation website for up-to-date datasets on Anvil and instructions on how to use them.
AnvilGPT
AnvilGPT is an LLM service that makes open source LLM models like LLaMA accessible to ACCESS researchers. It is hosted entirely with on-prem resources at Purdue, not only providing democratized access but adding another layer of control compared to commercial services. Chats, documents, and models are not shared between users nor used for training.
There are two modalities for interacting with AnvilGPT, UI, and API, and additional functionality for both modalities is under active development. This system is integrated with a PostgreSQL vector database in the backend to enable retrieval-augmented generation (RAG) functionality.
Note this service is a pilot and provides only limited safety measures. Models may hallucinate or generate offensive content. AnvilGPT should not be used for any illegal, harmful, or violent purposes.
Do not input, by any method, any data into these systems that your research institution would consider sensitive or proprietary. Do not input, by any method, any data into these systems that is regulated by State or Federal Law. This includes, but is not limited to, HIPAA data, Export Controlled data, personal identification numbers (e.g. SSNs) or biometric data.
Link to section 'Access' of 'AnvilGPT' Access
Access is currently being given on a per request basis. Navigate to https://anvilgpt.rcac.purdue.edu/ and log in using CILogon. Select ACCESS as the identity provider. This will create a pending account.
Next, reach out to the help desk with the subject/summary line “AnvilGPT Access Request”. In the description, provide a brief description of how you intend to use the service, your allocation number, and if you would like access to the UI, API, or both. Select "Some Other Question" as the user support issue and "Anvil" as the resource. An admin will assess and approve your request and get back to you.
User Interface
Link to section 'Chat Interface' of 'User Interface' Chat Interface
Link to section 'Model Selection' of 'User Interface' Model Selection
The chat interface allows you to select from a list of available models to interface with. This list includes both base models available to all users as well as any custom models you have created (covered below).
You may also select multiple models to compare the output for any prompt. In this case, your prompt will be sent to all selected models and the results displayed side-by-side.
If there are additional open-source models you would like to use that are not available in the drop-down, please send in a ticket request. We are able to provide access to most open-source models.
Link to section 'Sending a Message' of 'User Interface' Sending a Message
Sending a prompt is as simple as adding text into the message bar. By clicking the microphone or headset buttons you can also use a speech-to-text service or have a “call” with the model where you can speak it the model will speak back.
You can also add a document to give the model specific context to respond to the prompt with. If you would like this context to be persisted, upload it to documents or add it to a custom model as discussed below. To add an existing document that was previously uploaded into the document interface to the context of a chat, use ‘#’ and then the name of the document before entering your prompt.
When you get a model response, you can take various action including editing, copying, or reading the response out loud, and view statistics about the generation with the options available at the bottom of the response.
Link to section 'Other Controls' of 'User Interface' Other Controls
From the top left of the screen, you can access various controls which enable you to tweak the parameters and system prompt for the chat. To persist this information, use a custom model. From these options you are also able to download chats and change other settings related to the UI.
On the left of the screen you can create new chats, access your chat history, and access the workspace where you can upload documents and create custom models.
Link to section 'Workspace' of 'User Interface' Workspace
From the workspace you can upload documents and create models with a RAG functionality.
Link to section 'Documents' of 'User Interface' Documents
In the documents tab, use the ‘+’ button to upload documents. Select documents using the upload functionality then add it to a collection if you wish by specifying a name. The collection can be used to create different groupings of documents, for example to create custom RAGs focusing on different tasks. Documents will not be shared across users, but please do not upload documents with sensitive information or that are subject to regulations.
Link to section 'Models' of 'User Interface' Models
To create a custom model, navigate to the Models tab in the workspace and click “Create a model”.
From here, you can customize a model by specifying the base model, system prompt, and any context documents or collections of documents, among other customizations. Documents/collections must be uploaded in the document tab before they will be accessible here. Once the model has been created it will show up in the model list in the chat interface.
API
To use the API, you wil first need to find your API key, accessible from the UI. Click on your user avatar and navigate to Settings, and then the Account page in settings. Here you will be able to see the API Keys. Expand this section and copy either the JWT token or create an API key.
Use the following endpoint to connect to the API.
For an OpenAI API-compatible response, use https://anvilgpt.rcac.purdue.edu/api/chat/completions
For a streaming response, this will return results formated like:
data: {"id": "llama3.1:latest-dd3f8a12-a36f-4fcc-9537-02a9e7c1d9c8", "created": 1749755137, "model": "llama3.1:latest", "choices": [{"index": 0, "logprobs": null, "finish_reason": null, "delta": {"content": "I"}}], "object": "chat.completion.chunk"}
For a non-streaming response, this will return results formated like:
{"id":"llama3.1:latest-703c01b4-c58d-4f78-b7e3-f0df34a91ede","created":1749755251,"model":"llama3.1:latest","choices":[{"index":0,"logprobs":null,"finish_reason":"stop","message":{"content":"I don't have a personal name. I'm an AI designed to assist and communicate with users, so I'm often referred to as a \"chatbot\" or simply \"Assistant.\" If you'd like, I can generate a unique identifier for our conversation, but it won't be a traditional name. How can I help you today?","role":"assistant"}}],"object":"chat.completion","usage":{"response_token/s":180.38,"prompt_token/s":13403.27,"total_duration":397326193,"load_duration":13348992,"prompt_eval_count":15,"prompt_tokens":15,"prompt_eval_duration":1119130,"eval_count":69,"completion_tokens":69,"eval_duration":382515521,"approximate_total":"0h0m0s","total_tokens":84,"completion_tokens_details":{"reasoning_tokens":0,"accepted_prediction_tokens":0,"rejected_prediction_tokens":0}}}
To use the endpoint, insert your API key in the example Python code below:
import requests
url = "https://anvilgpt.rcac.purdue.edu/api/chat/completions"
headers = {
"Authorization": f"Bearer {jwt_token_or_api_key}",
"Content-Type": "application/json"
}
body = {
"model": "llama3.1:latest",
"messages": [
{
"role": "user",
"content": "What is your name?"
}
],
"stream": True
}
response = requests.post(url, headers=headers, json=body)
if response.status_code == 200:
print(response.text)
else:
raise Exception(f"Error: {response.status_code}, {response.text}")
This will return output in a JSON format along with metadata.
Gautschi User Guide
Gautschi is a Community Cluster optimized for communities running traditional, tightly-coupled science and engineering applications.
Link to section 'Overview of Gautschi' of 'Overview of Gautschi' Overview of Gautschi
Gautschi is a Community Cluster optimized for communities running traditional, tightly-coupled science and engineering applications. The Gautschi Community Cluster is equipped with both CPU and GPU compute nodes, each designed for specific computational tasks. The cluster includes Dell CPU compute nodes that feature dual 96-core AMD Epyc "Genoa" processors, providing 192 cores per node and 384 GB of memory. GPU compute nodes come with two 56-core Intel Xeon Platinum 8480+ processors, a total of 1031 GB of CPU memory, and eight NVIDIA H100 GPUs, each boasting 80 GB of memory. All compute nodes have 400 Gbps NDR Infiniband interconnect and service through 2030.
Access to the Gautschi cluster is being offered in units of 48-cores or one quarter share of a CPU node or 5 GPU-year package for GPU nodes. To purchase access to Gautschi today, go to the Cluster Access Purchase page. Please subscribe to our Community Cluster Program Mailing List to stay informed on the latest purchasing developments or contact us via email at rcac-cluster-purchase@lists.purdue.edu if you have any questions.
Link to section 'Gautschi Namesake' of 'Overview of Gautschi' Gautschi Namesake
Gautschi is named in honor of Dr. Walter Gautschi, the Professor in the Department of Computer Science and Mathematics at Purdue. More information about his life and impact on Purdue is available in a Biography of Gautschi.
Link to section 'Gautschi Specifications' of 'Overview of Gautschi' Gautschi Specifications
All Gautschi CPU compute nodes have 192 processing cores, 384 GB of memory, and 400 Gbps NDR Infiniband interconnects. Each Gautschi GPU compute node has eight NVIDIA H100 GPUs with 80 GB of GPU memory, 112 processing cores, 1032 GB of CPU memory, and 400 Gbps NDR Infiniband interconnects.
Front-Ends | Number of Nodes | Processors per Node | Cores per Node | Memory per Node | Retires in |
---|---|---|---|---|---|
8 | Two AMD EPYC 9654 96-Core Processor “Genoa” CPUs @ 2.4 GHz | 192 | 768 GB | 2030 |
Sub-Cluster | Number of Nodes | Processors per Node | Cores per Node | Memory per Node | Retires in |
---|---|---|---|---|---|
A | 338 | Two AMD EPYC 9654 96-Core Processor “Genoa” CPUs @ 2.4 GHz | 192 | 384 GB | 2030 |
B | 6 | Two AMD Epyc 9654 96-Core Processor “Genoa” CPUs @ 2.4GHz | 192 | 1.5 TB | 2030 |
G | 6 | Two AMD EPYC 9554 64-Core Processor “Genoa” CPUs @ 3.1GHz, Two NVIDIA L40S GPUs (48GB) |
128 | 384 GB | 2030 |
H | 20 | Two Intel Xeon Platinum 8480+ 56-Core CPUs @ 3.8GHz, Eight NVIDIA H100 GPUs (80GB) |
112 | 1031 GB | 2030 |
Gautschi nodes run Rocky Linux 9 and use Slurm (Simple Linux Utility for Resource Management) as the batch scheduler for resource and job management. The application of operating system patches occurs as security needs dictate.
On Gautschi, the following set of compiler and message-passing libraries for parallel code are recommended:
- GCC 14.1.0
- OpenMPI
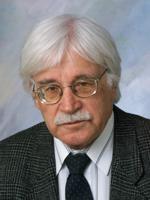
Link to section 'Walter Gautschi' of 'Biography of Gautschi' Walter Gautschi
Professor Gautschi is the Professor Emeritus of Computer Science and Mathematics in the Department of Computer Science at Purdue. Before coming to Purdue, Professor Gautschi did postdoctoral work as a Janggen-Pöhn Research Fellow at the National Institute of Applied Mathematics in Rome and at the Harvard Computation Laboratory. He also held positions at the National Bureau of Standards, the American University, the Oak Ridge National Laboratory, and the University of Tennessee. Since coming to Purdue, he has been a Fulbright Scholar at the Technical University of Munich and has held visiting appointments at the University of Wisconsin, Argonne National Laboratory, the Wright-Patterson Air Force Base, ETH Zurich, the University of Padova, and the University of Basel.
He has been a Fulbright Lecturer, an ACM National Lecturer, and a SIAM Visiting Lecturer. He is, or has been, on the editorial boards of SIAM Journal on Mathematical Analysis, Numerische Mathematik, Calcolo, and Mathematics of Computation, and has served as a special editor for Linear Algebra and Its Applications. From 1984 to 1995, he was the managing editor of Mathematics of Computation and, since 1991, an honorary editor of Numerische Mathematik. In 2001, Professor Gautschi was elected a Corresponding Member of the Bavarian Academy of Sciences and Humanities and, in the same year, a Foreign Member of the Academy of Sciences of Turin.
Link to section 'Accounts on Gautschi' of 'Accounts' Accounts on Gautschi
Link to section 'Obtaining an Account' of 'Accounts' Obtaining an Account
To obtain an account, you must be part of a research group which has purchased access to Gautschi. Refer to the Accounts / Access page for more details on how to request access.
Link to section 'Outside Collaborators' of 'Accounts' Outside Collaborators
A valid Purdue Career Account is required for access to any resource. If you do not currently have a valid Purdue Career Account you must have a current Purdue faculty or staff member file a Request for Privileges (R4P) before you can proceed.
Logging In
To submit jobs on Gautschi, log in to the submission host gautschi.rcac.purdue.edu
via SSH. This submission host is actually 8 front-end hosts: login00.gautschi
through login07.gautschi
. The login process randomly assigns one of these front-ends to each login to gautschi.rcac.purdue.edu
.
Purdue Login
Link to section 'SSH' of 'Purdue Login' SSH
- SSH to the cluster as usual.
- When asked for a password, type your password followed by "
,push
". - Your Purdue Duo client will receive a notification to approve the login.
Link to section 'Thinlinc' of 'Purdue Login' Thinlinc
- When asked for a password, type your password followed by "
,push
". - Your Purdue Duo client will receive a notification to approve the login.
- The native Thinlinc client will prompt for Duo approval twice due to the way Thinlinc works.
- The native Thinlinc client also supports key-based authentication.
Passwords
Gautschi supports either Purdue two-factor authentication (Purdue Login) or SSH keys.
SSH Client Software
Secure Shell or SSH is a way of establishing a secure connection between two computers. It uses public-key cryptography to authenticate the user with the remote computer and to establish a secure connection. Its usual function involves logging in to a remote machine and executing commands. There are many SSH clients available for all operating systems:
Linux / Solaris / AIX / HP-UX / Unix:
- The
ssh
command is pre-installed. Log in usingssh myusername@gautschi.rcac.purdue.edu
from a terminal.
Microsoft Windows:
- MobaXterm is a small, easy to use, full-featured SSH client. It includes X11 support for remote displays, SFTP capabilities, and limited SSH authentication forwarding for keys.
Mac OS X:
- The
ssh
command is pre-installed. You may start a local terminal window from "Applications->Utilities". Log in by typing the commandssh myusername@gautschi.rcac.purdue.edu
.
When prompted for password, enter your Purdue career account password followed by ",push
". Your Purdue Duo client will then receive a notification to approve the login.
SSH Keys
Link to section 'General overview' of 'SSH Keys' General overview
To connect to Gautschi using SSH keys, you must follow three high-level steps:
- Generate a key pair consisting of a private and a public key on your local machine.
- Copy the public key to the cluster and append it to
$HOME/.ssh/authorized_keys
file in your account. - Test if you can ssh from your local computer to the cluster without using your Purdue password.
Detailed steps for different operating systems and specific SSH client softwares are give below.
Link to section 'Mac and Linux:' of 'SSH Keys' Mac and Linux:
-
Run
ssh-keygen
in a terminal on your local machine. You may supply a filename and a passphrase for protecting your private key, but it is not mandatory. To accept the default settings, press Enter without specifying a filename.
Note: If you do not protect your private key with a passphrase, anyone with access to your computer could SSH to your account on Gautschi. -
By default, the key files will be stored in
~/.ssh/id_rsa
and~/.ssh/id_rsa.pub
on your local machine. -
Copy the contents of the public key into
$HOME/.ssh/authorized_keys
on the cluster with the following command. When asked for a password, type your password followed by ",push
". Your Purdue Duo client will receive a notification to approve the login.ssh-copy-id -i ~/.ssh/id_rsa.pub myusername@gautschi.rcac.purdue.edu
Note: use your actual Purdue account user name.
If your system does not have the
ssh-copy-id
command, use this instead:cat ~/.ssh/id_rsa.pub | ssh myusername@gautschi.rcac.purdue.edu "mkdir -p ~/.ssh && chmod 700 ~/.ssh && cat >> ~/.ssh/authorized_keys"
-
Test the new key by SSH-ing to the server. The login should now complete without asking for a password.
-
If the private key has a non-default name or location, you need to specify the key by
ssh -i my_private_key_name myusername@gautschi.rcac.purdue.edu
Link to section 'Windows:' of 'SSH Keys' Windows:
Programs | Instructions |
---|---|
MobaXterm | Open a local terminal and follow Linux steps |
Git Bash | Follow Linux steps |
Windows 10 PowerShell | Follow Linux steps |
Windows 10 Subsystem for Linux | Follow Linux steps |
PuTTY | Follow steps below |
PuTTY:
-
Launch PuTTYgen, keep the default key type (RSA) and length (2048-bits) and click Generate button.
The "Generate" button can be found under the "Actions" section of the PuTTY Key Generator interface. -
Once the key pair is generated:
Use the Save public key button to save the public key, e.g.
Documents\SSH_Keys\mylaptop_public_key.pub
Use the Save private key button to save the private key, e.g.
Documents\SSH_Keys\mylaptop_private_key.ppk
. When saving the private key, you can also choose a reminder comment, as well as an optional passphrase to protect your key, as shown in the image below. Note: If you do not protect your private key with a passphrase, anyone with access to your computer could SSH to your account on Gautschi.The PuTTY Key Generator form has inputs for the Key passphrase and optional reminder comment. From the menu of PuTTYgen, use the "Conversion -> Export OpenSSH key" tool to convert the private key into openssh format, e.g.
Documents\SSH_Keys\mylaptop_private_key.openssh
to be used later for Thinlinc. -
Configure PuTTY to use key-based authentication:
Launch PuTTY and navigate to "Connection -> SSH ->Auth" on the left panel, click Browse button under the "Authentication parameters" section and choose your private key, e.g. mylaptop_private_key.ppk
After clicking Connection -> SSH ->Auth panel, the "Browse" option can be found at the bottom of the resulting panel. Navigate back to "Session" on the left panel. Highlight "Default Settings" and click the "Save" button to ensure the change in place.
-
Connect to the cluster. When asked for a password, type your password followed by "
,push
". Your Purdue Duo client will receive a notification to approve the login. Copy the contents of public key from PuTTYgen as shown below and paste it into$HOME/.ssh/authorized_keys
. Please double-check that your text editor did not wrap or fold the pasted value (it should be one very long line).The "Public key" will look like a long string of random letters and numbers in a text box at the top of the window. - Test by connecting to the cluster. If successful, you will not be prompted for a password or receive a Duo notification. If you protected your private key with a passphrase in step 2, you will instead be prompted to enter your chosen passphrase when connecting.
SSH X11 Forwarding
SSH supports tunneling of X11 (X-Windows). If you have an X11 server running on your local machine, you may use X11 applications on remote systems and have their graphical displays appear on your local machine. These X11 connections are tunneled and encrypted automatically by your SSH client.
Link to section 'Installing an X11 Server' of 'SSH X11 Forwarding' Installing an X11 Server
To use X11, you will need to have a local X11 server running on your personal machine. Both free and commercial X11 servers are available for various operating systems.
Linux / Solaris / AIX / HP-UX / Unix:
- An X11 server is at the core of all graphical sessions. If you are logged in to a graphical environment on these operating systems, you are already running an X11 server.
- ThinLinc is an alternative to running an X11 server directly on your Linux computer. ThinLinc is a service that allows you to connect to a persistent remote graphical desktop session.
Microsoft Windows:
- ThinLinc is an alternative to running an X11 server directly on your Windows computer. ThinLinc is a service that allows you to connect to a persistent remote graphical desktop session.
- MobaXterm is a small, easy to use, full-featured SSH client. It includes X11 support for remote displays, SFTP capabilities, and limited SSH authentication forwarding for keys.
Mac OS X:
- X11 is available as an optional install on the Mac OS X install disks prior to 10.7/Lion. Run the installer, select the X11 option, and follow the instructions. For 10.7+ please download XQuartz.
- ThinLinc is an alternative to running an X11 server directly on your Mac computer. ThinLinc is a service that allows you to connect to a persistent remote graphical desktop session.
Link to section 'Enabling X11 Forwarding in your SSH Client' of 'SSH X11 Forwarding' Enabling X11 Forwarding in your SSH Client
Once you are running an X11 server, you will need to enable X11 forwarding/tunneling in your SSH client:
ssh
: X11 tunneling should be enabled by default. To be certain it is enabled, you may usessh -Y
.- MobaXterm: Select "New session" and "SSH." Under "Advanced SSH Settings" check the box for X11 Forwarding.
SSH will set the remote environment variable $DISPLAY
to "localhost:XX.YY"
when this is working correctly. If you had previously set your $DISPLAY
environment variable to your local IP or hostname, you must remove any set
/export
/setenv
of this variable from your login scripts. The environment variable $DISPLAY
must be left as SSH sets it, which is to a random local port address. Setting $DISPLAY
to an IP or hostname will not work.
ThinLinc
RCAC provides Cendio's ThinLinc as an alternative to running an X11 server directly on your computer. It allows you to run graphical applications or graphical interactive jobs directly on Gautschi through a persistent remote graphical desktop session.
ThinLinc is a service that allows you to connect to a persistent remote graphical desktop session. This service works very well over a high latency, low bandwidth, or off-campus connection compared to running an X11 server locally. It is also very helpful for Windows users who do not have an easy to use local X11 server, as little to no set up is required on your computer.
There are two ways in which to use ThinLinc: preferably through the native client or through a web browser.
Link to section 'Installing the ThinLinc native client' of 'ThinLinc' Installing the ThinLinc native client
The native ThinLinc client will offer the best experience especially over off-campus connections and is the recommended method for using ThinLinc. It is compatible with Windows, Mac OS X, and Linux.
- Download the ThinLinc client from the ThinLinc website.
- Start the ThinLinc client on your computer.
- In the client's login window, use desktop.gautschi.rcac.purdue.edu as the Server. Use your Purdue Career Account username and password, but append "
,push
" to your password. - Click the Connect button.
- Your Purdue Login Duo will receive a notification to approve your login.
- Continue to following section on connecting to Gautschi from ThinLinc.
Link to section 'Using ThinLinc through your web browser' of 'ThinLinc' Using ThinLinc through your web browser
The ThinLinc service can be accessed from your web browser as a convenience to installing the native client. This option works with no set up and is a good option for those on computers where you do not have privileges to install software. All that is required is an up-to-date web browser. Older versions of Internet Explorer may not work.
- Open a web browser and navigate to desktop.gautschi.rcac.purdue.edu.
- Log in with your Purdue Career Account username and password, but append "
,push
" to your password. - You may safely proceed past any warning messages from your browser.
- Your Purdue Login Duo will receive a notification to approve your login.
- Continue to the following section on connecting to Gautschi from ThinLinc.
Link to section 'Connecting to Gautschi from ThinLinc' of 'ThinLinc' Connecting to Gautschi from ThinLinc
- Once logged in, you will be presented with a remote Linux desktop running directly on a cluster front-end.
- Open the terminal application on the remote desktop.
- Once logged in to the Gautschi head node, you may use graphical editors, debuggers, software like Matlab, or run graphical interactive jobs. For example, to test the X forwarding connection issue the following command to launch the graphical editor gedit:
$ gedit
- This session will remain persistent even if you disconnect from the session. Any interactive jobs or applications you left running will continue running even if you are not connected to the session.
Link to section 'Tips for using ThinLinc native client' of 'ThinLinc' Tips for using ThinLinc native client
- To exit a full screen ThinLinc session press the F8 key on your keyboard (fn + F8 key for Mac users) and click to disconnect or exit full screen.
- Full screen mode can be disabled when connecting to a session by clicking the Options button and disabling full screen mode from the Screen tab.
Link to section 'Configure ThinLinc to use SSH Keys' of 'ThinLinc' Configure ThinLinc to use SSH Keys
- The web client does NOT support public-key authentication.
-
ThinLinc native client supports the use of an SSH key pair. For help generating and uploading keys to the cluster, see SSH Keys section in our user guide for details.
To set up SSH key authentication on the ThinLinc client:
-
Open the Options panel, and select Public key as your authentication method on the Security tab.
The "Options..." button in the ThinLinc Client can be found towards the bottom left, above the "Connect" button. -
In the options dialog, switch to the "Security" tab and select the "Public key" radio button:
The "Security" tab found in the options dialog, will be the last of available tabs. The "Public key" option can be found in the "Authentication method" options group. - Click OK to return to the ThinLinc Client login window. You should now see a Key field in place of the Password field.
-
In the Key field, type the path to your locally stored private key or click the ... button to locate and select the key on your local system. Note: If PuTTY is used to generate the SSH Key pairs, please choose the private key in the openssh format.
The ThinLinc Client login window will now display key field instead of a password field.
-
Software
Link to section 'Environment module' of 'Software' Environment module
Link to section 'Software catalog' of 'Software' Software catalog
Our clusters provide a number of software packages to users of the system via the module
command.
Link to section 'Environment Management with the Module Command' of 'Environment Management with the Module Command' Environment Management with the Module Command
The module command is the preferred method to manage your processing environment. With this command, you may load applications and compilers along with their libraries and paths. Modules are packages that you load and unload as needed.
Please use the module command and do not manually configure your environment, as staff may make changes to the specifics of various packages. If you use the module command to manage your environment, these changes will not be noticeable.
Link to section 'Hierarchy' of 'Environment Management with the Module Command' Hierarchy
Many modules have dependencies on other modules. For example, a particular openmpi
module requires a specific version of the Intel compiler to be loaded. Often, these dependencies are not clear to users of the module, and there are many modules which may conflict. Arranging modules in a hierarchical fashion makes this dependency clear. This arrangement also helps make the software stack easy to understand - your view of the modules will not be cluttered with a bunch of conflicting packages.
Your default module view on Gautschi will include a set of compilers and a set of basic software that has no dependencies (such as Matlab and Fluent). To make software available that depends on a compiler, you must first load the compiler, and then software which depends on it becomes available to you. In this way, all software you see when doing module avail is completely compatible with each other.
Link to section 'Using the Hierarchy' of 'Environment Management with the Module Command' Using the Hierarchy
Your default module view on Gautschi will include a set of compilers and a set of basic software that has no dependencies (such as Matlab and Fluent).
To see what modules are available on this system by default:
$ module avail
To see which versions of a specific compiler are available on this system:
$ module avail gcc
$ module avail intel
To continue further into the hierarchy of modules, you will need to choose a compiler. As an example, if you are planning on using the Intel compiler you will first want to load
the Intel compiler:
$ module load intel
With intel
loaded, you can repeat the avail
command, and at the bottom of the output you will see the section of additional software that the intel module provides:
$ module avail
Several of these new packages also provide additional software packages, such as MPI libraries. You can repeat the last two steps with one of the MPI packages such as openmpi
and you will have a few more software packages available to you.
If you are looking for a specific software package and do not see it in your default view, the module command provides a search function for searching the entire hierarchy tree of modules without the need for you to manually load
and avail
on every module.
To search for a software package:
$ module spider openmpi
------------------------------------------------------------------------------------------------------------------------------------------------------------------------------------------
openmpi:
------------------------------------------------------------------------------------------------------------------------------------------------------------------------------------------
Versions:
openmpi/4.1.6
openmpi/5.0.5
------------------------------------------------------------------------------------------------------------------------------------------------------------------------------------------
For detailed information about a specific "openmpi" package (including how to load the modules) use the module's full name.
Note that names that have a trailing (E) are extensions provided by other modules.
For example:
$ module spider openmpi/5.0.5
------------------------------------------------------------------------------------------------------------------------------------------------------------------------------------------
This will search for the openmpi
software package. If you do not specify a specific version of the package, you will be given a list of versions available on the system. Select the version you wish to use and spider
that to see how to access the module:
$ module spider openmpi/5.0.5
------------------------------------------------------------------------------------------------------------------------------------------------------------------------------------------
openmpi: openmpi/5.0.5
------------------------------------------------------------------------------------------------------------------------------------------------------------------------------------------
You will need to load all module(s) on any one of the lines below before the "openmpi/5.0.5" module is available to load.
gcc/11.4.1
gcc/14.1.0
Help:
An open source Message Passing Interface implementation. The Open MPI
Project is an open source Message Passing Interface implementation that
is developed and maintained by a consortium of academic, research, and
industry partners. Open MPI is therefore able to combine the expertise,
technologies, and resources from all across the High Performance
Computing community in order to build the best MPI library available.
Open MPI offers advantages for system and software vendors, application
developers and computer science researchers.
The output of this command will instruct you that you can load this module directly, or in the case of the above example, that you will need to first load a module or two. With the information provided with this command, you can now construct a load
command to load a version of OpenMPI into your environment:
$ module load gcc/14.1.0 openmpi/5.0.5
Some user communities may maintain copies of their domain software for others to use. For example, the Purdue Bioinformatics Core provides a wide set of bioinformatics software for use by any user of RCAC clusters via the bioinfo
module. The spider
command will also search this repository of modules. If it finds a software package available in the bioinfo module repository, the spider
command will instruct you to load the bioinfo module first.
Link to section 'Load / Unload a Module' of 'Environment Management with the Module Command' Load / Unload a Module
All modules consist of both a name and a version number. When loading a module, you may use only the name to load the default version, or you may specify which version you wish to load.
For each cluster, RCAC makes a recommendation regarding the set of compiler, math library, and MPI library for parallel code. To load the recommended set:
$ module load rcac
To verify what you loaded:
$ module list
To load the default version of a specific compiler, choose one of the following commands:
$ module load gcc
$ module load intel
To load a specific version of a compiler, include the version number:
$ module load gcc/11.2.0
When running a job, you must use the job submission file to load on the compute node(s) any relevant modules. Loading modules on the front end before submitting your job makes the software available to your session on the front-end, but not to your job submission script environment. You must load the necessary modules in your job submission script.
To unload a compiler or software package you loaded previously:
$ module unload gcc
$ module unload intel
$ module unload matlab
To unload all currently loaded modules and reset your environment:
$ module purge
Link to section 'Show Module Details' of 'Environment Management with the Module Command' Show Module Details
To learn more about what a module does to your environment, you may use the module show command.
$ module show matlab
Here is an example showing what loading the default Matlab does to the processing environment:
-------------------------------------------------------------------------------------------------------------------------------------------
/opt/spack/modulefiles/Core/matlab/R2022a.lua:
-------------------------------------------------------------------------------------------------------------------------------------------------------------------------------------
whatis("Name : matlab")
whatis("Version : R2022a")
...
setenv("MATLAB_HOME","/apps/spack/gautschi/apps/matlab/R2022a-gcc-8.5.0-u54n6sa")
setenv("RCAC_MATLAB_ROOT","/apps/spack/gautschi/apps/matlab/R2022a-gcc-8.5.0-u54n6sa")
setenv("RCAC_MATLAB_VERSION","R2022a")
setenv("MATLAB","/apps/spack/gautschi/apps/matlab/R2022a-gcc-8.5.0-u54n6sa")
setenv("MLROOT","/apps/spack/gautschi/apps/matlab/R2022a-gcc-8.5.0-u54n6sa")
setenv("ARCH","glnxa64")
append_path("PATH","/apps/spack/gautschi/apps/matlab/R2022a-gcc-8.5.0-u54n6sa/bin/glnxa64:/apps/spack/gautschi/apps/matlab/R2019a-gcc-4.8.5-jg35hvf/bin")
append_path("CMAKE_PREFIX_PATH","/apps/spack/gautschi/apps/matlab/R2022a-gcc-8.5.0-u54n6sa/")
append_path("LD_LIBRARY_PATH","/apps/spack/gautschi/apps/matlab/R2022a-gcc-8.5.0-u54n6sa/runtime/glnxa64:/apps/spack/gautschi/apps/matlab/R2022a-gcc-8.5.0-u54n6sa/bin/glnxa64")
Link to section 'GPU modules' of 'Environment Management with the Module Command' GPU modules
We use different module branch for GPU applications on Gautschi. To use the GPU modules, you can module load
the gpu module branch first:
$ module load modtree/gpu
After loading the modtree/gpu
, you are able to use module spider
to search modules or module load
to add the desired module to your environment.
For more information about Lmod:
Running Jobs
There is one method for submitting jobs to Gautschi. You may use SLURM to submit jobs to a partition on Gautschi. SLURM performs job scheduling. Jobs may be any type of program. You may use either the batch or interactive mode to run your jobs. Use the batch mode for finished programs; use the interactive mode only for debugging.
In this section, you'll find a few pages describing the basics of creating and submitting SLURM jobs. As well, a number of example SLURM jobs that you may be able to adapt to your own needs.
Basics of SLURM Jobs
The Simple Linux Utility for Resource Management (SLURM) is a system providing job scheduling and job management on compute clusters. With SLURM, a user requests resources and submits a job to a queue. The system will then take jobs from queues, allocate the necessary nodes, and execute them.
Do NOT run large, long, multi-threaded, parallel, or CPU-intensive jobs on a front-end login host. All users share the front-end hosts, and running anything but the smallest test job will negatively impact everyone's ability to use Gautschi. Always use SLURM to submit your work as a job.
Link to section 'Submitting a Job' of 'Basics of SLURM Jobs' Submitting a Job
The main steps to submitting a job are:
Follow the links below for information on these steps, and other basic information about jobs. A number of example SLURM jobs are also available.
Queues
On Gautschi, the required options for job submisison deviates from some of the other community clusters you might have experience using. In general every job submission will have four parts:
- The number and type of resources you want
- The account the resources should come out of
- The quality of service (QOS) this job expects from the resources
- The partition where the resources are located
If you have used other clusters, you will be familiar with first item. If you have not, you can read about how to format the request on our job submission page. The rest of this page will focus on the last three items as they are specific to Gautschi.
Link to section 'Accounts' of 'Queues' Accounts
On the Gautschi community cluster, users will have access to one or more accounts, also known as queues. These accounts are dedicated to and named after each partner who has purchased access to the cluster, and they provide partners and their researchers with priority access to their portion of the cluster. These accounts can be thought of as bank accounts that contain the resources a group has purchased access to which may include some number of cores, GPU hours or both. To see the list of accounts that you have access to on Gautschi as well as the resources they contain, you can use the command "slist".
On previous clusters, you may have had access to several other special accounts like "standby", "highmem", "gpu", etc. On Gautschi, these modes of access still exist, but they are no longer accounts. As well on Gautschi, you must explicitly define the account that you want to submit to using the "-A/--account=" option.
Link to section 'Quality of Service (QOS)' of 'Queues' Quality of Service (QOS)
On Gautschi, we use a Slurm concept called a Quality of Service or a QOS. A QOS can be thought of as a tag for a job that tells the scheduler how that job should be treated with respect to limits, priority, etc. The cluster administrators define the available QOSes as well as the policies for how each QOS should be treated on the cluster. A toy example of such a policy may be "no single user can have more than 200 jobs" that has been tagged with a QOS named "highpriority". There are two classes of QOSes and a job can have both:
- Partition QOSes: A partition QOS is a tag that is automatically added to your job when you submit to a partition that defines a partition QOS.
- Job QOSes: A Job QOS is a tag that you explicitly give to a job using the option "-q/--qos=". By explicitly tagging your jobs this way, you can choose the policy that each one of your jobs should abide by. We will describe the policies for the available job QOSes in the partition section below.
As an extended metaphor, if we think of a job as a package that we need to have shipped to some destination, then the partition can be thought of as the carrier we decide to ship our package with. That carrier is going to have some company policies that dictate how you need to label/pack that package, and that company policy is like the partition QOS. It is the policy that is enforced for simply deciding to submit to a particular partition. The Job QOS can then be thought of as the various different types of shipping options that carrier might offer. You might pay extra to have that package shipped overnight or have tracking information for it or you may choose to pay less and have your package arrive as available. Once we decide to go with a particular carrier, we are subject to their company policy, but we may negotiate how they handle that package by choosing one of their available shipping options. In the same way, when you choose to submit to a partition, you are subject to the limits enforced by the partition QOS and you may be able to ask for your job to be handled a particular way by specifying the job QOS, but that option has to be offered by the partition.
In order for a job to use a Job QOS, the user submitting the job must have access to the QOS, the account the job is being submitted to must accept the QOS, and the partition the job is being submitted to must accept the QOS. The following job QOSes every user and every account of Gautschi has access to:
- "normal": The "normal" QOS is the default job QOS on the cluster meaning if you do not explicitly list an alternative job QOS, your job will be tagged with this QOS. The policy for this QOS provides a high priority and does not add any additional limits.
- "standby": The "standby" QOS must be explicitly used if desired by using the option "-q standby" or "--qos=standby". The policy for this QOS gives access to idle resources on the cluster. Jobs tagged with this QOS are "low priority" jobs and are only allowed to run for up to four hours at a time, however the resources used by these jobs do not count against the resources in your Account. For users of our previous clusters, usage of this QOS replaces the previous -A standby style of submission.
Some of these QOSes may not be available in every partition. Each of the partitions in the following section will enumerate which of these QOSes are allowed in the partition.
Link to section 'Partitions' of 'Queues' Partitions
On Gautschi, the various types of nodes on the cluster are organized into distinct partitions. This allows CPU and GPU nodes to be charged separately and differently. This also means that Instead of only needing to specify the account name in the job script, the desired partition must also be specified. Each of these partitions is subject to different limitations and has a specific use case that will be described below.
Link to section 'CPU Partition' of 'Queues' CPU Partition
This partition contains the resources a group purchases access to when they purchase CPU resources on Gautschi and is made up of 336 Gautschi-A nodes. Each of these nodes contains two Zen 4 AMD EPYC 9654 96-core processors for a total of 192 cores and 384 GB of memory for a total of more than 64,000 cores in the partition. Memory in this partition is allocated proportional to your core request such that each core is given about 2 GB of memory per core requested. Submission to this partition can be accomplished by using the option: "-p cpu" or "--partition=cpu".
The purchasing model for this partition allows groups to purchase high priority access to some number of cores. When an account uses resources in this account by submitting a job tagged with the "normal" QOS, the cores used by that job are withdrawn from the account and deposited back into the account when the job terminates.
When using the CPU partition, jobs are tagged by the "normal" QOS by default, but they can be tagged with the "standby" QOS if explicitly submitted using the "-q standby" or "--qos=standby" option.
- Jobs tagged with the "normal" QOS are subject to the following policies:
- Jobs have a high priority and should not need to wait very long before starting.
- Any cores requested by these jobs are withdrawn from the account until the job terminates.
- These jobs can run for up to two weeks at a time.
- Jobs tagged with the "standby" QOS are subject to the following policies:
- Jobs have a low priority and there is no expectation of job start time. If the partition is very busy with jobs using the "normal" QOS or if you are requesting a very large job, then jobs using the "standby" QOS may take hours or days to start.
- These jobs can use idle resources on the cluster and as such cores requested by these jobs are not withdrawn from the account to which they were submitted.
- These jobs can run for up to four hours at a time.
Groups who purchased GPU resources on Gautschi will have the ability to run jobs in this partition tagged with the "standby" QOS described above, but will not be able to submit jobs tagged with the "normal" qos unless they have purchased CPU resources on the cluster.
Available QOSes: "normal", "standby"
Link to section 'AI Partition' of 'Queues' AI Partition
This partition contains the resources a group purchases access to when they purcahse GPU resources on Gautschi and is made up of 20 Gautschi-H nodes. Each of these nodes contains two Intel Xeon Platinum 8480+ 56-core processors and 8-way NVLinked H100s for a total of 160 H100s in the partition. CPU memory in this partition is allocated proportional to your core request such that each core is given about 9 GB of memory per core requested. Submission to this partition can be accomplished by using the option: "-p ai" or "--partition=ai".
The purchasing model for this cluster allows groups to purchase access to GPUs. When a group purchases access to a GPU for a year, they are given 365*24 hours of GPU time on the partition. The advantage to tracking resources this way is that if a group needs access to more than one GPU for a project, they can use multiple GPUs at once since instead of having to purchase additional GPUs, they just consume those GPU hours faster. When an account uses resources in this partition, the balance of GPU hours in their account is charged at a rate of at least one GPU hour for each GPU their job uses per hour (this is tracked by the minute), however the usage of some QOSes described below will charge usage at a slower rate.
When using the AI partition, jobs are tagged by the "normal" QOS by default, but they can be tagged with the "preemptible" QOS if explicitly submitted using the "-q preemptible" or "--qos=preemptible" option.
- Jobs tagged with the "normal" QOS are subject to the following policies:
- Jobs have a high priority and should not need to wait very long before starting.
- The account the job is submitted to will be charged 1 GPU hour for each GPU this job uses per hour (this is tracked by the minute).
- These jobs can run for up to two weeks at a time.
- Jobs tagged with the "preemptible" QOS subject to the following policies:
- Jobs have a low priority and there is no expectation of job start time. If the partition is very busy with jobs using the "normal" QOS or if you are requesting a very large job, then jobs using the "standby" QOS may take hours or days to start.
- The account the job is submitted to will be charged 0.25 GPU hour for each GPU this job uses per hour (this is tracked by the minute) which effectively allows jobs to use 4x as many resources as a job tagged with the "normal" qos for the same cost.
- These jobs can run for up to two weeks at a time.
- If there are not enough idle resources in this partition for a job tagged with the "normal" QOS to start, then this job may be cancelled to make room for that job. This means it is imperative to use checkpointing if using this QOS.
Available QOSes: "normal", "preemptible"
Link to section 'Highmem Partition' of 'Queues' Highmem Partition
This partition is made up of 6 Gautschi-B nodes which have four times as much memory as a standard Gautschi-A node, and access to this partition is given to all accounts on the cluster to enable work that has higher memory requirements. Each of these nodes contains two Zen 4 AMD EPYC 9654 96-core processors for a total of 192 cores and 1.5 TB of memory. Memory in this partition is allocated proportional to your core request such that each core is given about 8 GB of memory per core requested. Submission to this partition can be accomplished by using the option: "-p highmem" or "--partition=highmem".
When using the Highmem partition, jobs are tagged by the "normal" QOS by default, and this is the only QOS that is available for this partition, so there is no need to specify a QOS when using this partition. Additionally jobs are tagged by a highmem partition QOS that enforces the following policies
- There is no expectation of job start time as these nodes are a shared resources that are given as a bonus for purchasing access to high priority access to resources on Gautschi
- You can have 2 jobs running in this partition at once
- You can have 8 jobs submitted to thie partition at once
- Your jobs must use more than 48 of the 192 cores on the node otherwise your memory footprint would fit on a standard Gautschi-A node
- These jobs can run for up to 24 hours at a time.
Available QOSes: "normal"
Link to section 'Profiling Partition' of 'Queues' Profiling Partition
This partition is made up of 2 Gautschi-A nodes that have hardware performance counters enabled. By enabling hardware performance counters, profiling applications such as AMD MicroProf can track certain performance criteria for execution on the CPU such as L3 cache events, speculative execution misses, etc. Due to the fact that this allows greater visibility into the execution of each process, the nodes in this partition can only be used if your job uses the entire node. Submission to this partition can be accomplished by using the option: "-p profiling" or "--partition=profiling".
When using the profiling partition, jobs are tagged by the "normal" QOS by default, and this is the only QOS that is available for this partition, so there is no need to specify a QOS when using this partition. Additionally jobs are tagged by a profiling partition QOS that enforces the following policies
- There is no expectation of job start time as these nodes are a shared resources that are given as a bonus for purchasing access to high priority access to resources on Gautschi
- You can have 1 job running in this partition at once
- If you have a single process running on one of the nodes, you must use that entire node when submitting to this partition
- These jobs can run for up to 24 hours at a time.
This is a resource that is reserved for groups profiling their applications and we monitor to ensure that this partition is not being used simply for more compute.
Available QOSes: "normal"
Link to section 'Smallgpu Partiton' of 'Queues' Smallgpu Partiton
This partition is made up of 6 Gautschi-G nodes. Each of these nodes contains two NVIDIA L40s and two Zen 4 AMD EPYC 9554 64-core processors for a total of 128 cores and 384GB of memory. Memory in this partition is allocated proportional to your core request such that each core is given about 3 GB of memory per core requested. You should request cores proportional to the number of GPUs you are using in this partition (i.e. if you only need one of the two GPUs, you should request half of the cores on the node) Submission to this partition can be accomplished by using the option: "-p smallgpu" or "--partition=smallgpu".
When using the smallgpu partition, jobs are tagged by the "normal" QOS by default, and this is the only QOS that is available for this partition, so there is no need to specify a QOS when using this partition. Additionally jobs are tagged by a smallgpu partition QOS that enforces the following policies
- There is no expectation of job start time as these nodes are a shared resources that are given as a bonus for purchasing access to high priority access to resources on Gautschi
- You can use up to 2 GPUs in this partition at once
- You can have 8 jobs submitted to thie partition at once
- These jobs can run for up to 24 hours at a time.
Available QOSes: "normal"
Job Submission Script
To submit work to a SLURM queue, you must first create a job submission file. This job submission file is essentially a simple shell script. It will set any required environment variables, load any necessary modules, create or modify files and directories, and run any applications that you need:
#!/bin/bash
# FILENAME: myjobsubmissionfile
# Loads Matlab and sets the application up
module load matlab
# Change to the directory from which you originally submitted this job.
cd $SLURM_SUBMIT_DIR
# Runs a Matlab script named 'myscript'
matlab -nodisplay -singleCompThread -r myscript
Once your script is prepared, you are ready to submit your job.
Link to section 'Job Script Environment Variables' of 'Job Submission Script' Job Script Environment Variables
Name | Description |
---|---|
SLURM_SUBMIT_DIR | Absolute path of the current working directory when you submitted this job |
SLURM_JOBID | Job ID number assigned to this job by the batch system |
SLURM_JOB_NAME | Job name supplied by the user |
SLURM_JOB_NODELIST | Names of nodes assigned to this job |
SLURM_CLUSTER_NAME | Name of the cluster executing the job |
SLURM_SUBMIT_HOST | Hostname of the system where you submitted this job |
SLURM_JOB_PARTITION | Name of the original queue to which you submitted this job |
Submitting a Job
Once you have a job submission file, you may submit this script to SLURM using the sbatch
command. SLURM will find, or wait for, available resources matching your request and run your job there.
On Gautschi, in order to submit jobs, you need to specify the partition, account and Quality of Service (QoS) name to which you want to submit your jobs. To familiarize yourself with the partitions and QoS available on Gautschi, visit Gautschi Queues and Partitons. To check the available partitions on Gautschi, you can use the showpartitions
, and to check your available accounts you can use slist
commands. Slurm uses the term "Account" with the option -A
or --account=
to specify different batch accounts, the option -p
or --partition=
to select a specific partition for job submission, and the option -q
or --qos=
.
showpartitions
Partition statistics for cluster gautschi at Fri Dec 6 05:26:30 PM EST 2024
Partition #Nodes #CPU_cores Cores_pending Job_Nodes MaxJobTime Cores Mem/Node
Name State Total Idle Total Idle Resorc Other Min Max Day-hr:mn /node (GB)
ai up 20 20 2240 2240 0 0 1 infin infinite 112 1031
cpu up 336 300 64512 57600 0 0 1 infin infinite 192 386
highmem up 6 6 1152 1152 0 0 1 infin infinite 192 1547
smallgpu up 6 6 768 768 0 0 1 infin infinite 128 386
profiling up 2 2 384 384 0 0 1 infin infinite 192 386
Link to section 'CPU Partition' of 'Submitting a Job' CPU Partition
The CPU partition on Gautschi has two Quality of Service (QoS) levels: normal and standby. To submit your job to one compute node on cpu
partition and 'normal' QoS which has "high priority":
$ sbatch --nodes=1 --ntasks=1 --partition=cpu --account=accountname --qos=normal myjobsubmissionfile
$ sbatch -N1 -n1 -p cpu -A accountname -q normal myjobsubmissionfile
cpu
partition and 'standby' QoS which is has "low priority":
$ sbatch --nodes=1 --ntasks=1 --partition=cpu --account=accountname --qos=standby myjobsubmissionfile
$ sbatch -N1 -n1 -p cpu -A accountname -q standby myjobsubmissionfile
Link to section ' AI Partition' of 'Submitting a Job' AI Partition
The CPU partition on Gautschi has two Quality of Service (QoS) levels: normal and preemptible. To submit your job to one compute node requesting one GPU on ai
partition and 'normal' QoS which is has "high priority":
$ sbatch --nodes=1 --gpus-per-node=1 --ntasks=14 --partition=ai --account=accountname --qos=normal myjobsubmissionfile
$ sbatch -N1 --gpus-per-node=1 -n14 -p ai -A accountname -q normal myjobsubmissionfile
ai
partition and 'preemptible' QoS which has "high priority":
$ sbatch --nodes=1 --gpus-per-node=1 --ntasks=14 --partition=ai --account=accountname --qos=preemptible myjobsubmissionfile
$ sbatch -N1 --gpus-per-node=1 -n14 -p ai -A accountname -q preemptible myjobsubmissionfile
Link to section 'Highmem Partition' of 'Submitting a Job' Highmem Partition
To submit your job to a compute node on the highmem partition, you don’t need to specify the QoS name because only one QoS exists for this partition, and the default is normal. However, the highmem partition is suitable for jobs with memory requirements that exceed the capacity of a standard node, so the number of requested tasks should be appropriately high.
Link to section 'Profiling Partition' of 'Submitting a Job' Profiling Partition
To submit your job to a compute node on the profiling partition, you also don’t need to specify the QoS name because only one QoS exists for this partition, and the default is normal.
$ sbatch --nodes=1 --ntasks=1 --partition=profiling --account=accountname myjobsubmissionfile
$ sbatch -N1 -n1 -p profiling -A accountname myjobsubmissionfile
Link to section 'Smallgpu Partition' of 'Submitting a Job' Smallgpu Partition
To submit your job to a compute node on the smallgpu partition, you don’t need to specify the QoS name because only one QoS exists for this partition, and the default is normal. You should request cores proportional to the number of GPUs you are using in this partition (i.e. if you only need one of the two GPUs, you should request half of the cores on the node).
$ sbatch --nodes=1 --ntasks=64 --gpus-per-node=1 --partition=smallgpu --account=accountname myjobsubmissionfile
$ sbatch -N1 -n64 --gpus-per-node=1 -p smallgpu -A accountname myjobsubmissionfile
Link to section 'General Information' of 'Submitting a Job' General Information
By default, each job receives 30 minutes of wall time, or clock time. If you know that your job will not need more than a certain amount of time to run, request less than the maximum wall time, as this may allow your job to run sooner. To request the 1 hour and 30 minutes of wall time:
$ sbatch -t 01:30:00 -N=1 -n=1 -p=cpu -A=accountname -q=standby myjobsubmissionfile
The --nodes=
or -N
value indicates how many compute nodes you would like for your job, and --ntasks=
or -n
value indicates the number of tasks you want to run.
In some cases, you may want to request multiple nodes. To utilize multiple nodes, you will need to have a program or code that is specifically programmed to use multiple nodes such as with MPI. Simply requesting more nodes will not make your work go faster. Your code must support this ability.
To request 2 compute nodes:
$ sbatch -t 01:30:00 -N=2 -n=16 -p=cpu -A=accountname -q=standby myjobsubmissionfile
By default, jobs on Gautschi will share nodes with other jobs.
If more convenient, you may also specify any command line options to sbatch
from within your job submission file, using a special form of comment:
#!/bin/sh -l
# FILENAME: myjobsubmissionfile
#SBATCH --account=accountname
#SBATCH --nodes=1
#SBATCH --ntasks=1
#SBATCH --partition=cpu
#SBATCH --qos=normal
#SBATCH --time=1:30:00
#SBATCH --job-name myjobname
# Print the hostname of the compute node on which this job is running.
/bin/hostname
If an option is present in both your job submission file and on the command line, the option on the command line will take precedence.
After you submit your job with SBATCH
, it may wait in queue for minutes, hours, or even weeks. How long it takes for a job to start depends on the specific queue, the resources and time requested, and other jobs already waiting in that queue requested as well. It is impossible to say for sure when any given job will start. For best results, request no more resources than your job requires.
Once your job is submitted, you can monitor the job status, wait for the job to complete, and check the job output.
Checking Job Status
Once a job is submitted there are several commands you can use to monitor the progress of the job.
To see your jobs, use the squeue -u command and specify your username:
(Remember, in our SLURM environment a queue is referred to as an 'Account')
squeue -u myusername
JOBID USER ACCOUNT PART QOS NAME NODES TRES_PER_NODE CPUS TIME_LIMIT ST TIME
182792 myusername account_name cpu standby job1 1 N/A 192 4:00:00 R 35:54
185841 myusername account_name cpu normal job2 1 N/A 192 4:00:00 R 36:51
185844 myusername account_name smal normal job3 1 gres/gpu:1 64 8:00:00 R 22:11
To retrieve useful information about your queued or running job, use the scontrol show job command with your job's ID number. The output should look similar to the following:
scontrol show job 3519
JobId=3519 JobName=job.sub
UserId=myusername GroupId=mygroup MCS_label=N/A
Priority=1000 Nice=0 Account=myaccount QOS=normal
JobState=RUNNING Reason=None Dependency=(null)
Requeue=1 Restarts=0 BatchFlag=0 Reboot=0 ExitCode=0:0
RunTime=00:00:23 TimeLimit=04:00:00 TimeMin=N/A
SubmitTime=2025-02-24T14:31:19 EligibleTime=2025-02-24T14:31:19
AccrueTime=2025-02-24T14:31:19
StartTime=2025-02-24T14:32:36 EndTime=2025-02-24T18:32:36 Deadline=N/A
PreemptEligibleTime=2025-02-24T14:32:36 PreemptTime=None
SuspendTime=None SecsPreSuspend=0 LastSchedEval=2025-02-24T14:32:36 Scheduler=Backfill
Partition=cpu AllocNode:Sid=login02:4061246
ReqNodeList=(null) ExcNodeList=(null)
NodeList=a166
BatchHost=a166
NumNodes=1 NumCPUs=4 NumTasks=4 CPUs/Task=1 ReqB:S:C:T=0:0:*:*
ReqTRES=cpu=4,mem=7668M,node=1,gres/hp_cpu=4
AllocTRES=cpu=4,mem=7668M,node=1,gres/hp_cpu=4
Socks/Node=* NtasksPerN:B:S:C=0:0:*:* CoreSpec=*
MinCPUsNode=1 MinMemoryCPU=1917M MinTmpDiskNode=0
Features=(null) DelayBoot=00:00:00
OverSubscribe=OK Contiguous=0 Licenses=(null) Network=(null)
Command=/bin/sh
WorkDir=/home/myusername
TresPerJob=gres/hp_cpu=4
There are several useful bits of information in this output.
JobState
lets you know if the job is Pending, Running, Completed, or Held.RunTime and TimeLimit
will show how long the job has run and its maximum time.SubmitTime
is when the job was submitted to the cluster.NumNodes
,NumCPUs
,NumTasks
andCPUs/Task
are the number of Nodes, CPUs, Tasks, and CPUs per Task are shown.WorkDir
is the job's working directory.
Checking Job Output
Once a job is submitted, and has started, it will write its standard output and standard error to files that you can read.
SLURM catches output written to standard output and standard error - what would be printed to your screen if you ran your program interactively. Unless you specfied otherwise, SLURM will put the output in the directory where you submitted the job in a file named slurm-
followed by the job id
, with the extension out
. For example slurm-3509.out
. Note that both stdout and stderr will be written into the same file, unless you specify otherwise.
If your program writes its own output files, those files will be created as defined by the program. This may be in the directory where the program was run, or may be defined in a configuration or input file. You will need to check the documentation for your program for more details.
Link to section 'Redirecting Job Output' of 'Checking Job Output' Redirecting Job Output
It is possible to redirect job output to somewhere other than the default location with the --error
and --output
directives:
#!/bin/bash
#SBATCH --output=/home/myusername/joboutput/myjob.out
#SBATCH --error=/home/myusername/joboutput/myjob.out
# This job prints "Hello World" to output and exits
echo "Hello World"
Holding a Job
Sometimes you may want to submit a job but not have it run just yet. You may be wanting to allow lab mates to cut in front of you in the queue - so hold the job until their jobs have started, and then release yours.
To place a hold on a job before it starts running, use the scontrol hold job command:
$ scontrol hold job myjobid
Once a job has started running it can not be placed on hold.
To release a hold on a job, use the scontrol release job command:
$ scontrol release job myjobid
You find the job ID using the squeue command as explained in the SLURM Job Status section.
Job Dependencies
Dependencies are an automated way of holding and releasing jobs. Jobs with a dependency are held until the condition is satisfied. Once the condition is satisfied jobs only then become eligible to run and must still queue as normal.
Job dependencies may be configured to ensure jobs start in a specified order. Jobs can be configured to run after other job state changes, such as when the job starts or the job ends.
These examples illustrate setting dependencies in several ways. Typically dependencies are set by capturing and using the job ID from the last job submitted.
To run a job after job myjobid has started:
sbatch --dependency=after:myjobid myjobsubmissionfile
To run a job after job myjobid ends without error:
sbatch --dependency=afterok:myjobid myjobsubmissionfile
To run a job after job myjobid ends with errors:
sbatch --dependency=afternotok:myjobid myjobsubmissionfile
To run a job after job myjobid ends with or without errors:
sbatch --dependency=afterany:myjobid myjobsubmissionfile
To set more complex dependencies on multiple jobs and conditions:
sbatch --dependency=after:myjobid1:myjobid2:myjobid3,afterok:myjobid4 myjobsubmissionfile
Canceling a Job
To stop a job before it finishes or remove it from a queue, use the scancel command:
scancel myjobid
You find the job ID using the squeue command as explained in the SLURM Job Status section.
Example Jobs
A number of example jobs are available for you to look over and adapt to your own needs. The first few are generic examples, and latter ones go into specifics for particular software packages.
Generic SLURM Jobs
The following examples demonstrate the basics of SLURM jobs, and are designed to cover common job request scenarios. These example jobs will need to be modified to run your application or code.
Simple Job
Every SLURM job consists of a job submission file. A job submission file contains a list of commands that run your program and a set of resource (nodes, walltime, queue) requests. The resource requests can appear in the job submission file or can be specified at submit-time as shown below.
This simple example submits the job submission file hello.sub to the cpu queue on Gautschi and requests a single node:
#!/bin/bash
# FILENAME: hello.sub
# Show this ran on a compute node by running the hostname command.
hostname
echo "Hello World"
sbatch -A myallocation -p queue-name --nodes=1 --ntasks=1 --cpus-per-task=1 --time=00:01:00 hello.sub
Submitted batch job 3521
For a real job you would replace echo "Hello World"
with a command, or sequence of commands, that run your program.
After your job finishes running, the ls command will show a new file in your directory, the .out file:
ls -l
hello.sub
slurm-3521.out
The file slurm-3521.out contains the output and errors your program would have written to the screen if you had typed its commands at a command prompt:
cat slurm-3521.out
gautschi-a001.rcac.purdue.edu
Hello World
You should see the hostname of the compute node your job was executed on. Following should be the "Hello World" statement.
Multiple Node
In some cases, you may want to request multiple nodes. To utilize multiple nodes, you will need to have a program or code that is specifically programmed to use multiple nodes such as with MPI. Simply requesting more nodes will not make your work go faster. Your code must support this ability.
This example shows a request for multiple compute nodes. The job submission file contains a single command to show the names of the compute nodes allocated:
# FILENAME: myjobsubmissionfile.sub
#!/bin/bash
echo "$SLURM_JOB_NODELIST"
sbatch --nodes=2 --ntasks=384 --time=00:10:00 -A myallocation -p queue-name myjobsubmissionfile.sub
Compute nodes allocated:
gautschi-a[014-015]
The above example will allocate the total of 384 CPU cores across 2 nodes. Note that if your multi-node job requests fewer than each node's full 192 cores per node, by default Slurm provides no guarantee with respect to how this total is distributed between assigned nodes (i.e. the cores may not necessarily be split evenly). If you need specific arrangements of your tasks and cores, you can use --cpus-per-task= and/or --ntasks-per-node= flags. See Slurm documentation or man sbatch for more options.
Directives
So far these examples have shown submitting jobs with the resource requests on the sbatch
command line such as:
sbatch -A myallocation -p queue-name --nodes=1 --time=00:01:00 hello.sub
The resource requests can also be put into job submission file itself. Documenting the resource requests in the job submission is desirable because the job can be easily reproduced later. Details left in your command history are quickly lost. Arguments are specified with the #SBATCH
syntax:
#!/bin/bash # FILENAME: hello.sub
#SBATCH -A myallocation -p queue-name #SBATCH --nodes=1 --time=00:01:00 # Show this ran on a compute node by running the hostname command. hostname echo "Hello World"
The #SBATCH
directives must appear at the top of your submission file. SLURM will stop parsing directives as soon as it encounters a line that does not start with '#'. If you insert a directive in the middle of your script, it will be ignored.
This job can be then submitted with:
sbatch hello.sub
Interactive Jobs
Interactive jobs are run on compute nodes, while giving you a shell to interact with. They give you the ability to type commands or use a graphical interface in the same way as if you were on a front-end login host.
To submit an interactive job, use sinteractive to run a login shell on allocated resources.
sinteractive accepts most of the same resource requests as sbatch, so to request a login shell on the cpu account while allocating 2 nodes and 192 total cores, you might do:
sinteractive -A myallocation -p cpu -N2 -n384
To quit your interactive job:
exit
or Ctrl-D
The above example will allocate the total of 384 CPU cores across 2 nodes. Note that if your multi-node job requests fewer than each node's full 192 cores per node, by default Slurm provides no guarantee with respect to how this total is distributed between assigned nodes (i.e. the cores may not necessarily be split evenly). If you need specific arrangements of your tasks and cores, you can use --cpus-per-task= and/or --ntasks-per-node= flags. See Slurm documentation or man salloc for more options.
Serial Jobs
This shows how to submit one of the serial programs compiled in the section Compiling Serial Programs.
Create a job submission file:
#!/bin/bash
# FILENAME: serial_hello.sub
./serial_hello
Submit the job:
sbatch --nodes=1 --ntasks=1 --time=00:01:00 serial_hello.sub
After the job completes, view results in the output file:
cat slurm-myjobid.out
Runhost:gautschi-a009.rcac.purdue.edu
hello, world
If the job failed to run, then view error messages in the file slurm-myjobid.out.
Link to section 'Collecting System Resource Utilization Data' of 'Monitoring Resources' Collecting System Resource Utilization Data
Knowing the precise resource utilization an application had during a job, such as CPU load or memory, can be incredibly useful. This is especially the case when the application isn't performing as expected.
One approach is to run a program like htop
during an interactive job and keep an eye on system resources. You can get precise time-series data from nodes associated with your job using XDmod as well, online. But these methods don't gather telemetry in an automated fashion, nor do they give you control over the resolution or format of the data.
As a matter of course, a robust implementation of some HPC workload would include resource utilization data as a diagnostic tool in the event of some failure.
The monitor
utility is a simple command line system resource monitoring tool for gathering such telemetry and is available as a module.
module load monitor
Complete documentation is available online at resource-monitor.readthedocs.io. A full manual page is also available for reference, man monitor
.
In the context of a SLURM job you will need to put this monitoring task in the background to allow the rest of your job script to proceed. Be sure to interrupt these tasks at the end of your job.
#!/bin/bash
# FILENAME: monitored_job.sh
module load monitor
# track per-code CPU load
monitor cpu percent --all-cores >cpu-percent.log &
CPU_PID=$!
# track memory usage
monitor cpu memory >cpu-memory.log &
MEM_PID=$!
# your code here
# shut down the resource monitors
kill -s INT $CPU_PID $MEM_PID
A particularly elegant solution would be to include such tools in your prologue script and have the tear down in your epilogue script.
For large distributed jobs spread across multiple nodes, mpiexec
can be used to gather telemetry from all nodes in the job. The hostname is included in each line of output so that data can be grouped as such. A concise way of constructing the needed list of hostnames in SLURM is to simply use srun hostname | sort -u
.
#!/bin/bash
# FILENAME: monitored_job.sh
module load monitor
# track all CPUs (one monitor per host)
mpiexec -machinefile <(srun hostname | sort -u) \
monitor cpu percent --all-cores >cpu-percent.log &
CPU_PID=$!
# track memory on all hosts (one monitor per host)
mpiexec -machinefile <(srun hostname | sort -u) \
monitor cpu memory >cpu-memory.log &
MEM_PID=$!
# your code here
# shut down the resource monitors
kill -s INT $CPU_PID $MEM_PID
To get resource data in a more readily computable format, the monitor
program can be told to output in CSV format with the --csv
flag.
monitor cpu memory --csv >cpu-memory.csv
For a distributed job you will need to suppress the header lines otherwise one will be created by each host.
monitor cpu memory --csv | head -1 >cpu-memory.csv
mpiexec -machinefile <(srun hostname | sort -u) \
monitor cpu memory --csv --no-header >>cpu-memory.csv
Specific Applications
The following examples demonstrate job submission files for some common real-world applications. See the Generic SLURM Examples section for more examples on job submissions that can be adapted for use.
Python
Notice: Python 2.7 has reached end-of-life on Jan 1, 2020 (announcement). Please update your codes and your job scripts to use Python 3.
Python is a high-level, general-purpose, interpreted, dynamic programming language. We suggest using Anaconda which is a Python distribution made for large-scale data processing, predictive analytics, and scientific computing. For example, to use the default Anaconda distribution:
$ module load conda
For a full list of available Anaconda and Python modules enter:
$ module spider conda
Example Python Jobs
This section illustrates how to submit a small Python job to a SLURM queue.
Link to section 'Example 1: Hello world' of 'Example Python Jobs' Example 1: Hello world
Prepare a Python input file with an appropriate filename, here named hello.py
:
# FILENAME: hello.py
import string, sys
print("Hello, world!")
Prepare a job submission file with an appropriate filename, here named myjob.sub:
#!/bin/bash
# FILENAME: myjob.sub
module load conda
python hello.py
Hello, world!
Link to section 'Example 2: Matrix multiply' of 'Example Python Jobs' Example 2: Matrix multiply
Save the following script as matrix.py:
# Matrix multiplication program
x = [[3,1,4],[1,5,9],[2,6,5]]
y = [[3,5,8,9],[7,9,3,2],[3,8,4,6]]
result = [[sum(a*b for a,b in zip(x_row,y_col)) for y_col in zip(*y)] for x_row in x]
for r in result:
print(r)
Change the last line in the job submission file above to read:
python matrix.py
The standard output file from this job will result in the following matrix:
[28, 56, 43, 53]
[65, 122, 59, 73]
[63, 104, 54, 60]
Link to section 'Example 3: Sine wave plot using numpy and matplotlib packages' of 'Example Python Jobs' Example 3: Sine wave plot using numpy and matplotlib packages
Save the following script as sine.py:
import numpy as np
import matplotlib
matplotlib.use('Agg')
import matplotlib.pyplot as plt
x = np.linspace(-np.pi, np.pi, 201)
plt.plot(x, np.sin(x))
plt.xlabel('Angle [rad]')
plt.ylabel('sin(x)')
plt.axis('tight')
plt.savefig('sine.png')
Change your job submission file to submit this script and the job will output a png file and blank standard output and error files.
For more information about Python:
Managing Environments with Conda
Conda is a package manager in Anaconda that allows you to create and manage multiple environments where you can pick and choose which packages you want to use. To use Conda you must load an Anaconda module:
$ module load conda
Many packages are pre-installed in the global environment. To see these packages:
$ conda list
To create your own custom environment:
$ conda create --name MyEnvName python=3.8 FirstPackageName SecondPackageName -y
The --name option specifies that the environment created will be named MyEnvName. You can include as many packages as you require separated by a space. Including the -y option lets you skip the prompt to install the package. By default environments are created and stored in the $HOME/.conda directory.
To create an environment at a custom location:
$ conda create --prefix=$HOME/MyEnvName python=3.8 PackageName -y
To see a list of your environments:
$ conda env list
To remove unwanted environments:
$ conda remove --name MyEnvName --all
To add packages to your environment:
$ conda install --name MyEnvName PackageNames
To remove a package from an environment:
$ conda remove --name MyEnvName PackageName
Installing packages when creating your environment, instead of one at a time, will help you avoid dependency issues.
To activate or deactivate an environment you have created:
$ source activate MyEnvName
$ source deactivate MyEnvName
If you created your conda environment at a custom location using --prefix option, then you can activate or deactivate it using the full path.
$ source activate $HOME/MyEnvName
$ source deactivate $HOME/MyEnvName
To use a custom environment inside a job you must load the module and activate the environment inside your job submission script. Add the following lines to your submission script:
$ module load conda
$ source activate MyEnvName
For more information about Python:
Installing Packages
Installing Python packages in an Anaconda environment is recommended. One key advantage of Anaconda is that it allows users to install unrelated packages in separate self-contained environments. Individual packages can later be reinstalled or updated without impacting others. If you are unfamiliar with Conda environments, please check our Conda Guide.
To facilitate the process of creating and using Conda environments, we support a script (conda-env-mod) that generates a module file for an environment, as well as an optional Jupyter kernel to use this environment in a JupyterHub notebook.
You must load one of the anaconda modules in order to use this script.
$ module load conda
Step-by-step instructions for installing custom Python packages are presented below.
Link to section 'Step 1: Create a conda environment' of 'Installing Packages' Step 1: Create a conda environment
Users can use the conda-env-mod script to create an empty conda environment. This script needs either a name or a path for the desired environment. After the environment is created, it generates a module file for using it in future. Please note that conda-env-mod is different from the official conda-env script and supports a limited set of subcommands. Detailed instructions for using conda-env-mod can be found with the command conda-env-mod --help.
-
Example 1: Create a conda environment named mypackages in user's
$HOME
directory.$ conda-env-mod create -n mypackages
-
Example 2: Create a conda environment named mypackages at a custom location.
$ conda-env-mod create -p /depot/mylab/apps/mypackages
Please follow the on-screen instructions while the environment is being created. After finishing, the script will print the instructions to use this environment.
... ... ... Preparing transaction: ...working... done Verifying transaction: ...working... done Executing transaction: ...working... done +------------------------------------------------------+ | To use this environment, load the following modules: | | module load use.own | | module load conda-env/mypackages-py3.8.5 | +------------------------------------------------------+ Your environment "mypackages" was created successfully.
Note down the module names, as you will need to load these modules every time you want to use this environment. You may also want to add the module load lines in your jobscript, if it depends on custom Python packages.
By default, module files are generated in your $HOME/privatemodules directory. The location of module files can be customized by specifying the -m /path/to/modules option to conda-env-mod.
Note: The main differences between -p and -m are: 1) -p will change the location of packages to be installed for the env and the module file will still be located at the $HOME/privatemodules directory as defined in use.own. 2) -m will only change the location of the module file. So the method to load modules created with -m and -p are different, see Example 3 for details.
- Example 3: Create a conda environment named labpackages in your group's Data Depot space and place the module file at a shared location for the group to use.
$ conda-env-mod create -p /depot/mylab/apps/labpackages -m /depot/mylab/etc/modules ... ... ... Preparing transaction: ...working... done Verifying transaction: ...working... done Executing transaction: ...working... done +-------------------------------------------------------+ | To use this environment, load the following modules: | | module use /depot/mylab/etc/modules | | module load conda-env/labpackages-py3.8.5 | +-------------------------------------------------------+ Your environment "labpackages" was created successfully.
If you used a custom module file location, you need to run the module use command as printed by the command output above.
By default, only the environment and a module file are created (no Jupyter kernel). If you plan to use your environment in a JupyterHub notebook, you need to append a --jupyter flag to the above commands.
- Example 4: Create a Jupyter-enabled conda environment named labpackages in your group's Data Depot space and place the module file at a shared location for the group to use.
$ conda-env-mod create -p /depot/mylab/apps/labpackages -m /depot/mylab/etc/modules --jupyter ... ... ... Jupyter kernel created: "Python (My labpackages Kernel)" ... ... ... Your environment "labpackages" was created successfully.
Link to section 'Step 2: Load the conda environment' of 'Installing Packages' Step 2: Load the conda environment
-
The following instructions assume that you have used conda-env-mod script to create an environment named mypackages (Examples 1 or 2 above). If you used conda create instead, please use conda activate mypackages.
$ module load use.own $ module load conda-env/mypackages-py3.8.5
Note that the conda-env module name includes the Python version that it supports (Python 3.8.5 in this example). This is same as the Python version in the conda module.
-
If you used a custom module file location (Example 3 above), please use module use to load the conda-env module.
$ module use /depot/mylab/etc/modules $ module load conda-env/labpackages-py3.8.5
Link to section 'Step 3: Install packages' of 'Installing Packages' Step 3: Install packages
Now you can install custom packages in the environment using either conda install or pip install.
Link to section 'Installing with conda' of 'Installing Packages' Installing with conda
-
Example 1: Install OpenCV (open-source computer vision library) using conda.
$ conda install opencv
-
Example 2: Install a specific version of OpenCV using conda.
$ conda install opencv=4.5.5
-
Example 3: Install OpenCV from a specific anaconda channel.
$ conda install -c anaconda opencv
Link to section 'Installing with pip' of 'Installing Packages' Installing with pip
-
Example 4: Install pandas using pip.
$ pip install pandas
-
Example 5: Install a specific version of pandas using pip.
$ pip install pandas==1.4.3
Follow the on-screen instructions while the packages are being installed. If installation is successful, please proceed to the next section to test the packages.
Note: Do NOT run Pip with the --user argument, as that will install packages in a different location and might mess up your account environment.
Link to section 'Step 4: Test the installed packages' of 'Installing Packages' Step 4: Test the installed packages
To use the installed Python packages, you must load the module for your conda environment. If you have not loaded the conda-env module, please do so following the instructions at the end of Step 1.
$ module load use.own
$ module load conda-env/mypackages-py3.8.5
- Example 1: Test that OpenCV is available.
$ python -c "import cv2; print(cv2.__version__)"
- Example 2: Test that pandas is available.
$ python -c "import pandas; print(pandas.__version__)"
If the commands finished without errors, then the installed packages can be used in your program.
Link to section 'Additional capabilities of conda-env-mod script' of 'Installing Packages' Additional capabilities of conda-env-mod script
The conda-env-mod tool is intended to facilitate creation of a minimal Anaconda environment, matching module file and optionally a Jupyter kernel. Once created, the environment can then be accessed via familiar module load command, tuned and expanded as necessary. Additionally, the script provides several auxiliary functions to help manage environments, module files and Jupyter kernels.
General usage for the tool adheres to the following pattern:
$ conda-env-mod help
$ conda-env-mod <subcommand> <required argument> [optional arguments]
where required arguments are one of
- -n|--name ENV_NAME (name of the environment)
- -p|--prefix ENV_PATH (location of the environment)
and optional arguments further modify behavior for specific actions (e.g. -m to specify alternative location for generated module files).
Given a required name or prefix for an environment, the conda-env-mod script supports the following subcommands:
- create - to create a new environment, its corresponding module file and optional Jupyter kernel.
- delete - to delete existing environment along with its module file and Jupyter kernel.
- module - to generate just the module file for a given existing environment.
- kernel - to generate just the Jupyter kernel for a given existing environment (note that the environment has to be created with a --jupyter option).
- help - to display script usage help.
Using these subcommands, you can iteratively fine-tune your environments, module files and Jupyter kernels, as well as delete and re-create them with ease. Below we cover several commonly occurring scenarios.
Note: When you try to use conda-env-mod delete, remember to include the arguments as you create the environment (i.e. -p package_location and/or -m module_location).
Link to section 'Generating module file for an existing environment' of 'Installing Packages' Generating module file for an existing environment
If you already have an existing configured Anaconda environment and want to generate a module file for it, follow appropriate examples from Step 1 above, but use the module subcommand instead of the create one. E.g.
$ conda-env-mod module -n mypackages
and follow printed instructions on how to load this module. With an optional --jupyter flag, a Jupyter kernel will also be generated.
Note that the module name mypackages should be exactly the same with the older conda environment name. Note also that if you intend to proceed with a Jupyter kernel generation (via the --jupyter flag or a kernel subcommand later), you will have to ensure that your environment has ipython and ipykernel packages installed into it. To avoid this and other related complications, we highly recommend making a fresh environment using a suitable conda-env-mod create .... --jupyter command instead.
Link to section 'Generating Jupyter kernel for an existing environment' of 'Installing Packages' Generating Jupyter kernel for an existing environment
If you already have an existing configured Anaconda environment and want to generate a Jupyter kernel file for it, you can use the kernel subcommand. E.g.
$ conda-env-mod kernel -n mypackages
This will add a "Python (My mypackages Kernel)" item to the dropdown list of available kernels upon your next login to the JupyterHub.
Note that generated Jupiter kernels are always personal (i.e. each user has to make their own, even for shared environments). Note also that you (or the creator of the shared environment) will have to ensure that your environment has ipython and ipykernel packages installed into it.
Link to section 'Managing and using shared Python environments' of 'Installing Packages' Managing and using shared Python environments
Here is a suggested workflow for a common group-shared Anaconda environment with Jupyter capabilities:
The PI or lab software manager:
-
Creates the environment and module file (once):
$ module purge $ module load conda $ conda-env-mod create -p /depot/mylab/apps/labpackages -m /depot/mylab/etc/modules --jupyter
-
Installs required Python packages into the environment (as many times as needed):
$ module use /depot/mylab/etc/modules $ module load conda-env/labpackages-py3.8.5 $ conda install ....... # all the necessary packages
Lab members:
-
Lab members can start using the environment in their command line scripts or batch jobs simply by loading the corresponding module:
$ module use /depot/mylab/etc/modules $ module load conda-env/labpackages-py3.8.5 $ python my_data_processing_script.py .....
-
To use the environment in Jupyter notebooks, each lab member will need to create his/her own Jupyter kernel (once). This is because Jupyter kernels are private to individuals, even for shared environments.
$ module use /depot/mylab/etc/modules $ module load conda-env/labpackages-py3.8.5 $ conda-env-mod kernel -p /depot/mylab/apps/labpackages
A similar process can be devised for instructor-provided or individually-managed class software, etc.
Link to section 'Troubleshooting' of 'Installing Packages' Troubleshooting
- Python packages often fail to install or run due to dependency incompatibility with other packages. More specifically, if you previously installed packages in your home directory it is safer to clean those installations.
$ mv ~/.local ~/.local.bak $ mv ~/.cache ~/.cache.bak
- Unload all the modules.
$ module purge
- Clean up PYTHONPATH.
$ unset PYTHONPATH
- Next load the modules (e.g. anaconda) that you need.
$ module load conda/2024.02-py311 $ module load use.own $ module load conda-env/2024.02-py311
- Now try running your code again.
- Few applications only run on specific versions of Python (e.g. Python 3.6). Please check the documentation of your application if that is the case.
Installing Packages from Source
We maintain several Anaconda installations. Anaconda maintains numerous popular scientific Python libraries in a single installation. If you need a Python library not included with normal Python we recommend first checking Anaconda. For a list of modules currently installed in the Anaconda Python distribution:
$ module load conda
$ conda list
# packages in environment at /apps/spack/bell/apps/anaconda/2020.02-py37-gcc-4.8.5-u747gsx:
#
# Name Version Build Channel
_ipyw_jlab_nb_ext_conf 0.1.0 py37_0
_libgcc_mutex 0.1 main
alabaster 0.7.12 py37_0
anaconda 2020.02 py37_0
...
If you see the library in the list, you can simply import it into your Python code after loading the Anaconda module.
If you do not find the package you need, you should be able to install the library in your own Anaconda customization. First try to install it with Conda or Pip. If the package is not available from either Conda or Pip, you may be able to install it from source.
Use the following instructions as a guideline for installing packages from source. Make sure you have a download link to the software (usually it will be a tar.gz
archive file). You will substitute it on the wget line below.
We also assume that you have already created an empty conda environment as described in our Python package installation guide.
$ mkdir ~/src
$ cd ~/src
$ wget http://path/to/source/tarball/app-1.0.tar.gz
$ tar xzvf app-1.0.tar.gz
$ cd app-1.0
$ module load conda
$ module load use.own
$ module load conda-env/mypackages-py3.8.5
$ python setup.py install
$ cd ~
$ python
>>> import app
>>> quit()
The "import app" line should return without any output if installed successfully. You can then import the package in your python scripts.
If you need further help or run into any issues installing a library, contact us or drop by Coffee Hour for in-person help.
For more information about Python:
Link to section 'Distributed Training on RCAC Clusters' of 'Distributed Training' Distributed Training on RCAC Clusters
This page aims to provide users of RCAC resources a high-level overview of how distributed training works, along with complete working examples to serve as guides. This guide will cover the following:
- Single node DDP in PyTorch
- Multi-Node DDP in PyTorch
Link to section 'Single Node Distributed Data Parallel' of 'Distributed Training' Single Node Distributed Data Parallel
We will first focus on single node example of Distributed Data Parallelism (DDP) using 8 GPUs for an example mnist classification task. DDP aims to speed up training by training the model on multiple GPUs in parallel. Each instance of the model will receive its own batch of data, and compute its own gradients.
Importantly, before the update step, the gradients across each instance of the model will be accumulated and averaged. This prevents the weights of each of the models from diverging over time.
In this example, we will use torchrun
to spawn 8 processes on the node (one for each GPU). Each GPU will receive its own copy of the model, along with its own batch from the dataloader. Pytorch provides us with a comprehensive set of tooling to orchestrate the necessary environment and communications between all of the different processes. We can visualize the setup below. Each process (rank) on the node has its own GPU, with a complete copy of the model.
Link to section 'Slurm Script' of 'Distributed Training' Slurm Script
In the Slurm script, we are requesting a single Gautschi H node (with all 8 H100 GPUs) with a single task. Although we are only requesting a single task, 8 separate processes will be spawned by this task for distributed training.
Before we start training, we must specify the master port and address that will be used for rendezvous communications. This is where all of the individual processes sync up for communication. The Hostname will be formatted like h012.gautschi.rcac.purdue.edu
, since this job landed on the h012
node.
In the last line, torchrun
is ran only once, and is responsible for managing the spawning of processes (one for each GPU on this node), as well as setting environment variables that will be used within each process for establishing communication:
RANK, LOCAL_RANK, WORLD_SIZE, MASTER_ADDR, MASTER_PORT
Here, the rank (and local rank, which is more relevant for multi-node training, see below), world size, and rendezvous backend and location are all set.
#!/bin/bash
#SBATCH --job-name=ddp_singlenode
#SBATCH --nodes=1
#SBATCH --ntasks-per-node=1
#SBATCH --gpus-per-node=8
#SBATCH --cpus-per-task=14
#SBATCH --time=00:20:00
#SBATCH --constraint=H
#SBATCH --partition=ai
#SBATCH --qos=normal
#SBATCH --output=train_gautschi_singlenode.out
#SBATCH --account=rcac #Change to your account!
#Change these to match your environment!
ml conda
ml cuda
conda activate /depot/itap/user/envs/anaconda/gautschi/ddp_example
# Optional Logging (Uncomment these if you are having issues!)
# export NCCL_DEBUG=INFO
# export NCCL_DEBUG_SUBSYS=ALL
# export TORCH_CPP_LOG_LEVEL=INFO
# export TORCH_DISTRIBUTED_DEBUG=DETAIL
# Rendezvous settings (do this before so torchrun sees them)
# Will be used for establishing the rendezvous point for all processes
export MASTER_ADDR=$(hostname)
export MASTER_PORT=29500
echo "MASTER_ADDR: $MASTER_ADDR" #h012.gautschi.rcac.purdue.edu
echo "MASTER_PORT: $MASTER_PORT"
echo "SLURM_GPUS_ON_NODE: $SLURM_GPUS_PER_NODE" #8
torchrun --nproc_per_node=${SLURM_GPUS_PER_NODE} \
--rdzv_endpoint=${MASTER_ADDR}:${MASTER_PORT} \
ddp_train_gautschi.py
Link to section 'PyTorch Script' of 'Distributed Training' PyTorch Script
The Pytorch script largely looks like a standard Pytorch training script, but with modifications to allow for distributed training. With respect to DDP, the important portions of this script are as follows:
- The
dist.init_process_group(backend="nccl")
initializes the distributed process group. This allows for the collective communication across processes. Using environment variables set for each process by torchrun, it connects all processes to a rendezvous endpoint to allow for collective communication before training begins. Effectively, it ensures that all processes understand what their rank is, what all the other processes are, and that all processes are able to communicate with each other. This then allows each process to see information specific to it (such asget_rank()
). backend="nccl"
specifies the communication backend (Nvidia Collective Communications Library) for the sharing of gradients between processes.- The
sampler = DistributedSampler(train_dataset, num_replicas=world_size, rank=rank)
creates a sampler that will send different batches of your training dataset to each of the ranks (GPUs), which each contain their own copy of the model. - The
ddp_model = DDP(model, device_ids=[local_rank])
is arguably the most important portion of the code. When you wrap a pytorch model inDDP()
it transforms the model into a distributed model with all other ranks. This model will then share weighs with all other distributed models in all of the other ranks (using the nccl backend).
# (filename is ddp_train_gautschi.py)
import os
import torch
import torch.nn as nn
import torch.optim as optim
import torch.distributed as dist
from torch.nn.parallel import DistributedDataParallel as DDP
from torch.utils.data import DataLoader, DistributedSampler
from torchvision import datasets, transforms
import torch.nn.functional as F
import socket
def setup():
dist.init_process_group(backend="nccl")
def cleanup():
dist.destroy_process_group()
class MNISTModel(nn.Module):
'''This is a vanilla CNN compatible with the default mnist dataset!'''
def __init__(self):
super().__init__()
self.conv1 = nn.Conv2d(1, 32, kernel_size=3, padding=1)
self.conv2 = nn.Conv2d(32, 64, kernel_size=3, padding=1)
self.pool = nn.MaxPool2d(2)
self.fc1 = nn.Linear(64 * 7 * 7, 128)
self.fc2 = nn.Linear(128, 10)
def forward(self, x):
x = self.pool(F.relu(self.conv1(x)))
x = self.pool(F.relu(self.conv2(x)))
x = x.view(x.size(0), -1)
x = F.relu(self.fc1(x))
return self.fc2(x)
def train():
setup()
rank = dist.get_rank() #Will be the same as global rank for single-node!
world_size = dist.get_world_size() #Will be the #nodes * gpus/node!
local_rank = int(os.environ["LOCAL_RANK"]) #LOCAL_RANK managed by torchrun!
torch.cuda.set_device(local_rank) #LOCAL_RANK is just the GPU index to set!
device = torch.device("cuda", local_rank)
print(f"Rank:{rank}, local_rank:{local_rank}, hostname:{socket.gethostname()}, world_size = {world_size}", flush=True)
#Load standard mnist dataset !
transform = transforms.Compose([
transforms.ToTensor(),
transforms.Normalize((0.1307,), (0.3081,))
])
train_dataset = datasets.MNIST(root="./data", train=True, download=True, transform=transform)
#DistributedSampler will give each rank (gpu) its own batch!
sampler = DistributedSampler(train_dataset, num_replicas=world_size, rank=rank)
dataloader = DataLoader(train_dataset, batch_size=64, sampler=sampler, num_workers=4, pin_memory=True)
#Wrapping model in DDP allows for the sync of gradients across all ranks (gpus)!
model = MNISTModel().to(device)
ddp_model = DDP(model, device_ids=[local_rank])
optimizer = optim.Adam(ddp_model.parameters(), lr=0.001)
loss_fn = nn.CrossEntropyLoss()
#After that the "training loop" is basically identical to a
for epoch in range(5):
ddp_model.train()
sampler.set_epoch(epoch)
total_loss = 0.0
for batch_x, batch_y in dataloader:
batch_x = batch_x.to(device, non_blocking=True)
batch_y = batch_y.to(device, non_blocking=True)
optimizer.zero_grad()
output = ddp_model(batch_x)
loss = loss_fn(output, batch_y)
loss.backward()
optimizer.step()
total_loss += loss.item()
print(f"Rank {rank}, Epoch {epoch},Loss: {total_loss / len(dataloader):.4f}", flush=True)
print("done training", flush=True)
# Only save the model once (from the first rank!)
if rank == 0:
torch.save(ddp_model.state_dict(), "mnist_ddp_model.pth")
print("Model saved", flush=True)
cleanup()
if __name__ == "__main__":
train()
Link to section 'Output' of 'Distributed Training' Output
From the output, we see that the Python script is running 8 different times, with 8 separate ranks:
Rank:0, local_rank:0, hostname:h007.gautschi.rcac.purdue.edu, world_size = 8
Rank:2, local_rank:2, hostname:h007.gautschi.rcac.purdue.edu, world_size = 8
Rank:4, local_rank:4, hostname:h007.gautschi.rcac.purdue.edu, world_size = 8
Rank:3, local_rank:3, hostname:h007.gautschi.rcac.purdue.edu, world_size = 8
Rank:1, local_rank:1, hostname:h007.gautschi.rcac.purdue.edu, world_size = 8
Rank:7, local_rank:7, hostname:h007.gautschi.rcac.purdue.edu, world_size = 8
Rank:5, local_rank:5, hostname:h007.gautschi.rcac.purdue.edu, world_size = 8
Rank:6, local_rank:6, hostname:h007.gautschi.rcac.purdue.edu, world_size = 8
Link to section 'Multi Node Distributed Data Parallel' of 'Distributed Training' Multi Node Distributed Data Parallel
Things become a bit more complicated when we want to distribute training across multiple nodes. With a total world size of 16 (2 nodes, with 8 ranks each) each process will have a "global rank" (1-16) as well as a "local rank" on that node, which will correspond the the GPU ID:
Link to section 'Slurm Script' of 'Distributed Training' Slurm Script
In this Slurm script, we are now requesting two Gautschi H nodes (each with all 8 H100 GPUs), and specify that each node should have a single task. Again, the single task on each node will spawn 8 separate processes (corresponding to the 8 local ranks) per node.
Before we start training, we must specify the master port and address that will be used for rendezvous communications. This is where all of the individual processes sync up for communication. Since there are now multiple nodes, we must pull out the first node (from $SLURM_JOB_NODELIST
) to serve as the master.
When we run srun torchrun
here, and since we requested 1 task per node, torchrun is being ran once on each node, which in-turn spawns 8 processes (one for each rank) on each node.
#!/bin/bash
#SBATCH --job-name=ddp_multinode
#SBATCH --nodes=2
#SBATCH --ntasks-per-node=1
#SBATCH --gpus-per-node=8
#SBATCH --cpus-per-task=14
#SBATCH --time=00:05:00
#SBATCH --constraint=H
#SBATCH --partition=ai
#SBATCH --qos=normal
#SBATCH --output=train_gautschi_multinode.out
#SBATCH --account=rcac
ml conda
ml cuda
conda activate /depot/itap/user/envs/anaconda/gautschi/ddp_example
export MASTER_ADDR=$(scontrol show hostnames "$SLURM_JOB_NODELIST" | head -n1)
export MASTER_PORT=29500
srun torchrun \
--nnodes=${SLURM_NNODES} \
--node_rank=${SLURM_NODEID} \
--nproc_per_node=${SLURM_GPUS_PER_NODE} \
--rdzv_id=${SLURM_JOB_ID} \
--rdzv_backend=c10d \
--rdzv_endpoint=${MASTER_ADDR}:${MASTER_PORT} \
ddp_train_gautschi.py
Link to section 'PyTorch Script' of 'Distributed Training' PyTorch Script
Torchrun is handling all of the processes and environment variables that are needed to initiate the distributed process group, so no changes to the python script are needed!
Link to section 'Output' of 'Distributed Training' Output
From the output, we see that the Python script is now running 16 different times, with 8 processes on each node!
Rank:0, local_rank:0, hostname:h014.gautschi.rcac.purdue.edu, world_size = 16
Rank:8, local_rank:0, hostname:h016.gautschi.rcac.purdue.edu, world_size = 16
Rank:1, local_rank:1, hostname:h014.gautschi.rcac.purdue.edu, world_size = 16
Rank:3, local_rank:3, hostname:h014.gautschi.rcac.purdue.edu, world_size = 16
Rank:6, local_rank:6, hostname:h014.gautschi.rcac.purdue.edu, world_size = 16
Rank:4, local_rank:4, hostname:h014.gautschi.rcac.purdue.edu, world_size = 16
Rank:2, local_rank:2, hostname:h014.gautschi.rcac.purdue.edu, world_size = 16
Rank:7, local_rank:7, hostname:h014.gautschi.rcac.purdue.edu, world_size = 16
Rank:5, local_rank:5, hostname:h014.gautschi.rcac.purdue.edu, world_size = 16
Rank:9, local_rank:1, hostname:h016.gautschi.rcac.purdue.edu, world_size = 16
Rank:10, local_rank:2, hostname:h016.gautschi.rcac.purdue.edu, world_size = 16
Rank:15, local_rank:7, hostname:h016.gautschi.rcac.purdue.edu, world_size = 16
Rank:14, local_rank:6, hostname:h016.gautschi.rcac.purdue.edu, world_size = 16
Rank:11, local_rank:3, hostname:h016.gautschi.rcac.purdue.edu, world_size = 16
Rank:12, local_rank:4, hostname:h016.gautschi.rcac.purdue.edu, world_size = 16
Rank:13, local_rank:5, hostname:h016.gautschi.rcac.purdue.edu, world_size = 16
Link to section 'Investigating inter-process communications' of 'Distributed Training' Investigating inter-process communications
If you enabled detailed logging by uncommenting these variables:
export NCCL_DEBUG=INFO
export NCCL_DEBUG_SUBSYS=ALL
export TORCH_CPP_LOG_LEVEL=INFO
export TORCH_DISTRIBUTED_DEBUG=DETAIL
You will be able to see detailed information reported by the rendezvous and NCCL backends. (**WARNING** This will produce *very* large logs)
When doing gradient updating, you will see that NCCL is handling the synchronization of data between ranks (with the AllReduce
function):
h015:2073494:2073958 [2] NCCL INFO AllReduce: opCount 1e7 sendbuff 0x145d0be7e400 recvbuff 0x145d0be7e400 count 18816 datatype 7 op 0 root 0 comm 0x55956223bb90 [nranks=16] stream 0x55956223a9a0
You can also see that each of the ranks are frequently accessing the rendezvous backend (c10d
) for getting and setting information about other ranks:
[I521 16:05:33.213697937 TCPStoreLibUvBackend.cpp:827] [c10d - trace] set key:/default_pg/0//cuda//NCCL_4_trace_start address:[h005.gautschi.rcac.purdue.edu]:57348
Link to section 'FAQ' of 'Distributed Training' FAQ
- Is the
NCCL
"process group" backend different than the "rendezvous" backend? What's the difference?- The rendezvous backend (typically
c10d
) is what is used to allow different ranks to find, discover, and communicate with each other. Under the hood, it's effectively just a TCPStore key-value server that all ranks have access to. In the logs, you'll see each rank is often getting and setting values, which will be visible to all other ranks.
- The rendezvous backend (typically
* The NCCL
(Nvidia Collective Communications Library) process group backend is what is facilitating distributed operations of for GPUs. For example, NCCL
is handling the AllReduce
function, which is averaging gradients across GPUs when backpropgating in a DDP() model. There are other process group backends (gloo
, mpi
) but nccl
is strongly recommended for use with Nvidia GPUs.
- Do I need to use
torchrun
? What exactly istorchrun
doing?- You don't necessarily need to use
torchrun
, and could launch all processes withsrun
, or even manually. However, you'll need to ensure that the environment for each rank are set up properly, which would be tedious and error-prone without torchrun.
- You don't necessarily need to use
- How can I ensure all of the individual processes are synced?
- In the
setup()
function, you can add atorch.distributed.barrier()
line, which will prevent all processes from continuing until all have reached the barrier.
- In the
- Can I run distributed training in a container?
- Yes! You can use NGC (Nvidia GPU Cloud) containers to perform distributed training. Running with singularity may look something like this:
singularity run --nv /apps/ngc/images/nvcr.io_nvidia_pytorch:25.01-py3.sif \
torchrun --nproc_per_node=$SLURM_GPUS_PER_NODE \
--rdzv-backend=c10d \
--rdzv-endpoint=$MASTER_ADDR:$MASTER_PORT \
ddp_train_gautschi.py
R
R, a GNU project, is a language and environment for data manipulation, statistics, and graphics. It is an open source version of the S programming language. R is quickly becoming the language of choice for data science due to the ease with which it can produce high quality plots and data visualizations. It is a versatile platform with a large, growing community and collection of packages.
For more general information on R visit The R Project for Statistical Computing.
Loading Data into R
R is an environment for manipulating data. In order to manipulate data, it must be brought into the R environment. R has a function to read any file that data is stored in. Some of the most common file types like comma-separated variable(CSV) files have functions that come in the basic R packages. Other less common file types require additional packages to be installed. To read data from a CSV file into the R environment, enter the following command in the R prompt:
> read.csv(file = "path/to/data.csv", header = TRUE)
When R reads the file it creates an object that can then become the target of other functions. By default the read.csv() function will give the object the name of the .csv file. To assign a different name to the object created by read.csv enter the following in the R prompt:
> my_variable <- read.csv(file = "path/to/data.csv", header = FALSE)
To display the properties (structure) of loaded data, enter the following:
> str(my_variable)
For more functions and tutorials:
Installing R packages
Link to section 'Challenges of Managing R Packages in the Cluster Environment' of 'Installing R packages' Challenges of Managing R Packages in the Cluster Environment
- Different clusters have different hardware and softwares. So, if you have access to multiple clusters, you must install your R packages separately for each cluster.
- Each cluster has multiple versions of R and packages installed with one version of R may not work with another version of R. So, libraries for each R version must be installed in a separate directory.
- You can define the directory where your R packages will be installed using the environment variable
R_LIBS_USER
. - For your convenience, a sample ~/.Rprofile example file is provided that can be downloaded to your cluster account and renamed into
~/.Rprofile
(or appended to one) to customize your installation preferences. Detailed instructions.
Link to section 'Installing Packages' of 'Installing R packages' Installing Packages
-
Step 0: Set up installation preferences.
Follow the steps for setting up your~/.Rprofile
preferences. This step needs to be done only once. If you have created a~/.Rprofile
file previously on Gautschi, ignore this step. -
Step 1: Check if the package is already installed.
As part of the R installations on community clusters, a lot of R libraries are pre-installed. You can check if your package is already installed by opening an R terminal and entering the commandinstalled.packages()
. For example,module load r/4.4.1 R
installed.packages()["units",c("Package","Version")] Package Version "units" "0.8-1" quit()
If the package you are trying to use is already installed, simply load the library, e.g.,
library('units')
. Otherwise, move to the next step to install the package. -
Step 2: Load required dependencies. (if needed)
For simple packages you may not need this step. However, some R packages depend on other libraries. For example, thesf
package depends ongdal
andgeos
libraries. So, you will need to load the corresponding modules before installingsf
. Read the documentation for the package to identify which modules should be loaded.module load gdal module load geos
-
Step 3: Install the package.
Now install the desired package using the commandinstall.packages('package_name')
. R will automatically download the package and all its dependencies from CRAN and install each one. Your terminal will show the build progress and eventually show whether the package was installed successfully or not.R
install.packages('sf', repos="https://cran.case.edu/") Installing package into ‘/home/myusername/R/x86_64-pc-linux-gnu-library/4.4.1’ (as ‘lib’ is unspecified) trying URL 'https://cran.case.edu/src/contrib/sf_0.9-7.tar.gz' Content type 'application/x-gzip' length 4203095 bytes (4.0 MB) ================================================== downloaded 4.0 MB ... ... more progress messages ... ... ** testing if installed package can be loaded from final location ** testing if installed package keeps a record of temporary installation path * DONE (sf) The downloaded source packages are in ‘/tmp/RtmpSVAGio/downloaded_packages’
- Step 4: Troubleshooting. (if needed)
If Step 3 ended with an error, you need to investigate why the build failed. Most common reason for build failure is not loading the necessary modules.
Link to section 'Loading Libraries' of 'Installing R packages' Loading Libraries
Once you have packages installed you can load them with the library()
function as shown below:
library('packagename')
The package is now installed and loaded and ready to be used in R.
Link to section 'Example: Installing dplyr' of 'Installing R packages' Example: Installing dplyr
The following demonstrates installing the dplyr
package assuming the above-mentioned custom ~/.Rprofile
is in place (note its effect in the "Installing package into" information message):
module load r
R
install.packages('dplyr', repos="http://ftp.ussg.iu.edu/CRAN/")
Installing package into ‘/home/myusername/R/gautschi/4.4.1’
(as ‘lib’ is unspecified)
...
also installing the dependencies 'crayon', 'utf8', 'bindr', 'cli', 'pillar', 'assertthat', 'bindrcpp', 'glue', 'pkgconfig', 'rlang', 'Rcpp', 'tibble', 'BH', 'plogr'
...
...
...
The downloaded source packages are in
'/tmp/RtmpHMzm9z/downloaded_packages'
library(dplyr)
Attaching package: 'dplyr'
For more information about installing R packages:
RStudio
RStudio is a graphical integrated development environment (IDE) for R. RStudio is the most popular environment for developing both R scripts and packages. RStudio is provided on most Research systems.
There are two methods to launch RStudio on the cluster: command-line and application menu icon.
Link to section 'Launch RStudio by the command-line:' of 'RStudio' Launch RStudio by the command-line:
module load gcc
module load r
module load rstudio
rstudio
Note that RStudio is a graphical program and in order to run it you must have a local X11 server running or use Thinlinc Remote Desktop environment. See the ssh X11 forwarding section for more details.
Link to section 'Launch Rstudio by the application menu icon:' of 'RStudio' Launch Rstudio by the application menu icon:
- Log into desktop.gautschi.rcac.purdue.edu with web browser or ThinLinc client
- Click on the
Applications
drop down menu on the top left corner - Choose
Cluster Software
and thenRStudio
R and RStudio are free to download and run on your local machine. For more information about RStudio:
Running R jobs
This section illustrates how to submit a small R job to a SLURM queue. The example job computes a Pythagorean triple.
Prepare an R input file with an appropriate filename, here named myjob.R:
# FILENAME: myjob.R
# Compute a Pythagorean triple.
a = 3
b = 4
c = sqrt(a*a + b*b)
c # display result
Prepare a job submission file with an appropriate filename, here named myjob.sub:
#!/bin/bash
# FILENAME: myjob.sub
module load r
# --vanilla:
# --no-save: do not save datasets at the end of an R session
R --vanilla --no-save < myjob.R
For other examples or R jobs:
Singularity
On Gautschi, Singularity functionality is provided by Apptainer - see Apptainer section for details.
Apptainer
Note: Apptainer was formerly known as Singularity and is now a part of the Linux Foundation. When migrating from Singularity see the user compatibility documentation.
Link to section 'What is Apptainer?' of 'Apptainer' What is Apptainer?
Apptainer is an open-source container platform designed to be simple, fast, and secure. It allows the portability and reproducibility of operating systems and application environments through the use of Linux containers. It gives users complete control over their environment.
Apptainer is like Docker but tuned explicitly for HPC clusters. More information is available on the project’s website.
Link to section 'Features' of 'Apptainer' Features
- Run the latest applications on an Ubuntu or Centos userland
- Gain access to the latest developer tools
- Launch MPI programs easily
- Much more
Apptainer’s user guide is available at: apptainer.org/docs/user/main/introduction.html
Link to section 'Example' of 'Apptainer' Example
Here is an example using an Ubuntu 16.04 image on Gautschi:
apptainer exec /depot/itap/singularity/ubuntu1604.img cat /etc/lsb-release
DISTRIB_ID=Ubuntu
DISTRIB_RELEASE=16.04
DISTRIB_CODENAME=xenial
DISTRIB_DESCRIPTION="Ubuntu 16.04 LTS"
Here is another example using a Centos 7 image:
apptainer exec /depot/itap/singularity/centos7.img cat /etc/redhat-release
CentOS Linux release 7.3.1611 (Core)
Link to section 'Purdue Cluster Specific Notes' of 'Apptainer' Purdue Cluster Specific Notes
All service providers will integrate Apptainer slightly differently depending on site. The largest customization will be which default files are inserted into your images so that routine services will work.
Services we configure for your images include DNS settings and account information. File systems we overlay into your images are your home directory, scratch, Data Depot, and application file systems.
Here is a list of paths:
- /etc/resolv.conf
- /etc/hosts
- /home/$USER
- /apps
- /scratch
- /depot
This means that within the container environment these paths will be present and the same as outside the container. The /apps
, /scratch
, and /depot
directories will need to exist inside your container to work properly.
Link to section 'Creating Apptainer Images' of 'Apptainer' Creating Apptainer Images
You can build on your system or straight on the cluster (you do not need root privileges to build or run the container).
You can find information and documentation for how to install and use Apptainer on your system:
We have version 1.1.6
(or newer) on the cluster. Please note that installed versions may change throughout cluster life time, so when in doubt, please check exact version with a --version
command line flag:
apptainer --version
apptainer version 1.3.3-1.el9
Everything you need on how to build a container is available from their user guide. Below are merely some quick tips for getting your own containers built for Gautschi.
You can use a Definition File to both build your container and share its specification with collaborators (for the sake of reproducibility). Here is a simplistic example of such a file:
# FILENAME: Buildfile
Bootstrap: docker
From: ubuntu:18.04
%post
apt-get update && apt-get upgrade -y
mkdir /apps /depot /scratch
To build the image itself:
apptainer build ubuntu-18.04.sif Buildfile
The challenge with this approach however is that it must start from scratch if you decide to change something. In order to create a container image iteratively and interactively, you can use the --sandbox
option.
apptainer build --sandbox ubuntu-18.04 docker://ubuntu:18.04
This will not create a flat image file but a directory tree (i.e., a folder), the contents of which are the container's filesystem. In order to get a shell inside the container that allows you to modify it, user the --writable
option.
apptainer shell --writable ubuntu-18.04
Apptainer>
You can then proceed to install any libraries, software, etc. within the container. Then to create the final image file, exit
the shell and call the build
command once more on the sandbox.
apptainer build ubuntu-18.04.sif ubuntu-18.04
Finally, copy the new image to Gautschi and run it.
GPU Usage Monitoring
Link to section 'What is it?' of 'GPU Usage Monitoring' What is it?
To ensure that GPUs are effectively utilized on our cluster, we log and store the power, memory, and utilization of every GPU on our cluster every 10 seconds. RCAC uses this data to identify users that request large amounts of GPU hours but do not actually use them.
To assist users in optimizing their usage of requested GPU resources, we have created a GPU usage monitor tool that allows users to track their GPU utilization for jobs that they have submitted. The purpose of this tool is to provide users an interface to identify jobs or GPUs that they have requested but are not being effectively utilized.
Link to section 'How to use' of 'GPU Usage Monitoring' How to use
Our GPU Usage monitor is currently implemented on Gautschi
and Gilbreth
, and is available at the following location:
/apps/external/gpu_util/get_gpu_util
Link to section 'Seeing Logged Jobs' of 'GPU Usage Monitoring' Seeing Logged Jobs
You can check all the jobs that you've run with the -u
or --user_jobs
flag:
/apps/external/gpu_util/get_gpu_util --user_jobs
This will print a table of all the GPU jobs you have submitted to the cluster, with the job ID, start and end times, and the number of GPUs that that job had allocated to it:
It should be noted that we collect GPU usage information every 3 - 3.5 hours. If you have recently submitted a job, your job may not be available yet.
Link to section 'Inspecting a specific job' of 'GPU Usage Monitoring' Inspecting a specific job
If you want to view the GPU utilization of a specific job, you can use the -j
or --job_id
flag. For example, to see the utilization of job 32083, you may run the following:
/apps/external/gpu_util/get_gpu_util --job_id 32083
This will print a table of the GPU memory and utilization for each GPU that was allocated for the specified job:

Link to section 'Plotting Data' of 'GPU Usage Monitoring' Plotting Data
It is also possible to plot the GPU utilization and memory usage over time with the -p
or the --plot
flag:
/apps/external/gpu_util/get_gpu_util --job_id 32083 --plot
This will print two graphs. The first will be a 2-minute rolling average of the GPU utilization over time, and the second will be the percentage of memory used over time. Each GPU will be colored differently:
Link to section 'Saving GPU Data' of 'GPU Usage Monitoring' Saving GPU Data
If you would like to save the raw GPU utilization and memory usage data for your job, you can use the -s
or --save
flag:
/apps/external/gpu_util/get_gpu_util --job_id 32083 --save
This will download the GPU data for a specific job as:
{job_id}_gpu_usage.csv
Link to section 'Checking Account Usage (Group Managers only)' of 'GPU Usage Monitoring' Checking Account Usage (Group Managers only)
If you are a manager of a specific group, you are able to query all accounts owned by that group. You can check the usage of all members of your account over the previous week by running:
/apps/external/gpu_util/get_gpu_util -A accountname
If you would like a breakdown of the individual jobs ran within the past week, you can add the --records
flag:
/apps/external/gpu_util/get_gpu_util -A accountname --records
Further, the --job {jobid}
flag will also allow you to query the utilization of individual jobs that were ran on an account that you manage.
Link to section 'FAQ/Troubleshooting' of 'GPU Usage Monitoring' FAQ/Troubleshooting
- "Why doesn't my job show up in the list?"
- Although we log GPU utilization every 10 seconds, we only actually collect it every 3-3.5 hours. If you check later, your job should be listed.
- "Can other people see my data?"
- No, our interface only allows users to check the utilization of their own jobs.
- "Is this a good way to see the GPU Hours balance?"
- No, the primary purpose of this tool is to check that GPUs are actually being used, not to track billed GPU hours. To see the total GPU hours balance, please use the
slist
command.
- No, the primary purpose of this tool is to check that GPUs are actually being used, not to track billed GPU hours. To see the total GPU hours balance, please use the
File Storage and Transfer
Learn more about file storage transfer for Gautschi.
Storage Options
File storage options on RCAC systems include long-term storage (home directories, depot, Fortress) and short-term storage (scratch directories, /tmp directory). Each option has different performance and intended uses, and some options vary from system to system as well. Daily snapshots of home directories are provided for a limited time for accidental deletion recovery. Scratch directories and temporary storage are not backed up and old files are regularly purged from scratch and /tmp directories. More details about each storage option appear below.
Home Directory
Home directories are provided for long-term file storage. Each user has one home directory. You should use your home directory for storing important program files, scripts, input data sets, critical results, and frequently used files. You should store infrequently used files on Fortress. Your home directory becomes your current working directory, by default, when you log in.
Daily snapshots of your home directory are provided for a limited period of time in the event of accidental deletion. For additional security, you should store another copy of your files on more permanent storage, such as the Fortress HPSS Archive.
Your home directory physically resides on a GPFS storage system in the data center. To find the path to your home directory, first log in then immediately enter the following:
$ pwd
/home/myusername
Or from any subdirectory:
$ echo $HOME
/home/myusername
Your home directory has a quota limiting the total size of files you may store within. For more information, refer to the Storage Quotas / Limits Section.
Link to section 'Lost File Recovery' of 'Home Directory' Lost File Recovery
Nightly snapshots for 7 days, weekly snapshots for 4 weeks, and monthly snapshots for 3 months are kept. This means you will find snapshots from the last 7 nights, the last 4 Sundays, and the last 3 first of the months. Files are available going back between two and three months, depending on how long ago the last first of the month was. Snapshots beyond this are not kept. For additional security, you should store another copy of your files on more permanent storage, such as the Fortress HPSS Archive.
Link to section 'Performance' of 'Home Directory' Performance
Your home directory is medium-performance, non-purged space suitable for tasks like sharing data, editing files, developing and building software, and many other uses.
Your home directory is not designed or intended for use as high-performance working space for running data-intensive jobs with heavy I/O demands.
Scratch Space
Scratch directories are provided for short-term file storage only. The quota of your scratch directory is much greater than the quota of your home directory. You should use your scratch directory for storing temporary input files which your job reads or for writing temporary output files which you may examine after execution of your job. You should use your home directory and Fortress for longer-term storage or for holding critical results. The hsi and htar commands provide easy-to-use interfaces into the archive and can be used to copy files into the archive interactively or even automatically at the end of your regular job submission scripts.
Files in scratch directories are not recoverable. Files in scratch directories are not backed up. If you accidentally delete a file, a disk crashes, or old files are purged, they cannot be restored.
Files are purged from scratch directories not accessed or had content modified in 60 days. Owners of these files receive a notice one week before removal via email. Be sure to regularly check your Purdue email account or set up mail forwarding to an email account you do regularly check. For more information, please refer to our Scratch File Purging Policy.
All users may access scratch directories on Gautschi. To find the path to your scratch directory:
$ findscratch
/scratch/gautschi/myusername
The value of variable $RCAC_SCRATCH is your scratch directory path. Use this variable in any scripts. Your actual scratch directory path may change without warning, but this variable will remain current.
$ echo $RCAC_SCRATCH
/scratch/gautschi/myusername
Scratch directories are specific per cluster. I.e. only the /scratch/gautschi directory is available on Gautschi front-end and compute nodes. No other scratch directories are available on Gautschi.
Your scratch directory has a quota capping the total size and number of files you may store in it. For more information, refer to the section Storage Quotas / Limits.
Link to section 'Performance' of 'Scratch Space' Performance
Your scratch directory is located on a high-performance, large-capacity parallel filesystem engineered to provide work-area storage optimized for a wide variety of job types. It is designed to perform well with data-intensive computations, while scaling well to large numbers of simultaneous connections.
/tmp Directory
/tmp directories are provided for short-term file storage only. Each front-end and compute node has a /tmp directory. Your program may write temporary data to the /tmp
directory of the compute node on which it is running. That data is available for as long as your program is active. Once your program terminates, that temporary data is no longer available. When used properly, /tmp
may provide faster local storage to an active process than any other storage option. You should use your home directory and Fortress for longer-term storage or for holding critical results.
Backups are not performed for the /tmp
directory and removes files from /tmp
whenever space is low or whenever the system needs a reboot. In the event of a disk crash or file purge, files in /tmp
are not recoverable. You should copy any important files to more permanent storage.
Link to section 'Long-Term Storage' of 'Long-Term Storage' Long-Term Storage
Long-term Storage or Permanent Storage is available to users on the High Performance Storage System (HPSS), an archival storage system, called Fortress. Program files, data files and any other files which are not used often, but which must be saved, can be put in permanent storage. Fortress currently has over 10PB of capacity.
For more information about Fortress, how it works, and user guides, and how to obtain an account:
File Transfer
Gautschi supports several methods for file transfer. Use the links below to learn more about these methods.
SCP
SCP (Secure CoPy) is a simple way of transferring files between two machines that use the SSH protocol. SCP is available as a protocol choice in some graphical file transfer programs and also as a command line program on most Linux, Unix, and Mac OS X systems. SCP can copy single files, but will also recursively copy directory contents if given a directory name.
After Aug 17, 2020, the community clusters will not support password-based authentication for login. Methods that can be used include two-factor authentication (Purdue Login) or SSH keys. If you do not have SSH keys installed, you would need to type your Purdue Login response into the SFTP's "Password" prompt.
Link to section 'Command-line usage:' of 'SCP' Command-line usage:
You can transfer files both to and from Gautschi while initiating an SCP session on either some other computer or on Gautschi (in other words, directionality of connection and directionality of data flow are independent from each other). The scp
command appears somewhat similar to the familiar cp
command, with an extra user@host:file
syntax to denote files and directories on a remote host. Either Gautschi or another computer can be a remote.
-
Example: Initiating SCP session on some other computer (i.e. you are on some other computer, connecting to Gautschi):
(transfer TO Gautschi) (Individual files) $ scp sourcefile myusername@gautschi.rcac.purdue.edu:somedir/destinationfile $ scp sourcefile myusername@gautschi.rcac.purdue.edu:somedir/ (Recursive directory copy) $ scp -pr sourcedirectory/ myusername@gautschi.rcac.purdue.edu:somedir/ (transfer FROM Gautschi) (Individual files) $ scp myusername@gautschi.rcac.purdue.edu:somedir/sourcefile destinationfile $ scp myusername@gautschi.rcac.purdue.edu:somedir/sourcefile somedir/ (Recursive directory copy) $ scp -pr myusername@gautschi.rcac.purdue.edu:sourcedirectory somedir/
The -p flag is optional. When used, it will cause the transfer to preserve file attributes and permissions. The -r flag is required for recursive transfers of entire directories.
-
Example: Initiating SCP session on Gautschi (i.e. you are on Gautschi, connecting to some other computer):
(transfer TO Gautschi) (Individual files) $ scp myusername@$another.computer.example.com:sourcefile somedir/destinationfile $ scp myusername@$another.computer.example.com:sourcefile somedir/ (Recursive directory copy) $ scp -pr myusername@$another.computer.example.com:sourcedirectory/ somedir/ (transfer FROM Gautschi) (Individual files) $ scp somedir/sourcefile myusername@$another.computer.example.com:destinationfile $ scp somedir/sourcefile myusername@$another.computer.example.com:somedir/ (Recursive directory copy) $ scp -pr sourcedirectory myusername@$another.computer.example.com:somedir/
The -p flag is optional. When used, it will cause the transfer to preserve file attributes and permissions. The -r flag is required for recursive transfers of entire directories.
Link to section 'Software (SCP clients)' of 'SCP' Software (SCP clients)
Linux and other Unix-like systems:
- The
scp
command-line program should already be installed.
Microsoft Windows:
- MobaXterm
Free, full-featured, graphical Windows SSH, SCP, and SFTP client. - Command-line
scp
program can be installed as part of Windows Subsystem for Linux (WSL), or Git-Bash.
Mac OS X:
- The
scp
command-line program should already be installed. You may start a local terminal window from "Applications->Utilities". - Cyberduck is a full-featured and free graphical SFTP and SCP client.
FTP / SFTP
FTP is not supported on any research systems because it does not allow for secure transmission of data. Use SFTP instead, as described below.
SFTP (Secure File Transfer Protocol) is a reliable way of transferring files between two machines. SFTP is available as a protocol choice in some graphical file transfer programs and also as a command-line program on most Linux, Unix, and Mac OS X systems. SFTP has more features than SCP and allows for other operations on remote files, remote directory listing, and resuming interrupted transfers. Command-line SFTP cannot recursively copy directory contents; to do so, try using SCP or graphical SFTP client.
After Aug 17, 2020, the community clusters will not support password-based authentication for login. Methods that can be used include two-factor authentication (Purdue Login) or SSH keys. If you do not have SSH keys installed, you would need to type your Purdue Login response into the SFTP's "Password" prompt.
Link to section 'Command-line usage' of 'FTP / SFTP' Command-line usage
You can transfer files both to and from Gautschi while initiating an SFTP session on either some other computer or on Gautschi (in other words, directionality of connection and directionality of data flow are independent from each other). Once the connection is established, you use put
or get
subcommands between "local" and "remote" computers. Either Gautschi or another computer can be a remote.
-
Example: Initiating SFTP session on some other computer (i.e. you are on another computer, connecting to Gautschi):
$ sftp myusername@gautschi.rcac.purdue.edu (transfer TO Gautschi) sftp> put sourcefile somedir/destinationfile sftp> put -P sourcefile somedir/ (transfer FROM Gautschi) sftp> get sourcefile somedir/destinationfile sftp> get -P sourcefile somedir/ sftp> exit
The -P flag is optional. When used, it will cause the transfer to preserve file attributes and permissions.
-
Example: Initiating SFTP session on Gautschi (i.e. you are on Gautschi, connecting to some other computer):
$ sftp myusername@$another.computer.example.com (transfer TO Gautschi) sftp> get sourcefile somedir/destinationfile sftp> get -P sourcefile somedir/ (transfer FROM Gautschi) sftp> put sourcefile somedir/destinationfile sftp> put -P sourcefile somedir/ sftp> exit
The -P flag is optional. When used, it will cause the transfer to preserve file attributes and permissions.
Link to section 'Software (SFTP clients)' of 'FTP / SFTP' Software (SFTP clients)
Linux and other Unix-like systems:
- The
sftp
command-line program should already be installed.
Microsoft Windows:
- MobaXterm
Free, full-featured, graphical Windows SSH, SCP, and SFTP client. - Command-line
sftp
program can be installed as part of Windows Subsystem for Linux (WSL), or Git-Bash.
Mac OS X:
- The
sftp
command-line program should already be installed. You may start a local terminal window from "Applications->Utilities". - Cyberduck is a full-featured and free graphical SFTP and SCP client.
Globus
Link to section 'Globus' of 'Globus' Globus
Globus, previously known as Globus Online, is a powerful and easy to use file transfer service for transferring files virtually anywhere. It works within RCAC's various research storage systems; it connects between RCAC and remote research sites running Globus; and it connects research systems to personal systems. You may use Globus to connect to your home, scratch, and Fortress storage directories. Since Globus is web-based, it works on any operating system that is connected to the internet. The Globus Personal client is available on Windows, Linux, and Mac OS X. It is primarily used as a graphical means of transfer but it can also be used over the command line.
Link to section 'Link to section 'Globus Web:' of 'Globus' Globus Web:' of 'Globus' Link to section 'Globus Web:' of 'Globus' Globus Web:
- Navigate to http://transfer.rcac.purdue.edu
- Click "Proceed" to log in with your Purdue Career Account.
- On your first login it will ask to make a connection to a Globus account. Accept the conditions.
- Now you are at the main screen. Click "File Transfer" which will bring you to a two-panel interface (if you only see one panel, you can use selector in the top-right corner to switch the view).
- You will need to select one collection and file path on one side as the source, and the second collection on the other as the destination. This can be one of several Purdue endpoints, or another University, or even your personal computer (see Personal Client section below).
The RCAC collections are as follows. A search for "Purdue" will give you several suggested results you can choose from, or you can give a more specific search.
- Home directory and Scratch storage: "Gautschi Cluster Collection", however, you can start typing "gautschi" and it will suggest appropriate matches.
- Research Data Depot: "Purdue Research Computing - Data Depot", a search for "Depot" should provide appropriate matches to choose from.
- Fortress: "Purdue Fortress HPSS Archive", a search for "Fortress" should provide appropriate matches to choose from.
From here, select a file or folder in either side of the two-pane window, and then use the arrows in the top-middle of the interface to instruct Globus to move files from one side to the other. You can transfer files in either direction. You will receive an email once the transfer is completed.
Link to section 'Link to section 'Globus Personal Client setup:' of 'Globus' Globus Personal Client setup:' of 'Globus' Link to section 'Globus Personal Client setup:' of 'Globus' Globus Personal Client setup:
Globus Connect Personal is a small software tool you can install to make your own computer a Globus endpoint on its own. It is useful if you need to transfer files via Globus to and from your computer directly.
- On the "Collections" page from earlier, click "Get Globus Connect Personal" or download a version for your operating system it from here: Globus Connect Personal
- Name this particular personal system and follow the setup prompts to create your Globus Connect Personal endpoint.
- Your personal system is now available as a collection within the Globus transfer interface.
Link to section 'Link to section 'Globus Command Line:' of 'Globus' Globus Command Line:' of 'Globus' Link to section 'Globus Command Line:' of 'Globus' Globus Command Line:
Globus supports command line interface, allowing advanced automation of your transfers.
To use the recommended standalone Globus CLI application (the globus command):
- First time use: issue the globus login command and follow instructions for initial login.
- Commands for interfacing with the CLI can be found via Using the Command Line Interface, as well as the Globus CLI Examples pages.
Link to section 'Link to section 'Sharing Data with Outside Collaborators' of 'Globus' Sharing Data with Outside Collaborators' of 'Globus' Link to section 'Sharing Data with Outside Collaborators' of 'Globus' Sharing Data with Outside Collaborators
Globus allows convenient sharing of data with outside collaborators. Data can be shared with collaborators' personal computers or directly with many other computing resources at other institutions. See the Globus documentation on how to share data:
For links to more information, please see Globus Support page and RCAC Globus presentation.
Windows Network Drive / SMB
SMB (Server Message Block), also known as CIFS, is an easy to use file transfer protocol that is useful for transferring files between RCAC systems and a desktop or laptop. You may use SMB to connect to your home, scratch, and Fortress storage directories. The SMB protocol is available on Windows, Linux, and Mac OS X. It is primarily used as a graphical means of transfer but it can also be used over the command line.
Note: to access Gautschi through SMB file sharing, you must be on a Purdue campus network or connected through VPN.
Link to section 'Windows:' of 'Windows Network Drive / SMB' Windows:
- Windows 7: Click Windows menu > Computer, then click Map Network Drive in the top bar
- Windows 8 & 10: Tap the Windows key, type computer, select This PC, click Computer > Map Network Drive in the top bar
- Windows 11: Tap the Windows key, type File Explorer, select This PC, click Computer > Map Network Drive in the top bar
- In the folder location enter the following information and click Finish:
- To access your Gautschi home directory, enter \\home.gautschi.rcac.purdue.edu\gautschi-home.
- To access your scratch space on Gautschi, enter \\scratch.gautschi.rcac.purdue.edu\gautschi-scratch. Once mapped, you will be able to navigate to your scratch directory.
- Note: Use your career account login name and password when prompted. (You will not need to add "
,push
" nor use your Purdue Duo client.) - Your home or scratch directory should now be mounted as a drive in the Computer window.
Link to section 'Mac OS X:' of 'Windows Network Drive / SMB' Mac OS X:
- In the Finder, click Go > Connect to Server
- In the Server Address enter the following information and click Connect:
- To access your Gautschi home directory, enter smb://home.gautschi.rcac.purdue.edu/gautschi-home.
- To access your scratch space on Gautschi, enter smb://scratch.gautschi.rcac.purdue.edu/gautschi-scratch. Once mapped, you will be able to navigate to your scratch directory.
- Note: Use your career account login name and password when prompted. (You will not need to add "
,push
" nor use your Purdue Duo client.) - Your home or scratch directory should now be mounted as a drive in the Computer window.
Link to section 'Linux:' of 'Windows Network Drive / SMB' Linux:
- There are several graphical methods to connect in Linux depending on your desktop environment. Once you find out how to connect to a network server on your desktop environment, choose the Samba/SMB protocol and adapt the information from the Mac OS X section to connect.
- If you would like access via samba on the command line you may install smbclient which will give you FTP-like access and can be used as shown below. For all the possible ways to connect look at the Mac OS X instructions.
smbclient //home.gautschi.rcac.purdue.edu/gautschi-home -U myusername smbclient //scratch.gautschi.rcac.purdue.edu/gautschi-scratch -U myusername
- Note: Use your career account login name and password when prompted. (You will not need to add "
,push
" nor use your Purdue Duo client.)
HSI
HSI, the Hierarchical Storage Interface, is the preferred method of transferring files to and from Gautschi. HSI is designed to be a friendly interface for users of the High Performance Storage System (HPSS). It provides a familiar Unix-style environment for working within HPSS while automatically taking advantage of high-speed, parallel file transfers without requiring any special user knowledge.
HSI is provided on all research systems as the command hsi. HSI is also available for download for many operating systems.
Interactive usage:
$ hsi
*************************************************************************
* Purdue University
* High Performance Storage System (HPSS)
*************************************************************************
* This is the Purdue Data Archive, Fortress. For further information
* see http://www.rcac.purdue.edu/storage/fortress/
*
* If you are having problems with HPSS, please call IT/Operational
* Services at 49-44000 or send E-mail to rcac-help@purdue.edu.
*
*************************************************************************
Username: myusername UID: 12345 Acct: 12345(12345) Copies: 1 Firewall: off [hsi.3.5.8 Wed Sep 21 17:31:14 EDT 2011]
[Fortress HSI]/home/myusername->put data1.fits
put 'test' : '/home/myusername/test' ( 1024000000 bytes, 250138.1 KBS (cos=11))
[Fortress HSI]/home/myusername->lcd /tmp
[Fortress HSI]/home/myusername->get data1.fits
get '/tmp/data1.fits' : '/home/myusername/data1.fits' (2011/10/04 16:28:50 1024000000 bytes, 325844.9 KBS )
[Fortress HSI]/home/myusername->quit
Batch transfer file:
put data1.fits
put data2.fits
put data3.fits
put data4.fits
put data5.fits
put data6.fits
put data7.fits
put data8.fits
put data9.fits
Batch usage:
$ hsi < my_batch_transfer_file
*************************************************************************
* Purdue University
* High Performance Storage System (HPSS)
*************************************************************************
* This is the Purdue Data Archive, Fortress. For further information
* see http://www.rcac.purdue.edu/storage/fortress/
*
* If you are having problems with HPSS, please call IT/Operational
* Services at 49-44000 or send E-mail to rcac-help@purdue.edu.
*
*************************************************************************
Username: myusername UID: 12345 Acct: 12345(12345) Copies: 1 Firewall: off [hsi.3.5.8 Wed Sep 21 17:31:14 EDT 2011]
put 'data1.fits' : '/home/myusername/data1.fits' ( 1024000000 bytes, 250200.7 KBS (cos=11))
put 'data2.fits' : '/home/myusername/data2.fits' ( 1024000000 bytes, 258893.4 KBS (cos=11))
put 'data3.fits' : '/home/myusername/data3.fits' ( 1024000000 bytes, 222819.7 KBS (cos=11))
put 'data4.fits' : '/home/myusername/data4.fits' ( 1024000000 bytes, 224311.9 KBS (cos=11))
put 'data5.fits' : '/home/myusername/data5.fits' ( 1024000000 bytes, 323707.3 KBS (cos=11))
put 'data6.fits' : '/home/myusername/data6.fits' ( 1024000000 bytes, 320322.9 KBS (cos=11))
put 'data7.fits' : '/home/myusername/data7.fits' ( 1024000000 bytes, 253192.6 KBS (cos=11))
put 'data8.fits' : '/home/myusername/data8.fits' ( 1024000000 bytes, 253056.2 KBS (cos=11))
put 'data9.fits' : '/home/myusername/data9.fits' ( 1024000000 bytes, 323218.9 KBS (cos=11))
EOF detected on TTY - ending HSI session
For more information about HSI:
HTAR
HTAR (short for "HPSS TAR") is a utility program that writes TAR-compatible archive files directly onto Gautschi, without having to first create a local file. Its command line was originally based on tar, with a number of extensions added to provide extra features.
HTAR is provided on all research systems as the command htar. HTAR is also available for download for many operating systems.
Link to section 'Usage:' of 'HTAR' Usage:
Create a tar archive on Gautschi named data.tar including all files with the extension ".fits":
$ htar -cvf data.tar *.fits
HTAR: a data1.fits
HTAR: a data2.fits
HTAR: a data3.fits
HTAR: a data4.fits
HTAR: a data5.fits
HTAR: a /tmp/HTAR_CF_CHK_17953_1317760775
HTAR Create complete for data.tar. 5,120,006,144 bytes written for 5 member files, max threads: 3 Transfer time: 16.457 seconds (311.121 MB/s)
HTAR: HTAR SUCCESSFUL
Unpack a tar archive on Gautschi named data.tar into a scratch directory for use in a batch job:
$ cd $RCAC_SCRATCH/job_dir
$ htar -xvf data.tar
HTAR: x data1.fits, 1024000000 bytes, 2000001 media blocks
HTAR: x data2.fits, 1024000000 bytes, 2000001 media blocks
HTAR: x data3.fits, 1024000000 bytes, 2000001 media blocks
HTAR: x data4.fits, 1024000000 bytes, 2000001 media blocks
HTAR: x data5.fits, 1024000000 bytes, 2000001 media blocks
HTAR: Extract complete for data.tar, 5 files. total bytes read: 5,120,004,608 in 18.841 seconds (271.749 MB/s )
HTAR: HTAR SUCCESSFUL
Look at the contents of the data.tar HTAR archive on Gautschi:
$ htar -tvf data.tar
HTAR: -rw-r--r-- myusername/pucc 1024000000 2011-10-04 16:30 data1.fits
HTAR: -rw-r--r-- myusername/pucc 1024000000 2011-10-04 16:35 data2.fits
HTAR: -rw-r--r-- myusername/pucc 1024000000 2011-10-04 16:35 data3.fits
HTAR: -rw-r--r-- myusername/pucc 1024000000 2011-10-04 16:35 data4.fits
HTAR: -rw-r--r-- myusername/pucc 1024000000 2011-10-04 16:35 data5.fits
HTAR: -rw------- myusername/pucc 256 2011-10-04 16:39 /tmp/HTAR_CF_CHK_17953_1317760775
HTAR: Listing complete for data.tar, 6 files 6 total objects
HTAR: HTAR SUCCESSFUL
Unpack a single file, "data5.fits", from the tar archive on Gautschi named data.tar into a scratch directory.:
$ htar -xvf data.tar data5.fits
HTAR: x data5.fits, 1024000000 bytes, 2000001 media blocks
HTAR: Extract complete for data.tar, 1 files. total bytes read: 1,024,000,512 in 3.642 seconds (281.166 MB/s )
HTAR: HTAR SUCCESSFUL
Link to section 'HTAR Archive Verification' of 'HTAR' HTAR Archive Verification
HTAR allows different types of content verification while creating archives. Users can ask HTAR to verify the contents of an archive during (or after) creation using the '-Hverify' switch. The syntax of this option is:
$ htar -Hverify=option[,option...] ... other arguments ...
Option | Explanation |
---|---|
info |
Compares tar header info with the corresponding values in the index. |
crc |
Enables CRC checking of archive files for which a CRC was generated when the file is added to the archive. |
compare |
Enables a byte-by-byte comparison of archive member files and their local file counterparts. |
nocrc |
Disables CRC checking of archive files. |
nocompare |
Disables a byte-by-byte comparison of archive member files and their local file counterparts. |
Users can use a comma-separated list of options shown above, or a numeric value, or the wildcard all to specify the degree of verification. The numeric values for Hverify can be interpreted as follows:
0: Enables "info" verification.
1: Enables level 0 + "crc" verification.
2: Enables level 1 + "compare" verification.
all: Enables all comparison options.
An example to verify an archive during creation using checksums (crc):
htar -Hverify=1 -cvf abc.tar ./abc
An example to verify a previously created archive using checksums (crc):
htar -Hverify=1 -Kvf abc.tar
Please note that the time for verifying an archive increases as you increase the verification level. Carefully choose the option that suits your dataset best.
For details please see the HTAR Man Page.
For more information about HTAR:
HTAR has an individual file size limit of 64GB. If any files you are trying to archive with HTAR are greater than 64GB, then HTAR will immediately fail. This does not limit the number of files in the archive or the total overall size of the archive. To get around this limitation, try using the htar_large
command. It is slower than using htar
but it will work around the 64GB file size limit. This does not limit the number of files in the archive or the total overall size of the archive.
To get around this limitation, try using the htar_large
command. It is slower than using HTAR but it will work around the 64GB file size limit. The usage of htar_large
is almost the same as htar
except that htar_large
will not generate the tar index file. Thus, the -Hverify=1
option cannot be used since it's based on index file.
Storage Quota / Limits
Some limits are imposed on your disk usage on research systems. A quota is implemented on each filesystem. Each filesystem (home directory, scratch directory, etc.) may have a different limit. If you exceed the quota, you will not be able to save new files or new data to the filesystem until you delete or move data to long-term storage.
Link to section 'Checking Quota' of 'Storage Quota / Limits' Checking Quota
To check the current quotas of your home and scratch directories check the My Quota page or use the myquota
command:
$ myquota
Type Filesystem Size Limit Use Files Limit Use
==============================================================================
home myusername 5.0GB 25.0GB 20% - - -
scratch gautschi 220.7GB 100.0TB 0.22% 8k 2,000k 0.43%
The columns are as follows:
- Type: indicates home or scratch directory or your depot space.
- Filesystem: name of storage option.
- Size: sum of file sizes in bytes.
- Limit: allowed maximum on sum of file sizes in bytes.
- Use: percentage of file-size limit currently in use.
- Files: number of files and directories (not the size).
- Limit: allowed maximum on number of files and directories. It is possible, though unlikely, to reach this limit and not the file-size limit if you create a large number of very small files.
- Use: percentage of file-number limit currently in use.
If you find that you reached your quota in either your home directory or your scratch file directory, obtain estimates of your disk usage. Find the top-level directories which have a high disk usage, then study the subdirectories to discover where the heaviest usage lies.
To see in a human-readable format an estimate of the disk usage of your top-level directories in your home directory:
$ du -h --max-depth=1 $HOME >myfile
32K /home/myusername/mysubdirectory_1
529M /home/myusername/mysubdirectory_2
608K /home/myusername/mysubdirectory_3
The second directory is the largest of the three, so apply command du to it.
To see in a human-readable format an estimate of the disk usage of your top-level directories in your scratch file directory:
$ du -h --max-depth=1 $RCAC_SCRATCH >myfile
160K /scratch/gautschi/myusername
This strategy can be very helpful in figuring out the location of your largest usage. Move unneeded files and directories to long-term storage to free space in your home and scratch directories.
Link to section 'Increasing Quota' of 'Storage Quota / Limits' Increasing Quota
Link to section 'Home Directory' of 'Storage Quota / Limits' Home Directory
If you find you need additional disk space in your home directory, please consider archiving and compressing old files and moving them to long-term storage on the Fortress HPSS Archive, or purchase the Depot space for long-term storage. Unfortunately, it is not possible to increase your home directory quota beyond it's current level.
Link to section 'Scratch Space' of 'Storage Quota / Limits' Scratch Space
If you find you need additional disk space in your scratch space, please first consider archiving and compressing old files and moving them to long-term storage on the Fortress HPSS Archive. If you are unable to do so, you may ask for a quota increase by contacting support.
Link to section 'Storage Environment Variables' of 'Storage Environment Variables' Storage Environment Variables
Several environment variables are automatically defined for you to help you manage your storage. Use environment variables instead of actual paths whenever possible to avoid problems if the specific paths to any of these change.
Name | Description |
---|---|
HOME | /home/myusername |
PWD | path to your current directory |
RCAC_SCRATCH | /scratch/gautschi/myusername |
By convention, environment variable names are all uppercase. You may use them on the command line or in any scripts in place of and in combination with hard-coded values:
$ ls $HOME
...
$ ls $RCAC_SCRATCH/myproject
...
To find the value of any environment variable:
$ echo $RCAC_SCRATCH
/scratch/gautschi/myusername
To list the values of all environment variables:
$ env
USER=myusername
HOME=/home/myusername
RCAC_SCRATCH=/scratch/gautschi/myusername
...
You may create or overwrite an environment variable. To pass (export) the value of a variable in bash:
$ export MYPROJECT=$RCAC_SCRATCH/myproject
To assign a value to an environment variable in either tcsh or csh:
$ setenv MYPROJECT value
Link to section 'Archive and Compression' of 'Archive and Compression' Archive and Compression
There are several options for archiving and compressing groups of files or directories. The mostly commonly used options are:
Link to section 'tar' of 'Archive and Compression' tar
See the official documentation for tar for more information.
Saves many files together into a single archive file, and restores individual files from the archive. Includes automatic archive compression/decompression options and special features for incremental and full backups.
Examples:
(list contents of archive somefile.tar)
$ tar tvf somefile.tar
(extract contents of somefile.tar)
$ tar xvf somefile.tar
(extract contents of gzipped archive somefile.tar.gz)
$ tar xzvf somefile.tar.gz
(extract contents of bzip2 archive somefile.tar.bz2)
$ tar xjvf somefile.tar.bz2
(archive all ".c" files in current directory into one archive file)
$ tar cvf somefile.tar *.c
(archive and gzip-compress all files in a directory into one archive file)
$ tar czvf somefile.tar.gz somedirectory/
(archive and bzip2-compress all files in a directory into one archive file)
$ tar cjvf somefile.tar.bz2 somedirectory/
Other arguments for tar can be explored by using the man tar command.
Link to section 'gzip' of 'Archive and Compression' gzip
The standard compression system for all GNU software.
Examples:
(compress file somefile - also removes uncompressed file)
$ gzip somefile
(uncompress file somefile.gz - also removes compressed file)
$ gunzip somefile.gz
Link to section 'bzip2' of 'Archive and Compression' bzip2
See the official documentation for bzip for more information.
Strong, lossless data compressor based on the Burrows-Wheeler transform. Stronger compression than gzip.
Examples:
(compress file somefile - also removes uncompressed file)
$ bzip2 somefile
(uncompress file somefile.bz2 - also removes compressed file)
$ bunzip2 somefile.bz2
There are several other, less commonly used, options available as well:
- zip
- 7zip
- xz
Link to section 'Sharing Files from Gautschi' of 'Sharing' Sharing Files from Gautschi
Gautschi supports several methods for file sharing. Use the links below to learn more about these methods.
Link to section 'Sharing Data with Globus' of 'Globus' Sharing Data with Globus
Data on any RCAC resource can be shared with other users within Purdue or with collaborators at other institutions. Globus allows convenient sharing of data with outside collaborators. Data can be shared with collaborators' personal computers or directly with many other computing resources at other institutions.
To share files, login to https://transfer.rcac.purdue.edu, navigate to the endpoint (collection) of your choice, and follow instructions as described in Globus documentation on how to share data:
See also RCAC Globus presentation.
Link to section 'Sharing static content from your Data Depot space via the WWW' of 'WWW' Sharing static content from your Data Depot space via the WWW
Your research group can easily share static files (images, data, HTML) from your depot space via the WWW.
- Contact support to set up a "www" folder in your Data Depot space.
- Copy any files that you wish to share via the WWW into your Data Depot space's "www" folder.
- For example, cp /path/to/image.jpg /depot/mylab/www/, where mylab is your research group name.
- or upload to smb://datadepot.rcac.purdue.edu/depot/mylab/www, where mylab is your research group name.
- Your file is now accessible via your web browser at the URL https://www.datadepot.rcac.purdue.edu/mylab/image.jpg
Note that it is not possible to run web sites, dynamic content, interpreters (PHP, Perl, Python), or CGI scripts from this web site.
Gateway (Open OnDemand)
Gautschi's Gateway is an open-source HPC portal developed by the Ohio Supercomputing Center. Open OnDemand allows one to interact with HPC resources through a web browser and easily manage files, submit jobs, and interact with graphical applications directly in a browser, all with no software to install. Gautschi has an instance of OnDemand available that can be accessed via gateway.gautschi.rcac.purdue.edu.
Link to section 'Logging In' of 'Gateway (Open OnDemand)' Logging In
To log into Gateway:
- Navigate to gateway.gautschi.rcac.purdue.edu
- Log in using your Career account username and Purdue Login Duo client.
On the splash page you will see a quota usage report. If you are over 90% on any of your quotas a warning will be displayed. This information will update every 10-15 minutes while you are active on Gateway.
Link to section 'Apps' of 'Gateway (Open OnDemand)' Apps
There are a number of built-in apps in Gateway that can be accessed from the top menu bar. Below are links to documentation on each app.
Files
The Files app will let you access your files in your Home Directory, Scratch, and Data Depot spaces. The app lets you manage create, manage, and delete files and directories from your web browser. Navigate by double clicking on folders in the file explorer or by using the file tree on the left.
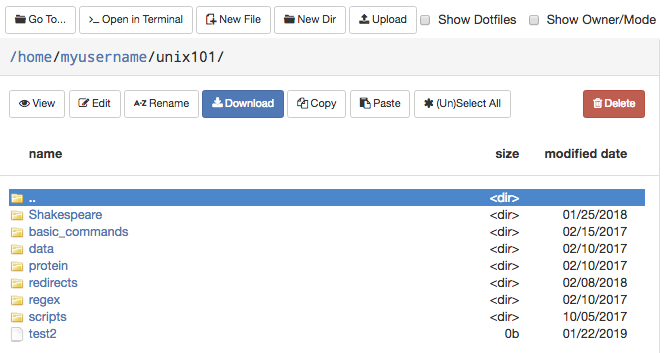
On the top row, there are buttons to:
- Go To: directly input a directory to navigate to
- Open in Terminal: launches the Shell app and navigates you to the current directory in the terminal
- New File: creates a new, empty file
- New Dir: creates a new, empty directory
- Upload: upload a file from your computer
Note: File uploads from your browser are limited to 100 GB per file. Be mindful that uploads over a few gigabytes may be unreliable through your browser, especially from off-campus connections. For very large files or off-campus transfers alternative methods such as Globus are highly recommended.
The second row of buttons lets you perform typical file management operations. The Edit button will open files in a fully fledged browser based text editor - it features syntax highlighting and vim and Emacs key bindings.
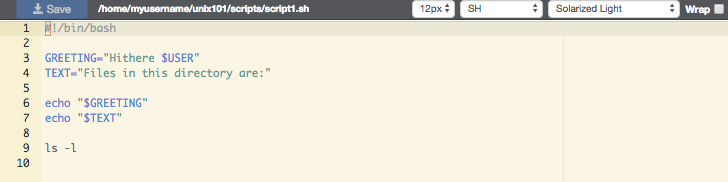
Jobs
There are two apps under the Jobs apps: Active Jobs and Job Composer. These are detailed below.
Link to section 'Active Jobs' of 'Jobs' Active Jobs
This shows you active SLURM jobs currently on the cluster. The default view will show you your current jobs, similar to squeue -u rices
. Using the button labeled "Your Jobs" in the upper right allows you to select different filters by queue (account). All accounts output by slist
will appear for you here. Using the arrow on the left hand side will expand the full job details.
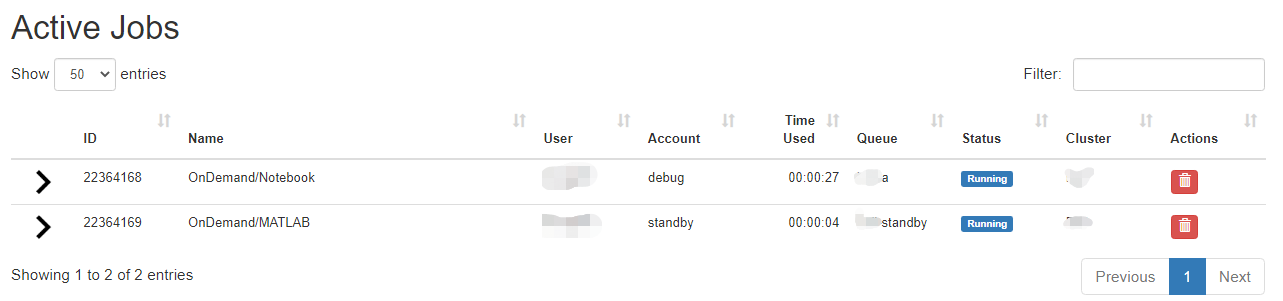
Link to section 'Job Composer' of 'Jobs' Job Composer
The Job Composer app allows you to create and submit jobs to the cluster. You can select from pre-defined templates (most of these are taken from the User Guide examples) or you can create your own templates for frequently used workflows.
Link to section 'Creating Job from Existing Template' of 'Jobs' Creating Job from Existing Template
Click "New Job" menu, then select "From Template":
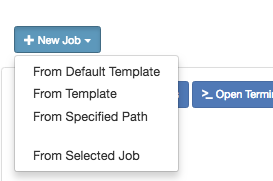
Then select from one of the available templates.
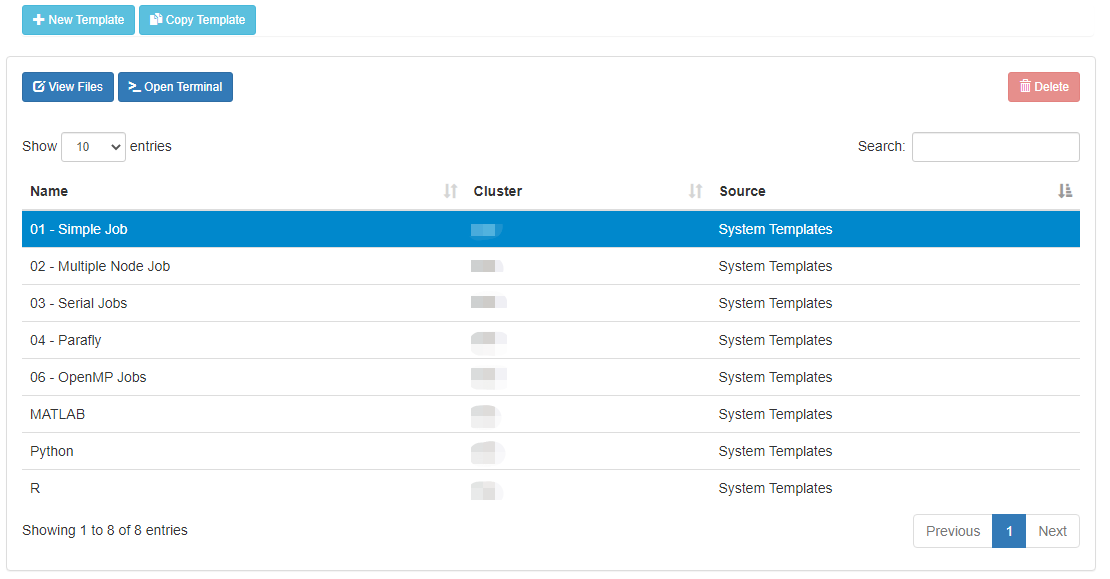
Click 'Create New Job' in second pane.
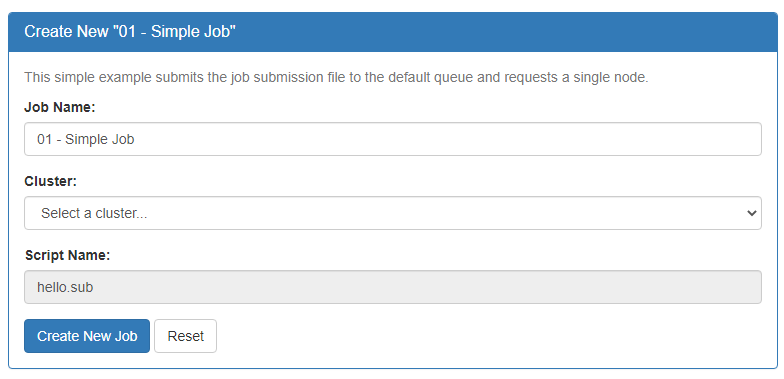
Your new job should be selected in your list of jobs. In the 'Submit Script' pane you can see the job script that was generated with an 'Open Editor' link to open the script in the built-in editor. Open the file in the editor and edit the script as necessary. By default the job will specify standby queue - this should be changed as appropriate, along with the node and walltime requests.
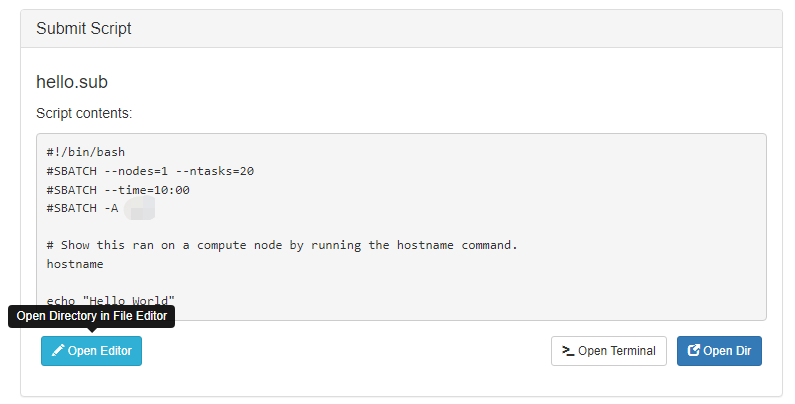
When you are finished with editing the job and are ready to submit, click the green 'Submit' button at the top of the job list. You can monitor progress from here or from the Active Jobs app. Once completed, you should see the output files appear:
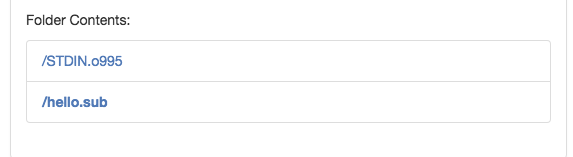
Clicking on one of the output files will open it in the file editor for your viewing.
Link to section 'Creating New Template' of 'Jobs' Creating New Template
First, prepare a template directory containing a template submission script along with any input files. Then, to import the job into the Job Composer app, click the 'Create New Template' button. Fill in the directory containing your template job script and files in the first box. Give it an appropriate name and notes.
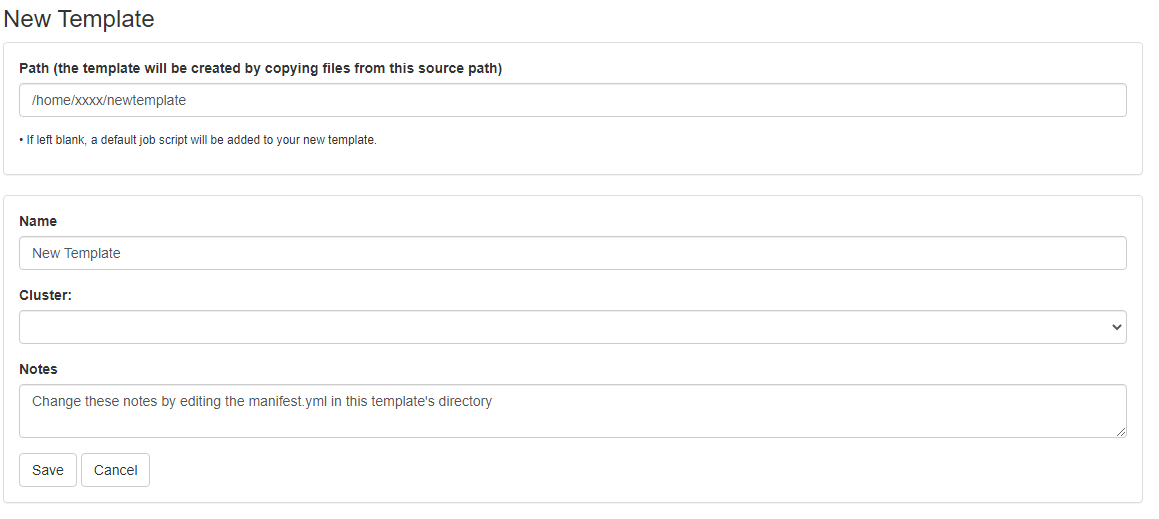
This template will now appear in your list of templates to choose from when composing jobs. You can now go create and submit a job from this new template.
Cluster Tools
The Cluster Tools menu contains cluster utilities. At the moment, only a terminal app is provided. Additional apps may be developed and provided in the future.
Link to section 'Shell Access' of 'Cluster Tools' Shell Access
Launching the shell app will provide you with a web-based terminal session on the cluster front-end. This is equivalent to using a standalone SSH client to connect to gautschi.rcac.purdue.edu
where you are connected to one several front-ends. The normal acceptable front-end use policy applies to access through the web-app. X11 Forwarding is not supported. Use of one of the interactive apps is recommended for graphical applications.
Interactive Apps
There are several interactive apps available through Gateway that can be accessed through the Interactive Apps dropdown menu. These apps are provided with a basic node and software configuration as a 'quick-launch' option to get your work up and running quickly. For simplicity, minimal options are provided - these apps are not intended for complex configuration/customization scenarios.
After you a submit an interactive app to the queue, Gateway will track and manage the session. Once it starts, you may connect and disconnect from the session in your browser, leaving the job running while you log out of your browser.
Each of the available apps are documented through the following links.
Compute Node Desktop
The Compute Node Desktop app will launch a graphical desktop session on a compute node. This is similar to using Thinlinc, however, this gives you a desktop directly on a compute node instead on a front-end. This app is useful if you have a custom application or application not directly available as an interactive app you would like to run inside Gateway.
To launch a desktop session on a compute node, select the Gautschi Compute Desktop app. From the submit form, select from the available options - the queue to which you wish to submit and the number of wallclock hours you wish to have job running. There is also a checkbox that enable a notification to your email when the job starts.
After the interactive job is submitted you will be taken to your list of active interactive app sessions. You can monitor the status of the job from here until it starts, or if you enabled the email notification, watch your Purdue email for the notification the job has started.
Once it is indicated the job has started you can connect to the desktop with the "Launch noVNC in New Tab" button. The session will be terminated after the wallclock hours you specified have elapsed or you terminate the session early with the "Delete" button from the list of sessions. Deleting the session when you are finished will free up queue resources for your lab mates and other users on the system.
MATLAB
The MATLAB app will launch a MATLAB session on a compute node and allow you to connect directly to it in a web browser.
To launch a MATLAB session on a compute node, select the MATLAB app. From the submit form, select from the available options - the version of MATLAB you are interested in running, the queue to which you wish to submit, and the number of wallclock hours you wish to have job running. There is also a checkbox that enable a notification to your email when the job starts.
After the interactive job is submitted you will be taken to your list of active interactive app sessions. You can monitor the status of the job from here until it starts, or if you enabled the email notification, watch your Purdue email for the notification the job has started.
Once it is indicated the job has started you can connect to the desktop with the "Launch noVNC in New Tab" button. The session will be terminated after the wallclock hours you specified have elapsed or you terminate the session early with the "Delete" button from the list of sessions. Deleting the session when you are finished will free up queue resources for your lab mates and other users on the system.
Jupyter Notebook
The Notebook app will launch a Notebook session on a compute node and allow you to connect directly to it in a web browser.
To launch a Notebook session on a compute node, select the Notebook app. From the submit form, select from the available options:
- Queue: This is a dropdown menu from which you can select a queue from all of the queues to which you have permission to submit.
- Walltime: This is a field which expects a number and represents how many hours you want to keep the session running. Note that this value should not exceed the maximum value given next to the selected queue name from the queue dropdown menu.
- Number of Cores/GPUs: This is a field which expects a number and represents the number of your resources your session is requesting. Note that the amount of memory allocated for your session is proportional to the number of cores or GPUs that you request for your job, so if your session is running out of memory, consider increasing this value.
- Use Jupyter Lab: This is a checkbox which, when checked, will run Jupyter Lab instead of Jupyter Notebook. Both of these applications are interfaces to Jupyter, and you can launch Jupyter notebooks from within Jupyter Lab. Jupyter Notebook is more "barebones" while Jupyter Lab has additional features such as the ability to interact with additional file types.
- E-mail Notice: This is a checkbox which, when checked, will send you an e-mail notification to your Purdue e-mail that your session is ready when the scheduler has found resources to dedicate to your session.
After the interactive job is submitted you will be taken to your list of active interactive app sessions. You can monitor the status of the job from here until it starts, or if you enabled the email notification, watch your Purdue email for the notification the job has started.
Once it is indicated the job has started you can connect to the desktop with the "Connect to Jupyter" button. Once connected, you can create new notebooks, selecting the currently available Anaconda versions available as modules, and any personally created Notebook kernels.
Often times you may want to use one of your existing Anaconda environments within your Jupyter session to use libraries specific to your workflow. In order to do so, you must ensure that the Anaconda environment you want to use contains the Python packages "IPyKernel" and "IPython" which are packages that are required by Jupyter. When you create a Jupyter session, Open OnDemand will check through your existing Anaconda environments and create a Jupyter kernel for any Anaconda environment that contains these two packages, and you will be able to select to use that kernel from within the application.
The session will be terminated after the number of hours you specified have elapsed or you terminate the session early with the "Delete" button from the list of sessions. Deleting the session when you are finished will free up queue resources for your lab mates and other users on the system.
RStudio Server
The RStudio app will launch a RStudio session on a compute node and allow you to connect directly to it in a web browser.
To launch a RStudio session on a compute node, select the RStudio app. From the submit form, select from the available options - the queue to which you wish to submit, and the number of wallclock hours you wish to have job running. There is also a checkbox that enable a notification to your email when the job starts.
After the interactive job is submitted you will be taken to your list of active interactive app sessions. You can monitor the status of the job from here until it starts, or if you enabled the email notification, watch your Purdue email for the notification the job has started.
Once it is indicated the job has started you can connect to the desktop with the "Connect to RStudio Server" button. The session will be terminated after the wallclock hours you specified have elapsed or you terminate the session early with the "Delete" button from the list of sessions. Deleting the session when you are finished will free up queue resources for your lab mates and other users on the system.
Compiling Source Code
Documentation on compiling source code on Gautschi.
Compiling GPU Programs
The Gautschi cluster nodes contain 6 GPUs that support CUDA and OpenCL. See the detailed hardware overview for the specifics on the GPUs in Gautschi. This section focuses on using CUDA.
A simple CUDA program has a basic workflow:
- Initialize an array on the host (CPU).
- Copy array from host memory to GPU memory.
- Apply an operation to array on GPU.
- Copy array from GPU memory to host memory.
Here is a sample CUDA program:
Both front-ends and GPU-enabled compute nodes have the CUDA tools and libraries available to compile CUDA programs. To compile a CUDA program, load CUDA, and use nvcc to compile the program:
$ module load gcc/11.4.1 cuda/12.6.0
$ nvcc gpu_hello.cu -o gpu_hello
./gpu_hello
No GPU specified, using first GPUhello, world
The example illustrates only how to copy an array between a CPU and its GPU but does not perform a serious computation.
The following program times three square matrix multiplications on a CPU and on the global and shared memory of a GPU:
$ module load cuda
$ nvcc mm.cu -o mm
$ ./mm 0
speedup
-------
Elapsed time in CPU: 6555.2 milliseconds
Elapsed time in GPU (global memory): 32.9 milliseconds 199.1
Elapsed time in GPU (shared memory): 3.0 milliseconds 2191.8
For best performance, the input array or matrix must be sufficiently large to overcome the overhead in copying the input and output data to and from the GPU.
For more information about NVIDIA, CUDA, and GPUs:
Compiling Hybrid Programs
A hybrid program combines both MPI and shared-memory to take advantage of compute clusters with multi-core compute nodes. Libraries for OpenMPI and Intel MPI (IMPI) and compilers which include OpenMP for C, C++, and Fortran are available.
Language | Header Files |
---|---|
Fortran 77 |
|
Fortran 90 |
|
Fortran 95 |
|
C |
|
C++ |
|
A few examples illustrate hybrid programs with task parallelism of OpenMP:
This example illustrates a hybrid program with loop-level (data) parallelism of OpenMP:
To see the available MPI libraries:
$ module avail impi
$ module avail openmpi
The following tables illustrate how to compile your hybrid (MPI/OpenMP) program. Any compiler flags accepted by Intel ifort/icc compilers are compatible with their respective MPI compiler.
Language | Command |
---|---|
Fortran 77 |
|
Fortran 90 |
|
Fortran 95 |
|
C |
|
C++ |
|
Language | Command |
---|---|
Fortran 77 |
|
Fortran 90 |
|
Fortran 95 |
|
C |
|
C++ |
|
The Intel and GNU compilers will not output anything for a successful compilation. Also, the Intel compiler does not recognize the suffix .f95
.
Compiling Serial Programs
A serial program is a single process which executes as a sequential stream of instructions on one processor core. Compilers capable of serial programming are available for C, C++, and versions of Fortran.
Here are a few sample serial programs:
- serial_hello.f
- serial_hello.f90
- serial_hello.f95
- serial_hello.c
-
To load a compiler, enter one of the following:
$ module load intel
$ module load gcc
Language | Intel Compiler | GNU Compiler | |
---|---|---|---|
Fortran 77 |
|
|
|
Fortran 90 |
|
|
|
Fortran 95 |
|
|
|
C |
|
|
|
C++ |
|
|
The Intel and GNU compilers will not output anything for a successful compilation. Also, the Intel compiler does not recognize the suffix ".f95".
Compiling MPI Programs
OpenMPI and Intel MPI (IMPI) are implementations of the Message-Passing Interface (MPI) standard. Libraries for these MPI implementations and compilers for C, C++, and Fortran are available on all clusters.
Language | Header Files |
---|---|
Fortran 77 |
|
Fortran 90 |
|
Fortran 95 |
|
C |
|
C++ |
|
Here are a few sample programs using MPI:
To see the available MPI libraries:
$ module avail openmpi
$ module avail impi
Language | Intel MPI | OpenMPI |
---|---|---|
Fortran 77 |
|
|
Fortran 90 |
|
|
Fortran 95 |
|
|
C |
|
|
C++ |
|
|
The Intel and GNU compilers will not output anything for a successful compilation. Also, the Intel compiler does not recognize the suffix ".f95".
Here is some more documentation from other sources on the MPI libraries:
Compiling OpenMP Programs
All compilers installed on Brown include OpenMP functionality for C, C++, and Fortran. An OpenMP program is a single process that takes advantage of a multi-core processor and its shared memory to achieve a form of parallel computing called multithreading. It distributes the work of a process over processor cores in a single compute node without the need for MPI communications.
Language | Header Files |
---|---|
Fortran 77 |
|
Fortran 90 |
|
Fortran 95 |
|
C |
|
C++ |
|
Sample programs illustrate task parallelism of OpenMP:
A sample program illustrates loop-level (data) parallelism of OpenMP:
To load a compiler, enter one of the following:
$ module load intel
$ module load gcc
Language | Intel Compiler | GNU Compiler |
---|---|---|
Fortran 77 |
|
|
Fortran 90 |
|
|
Fortran 95 |
|
|
C |
|
|
C++ |
|
|
The Intel and GNU compilers will not output anything for a successful compilation. Also, the Intel compiler does not recognize the suffix ".f95".
Here is some more documentation from other sources on OpenMP:
Frequently Asked Questions
Some common questions, errors, and problems are categorized below. Click the Expand Topics link in the upper right to see all entries at once. You can also use the search box above to search the user guide for any issues you are seeing.About Gautschi
Frequently asked questions about Gautschi.
Can you remove me from the Gautschi mailing list?
Your subscription in the Gautschi mailing list is tied to your account on Gautschi. If you are no longer using your account on Gautschi, your account can be deleted from the My Accounts page. Hover over the resource you wish to remove yourself from and click the red 'X' button. Your account and mailing list subscription will be removed overnight. Be sure to make a copy of any data you wish to keep first.
How is Gautschi different than other Community Clusters?
Gautschi differs from the previous Community Clusters in many significant aspects:
- Gautschi has a unique sales protocol. While previous clusters were available for sale based on compute resources, Gautschi is sold based on compute resource hours.
- Gautschi has eight NVIDIA H100 GPUs, each with 80 GB of GPU memory.
Do I need to do anything to my firewall to access Gautschi?
No firewall changes are needed to access Gautschi. However, to access data through Network Drives (i.e., CIFS, "Z: Drive"), you must be on a Purdue campus network or connected through VPN.
Does Gautschi have the same home directory as other clusters?
The Gautschi home directory and its contents are exclusive to Gautschi cluster front-end hosts and compute nodes. This home directory is not available on other RCAC machines but Gautschi. There is no automatic copying or synchronization between home directories.
At your discretion you can manually copy all or parts of your main research computing home to Gautschi using one of the suggested methods.
If you plan to use hsi
or htar
commands to access Fortress tape archive from Gautschi, please see also the keytab generation question for a temporary workaround to a potential caveat, while a permanent mitigation is being developed.
How should I launch common GUI applications on Gautschi?
When our users access Gautschi via ThinLinc, they can follow these steps to launch an interactive jobs.
- In the upper left corner, the user can click on Applications, then Cluster Software, where multiple software options are listed with interactive SLURM jobs.
- The GUI launcher starts with a window that prompts the user to select the desired version of the software to launch and guides them through the job submission process.
- The GUI launcher also makes it easy for users to view available accounts, the maximum wall times for each account, and the available computing resources with multiple help options at the bottom.
- After choosing the partition, the user is prompted to provide the computing resource they need for their job to run while adjusting the memory for them automatically.
- After requesting the resources the job will be submitted and waiting for slurm to allocate the computing resources.
Software
Frequently asked questions about software.
Cannot use pip after loading ml-toolkit modules
Neither pip nor ml-toolkit are available on Gautschi, although they are available on the other community clusters.
Gateway (Open OnDemand)
Gautschi's Gateway is an open-source HPC portal developed by the Ohio Supercomputing Center. Open OnDemand allows one to interact with HPC resources through a web browser and easily manage files, submit jobs, and interact with graphical applications directly in a browser, all with no software to install. Gautschi has an instance of OnDemand available that can be accessed via gateway.gautschi.rcac.purdue.edu.
Link to section 'Logging In' of 'Gateway (Open OnDemand)' Logging In
To log into Gateway:
- Navigate to gateway.gautschi.rcac.purdue.edu
- Log in using your Career account username and Purdue Login Duo client.
On the splash page you will see a quota usage report. If you are over 90% on any of your quotas a warning will be displayed. This information will update every 10-15 minutes while you are active on Gateway.
Link to section 'Apps' of 'Gateway (Open OnDemand)' Apps
There are a number of built-in apps in Gateway that can be accessed from the top menu bar. Below are links to documentation on each app.
Files
The Files app will let you access your files in your Home Directory, Scratch, and Data Depot spaces. The app lets you manage create, manage, and delete files and directories from your web browser. Navigate by double clicking on folders in the file explorer or by using the file tree on the left.
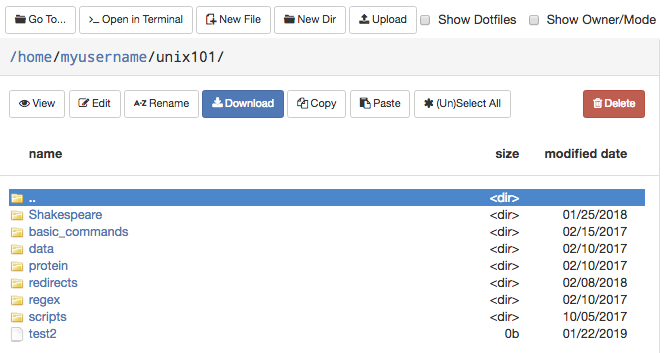
On the top row, there are buttons to:
- Go To: directly input a directory to navigate to
- Open in Terminal: launches the Shell app and navigates you to the current directory in the terminal
- New File: creates a new, empty file
- New Dir: creates a new, empty directory
- Upload: upload a file from your computer
Note: File uploads from your browser are limited to 100 GB per file. Be mindful that uploads over a few gigabytes may be unreliable through your browser, especially from off-campus connections. For very large files or off-campus transfers alternative methods such as Globus are highly recommended.
The second row of buttons lets you perform typical file management operations. The Edit button will open files in a fully fledged browser based text editor - it features syntax highlighting and vim and Emacs key bindings.
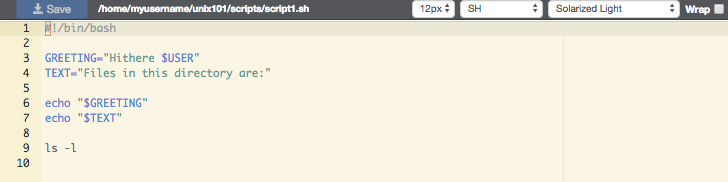
Jobs
There are two apps under the Jobs apps: Active Jobs and Job Composer. These are detailed below.
Link to section 'Active Jobs' of 'Jobs' Active Jobs
This shows you active SLURM jobs currently on the cluster. The default view will show you your current jobs, similar to squeue -u rices
. Using the button labeled "Your Jobs" in the upper right allows you to select different filters by queue (account). All accounts output by slist
will appear for you here. Using the arrow on the left hand side will expand the full job details.
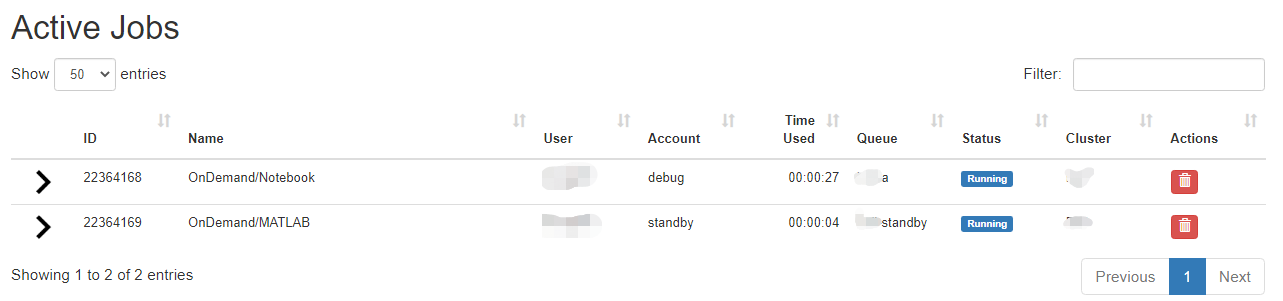
Link to section 'Job Composer' of 'Jobs' Job Composer
The Job Composer app allows you to create and submit jobs to the cluster. You can select from pre-defined templates (most of these are taken from the User Guide examples) or you can create your own templates for frequently used workflows.
Link to section 'Creating Job from Existing Template' of 'Jobs' Creating Job from Existing Template
Click "New Job" menu, then select "From Template":
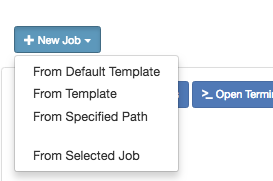
Then select from one of the available templates.
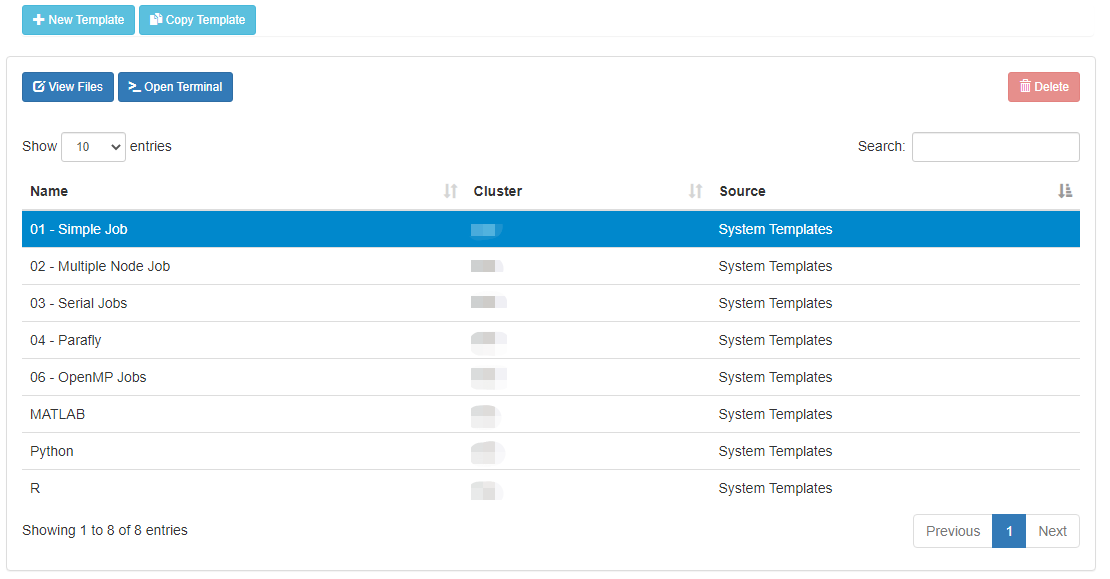
Click 'Create New Job' in second pane.
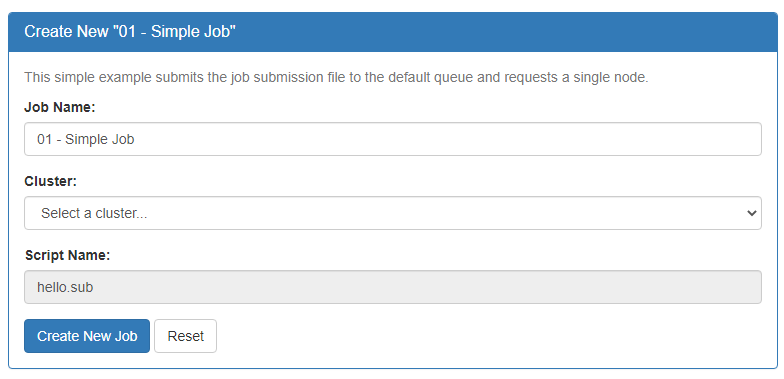
Your new job should be selected in your list of jobs. In the 'Submit Script' pane you can see the job script that was generated with an 'Open Editor' link to open the script in the built-in editor. Open the file in the editor and edit the script as necessary. By default the job will specify standby queue - this should be changed as appropriate, along with the node and walltime requests.
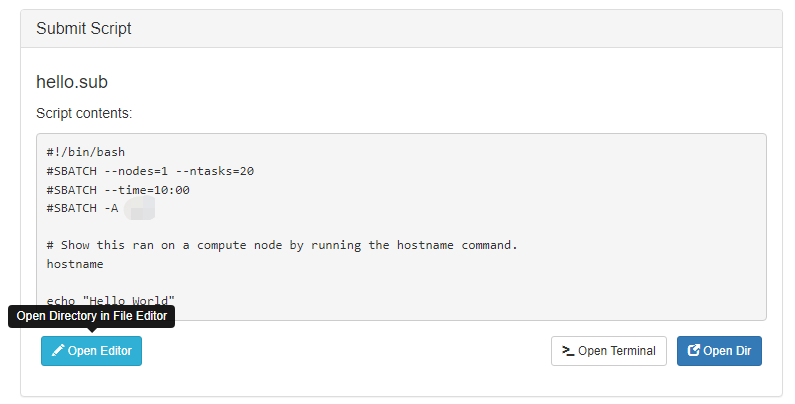
When you are finished with editing the job and are ready to submit, click the green 'Submit' button at the top of the job list. You can monitor progress from here or from the Active Jobs app. Once completed, you should see the output files appear:
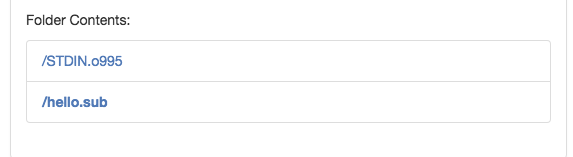
Clicking on one of the output files will open it in the file editor for your viewing.
Link to section 'Creating New Template' of 'Jobs' Creating New Template
First, prepare a template directory containing a template submission script along with any input files. Then, to import the job into the Job Composer app, click the 'Create New Template' button. Fill in the directory containing your template job script and files in the first box. Give it an appropriate name and notes.
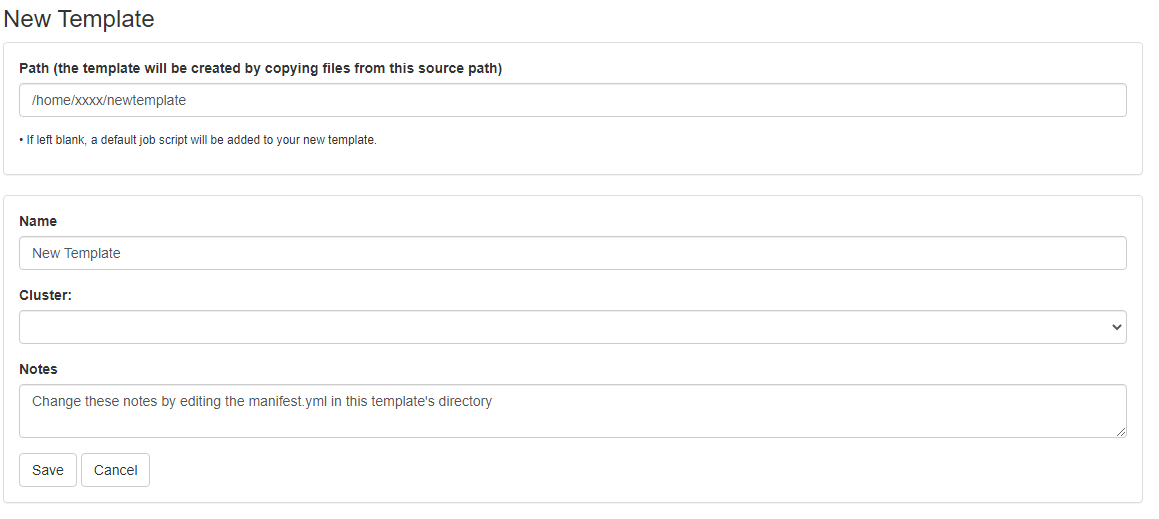
This template will now appear in your list of templates to choose from when composing jobs. You can now go create and submit a job from this new template.
Cluster Tools
The Cluster Tools menu contains cluster utilities. At the moment, only a terminal app is provided. Additional apps may be developed and provided in the future.
Link to section 'Shell Access' of 'Cluster Tools' Shell Access
Launching the shell app will provide you with a web-based terminal session on the cluster front-end. This is equivalent to using a standalone SSH client to connect to gautschi.rcac.purdue.edu
where you are connected to one several front-ends. The normal acceptable front-end use policy applies to access through the web-app. X11 Forwarding is not supported. Use of one of the interactive apps is recommended for graphical applications.
Interactive Apps
There are several interactive apps available through Gateway that can be accessed through the Interactive Apps dropdown menu. These apps are provided with a basic node and software configuration as a 'quick-launch' option to get your work up and running quickly. For simplicity, minimal options are provided - these apps are not intended for complex configuration/customization scenarios.
After you a submit an interactive app to the queue, Gateway will track and manage the session. Once it starts, you may connect and disconnect from the session in your browser, leaving the job running while you log out of your browser.
Each of the available apps are documented through the following links.
Compute Node Desktop
The Compute Node Desktop app will launch a graphical desktop session on a compute node. This is similar to using Thinlinc, however, this gives you a desktop directly on a compute node instead on a front-end. This app is useful if you have a custom application or application not directly available as an interactive app you would like to run inside Gateway.
To launch a desktop session on a compute node, select the Gautschi Compute Desktop app. From the submit form, select from the available options - the queue to which you wish to submit and the number of wallclock hours you wish to have job running. There is also a checkbox that enable a notification to your email when the job starts.
After the interactive job is submitted you will be taken to your list of active interactive app sessions. You can monitor the status of the job from here until it starts, or if you enabled the email notification, watch your Purdue email for the notification the job has started.
Once it is indicated the job has started you can connect to the desktop with the "Launch noVNC in New Tab" button. The session will be terminated after the wallclock hours you specified have elapsed or you terminate the session early with the "Delete" button from the list of sessions. Deleting the session when you are finished will free up queue resources for your lab mates and other users on the system.
MATLAB
The MATLAB app will launch a MATLAB session on a compute node and allow you to connect directly to it in a web browser.
To launch a MATLAB session on a compute node, select the MATLAB app. From the submit form, select from the available options - the version of MATLAB you are interested in running, the queue to which you wish to submit, and the number of wallclock hours you wish to have job running. There is also a checkbox that enable a notification to your email when the job starts.
After the interactive job is submitted you will be taken to your list of active interactive app sessions. You can monitor the status of the job from here until it starts, or if you enabled the email notification, watch your Purdue email for the notification the job has started.
Once it is indicated the job has started you can connect to the desktop with the "Launch noVNC in New Tab" button. The session will be terminated after the wallclock hours you specified have elapsed or you terminate the session early with the "Delete" button from the list of sessions. Deleting the session when you are finished will free up queue resources for your lab mates and other users on the system.
Jupyter Notebook - Deep Neural Networks Demo (GPU)
The Notebook app will launch a Notebook session on a compute node and allow you to connect directly to it in a web browser. It can be used to run GPU applications such as Tensorflow and Keras. Below is a demo of this to get you started.
- Download the demo notebook to your computer.
- Launch a Notebook session from the Gateway Interactive Apps menu:
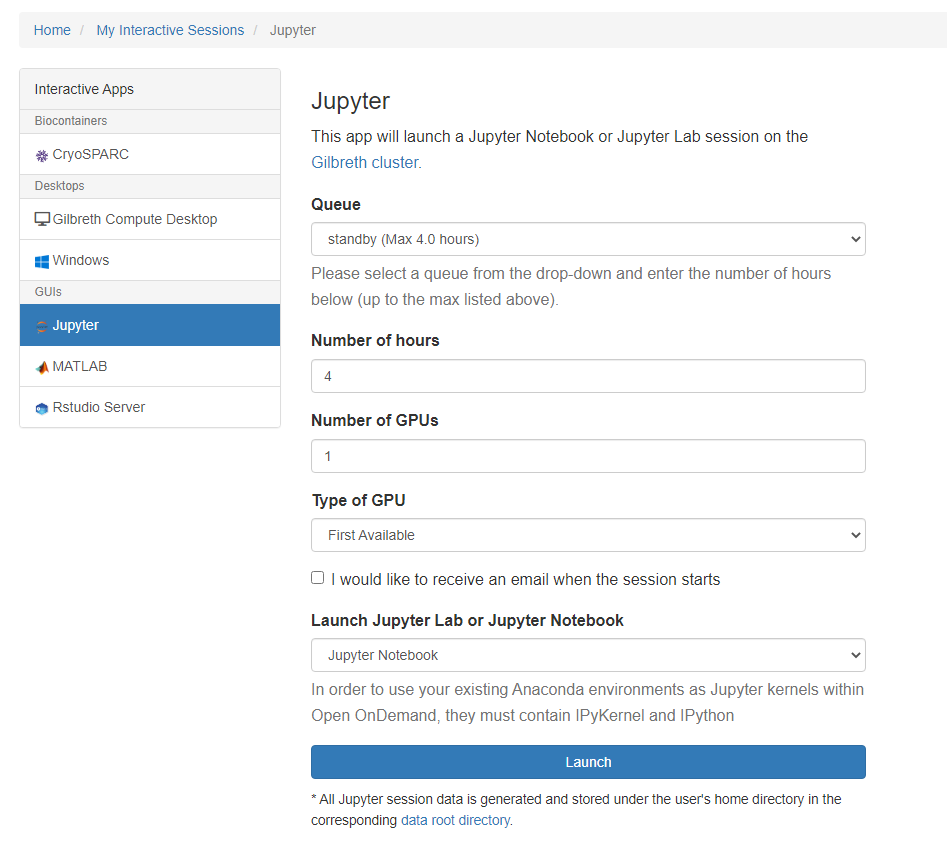
- Select the queue to which you wish to submit and enter the number of wallclock hours you require. Your notebook will be terminated after this number of hours elapses.
- Click Launch.
- Wait for your interactive session to change to Running state. This may take some time depending on how busy the queue and system is.
- Click on 'Connect to Jupyter' once the button appears.
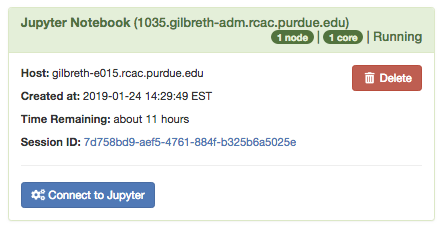
- Once in Jupyter, select 'Upload' in the upper right corner. You may wish to create a folder or change into a different directory to put the demo notebook first.
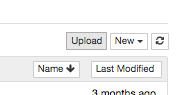
- Select the demo notebook file you downloaded earlier. Click the blue Upload button to complete the upload. Then click the
dnn.ipynb
item from the file list to launch the notebook. - You should now have the notebook loaded and you should be able to re-execute the code cells, or modify them to your needs.
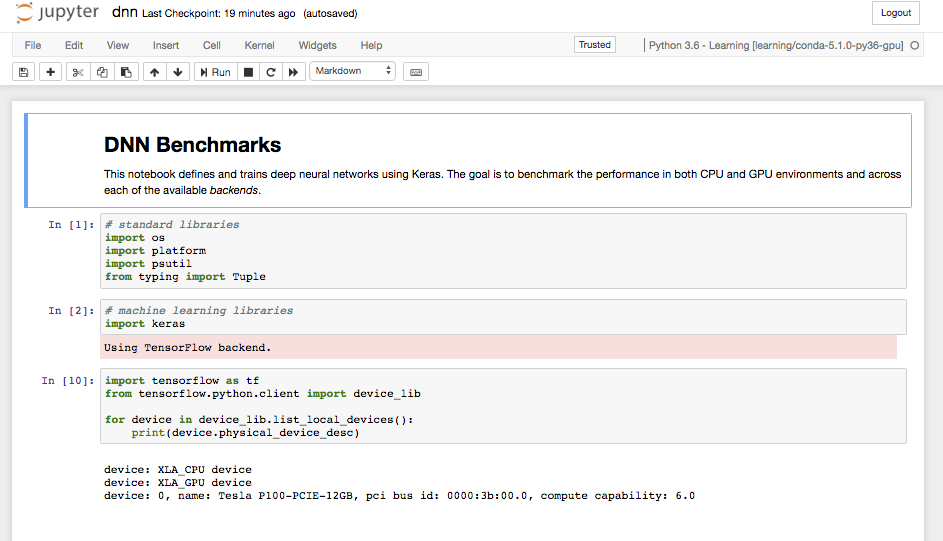
Jupyter Notebook
The Notebook app will launch a Notebook session on a compute node and allow you to connect directly to it in a web browser.
To launch a Notebook session on a compute node, select the Notebook app. From the submit form, select from the available options:
- Queue: This is a dropdown menu from which you can select a queue from all of the queues to which you have permission to submit.
- Walltime: This is a field which expects a number and represents how many hours you want to keep the session running. Note that this value should not exceed the maximum value given next to the selected queue name from the queue dropdown menu.
- Number of Cores/GPUs: This is a field which expects a number and represents the number of your resources your session is requesting. Note that the amount of memory allocated for your session is proportional to the number of cores or GPUs that you request for your job, so if your session is running out of memory, consider increasing this value.
- Use Jupyter Lab: This is a checkbox which, when checked, will run Jupyter Lab instead of Jupyter Notebook. Both of these applications are interfaces to Jupyter, and you can launch Jupyter notebooks from within Jupyter Lab. Jupyter Notebook is more "barebones" while Jupyter Lab has additional features such as the ability to interact with additional file types.
- E-mail Notice: This is a checkbox which, when checked, will send you an e-mail notification to your Purdue e-mail that your session is ready when the scheduler has found resources to dedicate to your session.
After the interactive job is submitted you will be taken to your list of active interactive app sessions. You can monitor the status of the job from here until it starts, or if you enabled the email notification, watch your Purdue email for the notification the job has started.
Once it is indicated the job has started you can connect to the desktop with the "Connect to Jupyter" button. Once connected, you can create new notebooks, selecting the currently available Anaconda versions available as modules, and any personally created Notebook kernels.
Often times you may want to use one of your existing Anaconda environments within your Jupyter session to use libraries specific to your workflow. In order to do so, you must ensure that the Anaconda environment you want to use contains the Python packages "IPyKernel" and "IPython" which are packages that are required by Jupyter. When you create a Jupyter session, Open OnDemand will check through your existing Anaconda environments and create a Jupyter kernel for any Anaconda environment that contains these two packages, and you will be able to select to use that kernel from within the application.
The session will be terminated after the number of hours you specified have elapsed or you terminate the session early with the "Delete" button from the list of sessions. Deleting the session when you are finished will free up queue resources for your lab mates and other users on the system.
RStudio Server
The RStudio app will launch a RStudio session on a compute node and allow you to connect directly to it in a web browser.
To launch a RStudio session on a compute node, select the RStudio app. From the submit form, select from the available options - the queue to which you wish to submit, and the number of wallclock hours you wish to have job running. There is also a checkbox that enable a notification to your email when the job starts.
After the interactive job is submitted you will be taken to your list of active interactive app sessions. You can monitor the status of the job from here until it starts, or if you enabled the email notification, watch your Purdue email for the notification the job has started.
Once it is indicated the job has started you can connect to the desktop with the "Connect to RStudio Server" button. The session will be terminated after the wallclock hours you specified have elapsed or you terminate the session early with the "Delete" button from the list of sessions. Deleting the session when you are finished will free up queue resources for your lab mates and other users on the system.
Windows Desktop
The Windows Desktop app will launch a Windows desktop session on a compute node. This is similar to using the Windows menu launcher through Thinlinc, however, this gives you a Windows desktop directly on a compute node instead on a front-end.
To launch a Windows session on a compute node, select the Windows Desktop app. From the submit form, select from the available options - choose from the basic Windows configuration or the GIS configured image, the queue to which you wish to submit, and the number of wallclock hours you wish to have job running. There is also a checkbox that enable a notification to your email when the job starts.
This will create a file in your scratch space called windows-base.qcow2
or windows-gis.qcow2
. If the file already exists, the existing image will be restarted. You can delete or rename the image at any time through the Files App to generate a fresh image. You can only have one instance of the image running at a time or corruption will occur. There are lock files to prevent this, but be mindful of this restriction. It is also recommended you make periodic backups of the image if you are making any modifications to it.
After the interactive job is submitted you will be taken to your list of active interactive app sessions. You can monitor the status of the job from here until it starts, or if you enabled the email notification, watch your Purdue email for the notification the job has started.
Once it is indicated the job has started you can connect to the desktop with the "Launch noVNC in New Tab" button. The session will be terminated after the wallclock hours you specified have elapsed or you terminate the session early with the "Delete" button from the list of sessions. Deleting the session when you are finished will free up queue resources for your lab mates and other users on the system.
Datasets
Please refer to our Federated Datasets Documentation website for up-to-date datasets on Anvil and instructions on how to use them.
Datasets
-iGenomes
To make the use of reference genomes easier, Illumina has developed a centralized resource called iGenomes. The most commonly used reference genome files are organized in a consistent structure for multiple genomes.
We have downloaded a copy of iGenomes onto our clusters, and developed them into environment modules to make them easy to use.
Link to section 'AGPv3' of 'iGenomes' AGPv3
Link to section 'Module' of 'iGenomes' Module
You can load the modules by:
module load datasets
module load igenomes/AGPv3
Link to section 'BDGP6' of 'iGenomes' BDGP6
Link to section 'Module' of 'iGenomes' Module
You can load the modules by:
module load datasets
module load igenomes/BDGP6
Link to section 'bosTau8' of 'iGenomes' bosTau8
Link to section 'Module' of 'iGenomes' Module
You can load the modules by:
module load datasets
module load igenomes/bosTau8
Link to section 'canFam3' of 'iGenomes' canFam3
The contents of the annotation directories were downloaded from UCSC on: July 17, 2015. SmallRNA annotation files were downloaded from miRBase release 21.Link to section 'Module' of 'iGenomes' Module
You can load the modules by:
module load datasets
module load igenomes/canFam3
Link to section 'CanFam3.1' of 'iGenomes' CanFam3.1
The contents of the annotation directories were downloaded from Ensembl on: July 17, 2015. Gene annotation files were downloaded from Ensembl release 81. SmallRNA annotation files were downloaded from miRBase release 21.Link to section 'Module' of 'iGenomes' Module
You can load the modules by:
module load datasets
module load igenomes/CanFam3.1
Link to section 'ce10' of 'iGenomes' ce10
The contents of the annotation directories were downloaded from UCSC on: July 17, 2015. SmallRNA annotation files were downloaded from miRBase release 21.Link to section 'Module' of 'iGenomes' Module
You can load the modules by:
module load datasets
module load igenomes/ce10
Link to section 'CHIMP2.1.4' of 'iGenomes' CHIMP2.1.4
The contents of the annotation directories were downloaded from Ensembl on: July 17, 2015. Gene annotation files were downloaded from Ensembl release 81. SmallRNA annotation files were downloaded from miRBase release 21.Link to section 'Module' of 'iGenomes' Module
You can load the modules by:
module load datasets
module load igenomes/CHIMP2.1.4
Link to section 'danRer10' of 'iGenomes' danRer10
Link to section 'Module' of 'iGenomes' Module
You can load the modules by:
module load datasets
module load igenomes/danRer10
Link to section 'dm6' of 'iGenomes' dm6
Link to section 'Module' of 'iGenomes' Module
You can load the modules by:
module load datasets
module load igenomes/dm6
Link to section 'EB1' of 'iGenomes' EB1
The contents of the annotation directories were downloaded from Ensembl on: July 17, 2015. Gene annotation files were downloaded from EnsemblGenomes release 27. Ensembl now uses the name 'ASM1942v1, June 2008' for this assembly.Link to section 'Module' of 'iGenomes' Module
You can load the modules by:
module load datasets
module load igenomes/EB1
Link to section 'EB2' of 'iGenomes' EB2
The contents of the annotation directories were downloaded from Ensembl on: July 17, 2015. Gene annotation files were downloaded from EnsemblGenomes release 27.Link to section 'Module' of 'iGenomes' Module
You can load the modules by:
module load datasets
module load igenomes/EB2
Link to section 'EF2' of 'iGenomes' EF2
The contents of the annotation directories were downloaded from Ensembl on: July 17, 2015. Annotation files in this directory and subdirectories were downloaded from EnsemblGenomes release 27. Ensembl now uses the name 'ASM294v2, May 2009' for this assembly.Link to section 'Module' of 'iGenomes' Module
You can load the modules by:
module load datasets
module load igenomes/EF2
Link to section 'equCab2' of 'iGenomes' equCab2
The contents of the annotation directories were downloaded from UCSC on: July 17, 2015. SmallRNA annotation files were downloaded from miRBase release 21.Link to section 'Module' of 'iGenomes' Module
You can load the modules by:
module load datasets
module load igenomes/equCab2
Link to section 'EquCab2' of 'iGenomes' EquCab2
The contents of the annotation directories were downloaded from Ensembl on: July 17, 2015. Gene annotation files were downloaded from Ensembl release 81. SmallRNA annotation files were downloaded from miRBase release 21.Link to section 'Module' of 'iGenomes' Module
You can load the modules by:
module load datasets
module load igenomes/EquCab2
Link to section 'galGal4' of 'iGenomes' galGal4
The contents of the annotation directories were downloaded from UCSC on: July 17, 2015. SmallRNA annotation files were downloaded from miRBase release 21.Link to section 'Module' of 'iGenomes' Module
You can load the modules by:
module load datasets
module load igenomes/galGal4
Link to section 'Galgal4' of 'iGenomes' Galgal4
Link to section 'Module' of 'iGenomes' Module
You can load the modules by:
module load datasets
module load igenomes/Galgal4
Link to section 'Gm01' of 'iGenomes' Gm01
The contents of the annotation directories were downloaded from Ensembl on: July 17, 2015. Gene annotation files were downloaded from EnsemblGenomes release 27. Ensembl now uses the name 'V1.0,' for this assembly. SmallRNA annotation files were downloaded from miRBase release 21.Link to section 'Module' of 'iGenomes' Module
You can load the modules by:
module load datasets
module load igenomes/Gm01
Link to section 'GRCh37' of 'iGenomes' GRCh37
The contents of the annotation directories were downloaded from Ensembl on: July 17, 2015. Gene annotation files were downloaded from Ensembl release 75. SmallRNA annotation files were downloaded from miRBase release 21.Link to section 'Module' of 'iGenomes' Module
You can load the modules by:
module load datasets
module load igenomes/GRCh37
Link to section 'GRCh38' of 'iGenomes' GRCh38
Link to section 'Module' of 'iGenomes' Module
You can load the modules by:
module load datasets
module load igenomes/GRCh38
Link to section 'GRCm38' of 'iGenomes' GRCm38
The contents of the annotation directories were downloaded from Ensembl on: July 17, 2015. Gene annotation files were downloaded from Ensembl release 81. SmallRNA annotation files were downloaded from miRBase release 21.Link to section 'Module' of 'iGenomes' Module
You can load the modules by:
module load datasets
module load igenomes/GRCm38
Link to section 'GRCz10' of 'iGenomes' GRCz10
Link to section 'Module' of 'iGenomes' Module
You can load the modules by:
module load datasets
module load igenomes/GRCz10
Link to section 'hg19' of 'iGenomes' hg19
The contents of the annotation directories were downloaded from UCSC on: July 17, 2015. SmallRNA annotation files were downloaded from miRBase release 21.Link to section 'Module' of 'iGenomes' Module
You can load the modules by:
module load datasets
module load igenomes/hg19
Link to section 'hg38' of 'iGenomes' hg38
Link to section 'Module' of 'iGenomes' Module
You can load the modules by:
module load datasets
module load igenomes/hg38
Link to section 'IRGSP-1.0' of 'iGenomes' IRGSP-1.0
Link to section 'Module' of 'iGenomes' Module
You can load the modules by:
module load datasets
module load igenomes/IRGSP-1.0
Link to section 'mm10' of 'iGenomes' mm10
The contents of the annotation directories were downloaded from UCSC on: July 17, 2015. SmallRNA annotation files were downloaded from miRBase release 21.Link to section 'Module' of 'iGenomes' Module
You can load the modules by:
module load datasets
module load igenomes/mm10
Link to section 'Mmul_1' of 'iGenomes' Mmul_1
The contents of the annotation directories were downloaded from Ensembl on: July 17, 2015. Gene annotation files were downloaded from Ensembl release 81. SmallRNA annotation files were downloaded from miRBase release 21.Link to section 'Module' of 'iGenomes' Module
You can load the modules by:
module load datasets
module load igenomes/Mmul_1
Link to section 'panTro4' of 'iGenomes' panTro4
The contents of the annotation directories were downloaded from UCSC on: July 17, 2015. SmallRNA annotation files were downloaded from miRBase release 21.Link to section 'Module' of 'iGenomes' Module
You can load the modules by:
module load datasets
module load igenomes/panTro4
Link to section 'R64-1-1' of 'iGenomes' R64-1-1
Link to section 'Module' of 'iGenomes' Module
You can load the modules by:
module load datasets
module load igenomes/R64-1-1
Link to section 'rn6' of 'iGenomes' rn6
Link to section 'Module' of 'iGenomes' Module
You can load the modules by:
module load datasets
module load igenomes/rn6
Link to section 'Rnor_5.0' of 'iGenomes' Rnor_5.0
Link to section 'Module' of 'iGenomes' Module
You can load the modules by:
module load datasets
module load igenomes/Rnor_5.0
Link to section 'Rnor_6.0' of 'iGenomes' Rnor_6.0
Link to section 'Module' of 'iGenomes' Module
You can load the modules by:
module load datasets
module load igenomes/Rnor_6.0
Link to section 'sacCer3' of 'iGenomes' sacCer3
The contents of this directory were downloaded from UCSC on: March 6, 2013 local modroot="/depot/itap/datasets/igenomes/Saccharomyces_cerevisiae/UCSC/sacCer3"Link to section 'Module' of 'iGenomes' Module
You can load the modules by:
module load datasets
module load igenomes/sacCer3
Link to section 'Sbi1' of 'iGenomes' Sbi1
The contents of the annotation directories were downloaded from Ensembl on: July 17, 2015. Gene annotation files were downloaded from EnsemblGenomes release 27. Ensembl now uses the name 'Sorbi1, Dec 2007' for this assembly. SmallRNA annotation files were downloaded from miRBase release 21.Link to section 'Module' of 'iGenomes' Module
You can load the modules by:
module load datasets
module load igenomes/Sbi1
Link to section 'Sscrofa10.2' of 'iGenomes' Sscrofa10.2
The contents of the annotation directories were downloaded from Ensembl on: July 17, 2015. Gene annotation files were downloaded from Ensembl release 81. SmallRNA annotation files were downloaded from miRBase release 21.Link to section 'Module' of 'iGenomes' Module
You can load the modules by:
module load datasets
module load igenomes/Sscrofa10.2
Link to section 'susScr3' of 'iGenomes' susScr3
The contents of the annotation directories were downloaded from UCSC on: July 17, 2015. SmallRNA annotation files were downloaded from miRBase release 21.Link to section 'Module' of 'iGenomes' Module
You can load the modules by:
module load datasets
module load igenomes/susScr3
Link to section 'TAIR10' of 'iGenomes' TAIR10
The contents of the annotation directories were downloaded from Ensembl on: July 17, 2015. Gene annotation files were downloaded from EnsemblGenomes release 27. SmallRNA annotation files were downloaded from miRBase release 21.Link to section 'Module' of 'iGenomes' Module
You can load the modules by:
module load datasets
module load igenomes/TAIR10
Link to section 'UMD3.1' of 'iGenomes' UMD3.1
The contents of the annotation directories were downloaded from Ensembl on: July 17, 2015. Gene annotation files were downloaded from Ensembl release 81. SmallRNA annotation files were downloaded from miRBase release 21.Link to section 'Module' of 'iGenomes' Module
You can load the modules by:
module load datasets
module load igenomes/UMD3.1
Link to section 'WBcel235' of 'iGenomes' WBcel235
Link to section 'Module' of 'iGenomes' Module
You can load the modules by:
module load datasets
module load igenomes/WBcel235
Software Catalog
-Compilers
Developer | Module name | C | C++ | Fortran |
---|---|---|---|---|
AMD | aocc | clang | clang | flang |
GNU | gcc | gcc | g++ | gfortran |
INTEL | intel | icc | icpc | ifort |
INTEL | intel-oneapi-compilers | icx | icpx | ifx |
NVIDIA | nvhpc | nvcc | nvc++ | nvfortran |
aocc
Link to section 'Description' of 'aocc' Description
The AOCC compiler system is a high performance, production quality code generation tool. The AOCC environment provides various options to developers when building and optimizing C, C++, and Fortran applications targeting 32-bit and 64-bit Linux® platforms.
Link to section 'Versions' of 'aocc' Versions
- Bell: 2.1.0
- Anvil: 3.1.0
Link to section 'Module' of 'aocc' Module
You can load the modules by:
module load aocc
gcc
Link to section 'Description' of 'gcc' Description
The GNU Compiler Collection includes front ends for C, C++, Objective-C, Fortran, Ada, and Go, as well as libraries for these languages.
Link to section 'Versions' of 'gcc' Versions
- Bell: 4.8.5, 6.3.0, 9.3.0, 10.2.0, 12.3.0
- Scholar: 4.8.5, 6.3.0, 9.3.0, 12.3.0
- Gilbreth: 4.8.5, 6.3.0, 9.3.0, 12.3.0
- Negishi: 8.5.0, 11.2.0, 12.2.0
- Anvil: 8.4.1, 10.2.0, 11.2.0
- Gautschi: 11.4.1, 14.1.0
Link to section 'Module' of 'gcc' Module
You can load the modules by:
module load gcc
Link to section 'Compiling Serial Programs' of 'gcc' Compiling Serial Programs
A serial program is a single process which executes as a sequential stream of instructions on one processor core. Compilers capable of serial programming are available for C, C++, and versions of Fortran.
Here are a few sample serial programs:
- serial_hello.f
- serial_hello.f90
- serial_hello.f95
- serial_hello.c
-
The following table illustrates how to compile your serial program: Language GCC Fortran 77 $ gfortran myprogram.f -o myprogram
Fortran 90 $ gfortran myprogram.f90 -o myprogram
Fortran 95 $ gfortran myprogram.f95 -o myprogram
C $ gcc myprogram.c -o myprogram
C++ $ g++ myprogram.cpp -o myprogram
intel
Link to section 'Description' of 'intel' Description
Intel Parallel Studio.
Link to section 'Versions' of 'intel' Versions
- Bell: 17.0.1.132, 19.0.5.281
- Scholar: 16.0.1.150, 17.0.1.132, 18.0.1.163, 19.0.3.199
- Gilbreth: 17.0.1.132, 19.0.5.281
- Negishi: 19.1.3.304
- Anvil: 19.0.5.281, 19.1.3.304, 2024.1
- Gautschi: 2024.1.0
Link to section 'Module' of 'intel' Module
You can load the modules by:
module load intel
Link to section 'Compiling serial programs' of 'intel' Compiling serial programs
A serial program is a single process which executes as a sequential stream of instructions on one processor core. Compilers capable of serial programming are available for C, C++, and versions of Fortran.
Here are a few sample serial programs:
- serial_hello.f
- serial_hello.f90
- serial_hello.f95
- serial_hello.c
-
The following table illustrates how to compile your serial program: Language Intel Compiler Fortran 77 $ ifort myprogram.f -o myprogram
Fortran 90 $ ifort myprogram.f90 -o myprogram
Fortran 95 $ ifort myprogram.f90 -o myprogram
C $ icc myprogram.c -o myprogram
C++ $ icc myprogram.cpp -o myprogram
The Intel compiler will not output anything for a successful compilation. Also, the Intel compiler does not recognize the suffix ".f95".
intel-oneapi-compilers
Link to section 'Description' of 'intel-oneapi-compilers' Description
icc, icpc, ifort, icx, icpx, ifx, and dpcpp.
Link to section 'Versions' of 'intel-oneapi-compilers' Versions
- Negishi: 2023.0.0, 2024.1
Link to section 'Module' of 'intel-oneapi-compilers' Module
You can load the modules by:
module load intel-oneapi-compilers
nvhpc
Link to section 'Description' of 'nvhpc' Description
The NVIDIA HPC SDK C, C++, and Fortran compilers support GPU acceleration of HPC modeling and simulation applications with standard C++ and Fortran, OpenACC® directives, and CUDA®. GPU-accelerated math libraries maximize performance on common HPC algorithms, and optimized communications libraries enable standards-based multi-GPU and scalable systems programming.
Link to section 'Homepage' of 'nvhpc' Homepage
https://developer.nvidia.com/hpc-sdk
Link to section 'Versions' of 'nvhpc' Versions
- Scholar: 22.11
- Gilbreth: 22.7
- Anvil: 21.7
Link to section 'Module' of 'nvhpc' Module
You can load the modules by:
module purge
module load nvhpc
Link to section 'Example' of 'nvhpc' Example
Below is the example to use nvcc to compile a simple hello-world cuda code.
Link to section 'Cuda code' of 'nvhpc' Cuda code
hello.cu
#include <stdio.h>
__global__ void cuda_hello(){
printf("Hello World from GPU!\n");
}
int main() {
cuda_hello<<<1,1>>>();
cudaDeviceSynchronize();
return 0;
}
</stdio.h>
Link to section 'Compile cuda code' of 'nvhpc' Compile cuda code
$ nvcc hello.cu -o hello
Link to section 'Run compiled code' of 'nvhpc' Run compiled code
$ ./hello
Hello World from GPU!
MPIs
Message Passing Interface (MPI) is a standardized and portable message-passing standard designed to function on parallel computing architectures.
impi
Link to section 'Description' of 'impi' Description
Intel MPI
Link to section 'Versions' of 'impi' Versions
- Bell: 2019.5.281
- Scholar: 2019.3.199
- Gilbreth: 2019.5.281
- Negishi: 2019.9.304
- Anvil: 2019.5.281
Link to section 'Module' of 'impi' Module
You can load the modules by:
module load intel
module load impi
intel-oneapi-mpi
Link to section 'Description' of 'intel-oneapi-mpi' Description
Intel MPI Library is a multifabric message-passing library that implements the open-source MPICH specification. Use the library to create, maintain, and test advanced, complex applications that perform better on high-performance computing HPC clusters based on Intel processors.
Link to section 'Versions' of 'intel-oneapi-mpi' Versions
- Negishi: 2021.8.0
- Gautschi: 2024.1
Link to section 'Module' of 'intel-oneapi-mpi' Module
You can load the modules by:
module load intel-oneapi-mpi
mvapich2
Link to section 'Description' of 'mvapich2' Description
Mvapich2 is a High-Performance MPI Library for clusters with diverse networks InfiniBand, Omni-Path, Ethernet/iWARP, and RoCE and computing platforms x86 Intel and AMD, ARM and OpenPOWER
Link to section 'Versions' of 'mvapich2' Versions
- Negishi: 2.3.7
- Anvil: 2.3.6
- Gautschi: 2.3.7-1
Link to section 'Module' of 'mvapich2' Module
You can load the modules by:
module load mvapich2
openmpi
Link to section 'Description' of 'openmpi' Description
An open source Message Passing Interface implementation.
Link to section 'Versions' of 'openmpi' Versions
- Bell: 3.1.4, 3.1.6, 4.0.5, 4.1.3, 4.1.5
- Scholar: 2.1.6, 3.1.6, 4.1.5
- Gilbreth: 3.1.6-gpu-cuda10, 3.1.6-gpu-cuda11, 4.1.5-gpu-cuda11, 4.1.5-gpu-cuda12
- Negishi: 4.1.4
- Anvil: 3.1.6, 4.0.6, 4.1.6
- Gautschi: 4.1.6, 5.0.5
Link to section 'Module' of 'openmpi' Module
You can load the modules by:
module load openmpi
Link to section 'Compile MPI Code' of 'openmpi' Compile MPI Code
Language | Command |
---|---|
Fortran 77 |
|
Fortran 90 |
|
Fortran 95 |
|
C |
|
C++ |
|
Link to section 'Run MPI Executables' of 'openmpi' Run MPI Executables
Create a job submission file:
#!/bin/bash
#SBATCH --nodes=2
#SBATCH --ntasks-per-node=128
#SBATCH --time=00:01:00
#SBATCH -A XXXX
srun -n 256 ./mpi_hello
SLURM can run an MPI program with the srun command. The number of processes is requested with the -n option. If you do not specify the -n option, it will default to the total number of processor cores you request from SLURM.
To run MPI executables, users can also use mpirun
or mpiexec
from openmpi. Note that mpiexec
and mpirun
are synonymous in openmpi.
mpirun -n number-of-processes [options] executable
Applications
Link to section 'AMD' of 'Applications' AMD
Link to section 'Audio/Visualization' of 'Applications' Audio/Visualization
Link to section 'Bioinformatics' of 'Applications' Bioinformatics
Link to section 'Climate' of 'Applications' Climate
Link to section 'Computational chemistry' of 'Applications' Computational chemistry
Link to section 'Container' of 'Applications' Container
Link to section 'Electrical engineering' of 'Applications' Electrical engineering
Link to section 'Fluid dynamics' of 'Applications' Fluid dynamics
Link to section 'Geospatial tools' of 'Applications' Geospatial tools
Link to section 'Libraries' of 'Applications' Libraries
- arpack-ng
- blis
- boost
- eigen
- fftw
- gmp
- ghostscript
- gsl
- hdf5
- hdf
- intel-mkl
- intel-oneapi-mkl
- intel-oneapi-tbb
- intel-rt
- libfabric
- libflame
- libiconv
- libmesh
- libpng
- libszip
- libtiff
- libv8
- libx11
- libxml2
- libxp
- libxscrnsaver
- libxslt
- mesa-glu
- mpfr
- netcdf-c
- netcdf-cxx4
- netcdf-fortran
- netcdf
- netlib-lapack
- oclfpga
- openblas
- parallel-netcdf
- petsc
- rocm
- sqlite
- swig
- ucx
- udunits2
- udunits
- util-linux-uuid
- xcb-util-image
- xcb-util-keysyms
- xcb-util-renderutil
- xcb-util-wm
- zlib
- zstd
Link to section 'Material science' of 'Applications' Material science
Link to section 'Mathematical/Statistics' of 'Applications' Mathematical/Statistics
Link to section 'ML toolkit' of 'Applications' ML toolkit
Link to section 'NVIDIA' of 'Applications' NVIDIA
Link to section 'Physics' of 'Applications' Physics
Link to section 'Programming languages' of 'Applications' Programming languages
Link to section 'System' of 'Applications' System
Link to section 'Text Editors' of 'Applications' Text Editors
Link to section 'Tools/Utilities' of 'Applications' Tools/Utilities
Link to section 'Workflow automation' of 'Applications' Workflow automation
abaqus
Link to section 'Description' of 'abaqus' Description
Abaqus is a software suite for Finite Element Analysis (FEA) developed by Dassault Systèmes.
Link to section 'Versions' of 'abaqus' Versions
- Bell: 2019, 2020, 2021, 2022, 2023
- Scholar: 2024
- Gilbreth: 2017, 2018, 2019, 2020, 2021, 2022
- Negishi: 2022, 2023, 2024
Link to section 'Module' of 'abaqus' Module
You can load the modules by:
module load abaqus
amber
Link to section 'Description' of 'amber' Description
AMBER (Assisted Model Building with Energy Refinement) is a package of molecular simulation programs.
Link to section 'Versions' of 'amber' Versions
- Bell: 16
- Gilbreth: 16
- Negishi: 20
- Anvil: 20
Link to section 'Module' of 'amber' Module
You can load the modules by:
module load amber
amdblis
Link to section 'Description' of 'amdblis' Description
AMD Optimized BLIS. BLIS is a portable software framework for instantiating high-performance BLAS-like dense linear algebra libraries.
Link to section 'Versions' of 'amdblis' Versions
- Anvil: 3.0
Link to section 'Module' of 'amdblis' Module
You can load the modules by:
module load amdblis
amdfftw
Link to section 'Description' of 'amdfftw' Description
FFTW AMD Optimized version is a comprehensive collection of fast C routines for computing the Discrete Fourier Transform DFT and various special cases thereof.
Link to section 'Versions' of 'amdfftw' Versions
- Anvil: 3.0
Link to section 'Module' of 'amdfftw' Module
You can load the modules by:
module load amdfftw
amdlibflame
Link to section 'Description' of 'amdlibflame' Description
libFLAME AMD Optimized version is a portable library for dense matrix computations, providing much of the functionality present in Linear Algebra Package LAPACK. It includes a compatibility layer, FLAPACK, which includes complete LAPACK implementation.
Link to section 'Versions' of 'amdlibflame' Versions
- Anvil: 3.0
Link to section 'Module' of 'amdlibflame' Module
You can load the modules by:
module load amdlibflame
amdlibm
Link to section 'Description' of 'amdlibm' Description
AMD LibM is a software library containing a collection of basic math functions optimized for x86-64 processor-based machines. It provides many routines from the list of standard C99 math functions. Applications can link into AMD LibM library and invoke math functions instead of compilers math functions for better accuracy and performance.
Link to section 'Versions' of 'amdlibm' Versions
- Anvil: 3.0
Link to section 'Module' of 'amdlibm' Module
You can load the modules by:
module load amdlibm
amdscalapack
Link to section 'Description' of 'amdscalapack' Description
ScaLAPACK is a library of high-performance linear algebra routines for parallel distributed memory machines. It depends on external libraries including BLAS and LAPACK for Linear Algebra computations.
Link to section 'Versions' of 'amdscalapack' Versions
- Anvil: 3.0
Link to section 'Module' of 'amdscalapack' Module
You can load the modules by:
module load amdscalapack
anaconda
Link to section 'Description' of 'anaconda' Description
Anaconda is a free and open-source distribution of the Python and R programming languages for scientific computing, that aims to simplify package management and deployment.
Link to section 'Versions' of 'anaconda' Versions
- Bell: 2019.10-py27, 2020.02-py37, 2020.11-py38, 2024.02-py311
- Gilbreth: 2019.10-py27, 2020.02-py37, 2020.11-py38, 2024.02-py311, 5.1.0-py27, 5.1.0-py36, 5.3.1-py27, 5.3.1-py37
- Negishi: 2021.05-py38, 2022.10-py39, 2024.02-py311
- Anvil: 2021.05-py38, 2024.02-py311
Link to section 'Module' of 'anaconda' Module
You can load the modules by:
module load anaconda
ansys
Link to section 'Description' of 'ansys' Description
Ansys is a CAE/multiphysics engineering simulation software that utilizes finite element analysis for numerically solving a wide variety of mechanical problems. The software contains a list of packages and can simulate many structural properties such as strength, toughness, elasticity, thermal expansion, fluid dynamics as well as acoustic and electromagnetic attributes.
Link to section 'Versions' of 'ansys' Versions
- Bell: 2019R3, 2020R1, 2021R2, 2022R1, 2022R2, 2023R1, 2023R2
- Scholar: 2022R1
- Gilbreth: 19.2, 2019R3, 2020R1, 2021R2, 2022R1, 2023R1, 2023R2
- Negishi: 2022R2, 2023R1, 2023R2, 2024R2
- Gautschi: 2024R2
Link to section 'Ansys Licensing' of 'ansys' Ansys Licensing
The Ansys licensing on our community clusters is maintained by Purdue ECN group. There are two types of licenses: teaching and research. For more information, please refer to ECN Ansys licensing page. If you are interested in purchasing your own research license, please send email to software@ecn.purdue.edu.
Link to section 'Ansys Workflow' of 'ansys' Ansys Workflow
Ansys software consists of several sub-packages such as Workbench and Fluent. Most simulations are performed using the Ansys Workbench console, a GUI interface to manage and edit the simulation workflow. It requires X11 forwarding for remote display so a SSH client software with X11 support or a remote desktop portal is required. Please see Logging In section for more details. To ensure preferred performance, ThinLinc remote desktop connection is highly recommended.
Typically users break down larger structures into small components in geometry with each of them modeled and tested individually. A user may start by defining the dimensions of an object, adding weight, pressure, temperature, and other physical properties.
Ansys Fluent is a computational fluid dynamics (CFD) simulation software known for its advanced physics modeling capabilities and accuracy. Fluent offers unparalleled analysis capabilities and provides all the tools needed to design and optimize new equipment and to troubleshoot existing installations.
In the following sections, we provide step-by-step instructions to lead you through the process of using Fluent. We will create a classical elbow pipe model and simulate the fluid dynamics when water flows through the pipe. The project files have been generated and can be downloaded via fluent_tutorial.zip.
Link to section 'Loading Ansys Module' of 'ansys' Loading Ansys Module
Different versions of Ansys are installed on the clusters and can be listed with module spider
or module avail
command in the terminal.
$ module avail ansys/
---------------------- Core Applications -----------------------------
ansys/2019R3 ansys/2020R1 ansys/2021R2 ansys/2022R1 (D)
Before launching Ansys Workbench, a specific version of Ansys module needs to be loaded. For example, you can module load ansys/2021R2
to use the latest Ansys 2021R2. If no version is specified, the default module -> (D) (ansys/2022R1
in this case) will be loaded. You can also check the loaded modules with module list
command.
Link to section 'Launching Ansys Workbench' of 'ansys' Launching Ansys Workbench
Open a terminal, enter rcac-runwb2
to launch Ansys Workbench.
You can also use runwb2
to launch Ansys Workbench. The main difference between runwb2
and rcac-runwb2
is that the latter sets the project folder to be in your scratch space. Ansys has an known bug that it might crash when the project folder is set to $HOME
on our systems.
ansysem
Link to section 'Description' of 'ansysem' Description
This module enables the use of ANSYSEM, a popular Electromechanical application.
Link to section 'Versions' of 'ansysem' Versions
- Bell: 2020r1, 2021r2
- Scholar: 2021r2
Link to section 'Module' of 'ansysem' Module
You can load the modules by:
module load ansysem
aocl
Link to section 'Description' of 'aocl' Description
AOCL are a set of numerical libraries tuned specifically for AMD EPYCTM processor family. They have a simple interface to take advantage of the latest hardware innovations.
Link to section 'Versions' of 'aocl' Versions
- Bell: 2.1
Link to section 'Module' of 'aocl' Module
You can load the modules by:
module load aocl
arpack-ng
Link to section 'Description' of 'arpack-ng' Description
ARPACK-NG is a collection of Fortran77 subroutines designed to solve large scale eigenvalue problems.
Link to section 'Versions' of 'arpack-ng' Versions
- Negishi: 3.8.0
- Anvil: 3.8.0
Link to section 'Module' of 'arpack-ng' Module
You can load the modules by:
module load arpack-ng
aws-cli
Link to section 'Description' of 'aws-cli' Description
The AWS Command Line Interface CLI is a unified tool to manage your AWS services from command line.
Link to section 'Versions' of 'aws-cli' Versions
- Bell: 2.4.15
- Scholar: 2.4.15
- Gilbreth: 2.4.15
- Negishi: 2.9.7
- Anvil: 2.4.15
Link to section 'Module' of 'aws-cli' Module
You can load the modules by:
module load aws-cli
bamtools
Link to section 'Description' of 'bamtools' Description
C++ API & command-line toolkit for working with BAM data.
Link to section 'Versions' of 'bamtools' Versions
- Anvil: 2.5.1, 2.5.2
- Bell: 2.5.1
- Negishi: 2.5.1
- Scholar: 2.5.1
- Gautschi: 2.5.1
Link to section 'Commands' of 'bamtools' Commands
- bamtools
Link to section 'Module' of 'bamtools' Module
You can load the modules by:
module load bamtools
Link to section 'Example job' of 'bamtools' Example job
To run bamtools our our clusters:
#!/bin/bash
#SBATCH -A Allocation # Allocation name
#SBATCH -t 20:00:00
#SBATCH -N 1
#SBATCH -n 24
#SBATCH -p PartitionName
#SBATCH --job-name=bamtools
#SBATCH --mail-type=FAIL,BEGIN,END
#SBATCH --error=%x-%J-%u.err
#SBATCH --output=%x-%J-%u.out
module load bamtools
bamtools convert -format fastq -in in.bam -out out.fastq
bbftp
Link to section 'Description' of 'bbftp' Description
bbFTP is a file transfer software. It implements its own transfer protocol, which is optimized for large files (larger than 2GB) and secure as it does not read the password in a file and encrypts the connection information.
Link to section 'Versions' of 'bbftp' Versions
- Bell: 3.2.1
Link to section 'Module' of 'bbftp' Module
You can load the modules by:
module load bbftp
beagle
Link to section 'Description' of 'beagle' Description
Beagle is a software package for phasing genotypes and for imputing ungenotyped markers.
Link to section 'Versions' of 'beagle' Versions
- Anvil: 5.1
- Bell: 5.1
- Negishi: 5.1
- Scholar: 5.1_24Aug19.3e8
- Gautschi: 5.1_24Aug19.3e8
Link to section 'Commands' of 'beagle' Commands
- beagle
Link to section 'Module' of 'beagle' Module
You can load the modules by:
module load beagle
Link to section 'Example job' of 'beagle' Example job
To run Beagle on our clusters:
#!/bin/bash
#SBATCH -A myAllocation
#SBATCH -t 1:00:00
#SBATCH -N 1
#SBATCH -n 1
#SBATCH --job-name=beagle
#SBATCH --mail-type=FAIL,BEGIN,END
#SBATCH --error=%x-%J-%u.err
#SBATCH --output=%x-%J-%u.out
module load beagle
beagle gt=test.vcf.gz out=test.out
beast2
Link to section 'Description' of 'beast2' Description
BEAST is a cross-platform program for Bayesian inference using MCMC of molecular sequences. It is entirely orientated towards rooted, time-measured phylogenies inferred using strict or relaxed molecular clock models. It can be used as a method of reconstructing phylogenies but is also a framework for testing evolutionary hypotheses without conditioning on a single tree topology.
Link to section 'Versions' of 'beast2' Versions
- Anvil: 2.6.3, 2.6.4, 2.6.6
- Bell: 2.6.3, 2.6.4, 2.6.6
- Negishi: 2.6.3, 2.6.4, 2.6.6
- Scholar: 2.6.3, 2.6.4, 2.6.6
- Gautschi: 2.6.3, 2.6.4, 2.6.6
Link to section 'Commands' of 'beast2' Commands
- applauncher
- beast
- beauti
- densitree
- loganalyser
- logcombiner
- packagemanager
- treeannotator
Link to section 'Module' of 'beast2' Module
You can load the modules by:
module load beast2
Link to section 'Example job' of 'beast2' Example job
To run BEAST 2 on our clusters:
#!/bin/bash
#SBATCH -A myQueue
#SBATCH -t 1:00:00
#SBATCH -N 1
#SBATCH -n 4
#SBATCH --job-name=beast2
#SBATCH --mail-type=FAIL,BEGIN,END
#SBATCH --error=%x-%J-%u.err
#SBATCH --output=%x-%J-%u.out
module load beast2
beast -threads 4 -prefix input input.xml
bismark
Link to section 'Description' of 'bismark' Description
A tool to map bisulfite converted sequence reads and determine cytosine methylation states
Link to section 'Versions' of 'bismark' Versions
- Anvil: 0.23.0, 0.24.0
- Bell: 0.23.0, 0.24.0
- Negishi: 0.23.0, 0.24.0
- Scholar: 0.23.0, 0.24.0
- Gautschi: 0.23.0, 0.24.0
Link to section 'Commands' of 'bismark' Commands
- bam2nuc
- bismark
- bismark2bedGraph
- bismark2report
- bismark2summary
- bismark_genome_preparation
- bismark_methylation_extractor
- coverage2cytosine
- deduplicate_bismark
- filter_non_conversion
- NOMe_filtering
Link to section 'Module' of 'bismark' Module
You can load the modules by:
module load bismark
Link to section 'Example job' of 'bismark' Example job
To run Bismark on our clusters:
#!/bin/bash
#SBATCH -A myAllocation # Allocation name
#SBATCH -t 1:00:00
#SBATCH -N 1
#SBATCH -n 12
#SBATCH --job-name=bismark
#SBATCH --mail-type=FAIL,BEGIN,END
#SBATCH --error=%x-%J-%u.err
#SBATCH --output=%x-%J-%u.out
module load bismark
bismark_genome_preparation --bowtie2 data/ref_genome
bismark --multicore 12 --genome data/ref_genome seq.fastq
blast-plus
Link to section 'Description' of 'blast-plus' Description
Basic Local Alignment Search Tool. BLAST finds regions of similarity between biological sequences. The program compares nucleotide or protein sequences to sequence databases and calculates the statistical significance.
Link to section 'Versions' of 'blast-plus' Versions
- Anvil: 2.12.0
Link to section 'Module' of 'blast-plus' Module
You can load the modules by:
module load blast-plus
Link to section 'BLAST Databases' of 'blast-plus' BLAST Databases
Local copies of the blast dabase can be found in the directory /anvil/datasets/ncbi/blast/latest
. The environment varialbe BLASTDB
was also set as /anvil/datasets/ncbi/blast/latest
. If users want to use cdd_delta
, env_nr
, env_nt
, nr
, nt
, pataa
, patnt
, pdbnt
, refseq_protein
, refseq_rna
, swissprot
, or tsa_nt
databases, do not need to provide the database path. Instead, just use the format like this -db nr
.
Link to section 'Example job' of 'blast-plus' Example job
To run bamtools our our clusters:
#!/bin/bash
#SBATCH -A myallocation # Allocation name
#SBATCH -t 20:00:00
#SBATCH -N 1
#SBATCH -n 24
#SBATCH -p PartitionName
#SBATCH --job-name=blast
#SBATCH --mail-type=FAIL,BEGIN,END
#SBATCH --error=%x-%J-%u.err
#SBATCH --output=%x-%J-%u.out
module load blast-plus
blastp -query protein.fasta -db nr -out test_out -num_threads 4
blis
Link to section 'Description' of 'blis' Description
BLIS is a portable software framework for instantiating high-performance BLAS-like dense linear algebra libraries.
Link to section 'Versions' of 'blis' Versions
- Negishi: 0.8.1, 0.9.0
- Anvil: 0.8.1
Link to section 'Module' of 'blis' Module
You can load the modules by:
module load blis
boost
Link to section 'Description' of 'boost' Description
Boost provides free peer-reviewed portable C++ source libraries, emphasizing libraries that work well with the C++ Standard Library.
Link to section 'Versions' of 'boost' Versions
- Bell: 1.68.0, 1.70.0, 1.80.0
- Scholar: 1.85.0
- Gilbreth: 1.66.0, 1.70.0, 1.79.0
- Negishi: 1.79.0, 1.80.0
- Anvil: 1.74.0
- Gautschi: 1.85.0
Link to section 'Module' of 'boost' Module
You can load the modules by:
module load boost
bowtie2
Link to section 'Description' of 'bowtie2' Description
Bowtie 2 is an ultrafast and memory-efficient tool for aligning sequencing reads to long reference sequences
Link to section 'Versions' of 'bowtie2' Versions
- Anvil: 2.4.2, 2.5.1
- Bell: 2.4.2, 2.5.1
- Negishi: 2.4.2, 2.5.1
- Scholar: 2.4.2, 2.5.1
- Gautschi: 2.4.2, 2.5.1
Link to section 'Module' of 'bowtie2' Module
You can load the modules by:
module load bowtie2
Link to section 'Example job' of 'bowtie2' Example job
To run Bowtie 2 on our clusters:
#!/bin/bash
#SBATCH -A myallocation # Allocation name
#SBATCH -t 1:00:00
#SBATCH -N 1
#SBATCH -n 1
#SBATCH --job-name=bowtie2
#SBATCH --mail-type=FAIL,BEGIN,END
#SBATCH --error=%x-%J-%u.err
#SBATCH --output=%x-%J-%u.out
module load bowtie2
bowtie2-build ref.fasta ref
bowtie2 -p 4 -x ref -1 input_1.fq -2 input_2.fq -S test.sam
bwa
Link to section 'Description' of 'bwa' Description
Burrow-Wheeler Aligner for pairwise alignment between DNA sequences.
Link to section 'Versions' of 'bwa' Versions
- Anvil: 0.7.17
- Bell: 0.7.17
- Negishi: 0.7.17
- Scholar: 0.7.17
- Gautschi: 0.7.17
Link to section 'Module' of 'bwa' Module
You can load the modules by:
module load bwa
Link to section 'Example job' of 'bwa' Example job
To run BWA on our clusters:
#!/bin/bash
#SBATCH -A myallocation # Allocation name
#SBATCH -t 1:00:00
#SBATCH -N 1
#SBATCH -n 1
#SBATCH --job-name=bwa
#SBATCH --mail-type=FAIL,BEGIN,END
#SBATCH --error=%x-%J-%u.err
#SBATCH --output=%x-%J-%u.out
module load bwa
bwa index ref.fasta
bwa mem ref.fasta input.fq > test.sam
bzip2
Link to section 'Description' of 'bzip2' Description
bzip2 is a freely available, patent free high-quality data compressor. It typically compresses files to within 10% to 15% of the best available techniques the PPM family of statistical compressors, whilst being around twice as fast at compression and six times faster at decompression.
Link to section 'Versions' of 'bzip2' Versions
- Bell: 1.0.8
- Gilbreth: 1.0.8
- Negishi: 1.0.8
- Anvil: 1.0.8
Link to section 'Module' of 'bzip2' Module
You can load the modules by:
module load bzip2
caffe
Link to section 'Description' of 'caffe' Description
Caffe is a deep learning framework made with expression, speed, and modularity in mind.
Link to section 'Versions' of 'caffe' Versions
- Bell: 1.0
- Gilbreth: 1.0.0
Link to section 'Module' of 'caffe' Module
You can load the modules by:
module load learning
module load caffe
cdo
Link to section 'Description' of 'cdo' Description
CDO is a collection of command line Operators to manipulate and analyse Climate and NWP model Data.
Link to section 'Versions' of 'cdo' Versions
- Bell: 1.9.5
- Scholar: 2.3.0
- Gilbreth: 1.9.5
- Negishi: 1.9.9
- Anvil: 1.9.9
- Gautschi: 2.3.0
Link to section 'Module' of 'cdo' Module
You can load the modules by:
module load cdo
cmake
Link to section 'Description' of 'cmake' Description
A cross-platform, open-source build system. CMake is a family of tools designed to build, test and package software.
Link to section 'Versions' of 'cmake' Versions
- Bell: 3.18.2, 3.20.6, 3.23.1, 3.30.1
- Scholar: 3.26.5
- Gilbreth: 3.15.4, 3.20.6
- Negishi: 3.23.1, 3.24.3
- Anvil: 3.20.0
- Gautschi: 3.30.2, 3.30.2
Link to section 'Module' of 'cmake' Module
You can load the modules by:
module load cmake
cntk
Link to section 'Description' of 'cntk' Description
The Microsoft Cognitive Toolkit is a unified deep-learning toolkit that describes neural networks as a series of computational steps via a directed graph.
Link to section 'Versions' of 'cntk' Versions
- Gilbreth: 2.6
Link to section 'Module' of 'cntk' Module
You can load the modules by:
module load learning
module load cntk
comsol
Link to section 'Description' of 'comsol' Description
Comsol Multiphysics (previously named Femlab) is a modeling package for the simulation of any physical process you can describe with partial differential equations (PDEs).
Link to section 'Versions' of 'comsol' Versions
- Bell: 5.3a, 5.4, 5.5_b359, 5.6, 6.0, 6.1, 6.2
- Scholar: 6.2
- Negishi: 6.1, 6.2
- Gautschi: 6.2
Link to section 'Module' of 'comsol' Module
You can load the modules by:
module load comsol
cp2k
Link to section 'Description' of 'cp2k' Description
CP2K is a quantum chemistry and solid state physics software package that can perform atomistic simulations of solid state, liquid, molecular, periodic, material, crystal, and biological systems
Link to section 'Versions' of 'cp2k' Versions
- Negishi: 20210311, 2022.1, 8.2
- Anvil: 8.2
- Bell: 20210311, 8.2
Link to section 'Module' of 'cp2k' Module
You can load the modules by:
module load cp2k
cplex
Link to section 'Description' of 'cplex' Description
IBM ILOG CPLEX Optimizer's mathematical programming technology enables decision optimization for improving efficiency, reducing costs, and increasing profitability.
Link to section 'Versions' of 'cplex' Versions
- Bell: 12.8.0
Link to section 'Module' of 'cplex' Module
You can load the modules by:
module load cplex
cuda
Link to section 'Description' of 'cuda' Description
CUDA is a parallel computing platform and programming model invented by NVIDIA. It enables dramatic increases in computing performance by harnessing the power of the graphics processing unit (GPU).
Link to section 'Versions' of 'cuda' Versions
- Scholar: 12.1.0
- Gilbreth: 8.0.61, 9.0.176, 10.0.130, 10.2.89, 11.0.3, 11.2.0, 11.7.0, 12.1.1
- Anvil: 11.0.3, 11.2.2, 11.4.2, 12.0.1
- Gautschi: 12.6.0
Link to section 'Module' of 'cuda' Module
You can load the modules by:
module load cuda
Link to section 'Monitor Activity and Drivers' of 'cuda' Monitor Activity and Drivers
Users can check the available GPUs, their current usage, installed version of the nvidia drivers, and running processes with the command nvidia-smi
. The output should look something like this:
User@gilbreth-fe00:~/cuda $ nvidia-smi
Sat May 27 23:26:14 2023
+-----------------------------------------------------------------------------+
| NVIDIA-SMI 515.48.07 Driver Version: 515.48.07 CUDA Version: 11.7 |
|-------------------------------+----------------------+----------------------+
| GPU Name Persistence-M| Bus-Id Disp.A | Volatile Uncorr. ECC |
| Fan Temp Perf Pwr:Usage/Cap| Memory-Usage | GPU-Util Compute M. |
| | | MIG M. |
|===============================+======================+======================|
| 0 NVIDIA A30 Off | 00000000:21:00.0 Off | 0 |
| N/A 29C P0 29W / 165W | 19802MiB / 24576MiB | 0% Default |
| | | Disabled |
+-------------------------------+----------------------+----------------------+
+-----------------------------------------------------------------------------+
| Processes: |
| GPU GI CI PID Type Process name GPU Memory |
| ID ID Usage |
|=============================================================================|
| 0 N/A N/A 29152 C python 9107MiB |
| 0 N/A N/A 53947 C ...020.11-py38/GP/bin/python 2611MiB |
| 0 N/A N/A 71769 C ...020.11-py38/GP/bin/python 1241MiB |
| 0 N/A N/A 72821 C ...8/TorchGPU_env/bin/python 2657MiB |
| 0 N/A N/A 91986 C ...2-4/internal/bin/gdesmond 931MiB |
+-----------------------------------------------------------------------------+
We can see that the node gilbreth-fe00
is running driver version 515.48.07 and is compatible with CUDA version 11.7. We do not recommend users to run jobs on front end nodes, but here we can see there are three python processes and one gdesmond process.
Link to section 'Compile a CUDA code' of 'cuda' Compile a CUDA code
The below vectorAdd.cu is modified from the textbook Learn CUDA Programming.
#include<stdio.h>
#include<stdlib.h>
#define N 512
void host_add(int *a, int *b, int *c) {
for(int idx=0;idx<N;idx++)
c[idx] = a[idx] + b[idx];
}
//basically just fills the array with index.
void fill_array(int *data) {
for(int idx=0;idx<N;idx++)
data[idx] = idx;
}
void print_output(int *a, int *b, int*c) {
for(int idx=0;idx<N;idx++)
printf("\n %d + %d = %d", a[idx] , b[idx], c[idx]);
}
int main(void) {
int *a, *b, *c;
int size = N * sizeof(int);
// Alloc space for host copies of a, b, c and setup input values
a = (int *)malloc(size); fill_array(a);
b = (int *)malloc(size); fill_array(b);
c = (int *)malloc(size);
host_add(a,b,c);
print_output(a,b,c);
free(a); free(b); free(c);
return 0;
}
We can compile the CUDA code by the CUDA nvcc compiler:
nvcc -o vector_addition vector_addition.cu
Link to section 'Example job script' of 'cuda' Example job script
#!/bin/bash
#SBATCH -A XXX
#SBATCH --nodes=1
#SBATCH --ntasks-per-node=20
#SBATCH --cpus-per-task=1
#SBATCH --gpus-per-node=1
#SBATCH --time 1:00:00
module purge
module load gcc/XXX
module load cuda/XXX
#compile the vector_addition.cu file
nvcc -o vector_addition vector_addition.cu
#runs the vector_addition program
./vector_addition
cudnn
Link to section 'Description' of 'cudnn' Description
cuDNN is a deep neural network library from Nvidia that provides a highly tuned implementation of many functions commonly used in deep machine learning applications.
Link to section 'Versions' of 'cudnn' Versions
- Gilbreth: cuda-8.0_6.0, cuda-8.0_7.1, cuda-9.0_7.3, cuda-9.0_7.4, cuda-10.0_7.5, cuda-10.2_8.0, cuda-11.0_8.0, cuda-11.2_8.1, cuda-11.7_8.6, cuda-12.1_8.9
- Anvil: cuda-11.0_8.0, cuda-11.2_8.1, cuda-11.4_8.2, cuda-12.0_8.8
Link to section 'Module' of 'cudnn' Module
You can load the modules by:
module load cudnn
cue-login-env
Link to section 'Description' of 'cue-login-env' Description
XSEDE Common User Environment Variables for Anvil. Load this module to have XSEDE Common User Environment variables defined for your shell session or job on Anvil. See detailed description at https://www.ideals.illinois.edu/bitstream/handle/2142/75910/XSEDE-CUE-Variable-Definitions-v1.1.pdf
Link to section 'Versions' of 'cue-login-env' Versions
- Anvil: 1.1
Link to section 'Module' of 'cue-login-env' Module
You can load the modules by:
module load cue-login-env
cufflinks
Link to section 'Description' of 'cufflinks' Description
Cufflinks assembles transcripts, estimates their abundances, and tests for differential expression and regulation in RNA-Seq samples.
Link to section 'Versions' of 'cufflinks' Versions
- Anvil: 2.2.1
- Bell: 2.2.1
- Negishi: 2.2.1
- Scholar: 2.2.1
- Gautschi: 2.2.1
Link to section 'Module' of 'cufflinks' Module
You can load the modules by:
module load cufflinks
Link to section 'Example job' of 'cufflinks' Example job
To run Cufflinks on our clusters:
#!/bin/bash
#SBATCH -A myallocation # Allocation name
#SBATCH -t 1:00:00
#SBATCH -N 1
#SBATCH -n 8
#SBATCH --job-name=cufflinks
#SBATCH --mail-type=FAIL,BEGIN,END
#SBATCH --error=%x-%J-%u.err
#SBATCH --output=%x-%J-%u.out
module load cufflinks
cufflinks -p 8 -G transcript.gtf --library-type fr-unstranded -o cufflinks_output tophat_out/accepted_hits.bam
curl
Link to section 'Description' of 'curl' Description
cURL is an open source command line tool and library for transferring data with URL syntax
Link to section 'Versions' of 'curl' Versions
- Bell: 7.63.0, 7.79.0, 8.7.1
- Scholar: 7.76.1
- Gilbreth: 7.79.0
- Negishi: 7.78.0, 7.85.0
- Anvil: 7.76.1
- Gautschi: 8.8.0
Link to section 'Module' of 'curl' Module
You can load the modules by:
module load curl
cutadapt
Link to section 'Description' of 'cutadapt' Description
Cutadapt finds and removes adapter sequences, primers, poly-A tails and other types of unwanted sequence from your high-throughput sequencing reads.
Link to section 'Versions' of 'cutadapt' Versions
- Anvil: 2.10, 3.4, 3.7
- Bell: 3.4, 3.7
- Negishi: 3.4, 3.7
- Scholar: 3.4, 3.7
- Gautschi: 3.4, 3.7
Link to section 'Module' of 'cutadapt' Module
You can load the modules by:
module load cutadapt
Link to section 'Example job' of 'cutadapt' Example job
To run Cutadapt on our clusters:
#!/bin/bash
#SBATCH -A myallocation # Allocation name
#SBATCH -t 1:00:00
#SBATCH -N 1
#SBATCH -n 1
#SBATCH --job-name=cutadapt
#SBATCH --mail-type=FAIL,BEGIN,END
#SBATCH --error=%x-%J-%u.err
#SBATCH --output=%x-%J-%u.out
module load cutadapt
cutadapt -a AACCGGTT -o output.fastq input.fastq
eigen
Link to section 'Description' of 'eigen' Description
Eigen is a C++ template library for linear algebra matrices, vectors, numerical solvers, and related algorithms.
Link to section 'Versions' of 'eigen' Versions
- Negishi: 3.3.9
- Anvil: 3.3.9
Link to section 'Module' of 'eigen' Module
You can load the modules by:
module load eigen
emacs
Link to section 'Description' of 'emacs' Description
The Emacs programmable text editor.
Link to section 'Versions' of 'emacs' Versions
- Negishi: 28.2
- Anvil: 27.2
Link to section 'Module' of 'emacs' Module
You can load the modules by:
module load emacs
envi
Link to section 'Description' of 'envi' Description
ENVI is the premier software solution for processing and analyzing geospatial imagery used by scientists, researchers, image analysts, and GIS professionals around the world.
Link to section 'Versions' of 'envi' Versions
- Bell: 5.5.2
- Gilbreth: 5.5.2
Link to section 'Module' of 'envi' Module
You can load the modules by:
module load envi
fastqc
Link to section 'Description' of 'fastqc' Description
A quality control tool for high throughput sequence data.
Link to section 'Versions' of 'fastqc' Versions
- Anvil: 0.11.9, 0.12.1
- Bell: 0.11.9, 0.12.1
- Negishi: 0.11.9, 0.12.1
- Scholar: 0.11.9, 0.12.1
- Gautschi: 0.11.9, 0.12.1
Link to section 'Module' of 'fastqc' Module
You can load the modules by:
module load fastqc
Link to section 'Example job' of 'fastqc' Example job
To run Fastqc on our clusters:
#!/bin/bash
#SBATCH -A myallocation # Allocation name
#SBATCH -t 1:00:00
#SBATCH -N 1
#SBATCH -n 4
#SBATCH --job-name=fastqc
#SBATCH --mail-type=FAIL,BEGIN,END
#SBATCH --error=%x-%J-%u.err
#SBATCH --output=%x-%J-%u.out
module load fastqc
fastqc -o fastqc_out -t 4 FASTQ1 FASTQ2
fasttree
Link to section 'Description' of 'fasttree' Description
FastTree infers approximately-maximum-likelihood phylogenetic trees from alignments of nucleotide or protein sequences. FastTree can handle alignments with up to a million of sequences in a reasonable amount of time and memory.
Link to section 'Versions' of 'fasttree' Versions
- Anvil: 2.1.10, 2.1.11
- Bell: 2.1.10, 2.1.11
- Negishi: 2.1.10, 2.1.11
- Scholar: 2.1.10, 2.1.11
- Gautschi: 2.1.10, 2.1.11
Link to section 'Module' of 'fasttree' Module
You can load the modules by:
module load fasttree
Link to section 'Example job using single CPU' of 'fasttree' Example job using single CPU
To run FastTree on our our clusters:
#!/bin/bash
#SBATCH -A myallocation # Allocation name
#SBATCH -t 20:00:00
#SBATCH -N 1
#SBATCH -n 1
#SBATCH --job-name=fasttree
#SBATCH --mail-type=FAIL,BEGIN,END
#SBATCH --error=%x-%J-%u.err
#SBATCH --output=%x-%J-%u.out
module load fasttree
FastTree alignmentfile > treefile
Link to section 'Example job using multiple CPUs' of 'fasttree' Example job using multiple CPUs
To run FastTree on our our clusters using multiple CPUs:
#!/bin/bash
#SBATCH -A myallocation # Allocation name
#SBATCH -t 20:00:00
#SBATCH -N 1
#SBATCH -n 24
#SBATCH --job-name=FastTreeMP
#SBATCH --mail-type=FAIL,BEGIN,END
#SBATCH --error=%x-%J-%u.err
#SBATCH --output=%x-%J-%u.out
module load fasttree
export OMP_NUM_THREADS=24
FastTreeMP alignmentfile > treefile
fastx-toolkit
Link to section 'fastx-toolkit' of 'fastx-toolkit' fastx-toolkit
Link to section 'Description' of 'fastx-toolkit' Description
The FASTX-Toolkit is a collection of command line tools for Short-Reads FASTA/FASTQ files preprocessing.
Link to section 'Versions' of 'fastx-toolkit' Versions
- Anvil: 0.0.14
Link to section 'Commands' of 'fastx-toolkit' Commands
- fasta_clipping_histogram.pl
- fasta_formatter
- fasta_nucleotide_changer
- fastq_masker
- fastq_quality_boxplot_graph.sh
- fastq_quality_converter
- fastq_quality_filter
- fastq_quality_trimmer
- fastq_to_fasta
- fastx_artifacts_filter
- fastx_barcode_splitter.pl
- fastx_clipper
- fastx_collapser
- fastx_nucleotide_distribution_graph.sh
- fastx_nucleotide_distribution_line_graph.sh
- fastx_quality_stats
- fastx_renamer
- fastx_reverse_complement
- fastx_trimmer
- fastx_uncollapser
Link to section 'Module' of 'fastx-toolkit' Module
You can load the modules by:
module load fastx-toolkit
Link to section 'Example job' of 'fastx-toolkit' Example job
To run FASTX-Toolkit on our clusters:
#!/bin/bash
#SBATCH -A myallocation # Allocation name
#SBATCH -t 1:00:00
#SBATCH -N 1
#SBATCH -n 1
#SBATCH --job-name=fastx_toolkit
#SBATCH --mail-type=FAIL,BEGIN,END
#SBATCH --error=%x-%J-%u.err
#SBATCH --output=%x-%J-%u.out
module load fastx_toolkit
ffmpeg
Link to section 'Description' of 'ffmpeg' Description
FFmpeg is a complete, cross-platform solution to record, convert and stream audio and video.
Link to section 'Versions' of 'ffmpeg' Versions
- Bell: 4.2.2
- Gilbreth: 4.2.1
- Negishi: 4.4.1
- Anvil: 4.2.2
Link to section 'Module' of 'ffmpeg' Module
You can load the modules by:
module load ffmpeg
fftw
Link to section 'Description' of 'fftw' Description
FFTW is a C subroutine library for computing the discrete Fourier transform DFT in one or more dimensions, of arbitrary input size, and of both real and complex data as well as of even/odd data, i.e. the discrete cosine/sine transforms or DCT/DST. We believe that FFTW, which is free software, should become the FFT library of choice for most applications.
Link to section 'Versions' of 'fftw' Versions
- Bell: 3.3.8, 3.3.10
- Scholar: 3.3.10
- Gilbreth: 3.3.7, 3.3.10
- Negishi: 3.3.10
- Anvil: 2.1.5, 3.3.8
- Gautschi: 3.3.10
Link to section 'Module' of 'fftw' Module
You can load the modules by:
module load fftw
gamess
Link to section 'Description' of 'gamess' Description
The General Atomic and Molecular Electronic Structure System (GAMESS) is a general ab initio quantum chemistry package.
Link to section 'Versions' of 'gamess' Versions
- Bell: 18.Aug.2016.R1, 30.Jun.2019.R1
- Scholar: 17.09-r2-libcchem
- Gilbreth: 30.Jun.2019, 17.09-r2-libcchem
Link to section 'Module' of 'gamess' Module
You can load the modules by:
module load gamess
gatk
Link to section 'Description' of 'gatk' Description
Genome Analysis Toolkit Variant Discovery in High-Throughput Sequencing Data
Link to section 'Versions' of 'gatk' Versions
- Anvil: 3.8, 4.1.8.1
- Bell: 3.8
- Negishi: 3.8
- Scholar: 3.8
- Gautschi: 3.8
Link to section 'Commands' of 'gatk' Commands
- gatk
Link to section 'Module' of 'gatk' Module
You can load the modules by:
module load gatk
Link to section 'Example job' of 'gatk' Example job
To run gatk our our clusters:
#!/bin/bash
#SBATCH -A myallocation # Allocation name
#SBATCH -t 20:00:00
#SBATCH -N 1
#SBATCH -n 24
#SBATCH -p PartitionName
#SBATCH --job-name=gatk
#SBATCH --mail-type=FAIL,BEGIN,END
#SBATCH --error=%x-%J-%u.err
#SBATCH --output=%x-%J-%u.out
module load gatk
gatk --java-options "-Xmx12G -XX:ParallelGCThreads=24" HaplotypeCaller -R hg38.fa -I 19P0126636WES.sorted.bam -O 19P0126636WES.HC.vcf --sample-name 19P0126636
gaussian09
Link to section 'Description' of 'gaussian09' Description
Gaussian is a general purpose computational chemistry software package initially released in 1970. It utilizes fundamental laws of quantum mechanics to predict energies, molecular structures, spectroscopic data (NMR, IR, UV) and much more advanced calculations. It provides state-of-the-art capabilities for electronic structure modeling.
Link to section 'Versions' of 'gaussian09' Versions
- Bell: E.01
- Scholar: E.01
Link to section 'Module' of 'gaussian09' Module
You can load the modules by:
module load gaussian09
gaussian16
Link to section 'Description' of 'gaussian16' Description
Gaussian is a general purpose computational chemistry software package initially released in 1970. It utilizes fundamental laws of quantum mechanics to predict energies, molecular structures, spectroscopic data (NMR, IR, UV) and much more advanced calculations. It provides state-of-the-art capabilities for electronic structure modeling.
Link to section 'Versions' of 'gaussian16' Versions
- Bell: B.01
- Scholar: B.01
- Gilbreth: A.03, B.01-gpu
- Negishi: B.01
Link to section 'Module' of 'gaussian16' Module
You can load the modules by:
module load gaussian16
gaussview
Link to section 'Description' of 'gaussview' Description
GaussView is a graphical interface used with Gaussian. It aids in the creation of Gaussian input files, enables the user to run Gaussian calculations from a graphical interface without the need for using a command line instruction, and helps in the interpretation of Gaussian output (e.g., you can use it to plot properties, animate vibrations, visualize computed spectra, etc.).
Link to section 'Versions' of 'gaussview' Versions
- Bell: 5.0.8, 6.0.16
- Scholar: 5.0.8, 6.0.16
- Gilbreth: 6.0.16
- Negishi: 6.0.16
Link to section 'Module' of 'gaussview' Module
You can load the modules by:
module load gaussview
gdal
Link to section 'Description' of 'gdal' Description
GDAL Geospatial Data Abstraction Library is a translator library for raster and vector geospatial data formats that is released under an X/MIT style Open Source license by the Open Source Geospatial Foundation. As a library, it presents a single raster abstract data model and vector abstract data model to the calling application for all supported formats. It also comes with a variety of useful command line utilities for data translation and processing.
Link to section 'Versions' of 'gdal' Versions
- Bell: 2.4.2, 3.4.2, 3.5.3, 3.5.3_sqlite3
- Scholar: 3.9.2-w7ljnyv
- Gilbreth: 2.4.2, 3.5.3, 3.5.3-grib
- Negishi: 2.4.4, 3.5.3
- Anvil: 2.4.4, 3.2.0
Link to section 'Module' of 'gdal' Module
You can load the modules by:
module load gdal
gdb
Link to section 'Description' of 'gdb' Description
GDB, the GNU Project debugger, allows you to see what is going on inside another program while it executes -- or what another program was doing at the moment it crashed.
Link to section 'Versions' of 'gdb' Versions
- Bell: 11.1
- Negishi: 11.1, 12.1
- Anvil: 11.1
Link to section 'Module' of 'gdb' Module
You can load the modules by:
module load gdb
geos
Link to section 'Description' of 'geos' Description
GEOS Geometry Engine - Open Source is a C++ port of the Java Topology Suite JTS. As such, it aims to contain the complete functionality of JTS in C++. This includes all the OpenGIS Simple Features for SQL spatial predicate functions and spatial operators, as well as specific JTS enhanced topology functions.
Link to section 'Versions' of 'geos' Versions
- Bell: 3.8.1, 3.9.4
- Gilbreth: 3.7.2, 3.9.4
- Negishi: 3.9.1
- Anvil: 3.8.1, 3.9.1
Link to section 'Module' of 'geos' Module
You can load the modules by:
module load geos
gmp
Link to section 'Description' of 'gmp' Description
GMP is a free library for arbitrary precision arithmetic, operating on signed integers, rational numbers, and floating-point numbers.
Link to section 'Versions' of 'gmp' Versions
- Bell: 6.1.2, 6.2.1, 6.3.0
- Gilbreth: 6.1.2
- Negishi: 6.2.1
- Anvil: 6.2.1
Link to section 'Module' of 'gmp' Module
You can load the modules by:
module load gmp
gmt
Link to section 'Description' of 'gmt' Description
GMT Generic Mapping Tools is an open source collection of about 80 command-line tools for manipulating geographic and Cartesian data sets including filtering, trend fitting, gridding, projecting, etc. and producing PostScript illustrations ranging from simple x-y plots via contour maps to artificially illuminated surfaces and 3D perspective views.
Link to section 'Versions' of 'gmt' Versions
- Bell: 5.4.4
- Gilbreth: 5.4.4
- Anvil: 6.1.0
- Negishi: 5.4.4, 6.2.0
Link to section 'Module' of 'gmt' Module
You can load the modules by:
module load gmt
gnuplot
Link to section 'Description' of 'gnuplot' Description
Gnuplot is a portable command-line driven graphing utility for Linux, OS/2, MS Windows, OSX, VMS, and many other platforms. The source code is copyrighted but freely distributed i.e., you don't have to pay for it. It was originally created to allow scientists and students to visualize mathematical functions and data interactively, but has grown to support many non-interactive uses such as web scripting. It is also used as a plotting engine by third-party applications like Octave. Gnuplot has been supported and under active development since 1986
Link to section 'Versions' of 'gnuplot' Versions
- Bell: 5.2.8
- Gilbreth: 5.2.7
- Negishi: 5.4.2
- Anvil: 5.4.2
Link to section 'Module' of 'gnuplot' Module
You can load the modules by:
module load gnuplot
gpaw
Link to section 'Description' of 'gpaw' Description
GPAW is a density-functional theory DFT Python code based on the projector-augmented wave PAW method and the atomic simulation environment ASE.
Link to section 'Versions' of 'gpaw' Versions
- Anvil: 21.1.0
Link to section 'Module' of 'gpaw' Module
You can load the modules by:
module load gpaw
grads
Link to section 'Description' of 'grads' Description
The Grid Analysis and Display System (GrADS) is an interactive desktop tool that is used for easy access, manipulation, and visualization of earth science data. GrADS has two data models for handling gridded and station data. GrADS supports many data file formats, including binary (stream or sequential), GRIB (version 1 and 2), NetCDF, HDF (version 4 and 5), and BUFR (for station data).
Link to section 'Versions' of 'grads' Versions
- Bell: 2.2.1
- Gilbreth: 2.2.1
- Negishi: 2.2.1
- Anvil: 2.2.1
Link to section 'Module' of 'grads' Module
You can load the modules by:
module load grads
gromacs
Link to section 'Description' of 'gromacs' Description
GROMACS GROningen MAchine for Chemical Simulations is a molecular dynamics package primarily designed for simulations of proteins, lipids and nucleic acids. It was originally developed in the Biophysical Chemistry department of University of Groningen, and is now maintained by contributors in universities and research centers across the world.
Link to section 'Versions' of 'gromacs' Versions
- Bell: 2018.4, 2019.2, 2020.3, 2022.3
- Scholar: 2018.2, 2020.2, 2021, 2021.3, 2024.3
- Gilbreth: 2018.2, 2018.4, 2019.2, 2020.2, 2021, 2021.3, 2024.1
- Negishi: 2020.3, 2022.3
- Anvil: 2019.6, 2020.2, 2021, 2021.2, 2021.3, 2024.1
- Gautschi: 2024.3
Link to section 'Module' of 'gromacs' Module
You can check available gromacs version by:
module spider gromacs
You can check how to load the gromacs module by the module's full name:
module spider gromacs/XXXX
Note: RCAC also installed some containerized gromacs modules.
To use these containerized modules, please following the instructions in the output of "module spider gromacs/XXXX"
You can load the modules by:
module load gromacs # for default version
module load gromacs/XXXX # for specific version
Link to section 'Usage' of 'gromacs' Usage
The GROMACS executable is gmx_mpi
and you can use gmx help commands
for help on a command.
For more details about how to run GROMACS, please check GROMACS.
Link to section 'Example job' of 'gromacs' Example job
#!/bin/bash
# FILENAME: myjobsubmissionfile
#SBATCH --nodes=2 # Total # of nodes
#SBATCH --ntasks=256 # Total # of MPI tasks
#SBATCH --time=1:30:00 # Total run time limit (hh:mm:ss)
#SBATCH -J myjobname # Job name
#SBATCH -o myjob.o%j # Name of stdout output file
#SBATCH -e myjob.e%j # Name of stderr error file
# Manage processing environment, load compilers and applications.
module purge
module load gcc/XXXX openmpi/XXXX # or module load intel/XXXX impi/XXXX | depends on the output of "module spider gromacs/XXXX"
module load gromacs/XXXX
module list
# Launch MPI code
gmx_mpi pdb2gmx -f my.pdb -o my_processed.gro -water spce
gmx_mpi grompp -f my.mdp -c my_processed.gro -p topol.top -o topol.tpr
srun -n $SLURM_NTASKS gmx_mpi mdrun -s topol.tpr
Link to section 'Note' of 'gromacs' Note
Using mpirun -np $SLURM_NTASKS gmx_mpi
or mpiexex -np $SLURM_NTASKS gmx_mpi
may not work for non-exclusive jobs on some clusters. Use srun -n $SLURM_NTASKS gmx_mpi
or mpirun gmx_mpi
instead. mpirun gmx_mpi
without specifying the number of ranks will automatically pick up the number of SLURM_NTASKS
and works fine.
gsl
Link to section 'Description' of 'gsl' Description
The GNU Scientific Library GSL is a numerical library for C and C++ programmers. It is free software under the GNU General Public License. The library provides a wide range of mathematical routines such as random number generators, special functions and least-squares fitting. There are over 1000 functions in total with an extensive test suite.
Link to section 'Versions' of 'gsl' Versions
- Bell: 2.4
- Gilbreth: 2.4
- Negishi: 2.4, 2.7.1
- Anvil: 2.4
Link to section 'Module' of 'gsl' Module
You can load the modules by:
module load gsl
gurobi
Link to section 'Description' of 'gurobi' Description
The Gurobi Optimizer was designed from the ground up to be the fastest, most powerful solver available for your LP, QP, QCP, and MIP MILP, MIQP, and MIQCP problems.
Link to section 'Versions' of 'gurobi' Versions
- Bell: 9.0.1, 9.5.1, 10.0.1
- Scholar: 11.0.3
- Anvil: 9.5.1
- Negishi: 10.0.1
- Gautschi: 11.0.3
Link to section 'Module' of 'gurobi' Module
You can load the modules by:
module load gurobi
gym
Link to section 'Description' of 'gym' Description
The OpenAI Gym: A toolkit for developing and comparing your reinforcement learning agents.
Link to section 'Versions' of 'gym' Versions
- Bell: 0.17.3
- Gilbreth: 0.10.9, 0.18.0
Link to section 'Module' of 'gym' Module
You can load the modules by:
module load learning
module load gym
hadoop
Link to section 'Description' of 'hadoop' Description
The Apache Hadoop software library is a framework that allows for the distributed processing of large data sets across clusters of computers using simple programming models.
Link to section 'Versions' of 'hadoop' Versions
- Bell: 2.7.7
- Gilbreth: 2.7.7
- Negishi: 3.3.2
- Anvil: 3.3.0
Link to section 'Module' of 'hadoop' Module
You can load the modules by:
module load hadoop
hdf
Link to section 'Description' of 'hdf' Description
HDF4 also known as HDF is a library and multi-object file format for storing and managing data between machines.
Link to section 'Versions' of 'hdf' Versions
- Bell: 4.2.15
- Gilbreth: 4.2.14
- Negishi: 4.2.15
- Anvil: 4.2.15
Link to section 'Module' of 'hdf' Module
You can load the modules by:
module load hdf
hdf5
Link to section 'Description' of 'hdf5' Description
HDF5 is a data model, library, and file format for storing and managing data. It supports an unlimited variety of datatypes, and is designed for flexible and efficient I/O and for high volume and complex data.
Link to section 'Versions' of 'hdf5' Versions
- Bell: 1.8.21, 1.10.6, 1.13.2
- Scholar: 1.14.3
- Gilbreth: 1.8.16, 1.10.5, 1.14.1
- Negishi: 1.13.2
- Anvil: 1.10.7
- Gautschi: 1.14.3
Link to section 'Module' of 'hdf5' Module
You can load the modules by:
module load hdf5
hpctoolkit
Link to section 'Description' of 'hpctoolkit' Description
HPCToolkit is an integrated suite of tools for measurement and analysis of program performance on computers ranging from multicore desktop systems to the nations largest supercomputers. By using statistical sampling of timers and hardware performance counters, HPCToolkit collects accurate measurements of a programs work, resource consumption, and inefficiency and attributes them to the full calling context in which they occur.
Link to section 'Versions' of 'hpctoolkit' Versions
- Negishi: 2022.05.15
- Anvil: 2021.03.01
Link to section 'Module' of 'hpctoolkit' Module
You can load the modules by:
module load hpctoolkit
hspice
Link to section 'Description' of 'hspice' Description
Hspice is a device level circuit simulator. Hspice takes a spice file as input and produces output describing the requested simulation of the circuit. It can also produce output files to be used by the AWAVES post processor.
Link to section 'Versions' of 'hspice' Versions
- Bell: 2017.12, 2019.06, 2020.12
- Negishi: 2020.12
Link to section 'Module' of 'hspice' Module
You can load the modules by:
module load hspice
htseq
Link to section 'Description' of 'htseq' Description
HTSeq is a Python package that provides infrastructure to process data from high-throughput sequencing assays.
Link to section 'Versions' of 'htseq' Versions
- Anvil: 0.11.2, 0.13.5, 1.99.2, 2.0.1, 2.0.2
- Bell: 0.13.5, 1.99.2, 2.0.1, 2.0.2
- Negishi: 0.13.5, 1.99.2, 2.0.1, 2.0.2
- Scholar: 0.13.5, 1.99.2, 2.0.1, 2.0.2, 2.0.2-py310
- Gautschi: 0.13.5, 1.99.2, 2.0.1, 2.0.2, 2.0.2-py310
Link to section 'Commands' of 'htseq' Commands
- htseq-count
- htseq-qa
Link to section 'Module' of 'htseq' Module
You can load the modules by:
module load htseq
Link to section 'Example job' of 'htseq' Example job
To run HTSeq on our clusters:
#!/bin/bash
#SBATCH -A myallocation # Allocation name
#SBATCH -t 1:00:00
#SBATCH -N 1
#SBATCH -n 1
#SBATCH --job-name=htseq
#SBATCH --mail-type=FAIL,BEGIN,END
#SBATCH --error=%x-%J-%u.err
#SBATCH --output=%x-%J-%u.out
module load htseq
htseq-count input.bam ref.gtf > test.out
hwloc
Link to section 'Description' of 'hwloc' Description
The Hardware Locality hwloc software project.
Link to section 'Versions' of 'hwloc' Versions
- Anvil: 1.11.13
Link to section 'Module' of 'hwloc' Module
You can load the modules by:
module load hwloc
hyper-shell
Link to section 'Description' of 'hyper-shell' Description
Process shell commands over a distributed, asynchronous queue.
Documentation: https://hyper-shell.readthedocs.io/en/latest/
Link to section 'Versions' of 'hyper-shell' Versions
- Bell: 1.8.3, 2.0.2, 2.4.0, 2.5.1
- Scholar: 2.5.2
- Gilbreth: 1.8.3, 2.0.2, 2.4.0, 2.5.1
- Negishi: 2.0.2, 2.1.0, 2.4.0, 2.5.1
- Anvil: 2.0.2, 2.2.0, 2.4.0, 2.5.1
- Gautschi: 2.5.2
Link to section 'Module' of 'hyper-shell' Module
You can load the modules by:
module load hyper-shell
hypre
Link to section 'Description' of 'hypre' Description
Hypre is a library of high performance preconditioners that features parallel multigrid methods for both structured and unstructured grid problems.
Link to section 'Versions' of 'hypre' Versions
- Bell: 2.18.1
Link to section 'Module' of 'hypre' Module
You can load the modules by:
module load hypre
idl
Link to section 'Description' of 'idl' Description
IDL is a data analysis language that provides powerful, core visualization and analysis functionality, and capabilities that allow data analysts and developers to leverage IDL's power in multiple software environments.
Link to section 'Versions' of 'idl' Versions
- Bell: 8.7
- Gilbreth: 8.7
Link to section 'Module' of 'idl' Module
You can load the modules by:
module load idl
intel-mkl
Link to section 'Description' of 'intel-mkl' Description
Intel Math Kernel Library (MKL) contains ScaLAPACK, LAPACK, Sparse Solver, BLAS, Sparse BLAS, CBLAS, GMP, FFTs, DFTs, VSL, VML, and Interval Arithmetic routines. MKL resides in the directory stored in the environment variable MKL_HOME, after loading a version of the Intel compiler with module.
Link to section 'Versions' of 'intel-mkl' Versions
- Bell: 2017.1.132, 2019.5.281, 2024.1
- Negishi: 2019.9.304
- Anvil: 2019.5.281, 2020.4.304
Link to section 'Module' of 'intel-mkl' Module
You can load the modules by:
module load intel-mkl
By using module load to load an Intel compiler your environment will have several variables set up to help link applications with MKL. Here are some example combinations of simplified linking options:
$ module load intel
$ echo $LINK_LAPACK
-L${MKL_HOME}/lib/intel64 -lmkl_intel_lp64 -lmkl_intel_thread -lmkl_core -liomp5 -lpthread
$ echo $LINK_LAPACK95
-L${MKL_HOME}/lib/intel64 -lmkl_lapack95_lp64 -lmkl_blas95_lp64 -lmkl_intel_lp64 -lmkl_intel_thread -lmkl_core -liomp5 -lpthread
RCAC recommends you use the provided variables to define MKL linking options in your compiling procedures. The Intel compiler modules also provide two other environment variables, LINK_LAPACK_STATIC and LINK_LAPACK95_STATIC that you may use if you need to link MKL statically.
RCAC recommends that you use dynamic linking of libguide. If so, define LD_LIBRARY_PATH such that you are using the correct version of libguide at run time. If you use static linking of libguide, then:
- If you use the Intel compilers, link in the libguide version that comes with the compiler (use the -openmp option).
- If you do not use the Intel compilers, link in the libguide version that comes with the Intel MKL above.
Here are some more documentation from other sources on the Intel MKL:
intel-oneapi-mkl
Link to section 'Description' of 'intel-oneapi-mkl' Description
Intel oneAPI Math Kernel Library Intel oneMKL; formerly Intel Math Kernel Library or Intel MKL, is a library of optimized math routines for science, engineering, and financial applications. Core math functions include BLAS, LAPACK, ScaLAPACK, sparse solvers, fast Fourier transforms, and vector math.
Link to section 'Versions' of 'intel-oneapi-mkl' Versions
- Negishi: 2023.0.0
Link to section 'Module' of 'intel-oneapi-mkl' Module
You can load the modules by:
module load intel-oneapi-mkl
intel-oneapi-tbb
Link to section 'Description' of 'intel-oneapi-tbb' Description
Intel oneAPI Threading Building Blocks oneTBB is a flexible performance library that simplifies the work of adding parallelism to complex applications across accelerated architectures, even if you are not a threading expert.
Link to section 'Versions' of 'intel-oneapi-tbb' Versions
- Negishi: 2021.8.0
Link to section 'Module' of 'intel-oneapi-tbb' Module
You can load the modules by:
module load intel-oneapi-tbb
julia
Link to section 'Description' of 'julia' Description
Julia is a flexible dynamic language, appropriate for scientific and numerical computing, with performance comparable to traditional statically-typed languages. One can write code in Julia that is nearly as fast as C. Julia features optional typing, multiple dispatch, and good performance, achieved using type inference and just-in-time (JIT) compilation, implemented using LLVM. It is multi-paradigm, combining features of imperative, functional, and object-oriented programming.
Link to section 'Versions' of 'julia' Versions
- Bell: 1.7.1, 1.8.1, 1.9.3
- Gilbreth: 1.7.1, 1.8.5, 1.9.3
- Negishi: 1.8.5, 1.9.3
- Anvil: 1.6.2, 1.9.3
- Gautschi: 1.11.1
- Scholar: 1.5.0, 2.4.2
Link to section 'Module' of 'julia' Module
You can load the modules by:
module load julia
Link to section 'Package installation' of 'julia' Package installation
Users do not have write permission to the default julia package installation destination. However, users can install packages into home directory under ~/.julia
.
Users can side step this by explicitly defining where to put julia packages:
$ export JULIA_DEPOT_PATH=$HOME/.julia
$ julia -e 'using Pkg; Pkg.add("PackageName")'
jupyter
Link to section 'Description' of 'jupyter' Description
Complete Jupyter Hub/Lab/Notebook environment.
Link to section 'Versions' of 'jupyter' Versions
- Anvil: 2.0.0
- Negishi: 3.1.1
- Gautschi: 1.1.1
Link to section 'Module' of 'jupyter' Module
You can load the modules by:
module load jupyter
jupyterhub
Link to section 'Description' of 'jupyterhub' Description
Complete Jupyter Hub/Lab/Notebook environment.
Link to section 'Versions' of 'jupyterhub' Versions
- Bell: 2.0.0
- Scholar: 2.0.0
- Gilbreth: 2.0.0
Link to section 'Module' of 'jupyterhub' Module
You can load the modules by:
module load jupyterhub
lammps
Link to section 'Description' of 'lammps' Description
LAMMPS is a classical molecular dynamics code with a focus on materials modelling. It’s an acronym for Large-scale Atomic/Molecular Massively Parallel Simulator.
LAMMPS has potentials for solid-state materials (metals, semiconductors) and soft matter (biomolecules, polymers) and coarse-grained or mesoscopic systems. It can be used to model atoms or, more generically, as a parallel particle simulator at the atomic, meso, or continuum scale.
Link to section 'Versions' of 'lammps' Versions
- Bell: 20200721, 20201029, 2022.5.04
- Scholar: 10Feb2021, 15Jun2020, 24Oct2018, 29Oct2020, 20240829
- Gilbreth: 10Feb2021, 15Jun2020, 24Oct2018, 29Oct2020
- Negishi: 2022.5.04, 20220623
- Anvil: 20210310, 20230802
- Gautschi: 20240829
Link to section 'Module' of 'lammps' Module
You can check available lammps version by:
module spider lammps
You can check how to load the lammps module by the module's full name:
module spider lammps/XXXX
You can load the modules by:
module load lammps # for default version
module load lammps/XXXX # for specific version
Link to section 'Usage' of 'lammps' Usage
LAMMPS reads command lines from an input file like "in.file". The LAMMPS executable is lmp
, to run the lammps input file, use the -in
command:
lmp -in in.file
For more details about how to run LAMMPS, please check LAMMPS.
Link to section 'Example job' of 'lammps' Example job
#!/bin/bash
# FILENAME: myjobsubmissionfile
#SBATCH --nodes=2 # Total # of nodes
#SBATCH --ntasks=256 # Total # of MPI tasks
#SBATCH --time=1:30:00 # Total run time limit (hh:mm:ss)
#SBATCH -J myjobname # Job name
#SBATCH -o myjob.o%j # Name of stdout output file
#SBATCH -e myjob.e%j # Name of stderr error file
# Manage processing environment, load compilers and applications.
module purge
module load gcc/XXXX openmpi/XXXX # or module load intel/XXXX impi/XXXX | depends on the output of "module spider lammps/XXXX"
module load lammps/XXXX
module list
# Launch MPI code
srun -n $SLURM_NTASKS lmp
Link to section 'Note' of 'lammps' Note
Using mpirun -np $SLURM_NTASKS lmp
or mpiexex -np $SLURM_NTASKS lmp
may not work for non-exclusive jobs on some clusters. Use srun -n $SLURM_NTASKS lmp
or mpirun lmp
instead. mpirun lmp
without specifying the number of ranks will automatically pick up the number of SLURM_NTASKS
and works fine.
launcher
Link to section 'Description' of 'launcher' Description
Framework for running large collections of serial or multi-threaded applications
Link to section 'Versions' of 'launcher' Versions
- Anvil: 3.9
- Negishi: 3.9
- Gautschi: 3.9
Link to section 'Module' of 'launcher' Module
You can load the modules by:
module load launcher
learning
Link to section 'Description' of 'learning' Description
The learning module loads the prerequisites (such as anaconda and cudnn ) and makes ML applications visible to the user
Link to section 'Versions' of 'learning' Versions
- Bell: conda-2020.11-py38-cpu
- Gilbreth: conda-5.1.0-py27-cpu, conda-5.1.0-py27-gpu, conda-5.1.0-py36-cpu, conda-5.1.0-py36-gpu, conda-2020.11-py38-cpu, conda-2020.11-py38-gpu
- Anvil: conda-2021.05-py38-gpu
Link to section 'Module' of 'learning' Module
You can load the modules by:
module load learning
Link to section 'Example job' of 'learning' Example job
This is the example jobscript for our cluster `Gilbreth`:
#!/bin/bash
#SBATCH -A myallocation # Allocation name
#SBATCH -t 20:00:00
#SBATCH -N 1
#SBATCH -n 10
#SBATCH --gpus-per-node=1
#SBATCH -p PartitionName
#SBATCH --job-name=learning
#SBATCH --mail-type=FAIL,BEGIN,END
#SBATCH --error=%x-%J-%u.err
#SBATCH --output=%x-%J-%u.out
module --force purge
module load learning/conda-2020.11-py38-gpu
module load ml-toolkit-gpu/pytorch/1.7.1
python torch.py
libfabric
Link to section 'Description' of 'libfabric' Description
The Open Fabrics Interfaces OFI is a framework focused on exporting fabric communication services to applications.
Link to section 'Versions' of 'libfabric' Versions
- Negishi: 1.12.0
- Anvil: 1.12.0
Link to section 'Module' of 'libfabric' Module
You can load the modules by:
module load libfabric
libflame
Link to section 'Description' of 'libflame' Description
libflame is a portable library for dense matrix computations, providing much of the functionality present in LAPACK, developed by current and former members of the Science of High-Performance Computing SHPC group in the Institute for Computational Engineering and Sciences at The University of Texas at Austin. libflame includes a compatibility layer, lapack2flame, which includes a complete LAPACK implementation.
Link to section 'Versions' of 'libflame' Versions
- Negishi: 5.2.0
- Anvil: 5.2.0
- Gautschi: 5.2.0
- Scholar: 5.2.0
Link to section 'Module' of 'libflame' Module
You can load the modules by:
module load libflame
libiconv
Link to section 'Description' of 'libiconv' Description
GNU libiconv provides an implementation of the iconv function and the iconv program for character set conversion.
Link to section 'Versions' of 'libiconv' Versions
- Bell: 1.16, 1.17
- Gilbreth: 1.16
- Negishi: 1.16
- Anvil: 1.16
Link to section 'Module' of 'libiconv' Module
You can load the modules by:
module load libiconv
libmesh
Link to section 'Description' of 'libmesh' Description
The libMesh library provides a framework for the numerical simulation of partial differential equations using arbitrary unstructured discretizations on serial and parallel platforms.
Link to section 'Versions' of 'libmesh' Versions
- Negishi: 1.7.1
- Anvil: 1.6.2
Link to section 'Module' of 'libmesh' Module
You can load the modules by:
module load libmesh
libszip
Link to section 'Description' of 'libszip' Description
Szip is an implementation of the extended-Rice lossless compression algorithm.
Link to section 'Versions' of 'libszip' Versions
- Bell: 2.1.1
- Negishi: 2.1.1
- Anvil: 2.1.1
Link to section 'Module' of 'libszip' Module
You can load the modules by:
module load libszip
libtiff
Link to section 'Description' of 'libtiff' Description
LibTIFF - Tag Image File Format TIFF Library and Utilities.
Link to section 'Versions' of 'libtiff' Versions
- Bell: 4.0.10, 4.4.0, 4.6.0
- Gilbreth: 4.0.10
- Negishi: 4.4.0
- Anvil: 4.1.0
Link to section 'Module' of 'libtiff' Module
You can load the modules by:
module load libtiff
libx11
Link to section 'Description' of 'libx11' Description
Xlib − C Language X Interface is a reference guide to the low-level C language interface to the X Window System protocol. It is neither a tutorial nor a user’s guide to programming the X Window System. Rather, it provides a detailed description of each function in the library as well as a discussion of the related background information.
Link to section 'Versions' of 'libx11' Versions
- Anvil: 1.7.0
- Negishi: 1.7.0
Link to section 'Module' of 'libx11' Module
You can load the modules by:
module load libx11
libxml2
Link to section 'Description' of 'libxml2' Description
Libxml2 is the XML C parser and toolkit developed for the Gnome project but usable outside of the Gnome platform, it is free software available under the MIT License.
Link to section 'Versions' of 'libxml2' Versions
- Bell: 2.9.9, 2.10.3
- Gilbreth: 2.9.9
- Negishi: 2.10.1
- Anvil: 2.9.10
Link to section 'Module' of 'libxml2' Module
You can load the modules by:
module load libxml2
mathematica
Link to section 'Description' of 'mathematica' Description
Mathematica is a technical computing environment and programming language with strong symbolic and numerical abilities.
Link to section 'Versions' of 'mathematica' Versions
- Bell: 11.3, 12.1, 12.3, 13.1, 14.1
- Scholar: 14.1
- Gilbreth: 11.3, 12.1, 12.3, 13.1, 14.1
- Negishi: 13.1, 14.1
- Gautschi: 14.1
Link to section 'Module' of 'mathematica' Module
You can load the modules by:
module load mathematica
Link to section 'Running Mathematica' of 'mathematica' Running Mathematica
Users can run Mathematica GUI in interactive jobs or run it as batch jobs.Link to section 'Interactive jobs' of 'mathematica' Interactive jobs
sinteractive -N1 -n24 -t4:00:00 -A standby
module load mathematica
Mathematica
Notice: The executable name has changed from Mathematica
to WolframNB
in version 14.1
Link to section 'Batch job' of 'mathematica' Batch job
To submit a sbatch job on our clusters:
#!/bin/bash
#SBATCH -A XXX
#SBATCH -t 10:00:00
#SBATCH -N 1
#SBATCH -n 24
#SBATCH --job-name=mathematica
#SBATCH --mail-type=FAIL,BEGIN,END
#SBATCH --error=%x-%J-%u.err
#SBATCH --output=%x-%J-%u.out
module load mathematica
math -noprompt < input.m
matlab
Link to section 'Description' of 'matlab' Description
MATLAB MATrix LABoratory is a multi-paradigm numerical computing environment and fourth-generation programming language. A proprietary programming language developed by MathWorks, MATLAB allows matrix manipulations, plotting of functions and data, implementation of algorithms, creation of user interfaces, and interfacing with programs written in other languages, including C, C++, C#, Java, Fortran and Python.
Link to section 'Versions' of 'matlab' Versions
- Bell: R2019a, R2020a, R2020b, R2021b, R2022a, R2023a
- Scholar: R2024a
- Gilbreth: R2017a, R2018a, R2019a, R2020a, R2022a, R2023a
- Negishi: R2021b, R2022a, R2023a
- Anvil: R2020b, R2021b, R2022a, R2023a
- Gautschi: R2024a
Link to section 'Module' of 'matlab' Module
You can load the modules by:
module load matlab
MATLAB, Simulink, Compiler, and several of the optional toolboxes are available to faculty, staff, and students. To see the kind and quantity of all MATLAB licenses plus the number that you are currently using you can use the matlab_licenses command:
$ module load matlab
$ matlab_licenses
The MATLAB client can be run in the front-end for application development, however, computationally intensive jobs must be run on compute nodes.
The following sections provide several examples illustrating how to submit MATLAB jobs to a Linux compute cluster.
Matlab Script (.m File)
This section illustrates how to submit a small, serial, MATLAB program as a job to a batch queue. This MATLAB program prints the name of the run host and gets three random numbers.
Prepare a MATLAB script myscript.m, and a MATLAB function file myfunction.m:
% FILENAME: myscript.m
% Display name of compute node which ran this job.
[c name] = system('hostname');
fprintf('\n\nhostname:%s\n', name);
% Display three random numbers.
A = rand(1,3);
fprintf('%f %f %f\n', A);
quit;
% FILENAME: myfunction.m
function result = myfunction ()
% Return name of compute node which ran this job.
[c name] = system('hostname');
result = sprintf('hostname:%s', name);
% Return three random numbers.
A = rand(1,3);
r = sprintf('%f %f %f', A);
result=strvcat(result,r);
end
Also, prepare a job submission file, here named myjob.sub. Run with the name of the script:
#!/bin/bash
# FILENAME: myjob.sub
echo "myjob.sub"
# Load module, and set up environment for Matlab to run
module load matlab
unset DISPLAY
# -nodisplay: run MATLAB in text mode; X11 server not needed
# -singleCompThread: turn off implicit parallelism
# -r: read MATLAB program; use MATLAB JIT Accelerator
# Run Matlab, with the above options and specifying our .m file
matlab -nodisplay -singleCompThread -r myscript
myjob.sub
< M A T L A B (R) >
Copyright 1984-2011 The MathWorks, Inc.
R2011b (7.13.0.564) 64-bit (glnxa64)
August 13, 2011
To get started, type one of these: helpwin, helpdesk, or demo.
For product information, visit www.mathworks.com.
hostname:resource-a001.rcac.purdue.edu
0.814724 0.905792 0.126987
Output shows that a processor core on one compute node (resource-a001) processed the job. Output also displays the three random numbers.
For more information about MATLAB:
Implicit Parallelism
MATLAB implements implicit parallelism which is automatic multithreading of many computations, such as matrix multiplication, linear algebra, and performing the same operation on a set of numbers. This is different from the explicit parallelism of the Parallel Computing Toolbox.
MATLAB offers implicit parallelism in the form of thread-parallel enabled functions. Since these processor cores, or threads, share a common memory, many MATLAB functions contain multithreading potential. Vector operations, the particular application or algorithm, and the amount of computation (array size) contribute to the determination of whether a function runs serially or with multithreading.
When your job triggers implicit parallelism, it attempts to allocate its threads on all processor cores of the compute node on which the MATLAB client is running, including processor cores running other jobs. This competition can degrade the performance of all jobs running on the node.
When you know that you are coding a serial job but are unsure whether you are using thread-parallel enabled operations, run MATLAB with implicit parallelism turned off. Beginning with the R2009b, you can turn multithreading off by starting MATLAB with -singleCompThread:
$ matlab -nodisplay -singleCompThread -r mymatlabprogram
When you are using implicit parallelism, make sure you request exclusive access to a compute node, as MATLAB has no facility for sharing nodes.
For more information about MATLAB's implicit parallelism:
Profile Manager
MATLAB offers two kinds of profiles for parallel execution: the 'local' profile and user-defined cluster profiles. The 'local' profile runs a MATLAB job on the processor core(s) of the same compute node, or front-end, that is running the client. To run a MATLAB job on compute node(s) different from the node running the client, you must define a Cluster Profile using the Cluster Profile Manager.
To prepare a user-defined cluster profile, use the Cluster Profile Manager in the Parallel menu. This profile contains the scheduler details (queue, nodes, processors, walltime, etc.) of your job submission. Ultimately, your cluster profile will be an argument to MATLAB functions like batch().
For your convenience, a generic cluster profile is provided that can be downloaded: myslurmprofile.settings
Please note that modifications are very likely to be required to make myslurmprofile.settings work. You may need to change values for number of nodes, number of workers, walltime, and submission queue specified in the file. As well, the generic profile itself depends on the particular job scheduler on the cluster, so you may need to download or create two or more generic profiles under different names. Each time you run a job using a Cluster Profile, make sure the specific profile you are using is appropriate for the job and the cluster.
To import the profile, start a MATLAB session and select Manage Cluster Profiles... from the Parallel menu. In the Cluster Profile Manager, select Import, navigate to the folder containing the profile, select myslurmprofile.settings and click OK. Remember that the profile will need to be customized for your specific needs. If you have any questions, please contact us.
For detailed information about MATLAB's Parallel Computing Toolbox, examples, demos, and tutorials:
Parallel Computing Toolbox (parfor)
The MATLAB Parallel Computing Toolbox (PCT) extends the MATLAB language with high-level, parallel-processing features such as parallel for loops, parallel regions, message passing, distributed arrays, and parallel numerical methods. It offers a shared-memory computing environment running on the local cluster profile in addition to your MATLAB client. Moreover, the MATLAB Distributed Computing Server (DCS) scales PCT applications up to the limit of your DCS licenses.
This section illustrates the fine-grained parallelism of a parallel for loop (parfor) in a pool job.
The following examples illustrate a method for submitting a small, parallel, MATLAB program with a parallel loop (parfor statement) as a job to a queue. This MATLAB program prints the name of the run host and shows the values of variables numlabs and labindex for each iteration of the parfor loop.
This method uses the job submission command to submit a MATLAB client which calls the MATLAB batch() function with a user-defined cluster profile.
Prepare a MATLAB pool program in a MATLAB script with an appropriate filename, here named myscript.m:
% FILENAME: myscript.m
% SERIAL REGION
[c name] = system('hostname');
fprintf('SERIAL REGION: hostname:%s\n', name)
numlabs = parpool('poolsize');
fprintf(' hostname numlabs labindex iteration\n')
fprintf(' ------------------------------- ------- -------- ---------\n')
tic;
% PARALLEL LOOP
parfor i = 1:8
[c name] = system('hostname');
name = name(1:length(name)-1);
fprintf('PARALLEL LOOP: %-31s %7d %8d %9d\n', name,numlabs,labindex,i)
pause(2);
end
% SERIAL REGION
elapsed_time = toc; % get elapsed time in parallel loop
fprintf('\n')
[c name] = system('hostname');
name = name(1:length(name)-1);
fprintf('SERIAL REGION: hostname:%s\n', name)
fprintf('Elapsed time in parallel loop: %f\n', elapsed_time)
The execution of a pool job starts with a worker executing the statements of the first serial region up to the parfor block, when it pauses. A set of workers (the pool) executes the parfor block. When they finish, the first worker resumes by executing the second serial region. The code displays the names of the compute nodes running the batch session and the worker pool.
Prepare a MATLAB script that calls MATLAB function batch() which makes a four-lab pool on which to run the MATLAB code in the file myscript.m. Use an appropriate filename, here named mylclbatch.m:
% FILENAME: mylclbatch.m
!echo "mylclbatch.m"
!hostname
pjob=batch('myscript','Profile','myslurmprofile','Pool',4,'CaptureDiary',true);
wait(pjob);
diary(pjob);
quit;
Prepare a job submission file with an appropriate filename, here named myjob.sub:
#!/bin/bash
# FILENAME: myjob.sub
echo "myjob.sub"
hostname
module load matlab
unset DISPLAY
matlab -nodisplay -r mylclbatch
One processor core runs myjob.sub and mylclbatch.m.
Once this job starts, a second job submission is made.
myjob.sub
< M A T L A B (R) >
Copyright 1984-2013 The MathWorks, Inc.
R2013a (8.1.0.604) 64-bit (glnxa64)
February 15, 2013
To get started, type one of these: helpwin, helpdesk, or demo.
For product information, visit www.mathworks.com.
mylclbatch.mresource-a000.rcac.purdue.edu
SERIAL REGION: hostname:resource-a000.rcac.purdue.edu
hostname numlabs labindex iteration
------------------------------- ------- -------- ---------
PARALLEL LOOP: resource-a001.rcac.purdue.edu 4 1 2
PARALLEL LOOP: resource-a002.rcac.purdue.edu 4 1 4
PARALLEL LOOP: resource-a001.rcac.purdue.edu 4 1 5
PARALLEL LOOP: resource-a002.rcac.purdue.edu 4 1 6
PARALLEL LOOP: resource-a003.rcac.purdue.edu 4 1 1
PARALLEL LOOP: resource-a003.rcac.purdue.edu 4 1 3
PARALLEL LOOP: resource-a004.rcac.purdue.edu 4 1 7
PARALLEL LOOP: resource-a004.rcac.purdue.edu 4 1 8
SERIAL REGION: hostname:resource-a001.rcac.purdue.edu
Elapsed time in parallel loop: 5.411486
To scale up this method to handle a real application, increase the wall time in the submission command to accommodate a longer running job. Secondly, increase the wall time of myslurmprofile by using the Cluster Profile Manager in the Parallel menu to enter a new wall time in the property SubmitArguments.
For more information about MATLAB Parallel Computing Toolbox:
Parallel Toolbox (spmd)
The MATLAB Parallel Computing Toolbox (PCT) extends the MATLAB language with high-level, parallel-processing features such as parallel for loops, parallel regions, message passing, distributed arrays, and parallel numerical methods. It offers a shared-memory computing environment with a maximum of eight MATLAB workers (labs, threads; versions R2009a) and 12 workers (labs, threads; version R2011a) running on the local configuration in addition to your MATLAB client. Moreover, the MATLAB Distributed Computing Server (DCS) scales PCT applications up to the limit of your DCS licenses.
This section illustrates how to submit a small, parallel, MATLAB program with a parallel region (spmd statement) as a MATLAB pool job to a batch queue.
This example uses the submission command to submit to compute nodes a MATLAB client which interprets a Matlab .m with a user-defined cluster profile which scatters the MATLAB workers onto different compute nodes. This method uses the MATLAB interpreter, the Parallel Computing Toolbox, and the Distributed Computing Server; so, it requires and checks out six licenses: one MATLAB license for the client running on the compute node, one PCT license, and four DCS licenses. Four DCS licenses run the four copies of the spmd statement. This job is completely off the front end.
Prepare a MATLAB script called myscript.m:
% FILENAME: myscript.m
% SERIAL REGION
[c name] = system('hostname');
fprintf('SERIAL REGION: hostname:%s\n', name)
p = parpool('4');
fprintf(' hostname numlabs labindex\n')
fprintf(' ------------------------------- ------- --------\n')
tic;
% PARALLEL REGION
spmd
[c name] = system('hostname');
name = name(1:length(name)-1);
fprintf('PARALLEL REGION: %-31s %7d %8d\n', name,numlabs,labindex)
pause(2);
end
% SERIAL REGION
elapsed_time = toc; % get elapsed time in parallel region
delete(p);
fprintf('\n')
[c name] = system('hostname');
name = name(1:length(name)-1);
fprintf('SERIAL REGION: hostname:%s\n', name)
fprintf('Elapsed time in parallel region: %f\n', elapsed_time)
quit;
Prepare a job submission file with an appropriate filename, here named myjob.sub. Run with the name of the script:
#!/bin/bash
# FILENAME: myjob.sub
echo "myjob.sub"
module load matlab
unset DISPLAY
matlab -nodisplay -r myscript
Run MATLAB to set the default parallel configuration to your job configuration:
$ matlab -nodisplay
>> parallel.defaultClusterProfile('myslurmprofile');
>> quit;
$
Once this job starts, a second job submission is made.
myjob.sub
< M A T L A B (R) >
Copyright 1984-2011 The MathWorks, Inc.
R2011b (7.13.0.564) 64-bit (glnxa64)
August 13, 2011
To get started, type one of these: helpwin, helpdesk, or demo.
For product information, visit www.mathworks.com.
SERIAL REGION: hostname:resource-a001.rcac.purdue.edu
Starting matlabpool using the 'myslurmprofile' profile ... connected to 4 labs.
hostname numlabs labindex
------------------------------- ------- --------
Lab 2:
PARALLEL REGION: resource-a002.rcac.purdue.edu 4 2
Lab 1:
PARALLEL REGION: resource-a001.rcac.purdue.edu 4 1
Lab 3:
PARALLEL REGION: resource-a003.rcac.purdue.edu 4 3
Lab 4:
PARALLEL REGION: resource-a004.rcac.purdue.edu 4 4
Sending a stop signal to all the labs ... stopped.
SERIAL REGION: hostname:resource-a001.rcac.purdue.edu
Elapsed time in parallel region: 3.382151
Output shows the name of one compute node (a001) that processed the job submission file myjob.sub and the two serial regions. The job submission scattered four processor cores (four MATLAB labs) among four different compute nodes (a001,a002,a003,a004) that processed the four parallel regions. The total elapsed time demonstrates that the jobs ran in parallel.
For more information about MATLAB Parallel Computing Toolbox:
Distributed Computing Server (parallel job)
The MATLAB Parallel Computing Toolbox (PCT) enables a parallel job via the MATLAB Distributed Computing Server (DCS). The tasks of a parallel job are identical, run simultaneously on several MATLAB workers (labs), and communicate with each other. This section illustrates an MPI-like program.
This section illustrates how to submit a small, MATLAB parallel job with four workers running one MPI-like task to a batch queue. The MATLAB program broadcasts an integer to four workers and gathers the names of the compute nodes running the workers and the lab IDs of the workers.
This example uses the job submission command to submit a Matlab script with a user-defined cluster profile which scatters the MATLAB workers onto different compute nodes. This method uses the MATLAB interpreter, the Parallel Computing Toolbox, and the Distributed Computing Server; so, it requires and checks out six licenses: one MATLAB license for the client running on the compute node, one PCT license, and four DCS licenses. Four DCS licenses run the four copies of the parallel job. This job is completely off the front end.
Prepare a MATLAB script named myscript.m :
% FILENAME: myscript.m
% Specify pool size.
% Convert the parallel job to a pool job.
parpool('4');
spmd
if labindex == 1
% Lab (rank) #1 broadcasts an integer value to other labs (ranks).
N = labBroadcast(1,int64(1000));
else
% Each lab (rank) receives the broadcast value from lab (rank) #1.
N = labBroadcast(1);
end
% Form a string with host name, total number of labs, lab ID, and broadcast value.
[c name] =system('hostname');
name = name(1:length(name)-1);
fmt = num2str(floor(log10(numlabs))+1);
str = sprintf(['%s:%d:%' fmt 'd:%d '], name,numlabs,labindex,N);
% Apply global concatenate to all str's.
% Store the concatenation of str's in the first dimension (row) and on lab #1.
result = gcat(str,1,1);
if labindex == 1
disp(result)
end
end % spmd
matlabpool close force;
quit;
Also, prepare a job submission, here named myjob.sub. Run with the name of the script:
# FILENAME: myjob.sub
echo "myjob.sub"
module load matlab
unset DISPLAY
# -nodisplay: run MATLAB in text mode; X11 server not needed
# -r: read MATLAB program; use MATLAB JIT Accelerator
matlab -nodisplay -r myscript
Run MATLAB to set the default parallel configuration to your appropriate Profile:
$ matlab -nodisplay
>> defaultParallelConfig('myslurmprofile');
>> quit;
$
Once this job starts, a second job submission is made.
myjob.sub
< M A T L A B (R) >
Copyright 1984-2011 The MathWorks, Inc.
R2011b (7.13.0.564) 64-bit (glnxa64)
August 13, 2011
To get started, type one of these: helpwin, helpdesk, or demo.
For product information, visit www.mathworks.com.
>Starting matlabpool using the 'myslurmprofile' configuration ... connected to 4 labs.
Lab 1:
resource-a006.rcac.purdue.edu:4:1:1000
resource-a007.rcac.purdue.edu:4:2:1000
resource-a008.rcac.purdue.edu:4:3:1000
resource-a009.rcac.purdue.edu:4:4:1000
Sending a stop signal to all the labs ... stopped.
Did not find any pre-existing parallel jobs created by matlabpool.
Output shows the name of one compute node (a006) that processed the job submission file myjob.sub. The job submission scattered four processor cores (four MATLAB labs) among four different compute nodes (a006,a007,a008,a009) that processed the four parallel regions.
To scale up this method to handle a real application, increase the wall time in the submission command to accommodate a longer running job. Secondly, increase the wall time of myslurmprofile by using the Cluster Profile Manager in the Parallel menu to enter a new wall time in the property SubmitArguments.
For more information about parallel jobs:
meep
Link to section 'Description' of 'meep' Description
Meep or MEEP is a free finite-difference time-domain FDTD simulation software package developed at MIT to model electromagnetic systems.
Link to section 'Versions' of 'meep' Versions
- Negishi: 1.20.0
- Anvil: 1.20.0
Link to section 'Module' of 'meep' Module
You can load the modules by:
module load meep
modtree
Link to section 'Description' of 'modtree' Description
ModuleTree or modtree helps users naviagate between different application stacks and sets up a default compiler and mpi environment.
Link to section 'Versions' of 'modtree' Versions
- Bell: deprecated, new
- Scholar: deprecated, recent
- Gilbreth: deprecated, new
- Negishi: cpu
- Anvil: cpu, gpu
- Gautschi: cpu
Link to section 'Module' of 'modtree' Module
You can load the modules by:
module load modtree
monitor
Link to section 'Description' of 'monitor' Description
System resource monitoring tool.
Link to section 'Versions' of 'monitor' Versions
- Anvil: 2.3.1
- Negishi: 2.3.1
- Gautschi: 2.3.1
Link to section 'Module' of 'monitor' Module
You can load the modules by:
module load monitor
mpc
Link to section 'Description' of 'mpc' Description
Gnu Mpc is a C library for the arithmetic of complex numbers with arbitrarily high precision and correct rounding of the result.
Link to section 'Versions' of 'mpc' Versions
- Bell: 1.1.0
- Gilbreth: 1.1.0
- Negishi: 1.1.0
- Anvil: 1.1.0
- Gautschi: 1.3.1
Link to section 'Module' of 'mpc' Module
You can load the modules by:
module load mpc
mpfr
Link to section 'Description' of 'mpfr' Description
The MPFR library is a C library for multiple-precision floating-point computations with correct rounding.
Link to section 'Versions' of 'mpfr' Versions
- Bell: 3.1.6, 4.2.1
- Gilbreth: 3.1.6
- Negishi: 4.0.2
- Anvil: 3.1.6, 4.0.2
Link to section 'Module' of 'mpfr' Module
You can load the modules by:
module load mpfr
mrbayes
Link to section 'Description' of 'mrbayes' Description
MrBayes is a program for Bayesian inference and model choice across a wide range of phylogenetic and evolutionary models. MrBayes uses Markov chain Monte Carlo MCMC methods to estimate the posterior distribution of model parameters.
Link to section 'Versions' of 'mrbayes' Versions
- Anvil: 3.2.7a
- Bell: 3.2.7
- Negishi: 3.2.7
- Scholar: 3.2.7
- Gautschi: 3.2.7
Link to section 'Module' of 'mrbayes' Module
You can load the modules by:
module load mrbayes
mxnet
Link to section 'Description' of 'mxnet' Description
NVIDIA Optimized Deep Learning Framework, powered by Apache MXNet is a deep learning framework designed for both efficiency and flexibility. It allows you to mix the flavors of symbolic programming and imperative programming to maximize efficiency and productivity.
Link to section 'Versions' of 'mxnet' Versions
- Gilbreth: 1.7.0
Link to section 'Module' of 'mxnet' Module
You can load the modules by:
module load mxnet
namd
Link to section 'Description' of 'namd' Description
NAMDis a parallel molecular dynamics code designed for high-performance simulation of large biomolecular systems.
Link to section 'Versions' of 'namd' Versions
- Anvil: 2.14, 3.0
- Bell: 2.15, 3.0
- Gilbreth: 2.13, 3.0
- Negishi: 2.14, 2.15, 3.0
- Scholar: 2.13-multinode, 2.13-singlenode, 3.0-alpha3-singlenode
- Gautschi: 3.0
Link to section 'Module' of 'namd' Module
You can load the modules by:
module load namd
nccl
Link to section 'Description' of 'nccl' Description
Optimized primitives for collective multi-GPU communication.
Link to section 'Versions' of 'nccl' Versions
- Anvil: cuda-11.0_2.11.4, cuda-11.2_2.8.4, cuda-11.4_2.11.4
Link to section 'Module' of 'nccl' Module
You can load the modules by:
module load modtree/gpu
module load nccl
nco
Link to section 'Description' of 'nco' Description
The NCO toolkit manipulates and analyzes data stored in netCDF-accessible formats
Link to section 'Versions' of 'nco' Versions
- Bell: 4.6.7
- Scholar: 5.2.4
- Gilbreth: 4.6.7
- Negishi: 4.9.3, 5.0.1
- Anvil: 4.9.3
- Gautschi: 5.2.4
Link to section 'Module' of 'nco' Module
You can load the modules by:
module load nco
ncview
Link to section 'Description' of 'ncview' Description
Simple viewer for NetCDF files.
Link to section 'Versions' of 'ncview' Versions
- Bell: 2.1.7
- Scholar: 2.1.9
- Gilbreth: 2.1.7
- Negishi: 2.1.9
- Anvil: 2.1.8
- Gautschi: 2.1.9
Link to section 'Module' of 'ncview' Module
You can load the modules by:
module load ncview
netcdf-c
Link to section 'Description' of 'netcdf-c' Description
NetCDF network Common Data Form is a set of software libraries and machine-independent data formats that support the creation, access, and sharing of array-oriented scientific data. This is the C distribution.
Link to section 'Versions' of 'netcdf-c' Versions
- Bell: 4.9.0
- Negishi: 4.9.0
- Anvil: 4.7.4
- Gautschi: 4.9.2
- Scholar: 4.9.2
Link to section 'Module' of 'netcdf-c' Module
You can load the modules by:
module load netcdf-c
netcdf-cxx4
Link to section 'Description' of 'netcdf-cxx4' Description
NetCDF network Common Data Form is a set of software libraries and machine-independent data formats that support the creation, access, and sharing of array-oriented scientific data. This is the C++ distribution.
Link to section 'Versions' of 'netcdf-cxx4' Versions
- Bell: 4.3.0, 4.3.1
- Scholar: 4.3.1
- Gilbreth: 4.3.0, 4.3.1
- Negishi: 4.3.1
- Anvil: 4.3.1
- Gautschi: 4.3.1
Link to section 'Module' of 'netcdf-cxx4' Module
You can load the modules by:
module load netcdf-cxx4
netcdf-fortran
Link to section 'Description' of 'netcdf-fortran' Description
NetCDF network Common Data Form is a set of software libraries and machine-independent data formats that support the creation, access, and sharing of array-oriented scientific data. This is the Fortran distribution.
Link to section 'Versions' of 'netcdf-fortran' Versions
- Bell: 4.4.4, 4.5.3, 4.6.0
- Scholar: 4.6.1
- Gilbreth: 4.4.4, 4.5.2, 4.6.1
- Negishi: 4.6.0
- Anvil: 4.5.3
- Gautschi: 4.6.1
Link to section 'Module' of 'netcdf-fortran' Module
You can load the modules by:
module load netcdf-fortran
netlib-lapack
Link to section 'Description' of 'netlib-lapack' Description
LAPACK version 3.X is a comprehensive FORTRAN library that does linear algebra operations including matrix inversions, least squared solutions to linear sets of equations, eigenvector analysis, singular value decomposition, etc. It is a very comprehensive and reputable package that has found extensive use in the scientific community.
Link to section 'Versions' of 'netlib-lapack' Versions
- Bell: 3.8.0
- Scholar: 3.11.0
- Gilbreth: 3.6.0, 3.8.0
- Negishi: 3.8.0
- Anvil: 3.8.0
- Gautschi: 3.11.0
Link to section 'Module' of 'netlib-lapack' Module
You can load the modules by:
module load netlib-lapack
numactl
Link to section 'Description' of 'numactl' Description
Simple NUMA policy support. It consists of a numactl program to run other programs with a specific NUMA policy and a libnuma shared library ("NUMA API") to set NUMA policy in applications.
Link to section 'Versions' of 'numactl' Versions
- Bell: 2.0.14
- Negishi: 2.0.14
- Anvil: 2.0.14
Link to section 'Module' of 'numactl' Module
You can load the modules by:
module load numactl
Link to section 'Usage' of 'numactl' Usage
numactl [ options ] command {arguments ...} command to run the program
Options:
-H, --hardware,
-m nodes, --membind=nodes, Use the memory on these NUMA nodes
-N nodes, --cpunodebind=nodes, Use the CPUs on these nodes
-C cpus, --physcpubind=cpus, Use these CPUs
nwchem
Link to section 'Description' of 'nwchem' Description
High-performance computational chemistry software
Link to section 'Versions' of 'nwchem' Versions
- Negishi: 7.0.2
- Anvil: 7.0.2
Link to section 'Module' of 'nwchem' Module
You can load the modules by:
module load nwchem
octave
Link to section 'Description' of 'octave' Description
GNU Octave is a high-level language, primarily intended for numerical computations. It provides a convenient command line interface for solving linear and nonlinear problems numerically, and for performing other numerical experiments using a language that is mostly compatible with Matlab. It may also be used as a batch-oriented language.
Link to section 'Versions' of 'octave' Versions
- Bell: 4.4.1
- Negishi: 7.3.0
- Anvil: 6.3.0
Link to section 'Module' of 'octave' Module
You can load the modules by:
module load octave
openblas
Link to section 'Description' of 'openblas' Description
OpenBLAS is an open source implementation of the BLAS API with many hand-crafted optimizations for specific processor types
Link to section 'Versions' of 'openblas' Versions
- Bell: 0.3.8, 0.3.21
- Scholar: 0.3.27
- Gilbreth: 0.2.20, 0.3.7, 0.3.21
- Negishi: 0.3.21
- Anvil: 0.3.17
- Gautschi: 0.3.27
Link to section 'Module' of 'openblas' Module
You can load the modules by:
module load openblas
openfoam
Link to section 'Description' of 'openfoam' Description
OpenFOAM is leading software for computational fluid dynamics (CFD).
Link to section 'Versions' of 'openfoam' Versions
- Bell: 5.x, 9-20211122
- Anvil: 8-20210316
- Negishi: 2312, 9-20211122
- Gautschi:2312
- Scholar:2312
Link to section 'Module' of 'openfoam' Module
You can load the modules by:
module load openmpi/5.0.5
module load openfoam
openjdk
Link to section 'Description' of 'openjdk' Description
The free and open-source java implementation
Link to section 'Versions' of 'openjdk' Versions
- Negishi: 1.8.0_265-b01, 11.0.17_8
- Anvil: 1.8.0_265-b01, 11.0.8_10
Link to section 'Module' of 'openjdk' Module
You can load the modules by:
module load openjdk
panoply
Link to section 'Description' of 'panoply' Description
Panoply is a Java-based cross-platform NetCDF, HDF and GRIB Data Viewer.
Link to section 'Versions' of 'panoply' Versions
- Bell: 4.11.6
- Scholar: 4.11.0
- Gilbreth: 4.11.0
Link to section 'Module' of 'panoply' Module
You can load the modules by:
module load panoply
papi
Link to section 'Description' of 'papi' Description
PAPI provides the tool designer and application engineer with a consistent interface and methodology for use of the performance counter hardware found in most major microprocessors. PAPI enables software engineers to see, in near real time, the relation between software performance and processor events. In addition Component PAPI provides access to a collection of components that expose performance measurement opportunities across the hardware and software stack.
Link to section 'Versions' of 'papi' Versions
- Negishi: 6.0.0.1
- Anvil: 6.0.0.1
Link to section 'Module' of 'papi' Module
You can load the modules by:
module load papi
parafly
Link to section 'Description' of 'parafly' Description
Run UNIX commands in parallel
Link to section 'Versions' of 'parafly' Versions
- Negishi: r2013
- Anvil: r2013
- Gautschi: r2013
Link to section 'Module' of 'parafly' Module
You can load the modules by:
module load parafly
parallel-netcdf
Link to section 'Description' of 'parallel-netcdf' Description
PnetCDF Parallel netCDF is a high-performance parallel I/O library for accessing files in format compatibility with Unidatas NetCDF, specifically the formats of CDF-1, 2, and 5.
Link to section 'Versions' of 'parallel-netcdf' Versions
- Bell: 1.11.2
- Negishi: 1.11.2
- Scholar: 1.12.3
- Anvil: 1.11.2
- Gautschi: 1.12.3
Link to section 'Module' of 'parallel-netcdf' Module
You can load the modules by:
module load parallel-netcdf
paraview
Link to section 'Description' of 'paraview' Description
ParaView is an open-source, multi-platform data analysis and visualization application.
Link to section 'Versions' of 'paraview' Versions
- Bell: 5.6.2
- Anvil: 5.9.1, 5.10.1
- Gilbreth: 5.9.0
- Negishi: 5.12.1, 5.9.1
- Scholar: 5.9.0, 5.13.0
Link to section 'Module' of 'paraview' Module
You can load the modules by:
module load paraview
perl-bioperl
Link to section 'Description' of 'perl-bioperl' Description
BioPerl is the product of a community effort to produce Perl code which is useful in biology. Examples include Sequence objects, Alignment objects and database searching objects. These objects not only do what they are advertised to do in the documentation, but they also interact - Alignment objects are made from the Sequence objects, Sequence objects have access to Annotation and SeqFeature objects and databases, Blast objects can be converted to Alignment objects, and so on. This means that the objects provide a coordinated and extensible framework to do computational biology.
Link to section 'Versions' of 'perl-bioperl' Versions
- Anvil: 1.7.2, 1.7.6
- Bell: 1.7.2
- Negishi: 1.7.2
- Scholar: 1.7.2-pl526
- Gautschi: 1.7.2-pl526
Link to section 'Commands' of 'perl-bioperl' Commands
- bp_aacomp
- bp_bioflat_index
- bp_biogetseq
- bp_chaos_plot
- bp_dbsplit
- bp_extract_feature_seq
- bp_fastam9_to_table
- bp_fetch
- bp_filter_search
- bp_find-blast-matches
- bp_gccalc
- bp_genbank2gff3
- bp_index
- bp_local_taxonomydb_query
- bp_make_mrna_protein
- bp_mask_by_search
- bp_mrtrans
- bp_mutate
- bp_nexus2nh
- bp_nrdb
- bp_oligo_count
- bp_process_gadfly
- bp_process_sgd
- bp_revtrans-motif
- bp_search2alnblocks
- bp_search2gff
- bp_search2table
- bp_search2tribe
- bp_seqconvert
- bp_seqcut
- bp_seq_length
- bp_seqpart
- bp_seqret
- bp_seqretsplit
- bp_split_seq
- bp_sreformat
- bp_taxid4species
- bp_taxonomy2tree
- bp_translate_seq
- bp_tree2pag
- bp_unflatten_seq
Link to section 'Module' of 'perl-bioperl' Module
You can load the modules by:
module load perl-bioperl
petsc
Link to section 'Description' of 'petsc' Description
PETSc is a suite of data structures and routines for the scalable parallel solution of scientific applications modeled by partial differential equations.
Link to section 'Versions' of 'petsc' Versions
- Negishi: 3.17.5, 3.18.3
- Anvil: 3.15.3
Link to section 'Module' of 'petsc' Module
You can load the modules by:
module load petsc
proj
Link to section 'Description' of 'proj' Description
PROJ is a generic coordinate transformation software, that transforms geospatial coordinates from one coordinate reference system CRS to another. This includes cartographic projections as well as geodetic transformations.
Link to section 'Versions' of 'proj' Versions
- Bell: 5.2.0, 8.1.0, 8.2.1
- Gilbreth: 5.2.0, 8.2.1
- Negishi: 5.2.0, 6.2.0, 8.1.0, 8.2.1
- Anvil: 5.2.0, 6.2.0
Link to section 'Module' of 'proj' Module
You can load the modules by:
module load proj
protobuf
Link to section 'Description' of 'protobuf' Description
Protocol Buffers (a.k.a., protobuf) are Google's language-neutral, platform-neutral, extensible mechanism for serializing structured data.
Link to section 'Versions' of 'protobuf' Versions
- Bell: 3.11.4
- Gilbreth: 3.0.2
- Negishi: 3.11.4, 3.18.0
- Anvil: 3.11.4
Link to section 'Module' of 'protobuf' Module
You can load the modules by:
module load protobuf
py-mpi4py
Link to section 'Description' of 'py-mpi4py' Description
mpi4py provides a Python interface to MPI or the Message-Passing Interface. It is useful for parallelizing Python scripts
Link to section 'Versions' of 'py-mpi4py' Versions
- Anvil: 3.0.3
Link to section 'Module' of 'py-mpi4py' Module
You can load the modules by:
module load py-mpi4py
python
Link to section 'Description' of 'python' Description
Native Python 3.9.5 including optimized libraries.
Link to section 'Versions' of 'python' Versions
- Anvil: 3.9.5
- Gautschi: 3.11.9
Link to section 'Module' of 'python' Module
You can load the modules by:
module load python
pytorch
Link to section 'Description' of 'pytorch' Description
PyTorch is a machine learning library with strong support for neural networks and deep learning. PyTorch also has a large user base and software ecosystem.
Link to section 'Versions' of 'pytorch' Versions
- Bell: 1.6.0
- Gilbreth: 1.0.0, 1.4.0, 1.7.0
- Anvil: 1.10.0
- Scholar: 1.5.0, 1.6.0, 1.8.0, 1.9.0, 1.10.0
Link to section 'Module' of 'pytorch' Module
You can load the modules by:
module load learning
module load pytorch
To check the loaded, version, run:
python -c "import torch; print(torch.__version__)"
qt
Link to section 'Description' of 'qt' Description
Qt is a comprehensive cross-platform C++ application framework.
Link to section 'Versions' of 'qt' Versions
- Bell: 5.12.5
- Gilbreth: 5.12.5
- Negishi: 5.15.2
- Anvil: 5.15.2
Link to section 'Module' of 'qt' Module
You can load the modules by:
module load qt
quantum-espresso
Link to section 'Description' of 'quantum-espresso' Description
Quantum ESPRESSO is an integrated suite of Open-Source computer codes for electronic-structure calculations and materials modeling at the nanoscale. It is based on density-functional theory, plane waves, and pseudopotentials.
Link to section 'Versions' of 'quantum-espresso' Versions
- Bell: 6.2.1, 6.3, 6.6
- Scholar: 7.3.1
- Negishi: 7.1
- Anvil: 6.7, 7.2, 7.3
Link to section 'Module' of 'quantum-espresso' Module
You can load the modules by:
module load quantum-espresso
r
Link to section 'Description' of 'r' Description
Linear and nonlinear modelling, statistical tests, time series analysis, classification, clustering, etc. Please consult the R project homepage for further information.
Link to section 'Versions' of 'r' Versions
- Bell: 3.6.3, 4.0.0, 4.1.1, 4.1.2, 4.2.2, 4.3.1, 4.4.1
- Scholar: 4.1.1, 4.4.1
- Gilbreth: 3.6.1, 3.6.3, 4.0.0, 4.1.2, 4.2.2, 4.3.1
- Negishi: 4.1.1, 4.2.2, 4.3.1, 4.4.1
- Anvil: 4.0.5, 4.1.0, 4.1.1, 4.4.1
- Gautschi: 4.1.1, 4.4.1
Link to section 'Module' of 'r' Module
You can load the modules by:
module load r
Link to section 'Setting Up R Preferences with .Rprofile' of 'r' Setting Up R Preferences with .Rprofile
Different clusters have different hardware and softwares. So, if you have access to multiple clusters, you must install your R packages separately for each cluster. Each cluster has multiple versions of R and packages installed with one version of R may not work with another version of R. So, libraries for each R version must be installed in a separate directory. You can define the directory where your R packages will be installed using the environment variable R_LIBS_USER
.
For your convenience, a sample .Rprofile example file is provided that can be downloaded to your cluster account and renamed into /.Rprofile
(or appended to one) to customize your installation preferences. Detailed instructions:
curl -#LO https://www.rcac.purdue.edu/files/knowledge/run/examples/apps/r/Rprofile_example
mv -ib Rprofile_example ~/.Rprofile
The above installation step needs to be done only once on each of the clusters you have access to. Now load the R module and run R to confirm the unique libPaths:
module load r/4.2.2
R
R> .libPaths()
[1] "/home/zhan4429/R/bell/4.2.2-gcc-9.3.0-xxbnk6s"
[2] "/apps/spack/bell/apps/r/4.2.2-gcc-9.3.0-xxbnk6s/rlib/R/library"
Link to section 'Challenging packages' of 'r' Challenging packages
Below are packages users may have difficulty in installation.
Link to section 'nloptr' of 'r' nloptr
In Bell, the installation may fail due to the default `cmake` version is too old. The solution is easy, users just need to load the newer versions of cmake
:
module load cmake/3.20.6
module load r
Rscript -e 'install.packages("nloptr")'
In Brown or other older clusters, because our system's cmake
and gcc
compilers are old, we may not be able to install the latest version of nloptr
. The walkaround is that users can install the older versions of nloptr
:
module load r
R
> myrepos = c("https://cran.case.edu")
> install.packages("devtools", repos = myrepos)
> library(devtools)
> install_version("nloptr", version = "> 1.2.2, < 2.0.0", repos = myrepos)
Link to section 'Error: C++17 standard requested but CXX17 is not defined' of 'r' Error: C++17 standard requested but CXX17 is not defined
When users want to install some packages, such as colourvalues
, the installation may fail due to Error: C++17 standard requested but CXX17 is not defined
. Please follow the below command to fix it:
module load r
module spider gcc
module load gcc/xxx ## the lateste gcc is recommended
mkdir -p ~/.R
echo 'CXX17 = g++ -std=gnu++17 -fPIC' > ~/.R/Makevars
R
> install.packages("xxxx")
Link to section 'RCurl' of 'r' RCurl
Some R packages rely on curl. When you install these packages such as RCurl, you may see such error:checking for curl-config... no Cannot find curl-config
To install such packages, you need to load the curl module:
module load curl
module load r
R
> install.packages("RCurl")
Link to section 'raster, stars and sf' of 'r' raster, stars and sf
These R packages have some dependencies. To install them, users will need to load several modules. Note that these modules have multiple versions, and the latest version is recommended. However, the default version may not be the latest version. To check the latest version, please runmodule spider XX
.
module spider gdal
module spider geos
module spider proj
module spider sqlite
module load gdal/XXX geos/XXX proj/XXX sqlite/XXX ## XXX is the version to use. The latest version is recommended.
module load r/XXX
R
> install.packages("raster")
install.packages("stars")
install.packages("sf")
Running R jobs
This section illustrates how to submit a small R job to a SLURM queue. The example job computes a Pythagorean triple.
Prepare an R input file with an appropriate filename, here named myjob.R:
# FILENAME: myjob.R
# Compute a Pythagorean triple.
a = 3
b = 4
c = sqrt(a*a + b*b)
c # display result
Prepare a job submission file with an appropriate filename, here named myjob.sub:
#!/bin/bash
# FILENAME: myjob.sub
module load r
# --vanilla:
# --no-save: do not save datasets at the end of an R session
R --vanilla --no-save < myjob.R
For other examples or R jobs:
Installing R packages
Link to section 'Challenges of Managing R Packages in the Cluster Environment' of 'Installing R packages' Challenges of Managing R Packages in the Cluster Environment
- Different clusters have different hardware and softwares. So, if you have access to multiple clusters, you must install your R packages separately for each cluster.
- Each cluster has multiple versions of R and packages installed with one version of R may not work with another version of R. So, libraries for each R version must be installed in a separate directory.
- You can define the directory where your R packages will be installed using the environment variable
R_LIBS_USER
. - For your convenience, a sample ~/.Rprofile example file is provided that can be downloaded to your cluster account and renamed into
~/.Rprofile
(or appended to one) to customize your installation preferences. Detailed instructions.
Link to section 'Installing Packages' of 'Installing R packages' Installing Packages
-
Step 0: Set up installation preferences.
Follow the steps for setting up your~/.Rprofile
preferences. This step needs to be done only once. If you have created a~/.Rprofile
file previously on a resource, ignore this step. -
Step 1: Check if the package is already installed.
As part of the R installations on community clusters, a lot of R libraries are pre-installed. You can check if your package is already installed by opening an R terminal and entering the commandinstalled.packages()
. For example,module load r/4.1.2 R
installed.packages()["units",c("Package","Version")] Package Version "units" "0.6-3" quit()
If the package you are trying to use is already installed, simply load the library, e.g.,
library('units')
. Otherwise, move to the next step to install the package. -
Step 2: Load required dependencies. (if needed)
For simple packages you may not need this step. However, some R packages depend on other libraries. For example, thesf
package depends ongdal
andgeos
libraries. So, you will need to load the corresponding modules before installingsf
. Read the documentation for the package to identify which modules should be loaded.module load gdal module load geos
-
Step 3: Install the package.
Now install the desired package using the commandinstall.packages('package_name')
. R will automatically download the package and all its dependencies from CRAN and install each one. Your terminal will show the build progress and eventually show whether the package was installed successfully or not.R
install.packages('sf', repos="https://cran.case.edu/") Installing package into ‘/home/myusername/R/the-resource/4.0.0’ (as ‘lib’ is unspecified) trying URL 'https://cran.case.edu/src/contrib/sf_0.9-7.tar.gz' Content type 'application/x-gzip' length 4203095 bytes (4.0 MB) ================================================== downloaded 4.0 MB ... ... more progress messages ... ... ** testing if installed package can be loaded from final location ** testing if installed package keeps a record of temporary installation path * DONE (sf) The downloaded source packages are in ‘/tmp/RtmpSVAGio/downloaded_packages’
- Step 4: Troubleshooting. (if needed)
If Step 3 ended with an error, you need to investigate why the build failed. Most common reason for build failure is not loading the necessary modules.
Link to section 'Loading Libraries' of 'Installing R packages' Loading Libraries
Once you have packages installed you can load them with the library()
function as shown below:
library('packagename')
The package is now installed and loaded and ready to be used in R.
Link to section 'Example: Installing dplyr' of 'Installing R packages' Example: Installing dplyr
The following demonstrates installing the dplyr
package assuming the above-mentioned custom ~/.Rprofile
is in place (note its effect in the "Installing package into" information message):
module load r
R
install.packages('dplyr', repos="http://ftp.ussg.iu.edu/CRAN/")
Installing package into ‘/home/myusername/R/the-resource/4.0.0’
(as ‘lib’ is unspecified)
...
also installing the dependencies 'crayon', 'utf8', 'bindr', 'cli', 'pillar', 'assertthat', 'bindrcpp', 'glue', 'pkgconfig', 'rlang', 'Rcpp', 'tibble', 'BH', 'plogr'
...
...
...
The downloaded source packages are in
'/tmp/RtmpHMzm9z/downloaded_packages'
library(dplyr)
Attaching package: 'dplyr'
For more information about installing R packages:
Loading Data into R
R is an environment for manipulating data. In order to manipulate data, it must be brought into the R environment. R has a function to read any file that data is stored in. Some of the most common file types like comma-separated variable(CSV) files have functions that come in the basic R packages. Other less common file types require additional packages to be installed. To read data from a CSV file into the R environment, enter the following command in the R prompt:
> read.csv(file = "path/to/data.csv", header = TRUE)
When R reads the file it creates an object that can then become the target of other functions. By default the read.csv() function will give the object the name of the .csv file. To assign a different name to the object created by read.csv enter the following in the R prompt:
> my_variable <- read.csv(file = "path/to/data.csv", header = FALSE)
To display the properties (structure) of loaded data, enter the following:
> str(my_variable)
For more functions and tutorials:
Setting Up R Preferences with .Rprofile
For your convenience, a sample ~/.Rprofile example file is provided that can be downloaded to your cluster account and renamed into ~/.Rprofile
(or appended to one). Follow these steps to download our recommended ~/.Rprofile
example and copy it into place:
curl -#LO https://www.rcac.purdue.edu/files/knowledge/run/examples/apps/r/Rprofile_example
mv -ib Rprofile_example ~/.Rprofile
The above installation step needs to be done only once on ${resource.name}. Now load the R module and run R:
module load r/4.1.2
R
.libPaths()
[1] "/home/myusername/R/the-resource/4.1.2-gcc-6.3.0-ymdumss"
[2] "/apps/spack/the-resource/apps/r/4.1.2-gcc-6.3.0-ymdumss/rlib/R/library"
.libPaths()
should output something similar to above if it is set up correctly.
You are now ready to install R packages into the dedicated directory /home/myusername/R/the-resource/4.1.2-gcc-6.3.0-ymdumss
.
rocm
Link to section 'Description' of 'rocm' Description
ROCm Application for Reporting System Info
Link to section 'Versions' of 'rocm' Versions
- Bell: 5.2.0
- Negishi: 6.0.0, 6.1.2
Link to section 'Module' of 'rocm' Module
You can load the modules by:
module load rocm
rstudio
Link to section 'Description' of 'rstudio' Description
This package installs Rstudio desktop from pre-compiled binaries available in the Rstudio website. The installer assumes that you are running on CentOS7/Redhat7/Fedora19. Please fix the download URL for other systems.
Link to section 'Versions' of 'rstudio' Versions
- Bell: 1.3.1073, 1.3.959, 2021.09, 2022.07, 2023.06, 2023.12
- Scholar: 2024.09
- Gilbreth: 1.2.1335, 1.3.959, 2021.09, 2022.07, 2023.06
- Negishi: 2023.06, 2024.04.2
- Anvil: 2021.09.0, 2024.04.2
- Gautschi: 2024.09
Link to section 'Module' of 'rstudio' Module
You can load the modules by:
module load rstudio
samtools
Link to section 'Description' of 'samtools' Description
SAM Tools provide various utilities for manipulating alignments in the SAM format, including sorting, merging, indexing and generating alignments in a per-position format
Link to section 'Versions' of 'samtools' Versions
- Anvil: 1.12, 1.15, 1.16, 1.17, 1.9
- Bell: 1.15, 1.16, 1.17, 1.9
- Negishi: 1.15, 1.16, 1.17, 1.9
- Scholar: 1.15, 1.16, 1.17, 1.9
- Gautschi: 1.9, 1.15, 1.16, 1.17
Link to section 'Commands' of 'samtools' Commands
- ace2sam
- blast2sam.pl
- bowtie2sam.pl
- export2sam.pl
- fasta-sanitize.pl
- interpolate_sam.pl
- maq2sam-long
- maq2sam-short
- md5fa
- md5sum-lite
- novo2sam.pl
- plot-ampliconstats
- plot-bamstats
- psl2sam.pl
- sam2vcf.pl
- samtools
- samtools.pl
- seq_cache_populate.pl
- soap2sam.pl
- wgsim
- wgsim_eval.pl
- zoom2sam.pl
Link to section 'Module' of 'samtools' Module
You can load the modules by:
module load samtools
Link to section 'Example job' of 'samtools' Example job
To run Samtools on our clusters:
#!/bin/bash
#SBATCH -A myallocation # Allocation name
#SBATCH -t 1:00:00
#SBATCH -N 1
#SBATCH -n 1
#SBATCH --job-name=samtools
#SBATCH --mail-type=FAIL,BEGIN,END
#SBATCH --error=%x-%J-%u.err
#SBATCH --output=%x-%J-%u.out
module load samtools
samtools sort my.sam > my_sorted.bam
samtools index my_sorted.bam
sentaurus
Link to section 'Description' of 'sentaurus' Description
Sentaurus is a suite of TCAD tools which simulates the fabrication, operation and reliability of semiconductor devices. The Sentaurus simulators use physical models to represent the wafer fabrication steps and device operation, thereby allowing the exploration and optimization of new semiconductor devices.
Link to section 'Versions' of 'sentaurus' Versions
- Bell: 2017.09, 2019.03
- Negishi: 2021.06, 2022.3
Link to section 'Module' of 'sentaurus' Module
You can load the modules by:
module load sentaurus
spark
Link to section 'Description' of 'spark' Description
Apache Spark is a fast and general engine for large-scale data processing.
Link to section 'Versions' of 'spark' Versions
- Bell: 2.4.4
- Gilbreth: 2.4.4
- Negishi: 3.1.1
- Anvil: 3.1.1
Link to section 'Module' of 'spark' Module
You can load the modules by:
module load spark
sqlite
Link to section 'Description' of 'sqlite' Description
SQLite3 is an SQL database engine in a C library. Programs that link the SQLite3 library can have SQL database access without running a separate RDBMS process.
Link to section 'Versions' of 'sqlite' Versions
- Bell: 3.30.1, 3.46.0
- Gilbreth: 3.30.1
- Negishi: 3.39.4
- Anvil: 3.35.5
Link to section 'Module' of 'sqlite' Module
You can load the modules by:
module load sqlite
stata-mp
Link to section 'Description' of 'stata-mp' Description
Stata/MP is the fastest and largest edition of Stata. Stata is a complete, integrated software package that provides all your data science needs—data manipulation, visualization, statistics, and automated reporting.
Link to section 'Versions' of 'stata-mp' Versions
- Bell: 17, 18
- Scholar: 17, 18
Link to section 'Module' of 'stata-mp' Module
You can load the modules by:
module load stata-mp
stata
Link to section 'Description' of 'stata' Description
Stata is a complete, integrated software package that provides all your data science needs—data manipulation, visualization, statistics, and automated reporting.
Link to section 'Versions' of 'stata' Versions
- Bell: 18
- Scholar: 17, 18
- Negishi: 18
- Gautschi: 18
Link to section 'Module' of 'stata' Module
You can load the modules by:
module load stata
subversion
Link to section 'Description' of 'subversion' Description
Apache Subversion - an open source version control system.
Link to section 'Versions' of 'subversion' Versions
- Bell: 1.12.2
Link to section 'Module' of 'subversion' Module
You can load the modules by:
module load subversion
swig
Link to section 'Description' of 'swig' Description
SWIG is an interface compiler that connects programs written in C and C++ with scripting languages such as Perl, Python, Ruby, and Tcl. It works by taking the declarations found in C/C++ header files and using them to generate the wrapper code that scripting languages need to access the underlying C/C++ code. In addition, SWIG provides a variety of customization features that let you tailor the wrapping process to suit your application.
Link to section 'Versions' of 'swig' Versions
- Negishi: 4.0.2
- Anvil: 4.0.2
Link to section 'Module' of 'swig' Module
You can load the modules by:
module load swig
tcl
Link to section 'Description' of 'tcl' Description
Tcl Tool Command Language is a very powerful but easy to learn dynamic programming language, suitable for a very wide range of uses, including web and desktop applications, networking, administration, testing and many more. Open source and business-friendly, Tcl is a mature yet evolving language that is truly cross platform, easily deployed and highly extensible.
Link to section 'Versions' of 'tcl' Versions
- Bell: 8.6.8, 8.6.12
- Gilbreth: 8.6.8
- Negishi: 8.6.11, 8.6.12
- Anvil: 8.6.11
Link to section 'Module' of 'tcl' Module
You can load the modules by:
module load tcl
tensorflow
Link to section 'Description' of 'tensorflow' Description
TensorFlow is an open source software library for numerical computation using data flow graphs. Nodes in the graph represent mathematical operations, while the graph edges represent the multidimensional data arrays (tensors) communicated between them. The flexible architecture allows you to deploy computation to one or more CPUs or GPUs in a desktop, server, or mobile device with a single API.
Link to section 'Versions' of 'tensorflow' Versions
- Bell: 2.3.0
- Anvil: 2.6.0
- Gilbreth: 2.4.0, 1.12.0
- Scholar: 1.15.2, 1.15.4, 1.15.5, 2.1.0, 2.2.0, 2.3.1, 2.5.0, 2.6.0
Link to section 'Module' of 'tensorflow' Module
You can load the modules by:
module load learning
module load tensorflow
To check the loaded version, run:
python -c "import tensorflow as tf; print(tf.__version__)"
texinfo
Link to section 'Description' of 'texinfo' Description
Texinfo is the official documentation format of the GNU project. It was invented by Richard Stallman and Bob Chassell many years ago, loosely based on Brian Reids Scribe and other formatting languages of the time. It is used by many non-GNU projects as well.
Link to section 'Versions' of 'texinfo' Versions
- Bell: 6.7
Link to section 'Module' of 'texinfo' Module
You can load the modules by:
module load texinfo
texlive
Link to section 'Description' of 'texlive' Description
TeX Live is a free software distribution for the TeX typesetting system. Heads up, its is not a reproducible installation. At any point only the most recent version can be installed. Older versions are included for backward compatibility, i.e., if you have that version already installed.
Link to section 'Versions' of 'texlive' Versions
- Bell: 20200406, 20220321
- Scholar: 20220321
- Gilbreth: 20200406
- Negishi: 20220321
- Anvil: 20200406
- Gautschi: 20220321
Link to section 'Module' of 'texlive' Module
You can load the modules by:
module load texlive
tflearn
Link to section 'Description' of 'tflearn' Description
TFlearn is a modular and transparent deep learning library built on top of Tensorflow. It was designed to provide a higher-level API to TensorFlow in order to facilitate and speed-up experimentations, while remaining fully transparent and compatible with it.
Link to section 'Versions' of 'tflearn' Versions
- Gilbreth: 0.3.2
Link to section 'Module' of 'tflearn' Module
You can load the modules by:
module load learning
module load tflearn
theano
Link to section 'Description' of 'theano' Description
Theano is a Python library that allows you to define, optimize, and evaluate mathematical expressions involving multi-dimensional arrays efficiently. Theano is most commonly used to perform Deep Learning and has excellent GPU support and integration through PyCUDA.
Link to section 'Versions' of 'theano' Versions
- Bell: 1.0.5
- Gilbreth: 1.0.3, 1.0.5
Link to section 'Module' of 'theano' Module
You can load the modules by:
module load learning
module load theano
thermocalc
Link to section 'Description' of 'thermocalc' Description
Thermo-Calc allows you to calculate the state for a given thermodynamic system to obtain insight.
Link to section 'Versions' of 'thermocalc' Versions
- Bell: 2019b, 2020a, 2021b
Link to section 'Module' of 'thermocalc' Module
You can load the modules by:
module load thermocalc
tk
Link to section 'Description' of 'tk' Description
Tk is a graphical user interface toolkit that takes developing desktop applications to a higher level than conventional approaches. Tk is the standard GUI not only for Tcl, but for many other dynamic languages, and can produce rich, native applications that run unchanged across Windows, Mac OS X, Linux and more.
Link to section 'Versions' of 'tk' Versions
- Bell: 8.6.8, 8.6.11
- Gilbreth: 8.6.8
- Negishi: 8.6.11
- Anvil: 8.6.11
- Gautschi: 8.6.11
Link to section 'Module' of 'tk' Module
You can load the modules by:
module load tk
tophat
Link to section 'Description' of 'tophat' Description
Spliced read mapper for RNA-Seq.
Link to section 'Versions' of 'tophat' Versions
- Anvil: 2.1.1, 2.1.2
- Bell: 2.1.1
- Negishi: 2.1.1
- Scholar: 2.1.1-py27
- Gautschi: 2.1.1-py27
Link to section 'Commands' of 'tophat' Commands
- bam2fastx
- bam_merge
- bed_to_juncs
- contig_to_chr_coords
- fix_map_ordering
- gtf_juncs
- gtf_to_fasta
- juncs_db
- long_spanning_reads
- map2gtf
- prep_reads
- sam_juncs
- samtools_0.1.18
- segment_juncs
- sra_to_solid
- tophat
- tophat2
- tophat-fusion-post
- tophat_reports
Link to section 'Module' of 'tophat' Module
You can load the modules by:
module load tophat
Link to section 'Example job' of 'tophat' Example job
To run TopHat on our clusters:
#!/bin/bash
#SBATCH -A myallocation # Allocation name
#SBATCH -t 1:00:00
#SBATCH -N 1
#SBATCH -n 1
#SBATCH --job-name=tophat
#SBATCH --mail-type=FAIL,BEGIN,END
#SBATCH --error=%x-%J-%u.err
#SBATCH --output=%x-%J-%u.out
module load tophat
tophat -r 20 test_ref reads_1.fq reads_2.fq
trimmomatic
Link to section 'Description' of 'trimmomatic' Description
A flexible read trimming tool for Illumina NGS data.
Link to section 'Versions' of 'trimmomatic' Versions
- Anvil: 0.39
- Bell: 0.39
- Negishi: 0.39
- Scholar: 0.39
- Gautschi: 0.39
Link to section 'Commands' of 'trimmomatic' Commands
- trimmomatic
Link to section 'Module' of 'trimmomatic' Module
You can load the modules by:
module load trimmomatic
Link to section 'Example job' of 'trimmomatic' Example job
To run Trimmomatic on our clusters:
#!/bin/bash
#SBATCH -A myallocation # Allocation name
#SBATCH -t 1:00:00
#SBATCH -N 1
#SBATCH -n 8
#SBATCH --job-name=trimmomatic
#SBATCH --mail-type=FAIL,BEGIN,END
#SBATCH --error=%x-%J-%u.err
#SBATCH --output=%x-%J-%u.out
module load trimmomatic
trimmomatic PE -threads 8 \
input_forward.fq.gz input_reverse.fq.gz \
output_forward_paired.fq.gz output_forward_unpaired.fq.gz \
output_reverse_paired.fq.gz output_reverse_unpaired.fq.gz \
ILLUMINACLIP:TruSeq3-PE.fa:2:30:10:2:True LEADING:3 TRAILING:3 MINLEN:36
ucx
Link to section 'Description' of 'ucx' Description
a communication library implementing high-performance messaging for MPI/PGAS frameworks
Link to section 'Versions' of 'ucx' Versions
- Anvil: 1.11.2
- Gilbreth: 1.13.0
Link to section 'Module' of 'ucx' Module
You can load the modules by:
module load ucx
udunits
Link to section 'Description' of 'udunits' Description
Automated units conversion
Link to section 'Versions' of 'udunits' Versions
- Negishi: 2.2.28
- Anvil: 2.2.28
Link to section 'Module' of 'udunits' Module
You can load the modules by:
module load udunits
udunits2
Link to section 'Description' of 'udunits2' Description
Automated units conversion
Link to section 'Versions' of 'udunits2' Versions
- Bell: 2.2.24
- Gilbreth: 2.2.24
Link to section 'Module' of 'udunits2' Module
You can load the modules by:
module load udunits2
valgrind
Link to section 'Description' of 'valgrind' Description
An instrumentation framework for building dynamic analysis.
Link to section 'Versions' of 'valgrind' Versions
- Bell: 3.15.0
- Scholar: 3.22.0
- Gilbreth: 3.13.0
- Negishi: 3.19.0
- Anvil: 3.15.0
Link to section 'Module' of 'valgrind' Module
You can load the modules by:
module load valgrind
vasp
Link to section 'Description' of 'vasp' Description
The Vienna Ab initio Simulation Package VASP is a computer program for atomic scale materials modelling, e.g. electronic structure calculations and quantum-mechanical molecular dynamics, from first principles.
Link to section 'Versions' of 'vasp' Versions
- Anvil: 5.4.4.pl2-vtst, 5.4.4.pl2, 6.3.0-sol, 6.3.0-vtst, 6.3.0-wannier90, 6.3.0
- Gilbreth: 6.4.1
- Negishi: 5.4.4.pl2, 6.4.1_vtst, 6.4.1
Link to section 'Module' of 'vasp' Module
You can load the modules by:
module load vasp
vcftools
Link to section 'Description' of 'vcftools' Description
VCFtools is a program package designed for working with VCF files, such as those generated by the 1000 Genomes Project. The aim of VCFtools is to provide easily accessible methods for working with complex genetic variation data in the form of VCF files.
Link to section 'Versions' of 'vcftools' Versions
- Anvil: 0.1.14, 0.1.16
- Bell: 0.1.16
- Negishi: 0.1.16
- Scholar: 0.1.16
- Gautschi: 0.1.16
Link to section 'Commands' of 'vcftools' Commands
- fill-aa
- fill-an-ac
- fill-fs
- fill-ref-md5
- vcf-annotate
- vcf-compare
- vcf-concat
- vcf-consensus
- vcf-contrast
- vcf-convert
- vcf-fix-newlines
- vcf-fix-ploidy
- vcf-indel-stats
- vcf-isec
- vcf-merge
- vcf-phased-join
- vcf-query
- vcf-shuffle-cols
- vcf-sort
- vcf-stats
- vcf-subset
- vcftools
- vcf-to-tab
- vcf-tstv
- vcf-validator
Link to section 'Module' of 'vcftools' Module
You can load the modules by:
module load vcftools
Link to section 'Example job' of 'vcftools' Example job
To run VCFtools on our clusters:
#!/bin/bash
#SBATCH -A myallocation # Allocation name
#SBATCH -t 1:00:00
#SBATCH -N 1
#SBATCH -n 1
#SBATCH --job-name=vcftools
#SBATCH --mail-type=FAIL,BEGIN,END
#SBATCH --error=%x-%J-%u.err
#SBATCH --output=%x-%J-%u.out
module load vcftools
vcftools --vcf input_data.vcf --chr 1 \
--from-bp 1000000 --to-bp 2000000
vim
Link to section 'Description' of 'vim' Description
Vim is a highly configurable text editor built to enable efficient text editing. It is an improved version of the vi editor distributed with most UNIX systems. Vim is often called a programmers editor, and so useful for programming that many consider it an entire IDE. Its not just for programmers, though. Vim is perfect for all kinds of text editing, from composing email to editing configuration files.
Link to section 'Versions' of 'vim' Versions
- Bell: 8.1.2141
- Gilbreth: 7.4.2367
- Scholar: 8.2
Link to section 'Module' of 'vim' Module
You can load the modules by:
module load vim
visit
Link to section 'Description' of 'visit' Description
VisIt is an Open Source, interactive, scalable, visualization, animation and analysis tool. Description
Link to section 'Versions' of 'visit' Versions
- Anvil: 3.1.4
- Negishi: 3.3.3
Link to section 'Module' of 'visit' Module
You can load the modules by:
module load visit
vlc
Link to section 'Description' of 'vlc' Description
VLC is a free and open source multimedia player for most multimedia formats.
Link to section 'Versions' of 'vlc' Versions
- Bell: 3.0.9.2
- Gilbreth: 3.0.9.2
- Anvil: 3.0.9.2
- Negishi: 3.0.9.2
Link to section 'Module' of 'vlc' Module
You can load the modules by:
module load vlc
vscode
Link to section 'Description' of 'vscode' Description
Visual Studio Code
Link to section 'Versions' of 'vscode' Versions
- Bell: 1.56, 1.59
- Gilbreth: 1.56, 1.59
- Anvil: 1.61.2
- Negishi: 1.79.2
Link to section 'Module' of 'vscode' Module
You can load the modules by:
module load vscode
vtk
Link to section 'Description' of 'vtk' Description
The Visualization Toolkit VTK is an open-source, freely available software system for 3D computer graphics, image processing and visualization.
Link to section 'Versions' of 'vtk' Versions
- Negishi: 9.0.0
- Anvil: 9.0.0
Link to section 'Module' of 'vtk' Module
You can load the modules by:
module load vtk
wannier90
Link to section 'Description' of 'wannier90' Description
Wannier90 is an open-source code released under GPLv2 for generating maximally-localized Wannier functions and using them to compute advanced electronic properties of materials with high efficiency and accuracy.
Link to section 'Versions' of 'wannier90' Versions
- Anvil: 3.1.0
- Bell: 3.1.0
Link to section 'Module' of 'wannier90' Module
You can load the modules by:
module load wannier90
xalt
Link to section 'Versions' of 'xalt' Versions
- Bell: 1.1.2
- Anvil: 2.10.45
- Scholar: 1.1.2, 2.7.1
- Gilbreth: 1.1.2
- Negishi: 3.0.2
Link to section 'Module' of 'xalt' Module
You can load the modules by:
module load xalt
zlib
Link to section 'Description' of 'zlib' Description
A free, general-purpose, legally unencumbered lossless data-compression library.
Link to section 'Versions' of 'zlib' Versions
- Bell: 1.2.11, 1.2.13
- Gilbreth: 1.2.11, 1.2.11-generic
- Negishi: 1.2.13
- Anvil: 1.2.11
Link to section 'Module' of 'zlib' Module
You can load the modules by:
module load zlib
zstd
Link to section 'Description' of 'zstd' Description
Zstandard, or zstd as short version, is a fast lossless compression algorithm, targeting real-time compression scenarios at zlib-level and better compression ratios. It's backed by a very fast entropy stage, provided by Huff0 and FSE library.
Link to section 'Versions' of 'zstd' Versions
- Negishi: 1.5.2
Link to section 'Module' of 'zstd' Module
You can load the modules by:
module load zstd
nextflow
Link to section 'Description' of 'nextflow' Description
Nextflow is a bioinformatics workflow manager that enables the development of portable and reproducible workflows. It supports deploying workflows on a variety of execution platforms including local, HPC schedulers, AWS Batch, Google Cloud Life Sciences, and Kubernetes. Additionally, it provides support for manage your workflow dependencies through built-in support for Conda, Spack, Docker, Podman, Singularity, Modules, and more.
Link to section 'Versions' of 'nextflow' Versions
- Negishi: 21.10.0, 22.10.1, 23.04.1
- Anvil: 21.10.0, 22.10.1
- Bell: 21.10.0, 22.10.4
- Scholar: 21.10.0
- Gautschi: 21.10.0
Link to section 'Module' of 'nextflow' Module
You can load the modules by:
module load nextflow
Note: Docker is not available on Purdue clusters, so use "-profile singularity", environment modules, or conda for running NextFlow pipelines.
Running Nextflow can be computing or memory intensive. Please do not run it on login nodes, as this might affect other users sharing the same login node with you.
Link to section 'Wrap nextflow into slurm jobscript' of 'nextflow' Wrap nextflow into slurm jobscript
The easiest method to use nextflow on clusters is to place the nextflow run command into a batch script and submitting it to Slurm with sbatch. The manager process will run on the allocated compute node, and all tasks are configured to use the local executor.
#!/bin/bash
#SBATCH -A myQueue
#SBATCH -t 1:00:00
#SBATCH -N 1
#SBATCH -n 12
#SBATCH --job-name=nextflow
#SBATCH --mail-type=FAIL,BEGIN,END
#SBATCH --error=%x-%J-%u.err
#SBATCH --output=%x-%J-%u.out
module load nextflow
nextflow run main.nf -profile singularity
Link to section 'Nextflow submits tasks as slurm jobs' of 'nextflow' Nextflow submits tasks as slurm jobs
Nextflow can also submit its tasks to Slurm instead of running them on the local host. Place the following file named nextflow.config in your Nextflow working directory:
process {
executor = 'slurm'
queueSize = 50
pollInterval = '1 min'
queueStatInterval = '5 min'
submitRateLimit = '10 sec'
}
Please do not change the above default configuration. Nextflow workflow manager process can generate a disruptive amount of communication requests to Slurm and the configuration file is used to reduce the frequency of those requests.
Link to section 'clusterOptions' of 'nextflow' clusterOptions
Inside the individual process definitions in your scripts, you will need to specify the clusterOptions variable to provide your queue and computing resources appropriate for that task. This can be done by adding something in the pattern of clusterOptions='-A standby -N1 -n1 -c12 -t 1:00:00' to the top of your task process blocks.
Below is a simple example to run Fastqc:
nextflow.enable.dsl=2
process FASTQC {
clusterOptions='-A standby -N1 -n1 -c4 -t 00:30:00'
input:
path reads
script:
"""
mkdir -p fastqc_out
module load biocontainers fastqc
fastqc -o fastqc_out ${reads}
"""
}
reads_ch = Channel.fromPath( 'reads/fastq/*.fastq.gz' )
workflow {
FASTQC(reads_ch)
}
Using clusterOptions='-A standby -N1 -n1 -c4 -t 00:30:00' , each nextflow task will be submitted to standby queue requesting 4 cores and 30 mins walltime.
grace
Link to section 'Description' of 'grace' Description
Grace is a WYSIWYG 2D plotting tool for the X Window System and M*tif.
Link to section 'Versions' of 'grace' Versions
-
Negishi: 5.1.25
Link to section 'Module' of 'grace' Module
You can load the modules by:
module load grace
imagemagick
Link to section 'Description' of 'imagemagick' Description
ImageMagick is a software suite to create, edit, compose, or convert bitmap images.
Link to section 'Versions' of 'imagemagick' Versions
- Bell: 7.0.8-7
- Negishi: 7.0.8-7
Link to section 'Module' of 'imagemagick' Module
You can load the modules by:
module load imagemagick
xcb-util-image
Link to section 'Description' of 'xcb-util-image' Description
The XCB util modules provides a number of libraries which sit on top of libxcb, the core X protocol library, and some of the extension libraries. These experimental libraries provide convenience functions and interfaces which make the raw X protocol more usable. Some of the libraries also provide client-side code which is not strictly part of the X protocol but which have traditionally been provided by Xlib.
Link to section 'Versions' of 'xcb-util-image' Versions
- Negishi: 0.4.0
- Anvil: 0.4.0
- Gautschi: 0.4.1
- Scholar: 0.4.1
Link to section 'Module' of 'xcb-util-image' Module
You can load the modules by:
module load xcb-util-image
xcb-util-keysyms
Link to section 'Description' of 'xcb-util-keysyms' Description
The XCB util modules provides a number of libraries which sit on top of libxcb, the core X protocol library, and some of the extension libraries. These experimental libraries provide convenience functions and interfaces which make the raw X protocol more usable. Some of the libraries also provide client-side code which is not strictly part of the X protocol but which have traditionally been provided by Xlib.
Link to section 'Versions' of 'xcb-util-keysyms' Versions
- Negishi: 0.4.0
- Anvil: 0.4.0
- Gautschi: 0.4.1
- Scholar: 0.4.1
Link to section 'Module' of 'xcb-util-keysyms' Module
You can load the modules by:
module load xcb-util-keysyms
xcb-util-renderutil
Link to section 'Description' of 'xcb-util-renderutil' Description
The XCB util modules provides a number of libraries which sit on top of libxcb, the core X protocol library, and some of the extension libraries. These experimental libraries provide convenience functions and interfaces which make the raw X protocol more usable. Some of the libraries also provide client-side code which is not strictly part of the X protocol but which have traditionally been provided by Xlib.
Link to section 'Versions' of 'xcb-util-renderutil' Versions
- Negishi: 0.3.9
- Anvil: 0.3.9
- Gautschi: 0.3.10
- Scholar: 0.3.10
Link to section 'Module' of 'xcb-util-renderutil' Module
You can load the modules by:
module load xcb-util-renderutil
xcb-util-wm
Link to section 'Description' of 'xcb-util-wm' Description
The XCB util modules provides a number of libraries which sit on top of libxcb, the core X protocol library, and some of the extension libraries. These experimental libraries provide convenience functions and interfaces which make the raw X protocol more usable. Some of the libraries also provide client-side code which is not strictly part of the X protocol but which have traditionally been provided by Xlib.
Link to section 'Versions' of 'xcb-util-wm' Versions
- Negishi: 0.4.1
- Anvil: 0.4.1
- Gautschi: 0.4.2
- Scholar: 0.4.2
Link to section 'Module' of 'xcb-util-wm' Module
You can load the modules by:
module load xcb-util-wm
libxscrnsaver
Link to section 'Description' of 'libxscrnsaver' Description
XScreenSaver - X11 Screen Saver extension client library
Link to section 'Versions' of 'libxscrnsaver' Versions
- Negishi: 1.2.2
- Anvil: 1.2.2
- Gautschi: 1.2.2
- Scholar: 1.2.2
Link to section 'Module' of 'libxscrnsaver' Module
You can load the modules by:
module load libxscrnsaver
libxslt
Link to section 'Description' of 'libxslt' Description
Libxslt is the XSLT C library developed for the GNOME project. XSLT itself is a an XML language to define transformation for XML. Libxslt is based on libxml2 the XML C library developed for the GNOME project. It also implements most of the EXSLT set of processor-portable extensions functions and some of Saxons evaluate and expressions extensions.
Link to section 'Versions' of 'libxslt' Versions
- Negishi: 1.1.33
- Anvil: 1.1.33
- Gautschi: 1.1.33
- Scholar: 1.1.33
Link to section 'Module' of 'libxslt' Module
You can load the modules by:
module load libxslt
mesa-glu
Link to section 'Description' of 'mesa-glu' Description
This package provides the Mesa OpenGL Utility library.
Link to section 'Versions' of 'mesa-glu' Versions
- Negishi: 9.0.2
- Anvil: 9.0.2
- Gautschi: 9.0.2
- Scholar: 9.0.2
Link to section 'Module' of 'mesa-glu' Module
You can load the modules by:
module load mesa-glu
motif
Link to section 'Description' of 'motif' Description
Motif - Graphical user interface GUI specification and the widget toolkit
Link to section 'Versions' of 'motif' Versions
- Negishi: 2.3.8
- Gautschi: 2.3.8
- Scholar: 2.3.8
Link to section 'Module' of 'motif' Module
You can load the modules by:
module load motif
Singularity
Note: Singularity was originally a project out of Lawrence Berkeley National Laboratory. It has now been spun off into a distinct offering under a new corporate entity under the name Sylabs Inc. This guide pertains to the open source community edition, SingularityCE.
Link to section 'What is Singularity?' of 'Singularity' What is Singularity?
Singularity is a new feature of the Community Clusters allowing the portability and reproducibility of operating system and application environments through the use of Linux containers. It gives users complete control over their environment.
Singularity is like Docker but tuned explicitly for HPC clusters. More information is available from the project’s website.
Link to section 'Features' of 'Singularity' Features
- Run the latest applications on an Ubuntu or Centos userland
- Gain access to the latest developer tools
- Launch MPI programs easily
- Much more
Singularity’s user guide is available at: sylabs.io/guides/3.8/user-guide
Link to section 'Example' of 'Singularity' Example
Here is an example using an Ubuntu 16.04 image on ${resource.name}:
singularity exec /depot/itap/singularity/ubuntu1604.img cat /etc/lsb-release
DISTRIB_ID=Ubuntu
DISTRIB_RELEASE=16.04
DISTRIB_CODENAME=xenial
DISTRIB_DESCRIPTION="Ubuntu 16.04 LTS"
Here is another example using a Centos 7 image:
singularity exec /depot/itap/singularity/centos7.img cat /etc/redhat-release
CentOS Linux release 7.2.1511 (Core)
Link to section 'Purdue Cluster Specific Notes' of 'Singularity' Purdue Cluster Specific Notes
All service providers will integrate Singularity slightly differently depending on site. The largest customization will be which default files are inserted into your images so that routine services will work.
Services we configure for your images include DNS settings and account information. File systems we overlay into your images are your home directory, scratch, Data Depot, and application file systems.
Here is a list of paths:
- /etc/resolv.conf
- /etc/hosts
- /home/$USER
- /apps
- /scratch
- /depot
This means that within the container environment these paths will be present and the same as outside the container. The /apps
, /scratch
, and /depot
directories will need to exist inside your container to work properly.
Link to section 'Creating Singularity Images' of 'Singularity' Creating Singularity Images
Due to how singularity containers work, you must have root privileges to build an image. Once you have a singularity container image built on your own system, you can copy the image file up to the cluster (you do not need root privileges to run the container).
You can find information and documentation for how to install and use singularity on your system:
We have version 3.8.0-1.el7
on the cluster. You will most likely not be able to run any container built with any singularity past that version. So be sure to follow the installation guide for version 3.8 on your system.
singularity --version
singularity version 3.8.0-1.el7
Everything you need on how to build a container is available from their user-guide. Below are merely some quick tips for getting your own containers built for ${resource.name}.
You can use a Definition File to both build your container and share its specification with collaborators (for the sake of reproducibility). Here is a simplistic example of such a file:
# FILENAME: Buildfile
Bootstrap: docker
From: ubuntu:18.04
%post
apt-get update && apt-get upgrade -y
mkdir /apps /depot /scratch
To build the image itself:
sudo singularity build ubuntu-18.04.sif Buildfile
The challenge with this approach however is that it must start from scratch if you decide to change something. In order to create a container image iteratively and interactively, you can use the --sandbox
option.
sudo singularity build --sandbox ubuntu-18.04 docker://ubuntu:18.04
This will not create a flat image file but a directory tree (i.e., a folder), the contents of which are the container's filesystem. In order to get a shell inside the container that allows you to modify it, user the --writable
option.
sudo singularity shell --writable ubuntu-18.04
Singularity: Invoking an interactive shell within container...
Singularity ubuntu-18.04.sandbox:~>
You can then proceed to install any libraries, software, etc. within the container. Then to create the final image file, exit
the shell and call the build
command once more on the sandbox.
sudo singularity build ubuntu-18.04.sif ubuntu-18.04
Finally, copy the new image to ${resource.name} and run it.
Apptainer
Note: Apptainer was formerly known as Singularity and is now a part of the Linux Foundation. When migrating from Singularity see the user compatibility documentation.
Link to section 'What is Apptainer?' of 'Apptainer' What is Apptainer?
Apptainer is an open-source container platform designed to be simple, fast, and secure. It allows the portability and reproducibility of operating systems and application environments through the use of Linux containers. It gives users complete control over their environment.
Apptainer is like Docker but tuned explicitly for HPC clusters. More information is available on the project’s website.
Link to section 'Features' of 'Apptainer' Features
- Run the latest applications on an Ubuntu or Centos userland
- Gain access to the latest developer tools
- Launch MPI programs easily
- Much more
Apptainer’s user guide is available at: apptainer.org/docs/user/main/introduction.html
Link to section 'Example' of 'Apptainer' Example
Here is an example using an Ubuntu 16.04 image on ${resource.name}:
apptainer exec /depot/itap/singularity/ubuntu1604.img cat /etc/lsb-release
DISTRIB_ID=Ubuntu
DISTRIB_RELEASE=16.04
DISTRIB_CODENAME=xenial
DISTRIB_DESCRIPTION="Ubuntu 16.04 LTS"
Here is another example using a Centos 7 image:
apptainer exec /depot/itap/singularity/centos7.img cat /etc/redhat-release
CentOS Linux release 7.3.1611 (Core)
Link to section 'Purdue Cluster Specific Notes' of 'Apptainer' Purdue Cluster Specific Notes
All service providers will integrate Apptainer slightly differently depending on site. The largest customization will be which default files are inserted into your images so that routine services will work.
Services we configure for your images include DNS settings and account information. File systems we overlay into your images are your home directory, scratch, Data Depot, and application file systems.
Here is a list of paths:
- /etc/resolv.conf
- /etc/hosts
- /home/$USER
- /apps
- /scratch
- /depot
This means that within the container environment these paths will be present and the same as outside the container. The /apps
, /scratch
, and /depot
directories will need to exist inside your container to work properly.
Link to section 'Creating Apptainer Images' of 'Apptainer' Creating Apptainer Images
You can build on your system or straight on the cluster (you do not need root privileges to build or run the container).
You can find information and documentation for how to install and use Apptainer on your system:
We have version 1.1.6
(or newer) on the cluster. Please note that installed versions may change throughout cluster life time, so when in doubt, please check exact version with a --version
command line flag:
apptainer --version
apptainer version 1.1.6-1
Everything you need on how to build a container is available from their user guide. Below are merely some quick tips for getting your own containers built for ${resource.name}.
You can use a Definition File to both build your container and share its specification with collaborators (for the sake of reproducibility). Here is a simplistic example of such a file:
# FILENAME: Buildfile
Bootstrap: docker
From: ubuntu:18.04
%post
apt-get update && apt-get upgrade -y
mkdir /apps /depot /scratch
To build the image itself:
apptainer build ubuntu-18.04.sif Buildfile
The challenge with this approach however is that it must start from scratch if you decide to change something. In order to create a container image iteratively and interactively, you can use the --sandbox
option.
apptainer build --sandbox ubuntu-18.04 docker://ubuntu:18.04
This will not create a flat image file but a directory tree (i.e., a folder), the contents of which are the container's filesystem. In order to get a shell inside the container that allows you to modify it, user the --writable
option.
apptainer shell --writable ubuntu-18.04
Apptainer>
You can then proceed to install any libraries, software, etc. within the container. Then to create the final image file, exit
the shell and call the build
command once more on the sandbox.
apptainer build ubuntu-18.04.sif ubuntu-18.04
Finally, copy the new image to ${resource.name} and run it.
tecplot
Link to section 'Description' of 'tecplot' Description
Tecplot 360 is a Computational Fluid Dynamics (CFD) and numerical simulation software package used in post-processing simulation results. It is also used in chemistry applications to visualize molecule structure by post-processing charge density data.
Link to section 'Versions' of 'tecplot' Versions
- Bell: 360-2017-R3, 360-2021-R1
- Scholar: 360-2017-R3
- Gilbreth: 360-2017-R3
- Negishi: 360-2022-R2, 360-2024-R1
- Gautschi: 360-2024-R1
Link to section 'Module' of 'tecplot' Module
You can load the modules by:
module load tecplot
ncl
Link to section 'Description' of 'ncl' Description
NCL is an interpreted language designed specifically for scientific data analysis and visualization. Supports NetCDF 3/4, GRIB 1/2, HDF 4/5, HDF-EOD 2/5, shapefile, ASCII, binary. Numerous analysis functions are built-in.
Link to section 'Versions' of 'ncl' Versions
- Bell: 6.4.0
- Scholar: 6.6.2
- Gilbreth: 6.4.0
- Negishi: 6.6.2
- Anvil: 6.4.0
- Gautschi: 6.6.2
Link to section 'Module' of 'ncl' Module
You can load the modules by:
module load ncl
vmd
Link to section 'Description' of 'vmd' Description
VMD is a molecular visualization program for displaying, animating, and analyzing large biomolecular systems using 3-D graphics and built-in scripting.
Link to section 'Versions' of 'vmd' Versions
- Bell: 1.9.3
- Gilbreth: 1.9.3
- Anvil: 1.9.3
Link to section 'Module' of 'vmd' Module
You can load the modules by:
module load vmd
nf-core
Link to section 'Description' of 'nf-core' Description
A community effort to collect a curated set of analysis pipelines built using Nextflow and tools to run the pipelines.
Home page: https://nf-co.re
Link to section 'Versions' of 'nf-core' Versions
- Anvil: 2.7.2, 2.8
- Bell: 2.8
- Negishi: 2.7.2, 2.8, 2.11.1
Link to section 'Commands' of 'nf-core' Commands
___ __ __ __ ___ /,-._.--~\
|\ | |__ __ / ` / \ |__) |__ } {
| \| | \__, \__/ | \ |___ \`-._,-`-,
`._,._,'
nf-core/tools version 2.8 - https://nf-co.re
Usage: nf-core [OPTIONS] COMMAND [ARGS]...
nf-core/tools provides a set of helper tools for use with nf-core Nextflow pipelines.
It is designed for both end-users running pipelines and also developers creating new pipelines.
╭─ Options ────────────────────────────────────────────────────────────────────────────────────────╮
│ --version Show the version and exit. │
│ --verbose -v Print verbose output to the console. │
│ --hide-progress Don't show progress bars. │
│ --log-file -l Save a verbose log to a file. │
│ --help -h Show this message and exit. │
╰──────────────────────────────────────────────────────────────────────────────────────────────────╯
╭─ Commands for users ─────────────────────────────────────────────────────────────────────────────╮
│ list List available nf-core pipelines with local info. │
│ launch Launch a pipeline using a web GUI or command line prompts. │
│ download Download a pipeline, nf-core/configs and pipeline singularity images. │
│ licences List software licences for a given workflow (DSL1 only). │
╰──────────────────────────────────────────────────────────────────────────────────────────────────╯
╭─ Commands for developers ────────────────────────────────────────────────────────────────────────╮
│ create Create a new pipeline using the nf-core template. │
│ lint Check pipeline code against nf-core guidelines. │
│ modules Commands to manage Nextflow DSL2 modules (tool wrappers). │
│ subworkflows Commands to manage Nextflow DSL2 subworkflows (tool wrappers). │
│ schema Suite of tools for developers to manage pipeline schema. │
│ bump-version Update nf-core pipeline version number. │
│ sync Sync a pipeline TEMPLATE branch with the nf-core template. │
╰──────────────────────────────────────────────────────────────────────────────────────────────────╯
Link to section 'Module' of 'nf-core' Module
You can load the modules by:
module load nf-core
Link to section 'List available pipelines' of 'nf-core' List available pipelines
To check all available pipelines:
$ nf-core list
,--./,-.
___ __ __ __ ___ /,-._.--~\
|\ | |__ __ / ` / \ |__) |__ } {
| \| | \__, \__/ | \ |___ \`-._,-`-,
`._,._,'
nf-core/tools version 2.8 - https://nf-co.re
┏━━━━━━━━━━━━━━━━━━━━━━┳━━━━━━━┳━━━━━━━━━━━━━━━━┳━━━━━━━━━━━━━━━┳━━━━━━━━━━━━━┳━━━━━━━━━━━━━━━━━━━━━━━┓
┃ Pipeline Name ┃ Stars ┃ Latest Release ┃ Released ┃ Last Pulled ┃ Have latest release? ┃
┡━━━━━━━━━━━━━━━━━━━━━━╇━━━━━━━╇━━━━━━━━━━━━━━━━╇━━━━━━━━━━━━━━━╇━━━━━━━━━━━━━╇━━━━━━━━━━━━━━━━━━━━━━━┩
│ funcscan │ 29 │ 1.1.0 │ 2 days ago │ - │ - │
│ smrnaseq │ 49 │ 2.2.0 │ 4 days ago │ - │ - │
│ rnafusion │ 95 │ 2.3.4 │ 4 days ago │ - │ - │
│ rnaseq │ 604 │ 3.11.2 │ 5 days ago │ 5 days ago │ No (dev - 4b7695a) │
│ demultiplex │ 26 │ 1.2.0 │ 5 days ago │ - │ - │
│ differentialabundan… │ 19 │ 1.2.0 │ 2 weeks ago │ 2 days ago │ Yes (v1.2.0) │
│ mhcquant │ 21 │ 2.4.1 │ 3 weeks ago │ - │ - │
│ viralintegration │ 8 │ 0.1.0 │ 1 months ago │ - │ - │
│ quantms │ 8 │ 1.1.1 │ 1 months ago │ - │ - │
│ viralrecon │ 93 │ 2.6.0 │ 1 months ago │ - │ - │
│ airrflow │ 24 │ 3.0 │ 1 months ago │ - │ - │
│ scrnaseq │ 81 │ 2.2.0 │ 1 months ago │ - │ - │
│ epitopeprediction │ 25 │ 2.2.1 │ 1 months ago │ - │ - │
│ isoseq │ 12 │ 1.1.4 │ 2 months ago │ - │ - │
│ taxprofiler │ 49 │ 1.0.0 │ 2 months ago │ 2 weeks ago │ No (master - c3f1adf) │
│ nanoseq │ 109 │ 3.1.0 │ 2 months ago │ - │ - │
│ cutandrun │ 41 │ 3.1 │ 2 months ago │ - │ - │
│ circdna │ 12 │ 1.0.2 │ 2 months ago │ - │ - │
│ ampliseq │ 111 │ 2.5.0 │ 2 months ago │ - │ - │
│ mag │ 126 │ 2.3.0 │ 2 months ago │ - │ - │
│ nascent │ 8 │ 2.1.1 │ 2 months ago │ - │ - │
│ phyloplace │ 3 │ 1.0.0 │ 2 months ago │ - │ - │
│ proteinfold │ 21 │ 1.0.0 │ 3 months ago │ - │ - │
│ crisprseq │ 8 │ 1.0 │ 3 months ago │ - │ - │
│ hic │ 48 │ 2.0.0 │ 3 months ago │ 2 weeks ago │ Yes (v2.0.0) │
│ sarek │ 235 │ 3.1.2 │ 4 months ago │ - │ - │
│ fetchngs │ 78 │ 1.9 │ 4 months ago │ - │ - │
│ methylseq │ 104 │ 2.3.0 │ 4 months ago │ - │ - │
│ atacseq │ 134 │ 2.0 │ 5 months ago │ - │ - │
│ eager │ 91 │ 2.4.6 │ 5 months ago │ 2 days ago │ Yes (v2.4.6) │
│ coproid │ 7 │ 1.1.1 │ 6 months ago │ - │ - │
│ hgtseq │ 16 │ 1.0.0 │ 6 months ago │ - │ - │
│ hlatyping │ 41 │ 2.0.0 │ 6 months ago │ - │ - │
│ chipseq │ 144 │ 2.0.0 │ 7 months ago │ - │ - │
│ rnavar │ 16 │ 1.0.0 │ 10 months ago │ - │ - │
│ mnaseseq │ 9 │ 1.0.0 │ 11 months ago │ - │ - │
│ hicar │ 3 │ 1.0.0 │ 12 months ago │ - │ - │
│ bamtofastq │ 8 │ 1.2.0 │ 1 years ago │ - │ - │
│ bacass │ 42 │ 2.0.0 │ 2 years ago │ 5 days ago │ Yes (v2.0.0) │
│ bactmap │ 41 │ 1.0.0 │ 2 years ago │ - │ - │
│ metaboigniter │ 10 │ 1.0.1 │ 2 years ago │ - │ - │
│ diaproteomics │ 10 │ 1.2.4 │ 2 years ago │ - │ - │
│ clipseq │ 13 │ 1.0.0 │ 2 years ago │ - │ - │
│ pgdb │ 3 │ 1.0.0 │ 2 years ago │ - │ - │
│ dualrnaseq │ 12 │ 1.0.0 │ 2 years ago │ - │ - │
│ cageseq │ 9 │ 1.0.2 │ 2 years ago │ - │ - │
│ proteomicslfq │ 29 │ 1.0.0 │ 3 years ago │ - │ - │
│ imcyto │ 20 │ 1.0.0 │ 3 years ago │ - │ - │
│ slamseq │ 4 │ 1.0.0 │ 3 years ago │ - │ - │
│ callingcards │ 1 │ dev │ - │ - │ - │
│ circrna │ 27 │ dev │ - │ - │ - │
│ fastquorum │ 8 │ dev │ - │ - │ - │
│ genomeannotator │ 9 │ dev │ - │ - │ - │
│ genomeassembler │ 12 │ dev │ - │ - │ - │
│ gwas │ 12 │ dev │ - │ - │ - │
│ lncpipe │ 25 │ dev │ - │ - │ - │
│ metapep │ 3 │ dev │ - │ - │ - │
│ metatdenovo │ 2 │ dev │ - │ - │ - │
│ nanostring │ 2 │ dev │ - │ - │ - │
│ pangenome │ 23 │ dev │ - │ 2 weeks ago │ No (a_brave_new_world │
│ │ │ │ │ │ - 6aa9b39) │
│ radseq │ 0 │ dev │ - │ - │ - │
│ raredisease │ 37 │ dev │ - │ - │ - │
│ rnadnavar │ 0 │ dev │ - │ - │ - │
│ rnasplice │ 3 │ dev │ - │ - │ - │
│ scflow │ 19 │ dev │ - │ - │ - │
│ spatialtranscriptom… │ 19 │ dev │ - │ - │ - │
│ spinningjenny │ 0 │ dev │ - │ - │ - │
│ variantcatalogue │ 3 │ dev │ - │ - │ - │
└──────────────────────┴───────┴────────────────┴───────────────┴─────────────┴───────────────────────┘
Link to section 'Download pipelines' of 'nf-core' Download pipelines
It is highly recommended to download pipelines to clusters before running them. Using singularity containers to run these pipelines are also recommended. Befor downloading, please set up environment variables NXF_SINGULARITY_CACHEDIR for singularity cache or NXF_CONDA_CACHEDIR for conda cache. Below is an example you can add to .bashrc for bash users.
export NXF_SINGULARITY_CACHEDIR="$SCRATCH/singularity/cache"
export NXF_CONDA_CACHEDIR="$SCRATCH/conda/cache"
Below is the example to download the rnaseq pipeline:
$ nf-core download rnaseq
,--./,-.
___ __ __ __ ___ /,-._.--~\
|\ | |__ __ / ` / \ |__) |__ } {
| \| | \__, \__/ | \ |___ \`-._,-`-,
`._,._,'
nf-core/tools version 2.8 - https://nf-co.re
? Select release / branch: (Use arrow keys)
» 3.11.2 [release]
3.11.1 [release]
3.11.0 [release]
3.10.1 [release]
3.10 [release]
3.9 [release]
3.8.1 [release]
3.8 [release]
3.7 [release]
3.6 [release]
3.5 [release]
3.4 [release]
3.3 [release]
3.2 [release]
3.1 [release]
3.0 [release]
2.0 [release]
1.4.2 [release]
1.4.1 [release]
1.4 [release]
1.3 [release]
1.2 [release]
1.1 [release]
1.0 [release]
Link to section 'Run the pipeline' of 'nf-core' Run the pipeline
If users have downloaded the rnaseq pipeline to a folder called nf-core under $HOME, users can use it to run RNAseq analysis. Here is an example for running RNAseq analysis with human samples.
#!/bin/bash
#SBATCH -A XXXX
#SBATCH --job-name=rnaseq
#SBATCH --output=slurm-%A.%a.out
#SBATCH --error=slurm-%A.%a.err
#SBATCH --nodes=1
#SBATCH --ntasks=1
#SBATCH --cpus-per-task=64
#SBATCH --time=24:00:00
#SBATCH --mail-type=all
module load nextflow
nextflow run $HOME/nf-core/nf-core-rnaseq-3.11.2/workflow/ \
--input samplesheet.csv --outdir results \
--genome GRCh37 -profile singularity
picard
Link to section 'Description' of 'picard' Description
Picard is a set of command line tools for manipulating high-throughput sequencing HTS data and formats such as SAM/BAM/CRAM and VCF.
Link to section 'Versions' of 'picard' Versions
- Anvil: 2.25.1, 2.25.7, 2.26.10, 3.1.1
- Bell: 2.25.1, 2.26.10, 3.1.1
- Negishi: 2.25.1, 2.26.10, 3.1.1
- Scholar: 2.25.1, 2.26.10
- Gautschi: 2.25.1, 2.26.10, 3.1.1
Link to section 'Commands' of 'picard' Commands
- picard
Link to section 'Module' of 'picard' Module
You can load the modules by:
module load picard
Link to section 'Example job' of 'picard' Example job
To run picard our our clusters:
#!/bin/bash
#SBATCH -A myallocation # Allocation name
#SBATCH -t 20:00:00
#SBATCH -N 1
#SBATCH -n 24
#SBATCH --job-name=picard
#SBATCH --mail-type=FAIL,BEGIN,END
#SBATCH --error=%x-%J-%u.err
#SBATCH --output=%x-%J-%u.out
module load picard
picard BuildBamIndex -Xmx64g I=19P0126636WES_sorted_md.bam
picard CreateSequenceDictionary -R hg38.fa -O hg38.dict
sratoolkit
Link to section 'Description' of 'sratoolkit' Description
The NCBI SRA Toolkit enables reading dumping of sequencing files from the SRA database and writing loading files into the .sra format.
Link to section 'Versions' of 'sratoolkit' Versions
- Anvil: 2.10.9
Link to section 'Module' of 'sratoolkit' Module
You can load the modules by:
module load sratoolkit
libv8
Link to section 'Description' of 'libv8' Description
Distributes the V8 JavaScript engine in binary and source forms in order to support fast builds of The Ruby Racer
Link to section 'Versions' of 'libv8' Versions
- Bell: 3.14
- Anvil: 6.7.17
Link to section 'Module' of 'libv8' Module
You can load the modules by:
module load libv8
quantumatk
Link to section 'Versions' of 'quantumatk' Versions
- Bell: 2020.09
- Negishi: 2020.09
Link to section 'Module' of 'quantumatk' Module
You can load the modules by:
module load quantumatk
sas
Link to section 'Description' of 'sas' Description
SAS is a commercial integrated system for statistical analysis, data mining, and graphics as well as many enterprise oriented additional features.
Link to section 'Versions' of 'sas' Versions
- Bell: 9.4
- Scholar: 9.4
- Gilbreth: 9.4
Link to section 'Module' of 'sas' Module
You can load the modules by:
module load sas
netcdf
Link to section 'Description' of 'netcdf' Description
NetCDF network Common Data Form is a set of software libraries and machine-independent data formats that support the creation, access, and sharing of array-oriented scientific data. This is the C distribution.
Link to section 'Versions' of 'netcdf' Versions
- Bell: 4.5.0, 4.7.4
- Scholar: 4.9.2
- Gilbreth: 4.5.0, 4.7.0, 4.9.2
Link to section 'Module' of 'netcdf' Module
You can load the modules by:
module load netcdf
or
module load netcdf-c
libxp
Link to section 'Description' of 'libxp' Description
libXp - X Print Client Library.
Link to section 'Versions' of 'libxp' Versions
- Negishi: 1.0.3
- Gautschi: 1.0.3
- Scholar: 1.0.3
Link to section 'Module' of 'libxp' Module
You can load the modules by:
module load libxp
parallel
Link to section 'Description' of 'parallel' Description
GNU parallel is a shell tool for executing jobs in parallel using one or more computers. A job can be a single command or a small script that has to be run for each of the lines in the input.
Link to section 'Versions' of 'parallel' Versions
- Bell: 20220522
- Negishi: 20220522
- Anvil: 20200822
- Gautschi: 20241022
Link to section 'Module' of 'parallel' Module
You can load the modules by:
module load parallel
Link to section 'Syntax' of 'parallel' Syntax
# Read commands to be run in parallel from an input file
parallel [OPTIONS] < CMDFILE
# Read command arguments on the command line
parallel [OPTIONS] COMMAND [ARGUMENTS] ::: ARGLIST
# Read command arguments from an input file
parallel [OPTIONS] COMMAND [ARGUMENTS] :::: ARGFILE
qemu
Link to section 'Description' of 'qemu' Description
QEMU is a generic and open source machine emulator and virtualizer.
Link to section 'Versions' of 'qemu' Versions
- Bell: 2.10.1, 4.1.0
- Gilbreth: 2.10.1
- Negishi: 4.2.1
- Anvil: 4.1.1, 6.2.0
Link to section 'Module' of 'qemu' Module
You can load the modules by:
module load qemu
totalview
Link to section 'Description' of 'totalview' Description
TotalView is a GUI-based source code defect analysis tool that gives you unprecedented control over processes and thread execution and visibility into program state and variables.
Link to section 'Versions' of 'totalview' Versions
- Bell: 2020.2.6, 2021.4.10
- Gilbreth: 2017.0.12, 2018.2.6, 2019.1.4, 2021.4.10
- Negishi: 2021.4.10
- Anvil: 2020.2.6
Link to section 'Module' of 'totalview' Module
You can load the modules by:
module load totalview
keras
Link to section 'Description' of 'keras' Description
Keras is a deep learning API written in Python, running on top of the machine learning platform TensorFlow. It was developed with a focus on enabling fast experimentation.
Link to section 'Versions' of 'keras' Versions
- Bell: 2.4.3
- Gilbreth: 2.2.4, 2.4.3
- Anvil: 2.6.0
Link to section 'Module' of 'keras' Module
You can load the modules by:
module load learning
module load keras
opencv
Link to section 'Description' of 'opencv' Description
OpenCV, Open source Computer Vision, is an open-source BSD-licensed library that includes several hundreds of computer vision algorithms. Written in optimized C/C++, the library can take advantage of multi-core processing.
Link to section 'Versions' of 'opencv' Versions
- Bell: 4.4.0
- Gilbreth: 3.4.3, 4.2.0, 4.5.1
- Anvil: 4.5.3
Link to section 'Module' of 'opencv' Module
You can load the modules by:
module load learning
module load opencv
libpng
Link to section 'Description' of 'libpng' Description
Libpng is the official open-source PNG reference library. It supports almost all PNG features, is extensible, and has been extensively tested for over 29 years.
Link to section 'Versions' of 'libpng' Versions
- Negishi: 1.5.30, 1.6.37
- Anvil: 1.6.37
- Gautschi: 1.6.37
- Scholar:1.6.37
Link to section 'Module' of 'libpng' Module
You can load the modules by:
module load libpng
ghostscript
Link to section 'Description' of 'ghostscript' Description
Ghostscript is an interpreter for the PostScript® language and PDF files. It is available under either the GNU GPL Affero license or licensed for commercial use from Artifex Software, Inc.
Link to section 'Versions' of 'ghostscript' Versions
- Negishi: 9.56.1
- Anvil: 9.56.1
- Gautschi: 9.56.1
Link to section 'Module' of 'ghostscript' Module
You can load the modules by:
module load libpng
cython
Link to section 'Description' of 'cython' Description
Cython is an optimising static compiler for both the Python programming language and the extended Cython programming language (based on Pyrex). It makes writing C extensions for Python as easy as Python itself.
Link to section 'Versions' of 'cython' Versions
- Gautschi: 3.0.10
Link to section 'Module' of 'cython' Module
You can load the modules by:
module load gcc
module load cython
util-linux-uuid
Link to section 'Description' of 'util-linux-uuid' Description
Util-linux is a suite of essential utilities for any Linux system.
Link to section 'Versions' of 'util-linux-uuid' Versions
- Negishi: 2.38.1
- Anvil: 2.38.1
- Gautschi: 2.38.1
- Scholar: 2.38.1
Link to section 'Module' of 'util-linux-uuid' Module
You can load the modules by:
module load util-linux-uuid
pandoc
Link to section 'Description' of 'pandoc' Description
If you need to convert files from one markup format into another, pandoc is your swiss-army knife.
Link to section 'Versions' of 'pandoc' Versions
- Gautschi: 2.19.2
Link to section 'Module' of 'pandoc' Module
You can load the modules by:
module load pandoc
r-devtools
Link to section 'Description' of 'r-devtools' Description
Tools to Make Developing R Packages Easier. Collection of package development tools.
Link to section 'Versions' of 'r-devtools' Versions
- Gautschi: 2.4.5
- Scholar: 2.4.5
Link to section 'Module' of 'r-devtools' Module
You can load the modules by:
module load r-devtools
r-formatr
Link to section 'Description' of 'r-formatr' Description
Format R Code Automatically. Provides a function tidy_source() to format R source code. Spaces and indent will be added to the code automatically, and comments will be preserved under certain conditions, so that R code will be more human-readable and tidy. There is also a Shiny app as a user interface in this package.
Link to section 'Versions' of 'r-formatr' Versions
- Gautschi: 1.14
- Scholar: 1.14
Link to section 'Module' of 'r-formatr' Module
You can load the modules by:
module load r-formatr
r-irkernel
Link to section 'Description' of 'r-irkernel' Description
Native R Kernel for the 'Jupyter Notebook'. The R kernel for the 'Jupyter' environment executes R code which the front-end ('Jupyter Notebook' or other front-ends) submits to the kernel via the network.
Link to section 'Versions' of 'r-irkernel' Versions
- Gautschi: 1.3.2
- Scholar: 1.3.2
Link to section 'Module' of 'r-irkernel' Module
You can load the modules by:
module load r-irkernel
r-plyr
Link to section 'Description' of 'r-plyr' Description
Tools for Splitting, Applying and Combining Data. A set of tools that solves a common set of problems: you need to break a big problem down into manageable pieces, operate on each piece and then put all the pieces back together. For example, you might want to fit a model to each spatial location or time point in your study, summarise data by panels or collapse high-dimensional arrays to simpler summary statistics. The development of 'plyr' has been generously supported by 'Becton Dickinson'.
Link to section 'Versions' of 'r-plyr' Versions
- Gautschi: 1.8.8
- Scholar: 1.8.8
Link to section 'Module' of 'r-plyr' Module
You can load the modules by:
module load r-plyr
r-reshape2
Link to section 'Description' of 'r-reshape2' Description
Flexibly Reshape Data: A Reboot of the Reshape Package. Flexibly restructure and aggregate data using just two functions: melt and dcast (or acast).
Link to section 'Versions' of 'r-reshape2' Versions
- Gautschi: 1.4.4
- Scholar: 1.4.4
Link to section 'Module' of 'r-reshape2' Module
You can load the modules by:
module load r-reshape2
r-shiny
Link to section 'Description' of 'r-shiny' Description
Web Application Framework for R. Makes it incredibly easy to build interactive web applications with R. Automatic "reactive" binding between inputs and outputs and extensive pre-built widgets make it possible to build beautiful, responsive, and powerful applications with minimal effort.
Link to section 'Versions' of 'r-shiny' Versions
- Gautschi: 1.8.1.1
Link to section 'Module' of 'r-shiny' Module
You can load the modules by:
module load r-shiny
duckdb
Link to section 'Description' of 'duckdb' Description
DuckDB is an in-process SQL OLAP database management system. Simple, feature-rich, fast & open source.
Link to section 'Versions' of 'duckdb' Versions
- Gautschi: 1.1.3
- Scholar: 1.0.0
Link to section 'Module' of 'duckdb' Module
You can load the modules by:
module load duckdb
intel-rt
Link to section 'Description' of 'intel-rt' Description
Intel® oneAPI Compiler redistributable libraries.
Link to section 'Versions' of 'intel-rt' Versions
- Gautschi: 2024.1.0
Link to section 'Module' of 'intel-rt' Module
You can load the modules by:
module load intel-rt
oclfpga
Link to section 'Description' of 'oclfpga' Description
Enables FPGA development using the Intel® oneAPI SYCL Compiler.
Link to section 'Versions' of 'oclfpga' Versions
- Gautschi: 2024.1.0
Link to section 'Module' of 'oclfpga' Module
You can load the modules by:
module load oclfpga
Utilities
Commonly used utilities.
archivemount
Link to section 'Availability' of 'archivemount' Availability
- Scholar
- Gilbreth
Link to section 'Module' of 'archivemount' Module
You can load the modules by:
module load utilities
module load archivemount
git
Link to section 'Availability' of 'git' Availability
- Scholar
- Gilbreth
Link to section 'Module' of 'git' Module
You can load the modules by:
module load utilities
module load git
grace
Link to section 'Availability' of 'grace' Availability
Link to section 'Module' of 'grace' Module
You can load the modules by:
module load utilities
module load grace
monitor
Link to section 'Description' of 'monitor' Description
system resource monitoring tool.
Link to section 'Availability' of 'monitor' Availability
- Bell
- Scholar
- Gilbreth
Link to section 'Module' of 'monitor' Module
You can load the modules by:
module load utilities
module load monitor
parafly
Link to section 'Availability' of 'parafly' Availability
- Bell
- Scholar
- Gilbreth
Link to section 'Module' of 'parafly' Module
You can load the modules by:
module load utilities
module load parafly
subversion
Link to section 'Availability' of 'subversion' Availability
- Scholar
- Gilbreth
Link to section 'Module' of 'subversion' Module
You can load the modules by:
module load utilities
module load subversion
vim
Link to section 'Availability' of 'vim' Availability
- Scholar
- Gilbreth
Link to section 'Module' of 'vim' Module
You can load the modules by:
module load utilities
module load vim
visit
Link to section 'Availability' of 'visit' Availability
Link to section 'Module' of 'visit' Module
You can load the modules by:
module load utilities
module load visit
vlc
Link to section 'Availability' of 'vlc' Availability
VLC is a free and open source multimedia player for most multimedia formats.
Link to section 'Versions' of 'vlc' Versions
- Bell: 3.0.9.2
- Gilbreth: 3.0.9.2
Link to section 'Module' of 'vlc' Module
You can load the modules by:
module load utilities
module load vlc
Biocontainers
Link to section 'What is BioContainers' of 'Biocontainers' What is BioContainers
The BioContainers project came from the idea of using the containers-based technologies such as Docker or rkt for bioinformatics software. Having a common and controllable environment for running software could help to deal with some of the current problems during software development and distribution. BioContainers is a community-driven project that provides the infrastructure and basic guidelines to create, manage and distribute bioinformatics containers with a special focus on omics fields such as proteomics, genomics, transcriptomics and metabolomics. . For more information, please visit BioContainers project.
Link to section 'Deployed Applications' of 'Biocontainers' Deployed Applications
- abacas
- abismal
- abpoa
- abricate
- abyss
- actc
- adapterremoval
- advntr
- afplot
- afterqc
- agat
- agfusion
- alfred
- alien-hunter
- alignstats
- allpathslg
- alphafold
- amptk
- ananse
- anchorwave
- angsd
- annogesic
- annovar
- antismash
- anvio
- any2fasta
- arcs
- asgal
- aspera-connect
- assembly-stats
- atac-seq-pipeline
- ataqv
- atram
- atropos
- augur
- augustus
- bactopia
- bali-phy
- bamgineer
- bamliquidator
- bam-readcount
- bamsurgeon
- bamtools
- bamutil
- barrnap
- basenji
- bayescan
- bazam
- bbmap
- bbtools
- bcftools
- bcl2fastq
- beagle
- beast2
- bedops
- bedtools
- bioawk
- biobambam
- bioconvert
- biopython
- bismark
- blasr
- blast
- blobtools
- bmge
- bowtie
- bowtie2
- bracken
- braker2
- brass
- breseq
- busco
- bustools
- bwa
- bwameth
- cactus
- cafe
- canu
- ccs
- cdbtools
- cd-hit
- cegma
- cellbender
- cellphonedb
- cellranger
- cellranger-arc
- cellranger-atac
- cellranger-dna
- cellrank
- cellrank-krylov
- cellsnp-lite
- celltypist
- centrifuge
- cfsan-snp-pipeline
- checkm-genome
- chewbbaca
- chopper
- chromap
- cicero
- circexplorer2
- circlator
- circompara2
- circos
- ciri2
- ciriquant
- clair3
- clairvoyante
- clearcnv
- clever-toolkit
- clonalframeml
- clust
- clustalw
- cnvkit
- cnvnator
- coinfinder
- concoct
- control-freec
- cooler
- coverm
- cramino
- crisprcasfinder
- crispresso2
- crispritz
- crossmap
- cross_match
- csvtk
- cufflinks
- cutadapt
- cuttlefish
- cyvcf2
- das_tool
- dbg2olc
- deconseq
- deepbgc
- deepconsensus
- deepsignal2
- deeptools
- deepvariant
- delly
- dendropy
- diamond
- dnaio
- dragonflye
- drep
- dropest
- drop-seq
- dsuite
- easysfs
- edta
- eggnog-mapper
- emboss
- ensembl-vep
- epic2
- evidencemodeler
- exonerate
- expansionhunter
- fasta3
- fastani
- fastp
- fastqc
- fastq_pair
- fastq-scan
- fastspar
- faststructure
- fasttree
- fastx_toolkit
- filtlong
- flye
- fraggenescan
- fraggenescanrs
- freebayes
- freyja
- fseq
- funannotate
- fwdpy11
- gadma
- gambit
- gamma
- gangstr
- gapfiller
- gatk
- gatk4
- gemma
- gemoma
- genemark
- genemarks-2
- genmap
- genomedata
- genomepy
- genomescope2
- genomicconsensus
- genrich
- getorganelle
- gfaffix
- gfastats
- gfatools
- gffcompare
- gffread
- gffutils
- gimmemotifs
- glimmer
- glimmerhmm
- glnexus
- gmap
- goatools
- graphlan
- graphmap
- gridss
- gseapy
- gtdbtk
- gubbins
- guppy
- hail
- hap.py
- helen
- hicexplorer
- hic-pro
- hifiasm
- hisat2
- hmmer
- homer
- how_are_we_stranded_here
- htseq
- htslib
- htstream
- humann
- hyphy
- idba
- igv
- impute2
- infernal
- instrain
- intarna
- interproscan
- iqtree
- isoquant
- isoseq3
- ivar
- jcvi
- kaiju
- kakscalculator2
- kallisto
- khmer
- kissde
- kissplice
- kissplice2refgenome
- kma
- kmc
- kmergenie
- kmer-jellyfish
- kneaddata
- kover
- kraken2
- krakentools
- lambda
- last
- lastz
- ldhat
- ldjump
- ldsc
- liftoff
- liftofftools
- lima
- links
- lofreq
- longphase
- longqc
- lra
- ltr_finder
- ltrpred
- lumpy-sv
- lyveset
- macrel
- macs2
- macs3
- mafft
- mageck
- magicblast
- maker
- manta
- mapcaller
- marginpolish
- mash
- mashmap
- mashtree
- masurca
- mauve
- maxbin2
- maxquant
- mcl
- mcscanx
- medaka
- megadepth
- megahit
- megan
- meme
- memes
- meraculous
- merqury
- meryl
- metabat
- metachip
- metaphlan
- metaseq
- methyldackel
- metilene
- mhm2
- microbedmm
- minialign
- miniasm
- minimap2
- minipolish
- miniprot
- mirdeep2
- mirtop
- mitofinder
- mlst
- mmseqs2
- mob_suite
- modbam2bed
- modeltest-ng
- momi
- mothur
- motus
- mrbayes
- multiqc
- mummer4
- muscle
- mutmap
- mykrobe
- n50
- nanofilt
- nanolyse
- nanoplot
- nanopolish
- ncbi-amrfinderplus
- ncbi-datasets
- ncbi-genome-download
- ncbi-table2asn
- neusomatic
- nextalign
- nextclade
- nextflow
- ngs-bits
- ngsld
- ngsutils
- orthofinder
- paml
- panacota
- panaroo
- pandaseq
- pandora
- pangolin
- panphlan
- parallel-fastq-dump
- parliament2
- parsnp
- pasta
- pbmm2
- pbptyper
- pcangsd
- peakranger
- pepper_deepvariant
- perl-bioperl
- phast
- phd2fasta
- phg
- phipack
- phrap
- phred
- phylosuite
- picard
- picrust2
- pilon
- pindel
- pirate
- piscem
- pixy
- plasmidfinder
- platon
- platypus
- plink
- plink2
- plotsr
- pomoxis
- poppunk
- popscle
- pplacer
- prinseq
- prodigal
- prokka
- proteinortho
- prothint
- pullseq
- purge_dups
- pvactools
- pyani
- pybedtools
- pybigwig
- pychopper
- pycoqc
- pyensembl
- pyfaidx
- pygenometracks
- pygenomeviz
- pyranges
- pysam
- pyvcf3
- qiime2
- qtlseq
- qualimap
- quast
- quickmirseq
- r
- racon
- ragout
- ragtag
- rapmap
- rasusa
- raven-assembler
- raxml
- raxml-ng
- reapr
- rebaler
- reciprocal_smallest_distance
- recycler
- regtools
- repeatmasker
- repeatmodeler
- repeatscout
- resfinder
- revbayes
- rmats
- rmats2sashimiplot
- rnaindel
- rnapeg
- rnaquast
- roary
- r-rnaseq
- r-rstudio
- r-scrnaseq
- rsem
- rseqc
- run_dbcan
- rush
- sage
- salmon
- sambamba
- samblaster
- samclip
- samplot
- samtools
- scanpy
- scarches
- scgen
- scirpy
- scvelo
- scvi-tools
- segalign
- seidr
- sepp
- seqcode
- seqkit
- seqyclean
- shapeit4
- shapeit5
- shasta
- shigeifinder
- shorah
- shortstack
- shovill
- sicer
- sicer2
- signalp4
- signalp6
- simug
- singlem
- ska
- skewer
- slamdunk
- smoove
- snakemake
- snap
- snap-aligner
- snaptools
- snippy
- snp-dists
- snpeff
- snpgenie
- snphylo
- snpsift
- snp-sites
- soapdenovo2
- sortmerna
- souporcell
- sourmash
- spaceranger
- spades
- sprod
- squeezemeta
- squid
- sra-tools
- srst2
- stacks
- star
- staramr
- starfusion
- stream
- stringdecomposer
- stringtie
- strique
- structure
- subread
- survivor
- svaba
- svtools
- svtyper
- swat
- syri
- talon
- targetp
- tassel
- taxonkit
- t-coffee
- tetranscripts
- tiara
- tigmint
- tobias
- tombo
- tophat
- tpmcalculator
- transabyss
- transdecoder
- transrate
- transvar
- trax
- treetime
- trimal
- trim-galore
- trimmomatic
- trinity
- trinotate
- trnascan-se
- trtools
- trust4
- trycycler
- ucsc_genome_toolkit
- unicycler
- usefulaf
- vadr
- vardict-java
- varlociraptor
- varscan
- vartrix
- vatools
- vcf2maf
- vcf2phylip
- vcf2tsvpy
- vcf-kit
- vcftools
- velocyto.py
- velvet
- veryfasttree
- vg
- viennarna
- vsearch
- weblogo
- whatshap
- wiggletools
- winnowmap
- wtdbg
abacas
Link to section 'Introduction' of 'abacas' Introduction
Abacas
is a tool for algorithm based automatic contiguation of assembled sequences.
For more information, please check its website: https://biocontainers.pro/tools/abacas and its home page: http://abacas.sourceforge.net.
Link to section 'Versions' of 'abacas' Versions
- 1.3.1
Link to section 'Commands' of 'abacas' Commands
- abacas.pl
- abacas.1.3.1.pl
Link to section 'Module' of 'abacas' Module
You can load the modules by:
module load biocontainers
module load abacas
Link to section 'Example job' of 'abacas' Example job
Using #!/bin/sh -l
as shebang in the slurm job script will cause the failure of some biocontainer modules. Please use #!/bin/bash
instead.
To run Abacas on our clusters:
#!/bin/bash
#SBATCH -A myallocation # Allocation name
#SBATCH -t 1:00:00
#SBATCH -N 1
#SBATCH -n 1
#SBATCH --job-name=abacas
#SBATCH --mail-type=FAIL,BEGIN,END
#SBATCH --error=%x-%J-%u.err
#SBATCH --output=%x-%J-%u.out
module --force purge
ml biocontainers abacas
abacas.pl -r cmm.fasta -q Cm.contigs.fasta -p nucmer -o out_prefix
abismal
Link to section 'Introduction' of 'abismal' Introduction
Another Bisulfite Mapping Algorithm (abismal) is a read mapping program for bisulfite sequencing in DNA methylation studies.
BioContainers: https://biocontainers.pro/tools/abismal
Home page: https://github.com/smithlabcode/abismal
Link to section 'Versions' of 'abismal' Versions
- 3.0.0
Link to section 'Commands' of 'abismal' Commands
- abismal
- abismalidx
- simreads
Link to section 'Module' of 'abismal' Module
You can load the modules by:
module load biocontainers
module load abismal
Link to section 'Example job' of 'abismal' Example job
Using #!/bin/sh -l
as shebang in the slurm job script will cause the failure of some biocontainer modules. Please use #!/bin/bash
instead.
To run abismal on our clusters:
#!/bin/bash
#SBATCH -A myallocation # Allocation name
#SBATCH -t 1:00:00
#SBATCH -N 1
#SBATCH -n 1
#SBATCH --job-name=abismal
#SBATCH --mail-type=FAIL,BEGIN,END
#SBATCH --error=%x-%J-%u.err
#SBATCH --output=%x-%J-%u.out
module --force purge
ml biocontainers abismal
abismalidx ~/.local/share/genomes/hg38/hg38.fa hg38
abpoa
Link to section 'Introduction' of 'abpoa' Introduction
abPOA: adaptive banded Partial Order Alignment
Home page: https://github.com/yangao07/abPOA
Link to section 'Versions' of 'abpoa' Versions
- 1.4.1
Link to section 'Commands' of 'abpoa' Commands
- abpoa
Link to section 'Module' of 'abpoa' Module
You can load the modules by:
module load biocontainers
module load abpoa
Link to section 'Example job' of 'abpoa' Example job
Using #!/bin/sh -l
as shebang in the slurm job script will cause the failure of some biocontainer modules. Please use #!/bin/bash
instead.
To run abpoa on our clusters:
#!/bin/bash
#SBATCH -A myallocation # Allocation name
#SBATCH -t 1:00:00
#SBATCH -N 1
#SBATCH -n 1
#SBATCH --job-name=abpoa
#SBATCH --mail-type=FAIL,BEGIN,END
#SBATCH --error=%x-%J-%u.err
#SBATCH --output=%x-%J-%u.out
module --force purge
ml biocontainers abpoa
abpoa seq.fa > cons.fa
abricate
Link to section 'Introduction' of 'abricate' Introduction
Abricate
is a tool for mass screening of contigs for antimicrobial resistance or virulence genes.
For more information, please check its website: https://biocontainers.pro/tools/abricate and its home page on Github.
Link to section 'Versions' of 'abricate' Versions
- 1.0.1
Link to section 'Commands' of 'abricate' Commands
- abricate
Link to section 'Module' of 'abricate' Module
You can load the modules by:
module load biocontainers
module load abricate
Link to section 'Example job' of 'abricate' Example job
Using #!/bin/sh -l
as shebang in the slurm job script will cause the failure of some biocontainer modules. Please use #!/bin/bash
instead.
To run Abricate on our clusters:
#!/bin/bash
#SBATCH -A myallocation # Allocation name
#SBATCH -t 1:00:00
#SBATCH -N 1
#SBATCH -n 8
#SBATCH --job-name=abricate
#SBATCH --mail-type=FAIL,BEGIN,END
#SBATCH --error=%x-%J-%u.err
#SBATCH --output=%x-%J-%u.out
module --force purge
ml biocontainers abricate
abricate --threads 8 *.fasta
abyss
Link to section 'Introduction' of 'abyss' Introduction
ABySS
is a de novo sequence assembler intended for short paired-end reads and genomes of all sizes.
For more information, please check its website: https://biocontainers.pro/tools/abyss and its home page on Github.
Link to section 'Versions' of 'abyss' Versions
- 2.3.2
- 2.3.4
- 2.3.8
Link to section 'Commands' of 'abyss' Commands
- ABYSS
- ABYSS-P
- AdjList
- Consensus
- DAssembler
- DistanceEst
- DistanceEst-ssq
- KAligner
- MergeContigs
- MergePaths
- Overlap
- ParseAligns
- PathConsensus
- PathOverlap
- PopBubbles
- SimpleGraph
- abyss-align
- abyss-bloom
- abyss-bloom-dbg
- abyss-bowtie
- abyss-bowtie2
- abyss-bwa
- abyss-bwamem
- abyss-bwasw
- abyss-db-txt
- abyss-dida
- abyss-fac
- abyss-fatoagp
- abyss-filtergraph
- abyss-fixmate
- abyss-fixmate-ssq
- abyss-gapfill
- abyss-gc
- abyss-index
- abyss-junction
- abyss-kaligner
- abyss-layout
- abyss-longseqdist
- abyss-map
- abyss-map-ssq
- abyss-mergepairs
- abyss-overlap
- abyss-paired-dbg
- abyss-paired-dbg-mpi
- abyss-pe
- abyss-rresolver-short
- abyss-samtoafg
- abyss-scaffold
- abyss-sealer
- abyss-stack-size
- abyss-tabtomd
- abyss-todot
- abyss-tofastq
- konnector
- logcounter
Link to section 'Module' of 'abyss' Module
You can load the modules by:
module load biocontainers
module load abyss
Link to section 'Example job' of 'abyss' Example job
Using #!/bin/sh -l
as shebang in the slurm job script will cause the failure of some biocontainer modules. Please use #!/bin/bash
instead.
To run abyss on our our clusters:
#!/bin/bash
#SBATCH -A myallocation # Allocation name
#SBATCH -t 1:00:00
#SBATCH -N 1
#SBATCH -n 4
#SBATCH --job-name=abyss
#SBATCH --mail-type=FAIL,BEGIN,END
#SBATCH --error=%x-%J-%u.err
#SBATCH --output=%x-%J-%u.out
module --force purge
ml biocontainers abyss
abyss-pe np=4 k=25 name=test B=1G \
in='test-data/reads1.fastq test-data/reads2.fastq'
actc
Link to section 'Introduction' of 'actc' Introduction
Actc is used to align subreads to ccs reads.
Home page: https://github.com/PacificBiosciences/actc
Link to section 'Versions' of 'actc' Versions
- 0.2.0
Link to section 'Commands' of 'actc' Commands
- actc
Link to section 'Module' of 'actc' Module
You can load the modules by:
module load biocontainers
module load actc
Link to section 'Example job' of 'actc' Example job
Using #!/bin/sh -l
as shebang in the slurm job script will cause the failure of some biocontainer modules. Please use #!/bin/bash
instead.
To run actc on our clusters:
#!/bin/bash
#SBATCH -A myallocation # Allocation name
#SBATCH -t 1:00:00
#SBATCH -N 1
#SBATCH -n 1
#SBATCH --job-name=actc
#SBATCH --mail-type=FAIL,BEGIN,END
#SBATCH --error=%x-%J-%u.err
#SBATCH --output=%x-%J-%u.out
module --force purge
ml biocontainers actc
actc subreads.bam ccs.bam subreads_to_ccs.bam
adapterremoval
Link to section 'Introduction' of 'adapterremoval' Introduction
AdapterRemoval searches for and removes adapter sequences from High-Throughput Sequencing (HTS) data and (optionally) trims low quality bases from the 3' end of reads following adapter removal. AdapterRemoval can analyze both single end and paired end data, and can be used to merge overlapping paired-ended reads into (longer) consensus sequences. Additionally, AdapterRemoval can construct a consensus adapter sequence for paired-ended reads, if which this information is not available.
BioContainers: https://biocontainers.pro/tools/adapterremoval
Home page: https://github.com/MikkelSchubert/adapterremoval
Link to section 'Versions' of 'adapterremoval' Versions
- 2.3.3
Link to section 'Commands' of 'adapterremoval' Commands
- AdapterRemoval
Link to section 'Module' of 'adapterremoval' Module
You can load the modules by:
module load biocontainers
module load adapterremoval
Link to section 'Example job' of 'adapterremoval' Example job
Using #!/bin/sh -l
as shebang in the slurm job script will cause the failure of some biocontainer modules. Please use #!/bin/bash
instead.
To run adapterremoval on our clusters:
#!/bin/bash
#SBATCH -A myallocation # Allocation name
#SBATCH -t 1:00:00
#SBATCH -N 1
#SBATCH -n 1
#SBATCH --job-name=adapterremoval
#SBATCH --mail-type=FAIL,BEGIN,END
#SBATCH --error=%x-%J-%u.err
#SBATCH --output=%x-%J-%u.out
module --force purge
ml biocontainers adapterremoval
AdapterRemoval --file1 input_1.fastq --file2 input_2.fastq
advntr
Link to section 'Introduction' of 'advntr' Introduction
Advntr
is a tool for genotyping Variable Number Tandem Repeats (VNTR) from sequence data.
For more information, please check its website: https://biocontainers.pro/tools/advntr and its home page on Github.
Link to section 'Versions' of 'advntr' Versions
- 1.4.0
- 1.5.0
Link to section 'Commands' of 'advntr' Commands
- advntr
Link to section 'Module' of 'advntr' Module
You can load the modules by:
module load biocontainers
module load advntr
Link to section 'Example job' of 'advntr' Example job
Using #!/bin/sh -l
as shebang in the slurm job script will cause the failure of some biocontainer modules. Please use #!/bin/bash
instead.
To run Advntr on our clusters:
#!/bin/bash
#SBATCH -A myallocation # Allocation name
#SBATCH -t 1:00:00
#SBATCH -N 1
#SBATCH -n 1
#SBATCH --job-name=advntr
#SBATCH --mail-type=FAIL,BEGIN,END
#SBATCH --error=%x-%J-%u.err
#SBATCH --output=%x-%J-%u.out
module --force purge
ml biocontainers advntr
advntr addmodel -r chr21.fa -p CGCGGGGCGGGG -s 45196324 -e 45196360 -c chr21
advntr genotype --vntr_id 1 --alignment_file CSTB_2_5_testdata.bam --working_directory working_dir
afplot
Link to section 'Introduction' of 'afplot' Introduction
Afplot
is a tool to plot allele frequencies in VCF files.
For more information, please check its website: https://biocontainers.pro/tools/afplot and its home page on Github.
Link to section 'Versions' of 'afplot' Versions
- 0.2.1
Link to section 'Commands' of 'afplot' Commands
- afplot
Link to section 'Module' of 'afplot' Module
You can load the modules by:
module load biocontainers
module load afplot
Link to section 'Example job' of 'afplot' Example job
Using #!/bin/sh -l
as shebang in the slurm job script will cause the failure of some biocontainer modules. Please use #!/bin/bash
instead.
To run afplot on our our clusters:
#!/bin/bash
#SBATCH -A myallocation # Allocation name
#SBATCH -t 1:00:00
#SBATCH -N 1
#SBATCH -n 1
#SBATCH --job-name=afplot
#SBATCH --mail-type=FAIL,BEGIN,END
#SBATCH --error=%x-%J-%u.err
#SBATCH --output=%x-%J-%u.out
module --force purge
ml biocontainers afplot
afplot whole-genome histogram -v my_vcf.gz -l my_label -s my_sample -o mysample.histogram.png
afterqc
Link to section 'Introduction' of 'afterqc' Introduction
Afterqc
is a tool for quality control of FASTQ data produced by HiSeq 2000/2500/3000/4000, Nextseq 500/550, MiniSeq, and Illumina 1.8 or newer.
For more information, please check its website: https://biocontainers.pro/tools/afterqc and its home page on Github.
Link to section 'Versions' of 'afterqc' Versions
- 0.9.7
Link to section 'Commands' of 'afterqc' Commands
- after.py
Link to section 'Module' of 'afterqc' Module
You can load the modules by:
module load biocontainers
module load afterqc
Link to section 'Example job' of 'afterqc' Example job
Using #!/bin/sh -l
as shebang in the slurm job script will cause the failure of some biocontainer modules. Please use #!/bin/bash
instead.
To run blobtools on our our clusters:
#!/bin/bash
#SBATCH -A myallocation # Allocation name
#SBATCH -t 1:00:00
#SBATCH -N 1
#SBATCH -n 1
#SBATCH --job-name=afterqc
#SBATCH --mail-type=FAIL,BEGIN,END
#SBATCH --error=%x-%J-%u.err
#SBATCH --output=%x-%J-%u.out
module --force purge
ml biocontainers afterqc
after.py -1 SRR11941281_1.fastq.paired.fq -2 SRR11941281_2.fastq.paired.fq
agat
Link to section 'Introduction' of 'agat' Introduction
Agat
is a suite of tools to handle gene annotations in any GTF/GFF format.
For more information, please check its website: https://biocontainers.pro/tools/agat and its home page on Github.
Link to section 'Versions' of 'agat' Versions
- 0.8.1
Link to section 'Commands' of 'agat' Commands
- agat_convert_bed2gff.pl
- agat_convert_embl2gff.pl
- agat_convert_genscan2gff.pl
- agat_convert_mfannot2gff.pl
- agat_convert_minimap2_bam2gff.pl
- agat_convert_sp_gff2bed.pl
- agat_convert_sp_gff2gtf.pl
- agat_convert_sp_gff2tsv.pl
- agat_convert_sp_gff2zff.pl
- agat_convert_sp_gxf2gxf.pl
- agat_sp_Prokka_inferNameFromAttributes.pl
- agat_sp_add_introns.pl
- agat_sp_add_start_and_stop.pl
- agat_sp_alignment_output_style.pl
- agat_sp_clipN_seqExtremities_and_fixCoordinates.pl
- agat_sp_compare_two_BUSCOs.pl
- agat_sp_compare_two_annotations.pl
- agat_sp_complement_annotations.pl
- agat_sp_ensembl_output_style.pl
- agat_sp_extract_attributes.pl
- agat_sp_extract_sequences.pl
- agat_sp_filter_by_ORF_size.pl
- agat_sp_filter_by_locus_distance.pl
- agat_sp_filter_by_mrnaBlastValue.pl
- agat_sp_filter_feature_by_attribute_presence.pl
- agat_sp_filter_feature_by_attribute_value.pl
- agat_sp_filter_feature_from_keep_list.pl
- agat_sp_filter_feature_from_kill_list.pl
- agat_sp_filter_gene_by_intron_numbers.pl
- agat_sp_filter_gene_by_length.pl
- agat_sp_filter_incomplete_gene_coding_models.pl
- agat_sp_filter_record_by_coordinates.pl
- agat_sp_fix_cds_phases.pl
- agat_sp_fix_features_locations_duplicated.pl
- agat_sp_fix_fusion.pl
- agat_sp_fix_longest_ORF.pl
- agat_sp_fix_overlaping_genes.pl
- agat_sp_fix_small_exon_from_extremities.pl
- agat_sp_flag_premature_stop_codons.pl
- agat_sp_flag_short_introns.pl
- agat_sp_functional_statistics.pl
- agat_sp_keep_longest_isoform.pl
- agat_sp_kraken_assess_liftover.pl
- agat_sp_list_short_introns.pl
- agat_sp_load_function_from_protein_align.pl
- agat_sp_manage_IDs.pl
- agat_sp_manage_UTRs.pl
- agat_sp_manage_attributes.pl
- agat_sp_manage_functional_annotation.pl
- agat_sp_manage_introns.pl
- agat_sp_merge_annotations.pl
- agat_sp_prokka_fix_fragmented_gene_annotations.pl
- agat_sp_sensitivity_specificity.pl
- agat_sp_separate_by_record_type.pl
- agat_sp_statistics.pl
- agat_sp_webApollo_compliant.pl
- agat_sq_add_attributes_from_tsv.pl
- agat_sq_add_hash_tag.pl
- agat_sq_add_locus_tag.pl
- agat_sq_count_attributes.pl
- agat_sq_filter_feature_from_fasta.pl
- agat_sq_list_attributes.pl
- agat_sq_manage_IDs.pl
- agat_sq_manage_attributes.pl
- agat_sq_mask.pl
- agat_sq_remove_redundant_entries.pl
- agat_sq_repeats_analyzer.pl
- agat_sq_rfam_analyzer.pl
- agat_sq_split.pl
- agat_sq_stat_basic.pl
Link to section 'Module' of 'agat' Module
You can load the modules by:
module load biocontainers
module load agat
Link to section 'Example job' of 'agat' Example job
Using #!/bin/sh -l
as shebang in the slurm job script will cause the failure of some biocontainer modules. Please use #!/bin/bash
instead.
To run Agat on our our clusters:
#!/bin/bash
#SBATCH -A myallocation # Allocation name
#SBATCH -t 1:00:00
#SBATCH -N 1
#SBATCH -n 1
#SBATCH --job-name=agat
#SBATCH --mail-type=FAIL,BEGIN,END
#SBATCH --error=%x-%J-%u.err
#SBATCH --output=%x-%J-%u.out
module --force purge
ml biocontainers agat
agat_convert_sp_gff2bed.pl --gff genes.gff -o genes.bed
agfusion
Link to section 'Introduction' of 'agfusion' Introduction
AGFusion (pronounced 'A G Fusion') is a python package for annotating gene fusions from the human or mouse genomes.
Docker hub: https://hub.docker.com/r/mgibio/agfusion
Home page: https://github.com/murphycj/AGFusion
Link to section 'Versions' of 'agfusion' Versions
- 1.3.11
Link to section 'Commands' of 'agfusion' Commands
- agfusion
Link to section 'Module' of 'agfusion' Module
You can load the modules by:
module load biocontainers
module load agfusion
Link to section 'Example job' of 'agfusion' Example job
Using #!/bin/sh -l
as shebang in the slurm job script will cause the failure of some biocontainer modules. Please use #!/bin/bash
instead.
To run agfusion on our clusters:
#!/bin/bash
#SBATCH -A myallocation # Allocation name
#SBATCH -t 1:00:00
#SBATCH -N 1
#SBATCH -n 1
#SBATCH --job-name=agfusion
#SBATCH --mail-type=FAIL,BEGIN,END
#SBATCH --error=%x-%J-%u.err
#SBATCH --output=%x-%J-%u.out
module --force purge
ml biocontainers agfusion
alfred
Link to section 'Introduction' of 'alfred' Introduction
Alfred
is an efficient and versatile command-line application that computes multi-sample quality control metrics in a read-group aware manner.
For more information, please check its website: https://biocontainers.pro/tools/alfred and its home page on Github.
Link to section 'Versions' of 'alfred' Versions
- 0.2.5
- 0.2.6
Link to section 'Commands' of 'alfred' Commands
- alfred
Link to section 'Module' of 'alfred' Module
You can load the modules by:
module load biocontainers
module load alfred
Link to section 'Example job' of 'alfred' Example job
Using #!/bin/sh -l
as shebang in the slurm job script will cause the failure of some biocontainer modules. Please use #!/bin/bash
instead.
To run Alfred on our clusters:
#!/bin/bash
#SBATCH -A myallocation # Allocation name
#SBATCH -t 1:00:00
#SBATCH -N 1
#SBATCH -n 1
#SBATCH --job-name=alfred
#SBATCH --mail-type=FAIL,BEGIN,END
#SBATCH --error=%x-%J-%u.err
#SBATCH --output=%x-%J-%u.out
module --force purge
ml biocontainers alfred
alfred qc -r genome.fasta -o qc.tsv.gz sorted.bam
alien-hunter
Link to section 'Introduction' of 'alien-hunter' Introduction
Alien-hunter
is an application for the prediction of putative Horizontal Gene Transfer (HGT) events with the implementation of Interpolated Variable Order Motifs (IVOMs).
For more information, please check its website: https://biocontainers.pro/tools/alien-hunter.
Link to section 'Versions' of 'alien-hunter' Versions
- 1.7.7
Link to section 'Commands' of 'alien-hunter' Commands
- alien_hunter
Link to section 'Module' of 'alien-hunter' Module
You can load the modules by:
module load biocontainers
module load alien_hunter
Link to section 'Example job' of 'alien-hunter' Example job
Using #!/bin/sh -l
as shebang in the slurm job script will cause the failure of some biocontainer modules. Please use #!/bin/bash
instead.
To run Alien_hunter on our our clusters:
#!/bin/bash
#SBATCH -A myallocation # Allocation name
#SBATCH -t 1:00:00
#SBATCH -N 1
#SBATCH -n 1
#SBATCH --job-name=alien_hunter
#SBATCH --mail-type=FAIL,BEGIN,END
#SBATCH --error=%x-%J-%u.err
#SBATCH --output=%x-%J-%u.out
module --force purge
ml biocontainers alien_hunter
alien_hunter genome.fasta output
alignstats
Link to section 'Introduction' of 'alignstats' Introduction
AlignStats produces various alignment, whole genome coverage, and capture coverage metrics for sequence alignment files in SAM, BAM, and CRAM format.
BioContainers: https://biocontainers.pro/tools/alignstats
Home page: https://github.com/jfarek/alignstats
Link to section 'Versions' of 'alignstats' Versions
- 0.9.1
Link to section 'Commands' of 'alignstats' Commands
- alignstats
Link to section 'Module' of 'alignstats' Module
You can load the modules by:
module load biocontainers
module load alignstats
Link to section 'Example job' of 'alignstats' Example job
Using #!/bin/sh -l
as shebang in the slurm job script will cause the failure of some biocontainer modules. Please use #!/bin/bash
instead.
To run alignstats on our clusters:
#!/bin/bash
#SBATCH -A myallocation # Allocation name
#SBATCH -t 1:00:00
#SBATCH -N 1
#SBATCH -n 1
#SBATCH --job-name=alignstats
#SBATCH --mail-type=FAIL,BEGIN,END
#SBATCH --error=%x-%J-%u.err
#SBATCH --output=%x-%J-%u.out
module --force purge
ml biocontainers alignstats
alignstats -C -i input.bam -o report.txt
allpathslg
Link to section 'Introduction' of 'allpathslg' Introduction
Allpathslg
is a whole-genome shotgun assembler that can generate high-quality genome assemblies using short reads.
For more information, please check its website: https://biocontainers.pro/tools/allpathslg and its home page: https://bioinformaticshome.com/tools/wga/descriptions/Allpaths-LG.html.
Link to section 'Versions' of 'allpathslg' Versions
- 52488
Link to section 'Commands' of 'allpathslg' Commands
- PrepareAllPathsInputs.pl
- RunAllPathsLG
- CacheLibs.pl
- Fasta2Fastb
Link to section 'Module' of 'allpathslg' Module
You can load the modules by:
module load biocontainers
module load allpathslg
Link to section 'Example job' of 'allpathslg' Example job
Using #!/bin/sh -l
as shebang in the slurm job script will cause the failure of some biocontainer modules. Please use #!/bin/bash
instead.
To run Allpathslg on our our clusters:
#!/bin/bash
#SBATCH -A myallocation # Allocation name
#SBATCH -t 1:00:00
#SBATCH -N 1
#SBATCH -n 24
#SBATCH --job-name=allpathslg
#SBATCH --mail-type=FAIL,BEGIN,END
#SBATCH --error=%x-%J-%u.err
#SBATCH --output=%x-%J-%u.out
module --force purge
ml biocontainers allpathslg
PrepareAllPathsInputs.pl \
DATA_DIR=data \
PLOIDY=1 \
IN_GROUPS_CSV=in_groups.csv\
IN_LIBS_CSV=in_libs.csv\
OVERWRITE=True\
RunAllPathsLG PRE=allpathlg REFERENCE_NAME=test.genome \
DATA_SUBDIR=data RUN=myrun TARGETS=standard \
SUBDIR=test OVERWRITE=True
alphafold
Link to section 'Introduction' of 'alphafold' Introduction
Alphafold
is a protein structure prediction tool developed by DeepMind (Google). It uses a novel machine learning approach to predict 3D protein structures from primary sequences alone. The source code is available on Github. It has been deployed in all RCAC clusters, supporting both CPU and GPU.
It also relies on a huge database. The full database ( 2.2TB) has been downloaded and setup for users.
Protein struction prediction by alphafold is performed in the following steps:
- Search the amino acid sequence in uniref90 database by jackhmmer (using CPU)
- Search the amino acid sequence in mgnify database by jackhmmer (using CPU)
- Search the amino acid sequence in pdb70 database (for monomers) or pdb_seqres database (for multimers) by hhsearch (using CPU)
- Search the amino acid sequence in bfd database and uniclust30 (updated to uniref30 since v2.3.0) database by hhblits (using CPU)
- Search structure templates in pdb_mmcif database (using CPU)
- Search the amino acid sequence in uniprot database (for multimers) by jackhmmer (using CPU)
- Predict 3D structure by machine learning (using CPU or GPU)
- Structure optimisation with OpenMM (using CPU or GPU)
Link to section 'Versions' of 'alphafold' Versions
- 2.1.1
- 2.2.0
- 2.2.3
- 2.3.0
- 2.3.1
- 2.3.2
Link to section 'Commands' of 'alphafold' Commands
run_alphafold.sh
Link to section 'Module' of 'alphafold' Module
You can load the modules by:
module load biocontainers
module load alphafold
Link to section 'Usage' of 'alphafold' Usage
The usage of Alphafold on our cluster is very straightford, users can create a flagfile containing the database path information:
run_alphafold.sh --flagfile=full_db.ff --fasta_paths=XX --output_dir=XX ...
Users can check its detailed user guide in its Github.
Link to section 'full_db.ff' of 'alphafold' full_db.ff
Example contents of full_db.ff:
--db_preset=full_dbs
--bfd_database_path=/depot/itap/datasets/alphafold/db/bfd/bfd_metaclust_clu_complete_id30_c90_final_seq.sorted_opt
--data_dir=/depot/itap/datasets/alphafold/db/
--uniref90_database_path=/depot/itap/datasets/alphafold/db/uniref90/uniref90.fasta
--mgnify_database_path=/depot/itap/datasets/alphafold/db/mgnify/mgy_clusters_2018_12.fa
--uniclust30_database_path=/depot/itap/datasets/alphafold/db/uniclust30/uniclust30_2018_08/uniclust30_2018_08
--pdb70_database_path=/depot/itap/datasets/alphafold/db/pdb70/pdb70
--template_mmcif_dir=/depot/itap/datasets/alphafold/db/pdb_mmcif/mmcif_files
--max_template_date=2022-01-29
--obsolete_pdbs_path=/depot/itap/datasets/alphafold/db/pdb_mmcif/obsolete.dat
--hhblits_binary_path=/usr/bin/hhblits
--hhsearch_binary_path=/usr/bin/hhsearch
--jackhmmer_binary_path=/usr/bin/jackhmmer
--kalign_binary_path=/usr/bin/kalign
Since Version v2.2.0, the AlphaFold-Multimer model parameters has been updated. The updated full database is stored in depot/itap/datasets/alphafold/db_20221014
. For ACCESS Anvil, the database is stored in /anvil/datasets/alphafold/db_20221014
. Users need to update the flagfile using the updated database:
run_alphafold.sh --flagfile=full_db_20221014.ff --fasta_paths=XX --output_dir=XX ...
Link to section 'full_db_20221014.ff (for alphafold v2)' of 'alphafold' full_db_20221014.ff (for alphafold v2)
Example contents of full_db_20221014.ff (For ACCESS Anvil, please change depot/itap
to anvil
):
--db_preset=full_dbs
--bfd_database_path=/depot/itap/datasets/alphafold/db_20221014/bfd/bfd_metaclust_clu_complete_id30_c90_final_seq.sorted_opt
--data_dir=/depot/itap/datasets/alphafold/db_20221014/
--uniref90_database_path=/depot/itap/datasets/alphafold/db_20221014/uniref90/uniref90.fasta
--mgnify_database_path=/depot/itap/datasets/alphafold/db_20221014/mgnify/mgy_clusters_2018_12.fa
--uniclust30_database_path=/depot/itap/datasets/alphafold/db_20221014/uniclust30/uniclust30_2018_08/uniclust30_2018_08
--pdb_seqres_database_path=/depot/itap/datasets/alphafold/db_20221014/pdb_seqres/pdb_seqres.txt
--uniprot_database_path=/depot/itap/datasets/alphafold/db_20221014/uniprot/uniprot.fasta
--template_mmcif_dir=/depot/itap/datasets/alphafold/db_20221014/pdb_mmcif/mmcif_files
--obsolete_pdbs_path=/depot/itap/datasets/alphafold/db_20221014/pdb_mmcif/obsolete.dat
--hhblits_binary_path=/usr/bin/hhblits
--hhsearch_binary_path=/usr/bin/hhsearch
--jackhmmer_binary_path=/usr/bin/jackhmmer
--kalign_binary_path=/usr/bin/kalign
Since Version v2.3.0, the AlphaFold-Multimer model parameters has been updated. The updated full database is stored in depot/itap/datasets/alphafold/db_20230311
. For ACCESS Anvil, the database is stored in /anvil/datasets/alphafold/db_20230311
. Users need to update the flagfile using the updated database:
run_alphafold.sh --flagfile=full_db_20230311.ff --fasta_paths=XX --output_dir=XX ...
Since Version v2.3.0, uniclust30_database_path
has been changed to uniref30_database_path
.
Link to section 'full_db_20230311.ff (for alphafold v3)' of 'alphafold' full_db_20230311.ff (for alphafold v3)
Example contents of full_db_20230311.ff for monomer (For ACCESS Anvil, please change depot/itap
to anvil
):
--db_preset=full_dbs
--bfd_database_path=/depot/itap/datasets/alphafold/db_20230311/bfd/bfd_metaclust_clu_complete_id30_c90_final_seq.sorted_opt
--data_dir=/depot/itap/datasets/alphafold/db_20230311/
--uniref90_database_path=/depot/itap/datasets/alphafold/db_20230311/uniref90/uniref90.fasta
--mgnify_database_path=/depot/itap/datasets/alphafold/db_20230311/mgnify/mgy_clusters_2022_05.fa
--uniref30_database_path=/depot/itap/datasets/alphafold/db_20230311/uniref30/UniRef30_2021_03
--pdb70_database_path=/depot/itap/datasets/alphafold/db_20230311/pdb70/pdb70
--template_mmcif_dir=/depot/itap/datasets/alphafold/db_20230311/pdb_mmcif/mmcif_files
--obsolete_pdbs_path=/depot/itap/datasets/alphafold/db_20230311/pdb_mmcif/obsolete.dat
--hhblits_binary_path=/usr/bin/hhblits
--hhsearch_binary_path=/usr/bin/hhsearch
--jackhmmer_binary_path=/usr/bin/jackhmmer
--kalign_binary_path=/usr/bin/kalign
Example contents of full_db_20230311.ff for multimer (For ACCESS Anvil, please change depot/itap
to anvil
):
--db_preset=full_dbs
--bfd_database_path=/depot/itap/datasets/alphafold/db_20230311/bfd/bfd_metaclust_clu_complete_id30_c90_final_seq.sorted_opt
--data_dir=/depot/itap/datasets/alphafold/db_20230311/
--uniref90_database_path=/depot/itap/datasets/alphafold/db_20230311/uniref90/uniref90.fasta
--mgnify_database_path=/depot/itap/datasets/alphafold/db_20230311/mgnify/mgy_clusters_2022_05.fa
--uniref30_database_path=/depot/itap/datasets/alphafold/db_20230311/uniref30/UniRef30_2021_03
--pdb_seqres_database_path=/depot/itap/datasets/alphafold/db_20230311/pdb_seqres/pdb_seqres.txt
--uniprot_database_path=/depot/itap/datasets/alphafold/db_20230311/uniprot/uniprot.fasta
--template_mmcif_dir=/depot/itap/datasets/alphafold/db_20230311/pdb_mmcif/mmcif_files
--obsolete_pdbs_path=/depot/itap/datasets/alphafold/db_20230311/pdb_mmcif/obsolete.dat
--hhblits_binary_path=/usr/bin/hhblits
--hhsearch_binary_path=/usr/bin/hhsearch
--jackhmmer_binary_path=/usr/bin/jackhmmer
--kalign_binary_path=/usr/bin/kalign
Link to section 'Example job using CPU' of 'alphafold' Example job using CPU
Using #!/bin/sh -l
as shebang in the slurm job script will cause the failure of some biocontainer modules. Please use #!/bin/bash
instead.
Notice that since version 2.2.0, the parameter --use_gpu_relax=False
is required.
To run alphafold using CPU:
#!/bin/bash
#SBATCH -A myallocation # Allocation name
#SBATCH -t 20:00:00
#SBATCH -N 1
#SBATCH -n 24
#SBATCH --job-name=alphafold
#SBATCH --mail-type=FAIL,BEGIN,END
#SBATCH --error=%x-%J-%u.err
#SBATCH --output=%x-%J-%u.out
module --force purge
ml biocontainers alphafold/2.3.1
run_alphafold.sh --flagfile=full_db_20230311.ff \
--fasta_paths=sample.fasta --max_template_date=2022-02-01 \
--output_dir=af2_full_out --model_preset=monomer \
--use_gpu_relax=False
Link to section 'Example job using GPU' of 'alphafold' Example job using GPU
Using #!/bin/sh -l
as shebang in the slurm job script will cause the failure of some biocontainer modules. Please use #!/bin/bash
instead.
Notice that since version 2.2.0, the parameter --use_gpu_relax=True
is required.
To run alphafold using GPU:
#!/bin/bash
#SBATCH -A myallocation # Allocation name
#SBATCH -t 20:00:00
#SBATCH -N 1
#SBATCH -n 11
#SBATCH --gres=gpu:1
#SBATCH --job-name=alphafold
#SBATCH --mail-type=FAIL,BEGIN,END
#SBATCH --error=%x-%J-%u.err
#SBATCH --output=%x-%J-%u.out
module --force purge
ml biocontainers alphafold/2.3.1
run_alphafold.sh --flagfile=full_db_20230311.ff \
--fasta_paths=sample.fasta --max_template_date=2022-02-01 \
--output_dir=af2_full_out --model_preset=monomer \
--use_gpu_relax=True
amptk
Link to section 'Introduction' of 'amptk' Introduction
Amptk
is a series of scripts to process NGS amplicon data using USEARCH and VSEARCH, it can also be used to process any NGS amplicon data and includes databases setup for analysis of fungal ITS, fungal LSU, bacterial 16S, and insect COI amplicons.
For more information, please check its website: https://biocontainers.pro/tools/amptk and its home page on Github.
Link to section 'Versions' of 'amptk' Versions
- 1.5.4
Link to section 'Commands' of 'amptk' Commands
- amptk
Link to section 'Module' of 'amptk' Module
You can load the modules by:
module load biocontainers
module load amptk
Link to section 'Example job' of 'amptk' Example job
Using #!/bin/sh -l
as shebang in the slurm job script will cause the failure of some biocontainer modules. Please use #!/bin/bash
instead.
To run Amptk on our our clusters:
#!/bin/bash
#SBATCH -A myallocation # Allocation name
#SBATCH -t 1:00:00
#SBATCH -N 1
#SBATCH -n 4
#SBATCH --job-name=amptk
#SBATCH --mail-type=FAIL,BEGIN,END
#SBATCH --error=%x-%J-%u.err
#SBATCH --output=%x-%J-%u.out
module --force purge
ml biocontainers amptk
amptk illumina -i test_data/illumina_test_data -o miseq -f fITS7 -r ITS4 --cpus 4
ananse
Link to section 'Introduction' of 'ananse' Introduction
ANANSE is a computational approach to infer enhancer-based gene regulatory networks (GRNs) and to identify key transcription factors between two GRNs.
BioContainers: https://biocontainers.pro/tools/ananse
Home page: https://github.com/vanheeringen-lab/ANANSE
Link to section 'Versions' of 'ananse' Versions
- 0.4.0
Link to section 'Commands' of 'ananse' Commands
- ananse
Link to section 'Module' of 'ananse' Module
You can load the modules by:
module load biocontainers
module load ananse
Link to section 'Example job' of 'ananse' Example job
Using #!/bin/sh -l
as shebang in the slurm job script will cause the failure of some biocontainer modules. Please use #!/bin/bash
instead.
To run ananse on our clusters:
#!/bin/bash
#SBATCH -A myallocation # Allocation name
#SBATCH -t 1:00:00
#SBATCH -N 1
#SBATCH -n 1
#SBATCH --job-name=ananse
#SBATCH --mail-type=FAIL,BEGIN,END
#SBATCH --error=%x-%J-%u.err
#SBATCH --output=%x-%J-%u.out
module --force purge
ml biocontainers ananse
mkdir -p ANANSE.REMAP.model.v1.0
wget https://zenodo.org/record/4768075/files/ANANSE.REMAP.model.v1.0.tgz
tar xvzf ANANSE.REMAP.model.v1.0.tgz -C ANANSE.REMAP.model.v1.0
rm ANANSE.REMAP.model.v1.0.tgz
wget https://zenodo.org/record/4769814/files/ANANSE_example_data.tgz
tar xvzf ANANSE_example_data.tgz
rm ANANSE_example_data.tgz
ananse binding -H ANANSE_example_data/H3K27ac/fibroblast*bam -A ANANSE_example_data/ATAC/fibroblast*bam -R ANANSE.REMAP.model.v1.0/ -o fibroblast.binding
ananse binding -H ANANSE_example_data/H3K27ac/heart*bam -A ANANSE_example_data/ATAC/heart*bam -R ANANSE.REMAP.model.v1.0/ -o heart.binding
ananse network -b fibroblast.binding/binding.h5 -e ANANSE_example_data/RNAseq/fibroblast*TPM.txt -n 4 -o fibroblast.network.txt
ananse network -b heart.binding/binding.h5 -e ANANSE_example_data/RNAseq/heart*TPM.txt -n 4 -o heart.network.txt
ananse influence -s fibroblast.network.txt -t heart.network.txt -d ANANSE_example_data/RNAseq/fibroblast2heart_degenes.csv -p -o fibroblast2heart.influence.txt
anchorwave
Link to section 'Introduction' of 'anchorwave' Introduction
Anchorwave
is used for sensitive alignment of genomes with high sequence diversity, extensive structural polymorphism and whole-genome duplication variation.
For more information, please check its website: https://biocontainers.pro/tools/anchorwave and its home page on Github.
Link to section 'Versions' of 'anchorwave' Versions
- 1.0.1
- 1.1.1
Link to section 'Commands' of 'anchorwave' Commands
- anchorwave
- gmap_build
- gmap
- minimap2
Link to section 'Module' of 'anchorwave' Module
You can load the modules by:
module load biocontainers
module load anchorwave
Link to section 'Example job' of 'anchorwave' Example job
Using #!/bin/sh -l
as shebang in the slurm job script will cause the failure of some biocontainer modules. Please use #!/bin/bash
instead.
To run Anchorwave on our our clusters:
#!/bin/bash
#SBATCH -A myallocation # Allocation name
#SBATCH -t 1:00:00
#SBATCH -N 1
#SBATCH -n 4
#SBATCH --job-name=anchorwave
#SBATCH --mail-type=FAIL,BEGIN,END
#SBATCH --error=%x-%J-%u.err
#SBATCH --output=%x-%J-%u.out
module --force purge
ml biocontainers anchorwave
anchorwave gff2seq -i Zea_mays.AGPv4.34.gff3 -r Zea_mays.AGPv4.dna.toplevel.fa -o cds.fa
angsd
ANGSD
is a software for analyzing next generation sequencing data. Detailed usage can be found here: http://www.popgen.dk/angsd/index.php/ANGSD.
Link to section 'Versions' of 'angsd' Versions
- 0.935
- 0.937
- 0.939
- 0.940
Link to section 'Commands' of 'angsd' Commands
- angsd
- realSFS
- msToGlf
- thetaStat
- supersim
Link to section 'Module' of 'angsd' Module
You can load the modules by:
module load biocontainers
module load angsd/0.937
Link to section 'Example job' of 'angsd' Example job
Using #!/bin/sh -l
as shebang in the slurm job script will cause the failure of some biocontainer modules. Please use #!/bin/bash
instead.
To run angsd on our our clusters:
#!/bin/bash
#SBATCH -A myallocation # Allocation name
#SBATCH -t 20:00:00
#SBATCH -N 1
#SBATCH -n 24
#SBATCH --job-name=angsd
#SBATCH --mail-type=FAIL,BEGIN,END
#SBATCH --error=%x-%J-%u.err
#SBATCH --output=%x-%J-%u.out
module --force purge
ml biocontainers angsd/0.937
angsd -b bam.filelist -GL 1 -doMajorMinor 1 -doMaf 2 -P 5 -minMapQ 30 -minQ 20 -minMaf 0.05
annogesic
Link to section 'Introduction' of 'annogesic' Introduction
ANNOgesic is the swiss army knife for RNA-Seq based annotation of bacterial/archaeal genomes.
Docker hub: https://hub.docker.com/r/silasysh/annogesic
Home page: https://github.com/Sung-Huan/ANNOgesic
Link to section 'Versions' of 'annogesic' Versions
- 1.1.0
Link to section 'Commands' of 'annogesic' Commands
- annogesic
Link to section 'Module' of 'annogesic' Module
You can load the modules by:
module load biocontainers
module load annogesic
Link to section 'Example job' of 'annogesic' Example job
Using #!/bin/sh -l
as shebang in the slurm job script will cause the failure of some biocontainer modules. Please use #!/bin/bash
instead.
To run annogesic on our clusters:
#!/bin/bash
#SBATCH -A myallocation # Allocation name
#SBATCH -t 1:00:00
#SBATCH -N 1
#SBATCH -n 1
#SBATCH --job-name=annogesic
#SBATCH --mail-type=FAIL,BEGIN,END
#SBATCH --error=%x-%J-%u.err
#SBATCH --output=%x-%J-%u.out
module --force purge
ml biocontainers annogesic
ANNOGESIC_FOLDER=ANNOgesic
annogesic \
update_genome_fasta \
-c $ANNOGESIC_FOLDER/input/references/fasta_files/NC_009839.1.fa \
-m $ANNOGESIC_FOLDER/input/mutation_tables/mutation.csv \
-u NC_test.1 \
-pj $ANNOGESIC_FOLDER
annovar
Link to section 'Introduction' of 'annovar' Introduction
ANNOVAR
is an efficient software tool to utilize update-to-date information to functionally annotate genetic variants detected from diverse genomes (including human genome hg18, hg19, hg38, as well as mouse, worm, fly, yeast and many others).
For more information, please check its website: https://annovar.openbioinformatics.org/en/latest/.
Link to section 'Versions' of 'annovar' Versions
- 2022-01-13
Link to section 'Commands' of 'annovar' Commands
- annotate_variation.pl
- coding_change.pl
- convert2annovar.pl
- retrieve_seq_from_fasta.pl
- table_annovar.pl
- variants_reduction.pl
Link to section 'Module' of 'annovar' Module
You can load the modules by:
module load biocontainers
module load annovar
Link to section 'Example job' of 'annovar' Example job
Using #!/bin/sh -l
as shebang in the slurm job script will cause the failure of some biocontainer modules. Please use #!/bin/bash
instead.
To run ANNOVAR on our our clusters:
#!/bin/bash
#SBATCH -A myallocation # Allocation name
#SBATCH -t 1:00:00
#SBATCH -N 1
#SBATCH -n 4
#SBATCH --job-name=annovar
#SBATCH --mail-type=FAIL,BEGIN,END
#SBATCH --error=%x-%J-%u.err
#SBATCH --output=%x-%J-%u.out
module --force purge
ml biocontainers annovar
annotate_variation.pl --buildver hg19 --downdb seq humandb/hg19_seq
convert2annovar.pl -format region -seqdir humandb/hg19_seq/ chr1:2000001-2000003
antismash
Link to section 'Introduction' of 'antismash' Introduction
Antismash
Antismash allows the rapid genome-wide identification, annotation and analysis of secondary metabolite biosynthesis gene clusters in bacterial and fungal genomes.
For more information, please check its website: https://biocontainers.pro/tools/antismash and its home page: https://docs.antismash.secondarymetabolites.org.
Link to section 'Versions' of 'antismash' Versions
- 5.1.2
- 6.0.1
- 6.1.0
Link to section 'Commands' of 'antismash' Commands
- antismash
Link to section 'Module' of 'antismash' Module
You can load the modules by:
module load biocontainers
module load antismash
Link to section 'Example job' of 'antismash' Example job
Using #!/bin/sh -l
as shebang in the slurm job script will cause the failure of some biocontainer modules. Please use #!/bin/bash
instead.
To run Antismash on our our clusters:
#!/bin/bash
#SBATCH -A myallocation # Allocation name
#SBATCH -t 1:00:00
#SBATCH -N 1
#SBATCH -n 4
#SBATCH --job-name=antismash
#SBATCH --mail-type=FAIL,BEGIN,END
#SBATCH --error=%x-%J-%u.err
#SBATCH --output=%x-%J-%u.out
module --force purge
ml biocontainers antismash
antismash --cb-general --cb-knownclusters --cb-subclusters --asf --pfam2go --smcog-trees seq.gbk
anvio
Link to section 'Introduction' of 'anvio' Introduction
Anvio
is an analysis and visualization platform for 'omics data.
For more information, please check its website: https://biocontainers.pro/tools/anvio and its home page on Github.
Link to section 'Versions' of 'anvio' Versions
- 7.0
- 7.1_main
- 7.1_structure
Link to section 'Commands' of 'anvio' Commands
- anvi-analyze-synteny
- anvi-cluster-contigs
- anvi-compute-ani
- anvi-compute-completeness
- anvi-compute-functional-enrichment
- anvi-compute-gene-cluster-homogeneity
- anvi-compute-genome-similarity
- anvi-convert-trnaseq-database
- anvi-db-info
- anvi-delete-collection
- anvi-delete-hmms
- anvi-delete-misc-data
- anvi-delete-state
- anvi-dereplicate-genomes
- anvi-display-contigs-stats
- anvi-display-metabolism
- anvi-display-pan
- anvi-display-structure
- anvi-estimate-genome-completeness
- anvi-estimate-genome-taxonomy
- anvi-estimate-metabolism
- anvi-estimate-scg-taxonomy
- anvi-estimate-trna-taxonomy
- anvi-experimental-organization
- anvi-export-collection
- anvi-export-contigs
- anvi-export-functions
- anvi-export-gene-calls
- anvi-export-gene-coverage-and-detection
- anvi-export-items-order
- anvi-export-locus
- anvi-export-misc-data
- anvi-export-splits-and-coverages
- anvi-export-splits-taxonomy
- anvi-export-state
- anvi-export-structures
- anvi-export-table
- anvi-gen-contigs-database
- anvi-gen-fixation-index-matrix
- anvi-gen-gene-consensus-sequences
- anvi-gen-gene-level-stats-databases
- anvi-gen-genomes-storage
- anvi-gen-network
- anvi-gen-phylogenomic-tree
- anvi-gen-structure-database
- anvi-gen-variability-matrix
- anvi-gen-variability-network
- anvi-gen-variability-profile
- anvi-get-aa-counts
- anvi-get-codon-frequencies
- anvi-get-enriched-functions-per-pan-group
- anvi-get-sequences-for-gene-calls
- anvi-get-sequences-for-gene-clusters
- anvi-get-sequences-for-hmm-hits
- anvi-get-short-reads-from-bam
- anvi-get-short-reads-mapping-to-a-gene
- anvi-get-split-coverages
- anvi-help
- anvi-import-collection
- anvi-import-functions
- anvi-import-items-order
- anvi-import-misc-data
- anvi-import-state
- anvi-import-taxonomy-for-genes
- anvi-import-taxonomy-for-layers
- anvi-init-bam
- anvi-inspect
- anvi-interactive
- anvi-matrix-to-newick
- anvi-mcg-classifier
- anvi-merge
- anvi-merge-bins
- anvi-meta-pan-genome
- anvi-migrate
- anvi-oligotype-linkmers
- anvi-pan-genome
- anvi-profile
- anvi-push
- anvi-refine
- anvi-rename-bins
- anvi-report-linkmers
- anvi-run-hmms
- anvi-run-interacdome
- anvi-run-kegg-kofams
- anvi-run-ncbi-cogs
- anvi-run-pfams
- anvi-run-scg-taxonomy
- anvi-run-trna-taxonomy
- anvi-run-workflow
- anvi-scan-trnas
- anvi-script-add-default-collection
- anvi-script-augustus-output-to-external-gene-calls
- anvi-script-calculate-pn-ps-ratio
- anvi-script-checkm-tree-to-interactive
- anvi-script-compute-ani-for-fasta
- anvi-script-enrichment-stats
- anvi-script-estimate-genome-size
- anvi-script-filter-fasta-by-blast
- anvi-script-fix-homopolymer-indels
- anvi-script-gen-CPR-classifier
- anvi-script-gen-distribution-of-genes-in-a-bin
- anvi-script-gen-help-pages
- anvi-script-gen-hmm-hits-matrix-across-genomes
- anvi-script-gen-programs-network
- anvi-script-gen-programs-vignette
- anvi-script-gen-pseudo-paired-reads-from-fastq
- anvi-script-gen-scg-domain-classifier
- anvi-script-gen-short-reads
- anvi-script-gen_stats_for_single_copy_genes.R
- anvi-script-gen_stats_for_single_copy_genes.py
- anvi-script-gen_stats_for_single_copy_genes.sh
- anvi-script-get-collection-info
- anvi-script-get-coverage-from-bam
- anvi-script-get-hmm-hits-per-gene-call
- anvi-script-get-primer-matches
- anvi-script-merge-collections
- anvi-script-pfam-accessions-to-hmms-directory
- anvi-script-predict-CPR-genomes
- anvi-script-process-genbank
- anvi-script-process-genbank-metadata
- anvi-script-reformat-fasta
- anvi-script-run-eggnog-mapper
- anvi-script-snvs-to-interactive
- anvi-script-tabulate
- anvi-script-transpose-matrix
- anvi-script-variability-to-vcf
- anvi-script-visualize-split-coverages
- anvi-search-functions
- anvi-self-test
- anvi-setup-interacdome
- anvi-setup-kegg-kofams
- anvi-setup-ncbi-cogs
- anvi-setup-pdb-database
- anvi-setup-pfams
- anvi-setup-scg-taxonomy
- anvi-setup-trna-taxonomy
- anvi-show-collections-and-bins
- anvi-show-misc-data
- anvi-split
- anvi-summarize
- anvi-trnaseq
- anvi-update-db-description
- anvi-update-structure-database
- anvi-upgrade
Link to section 'Module' of 'anvio' Module
You can load the modules by:
module load biocontainers
module load anvio
Link to section 'Example job' of 'anvio' Example job
Using #!/bin/sh -l
as shebang in the slurm job script will cause the failure of some biocontainer modules. Please use #!/bin/bash
instead.
To run Anvio on our our clusters:
#!/bin/bash
#SBATCH -A myallocation # Allocation name
#SBATCH -t 1:00:00
#SBATCH -N 1
#SBATCH -n 8
#SBATCH --job-name=anvio
#SBATCH --mail-type=FAIL,BEGIN,END
#SBATCH --error=%x-%J-%u.err
#SBATCH --output=%x-%J-%u.out
module --force purge
ml biocontainers anvio
anvi-script-reformat-fasta assembly.fa -o contigs.fa -l 1000 --simplify-names --seq-type NT
anvi-gen-contigs-database -f contigs.fa -o contigs.db -n 'An example contigs database' --num-threads 8
anvi-display-contigs-stats contigs.db
anvi-setup-ncbi-cogs --cog-data-dir $PWD --num-threads 8 --just-do-it --reset
anvi-run-ncbi-cogs -c contigs.db --cog-data-dir COG20 --num-threads 8
any2fasta
Link to section 'Introduction' of 'any2fasta' Introduction
Any2fasta can convert various sequence formats to FASTA.
BioContainers: https://biocontainers.pro/tools/any2fasta
Home page: https://github.com/tseemann/any2fasta
Link to section 'Versions' of 'any2fasta' Versions
- 0.4.2
Link to section 'Commands' of 'any2fasta' Commands
- any2fasta
Link to section 'Module' of 'any2fasta' Module
You can load the modules by:
module load biocontainers
module load any2fasta
Link to section 'Example job' of 'any2fasta' Example job
Using #!/bin/sh -l
as shebang in the slurm job script will cause the failure of some biocontainer modules. Please use #!/bin/bash
instead.
To run any2fasta on our clusters:
#!/bin/bash
#SBATCH -A myallocation # Allocation name
#SBATCH -t 1:00:00
#SBATCH -N 1
#SBATCH -n 1
#SBATCH --job-name=any2fasta
#SBATCH --mail-type=FAIL,BEGIN,END
#SBATCH --error=%x-%J-%u.err
#SBATCH --output=%x-%J-%u.out
module --force purge
ml biocontainers any2fasta
any2fasta input.gff > out.fasta
arcs
Link to section 'Introduction' of 'arcs' Introduction
ARCS is a tool for scaffolding genome sequence assemblies using linked or long read sequencing data.
Home page: https://github.com/bcgsc/arcs
Link to section 'Versions' of 'arcs' Versions
- 1.2.4
Link to section 'Commands' of 'arcs' Commands
- arcs
- arcs-make
Link to section 'Module' of 'arcs' Module
You can load the modules by:
module load biocontainers
module load arcs
Link to section 'Example job' of 'arcs' Example job
Using #!/bin/sh -l
as shebang in the slurm job script will cause the failure of some biocontainer modules. Please use #!/bin/bash
instead.
To run arcs on our clusters:
#!/bin/bash
#SBATCH -A myallocation # Allocation name
#SBATCH -t 1:00:00
#SBATCH -N 1
#SBATCH -n 1
#SBATCH --job-name=arcs
#SBATCH --mail-type=FAIL,BEGIN,END
#SBATCH --error=%x-%J-%u.err
#SBATCH --output=%x-%J-%u.out
module --force purge
ml biocontainers arcs
asgal
Link to section 'Introduction' of 'asgal' Introduction
ASGAL
(Alternative Splicing Graph ALigner) is a tool for detecting the alternative splicing events expressed in a RNA-Seq sample with respect to a gene annotation.
Docker hub: https://hub.docker.com/r/algolab/asgal and its home page on Github.
Link to section 'Versions' of 'asgal' Versions
- 1.1.7
Link to section 'Commands' of 'asgal' Commands
- asgal
Link to section 'Module' of 'asgal' Module
You can load the modules by:
module load biocontainers
module load asgal
Link to section 'Example job' of 'asgal' Example job
Using #!/bin/sh -l
as shebang in the slurm job script will cause the failure of some biocontainer modules. Please use #!/bin/bash
instead.
To run ASGAL on our clusters:
#!/bin/bash
#SBATCH -A myallocation # Allocation name
#SBATCH -t 1:00:00
#SBATCH -N 1
#SBATCH -n 1
#SBATCH --job-name=asgal
#SBATCH --mail-type=FAIL,BEGIN,END
#SBATCH --error=%x-%J-%u.err
#SBATCH --output=%x-%J-%u.out
module --force purge
ml biocontainers asgal
asgal -g input/genome.fa \
-a input/annotation.gtf \
-s input/sample_1.fa -o outputFolder
assembly-stats
Link to section 'Introduction' of 'assembly-stats' Introduction
Assembly-stats
is a tool to get assembly statistics from FASTA and FASTQ files.
For more information, please check its website: https://biocontainers.pro/tools/assembly-stats and its home page on Github.
Link to section 'Versions' of 'assembly-stats' Versions
- 1.0.1
Link to section 'Commands' of 'assembly-stats' Commands
- assembly-stats
Link to section 'Module' of 'assembly-stats' Module
You can load the modules by:
module load biocontainers
module load assembly-stats
Link to section 'Example job' of 'assembly-stats' Example job
Using #!/bin/sh -l
as shebang in the slurm job script will cause the failure of some biocontainer modules. Please use #!/bin/bash
instead.
To run Assembly-stats on our our clusters:
#!/bin/bash
#SBATCH -A myallocation # Allocation name
#SBATCH -t 00:10:00
#SBATCH -N 1
#SBATCH -n 1
#SBATCH --job-name=assembly-stats
#SBATCH --mail-type=FAIL,BEGIN,END
#SBATCH --error=%x-%J-%u.err
#SBATCH --output=%x-%J-%u.out
module --force purge
ml biocontainers assembly-stats
assembly-stats seq.fasta
atac-seq-pipeline
Link to section 'Introduction' of 'atac-seq-pipeline' Introduction
The ENCODE ATAC-seq pipeline is used for quality control and statistical signal processing of short-read sequencing data, producing alignments and measures of enrichment. It was developed by Anshul Kundaje's lab at Stanford University.
Docker hub: https://hub.docker.com/r/encodedcc/atac-seq-pipeline
Home page: https://www.encodeproject.org/atac-seq/
Link to section 'Versions' of 'atac-seq-pipeline' Versions
- 2.1.3
Link to section 'Commands' of 'atac-seq-pipeline' Commands
- 10x_bam2fastq
- SAMstats
- SAMstatsParallel
- ace2sam
- aggregate_scores_in_intervals.py
- align_print_template.py
- alignmentSieve
- annotate.py
- annotateBed
- axt_extract_ranges.py
- axt_to_fasta.py
- axt_to_lav.py
- axt_to_maf.py
- bamCompare
- bamCoverage
- bamPEFragmentSize
- bamToBed
- bamToFastq
- bed12ToBed6
- bedToBam
- bedToIgv
- bed_bigwig_profile.py
- bed_build_windows.py
- bed_complement.py
- bed_count_by_interval.py
- bed_count_overlapping.py
- bed_coverage.py
- bed_coverage_by_interval.py
- bed_diff_basewise_summary.py
- bed_extend_to.py
- bed_intersect.py
- bed_intersect_basewise.py
- bed_merge_overlapping.py
- bed_rand_intersect.py
- bed_subtract_basewise.py
- bedpeToBam
- bedtools
- bigwigCompare
- blast2sam.pl
- bnMapper.py
- bowtie2sam.pl
- bwa
- chardetect
- closestBed
- clusterBed
- complementBed
- compress
- computeGCBias
- computeMatrix
- computeMatrixOperations
- correctGCBias
- coverageBed
- createDiff
- cutadapt
- cygdb
- cython
- cythonize
- deeptools
- div_snp_table_chr.py
- download_metaseq_example_data.py
- estimateReadFiltering
- estimateScaleFactor
- expandCols
- export2sam.pl
- faidx
- fastaFromBed
- find_in_sorted_file.py
- flankBed
- gene_fourfold_sites.py
- genomeCoverageBed
- getOverlap
- getSeq_genome_wN
- getSeq_genome_woN
- get_objgraph
- get_scores_in_intervals.py
- gffutils-cli
- groupBy
- gsl-config
- gsl-histogram
- gsl-randist
- idr
- int_seqs_to_char_strings.py
- interpolate_sam.pl
- intersectBed
- intersection_matrix.py
- interval_count_intersections.py
- interval_join.py
- intron_exon_reads.py
- jsondiff
- lav_to_axt.py
- lav_to_maf.py
- line_select.py
- linksBed
- lzop_build_offset_table.py
- mMK_bitset.py
- macs2
- maf_build_index.py
- maf_chop.py
- maf_chunk.py
- maf_col_counts.py
- maf_col_counts_all.py
- maf_count.py
- maf_covered_ranges.py
- maf_covered_regions.py
- maf_div_sites.py
- maf_drop_overlapping.py
- maf_extract_chrom_ranges.py
- maf_extract_ranges.py
- maf_extract_ranges_indexed.py
- maf_filter.py
- maf_filter_max_wc.py
- maf_gap_frequency.py
- maf_gc_content.py
- maf_interval_alignibility.py
- maf_limit_to_species.py
- maf_mapping_word_frequency.py
- maf_mask_cpg.py
- maf_mean_length_ungapped_piece.py
- maf_percent_columns_matching.py
- maf_percent_identity.py
- maf_print_chroms.py
- maf_print_scores.py
- maf_randomize.py
- maf_region_coverage_by_src.py
- maf_select.py
- maf_shuffle_columns.py
- maf_species_in_all_files.py
- maf_split_by_src.py
- maf_thread_for_species.py
- maf_tile.py
- maf_tile_2.py
- maf_tile_2bit.py
- maf_to_axt.py
- maf_to_concat_fasta.py
- maf_to_fasta.py
- maf_to_int_seqs.py
- maf_translate_chars.py
- maf_truncate.py
- maf_word_frequency.py
- makeBAM.sh
- makeDiff.sh
- makeFastq.sh
- make_unique
- makepBAM_genome.sh
- makepBAM_transcriptome.sh
- mapBed
- maq2sam-long
- maq2sam-short
- maskFastaFromBed
- mask_quality.py
- mergeBed
- metaseq-cli
- multiBamCov
- multiBamSummary
- multiBigwigSummary
- multiIntersectBed
- nib_chrom_intervals_to_fasta.py
- nib_intervals_to_fasta.py
- nib_length.py
- novo2sam.pl
- nucBed
- one_field_per_line.py
- out_to_chain.py
- pairToBed
- pairToPair
- pbam2bam
- pbam_mapped_transcriptome
- pbt_plotting_example.py
- peak_pie.py
- plot-bamstats
- plotCorrelation
- plotCoverage
- plotEnrichment
- plotFingerprint
- plotHeatmap
- plotPCA
- plotProfile
- prefix_lines.py
- pretty_table.py
- print_unique
- psl2sam.pl
- py.test
- pybabel
- pybedtools
- pygmentize
- pytest
- python-argcomplete-check-easy-install-script
- python-argcomplete-tcsh
- qv_to_bqv.py
- randomBed
- random_lines.py
- register-python-argcomplete
- sam2vcf.pl
- samtools
- samtools.pl
- seq_cache_populate.pl
- shiftBed
- shuffleBed
- slopBed
- soap2sam.pl
- sortBed
- speedtest.py
- subtractBed
- table_add_column.py
- table_filter.py
- tagBam
- tfloc_summary.py
- ucsc_gene_table_to_intervals.py
- undill
- unionBedGraphs
- varfilter.py
- venn_gchart.py
- venn_mpl.py
- wgsim
- wgsim_eval.pl
- wiggle_to_array_tree.py
- wiggle_to_binned_array.py
- wiggle_to_chr_binned_array.py
- wiggle_to_simple.py
- windowBed
- windowMaker
- zoom2sam.pl
Link to section 'Module' of 'atac-seq-pipeline' Module
You can load the modules by:
module load biocontainers
module load atac-seq-pipeline
Link to section 'Example job' of 'atac-seq-pipeline' Example job
Using #!/bin/sh -l
as shebang in the slurm job script will cause the failure of some biocontainer modules. Please use #!/bin/bash
instead.
To run atac-seq-pipeline on our clusters:
#!/bin/bash
#SBATCH -A myallocation # Allocation name
#SBATCH -t 1:00:00
#SBATCH -N 1
#SBATCH -n 1
#SBATCH --job-name=atac-seq-pipeline
#SBATCH --mail-type=FAIL,BEGIN,END
#SBATCH --error=%x-%J-%u.err
#SBATCH --output=%x-%J-%u.out
module --force purge
ml biocontainers atac-seq-pipeline
ataqv
Link to section 'Introduction' of 'ataqv' Introduction
Ataqv
is a toolkit for measuring and comparing ATAC-seq results, made in the Parker lab at the University of Michigan.
For more information, please check its website: https://biocontainers.pro/tools/ataqv and its home page on Github.
Link to section 'Versions' of 'ataqv' Versions
- 1.3.0
Link to section 'Commands' of 'ataqv' Commands
- ataqv
Link to section 'Module' of 'ataqv' Module
You can load the modules by:
module load biocontainers
module load ataqv
Link to section 'Example job' of 'ataqv' Example job
Using #!/bin/sh -l
as shebang in the slurm job script will cause the failure of some biocontainer modules. Please use #!/bin/bash
instead.
To run Ataqv on our clusters:
#!/bin/bash
#SBATCH -A myallocation # Allocation name
#SBATCH -t 1:00:00
#SBATCH -N 1
#SBATCH -n 1
#SBATCH --job-name=ataqv
#SBATCH --mail-type=FAIL,BEGIN,END
#SBATCH --error=%x-%J-%u.err
#SBATCH --output=%x-%J-%u.out
module --force purge
ml biocontainers ataqv
ataqv --peak-file sample_1_peaks.broadPeak \
--name sample_1 --metrics-file sample_1.ataqv.json.gz \
--excluded-region-file hg19.blacklist.bed.gz \
--tss-file hg19.tss.refseq.bed.gz \
--ignore-read-groups human sample_1.md.bam \
> sample_1.ataqv.out
ataqv --peak-file sample_2_peaks.broadPeak \
--name sample_2 --metrics-file sample_2.ataqv.json.gz \
--excluded-region-file hg19.blacklist.bed.gz \
--tss-file hg19.tss.refseq.bed.gz \
--ignore-read-groups human sample_2.md.bam \
> sample_2.ataqv.out
ataqv --peak-file sample_3_peaks.broadPeak \
--name sample_3 --metrics-file sample_3.ataqv.json.gz \
--excluded-region-file hg19.blacklist.bed.gz \
--tss-file hg19.tss.refseq.bed.gz \
--ignore-read-groups human sample_3.md.bam \
> sample_3.ataqv.out
mkarv my_fantastic_experiment sample_1.ataqv.json.gz sample_2.ataqv.json.gz sample_3.ataqv.json.gz
atram
aTRAM
(automated target restricted assembly method) is an iterative assembler that performs reference-guided local de novo assemblies using a variety of available methods.
Detailed usage can be found here: https://bioinformaticshome.com/tools/wga/descriptions/aTRAM.html
Link to section 'Versions' of 'atram' Versions
- 2.4.3
Link to section 'Commands' of 'atram' Commands
- atram.py
- atram_preprocessor.py
- atram_stitcher.py
Link to section 'Module' of 'atram' Module
You can load the modules by:
module load biocontainers
module load atram/2.4.3
Link to section 'Example job' of 'atram' Example job
Using #!/bin/sh -l
as shebang in the slurm job script will cause the failure of some biocontainer modules. Please use #!/bin/bash
instead.
To run aTRAM on our our clusters:
#!/bin/bash
#SBATCH -A myallocation # Allocation name
#SBATCH -t 20:00:00
#SBATCH -N 1
#SBATCH -n 24
#SBATCH --job-name=atram
#SBATCH --mail-type=FAIL,BEGIN,END
#SBATCH --error=%x-%J-%u.err
#SBATCH --output=%x-%J-%u.out
module --force purge
ml biocontainers atram/2.4.3a
atram_preprocessor.py --blast-db=atram_db \
--end-1=data/tutorial_end_1.fasta.gz \
--end-2=data/tutorial_end_2.fasta.gz \
--gzip
atram.py --query=tutorial-query.pep.fasta \
--blast-db=atram_db \
--output=output \
--assembler=velvet
atropos
Link to section 'Introduction' of 'atropos' Introduction
Atropos
is a tool for specific, sensitive, and speedy trimming of NGS reads.
For more information, please check its website: https://biocontainers.pro/tools/atropos and its home page on Github.
Link to section 'Versions' of 'atropos' Versions
- 1.1.17
- 1.1.31
Link to section 'Commands' of 'atropos' Commands
- atropos
Link to section 'Module' of 'atropos' Module
You can load the modules by:
module load biocontainers
module load atropos
Link to section 'Example job' of 'atropos' Example job
Using #!/bin/sh -l
as shebang in the slurm job script will cause the failure of some biocontainer modules. Please use #!/bin/bash
instead.
To run Atropos on our clusters:
#!/bin/bash
#SBATCH -A myallocation # Allocation name
#SBATCH -t 1:00:00
#SBATCH -N 1
#SBATCH -n 4
#SBATCH --job-name=atropos
#SBATCH --mail-type=FAIL,BEGIN,END
#SBATCH --error=%x-%J-%u.err
#SBATCH --output=%x-%J-%u.out
module --force purge
ml biocontainers atropos
atropos --threads 4 \
-a AGATCGGAAGAGCACACGTCTGAACTCCAGTCACGAGTTA \
-o trimmed1.fq.gz -p trimmed2.fq.gz \
-pe1 SRR13176582_1.fastq -pe2 SRR13176582_2.fastq
augur
Link to section 'Introduction' of 'augur' Introduction
Augur
is the bioinformatics toolkit we use to track evolution from sequence and serological data.
For more information, please check its website: https://biocontainers.pro/tools/augur and its home page on Github.
Link to section 'Versions' of 'augur' Versions
- 14.0.0
- 15.0.0
Link to section 'Commands' of 'augur' Commands
- augur
Link to section 'Module' of 'augur' Module
You can load the modules by:
module load biocontainers
module load augur
Link to section 'Example job' of 'augur' Example job
Using #!/bin/sh -l
as shebang in the slurm job script will cause the failure of some biocontainer modules. Please use #!/bin/bash
instead.
To run Augur on our our clusters:
#!/bin/bash
#SBATCH -A myallocation # Allocation name
#SBATCH -t 1:00:00
#SBATCH -N 1
#SBATCH -n 1
#SBATCH --job-name=augur
#SBATCH --mail-type=FAIL,BEGIN,END
#SBATCH --error=%x-%J-%u.err
#SBATCH --output=%x-%J-%u.out
module --force purge
ml biocontainers augur
mkdir -p results
augur index --sequences zika-tutorial/data/sequences.fasta \
--output results/sequence_index.tsv
augur filter --sequences zika-tutorial/data/sequences.fasta \
--sequence-index results/sequence_index.tsv \
--metadata zika-tutorial/data/metadata.tsv \
--exclude zika-tutorial/config/dropped_strains.txt \
--output results/filtered.fasta \
--group-by country year month \
--sequences-per-group 20 \
--min-date 2012
augur align --sequences results/filtered.fasta \
--reference-sequence zika-tutorial/config/zika_outgroup.gb \
--output results/aligned.fasta \
--fill-gaps
augur tree --alignment results/aligned.fasta \
--output results/tree_raw.nwk
augur refine --tree results/tree_raw.nwk \
--alignment results/aligned.fasta \
--metadata zika-tutorial/data/metadata.tsv \
--output-tree results/tree.nwk \
--output-node-data results/branch_lengths.json \
--timetree \
--coalescent opt \
--date-confidence \
--date-inference marginal \
--clock-filter-iqd 4
augustus
Link to section 'Introduction' of 'augustus' Introduction
AUGUSTUS
is a program that predicts genes in eukaryotic genomic sequences.
For more information, please check its website: https://bioinf.uni-greifswald.de/augustus/.
Link to section 'Versions' of 'augustus' Versions
- 3.4.0
- 3.5.0
Commands
- aln2wig
- augustus
- bam2wig
- bam2wig-dist
- consensusFinder
- curve2hints
- etraining
- fastBlockSearch
- filterBam
- getSeq
- getSeq-dist
- homGeneMapping
- joingenes
- prepareAlign
Link to section 'Module' of 'augustus' Module
You can load the modules by:
module load biocontainers
module load augustus/3.4.0
Link to section 'Example job' of 'augustus' Example job
Using #!/bin/sh -l
as shebang in the slurm job script will cause the failure of some biocontainer modules. Please use #!/bin/bash
instead.
To run AUGUSTUS on our cluster:
#!/bin/bash
#SBATCH -A myallocation # Allocation name
#SBATCH -t 10:00:00
#SBATCH -N 1
#SBATCH -n 24
#SBATCH --job-name=AUGUSTUS
#SBATCH --mail-type=FAIL,BEGIN,END
#SBATCH --error=%x-%J-%u.err
#SBATCH --output=%x-%J-%u.out
module --force purge
ml biocontainers augustus/3.4.0
augustus --species=botrytis_cinerea genome.fasta > annotation.gff
bactopia
Link to section 'Introduction' of 'bactopia' Introduction
Bactopia is a flexible pipeline for complete analysis of bacterial genomes. The goal of Bactopia is to process your data with a broad set of tools, so that you can get to the fun part of analyses quicker!
Docker hub: https://hub.docker.com/r/bactopia/bactopia
Home page: https://github.com/bactopia/bactopia
Link to section 'Versions' of 'bactopia' Versions
- 2.0.3
- 2.1.1
- 2.2.0
- 3.0.0
Link to section 'Commands' of 'bactopia' Commands
- bactopia
Link to section 'Module' of 'bactopia' Module
You can load the modules by:
module load biocontainers
module load bactopia
Link to section 'Example job' of 'bactopia' Example job
Using #!/bin/sh -l
as shebang in the slurm job script will cause the failure of some biocontainer modules. Please use #!/bin/bash
instead.
To run bactopia on our clusters:
#!/bin/bash
#SBATCH -A myallocation # Allocation name
#SBATCH -t 1:00:00
#SBATCH -N 1
#SBATCH -n 12
#SBATCH --job-name=bactopia
#SBATCH --mail-type=FAIL,BEGIN,END
#SBATCH --error=%x-%J-%u.err
#SBATCH --output=%x-%J-%u.out
module --force purge
ml biocontainers bactopia
bactopia datasets \
--ariba "vfdb_core,card" \
--species "Staphylococcus aureus" \
--include_genus \
--limit 100 \
--cpus 12
bactopia --accession SRX4563634 \
--datasets datasets/ \
--species "Staphylococcus aureus" \
--coverage 100 \
--genome_size median \
--outdir ena-single-sample \
--max_cpus 12
bali-phy
Link to section 'Introduction' of 'bali-phy' Introduction
Bali-phy is a tool for bayesian co-estimation of phylogenies and multiple alignments via MCMC.
BioContainers: https://biocontainers.pro/tools/bali-phy
Home page: https://github.com/bredelings/BAli-Phy
Link to section 'Versions' of 'bali-phy' Versions
- 3.6.0
Link to section 'Commands' of 'bali-phy' Commands
- bali-phy
Link to section 'Module' of 'bali-phy' Module
You can load the modules by:
module load biocontainers
module load bali-phy
Link to section 'Example job' of 'bali-phy' Example job
Using #!/bin/sh -l
as shebang in the slurm job script will cause the failure of some biocontainer modules. Please use #!/bin/bash
instead.
To run bali-phy on our clusters:
#!/bin/bash
#SBATCH -A myallocation # Allocation name
#SBATCH -t 1:00:00
#SBATCH -N 1
#SBATCH -n 1
#SBATCH --job-name=bali-phy
#SBATCH --mail-type=FAIL,BEGIN,END
#SBATCH --error=%x-%J-%u.err
#SBATCH --output=%x-%J-%u.out
module --force purge
ml biocontainers bali-phy
bali-phy examples/sequences/ITS/ITS1.fasta 5.8S.fasta ITS2.fasta --test
bali-phy examples/sequences/5S-rRNA/5d-clustalw.fasta -S gtr+Rates.gamma[4]+inv -n 5d-free
bam-readcount
Link to section 'Introduction' of 'bam-readcount' Introduction
Bam-readcount
is a utility that runs on a BAM or CRAM file and generates low-level information about sequencing data at specific nucleotide positions.
Docker hub: https://hub.docker.com/r/mgibio/bam-readcount and its home page on Github.
Link to section 'Versions' of 'bam-readcount' Versions
- 1.0.0
Link to section 'Commands' of 'bam-readcount' Commands
- bam-readcount
Link to section 'Module' of 'bam-readcount' Module
You can load the modules by:
module load biocontainers
module load bam-readcount
Link to section 'Example job' of 'bam-readcount' Example job
Using #!/bin/sh -l
as shebang in the slurm job script will cause the failure of some biocontainer modules. Please use #!/bin/bash
instead.
To run Bam-readcount on our clusters:
#!/bin/bash
#SBATCH -A myallocation # Allocation name
#SBATCH -t 1:00:00
#SBATCH -N 1
#SBATCH -n 1
#SBATCH --job-name=bam-readcount
#SBATCH --mail-type=FAIL,BEGIN,END
#SBATCH --error=%x-%J-%u.err
#SBATCH --output=%x-%J-%u.out
module --force purge
ml biocontainers bam-readcount
bam-readcount -f Homo_sapiens.GRCh38.dna.primary_assembly.fa Aligned.sortedByCoord.out.bam
bamgineer
Link to section 'Introduction' of 'bamgineer' Introduction
Bamgineer
is a tool that can be used to introduce user-defined haplotype-phased allele-specific copy number variations (CNV) into an existing Binary Alignment Mapping (BAM) file with demonstrated applicability to simulate somatic cancer CNVs in phased whole-genome sequencing datasets.
Docker hub: https://hub.docker.com/r/suluxan/bamgineer-v2 and its home page on Github.
Link to section 'Versions' of 'bamgineer' Versions
- 1.1
Link to section 'Commands' of 'bamgineer' Commands
- simulate.py
Link to section 'Module' of 'bamgineer' Module
You can load the modules by:
module load biocontainers
module load bamgineer
Link to section 'Example job' of 'bamgineer' Example job
Using #!/bin/sh -l
as shebang in the slurm job script will cause the failure of some biocontainer modules. Please use #!/bin/bash
instead.
To run Bamgineer on our clusters:
#!/bin/bash
#SBATCH -A myallocation # Allocation name
#SBATCH -t 1:00:00
#SBATCH -N 1
#SBATCH -n 1
#SBATCH --job-name=bamgineer
#SBATCH --mail-type=FAIL,BEGIN,END
#SBATCH --error=%x-%J-%u.err
#SBATCH --output=%x-%J-%u.out
module --force purge
ml biocontainers bamgineer
simulate.py -config inputs/config.cfg \
-splitbamdir splitbams \
-cnv_bed inputs/cnv.bed \
-vcf inputs/normal_het.vcf \
-exons inputs/exons.bed \
-outbam tumour.bam \
-results outputs \
-cancertype LUAC1
bamliquidator
Link to section 'Introduction' of 'bamliquidator' Introduction
Bamliquidator
is a set of tools for analyzing the density of short DNA sequence read alignments in the BAM file format.
Docker hub: https://hub.docker.com/r/bioliquidator/bamliquidator/ and its home page on Github.
Link to section 'Versions' of 'bamliquidator' Versions
- 1.5.2
Link to section 'Commands' of 'bamliquidator' Commands
- bamliquidator
- bamliquidator_bins
- bamliquidator_regions
- bamliquidatorbatch
Link to section 'Module' of 'bamliquidator' Module
You can load the modules by:
module load biocontainers
module load bamliquidator
Link to section 'Example job' of 'bamliquidator' Example job
Using #!/bin/sh -l
as shebang in the slurm job script will cause the failure of some biocontainer modules. Please use #!/bin/bash
instead.
To run Bamliquidator on our clusters:
#!/bin/bash
#SBATCH -A myallocation # Allocation name
#SBATCH -t 1:00:00
#SBATCH -N 1
#SBATCH -n 1
#SBATCH --job-name=bamliquidator
#SBATCH --mail-type=FAIL,BEGIN,END
#SBATCH --error=%x-%J-%u.err
#SBATCH --output=%x-%J-%u.out
module --force purge
ml biocontainers bamliquidator
bamsurgeon
Link to section 'Introduction' of 'bamsurgeon' Introduction
Bamsurgeon
are tools for adding mutations to .bam files, used for testing mutation callers.
Docker hub: https://hub.docker.com/r/lethalfang/bamsurgeon and its home page on Github.
Link to section 'Versions' of 'bamsurgeon' Versions
- 1.2
Link to section 'Commands' of 'bamsurgeon' Commands
- addindel.py
- addsnv.py
- addsv.py
Link to section 'Module' of 'bamsurgeon' Module
You can load the modules by:
module load biocontainers
module load bamsurgeon
Link to section 'Example job' of 'bamsurgeon' Example job
Using #!/bin/sh -l
as shebang in the slurm job script will cause the failure of some biocontainer modules. Please use #!/bin/bash
instead.
To run Bamsurgeon on our clusters:
#!/bin/bash
#SBATCH -A myallocation # Allocation name
#SBATCH -t 1:00:00
#SBATCH -N 1
#SBATCH -n 1
#SBATCH --job-name=bamsurgeon
#SBATCH --mail-type=FAIL,BEGIN,END
#SBATCH --error=%x-%J-%u.err
#SBATCH --output=%x-%J-%u.out
module --force purge
ml biocontainers bamsurgeon
addsv.py -p 1 -v test_sv.txt -f testregion_realign.bam \
-r reference.fasta -o testregion_sv_mut.bam \
--aligner mem --keepsecondary --seed 1234 \
--inslib test_inslib.fa
bamtools
Link to section 'Introduction' of 'bamtools' Introduction
BamTools
is a programmer API and an end-user toolkit for handling BAM files. This container provides a toolkit-only version (no API to build against).
For more information, please check its website: https://biocontainers.pro/tools/bamtools and its home page on Github.
Link to section 'Versions' of 'bamtools' Versions
- 2.5.1
Link to section 'Commands' of 'bamtools' Commands
- bamtools
Link to section 'Module' of 'bamtools' Module
You can load the modules by:
module load biocontainers
module load bamtools
Link to section 'Example job' of 'bamtools' Example job
Using #!/bin/sh -l
as shebang in the slurm job script will cause the failure of some biocontainer modules. Please use #!/bin/bash
instead.
To run BamTools on our clusters:
#!/bin/bash
#SBATCH -A myallocation # Allocation name
#SBATCH -t 1:00:00
#SBATCH -N 1
#SBATCH -n 1
#SBATCH --job-name=bamtools
#SBATCH --mail-type=FAIL,BEGIN,END
#SBATCH -ddd-error=%x-%J-%u.err
#SBATCH --output=%x-%J-%u.out
module --force purge
ml biocontainers bamtools
bamtools convert -format fastq -in in.bam -out out.fastq
bamutil
Link to section 'Introduction' of 'bamutil' Introduction
Bamutil
is a collection of programs for working on SAM/BAM files.
For more information, please check its website: https://biocontainers.pro/tools/bamutil and its home page on Github.
Link to section 'Versions' of 'bamutil' Versions
- 1.0.15
Link to section 'Commands' of 'bamutil' Commands
- bam
Link to section 'Module' of 'bamutil' Module
You can load the modules by:
module load biocontainers
module load bamutil
Link to section 'Example job' of 'bamutil' Example job
Using #!/bin/sh -l
as shebang in the slurm job script will cause the failure of some biocontainer modules. Please use #!/bin/bash
instead.
To run Bamutil on our clusters:
#!/bin/bash
#SBATCH -A myallocation # Allocation name
#SBATCH -t 1:00:00
#SBATCH -N 1
#SBATCH -n 1
#SBATCH --job-name=bamutil
#SBATCH --mail-type=FAIL,BEGIN,END
#SBATCH --error=%x-%J-%u.err
#SBATCH --output=%x-%J-%u.out
module --force purge
ml biocontainers bamutil
bam validate --params --in test/testFiles/testInvalid.sam --refFile test/testFilesLibBam/chr1_partial.fa --v --noph 2> results/validateInvalid.txt
bam convert --params --in test/testFiles/testFilter.bam --out results/convertBam.sam --noph 2> results/convertBam.log
bam splitChromosome --in test/testFile/sortedBam1.bam --out results/splitSortedBam --noph 2> results/splitChromosome.txt
bam stats --basic --in test/testFiles/testFilter.sam --noph 2> results/basicStats.txt
bam gapInfo --in test/testFiles/testGapInfo.sam --out results/gapInfo.txt --noph 2> results/gapInfo.log
bam findCigars --in test/testFiles/testRevert.sam --out results/cigarNonM.sam --nonM --noph 2> results/cigarNonM.log
barrnap
Link to section 'Introduction' of 'barrnap' Introduction
Barrnap
: BAsic Rapid Ribosomal RNA Predictor.
For more information, please check its website: https://biocontainers.pro/tools/barrnap and its home page on Github.
Link to section 'Versions' of 'barrnap' Versions
- 0.9.4
Link to section 'Commands' of 'barrnap' Commands
- barrnap
Link to section 'Module' of 'barrnap' Module
You can load the modules by:
module load biocontainers
module load barrnap
Link to section 'Example job' of 'barrnap' Example job
Using #!/bin/sh -l
as shebang in the slurm job script will cause the failure of some biocontainer modules. Please use #!/bin/bash
instead.
To run Barrnap on our clusters:
#!/bin/bash
#SBATCH -A myallocation # Allocation name
#SBATCH -t 1:00:00
#SBATCH -N 1
#SBATCH -n 1
#SBATCH --job-name=barrnap
#SBATCH --mail-type=FAIL,BEGIN,END
#SBATCH --error=%x-%J-%u.err
#SBATCH --output=%x-%J-%u.out
module --force purge
ml biocontainers barrnap
barrnap --kingdom bac -o bac_16s.fasta < bac_genome.fasta > bac_16s.gff3
barrnap --kingdom euk -o euk_16s.fasta < euk_genome.fasta > euk_16s.gff3
basenji
Link to section 'Introduction' of 'basenji' Introduction
Basenji
is a tool for sequential regulatory activity predictions with deep convolutional neural networks.
For more information, please check its website: https://biocontainers.pro/tools/basenji and its home page on Github.
Link to section 'Versions' of 'basenji' Versions
- 0.5.1
Link to section 'Commands' of 'basenji' Commands
- akita_data.py
- akita_data_read.py
- akita_data_write.py
- akita_predict.py
- akita_sat_plot.py
- akita_sat_vcf.py
- akita_scd.py
- akita_scd_multi.py
- akita_test.py
- akita_train.py
- bam_cov.py
- basenji_annot_chr.py
- basenji_bench_classify.py
- basenji_bench_gtex.py
- basenji_bench_gtex_cmp.py
- basenji_bench_phylop.py
- basenji_bench_phylop_folds.py
- basenji_cmp.py
- basenji_data.py
- basenji_data2.py
- basenji_data_align.py
- basenji_data_gene.py
- basenji_data_hic_read.py
- basenji_data_hic_write.py
- basenji_data_read.py
- basenji_data_write.py
- basenji_fetch_app.py
- basenji_fetch_app1.py
- basenji_fetch_app2.py
- basenji_fetch_norm.py
- basenji_fetch_vcf.py
- basenji_gtex_folds.py
- basenji_hdf5_genes.py
- basenji_hidden.py
- basenji_map.py
- basenji_map_genes.py
- basenji_map_seqs.py
- basenji_motifs.py
- basenji_motifs_denovo.py
- basenji_norm_h5.py
- basenji_predict.py
- basenji_predict_bed.py
- basenji_predict_bed_multi.py
- basenji_sad.py
- basenji_sad_multi.py
- basenji_sad_norm.py
- basenji_sad_ref.py
- basenji_sad_ref_multi.py
- basenji_sad_table.py
- basenji_sat_bed.py
- basenji_sat_bed_multi.py
- basenji_sat_folds.py
- basenji_sat_plot.py
- basenji_sat_plot2.py
- basenji_sat_vcf.py
- basenji_sed.py
- basenji_sed_multi.py
- basenji_sedg.py
- basenji_test.py
- basenji_test_folds.py
- basenji_test_genes.py
- basenji_test_reps.py
- basenji_test_specificity.py
- basenji_train.py
- basenji_train1.py
- basenji_train2.py
- basenji_train_folds.py
- basenji_train_hic.py
- basenji_train_reps.py
- save_model.py
- sonnet_predict_bed.py
- sonnet_sad.py
- sonnet_sad_multi.py
- sonnet_sat_bed.py
- sonnet_sat_vcf.py
- tfr_bw.py
- tfr_hdf5.py
- tfr_qc.py
- upgrade_tf1.py
Link to section 'Module' of 'basenji' Module
You can load the modules by:
module load biocontainers
module load basenji
Link to section 'Example job' of 'basenji' Example job
Using #!/bin/sh -l
as shebang in the slurm job script will cause the failure of some biocontainer modules. Please use #!/bin/bash
instead.
To run Basenji on our clusters:
#!/bin/bash
#SBATCH -A myallocation # Allocation name
#SBATCH -t 1:00:00
#SBATCH -N 1
#SBATCH -n 1
#SBATCH --job-name=basenji
#SBATCH --mail-type=FAIL,BEGIN,END
#SBATCH --error=%x-%J-%u.err
#SBATCH --output=%x-%J-%u.out
module --force purge
ml biocontainers basenji
bazam
Link to section 'Introduction' of 'bazam' Introduction
Bazam is a tool to extract paired reads in FASTQ format from coordinate sorted BAM files.
For more information, please check:
Docker hub: https://hub.docker.com/r/dockanomics/bazam
Home page: https://github.com/ssadedin/bazam
Link to section 'Versions' of 'bazam' Versions
- 1.0.1
Link to section 'Commands' of 'bazam' Commands
- bazam
Link to section 'Module' of 'bazam' Module
You can load the modules by:
module load biocontainers
module load bazam
Link to section 'Example job' of 'bazam' Example job
Using #!/bin/sh -l
as shebang in the slurm job script will cause the failure of some biocontainer modules. Please use #!/bin/bash
instead.
To run bazam on our clusters:
#!/bin/bash
#SBATCH -A myallocation # Allocation name
#SBATCH -t 1:00:00
#SBATCH -N 1
#SBATCH -n 1
#SBATCH --job-name=bazam
#SBATCH --mail-type=FAIL,BEGIN,END
#SBATCH --error=%x-%J-%u.err
#SBATCH --output=%x-%J-%u.out
module --force purge
ml biocontainers bazam
bbmap
Link to section 'Introduction' of 'bbmap' Introduction
Bbmap
is a short read aligner, as well as various other bioinformatic tools.
For more information, please check its website: https://biocontainers.pro/tools/bbmap and its home page on Sourceforge.
Link to section 'Versions' of 'bbmap' Versions
- 38.93
- 38.96
Link to section 'Commands' of 'bbmap' Commands
- addadapters.sh
- a_sample_mt.sh
- bbcountunique.sh
- bbduk.sh
- bbest.sh
- bbfakereads.sh
- bbmap.sh
- bbmapskimmer.sh
- bbmask.sh
- bbmerge-auto.sh
- bbmergegapped.sh
- bbmerge.sh
- bbnorm.sh
- bbqc.sh
- bbrealign.sh
- bbrename.sh
- bbsketch.sh
- bbsplitpairs.sh
- bbsplit.sh
- bbstats.sh
- bbversion.sh
- bbwrap.sh
- calcmem.sh
- calctruequality.sh
- callpeaks.sh
- callvariants2.sh
- callvariants.sh
- clumpify.sh
- commonkmers.sh
- comparesketch.sh
- comparevcf.sh
- consect.sh
- countbarcodes.sh
- countgc.sh
- countsharedlines.sh
- crossblock.sh
- crosscontaminate.sh
- cutprimers.sh
- decontaminate.sh
- dedupe2.sh
- dedupebymapping.sh
- dedupe.sh
- demuxbyname.sh
- diskbench.sh
- estherfilter.sh
- explodetree.sh
- filterassemblysummary.sh
- filterbarcodes.sh
- filterbycoverage.sh
- filterbyname.sh
- filterbysequence.sh
- filterbytaxa.sh
- filterbytile.sh
- filterlines.sh
- filtersam.sh
- filtersubs.sh
- filtervcf.sh
- fungalrelease.sh
- fuse.sh
- getreads.sh
- gi2ancestors.sh
- gi2taxid.sh
- gitable.sh
- grademerge.sh
- gradesam.sh
- idmatrix.sh
- idtree.sh
- invertkey.sh
- kcompress.sh
- khist.sh
- kmercountexact.sh
- kmercountmulti.sh
- kmercoverage.sh
- loadreads.sh
- loglog.sh
- makechimeras.sh
- makecontaminatedgenomes.sh
- makepolymers.sh
- mapPacBio.sh
- matrixtocolumns.sh
- mergebarcodes.sh
- mergeOTUs.sh
- mergesam.sh
- msa.sh
- mutate.sh
- muxbyname.sh
- normandcorrectwrapper.sh
- partition.sh
- phylip2fasta.sh
- pileup.sh
- plotgc.sh
- postfilter.sh
- printtime.sh
- processfrag.sh
- processspeed.sh
- randomreads.sh
- readlength.sh
- reducesilva.sh
- reformat.sh
- removebadbarcodes.sh
- removecatdogmousehuman.sh
- removehuman2.sh
- removehuman.sh
- removemicrobes.sh
- removesmartbell.sh
- renameimg.sh
- rename.sh
- repair.sh
- replaceheaders.sh
- representative.sh
- rqcfilter.sh
- samtoroc.sh
- seal.sh
- sendsketch.sh
- shred.sh
- shrinkaccession.sh
- shuffle.sh
- sketchblacklist.sh
- sketch.sh
- sortbyname.sh
- splitbytaxa.sh
- splitnextera.sh
- splitsam4way.sh
- splitsam6way.sh
- splitsam.sh
- stats.sh
- statswrapper.sh
- streamsam.sh
- summarizecrossblock.sh
- summarizemerge.sh
- summarizequast.sh
- summarizescafstats.sh
- summarizeseal.sh
- summarizesketch.sh
- synthmda.sh
- tadpipe.sh
- tadpole.sh
- tadwrapper.sh
- taxonomy.sh
- taxserver.sh
- taxsize.sh
- taxtree.sh
- testfilesystem.sh
- testformat2.sh
- testformat.sh
- tetramerfreq.sh
- textfile.sh
- translate6frames.sh
- unicode2ascii.sh
- webcheck.sh
Link to section 'Module' of 'bbmap' Module
You can load the modules by:
module load biocontainers
module load bbmap
Link to section 'Example job' of 'bbmap' Example job
Using #!/bin/sh -l
as shebang in the slurm job script will cause the failure of some biocontainer modules. Please use #!/bin/bash
instead.
To run Bbmap on our clusters:
#!/bin/bash
#SBATCH -A myallocation # Allocation name
#SBATCH -t 1:00:00
#SBATCH -N 1
#SBATCH -n 1
#SBATCH --job-name=bbmap
#SBATCH --mail-type=FAIL,BEGIN,END
#SBATCH --error=%x-%J-%u.err
#SBATCH --output=%x-%J-%u.out
module --force purge
ml biocontainers bbmap
stats.sh in=SRR11234553_1.fastq > stats_out.txt
statswrapper.sh *.fastq > statswrapper_out.txt
pileup.sh in=map1.sam out=pileup_out.txt
readlength.sh in=SRR11234553_1.fastq in2=SRR11234553_2.fastq > readlength_out.txt
kmercountexact.sh in=SRR11234553_1.fastq in2=SRR11234553_2.fastq out=kmer_test.out khist=kmer.khist peaks=kmer.peak
bbmask.sh in=SRR11234553_1.fastq out=test.mark sam=map1.sam
bbtools
Link to section 'Introduction' of 'bbtools' Introduction
BBTools is a suite of fast, multithreaded bioinformatics tools designed for analysis of DNA and RNA sequence data.
Docker hub: https://hub.docker.com/r/staphb/bbtools
Home page: https://jgi.doe.gov/data-and-tools/software-tools/bbtools/
Link to section 'Versions' of 'bbtools' Versions
- 39.00
Link to section 'Commands' of 'bbtools' Commands
- Xcalcmem.sh
- a_sample_mt.sh
- addadapters.sh
- addssu.sh
- adjusthomopolymers.sh
- alltoall.sh
- analyzeaccession.sh
- analyzegenes.sh
- analyzesketchresults.sh
- applyvariants.sh
- bbcms.sh
- bbcountunique.sh
- bbduk.sh
- bbest.sh
- bbfakereads.sh
- bbmap.sh
- bbmapskimmer.sh
- bbmask.sh
- bbmerge-auto.sh
- bbmerge.sh
- bbnorm.sh
- bbrealign.sh
- bbrename.sh
- bbsketch.sh
- bbsplit.sh
- bbsplitpairs.sh
- bbstats.sh
- bbversion.sh
- bbwrap.sh
- bloomfilter.sh
- calcmem.sh
- calctruequality.sh
- callgenes.sh
- callpeaks.sh
- callvariants.sh
- callvariants2.sh
- clumpify.sh
- commonkmers.sh
- comparegff.sh
- comparesketch.sh
- comparessu.sh
- comparevcf.sh
- consect.sh
- consensus.sh
- countbarcodes.sh
- countgc.sh
- countsharedlines.sh
- crossblock.sh
- crosscontaminate.sh
- cutgff.sh
- cutprimers.sh
- decontaminate.sh
- dedupe.sh
- dedupe2.sh
- dedupebymapping.sh
- demuxbyname.sh
- diskbench.sh
- estherfilter.sh
- explodetree.sh
- fetchproks.sh
- filterassemblysummary.sh
- filterbarcodes.sh
- filterbycoverage.sh
- filterbyname.sh
- filterbysequence.sh
- filterbytaxa.sh
- filterbytile.sh
- filterlines.sh
- filterqc.sh
- filtersam.sh
- filtersilva.sh
- filtersubs.sh
- filtervcf.sh
- fixgaps.sh
- fungalrelease.sh
- fuse.sh
- gbff2gff.sh
- getreads.sh
- gi2ancestors.sh
- gi2taxid.sh
- gitable.sh
- grademerge.sh
- gradesam.sh
- icecreamfinder.sh
- icecreamgrader.sh
- icecreammaker.sh
- idmatrix.sh
- idtree.sh
- invertkey.sh
- kapastats.sh
- kcompress.sh
- keepbestcopy.sh
- khist.sh
- kmercountexact.sh
- kmercountmulti.sh
- kmercoverage.sh
- kmerfilterset.sh
- kmerlimit.sh
- kmerlimit2.sh
- kmerposition.sh
- kmutate.sh
- lilypad.sh
- loadreads.sh
- loglog.sh
- makechimeras.sh
- makecontaminatedgenomes.sh
- makepolymers.sh
- mapPacBio.sh
- matrixtocolumns.sh
- mergeOTUs.sh
- mergebarcodes.sh
- mergepgm.sh
- mergeribo.sh
- mergesam.sh
- mergesketch.sh
- mergesorted.sh
- msa.sh
- mutate.sh
- muxbyname.sh
- partition.sh
- phylip2fasta.sh
- pileup.sh
- plotflowcell.sh
- plotgc.sh
- postfilter.sh
- printtime.sh
- processfrag.sh
- processhi-c.sh
- processspeed.sh
- randomgenome.sh
- randomreads.sh
- readlength.sh
- readqc.sh
- reducesilva.sh
- reformat.sh
- reformatpb.sh
- removebadbarcodes.sh
- removecatdogmousehuman.sh
- removehuman.sh
- removehuman2.sh
- removemicrobes.sh
- removesmartbell.sh
- rename.sh
- renameimg.sh
- repair.sh
- replaceheaders.sh
- representative.sh
- rqcfilter.sh
- rqcfilter2.sh
- runhmm.sh
- samtoroc.sh
- seal.sh
- sendsketch.sh
- shred.sh
- shrinkaccession.sh
- shuffle.sh
- shuffle2.sh
- sketch.sh
- sketchblacklist.sh
- sketchblacklist2.sh
- sortbyname.sh
- splitbytaxa.sh
- splitnextera.sh
- splitribo.sh
- splitsam.sh
- splitsam4way.sh
- splitsam6way.sh
- stats.sh
- statswrapper.sh
- streamsam.sh
- subsketch.sh
- summarizecontam.sh
- summarizecoverage.sh
- summarizecrossblock.sh
- summarizemerge.sh
- summarizequast.sh
- summarizescafstats.sh
- summarizeseal.sh
- summarizesketch.sh
- synthmda.sh
- tadpipe.sh
- tadpole.sh
- tadwrapper.sh
- taxonomy.sh
- taxserver.sh
- taxsize.sh
- taxtree.sh
- testfilesystem.sh
- testformat.sh
- testformat2.sh
- tetramerfreq.sh
- textfile.sh
- translate6frames.sh
- unicode2ascii.sh
- unzip.sh
- vcf2gff.sh
- webcheck.sh
Link to section 'Module' of 'bbtools' Module
You can load the modules by:
module load biocontainers
module load bbtools
Link to section 'Example job' of 'bbtools' Example job
Using #!/bin/sh -l
as shebang in the slurm job script will cause the failure of some biocontainer modules. Please use #!/bin/bash
instead.
To run bbtools on our clusters:
#!/bin/bash
#SBATCH -A myallocation # Allocation name
#SBATCH -t 1:00:00
#SBATCH -N 1
#SBATCH -n 1
#SBATCH --job-name=bbtools
#SBATCH --mail-type=FAIL,BEGIN,END
#SBATCH --error=%x-%J-%u.err
#SBATCH --output=%x-%J-%u.out
module --force purge
ml biocontainers bbtools
bcftools
Link to section 'Introduction' of 'bcftools' Introduction
Bcftools
is a program for variant calling and manipulating files in the Variant Call Format (VCF) and its binary counterpart BCF.
For more information, please check its website: https://biocontainers.pro/tools/bcftools and its home page on Github.
Link to section 'Versions' of 'bcftools' Versions
- 1.13
- 1.14
- 1.17
Link to section 'Commands' of 'bcftools' Commands
- bcftools
- color-chrs.pl
- guess-ploidy.py
- plot-roh.py
- plot-vcfstats
- run-roh.pl
- vcfutils.pl
Link to section 'Module' of 'bcftools' Module
You can load the modules by:
module load biocontainers
module load bcftools
Link to section 'Example job' of 'bcftools' Example job
Using #!/bin/sh -l
as shebang in the slurm job script will cause the failure of some biocontainer modules. Please use #!/bin/bash
instead.
To run Bcftools on our clusters:
#!/bin/bash
#SBATCH -A myallocation # Allocation name
#SBATCH -t 1:00:00
#SBATCH -N 1
#SBATCH -n 1
#SBATCH --job-name=bcftools
#SBATCH --mail-type=FAIL,BEGIN,END
#SBATCH --error=%x-%J-%u.err
#SBATCH --output=%x-%J-%u.out
module --force purge
ml biocontainers bcftools
bcftools query -f '%CHROM %POS %REF %ALT\n' file.bcf
bcftools polysomy -v -o outdir/ file.vcf
# Variant calling
bcftools mpileup -f reference.fa alignments.bam | bcftools call -mv -Ob -o calls.bcf
bcl2fastq
Link to section 'Introduction' of 'bcl2fastq' Introduction
bcl2fastq Conversion Software both demultiplexes data and converts BCL files generated by Illumina sequencing systems to standard FASTQ file formats for downstream analysis.
Docker hub: https://hub.docker.com/r/gcfntnu/bcl2fastq
Home page: https://support.illumina.com/sequencing/sequencing_software/bcl2fastq-conversion-software.html
Link to section 'Versions' of 'bcl2fastq' Versions
- 2.20.0
Link to section 'Commands' of 'bcl2fastq' Commands
- bcl2fastq
Link to section 'Module' of 'bcl2fastq' Module
You can load the modules by:
module load biocontainers
module load bcl2fastq
Link to section 'Example job' of 'bcl2fastq' Example job
Using #!/bin/sh -l
as shebang in the slurm job script will cause the failure of some biocontainer modules. Please use #!/bin/bash
instead.
To run bcl2fastq on our clusters:
#!/bin/bash
#SBATCH -A myallocation # Allocation name
#SBATCH -t 1:00:00
#SBATCH -N 1
#SBATCH -n 1
#SBATCH --job-name=bcl2fastq
#SBATCH --mail-type=FAIL,BEGIN,END
#SBATCH --error=%x-%J-%u.err
#SBATCH --output=%x-%J-%u.out
module --force purge
ml biocontainers bcl2fastq
beagle
Link to section 'Introduction' of 'beagle' Introduction
Beagle
is a software package for phasing genotypes and for imputing ungenotyped markers. Start it with: beagle [java options] [arguments]
Note: Bref is not installed in this container.
For more information, please check its website: https://biocontainers.pro/tools/beagle and its home page: https://faculty.washington.edu/browning/beagle/beagle.html.
Link to section 'Versions' of 'beagle' Versions
- 5.1_24Aug19.3e8
Link to section 'Commands' of 'beagle' Commands
- beagle
Link to section 'Module' of 'beagle' Module
You can load the modules by:
module load biocontainers
module load beagle
Link to section 'Example job' of 'beagle' Example job
Using #!/bin/sh -l
as shebang in the slurm job script will cause the failure of some biocontainer modules. Please use #!/bin/bash
instead.
To run Beagle on our clusters:
#!/bin/bash
#SBATCH -A myallocation # Allocation name
#SBATCH -t 1:00:00
#SBATCH -N 1
#SBATCH -n 1
#SBATCH --job-name=beagle
#SBATCH --mail-type=FAIL,BEGIN,END
#SBATCH --error=%x-%J-%u.err
#SBATCH --output=%x-%J-%u.out
module --force purge
ml biocontainers beagle
beagle gt=test.vcf.gz out=test.out
beast2
Link to section 'Introduction' of 'beast2' Introduction
BEAST 2
is a cross-platform program for Bayesian phylogenetic analysis of molecular sequences.
For more information, please check its website: https://biocontainers.pro/tools/beast2 and its home page: https://www.beast2.org.
Link to section 'Versions' of 'beast2' Versions
- 2.6.3
- 2.6.4
- 2.6.6
Link to section 'Commands' of 'beast2' Commands
- applauncher
- beast
- beauti
- densitree
- loganalyser
- logcombiner
- packagemanager
- treeannotator
Link to section 'Module' of 'beast2' Module
You can load the modules by:
module load biocontainers
module load beast2
Link to section 'Example job' of 'beast2' Example job
Using #!/bin/sh -l
as shebang in the slurm job script will cause the failure of some biocontainer modules. Please use #!/bin/bash
instead.
To run BEAST 2 on our clusters:
#!/bin/bash
#SBATCH -A myallocation # Allocation name
#SBATCH -t 1:00:00
#SBATCH -N 1
#SBATCH -n 4
#SBATCH --job-name=beast2
#SBATCH --mail-type=FAIL,BEGIN,END
#SBATCH --error=%x-%J-%u.err
#SBATCH --output=%x-%J-%u.out
module --force purge
ml biocontainers beast2
beast -threads 4 -prefix input input.xml
bedops
Link to section 'Introduction' of 'bedops' Introduction
Bedops
is a software package for manipulating and analyzing genomic interval data.
For more information, please check its website: https://biocontainers.pro/tools/bedops and its home page: https://bedops.readthedocs.io/en/latest/.
Link to section 'Versions' of 'bedops' Versions
- 2.4.39
Link to section 'Commands' of 'bedops' Commands
- bam2bed
- bam2bed-float128
- bam2bed_gnuParallel
- bam2bed_gnuParallel-float128
- bam2bed_gnuParallel-megarow
- bam2bed_gnuParallel-typical
- bam2bed-megarow
- bam2bed_sge
- bam2bed_sge-float128
- bam2bed_sge-megarow
- bam2bed_sge-typical
- bam2bed_slurm
- bam2bed_slurm-float128
- bam2bed_slurm-megarow
- bam2bed_slurm-typical
- bam2bed-typical
- bam2starch
- bam2starch-float128
- bam2starch_gnuParallel
- bam2starch_gnuParallel-float128
- bam2starch_gnuParallel-megarow
- bam2starch_gnuParallel-typical
- bam2starch-megarow
- bam2starch_sge
- bam2starch_sge-float128
- bam2starch_sge-megarow
- bam2starch_sge-typical
- bam2starch_slurm
- bam2starch_slurm-float128
- bam2starch_slurm-megarow
- bam2starch_slurm-typical
- bam2starch-typical
- bedextract
- bedextract-float128
- bedextract-megarow
- bedextract-typical
- bedmap
- bedmap-float128
- bedmap-megarow
- bedmap-typical
- bedops
- bedops-float128
- bedops-megarow
- bedops-typical
- closest-features
- closest-features-float128
- closest-features-megarow
- closest-features-typical
- convert2bed
- convert2bed-float128
- convert2bed-megarow
- convert2bed-typical
- gff2bed
- gff2bed-float128
- gff2bed-megarow
- gff2bed-typical
- gff2starch
- gff2starch-float128
- gff2starch-megarow
- gff2starch-typical
- gtf2bed
- gtf2bed-float128
- gtf2bed-megarow
- gtf2bed-typical
- gtf2starch
- gtf2starch-float128
- gtf2starch-megarow
- gtf2starch-typical
- gvf2bed
- gvf2bed-float128
- gvf2bed-megarow
- gvf2bed-typical
- gvf2starch
- gvf2starch-float128
- gvf2starch-megarow
- gvf2starch-typical
- psl2bed
- psl2bed-float128
- psl2bed-megarow
- psl2bed-typical
- psl2starch
- psl2starch-float128
- psl2starch-megarow
- psl2starch-typical
- rmsk2bed
- rmsk2bed-float128
- rmsk2bed-megarow
- rmsk2bed-typical
- rmsk2starch
- rmsk2starch-float128
- rmsk2starch-megarow
- rmsk2starch-typical
- sam2bed
- sam2bed-float128
- sam2bed-megarow
- sam2bed-typical
- sam2starch
- sam2starch-float128
- sam2starch-megarow
- sam2starch-typical
- sort-bed
- sort-bed-float128
- sort-bed-megarow
- sort-bed-typical
- starch
- starchcat
- starchcat-float128
- starchcat-megarow
- starchcat-typical
- starchcluster_gnuParallel
- starchcluster_gnuParallel-float128
- starchcluster_gnuParallel-megarow
- starchcluster_gnuParallel-typical
- starchcluster_sge
- starchcluster_sge-float128
- starchcluster_sge-megarow
- starchcluster_sge-typical
- starchcluster_slurm
- starchcluster_slurm-float128
- starchcluster_slurm-megarow
- starchcluster_slurm-typical
- starch-diff
- starch-diff-float128
- starch-diff-megarow
- starch-diff-typical
- starch-float128
- starch-megarow
- starchstrip
- starchstrip-float128
- starchstrip-megarow
- starchstrip-typical
- starch-typical
- switch-BEDOPS-binary-type
- unstarch
- unstarch-float128
- unstarch-megarow
- unstarch-typical
- update-sort-bed-migrate-candidates
- update-sort-bed-migrate-candidates-float128
- update-sort-bed-migrate-candidates-megarow
- update-sort-bed-migrate-candidates-typical
- update-sort-bed-slurm
- update-sort-bed-slurm-float128
- update-sort-bed-slurm-megarow
- update-sort-bed-slurm-typical
- update-sort-bed-starch-slurm
- update-sort-bed-starch-slurm-float128
- update-sort-bed-starch-slurm-megarow
- update-sort-bed-starch-slurm-typical
- vcf2bed
- vcf2bed-float128
- vcf2bed-megarow
- vcf2bed-typical
- vcf2starch
- vcf2starch-float128
- vcf2starch-megarow
- vcf2starch-typical
- wig2bed
- wig2bed-float128
- wig2bed-megarow
- wig2bed-typical
- wig2starch
- wig2starch-float128
- wig2starch-megarow
- wig2starch-typical
Link to section 'Module' of 'bedops' Module
You can load the modules by:
module load biocontainers
module load bedops
Link to section 'Example job' of 'bedops' Example job
Using #!/bin/sh -l
as shebang in the slurm job script will cause the failure of some biocontainer modules. Please use #!/bin/bash
instead.
To run Bedops on our clusters:
#!/bin/bash
#SBATCH -A myallocation # Allocation name
#SBATCH -t 1:00:00
#SBATCH -N 1
#SBATCH -n 1
#SBATCH --job-name=bedops
#SBATCH --mail-type=FAIL,BEGIN,END
#SBATCH --error=%x-%J-%u.err
#SBATCH --output=%x-%J-%u.out
module --force purge
ml biocontainers bedops
bedops -m 001.merge.001.test > 001.merge.001.observed
bedops -c 001.merge.001.test > 001.complement.001.observed
bedops -i 001.intersection.001a.test 001.intersection.001b.test > 001.intersection.001.observed
bedtools
Link to section 'Introduction' of 'bedtools' Introduction
Bedtools
is an extensive suite of utilities for genome arithmetic and comparing genomic features in BED format.
For more information, please check its website: https://biocontainers.pro/tools/bedtools and its home page on Github.
Link to section 'Versions' of 'bedtools' Versions
- 2.30.0
Link to section 'Commands' of 'bedtools' Commands
- annotateBed
- bamToBed
- bamToFastq
- bed12ToBed6
- bedpeToBam
- bedToBam
- bedToIgv
- bedtools
- closestBed
- clusterBed
- complementBed
- coverageBed
- expandCols
- fastaFromBed
- flankBed
- genomeCoverageBed
- getOverlap
- groupBy
- intersectBed
- linksBed
- mapBed
- maskFastaFromBed
- mergeBed
- multiBamCov
- multiIntersectBed
- nucBed
- pairToBed
- pairToPair
- randomBed
- shiftBed
- shuffleBed
- slopBed
- sortBed
- subtractBed
- tagBam
- unionBedGraphs
- windowBed
- windowMaker
Link to section 'Module' of 'bedtools' Module
You can load the modules by:
module load biocontainers
module load bedtools
Link to section 'Example job' of 'bedtools' Example job
Using #!/bin/sh -l
as shebang in the slurm job script will cause the failure of some biocontainer modules. Please use #!/bin/bash
instead.
To run Bedtools on our clusters:
#!/bin/bash
#SBATCH -A myallocation # Allocation name
#SBATCH -t 1:00:00
#SBATCH -N 1
#SBATCH -n 1
#SBATCH --job-name=bedtools
#SBATCH --mail-type=FAIL,BEGIN,END
#SBATCH --error=%x-%J-%u.err
#SBATCH --output=%x-%J-%u.out
module --force purge
ml biocontainers bedtools
bedtools intersect -a a.bed -b b.bed
bedtools annotate -i variants.bed -files genes.bed conserve.bed known_var.bed
bioawk
Link to section 'Introduction' of 'bioawk' Introduction
Bioawk
is an extension to Brian Kernighan's awk, adding the support of several common biological data formats, including optionally gzipped BED, GFF, SAM, VCF, FASTA/Q and TAB-delimited formats with column names.
For more information, please check its website: https://biocontainers.pro/tools/bioawk and its home page on Github.
Link to section 'Versions' of 'bioawk' Versions
- 1.0
Link to section 'Commands' of 'bioawk' Commands
- bioawk
Link to section 'Module' of 'bioawk' Module
You can load the modules by:
module load biocontainers
module load bioawk
Link to section 'Example job' of 'bioawk' Example job
Using #!/bin/sh -l
as shebang in the slurm job script will cause the failure of some biocontainer modules. Please use #!/bin/bash
instead.
To run Bioawk on our clusters:
#!/bin/bash
#SBATCH -A myallocation # Allocation name
#SBATCH -t 1:00:00
#SBATCH -N 1
#SBATCH -n 1
#SBATCH --job-name=bioawk
#SBATCH --mail-type=FAIL,BEGIN,END
#SBATCH --error=%x-%J-%u.err
#SBATCH --output=%x-%J-%u.out
module --force purge
ml biocontainers bioawk
bioawk -c fastx '{print ">"$name;print revcomp($seq)}' seq.fa.gz
biobambam
Link to section 'Introduction' of 'biobambam' Introduction
Biobambam
is a collection of tools for early stage alignment file processing.
For more information, please check its website: https://biocontainers.pro/tools/biobambam and its home page on Gitlab.
Link to section 'Versions' of 'biobambam' Versions
- 2.0.183
Link to section 'Commands' of 'biobambam' Commands
- bam12auxmerge
- bam12split
- bam12strip
- bamadapterclip
- bamadapterfind
- bamalignfrac
- bamauxmerge
- bamauxmerge2
- bamauxsort
- bamcat
- bamchecksort
- bamclipXT
- bamclipreinsert
- bamcollate2
- bamdepth
- bamdepthintersect
- bamdifference
- bamdownsamplerandom
- bamexplode
- bamexploderef
- bamfastcat
- bamfastexploderef
- bamfastnumextract
- bamfastsplit
- bamfeaturecount
- bamfillquery
- bamfilteraux
- bamfiltereofblocks
- bamfilterflags
- bamfilterheader
- bamfilterheader2
- bamfilterk
- bamfilterlength
- bamfiltermc
- bamfilternames
- bamfilterrefid
- bamfilterrg
- bamfixmateinformation
- bamfixpairinfo
- bamflagsplit
- bamindex
- bamintervalcomment
- bamintervalcommenthist
- bammapdist
- bammarkduplicates
- bammarkduplicates2
- bammarkduplicatesopt
- bammaskflags
- bammdnm
- bammerge
- bamnumericalindex
- bamnumericalindexstats
- bamrank
- bamranksort
- bamrecalculatecigar
- bamrecompress
- bamrefextract
- bamrefinterval
- bamreheader
- bamreplacechecksums
- bamreset
- bamscrapcount
- bamseqchksum
- bamsormadup
- bamsort
- bamsplit
- bamsplitdiv
- bamstreamingmarkduplicates
- bamtofastq
- bamvalidate
- bamzztoname
Link to section 'Module' of 'biobambam' Module
You can load the modules by:
module load biocontainers
module load biobambam
Link to section 'Example job' of 'biobambam' Example job
Using #!/bin/sh -l
as shebang in the slurm job script will cause the failure of some biocontainer modules. Please use #!/bin/bash
instead.
To run Biobambam on our clusters:
#!/bin/bash
#SBATCH -A myallocation # Allocation name
#SBATCH -t 1:00:00
#SBATCH -N 1
#SBATCH -n 8
#SBATCH --job-name=biobambam
#SBATCH --mail-type=FAIL,BEGIN,END
#SBATCH --error=%x-%J-%u.err
#SBATCH --output=%x-%J-%u.out
module --force purge
ml biocontainers biobambam
bammarkduplicates I=Aligned.sortedByCoord.out.bam O=out.bam D=duplcate_out
bamsort I=Aligned.sortedByCoord.out.bam O=sorted.bam sortthreads=8
bamtofastq filename=Aligned.sortedByCoord.out.bam outputdir=fastq_out
bioconvert
Link to section 'Introduction' of 'bioconvert' Introduction
Bioconvert
is a collaborative project to facilitate the interconversion of life science data from one format to another.
For more information, please check its website: https://biocontainers.pro/tools/bioconvert.
Link to section 'Versions' of 'bioconvert' Versions
- 0.4.3
- 0.5.2
- 0.6.1
- 0.6.2
Link to section 'Commands' of 'bioconvert' Commands
- bioconvert
Link to section 'Module' of 'bioconvert' Module
You can load the modules by:
module load biocontainers
module load bioconvert
Link to section 'Example job' of 'bioconvert' Example job
Using #!/bin/sh -l
as shebang in the slurm job script will cause the failure of some biocontainer modules. Please use #!/bin/bash
instead.
To run Bioconvert on our clusters:
#!/bin/bash
#SBATCH -A myallocation # Allocation name
#SBATCH -t 1:00:00
#SBATCH -N 1
#SBATCH -n 1
#SBATCH --job-name=bioconvert
#SBATCH --mail-type=FAIL,BEGIN,END
#SBATCH --error=%x-%J-%u.err
#SBATCH --output=%x-%J-%u.out
module --force purge
ml biocontainers bioconvert
bioconvert fastq2fasta input.fastq output.fa
biopython
Link to section 'Introduction' of 'biopython' Introduction
Biopython
is a set of freely available tools for biological computation written in Python.
For more information, please check its website: https://biocontainers.pro/tools/biopython and its home page: https://biopython.org.
Link to section 'Versions' of 'biopython' Versions
- 1.70-np112py27
- 1.70-np112py36
- 1.78
Link to section 'Commands' of 'biopython' Commands
- easy_install
- f2py
- f2py3
- idle3
- pip
- pip3
- pydoc
- pydoc3
- python
- python3
- python3-config
- python3.9
- python3.9-config
- wheel
Link to section 'Module' of 'biopython' Module
You can load the modules by:
module load biocontainers
module load biopython
Link to section 'Interactive job' of 'biopython' Interactive job
To run biopython interactively on our clusters:
(base) UserID@bell-fe00:~ $ sinteractive -N1 -n12 -t4:00:00 -A myallocation
salloc: Granted job allocation 12345869
salloc: Waiting for resource configuration
salloc: Nodes bell-a008 are ready for job
(base) UserID@bell-a008:~ $ module load biocontainers biopython
(base) UserID@bell-a008:~ $ python
Python 3.9.1 | packaged by conda-forge | (default, Jan 26 2021, 01:34:10)
[GCC 9.3.0] on linux
Type "help", "copyright", "credits" or "license" for more information.
>>> from Bio import SeqIO
>>> with open("input.gb") as input_handle:
for record in SeqIO.parse(input_handle, "genbank"):
print(record)
Link to section 'Batch job' of 'biopython' Batch job
Using #!/bin/sh -l
as shebang in the slurm job script will cause the failure of some biocontainer modules. Please use #!/bin/bash
instead.
To run Biopython on our clusters:
#!/bin/bash
#SBATCH -A myallocation # Allocation name
#SBATCH -t 1:00:00
#SBATCH -N 1
#SBATCH -n 1
#SBATCH --job-name=biopython
#SBATCH --mail-type=FAIL,BEGIN,END
#SBATCH --error=%x-%J-%u.err
#SBATCH --output=%x-%J-%u.out
module --force purge
ml biocontainers biopython
python script.py
bismark
Link to section 'Introduction' of 'bismark' Introduction
Bismark
is a tool to map bisulfite treated sequencing reads to a genome of interest and perform methylation calls in a single step.
For more information, please check its website: https://biocontainers.pro/tools/bismark and its home page on Github.
Link to section 'Versions' of 'bismark' Versions
- 0.23.0
Link to section 'Commands' of 'bismark' Commands
- bismark
- bam2nuc
- bismark2bedGraph
- bismark2report
- bismark2summary
- bismark_genome_preparation
- bismark_methylation_extractor
- copy_bismark_files_for_release.pl
- coverage2cytosine
- deduplicate_bismark
- filter_non_conversion
- methylation_consistency
Link to section 'Dependencies' of 'bismark' Dependencies
Bowtie v2.4.2
, Samtools v1.12
, HISAT2 v2.2.1
were included in the container image. So users do not need to provide the dependency path in the bismark parameter.
Link to section 'Module' of 'bismark' Module
You can load the modules by:
module load biocontainers
module load bismark
Link to section 'Example job' of 'bismark' Example job
Using #!/bin/sh -l
as shebang in the slurm job script will cause the failure of some biocontainer modules. Please use #!/bin/bash
instead.
To run Bismark on our clusters:
#!/bin/bash
#SBATCH -A myallocation # Allocation name
#SBATCH -t 1:00:00
#SBATCH -N 1
#SBATCH -n 12
#SBATCH --job-name=bismark
#SBATCH --mail-type=FAIL,BEGIN,END
#SBATCH --error=%x-%J-%u.err
#SBATCH --output=%x-%J-%u.out
module --force purge
ml biocontainers bismark
bismark_genome_preparation --bowtie2 data/ref_genome
bismark --multicore 12 --genome data/ref_genome seq.fastq
blasr
Link to section 'Introduction' of 'blasr' Introduction
Blasr
Blasr is a read mapping program that maps reads to positions in a genome by clustering short exact matches between the read and the genome, and scoring clusters using alignment.
For more information, please check its website: https://biocontainers.pro/tools/blasr.
Link to section 'Versions' of 'blasr' Versions
- 5.3.5
Link to section 'Commands' of 'blasr' Commands
- blasr
Link to section 'Module' of 'blasr' Module
You can load the modules by:
module load biocontainers
module load blasr
Link to section 'Example job' of 'blasr' Example job
Using #!/bin/sh -l
as shebang in the slurm job script will cause the failure of some biocontainer modules. Please use #!/bin/bash
instead.
To run Blasr on our clusters:
#!/bin/bash
#SBATCH -A myallocation # Allocation name
#SBATCH -t 1:00:00
#SBATCH -N 1
#SBATCH -n 1
#SBATCH --job-name=blasr
#SBATCH --mail-type=FAIL,BEGIN,END
#SBATCH --error=%x-%J-%u.err
#SBATCH --output=%x-%J-%u.out
module --force purge
ml biocontainers blasr
blasr reads.bas.h5 ecoli_K12.fasta -sam
blast
Link to section 'Introduction' of 'blast' Introduction
BLAST
(Basic Local Alignment Search Tool) finds regions of similarity between biological sequences. The program compares nucleotide or protein sequences to sequence databases and calculates the statistical significance.
For more information, please check its website: https://biocontainers.pro/tools/blast and its home page: https://blast.ncbi.nlm.nih.gov/Blast.cgi?CMD=Web&PAGE_TYPE=BlastHome.
Link to section 'Versions' of 'blast' Versions
- 2.11.0
- 2.13.0
Link to section 'Commands' of 'blast' Commands
- blastn
- blastp
- blastx
- blast_formatter
- amino-acid-composition
- between-two-genes
- blastdbcheck
- blastdbcmd
- blastdb_aliastool
- cleanup-blastdb-volumes.py
- deltablast
- dustmasker
- eaddress
- eblast
- get_species_taxids.sh
- legacy_blast.pl
- makeblastdb
- makembindex
- makeprofiledb
- psiblast
- rpsblast
- rpstblastn
- run-ncbi-converter
- segmasker
- tblastn
- tblastx
- update_blastdb.pl
- windowmasker
Link to section 'Module' of 'blast' Module
You can load the modules by:
module load biocontainers
module load blast
Link to section 'BLAST Databases' of 'blast' BLAST Databases
Local copies of the blast dabase can be found in the directory /depot/itap/datasets/blast/latest/. The environment varialbe BLASTDB
was also set as /depot/itap/datasets/blast/latest/
. If users want to use cdd_delta
, env_nr
, env_nt
, nr
, nt
, pataa
, patnt
, pdbnt
, refseq_protein
, refseq_rna
, swissprot
, or tsa_nt
databases, do not need to provide the database path. Instead, just use the format like this -db nr
.
Link to section 'Example job' of 'blast' Example job
Using #!/bin/sh -l
as shebang in the slurm job script will cause the failure of some biocontainer modules. Please use #!/bin/bash
instead.
To run BLAST on our clusters:
#!/bin/bash
#SBATCH -A myallocation # Allocation name
#SBATCH -t 1:00:00
#SBATCH -N 1
#SBATCH -n 1
#SBATCH --job-name=blast
#SBATCH --mail-type=FAIL,BEGIN,END
#SBATCH --error=%x-%J-%u.err
#SBATCH --output=%x-%J-%u.out
module --force purge
ml biocontainers blast
blastp -query protein.fasta -db nr -out test_out -num_threads 4
blobtools
BlobTools
is a modular command-line solution for visualisation, quality control and taxonomic partitioning of genome datasets.
Detailed usage can be found here: https://github.com/DRL/blobtools
Link to section 'Versions' of 'blobtools' Versions
- 1.1.1
Link to section 'Commands' of 'blobtools' Commands
- blobtools
Link to section 'Module' of 'blobtools' Module
You can load the modules by:
module load biocontainers
module load blobtools/1.1.1
Link to section 'Example job' of 'blobtools' Example job
Using #!/bin/sh -l
as shebang in the slurm job script will cause the failure of some biocontainer modules. Please use #!/bin/bash
instead.
To run blobtools on our our clusters:
#!/bin/bash
#SBATCH -A myallocation # Allocation name
#SBATCH -t 1:00:00
#SBATCH -N 1
#SBATCH -n 4
#SBATCH --job-name=blobtools
#SBATCH --mail-type=FAIL,BEGIN,END
#SBATCH --error=%x-%J-%u.err
#SBATCH --output=%x-%J-%u.out
module --force purge
ml biocontainers blobtools/1.1.1
blobtools create -i example/assembly.fna -b example/mapping_1.sorted.bam -t example/blast.out -o test && \
blobtools view -i test.blobDB.json && \
blobtools plot -i test.blobDB.json
bmge
Link to section 'Introduction' of 'bmge' Introduction
Bmge
is a program that selects regions in a multiple sequence alignment that are suited for phylogenetic inference.
For more information, please check its website: https://biocontainers.pro/tools/bmge and its home page: https://bioweb.pasteur.fr/packages/pack@BMGE@1.12.
Link to section 'Versions' of 'bmge' Versions
- 1.12
Link to section 'Commands' of 'bmge' Commands
- bmge
Link to section 'Module' of 'bmge' Module
You can load the modules by:
module load biocontainers
module load bmge
Link to section 'Example job' of 'bmge' Example job
Using #!/bin/sh -l
as shebang in the slurm job script will cause the failure of some biocontainer modules. Please use #!/bin/bash
instead.
To run Bmge on our clusters:
#!/bin/bash
#SBATCH -A myallocation # Allocation name
#SBATCH -t 1:00:00
#SBATCH -N 1
#SBATCH -n 1
#SBATCH --job-name=bmge
#SBATCH --mail-type=FAIL,BEGIN,END
#SBATCH --error=%x-%J-%u.err
#SBATCH --output=%x-%J-%u.out
module --force purge
ml biocontainers bmge
bmge -i seq.fa -t AA -o out.phy
bowtie
Link to section 'Introduction' of 'bowtie' Introduction
Bowtie
is an ultrafast, memory-efficient short read aligner. It aligns short DNA sequences (reads) to the human genome at a rate of over 25 million 35-bp reads per hour. Bowtie indexes the genome with a Burrows-Wheeler index to keep its memory footprint small: typically about 2.2 GB for the human genome (2.9 GB for paired-end).
For more information, please check its website: https://biocontainers.pro/tools/bowtie and its home page: http://bowtie-bio.sourceforge.net/.
Link to section 'Versions' of 'bowtie' Versions
- 1.3.1
Link to section 'Commands' of 'bowtie' Commands
- bowtie
- bowtie-build
- bowtie-inspect
Link to section 'Module' of 'bowtie' Module
You can load the modules by:
module load biocontainers
module load bowtie
Link to section 'Example job' of 'bowtie' Example job
Using #!/bin/sh -l
as shebang in the slurm job script will cause the failure of some biocontainer modules. Please use #!/bin/bash
instead.
To run Bowtie on our clusters:
#!/bin/bash
#SBATCH -A myallocation # Allocation name
#SBATCH -t 1:00:00
#SBATCH -N 1
#SBATCH -n 1
#SBATCH --job-name=bowtie
#SBATCH --mail-type=FAIL,BEGIN,END
#SBATCH --error=%x-%J-%u.err
#SBATCH --output=%x-%J-%u.out
module --force purge
ml biocontainers bowtie
bowtie-build ref.fasta ref
bowtie -p 4 -x ref -1 input_1.fq -2 input_2.fq -S test.sam
bowtie2
Link to section 'Introduction' of 'bowtie2' Introduction
Bowtie 2
is an ultrafast and memory-efficient tool for aligning sequencing reads to long reference sequences. It is particularly good at aligning reads of about 50 up to 100s or 1,000s of characters, and particularly good at aligning to relatively long (e.g. mammalian) genomes. Bowtie 2 indexes the genome with an FM Index to keep its memory footprint small: for the human genome, its memory footprint is typically around 3.2 GB. Bowtie 2 supports gapped, local, and paired-end alignment modes.
For more information, please check its website: https://biocontainers.pro/tools/bowtie2 and its home page on Github.
Link to section 'Versions' of 'bowtie2' Versions
- 2.4.2
- 2.5.1
Link to section 'Commands' of 'bowtie2' Commands
- bowtie2
- bowtie2-build
- bowtie2-inspect
Link to section 'Module' of 'bowtie2' Module
You can load the modules by:
module load biocontainers
module load bowtie2
Link to section 'Example job' of 'bowtie2' Example job
Using #!/bin/sh -l
as shebang in the slurm job script will cause the failure of some biocontainer modules. Please use #!/bin/bash
instead.
To run Bowtie 2 on our clusters:
#!/bin/bash
#SBATCH -A myallocation # Allocation name
#SBATCH -t 1:00:00
#SBATCH -N 1
#SBATCH -n 1
#SBATCH --job-name=bowtie2
#SBATCH --mail-type=FAIL,BEGIN,END
#SBATCH --error=%x-%J-%u.err
#SBATCH --output=%x-%J-%u.out
module --force purge
ml biocontainers bowtie2
bowtie2-build ref.fasta ref
bowtie2 -p 4 -x ref -1 input_1.fq -2 input_2.fq -S test.sam
bracken
Bracken
(Bayesian Reestimation of Abundance with KrakEN) is a highly accurate statistical method that computes the abundance of species in DNA sequences from a metagenomics sample.
Detailed usage can be found here: https://github.com/jenniferlu717/Bracken
Inside the bracken
container image, kraken2
was also installed. As a result, when you load bracken/2.6.1-py37
, kraken version 2.1.1
will be automatically loaded. Please do not load kraken2
module together with bracken
module to avoid conflict.
Link to section 'Versions' of 'bracken' Versions
- 2.6.1
- 2.7
Link to section 'Commands' of 'bracken' Commands
- bracken
- bracken-build
- combine_bracken_outputs.py
- kraken2
- kraken2-build
- kraken2-inspect
- combine_bracken_outputs.py
- est_abundance.py
- generate_kmer_distribution.py
Link to section 'Module' of 'bracken' Module
You can load the modules by:
module load biocontainers
module load bracken/2.6.1-py37
Link to section 'Example job' of 'bracken' Example job
Using #!/bin/sh -l
as shebang in the slurm job script will cause the failure of some biocontainer modules. Please use #!/bin/bash
instead.
To run bracken on our our clusters:
#!/bin/bash
#SBATCH -A myallocation # Allocation name
#SBATCH -t 10:00:00
#SBATCH -N 1
#SBATCH -n 24
#SBATCH --job-name=bracken
#SBATCH --mail-type=FAIL,BEGIN,END
#SBATCH --error=%x-%J-%u.err
#SBATCH --output=%x-%J-%u.out
module --force purge
ml biocontainers bracken/2.6.1-py37
DATABASE=minikraken2_v2_8GB_201904_UPDATE
kraken2 --threads 24 --report kranken2.report --db $DATABASE --paired --classified-out cseqs#.fq SRR5043021_1.fastq SRR5043021_2.fastq
bracken -d $DATABASE -i kranken2.report -o bracken_output -w bracken.report
braker2
Link to section 'Introduction' of 'braker2' Introduction
BRAKER
is a pipeline for fully automated prediction of protein coding gene structures with GeneMark-ES/ET and AUGUSTUS in novel eukaryotic genomes.
For more information. please check its github repository https://github.com/Gaius-Augustus/BRAKER.
Link to section 'Versions' of 'braker2' Versions
- 2.1.6
Commands
braker.pl
Helper command
Since BRAKER
is a pipeline that trains AUGUSTUS
, i.e. writes species specific parameter files, BRAKER needs writing access to the configuration directory of AUGUSTUS that contains such files. This installation comes with a stub of AUGUSTUS configuration files, but you must
copy them out from the container into a location where you have write permissions.
A helper command copy_augustus_config
is provided to simplify the task. Follow the procedure below to put the config files in your scratch space:
$ mkdir -p $RCAC_SCRATCH/augustus
$ copy_augustus_config $RCAC_SCRATCH/augustus
$ export AUGUSTUS_CONFIG_PATH=$RCAC_SCRATCH/augustus/config
Link to section 'Module' of 'braker2' Module
You can load the modules by:
module load biocontainers
module load braker2/2.1.6
Link to section 'Example job' of 'braker2' Example job
Using #!/bin/sh -l
as shebang in the slurm job script will cause the failure of some biocontainer modules. Please use #!/bin/bash
instead.
To run BRAKER on our cluster:
#!/bin/bash
#SBATCH -A myallocation # Allocation name
#SBATCH -t 10:00:00
#SBATCH -N 1
#SBATCH -n 24
#SBATCH --job-name=BRAKER2
#SBATCH --mail-type=FAIL,BEGIN,END
#SBATCH --error=%x-%J-%u.err
#SBATCH --output=%x-%J-%u.out
module --force purge
ml biocontainers braker2/2.1.6
# The augustus config step is only required for the first time to use BRAKER2
mkdir -p $RCAC_SCRATCH/augustus
copy_augustus_config $RCAC_SCRATCH/augustus
export AUGUSTUS_CONFIG_PATH=$RCAC_SCRATCH/augustus/config
braker.pl --genome genome.fa --bam RNAseq.bam --softmasking --cores 24
brass
Link to section 'Introduction' of 'brass' Introduction
Brass
is used to analyze one or more related BAM files of paired-end sequencing to determine potential rearrangement breakpoints.
For more information, please check its website: https://quay.io/repository/wtsicgp/brass and its home page on Github.
Link to section 'Versions' of 'brass' Versions
- 6.3.4
Link to section 'Commands' of 'brass' Commands
- brass-assemble
- brass_bedpe2vcf.pl
- brass_foldback_reads.pl
- brass-group
- brassI_filter.pl
- brassI_np_in.pl
- brassI_pre_filter.pl
- brassI_prep_bam.pl
- brass.pl
Link to section 'Module' of 'brass' Module
You can load the modules by:
module load biocontainers
module load brass
Link to section 'Example job' of 'brass' Example job
Using #!/bin/sh -l
as shebang in the slurm job script will cause the failure of some biocontainer modules. Please use #!/bin/bash
instead.
To run Brass on our clusters:
#!/bin/bash
#SBATCH -A myallocation # Allocation name
#SBATCH -t 1:00:00
#SBATCH -N 1
#SBATCH -n 4
#SBATCH --job-name=brass
#SBATCH --mail-type=FAIL,BEGIN,END
#SBATCH --error=%x-%J-%u.err
#SBATCH --output=%x-%J-%u.out
module --force purge
ml biocontainers brass
brass.pl -c 4 -o myout -t tumour.bam -n normal.bam
breseq
Link to section 'Introduction' of 'breseq' Introduction
Breseq
is a computational pipeline for the analysis of short-read re-sequencing data.
For more information, please check its website: https://biocontainers.pro/tools/breseq and its home page on Github.
Link to section 'Versions' of 'breseq' Versions
- 0.36.1
Link to section 'Commands' of 'breseq' Commands
- breseq
Link to section 'Module' of 'breseq' Module
You can load the modules by:
module load biocontainers
module load breseq
Link to section 'Example job' of 'breseq' Example job
Using #!/bin/sh -l
as shebang in the slurm job script will cause the failure of some biocontainer modules. Please use #!/bin/bash
instead.
To run Breseq on our clusters:
#!/bin/bash
#SBATCH -A myallocation # Allocation name
#SBATCH -t 1:00:00
#SBATCH -N 1
#SBATCH -n 1
#SBATCH --job-name=breseq
#SBATCH --mail-type=FAIL,BEGIN,END
#SBATCH --error=%x-%J-%u.err
#SBATCH --output=%x-%J-%u.out
module --force purge
ml biocontainers breseq
busco
Link to section 'Introduction' of 'busco' Introduction
BUSCO
(Benchmarking sets of Universal Single-Copy Orthologs) provides measures for quantitative assessment of genome assembly, gene set, and transcriptome completeness based on evolutionarily informed expectations of gene content from near-universal single-copy orthologs.
Detailed information can be found here: https://gitlab.com/ezlab/busco/
Link to section 'Versions' of 'busco' Versions
- 5.2.2
- 5.3.0
- 5.4.1
- 5.4.3
- 5.4.4
- 5.4.5
- 5.4.7
Commands
- busco - generate_plot.py
Helper command
Augustus is a gene prediction program for eukaryotes which is required by BUSCO. Augustus requires a writable configuration directory. This installation comes with a stub of AUGUSTUS configuration files, but you must
copy them out from the container into a location where you have write permissions.
A helper command copy_augustus_config
is provided to simplify the task. Follow the procedure below to put the config files in your scratch space:
$ mkdir -p $RCAC_SCRATCH/augustus
$ copy_augustus_config $RCAC_SCRATCH/augustus
$ export AUGUSTUS_CONFIG_PATH=$RCAC_SCRATCH/augustus/config
Link to section 'Module' of 'busco' Module
You can load the modules by:
module load biocontainers
module load busco
Link to section 'Example job for prokaryotic genomes' of 'busco' Example job for prokaryotic genomes
Using #!/bin/sh -l
as shebang in the slurm job script will cause the failure of some biocontainer modules. Please use #!/bin/bash
instead.
To run BUSCO on our cluster:
#!/bin/bash
#SBATCH -A myallocation # Allocation name
#SBATCH -t 1:00:00
#SBATCH -N 1
#SBATCH -n 12
#SBATCH --job-name=BUSCO
#SBATCH --mail-type=FAIL,BEGIN,END
#SBATCH --error=%x-%J-%u.err
#SBATCH --output=%x-%J-%u.out
module --force purge
ml biocontainers busco
## Print the full lineage datasets, and find the dataset fitting your organism.
busco --list-datasets
## run the evaluation
busco -f -c 12 -l actinobacteria_class_odb10 -i bacteria_genome.fasta -o busco_out -m genome
## generate a simple summary plot
generate_plot.py -wd busco_out
Link to section 'Example job for eukaryotic genomes' of 'busco' Example job for eukaryotic genomes
Using #!/bin/sh -l
as shebang in the slurm job script will cause the failure of some biocontainer modules. Please use #!/bin/bash
instead.
To run BUSCO on our cluster:
#!/bin/bash
#SBATCH -A myallocation # Allocation name
#SBATCH -t 1:00:00
#SBATCH -N 1
#SBATCH -n 12
#SBATCH --job-name=BUSCO
#SBATCH --mail-type=FAIL,BEGIN,END
#SBATCH --error=%x-%J-%u.err
#SBATCH --output=%x-%J-%u.out
module --force purge
ml biocontainers busco
## The augustus config step is only required for the first time to use BUSCO
mkdir -p $RCAC_SCRATCH/augustus
copy_augustus_config $RCAC_SCRATCH/augustus
## This is required for eukaryotic genomes
export AUGUSTUS_CONFIG_PATH=$RCAC_SCRATCH/augustus/config
## Print the full lineage datasets, and find the dataset fitting your organism.
busco --list-datasets
## run the evaluation
busco -f -c 12 -l fungi_odb10 -i fungi_protein.fasta -o busco_out_protein -m protein
busco -f -c 12 --augustus -l fungi_odb10 -i fungi_genome.fasta -o busco_out_genome -m genome
## generate a simple summary plot
generate_plot.py -wd busco_out_protein
generate_plot.py -wd busco_out_genome
bustools
Link to section 'Introduction' of 'bustools' Introduction
Bustools
is a program for manipulating BUS files for single cell RNA-Seq datasets.
For more information, please check its website: https://biocontainers.pro/tools/bustools and its home page on Github.
Link to section 'Versions' of 'bustools' Versions
- 0.41.0
Link to section 'Commands' of 'bustools' Commands
- bustools
Link to section 'Module' of 'bustools' Module
You can load the modules by:
module load biocontainers
module load bustools
Link to section 'Example job' of 'bustools' Example job
Using #!/bin/sh -l
as shebang in the slurm job script will cause the failure of some biocontainer modules. Please use #!/bin/bash
instead.
To run Bustools on our clusters:
#!/bin/bash
#SBATCH -A myallocation # Allocation name
#SBATCH -t 1:00:00
#SBATCH -N 1
#SBATCH -n 1
#SBATCH --job-name=bustools
#SBATCH --mail-type=FAIL,BEGIN,END
#SBATCH --error=%x-%J-%u.err
#SBATCH --output=%x-%J-%u.out
module --force purge
ml biocontainers bustools
bustools capture -s -o cDNA_capture.bus -c cDNA_transcripts.to_capture.txt -e matrix.ec -t transcripts.txt output.correct.sort.bus
bustools count -o u -g cDNA_introns_t2g.txt -e matrix.ec -t transcripts.txt --genecounts cDNA_capture.bus
bwa
Link to section 'Introduction' of 'bwa' Introduction
BWA
(Burrows-Wheeler Aligner) is a fast, accurate, memory-efficient aligner for short and long sequencing reads.
For more information, please check its website: https://biocontainers.pro/tools/bwa and its home page: http://bio-bwa.sourceforge.net.
Link to section 'Versions' of 'bwa' Versions
- 0.7.17
Link to section 'Commands' of 'bwa' Commands
- bwa
- qualfa2fq.pl
- xa2multi.pl
Link to section 'Module' of 'bwa' Module
You can load the modules by:
module load biocontainers
module load bwa
Link to section 'Example job' of 'bwa' Example job
Using #!/bin/sh -l
as shebang in the slurm job script will cause the failure of some biocontainer modules. Please use #!/bin/bash
instead.
To run BWA on our clusters:
#!/bin/bash
#SBATCH -A myallocation # Allocation name
#SBATCH -t 1:00:00
#SBATCH -N 1
#SBATCH -n 1
#SBATCH --job-name=bwa
#SBATCH --mail-type=FAIL,BEGIN,END
#SBATCH --error=%x-%J-%u.err
#SBATCH --output=%x-%J-%u.out
module --force purge
ml biocontainers bwa
bwa index ref.fasta
bwa mem ref.fasta input.fq > test.sam
bwameth
Link to section 'Introduction' of 'bwameth' Introduction
Bwameth is a tool for fast and accurante alignment of BS-Seq reads.
BioContainers: https://biocontainers.pro/tools/bwameth
Home page: https://github.com/brentp/bwa-meth
Link to section 'Versions' of 'bwameth' Versions
- 0.2.5
Link to section 'Commands' of 'bwameth' Commands
- bwameth.py
Link to section 'Module' of 'bwameth' Module
You can load the modules by:
module load biocontainers
module load bwameth
Link to section 'Example job' of 'bwameth' Example job
Using #!/bin/sh -l
as shebang in the slurm job script will cause the failure of some biocontainer modules. Please use #!/bin/bash
instead.
To run bwameth on our clusters:
#!/bin/bash
#SBATCH -A myallocation # Allocation name
#SBATCH -t 1:00:00
#SBATCH -N 1
#SBATCH -n 1
#SBATCH --job-name=bwameth
#SBATCH --mail-type=FAIL,BEGIN,END
#SBATCH --error=%x-%J-%u.err
#SBATCH --output=%x-%J-%u.out
module --force purge
ml biocontainers bwameth
cactus
Link to section 'Introduction' of 'cactus' Introduction
Cactus
is a reference-free whole-genome multiple alignment program.
For more information, please check its website: https://biocontainers.pro/tools/cactus and its home page on Github.
Link to section 'Versions' of 'cactus' Versions
- 2.0.5
- 2.2.1
- 2.2.3-gpu
- 2.2.3
- 2.4.0-gpu
- 2.4.0
- 2.5.2
- 2.6.5
Link to section 'Commands' of 'cactus' Commands
- cactus
- cactus-align
- cactus-align-batch
- cactus-blast
- cactus-graphmap
- cactus-graphmap-join
- cactus-graphmap-split
- cactus-minigraph
- cactus-prepare
- cactus-prepare-toil
- cactus-preprocess
- cactus-refmap
- cactus2hal-stitch.sh
- cactus2hal.py
- cactusAPITests
- cactus_analyseAssembly
- cactus_barTests
- cactus_batch_mergeChunks
- cactus_chain
- cactus_consolidated
- cactus_covered_intervals
- cactus_fasta_fragments.py
- cactus_fasta_softmask_intervals.py
- cactus_filterSmallFastaSequences.py
- cactus_halGeneratorTests
- cactus_local_alignment.py
- cactus_makeAlphaNumericHeaders.py
- cactus_softmask2hardmask
Link to section 'Module' of 'cactus' Module
You can load the modules by:
module load biocontainers
module load cactus
Link to section 'Example job' of 'cactus' Example job
Using #!/bin/sh -l
as shebang in the slurm job script will cause the failure of some biocontainer modules. Please use #!/bin/bash
instead.
To run Cactus on our clusters:
#!/bin/bash
#SBATCH -A myallocation # Allocation name
#SBATCH -t 1:00:00
#SBATCH -N 1
#SBATCH -n 1
#SBATCH --job-name=cactus
#SBATCH --mail-type=FAIL,BEGIN,END
#SBATCH --error=%x-%J-%u.err
#SBATCH --output=%x-%J-%u.out
module --force purge
ml biocontainers cactus
wget https://raw.githubusercontent.com/ComparativeGenomicsToolkit/cactus/master/examples/evolverMammals.txt
cactus jobStore evolverMammals.txt evolverMammals.hal
cafe
Link to section 'Introduction' of 'cafe' Introduction
Cafe
is a computational tool for the study of gene family evolution.
For more information, please check its website: https://biocontainers.pro/tools/cafe and its home page on Github.
Link to section 'Versions' of 'cafe' Versions
- 4.2.1
- 5.0.0
Link to section 'Commands' of 'cafe' Commands
- cafe
Link to section 'Module' of 'cafe' Module
You can load the modules by:
module load biocontainers
module load cafe
Link to section 'Example job' of 'cafe' Example job
Using #!/bin/sh -l
as shebang in the slurm job script will cause the failure of some biocontainer modules. Please use #!/bin/bash
instead.
To run Cafe on our clusters:
#!/bin/bash
#SBATCH -A myallocation # Allocation name
#SBATCH -t 1:00:00
#SBATCH -N 1
#SBATCH -n 1
#SBATCH --job-name=cafe
#SBATCH --mail-type=FAIL,BEGIN,END
#SBATCH --error=%x-%J-%u.err
#SBATCH --output=%x-%J-%u.out
module --force purge
ml biocontainers cafe
#To get a list of commands just call CAFE with the -h or --help arguments
cafe5 -h
#To estimate lambda with no among family rate variation issue the command
cafe5 -i mammal_gene_families.txt -t mammal_tree.txt
canu
Canu
is a single molecule sequence assembler for genomes large and small.
Detailed usage can be found here: https://github.com/marbl/canu
Link to section 'Versions' of 'canu' Versions
- 2.1.1
- 2.2
Link to section 'Commands' of 'canu' Commands
- canu
Link to section 'Module' of 'canu' Module
You can load the modules by:
module load biocontainers
module load canu/2.2
Link to section 'Example job' of 'canu' Example job
Using #!/bin/sh -l
as shebang in the slurm job script will cause the failure of some biocontainer modules. Please use #!/bin/bash
instead.
To run canu on our our clusters:
#!/bin/bash
#SBATCH -A myallocation # Allocation name
#SBATCH -t 20:00:00
#SBATCH -N 1
#SBATCH -n 24
#SBATCH --job-name=canu
#SBATCH --mail-type=FAIL,BEGIN,END
#SBATCH --error=%x-%J-%u.err
#SBATCH --output=%x-%J-%u.out
module --force purge
ml biocontainers canu/2.2
canu -p Cm -d clavibacter_pacbio genomeSize=3.4m -pacbio *.fastq
ccs
Link to section 'Introduction' of 'ccs' Introduction
Pbccs is a tool to generate Highly Accurate Single-Molecule Consensus Reads (HiFi Reads).
BioContainers: https://biocontainers.pro/tools/pbccs
Home page: https://github.com/PacificBiosciences/ccs
Link to section 'Versions' of 'ccs' Versions
- 6.4.0
Link to section 'Commands' of 'ccs' Commands
- ccs
Link to section 'Module' of 'ccs' Module
You can load the modules by:
module load biocontainers
module load ccs
Link to section 'Example job' of 'ccs' Example job
Using #!/bin/sh -l
as shebang in the slurm job script will cause the failure of some biocontainer modules. Please use #!/bin/bash
instead.
To run ccs on our clusters:
#!/bin/bash
#SBATCH -A myallocation # Allocation name
#SBATCH -t 1:00:00
#SBATCH -N 1
#SBATCH -n 1
#SBATCH --job-name=ccs
#SBATCH --mail-type=FAIL,BEGIN,END
#SBATCH --error=%x-%J-%u.err
#SBATCH --output=%x-%J-%u.out
module --force purge
ml biocontainers ccs
ccs --all subreads.bam ccs.bam
cd-hit
Link to section 'Introduction' of 'cd-hit' Introduction
Cd-hit
is a very widely used program for clustering and comparing protein or nucleotide sequences.
For more information, please check its website: https://biocontainers.pro/tools/cd-hit and its home page on Github.
Link to section 'Versions' of 'cd-hit' Versions
- 4.8.1
Link to section 'Commands' of 'cd-hit' Commands
- FET.pl
- cd-hit
- cd-hit-2d
- cd-hit-2d-para.pl
- cd-hit-454
- cd-hit-clstr_2_blm8.pl
- cd-hit-div
- cd-hit-div.pl
- cd-hit-est
- cd-hit-est-2d
- cd-hit-para.pl
- clstr2tree.pl
- clstr2txt.pl
- clstr2xml.pl
- clstr_cut.pl
- clstr_list.pl
- clstr_list_sort.pl
- clstr_merge.pl
- clstr_merge_noorder.pl
- clstr_quality_eval.pl
- clstr_quality_eval_by_link.pl
- clstr_reduce.pl
- clstr_renumber.pl
- clstr_rep.pl
- clstr_reps_faa_rev.pl
- clstr_rev.pl
- clstr_select.pl
- clstr_select_rep.pl
- clstr_size_histogram.pl
- clstr_size_stat.pl
- clstr_sort_by.pl
- clstr_sort_prot_by.pl
- clstr_sql_tbl.pl
- clstr_sql_tbl_sort.pl
- make_multi_seq.pl
- plot_2d.pl
- plot_len1.pl
Link to section 'Module' of 'cd-hit' Module
You can load the modules by:
module load biocontainers
module load cd-hit
Link to section 'Example job' of 'cd-hit' Example job
Using #!/bin/sh -l
as shebang in the slurm job script will cause the failure of some biocontainer modules. Please use #!/bin/bash
instead.
To run Cd-hit on our clusters:
#!/bin/bash
#SBATCH -A myallocation # Allocation name
#SBATCH -t 1:00:00
#SBATCH -N 1
#SBATCH -n 1
#SBATCH --job-name=cd-hit
#SBATCH --mail-type=FAIL,BEGIN,END
#SBATCH --error=%x-%J-%u.err
#SBATCH --output=%x-%J-%u.out
module --force purge
ml biocontainers cd-hit
cd-hit -i Cm_pep.fasta -o Cmdb90 -c 0.9 -n 5 -M 16000 -T 8
cd-hit-est -i Cm_dna.fasta -o Cmdb90_nt -c 0.9 -n 5 -M 16000 -T 8
cdbtools
Link to section 'Introduction' of 'cdbtools' Introduction
Cdbtools
is a collection of tools used for creating indices for quick retrieval of any particular sequences from large multi-FASTA files.
For more information, please check its website: https://biocontainers.pro/tools/cdbtools.
Link to section 'Versions' of 'cdbtools' Versions
- 0.99
Link to section 'Commands' of 'cdbtools' Commands
- cdbfasta
- cdbyank
Link to section 'Module' of 'cdbtools' Module
You can load the modules by:
module load biocontainers
module load cdbtools
Link to section 'Example job' of 'cdbtools' Example job
Using #!/bin/sh -l
as shebang in the slurm job script will cause the failure of some biocontainer modules. Please use #!/bin/bash
instead.
To run Cdbtools on our clusters:
#!/bin/bash
#SBATCH -A myallocation # Allocation name
#SBATCH -t 1:00:00
#SBATCH -N 1
#SBATCH -n 1
#SBATCH --job-name=cdbtools
#SBATCH --mail-type=FAIL,BEGIN,END
#SBATCH --error=%x-%J-%u.err
#SBATCH --output=%x-%J-%u.out
module --force purge
ml biocontainers cdbtools
cdbfasta genome.fa
cdbyank -a 'seq_1' genome.fa.cidx
cegma
Link to section 'Introduction' of 'cegma' Introduction
CEGMA (Core Eukaryotic Genes Mapping Approach) is a pipeline for building a set of high reliable set of gene annotations in virtually any eukaryotic genome.
Docker hub: https://hub.docker.com/r/chrishah/cegma
Home page: https://github.com/KorfLab/CEGMA_v2
Link to section 'Versions' of 'cegma' Versions
- 2.5
Link to section 'Commands' of 'cegma' Commands
- cegma
Link to section 'Module' of 'cegma' Module
You can load the modules by:
module load biocontainers
module load cegma
Link to section 'Example job' of 'cegma' Example job
Using #!/bin/sh -l
as shebang in the slurm job script will cause the failure of some biocontainer modules. Please use #!/bin/bash
instead.
To run cegma on our clusters:
#!/bin/bash
#SBATCH -A myallocation # Allocation name
#SBATCH -t 1:00:00
#SBATCH -N 1
#SBATCH -n 1
#SBATCH --job-name=cegma
#SBATCH --mail-type=FAIL,BEGIN,END
#SBATCH --error=%x-%J-%u.err
#SBATCH --output=%x-%J-%u.out
module --force purge
ml biocontainers cegma
cegma --genome genome.fasta -o output
cellbender
Link to section 'Introduction' of 'cellbender' Introduction
Cellbender
is a software package for eliminating technical artifacts from high-throughput single-cell RNA sequencing (scRNA-seq) data.
For more information, please check its website: https://biocontainers.pro/tools/cellbender and its home page on Github.
Link to section 'Versions' of 'cellbender' Versions
- 0.2.0
- 0.2.2
Link to section 'Commands' of 'cellbender' Commands
- cellbender
Link to section 'Module' of 'cellbender' Module
You can load the modules by:
module load biocontainers
module load cellbender
Link to section 'Example job' of 'cellbender' Example job
Using #!/bin/sh -l
as shebang in the slurm job script will cause the failure of some biocontainer modules. Please use #!/bin/bash
instead.
To run Cellbender on our clusters:
#!/bin/bash
#SBATCH -A myallocation # Allocation name
#SBATCH -t 10:00:00
#SBATCH -N 1
#SBATCH -n 24
#SBATCH --job-name=cellbender
#SBATCH --mail-type=FAIL,BEGIN,END
#SBATCH --error=%x-%J-%u.err
#SBATCH --output=%x-%J-%u.out
module --force purge
ml biocontainers cellbender
cellbender remove-background \
--input cellranger/test_count/run_count_1kpbmcs/outs/raw_feature_bc_matrix.h5 \
--output output_cpu.h5 \
--expected-cells 1000 \
--total-droplets-included 20000 \
--fpr 0.01 \
--epochs 150
cellphonedb
Link to section 'Introduction' of 'cellphonedb' Introduction
CellPhoneDB is a publicly available repository of curated receptors, ligands and their interactions.
Docker hub: https://hub.docker.com/r/eagleshot/cellphonedb
Home page: https://github.com/Teichlab/cellphonedb
Link to section 'Versions' of 'cellphonedb' Versions
- 2.1.7
Link to section 'Commands' of 'cellphonedb' Commands
- cellphonedb
Link to section 'Module' of 'cellphonedb' Module
You can load the modules by:
module load biocontainers
module load cellphonedb
Link to section 'Example job' of 'cellphonedb' Example job
Using #!/bin/sh -l
as shebang in the slurm job script will cause the failure of some biocontainer modules. Please use #!/bin/bash
instead.
To run cellphonedb on our clusters:
#!/bin/bash
#SBATCH -A myallocation # Allocation name
#SBATCH -t 1:00:00
#SBATCH -N 1
#SBATCH -n 1
#SBATCH --job-name=cellphonedb
#SBATCH --mail-type=FAIL,BEGIN,END
#SBATCH --error=%x-%J-%u.err
#SBATCH --output=%x-%J-%u.out
module --force purge
ml biocontainers cellphonedb
cellranger
Cellranger
is a set of analysis pipelines that process Chromium single-cell data to align reads, generate feature-barcode matrices, perform clustering and other secondary analysis, and more.
Detailed usage can be found here: https://support.10xgenomics.com/single-cell-gene-expression/software/pipelines/latest/what-is-cell-ranger.
Link to section 'Versions' of 'cellranger' Versions
- 6.0.1
- 6.1.1
- 6.1.2
- 7.0.0
- 7.0.1
- 7.1.0
Link to section 'Commands' of 'cellranger' Commands
- cellranger mkfastq
- cellranger count
- cellranger aggr
- cellranger reanalyze
- cellranger multi
Link to section 'Module' of 'cellranger' Module
You can load the modules by:
module load biocontainers
module load cellranger
Link to section 'Example job' of 'cellranger' Example job
Using #!/bin/sh -l
as shebang in the slurm job script will cause the failure of some biocontainer modules. Please use #!/bin/bash
instead.
To run cellranger our our clusters:
#!/bin/bash
#SBATCH -A myallocation # Allocation name
#SBATCH -t 20:00:00
#SBATCH -N 1
#SBATCH -n 48
#SBATCH --job-name=cellranger
#SBATCH --mail-type=FAIL,BEGIN,END
#SBATCH --error=%x-%J-%u.err
#SBATCH --output=%x-%J-%u.out
module --force purge
ml biocontainers cellranger
cellranger count --id=run_count_1kpbmcs --fastqs=pbmc_1k_v3_fastqs --sample=pbmc_1k_v3 --transcriptome=refdata-gex-GRCh38-2020-A
cellranger-arc
Link to section 'Introduction' of 'cellranger-arc' Introduction
Cell Ranger ARC is a set of analysis pipelines that process Chromium Single Cell Multiome ATAC + Gene Expression sequencing data to generate a variety of analyses pertaining to gene expression (GEX), chromatin accessibility, and their linkage. Furthermore, since the ATAC and GEX measurements are on the very same cell, we are able to perform analyses that link chromatin accessibility and GEX.
Docker hub: https://hub.docker.com/r/cumulusprod/cellranger-arc
Home page: https://support.10xgenomics.com/single-cell-multiome-atac-gex/software/pipelines/latest/what-is-cell-ranger-arc
Link to section 'Versions' of 'cellranger-arc' Versions
- 2.0.2_patched
- 2.0.2
Link to section 'Commands' of 'cellranger-arc' Commands
- cellranger-arc
Link to section 'Module' of 'cellranger-arc' Module
You can load the modules by:
module load biocontainers
module load cellranger-arc
Link to section 'Example job' of 'cellranger-arc' Example job
Using #!/bin/sh -l
as shebang in the slurm job script will cause the failure of some biocontainer modules. Please use #!/bin/bash
instead.
To run cellranger-arc on our clusters:
#!/bin/bash
#SBATCH -A myallocation # Allocation name
#SBATCH -t 1:00:00
#SBATCH -N 1
#SBATCH -n 1
#SBATCH --job-name=cellranger-arc
#SBATCH --mail-type=FAIL,BEGIN,END
#SBATCH --error=%x-%J-%u.err
#SBATCH --output=%x-%J-%u.out
module --force purge
ml biocontainers cellranger-arc
cellranger-atac
Link to section 'Introduction' of 'cellranger-atac' Introduction
Cellranger-atac
is a set of analysis pipelines that process Chromium Single Cell ATAC data.
Docker hub: https://hub.docker.com/r/cumulusprod/cellranger-atac and its home page: https://support.10xgenomics.com/single-cell-atac/software/pipelines/latest/algorithms/overview.
Link to section 'Versions' of 'cellranger-atac' Versions
- 2.0.0
- 2.1.0
Link to section 'Commands' of 'cellranger-atac' Commands
- cellranger-atac
Link to section 'Module' of 'cellranger-atac' Module
You can load the modules by:
module load biocontainers
module load cellranger-atac
Link to section 'Example job' of 'cellranger-atac' Example job
Using #!/bin/sh -l
as shebang in the slurm job script will cause the failure of some biocontainer modules. Please use #!/bin/bash
instead.
To run Cellranger-atac on our clusters:
#!/bin/bash
#SBATCH -A myallocation # Allocation name
#SBATCH -t 1:00:00
#SBATCH -N 1
#SBATCH -n 8
#SBATCH --mem=64G
#SBATCH --job-name=cellranger-atac
#SBATCH --mail-type=FAIL,BEGIN,END
#SBATCH --error=%x-%J-%u.err
#SBATCH --output=%x-%J-%u.out
module --force purge
ml biocontainers cellranger-atac
cellranger-atac count --id=sample345 \
--reference=refdata-cellranger-arc-GRCh38-2020-A-2.0.0 \
--fastqs=runs/HAWT7ADXX/outs/fastq_path \
--sample=mysample \
--localcores=8 \
--localmem=64
cellranger-dna
Link to section 'Introduction' of 'cellranger-dna' Introduction
Cell Ranger DNA is a set of analysis pipelines that process Chromium single cell DNA sequencing output to align reads, identify copy number variation (CNV), and compare heterogeneity among cells.
Home page: https://support.10xgenomics.com/single-cell-dna/software/pipelines/latest/what-is-cell-ranger-dna
Link to section 'Versions' of 'cellranger-dna' Versions
- 1.1.0
Link to section 'Commands' of 'cellranger-dna' Commands
- cellranger-dna
Link to section 'Module' of 'cellranger-dna' Module
You can load the modules by:
module load biocontainers
module load cellranger-dna
Link to section 'Example job' of 'cellranger-dna' Example job
Using #!/bin/sh -l
as shebang in the slurm job script will cause the failure of some biocontainer modules. Please use #!/bin/bash
instead.
To run cellranger-dna on our clusters:
#!/bin/bash
#SBATCH -A myallocation # Allocation name
#SBATCH -t 1:00:00
#SBATCH -N 1
#SBATCH -n 1
#SBATCH --job-name=cellranger-dna
#SBATCH --mail-type=FAIL,BEGIN,END
#SBATCH --error=%x-%J-%u.err
#SBATCH --output=%x-%J-%u.out
module --force purge
ml biocontainers cellranger-dna
cellrank
CellRank
a toolkit to uncover cellular dynamics based on Markov state modeling of single-cell data. Detailed information about CellRank can be found here: https://cellrank.readthedocs.io/en/stable/.
Link to section 'Versions' of 'cellrank' Versions
- 1.5.1
Link to section 'Commands' of 'cellrank' Commands
- python
- python3
Link to section 'Module' of 'cellrank' Module
You can load the modules by:
module load biocontainers
module load cellrank/1.5.1
The CellRank container also contained scVelo and scanpy. When you want to use CellRank, do not load scVelo or scanpy.
Link to section 'Interactive job' of 'cellrank' Interactive job
To run CellRank interactively on our clusters:
(base) UserID@bell-fe00:~ $ sinteractive -N1 -n12 -t4:00:00 -A myallocation
salloc: Granted job allocation 12345869
salloc: Waiting for resource configuration
salloc: Nodes bell-a008 are ready for job
(base) UserID@bell-a008:~ $ module load biocontainers cellrank/1.5.1
(base) UserID@bell-a008:~ $ python
Python 3.9.9 | packaged by conda-forge | (main, Dec 20 2021, 02:41:03)
[GCC 9.4.0] :: Anaconda, Inc. on linux
Type "help", "copyright", "credits" or "license" for more information.
>>> import scanpy as sc
>>> import scvelo as scv
>>> import cellrank as cr
>>> import numpy as np
>>> scv.settings.verbosity = 3
>>> scv.settings.set_figure_params("scvelo")
>>> cr.settings.verbosity = 2
Link to section 'Batch job' of 'cellrank' Batch job
Using #!/bin/sh -l
as shebang in the slurm job script will cause the failure of some biocontainer modules. Please use #!/bin/bash
instead.
To submit a sbatch job on our clusters:
#!/bin/bash
#SBATCH -A myallocation # Allocation name
#SBATCH -t 10:00:00
#SBATCH -N 1
#SBATCH -n 24
#SBATCH --job-name=cellrank
#SBATCH --mail-type=FAIL,BEGIN,END
#SBATCH --error=%x-%J-%u.err
#SBATCH --output=%x-%J-%u.out
module --force purge
ml biocontainers cellrank/1.5.1
python script.py
cellrank-krylov
CellRank
a toolkit to uncover cellular dynamics based on Markov state modeling of single-cell data. CellRank-krylov
is CellRank
installed with extra libraries, enabling it to have better performance for large datasets (>15k cells).
Detailed information about CellRank can be found here: https://cellrank.readthedocs.io/en/stable/.
Link to section 'Versions' of 'cellrank-krylov' Versions
- 1.5.1
Link to section 'Commands' of 'cellrank-krylov' Commands
- python
- python3
Link to section 'Module' of 'cellrank-krylov' Module
You can load the modules by:
module load biocontainers
module load cellrank-krylov/1.5.1
The CellRank container also contained scVelo and scanpy. When you want to use CellRank, do not load scVelo or scanpy.
Link to section 'Interactive job' of 'cellrank-krylov' Interactive job
To run CellRank-krylov interactively on our clusters:
(base) UserID@bell-fe00:~ $ sinteractive -N1 -n12 -t4:00:00 -A myallocation
salloc: Granted job allocation 12345869
salloc: Waiting for resource configuration
salloc: Nodes bell-a008 are ready for job
(base) UserID@bell-a008:~ $ module load biocontainers cellrank-krylov/1.5.1
(base) UserID@bell-a008:~ $ python
Python 3.9.9 | packaged by conda-forge | (main, Dec 20 2021, 02:41:03)
[GCC 9.4.0] :: Anaconda, Inc. on linux
Type "help", "copyright", "credits" or "license" for more information.
>>> import scanpy as sc
>>> import scvelo as scv
>>> import cellrank as cr
>>> import numpy as np
>>> scv.settings.verbosity = 3
>>> scv.settings.set_figure_params("scvelo")
>>> cr.settings.verbosity = 2
Link to section 'Batch job' of 'cellrank-krylov' Batch job
Using #!/bin/sh -l
as shebang in the slurm job script will cause the failure of some biocontainer modules. Please use #!/bin/bash
instead.
To submit a sbatch job on our clusters:
#!/bin/bash
#SBATCH -A myallocation # Allocation name
#SBATCH -t 10:00:00
#SBATCH -N 1
#SBATCH -n 24
#SBATCH --job-name=cellrank-krylov
#SBATCH --mail-type=FAIL,BEGIN,END
#SBATCH --error=%x-%J-%u.err
#SBATCH --output=%x-%J-%u.out
module --force purge
ml biocontainers cellrank-krylov/1.5.1
python script.py
cellsnp-lite
Link to section 'Introduction' of 'cellsnp-lite' Introduction
cellSNP
aims to pileup the expressed alleles in single-cell or bulk RNA-seq data, which can be directly used for donor deconvolution in multiplexed single-cell RNA-seq data, particularly with vireo, which assigns cells to donors and detects doublets, even without genotyping reference.
For more information, please check its website: https://biocontainers.pro/tools/cellsnp-lite and its home page on Github.
Link to section 'Versions' of 'cellsnp-lite' Versions
- 1.2.2
Link to section 'Commands' of 'cellsnp-lite' Commands
- cellsnp-lite
Link to section 'Module' of 'cellsnp-lite' Module
You can load the modules by:
module load biocontainers
module load cellsnp-lite
Link to section 'Example job' of 'cellsnp-lite' Example job
Using #!/bin/sh -l
as shebang in the slurm job script will cause the failure of some biocontainer modules. Please use #!/bin/bash
instead.
To run cellSNP on our clusters:
#!/bin/bash
#SBATCH -A myallocation # Allocation name
#SBATCH -t 1:00:00
#SBATCH -N 1
#SBATCH -n 8
#SBATCH --job-name=cellsnp-lite
#SBATCH --mail-type=FAIL,BEGIN,END
#SBATCH --error=%x-%J-%u.err
#SBATCH --output=%x-%J-%u.out
module --force purge
ml biocontainers cellsnp-lite
cellsnp-lite -s sample.bam -b barcode.tsv -O cellsnp_out -p 8 --minMAF 0.1 --minCOUNT 100
celltypist
Link to section 'Introduction' of 'celltypist' Introduction
Celltypist
is a tool for semi-automatic cell type annotation.
For more information, please check its website: https://biocontainers.pro/tools/celltypist and its home page on Github.
Link to section 'Versions' of 'celltypist' Versions
- 0.2.0
- 1.1.0
Link to section 'Commands' of 'celltypist' Commands
- celltypist
- python
- python3
Link to section 'Module' of 'celltypist' Module
You can load the modules by:
module load biocontainers
module load celltypist
Link to section 'Example job' of 'celltypist' Example job
Using #!/bin/sh -l
as shebang in the slurm job script will cause the failure of some biocontainer modules. Please use #!/bin/bash
instead.
To run Celltypist on our clusters:
#!/bin/bash
#SBATCH -A myallocation # Allocation name
#SBATCH -t 1:00:00
#SBATCH -N 1
#SBATCH -n 8
#SBATCH --job-name=celltypist
#SBATCH --mail-type=FAIL,BEGIN,END
#SBATCH --error=%x-%J-%u.err
#SBATCH --output=%x-%J-%u.out
module --force purge
ml biocontainers celltypist
celltypist --indata demo_2000_cells.h5ad --model Immune_All_Low.pkl --outdir output
centrifuge
Link to section 'Introduction' of 'centrifuge' Introduction
Centrifuge
is a novel microbial classification engine that enables rapid, accurate, and sensitive labeling of reads and quantification of species on desktop computers.
For more information, please check its website: https://biocontainers.pro/tools/centrifuge and its home page: http://www.ccb.jhu.edu/software/centrifuge/.
Link to section 'Versions' of 'centrifuge' Versions
- 1.0.4_beta
Link to section 'Commands' of 'centrifuge' Commands
- centrifuge
- centrifuge-BuildSharedSequence.pl
- centrifuge-RemoveEmptySequence.pl
- centrifuge-RemoveN.pl
- centrifuge-build
- centrifuge-build-bin
- centrifuge-class
- centrifuge-compress.pl
- centrifuge-download
- centrifuge-inspect
- centrifuge-inspect-bin
- centrifuge-kreport
- centrifuge-sort-nt.pl
- centrifuge_evaluate.py
- centrifuge_simulate_reads.py
Link to section 'Module' of 'centrifuge' Module
You can load the modules by:
module load biocontainers
module load centrifuge
Link to section 'Example job' of 'centrifuge' Example job
Using #!/bin/sh -l
as shebang in the slurm job script will cause the failure of some biocontainer modules. Please use #!/bin/bash
instead.
To run Centrifuge on our clusters:
#!/bin/bash
#SBATCH -A myallocation # Allocation name
#SBATCH -t 1:00:00
#SBATCH -N 1
#SBATCH -n 1
#SBATCH --job-name=centrifuge
#SBATCH --mail-type=FAIL,BEGIN,END
#SBATCH --error=%x-%J-%u.err
#SBATCH --output=%x-%J-%u.out
module --force purge
ml biocontainers centrifuge
centrifuge-download -o taxonomy taxonomy
centrifuge-download -o library -m -d "archaea,bacteria,viral" refseq > seqid2taxid.map
cat library/*/*.fna > input-sequences.fna
centrifuge-build -p 8 --conversion-table seqid2taxid.map \
--taxonomy-tree taxonomy/nodes.dmp --name-table taxonomy/names.dmp \
input-sequences.fna abv
cfsan-snp-pipeline
Link to section 'Introduction' of 'cfsan-snp-pipeline' Introduction
The CFSAN SNP Pipeline is a Python-based system for the production of SNP matrices from sequence data used in the phylogenetic analysis of pathogenic organisms sequenced from samples of interest to food safety.
Docker hub: https://hub.docker.com/r/staphb/cfsan-snp-pipeline
Home page: https://github.com/CFSAN-Biostatistics/snp-pipeline
Link to section 'Versions' of 'cfsan-snp-pipeline' Versions
- 2.2.1
Link to section 'Commands' of 'cfsan-snp-pipeline' Commands
- cfsan_snp_pipeline
Link to section 'Module' of 'cfsan-snp-pipeline' Module
You can load the modules by:
module load biocontainers
module load cfsan-snp-pipeline
Link to section 'Example job' of 'cfsan-snp-pipeline' Example job
Using #!/bin/sh -l
as shebang in the slurm job script will cause the failure of some biocontainer modules. Please use #!/bin/bash
instead.
To run cfsan-snp-pipeline on our clusters:
#!/bin/bash
#SBATCH -A myallocation # Allocation name
#SBATCH -t 1:00:00
#SBATCH -N 1
#SBATCH -n 1
#SBATCH --job-name=cfsan-snp-pipeline
#SBATCH --mail-type=FAIL,BEGIN,END
#SBATCH --error=%x-%J-%u.err
#SBATCH --output=%x-%J-%u.out
module --force purge
ml biocontainers cfsan-snp-pipeline
checkm-genome
Link to section 'Introduction' of 'checkm-genome' Introduction
CheckM provides a set of tools for assessing the quality of genomes recovered from isolates, single cells, or metagenomes.
BioContainers: https://biocontainers.pro/tools/checkm-genome
Home page: https://github.com/Ecogenomics/CheckM
Link to section 'Versions' of 'checkm-genome' Versions
- 1.2.0
- 1.2.2
Link to section 'Commands' of 'checkm-genome' Commands
- checkm-genome
Link to section 'Module' of 'checkm-genome' Module
You can load the modules by:
module load biocontainers
module load checkm-genome
Link to section 'Example job' of 'checkm-genome' Example job
Using #!/bin/sh -l
as shebang in the slurm job script will cause the failure of some biocontainer modules. Please use #!/bin/bash
instead.
To run checkm-genome on our clusters:
#!/bin/bash
#SBATCH -A myallocation # Allocation name
#SBATCH -t 1:00:00
#SBATCH -N 1
#SBATCH -n 8
#SBATCH --job-name=checkm-genome
#SBATCH --mail-type=FAIL,BEGIN,END
#SBATCH --error=%x-%J-%u.err
#SBATCH --output=%x-%J-%u.out
module --force purge
ml biocontainers checkm-genome
checkm lineage_wf -t 8 -x fa bins checkm
chewbbaca
Link to section 'Introduction' of 'chewbbaca' Introduction
chewBBACA is a comprehensive pipeline including a set of functions for the creation and validation of whole genome and core genome MultiLocus Sequence Typing (wg/cgMLST) schemas, providing an allele calling algorithm based on Blast Score Ratio that can be run in multiprocessor settings and a set of functions to visualize and validate allele variation in the loci. chewBBACA performs the schema creation and allele calls on complete or draft genomes resulting from de novo assemblers.
BioContainers: https://biocontainers.pro/tools/chewbbaca
Home page: https://github.com/B-UMMI/chewBBACA
Link to section 'Versions' of 'chewbbaca' Versions
- 2.8.5
Link to section 'Commands' of 'chewbbaca' Commands
- chewBBACA.py
Link to section 'Module' of 'chewbbaca' Module
You can load the modules by:
module load biocontainers
module load chewbbaca
Link to section 'Example job' of 'chewbbaca' Example job
Using #!/bin/sh -l
as shebang in the slurm job script will cause the failure of some biocontainer modules. Please use #!/bin/bash
instead.
To run chewbbaca on our clusters:
#!/bin/bash
#SBATCH -A myallocation # Allocation name
#SBATCH -t 1:00:00
#SBATCH -N 1
#SBATCH -n 4
#SBATCH --job-name=chewbbaca
#SBATCH --mail-type=FAIL,BEGIN,END
#SBATCH --error=%x-%J-%u.err
#SBATCH --output=%x-%J-%u.out
module --force purge
ml biocontainers chewbbaca
chewBBACA.py CreateSchema -i complete_genomes/ -o tutorial_schema --ptf Streptococcus_agalactiae.trn --cpu 4
chewBBACA.py AlleleCall -i complete_genomes/ -g tutorial_schema/schema_seed -o results32_wgMLST --cpu 4
chopper
Link to section 'Introduction' of 'chopper' Introduction
Chopper is Rust implementation of NanoFilt+NanoLyse, both originally written in Python. This tool, intended for long read sequencing such as PacBio or ONT, filters and trims a fastq file. Filtering is done on average read quality and minimal or maximal read length, and applying a headcrop (start of read) and tailcrop (end of read) while printing the reads passing the filter.
BioContainers: https://biocontainers.pro/tools/chopper
Home page: https://github.com/wdecoster/chopper
Link to section 'Versions' of 'chopper' Versions
- 0.2.0
Link to section 'Commands' of 'chopper' Commands
- chopper
Link to section 'Module' of 'chopper' Module
You can load the modules by:
module load biocontainers
module load chopper
Link to section 'Example job' of 'chopper' Example job
Using #!/bin/sh -l
as shebang in the slurm job script will cause the failure of some biocontainer modules. Please use #!/bin/bash
instead.
To run chopper on our clusters:
#!/bin/bash
#SBATCH -A myallocation # Allocation name
#SBATCH -t 1:00:00
#SBATCH -N 1
#SBATCH -n 1
#SBATCH --job-name=chopper
#SBATCH --mail-type=FAIL,BEGIN,END
#SBATCH --error=%x-%J-%u.err
#SBATCH --output=%x-%J-%u.out
module --force purge
ml biocontainers chopper
chromap
Link to section 'Introduction' of 'chromap' Introduction
Chromap is an ultrafast method for aligning and preprocessing high throughput chromatin profiles.
BioContainers: https://biocontainers.pro/tools/chromap
Home page: https://github.com/haowenz/chromap
Link to section 'Versions' of 'chromap' Versions
- 0.2.2
Link to section 'Commands' of 'chromap' Commands
- chromap
Link to section 'Module' of 'chromap' Module
You can load the modules by:
module load biocontainers
module load chromap
Link to section 'Example job' of 'chromap' Example job
Using #!/bin/sh -l
as shebang in the slurm job script will cause the failure of some biocontainer modules. Please use #!/bin/bash
instead.
To run chromap on our clusters:
#!/bin/bash
#SBATCH -A myallocation # Allocation name
#SBATCH -t 1:00:00
#SBATCH -N 1
#SBATCH -n 1
#SBATCH --job-name=chromap
#SBATCH --mail-type=FAIL,BEGIN,END
#SBATCH --error=%x-%J-%u.err
#SBATCH --output=%x-%J-%u.out
module --force purge
ml biocontainers chromap
cicero
Link to section 'Introduction' of 'cicero' Introduction
CICERO
(Clipped-reads Extended for RNA Optimization) is an assembly-based algorithm to detect diverse classes of driver gene fusions from RNA-seq.
For more information, please check its home page on Github.
Link to section 'Versions' of 'cicero' Versions
- 1.8.1
Link to section 'Commands' of 'cicero' Commands
- Cicero.sh
Link to section 'Module' of 'cicero' Module
You can load the modules by:
module load biocontainers
module load cicero
Link to section 'Example job' of 'cicero' Example job
Using #!/bin/sh -l
as shebang in the slurm job script will cause the failure of some biocontainer modules. Please use #!/bin/bash
instead.
To run CICERO on our clusters:
#!/bin/bash
#SBATCH -A myallocation # Allocation name
#SBATCH -t 1:00:00
#SBATCH -N 1
#SBATCH -n 1
#SBATCH --job-name=cicero
#SBATCH --mail-type=FAIL,BEGIN,END
#SBATCH --error=%x-%J-%u.err
#SBATCH --output=%x-%J-%u.out
module --force purge
ml biocontainers cicero
circexplorer2
Link to section 'Introduction' of 'circexplorer2' Introduction
CIRCexplorer2 is a comprehensive and integrative circular RNA analysis toolset. It is the successor of CIRCexplorer with plenty of new features to facilitate circular RNA identification and characterization.
BioContainers: https://biocontainers.pro/tools/circexplorer2
Home page: https://github.com/YangLab/CIRCexplorer2
Link to section 'Versions' of 'circexplorer2' Versions
- 2.3.8
Link to section 'Commands' of 'circexplorer2' Commands
- CIRCexplorer2
- fast_circ.py
- fetch_ucsc.py
Link to section 'Module' of 'circexplorer2' Module
You can load the modules by:
module load biocontainers
module load circexplorer2
Link to section 'Example job' of 'circexplorer2' Example job
Using #!/bin/sh -l
as shebang in the slurm job script will cause the failure of some biocontainer modules. Please use #!/bin/bash
instead.
To run circexplorer2 on our clusters:
#!/bin/bash
#SBATCH -A myallocation # Allocation name
#SBATCH -t 1:00:00
#SBATCH -N 1
#SBATCH -n 1
#SBATCH --job-name=circexplorer2
#SBATCH --mail-type=FAIL,BEGIN,END
#SBATCH --error=%x-%J-%u.err
#SBATCH --output=%x-%J-%u.out
module --force purge
ml biocontainers circexplorer2
circlator
Link to section 'Introduction' of 'circlator' Introduction
Circlator
is a tool to circularize genome assemblies.
Docker hub: https://hub.docker.com/r/sangerpathogens/circlator and its home page on Github.
Link to section 'Versions' of 'circlator' Versions
- 1.5.5
Link to section 'Commands' of 'circlator' Commands
- circlator
- python3
Link to section 'Module' of 'circlator' Module
You can load the modules by:
module load biocontainers
module load circlator
Link to section 'Example job' of 'circlator' Example job
Using #!/bin/sh -l
as shebang in the slurm job script will cause the failure of some biocontainer modules. Please use #!/bin/bash
instead.
To run Circlator on our clusters:
#!/bin/bash
#SBATCH -A myallocation # Allocation name
#SBATCH -t 1:00:00
#SBATCH -N 1
#SBATCH -n 1
#SBATCH --job-name=circlator
#SBATCH --mail-type=FAIL,BEGIN,END
#SBATCH --error=%x-%J-%u.err
#SBATCH --output=%x-%J-%u.out
module --force purge
ml biocontainers circlator
circlator minimus2 minimus2_test_run_minimus2.in.fa minimus2_test
circompara2
Link to section 'Introduction' of 'circompara2' Introduction
CirComPara2 is a computational pipeline to detect, quantify, and correlate expression of linear and circular RNAs from RNA-seq data that combines multiple circRNA-detection methods.
Home page: https://github.com/egaffo/circompara2
Link to section 'Versions' of 'circompara2' Versions
- 0.1.2.1
Link to section 'Commands' of 'circompara2' Commands
- python
- Rscript
- circompara2
- CIRCexplorer2
- CIRCexplorer_compare.R
- CIRI.pl
- DCC
- DCC_patch_CombineCounts.py
- QRE_finder.py
- STAR
- bedtools
- bowtie
- bowtie-build
- bowtie-inspect
- bowtie2
- bowtie2-build
- bowtie2-inspect
- bwa
- ccp_circrna_expression.R
- cfinder_compare.R
- chimoutjunc_to_bed.py
- ciri_compare.R
- collect_read_stats.R
- convert_circrna_collect_tables.py
- cuffcompare
- cuffdiff
- cufflinks
- cuffmerge
- cuffnorm
- cuffquant
- dcc_compare.R
- dcc_fix_strand.R
- fasta_len.py
- fastq_rev_comp.py
- fastqc
- filterCirc.awk
- filterSpliceSiteCircles.pl
- filter_and_cast_circexp.R
- filter_fastq_reads.py
- filter_findcirc_res.R
- filter_segemehl.R
- find_circ.py
- findcirc_compare.R
- gene_annotation.R
- get_ce2_bwa_bks_reads.R
- get_ce2_bwa_circ_reads.py
- get_ce2_segemehl_bks_reads.R
- get_ce2_star_bks_reads.R
- get_ce2_th_bks_reads.R
- get_circompara_counts.R
- get_circrnaFinder_bks_reads.R
- get_ciri_bks_reads.R
- get_dcc_bks_reads.R
- get_findcirc_bks_reads.R
- get_gene_expression_files.R
- get_stringtie_rawcounts.R
- gffread
- gtfToGenePred
- gtf_collapse_features.py
- gtf_to_sam
- haarz.x
- hisat2
- hisat2-build
- htseq-count
- install_R_libs.R
- nrForwardSplicedReads.pl
- parallel
- pip
- postProcessStarAlignment.pl
- samtools
- samtools_v0
- scons
- segemehl.x
- split_start_end_gtf.py
- starCirclesToBed.pl
- stringtie
- testrealign_compare.R
- tophat2
- trim_read_header.py
- trimmomatic-0.39.jar
- unmapped2anchors.py
- cf_filterChimout.awk
- circompara
- get_unmapped_reads_from_bam.sh
- install_circompara
- make_circrna_html
- make_indexes
Link to section 'Module' of 'circompara2' Module
You can load the modules by:
module load biocontainers
module load circompara2
Link to section 'Example job' of 'circompara2' Example job
Using #!/bin/sh -l
as shebang in the slurm job script will cause the failure of some biocontainer modules. Please use #!/bin/bash
instead.
To run circompara2 on our clusters:
#!/bin/bash
#SBATCH -A myallocation # Allocation name
#SBATCH -t 1:00:00
#SBATCH -N 1
#SBATCH -n 1
#SBATCH --job-name=circompara2
#SBATCH --mail-type=FAIL,BEGIN,END
#SBATCH --error=%x-%J-%u.err
#SBATCH --output=%x-%J-%u.out
module --force purge
ml biocontainers circompara2
circos
Link to section 'Introduction' of 'circos' Introduction
Circos
is a software package for visualizing data and information.
For more information, please check its website: https://biocontainers.pro/tools/circos and its home page: http://circos.ca.
Link to section 'Versions' of 'circos' Versions
- 0.69.8
Link to section 'Commands' of 'circos' Commands
- circos
Link to section 'Module' of 'circos' Module
You can load the modules by:
module load biocontainers
module load circos
Link to section 'Example job' of 'circos' Example job
Using #!/bin/sh -l
as shebang in the slurm job script will cause the failure of some biocontainer modules. Please use #!/bin/bash
instead.
To run Circos on our clusters:
#!/bin/bash
#SBATCH -A myallocation # Allocation name
#SBATCH -t 1:00:00
#SBATCH -N 1
#SBATCH -n 1
#SBATCH --job-name=circos
#SBATCH --mail-type=FAIL,BEGIN,END
#SBATCH --error=%x-%J-%u.err
#SBATCH --output=%x-%J-%u.out
module --force purge
ml biocontainers circos
circos -conf circos.conf
ciri2
Link to section 'Introduction' of 'ciri2' Introduction
CIRI2: Circular RNA identification based on multiple seed matching
Home page: https://sourceforge.net/projects/ciri/files/CIRI2/
Link to section 'Versions' of 'ciri2' Versions
- 2.0.6
Link to section 'Commands' of 'ciri2' Commands
- CIRI2.pl
Link to section 'Module' of 'ciri2' Module
You can load the modules by:
module load biocontainers
module load ciri2
Link to section 'Example job' of 'ciri2' Example job
Using #!/bin/sh -l
as shebang in the slurm job script will cause the failure of some biocontainer modules. Please use #!/bin/bash
instead.
To run ciri2 on our clusters:
#!/bin/bash
#SBATCH -A myallocation # Allocation name
#SBATCH -t 1:00:00
#SBATCH -N 1
#SBATCH -n 1
#SBATCH --job-name=ciri2
#SBATCH --mail-type=FAIL,BEGIN,END
#SBATCH --error=%x-%J-%u.err
#SBATCH --output=%x-%J-%u.out
module --force purge
ml biocontainers ciri2
ciriquant
Link to section 'Introduction' of 'ciriquant' Introduction
CIRIquant
is a comprehensive analysis pipeline for circRNA detection and quantification in RNA-Seq data.
Docker hub: https://hub.docker.com/r/mortreux/ciriquant and its home page on Github.
Link to section 'Versions' of 'ciriquant' Versions
- 1.1.2
Link to section 'Commands' of 'ciriquant' Commands
- CIRIquant
Link to section 'Module' of 'ciriquant' Module
You can load the modules by:
module load biocontainers
module load ciriquant
Link to section 'config.yml ' of 'ciriquant' config.yml
All required dependencies have been installed within the CIRIquant container image. But users still need toprovide the PATH of these executables in `config.yml`. Please use the below `config.yml` as example:
name: hg38
tools:
bwa: /bin/bwa
hisat2: /bin/hisat2
stringtie: /bin/stringtie
samtools: /usr/local/bin/samtools
reference:
fasta: reference/Homo_sapiens.GRCh38.dna.primary_assembly.fa
gtf: reference/Homo_sapiens.GRCh38.105.gtf
bwa_index: reference/Homo_sapiens.GRCh38.dna.primary_assembly.fa
hisat_index: reference/hg38_hisat2
Link to section 'Example job' of 'ciriquant' Example job
Using #!/bin/sh -l
as shebang in the slurm job script will cause the failure of some biocontainer modules. Please use #!/bin/bash
instead.
To run CIRIquant on our clusters:
#!/bin/bash
#SBATCH -A myallocation # Allocation name
#SBATCH -t 10:00:00
#SBATCH -N 1
#SBATCH -n 64
#SBATCH --job-name=ciriquant
#SBATCH --mail-type=FAIL,BEGIN,END
#SBATCH --error=%x-%J-%u.err
#SBATCH --output=%x-%J-%u.out
module --force purge
ml biocontainers ciriquant
CIRIquant -t 64 -1 SRR12095148_1.fastq -2 SRR12095148_2.fastq --config config.yml -o Output -p test
clair3
Link to section 'Introduction' of 'clair3' Introduction
Clair3 is a germline small variant caller for long-reads. Clair3 makes the best of two major method categories: pileup calling handles most variant candidates with speed, and full-alignment tackles complicated candidates to maximize precision and recall. Clair3 runs fast and has superior performance, especially at lower coverage. Clair3 is simple and modular for easy deployment and integration.
Docker hub: https://hub.docker.com/r/hkubal/clair3
Home page: https://github.com/HKU-BAL/Clair3
Link to section 'Versions' of 'clair3' Versions
- 0.1-r11
- 0.1-r12
Link to section 'Commands' of 'clair3' Commands
- run_clair3.sh
Link to section 'Module' of 'clair3' Module
You can load the modules by:
module load biocontainers
module load clair3
Model_path
model_path
is in /opt/models/
. The parameter will be like this --model_path="/opt/models/MODEL_NAME"
Link to section 'Example job' of 'clair3' Example job
Using #!/bin/sh -l
as shebang in the slurm job script will cause the failure of some biocontainer modules. Please use #!/bin/bash
instead.
To run clair3 on our clusters:
#!/bin/bash
#SBATCH -A myallocation # Allocation name
#SBATCH -t 1:00:00
#SBATCH -N 1
#SBATCH -n 1
#SBATCH --job-name=clair3
#SBATCH --mail-type=FAIL,BEGIN,END
#SBATCH --error=%x-%J-%u.err
#SBATCH --output=%x-%J-%u.out
module --force purge
ml biocontainers clair3
run_clair3.sh \
--bam_fn=input.bam \
--ref_fn=ref.fasta \
--threads=12 \
--platform=ont \
--model_path="/opt/models/ont" \
--output=output
clairvoyante
Link to section 'Introduction' of 'clairvoyante' Introduction
Clairvoyante is a deep neural network based variant caller.
Docker hub: https://hub.docker.com/r/lifebitai/clairvoyante
Home page: https://github.com/aquaskyline/Clairvoyante
Link to section 'Versions' of 'clairvoyante' Versions
- 1.02
Link to section 'Commands' of 'clairvoyante' Commands
- clairvoyante.py
Link to section 'Module' of 'clairvoyante' Module
You can load the modules by:
module load biocontainers
module load clairvoyante
Link to section 'Example job' of 'clairvoyante' Example job
Using #!/bin/sh -l
as shebang in the slurm job script will cause the failure of some biocontainer modules. Please use #!/bin/bash
instead.
To run clairvoyante on our clusters:
#!/bin/bash
#SBATCH -A myallocation # Allocation name
#SBATCH -t 1:00:00
#SBATCH -N 1
#SBATCH -n 1
#SBATCH --job-name=clairvoyante
#SBATCH --mail-type=FAIL,BEGIN,END
#SBATCH --error=%x-%J-%u.err
#SBATCH --output=%x-%J-%u.out
module --force purge
ml biocontainers clairvoyante
cd training
clairvoyante.py callVarBam \
--chkpnt_fn ../trainedModels/fullv3-illumina-novoalign-hg001+hg002-hg38/learningRate1e-3.epoch500 \
--bam_fn ../testingData/chr21/chr21.bam \
--ref_fn ../testingData/chr21/chr21.fa \
--bed_fn ../testingData/chr21/chr21.bed \
--call_fn chr21_calls.vcf \
--ctgName chr21
clearcnv
Link to section 'Introduction' of 'clearcnv' Introduction
ClearCNV: CNV calling from NGS panel data in the presence of ambiguity and noise.
BioContainers: https://biocontainers.pro/tools/clearcnv
Home page: https://github.com/bihealth/clear-cnv
Link to section 'Versions' of 'clearcnv' Versions
- 0.306
Link to section 'Commands' of 'clearcnv' Commands
- clearCNV
Link to section 'Module' of 'clearcnv' Module
You can load the modules by:
module load biocontainers
module load clearcnv
Link to section 'Example job' of 'clearcnv' Example job
Using #!/bin/sh -l
as shebang in the slurm job script will cause the failure of some biocontainer modules. Please use #!/bin/bash
instead.
To run clearcnv on our clusters:
#!/bin/bash
#SBATCH -A myallocation # Allocation name
#SBATCH -t 1:00:00
#SBATCH -N 1
#SBATCH -n 1
#SBATCH --job-name=clearcnv
#SBATCH --mail-type=FAIL,BEGIN,END
#SBATCH --error=%x-%J-%u.err
#SBATCH --output=%x-%J-%u.out
module --force purge
ml biocontainers clearcnv
clever-toolkit
Link to section 'Introduction' of 'clever-toolkit' Introduction
Clever-toolkit is a collection of tools to discover and genotype structural variations in genomes from paired-end sequencing reads. The main software is written in C++ with some auxiliary scripts in Python.
BioContainers: https://biocontainers.pro/tools/clever-toolkit
Home page: https://bitbucket.org/tobiasmarschall/clever-toolkit/src/master/
Link to section 'Versions' of 'clever-toolkit' Versions
- 2.4
Link to section 'Commands' of 'clever-toolkit' Commands
- clever
- laser
- bam-to-alignment-priors
- split-priors-by-chromosome
- clever-core
- postprocess-predictions
- evaluate-sv-predictions
- split-reads
- laser-core
- laser-recalibrate
- genotyper
- insert-length-histogram
- add-score-tags-to-bam
- bam2fastq
- remove-redundant-variations
- precompute-distributions
- extract-bad-reads
- filter-variations
- merge-to-vcf
- multiline-to-xa
- filter-bam
- read-group-stats
Link to section 'Module' of 'clever-toolkit' Module
You can load the modules by:
module load biocontainers
module load clever-toolkit
Link to section 'Example job' of 'clever-toolkit' Example job
Using #!/bin/sh -l
as shebang in the slurm job script will cause the failure of some biocontainer modules. Please use #!/bin/bash
instead.
To run clever-toolkit on our clusters:
#!/bin/bash
#SBATCH -A myallocation # Allocation name
#SBATCH -t 1:00:00
#SBATCH -N 1
#SBATCH -n 1
#SBATCH --job-name=clever-toolkit
#SBATCH --mail-type=FAIL,BEGIN,END
#SBATCH --error=%x-%J-%u.err
#SBATCH --output=%x-%J-%u.out
module --force purge
ml biocontainers clever-toolkit
cat mapped.bam | bam2fastq output_1.fq output_2.fq
clonalframeml
Link to section 'Introduction' of 'clonalframeml' Introduction
ClonalFrameML is a software package that performs efficient inference of recombination in bacterial genomes.
BioContainers: https://biocontainers.pro/tools/clonalframeml
Home page: https://github.com/xavierdidelot/ClonalFrameML
Link to section 'Versions' of 'clonalframeml' Versions
- 1.11
Link to section 'Commands' of 'clonalframeml' Commands
- ClonalFrameML
Link to section 'Module' of 'clonalframeml' Module
You can load the modules by:
module load biocontainers
module load clonalframeml
Link to section 'Example job' of 'clonalframeml' Example job
Using #!/bin/sh -l
as shebang in the slurm job script will cause the failure of some biocontainer modules. Please use #!/bin/bash
instead.
To run clonalframeml on our clusters:
#!/bin/bash
#SBATCH -A myallocation # Allocation name
#SBATCH -t 1:00:00
#SBATCH -N 1
#SBATCH -n 1
#SBATCH --job-name=clonalframeml
#SBATCH --mail-type=FAIL,BEGIN,END
#SBATCH --error=%x-%J-%u.err
#SBATCH --output=%x-%J-%u.out
module --force purge
ml biocontainers clonalframeml
clust
Link to section 'Introduction' of 'clust' Introduction
Clust is a fully automated method for identification of clusters (groups) of genes that are consistently co-expressed (well-correlated) in one or more heterogeneous datasets from one or multiple species.
BioContainers: https://biocontainers.pro/tools/clust
Home page: https://github.com/baselabujamous/clust
Link to section 'Versions' of 'clust' Versions
- 1.17.0
Link to section 'Commands' of 'clust' Commands
- clust
Link to section 'Module' of 'clust' Module
You can load the modules by:
module load biocontainers
module load clust
Link to section 'Example job' of 'clust' Example job
Using #!/bin/sh -l
as shebang in the slurm job script will cause the failure of some biocontainer modules. Please use #!/bin/bash
instead.
To run clust on our clusters:
#!/bin/bash
#SBATCH -A myallocation # Allocation name
#SBATCH -t 1:00:00
#SBATCH -N 1
#SBATCH -n 1
#SBATCH --job-name=clust
#SBATCH --mail-type=FAIL,BEGIN,END
#SBATCH --error=%x-%J-%u.err
#SBATCH --output=%x-%J-%u.out
module --force purge
ml biocontainers clust
clustalw
Link to section 'Introduction' of 'clustalw' Introduction
Clustalw
is a general purpose multiple alignment program for DNA or proteins.
For more information, please check its website: https://biocontainers.pro/tools/clustalw and its home page: http://www.clustal.org/clustal2/.
Link to section 'Versions' of 'clustalw' Versions
- 2.1
Link to section 'Commands' of 'clustalw' Commands
- clustalw
Link to section 'Module' of 'clustalw' Module
You can load the modules by:
module load biocontainers
module load clustalw
Link to section 'Example job' of 'clustalw' Example job
Using #!/bin/sh -l
as shebang in the slurm job script will cause the failure of some biocontainer modules. Please use #!/bin/bash
instead.
To run Clustalw on our clusters:
#!/bin/bash
#SBATCH -A myallocation # Allocation name
#SBATCH -t 1:00:00
#SBATCH -N 1
#SBATCH -n 1
#SBATCH --job-name=clustalw
#SBATCH --mail-type=FAIL,BEGIN,END
#SBATCH --error=%x-%J-%u.err
#SBATCH --output=%x-%J-%u.out
module --force purge
ml biocontainers clustalw
clustalw -tree -align -infile=seq.faa
cnvkit
Link to section 'Introduction' of 'cnvkit' Introduction
CNVkit
is a command-line toolkit and Python library for detecting copy number variants and alterations genome-wide from high-throughput sequencing.
For more information, please check its website: https://biocontainers.pro/tools/cnvkit and its home page on Github.
Link to section 'Versions' of 'cnvkit' Versions
- 0.9.9-py
Link to section 'Commands' of 'cnvkit' Commands
- cnvkit.py
- cnv_annotate.py
- cnv_expression_correlate.py
- cnv_updater.py
Link to section 'Module' of 'cnvkit' Module
You can load the modules by:
module load biocontainers
module load cnvkit
Link to section 'Example job' of 'cnvkit' Example job
Using #!/bin/sh -l
as shebang in the slurm job script will cause the failure of some biocontainer modules. Please use #!/bin/bash
instead.
To run CNVkit on our clusters:
#!/bin/bash
#SBATCH -A myallocation # Allocation name
#SBATCH -t 1:00:00
#SBATCH -N 1
#SBATCH -n 1
#SBATCH --job-name=cnvkit
#SBATCH --mail-type=FAIL,BEGIN,END
#SBATCH --error=%x-%J-%u.err
#SBATCH --output=%x-%J-%u.out
module --force purge
ml biocontainers cnvkit
cnvkit.py batch *Tumor.bam --normal *Normal.bam \
--targets my_baits.bed --fasta hg19.fasta \
--access data/access-5kb-mappable.hg19.bed \
--output-reference my_reference.cnn
--output-dir example/
cnvnator
Link to section 'Introduction' of 'cnvnator' Introduction
Cnvnator
is a tool for discovery and characterization of copy number variation (CNV) in population genome sequencing data.
For more information, please check its website: https://biocontainers.pro/tools/cnvnator and its home page on Github.
Link to section 'Versions' of 'cnvnator' Versions
- 0.4.1
Link to section 'Commands' of 'cnvnator' Commands
- cnvnator
- cnvnator2VCF.pl
- plotbaf.py
- plotcircular.py
- plotrdbaf.py
- pytools.py
Link to section 'Module' of 'cnvnator' Module
You can load the modules by:
module load biocontainers
module load cnvnator
Link to section 'Example job' of 'cnvnator' Example job
Using #!/bin/sh -l
as shebang in the slurm job script will cause the failure of some biocontainer modules. Please use #!/bin/bash
instead.
To run Cnvnator on our clusters:
#!/bin/bash
#SBATCH -A myallocation # Allocation name
#SBATCH -t 1:00:00
#SBATCH -N 1
#SBATCH -n 1
#SBATCH --job-name=cnvnator
#SBATCH --mail-type=FAIL,BEGIN,END
#SBATCH --error=%x-%J-%u.err
#SBATCH --output=%x-%J-%u.out
module --force purge
ml biocontainers cnvnator
cnvnator -root file.root -tree file.bam -chrom $(seq 1 22) X Y
plotcircular.py file.root
coinfinder
Link to section 'Introduction' of 'coinfinder' Introduction
Coinfinder is an algorithm and software tool that detects genes which associate and dissociate with other genes more often than expected by chance in pangenomes.
BioContainers: https://biocontainers.pro/tools/coinfinder
Home page: https://github.com/fwhelan/coinfinder
Link to section 'Versions' of 'coinfinder' Versions
- 1.2.0
Link to section 'Commands' of 'coinfinder' Commands
- coinfinder
Link to section 'Module' of 'coinfinder' Module
You can load the modules by:
module load biocontainers
module load coinfinder
Link to section 'Example job' of 'coinfinder' Example job
Using #!/bin/sh -l
as shebang in the slurm job script will cause the failure of some biocontainer modules. Please use #!/bin/bash
instead.
To run coinfinder on our clusters:
#!/bin/bash
#SBATCH -A myallocation # Allocation name
#SBATCH -t 1:00:00
#SBATCH -N 1
#SBATCH -n 1
#SBATCH --job-name=coinfinder
#SBATCH --mail-type=FAIL,BEGIN,END
#SBATCH --error=%x-%J-%u.err
#SBATCH --output=%x-%J-%u.out
module --force purge
ml biocontainers coinfinder
coinfinder -i coinfinder-manuscript/gene_presence_absence.csv \
-I -p coinfinder-manuscript/core-gps_fasttree.newick \
-o output
concoct
CONCOCT
: Clustering cONtigs with COverage and ComposiTion.
Detailed usage can be found here: https://github.com/BinPro/CONCOCT
Link to section 'Versions' of 'concoct' Versions
- 1.1.0
Link to section 'Commands' of 'concoct' Commands
- concoct
- concoct_refine
- concoct_coverage_table.py
- cut_up_fasta.py
- extract_fasta_bins.py
- merge_cutup_clustering.py
Link to section 'Module' of 'concoct' Module
You can load the modules by:
module load biocontainers
module load concoct/1.1.0-py38
Link to section 'Example job' of 'concoct' Example job
Using #!/bin/sh -l
as shebang in the slurm job script will cause the failure of some biocontainer modules. Please use #!/bin/bash
instead.
To run concoct on our our clusters:
#!/bin/bash
#SBATCH -A myallocation # Allocation name
#SBATCH -t 20:00:00
#SBATCH -N 1
#SBATCH -n 24
#SBATCH --job-name=concoct
#SBATCH --mail-type=FAIL,BEGIN,END
#SBATCH --error=%x-%J-%u.err
#SBATCH --output=%x-%J-%u.out
module --force purge
ml biocontainers concoct/1.1.0-py38
cut_up_fasta.py final.contigs.fa -c 10000 -o 0 --merge_last -b contigs_10K.bed > contigs_10K.fa
concoct_coverage_table.py contigs_10K.bed SRR1976948_sorted.bam > coverage_table.tsv
concoct --composition_file contigs_10K.fa --coverage_file coverage_table.tsv -b concoct_output/
control-freec
Link to section 'Introduction' of 'control-freec' Introduction
Control-freec
is a tool for detection of copy-number changes and allelic imbalances (including LOH) using deep-sequencing data.
For more information, please check its website: https://biocontainers.pro/tools/control-freec and its home page on Github.
Link to section 'Versions' of 'control-freec' Versions
- 11.6
Link to section 'Commands' of 'control-freec' Commands
- freec
Link to section 'Module' of 'control-freec' Module
You can load the modules by:
module load biocontainers
module load control-freec
Link to section 'Example job' of 'control-freec' Example job
Using #!/bin/sh -l
as shebang in the slurm job script will cause the failure of some biocontainer modules. Please use #!/bin/bash
instead.
To run Control-freec on our clusters:
#!/bin/bash
#SBATCH -A myallocation # Allocation name
#SBATCH -t 1:00:00
#SBATCH -N 1
#SBATCH -n 1
#SBATCH --job-name=control-freec
#SBATCH --mail-type=FAIL,BEGIN,END
#SBATCH --error=%x-%J-%u.err
#SBATCH --output=%x-%J-%u.out
module --force purge
ml biocontainers control-freec
freec -conf config_chr19.txt
cooler

Link to section 'Introduction' of 'cooler' Introduction
Cooler
is a support library for a sparse, compressed, binary persistent storage format, also called cooler, used to store genomic interaction data, such as Hi-C contact matrices.
For more information, please check its website: https://biocontainers.pro/tools/cooler and its home page on Github.
Link to section 'Versions' of 'cooler' Versions
- 0.8.11
Link to section 'Commands' of 'cooler' Commands
- cooler
- python
- python3
Link to section 'Module' of 'cooler' Module
You can load the modules by:
module load biocontainers
module load Cooler
Link to section 'Interactive job' of 'cooler' Interactive job
To run Cooler interactively on our clusters:
(base) UserID@bell-fe00:~ $ sinteractive -N1 -n12 -t4:00:00 -A myallocation
salloc: Granted job allocation 12345869
salloc: Waiting for resource configuration
salloc: Nodes bell-a008 are ready for job
(base) UserID@bell-a008:~ $ module load biocontainers cooler
(base) UserID@bell-a008:~ $ python
Python 3.9.7 | packaged by conda-forge | (default, Sep 29 2021, 19:20:46)
[GCC 9.4.0] on linux
Type "help", "copyright", "credits" or "license" for more information.
>>> import cooler
Link to section 'Batch job' of 'cooler' Batch job
Using #!/bin/sh -l
as shebang in the slurm job script will cause the failure of some biocontainer modules. Please use #!/bin/bash
instead.
To run Cooler batch jobs on our clusters:
#!/bin/bash
#SBATCH -A myallocation # Allocation name
#SBATCH -t 1:00:00
#SBATCH -N 1
#SBATCH -n 1
#SBATCH --job-name=cooler
#SBATCH --mail-type=FAIL,BEGIN,END
#SBATCH --error=%x-%J-%u.err
#SBATCH --output=%x-%J-%u.out
module --force purge
ml biocontainers cooler
cooler info data/Rao2014-GM12878-MboI-allreps-filtered.1000kb.cool
cooler info -f bin-size data/Rao2014-GM12878-MboI-allreps-filtered.1000kb.cool
cooler info -m data/Rao2014-GM12878-MboI-allreps-filtered.1000kb.cool
cooler tree data/Rao2014-GM12878-MboI-allreps-filtered.1000kb.cool
cooler attrs data/Rao2014-GM12878-MboI-allreps-filtered.1000kb.cool
coverm
Link to section 'Introduction' of 'coverm' Introduction
Coverm
is a configurable, easy to use and fast DNA read coverage and relative abundance calculator focused on metagenomics applications.
For more information, please check its website: https://biocontainers.pro/tools/coverm and its home page on Github.
Link to section 'Versions' of 'coverm' Versions
- 0.6.1
Link to section 'Commands' of 'coverm' Commands
- coverm
Link to section 'Module' of 'coverm' Module
You can load the modules by:
module load biocontainers
module load coverm
Link to section 'Example job' of 'coverm' Example job
Using #!/bin/sh -l
as shebang in the slurm job script will cause the failure of some biocontainer modules. Please use #!/bin/bash
instead.
To run Coverm on our clusters:
#!/bin/bash
#SBATCH -A myallocation # Allocation name
#SBATCH -t 1:00:00
#SBATCH -N 1
#SBATCH -n 1
#SBATCH --job-name=coverm
#SBATCH --mail-type=FAIL,BEGIN,END
#SBATCH --error=%x-%J-%u.err
#SBATCH --output=%x-%J-%u.out
module --force purge
ml biocontainers coverm
coverm genome --genome-fasta-files xcc.fasta --coupled SRR11234553_1.fastq SRR11234553_2.fastq
cramino
Link to section 'Introduction' of 'cramino' Introduction
Cramino is a tool for quick quality assessment of cram and bam files, intended for long read sequencing.
Docker hub: https://hub.docker.com/r/alexanrna/cramino
Home page: https://github.com/wdecoster/cramino
Link to section 'Versions' of 'cramino' Versions
- 0.9.6
Link to section 'Commands' of 'cramino' Commands
- cramino
Link to section 'Module' of 'cramino' Module
You can load the modules by:
module load biocontainers
module load cramino
Link to section 'Example job' of 'cramino' Example job
Using #!/bin/sh -l
as shebang in the slurm job script will cause the failure of some biocontainer modules. Please use #!/bin/bash
instead.
To run cramino on our clusters:
#!/bin/bash
#SBATCH -A myallocation # Allocation name
#SBATCH -t 1:00:00
#SBATCH -N 1
#SBATCH -n 1
#SBATCH --job-name=cramino
#SBATCH --mail-type=FAIL,BEGIN,END
#SBATCH --error=%x-%J-%u.err
#SBATCH --output=%x-%J-%u.out
module --force purge
ml biocontainers cramino
crisprcasfinder
CRISPRCasFinder
==============================
CRISPRCasFinder
enables the easy detection of CRISPRs and cas genes in user-submitted sequence data. It is an updated, improved, and integrated version of CRISPRFinder and CasFinder.
Detailed usage can be found here: https://github.com/dcouvin/CRISPRCasFinder
Link to section 'Versions' of 'crisprcasfinder' Versions
- 4.2.20
Link to section 'Commands' of 'crisprcasfinder' Commands
- CRISPRCasFinder.pl
Link to section 'Module' of 'crisprcasfinder' Module
You can load the modules by:
module load biocontainers
module load crisprcasfinder/4.2.20
Link to section 'Example job' of 'crisprcasfinder' Example job
Using #!/bin/sh -l
as shebang in the slurm job script will cause the failure of some biocontainer modules. Please use #!/bin/bash
instead.
To run CRISPRCasFinder on our our clusters:
#!/bin/bash
#SBATCH -A myallocation # Allocation name
#SBATCH -t 2:00:00
#SBATCH -N 1
#SBATCH -n 12
#SBATCH --job-name=CRISPRCasFinder
#SBATCH --mail-type=FAIL,BEGIN,END
#SBATCH --error=%x-%J-%u.err
#SBATCH --output=%x-%J-%u.out
module --force purge
ml biocontainers crisprcasfinder/4.2.20
CRISPRCasFinder.pl -in install_test/sequence.fasta -cas -cf CasFinder-2.0.3 -def G -keep
crispresso2
Link to section 'Introduction' of 'crispresso2' Introduction
CRISPResso2 is a software pipeline designed to enable rapid and intuitive interpretation of genome editing experiments.
Docker hub: https://hub.docker.com/r/pinellolab/crispresso2
Home page: https://github.com/pinellolab/CRISPResso2
Link to section 'Versions' of 'crispresso2' Versions
- 2.2.10
- 2.2.11a
- 2.2.8
- 2.2.9
Link to section 'Commands' of 'crispresso2' Commands
- CRISPResso
- CRISPRessoAggregate
- CRISPRessoBatch
- CRISPRessoCompare
- CRISPRessoPooled
- CRISPRessoPooledWGSCompare
- CRISPRessoWGS
Link to section 'Module' of 'crispresso2' Module
You can load the modules by:
module load biocontainers
module load crispresso2
Link to section 'Example job' of 'crispresso2' Example job
Using #!/bin/sh -l
as shebang in the slurm job script will cause the failure of some biocontainer modules. Please use #!/bin/bash
instead.
To run crispresso2 on our clusters:
#!/bin/bash
#SBATCH -A myallocation # Allocation name
#SBATCH -t 1:00:00
#SBATCH -N 1
#SBATCH -n 1
#SBATCH --job-name=crispresso2
#SBATCH --mail-type=FAIL,BEGIN,END
#SBATCH --error=%x-%J-%u.err
#SBATCH --output=%x-%J-%u.out
module --force purge
ml biocontainers crispresso2
CRISPResso --fastq_r1 nhej.r1.fastq.gz --fastq_r2 nhej.r2.fastq.gz -n nhej --amplicon_seq \
AATGTCCCCCAATGGGAAGTTCATCTGGCACTGCCCACAGGTGAGGAGGTCATGATCCCCTTCTGGAGCTCCCAACGGGCCGTGGTCTGGTTCATCATCTGTAAGAATGGCTTCAAGAGGCTCGGCTGTGGTT
crispritz
Link to section 'Introduction' of 'crispritz' Introduction
Crispritz
is a software package containing 5 different tools dedicated to perform predictive analysis and result assessment on CRISPR/Cas experiments.
For more information, please check its website: https://biocontainers.pro/tools/crispritz and its home page on Github.
Link to section 'Versions' of 'crispritz' Versions
- 2.6.5
Link to section 'Commands' of 'crispritz' Commands
- crispritz.py
Link to section 'Module' of 'crispritz' Module
You can load the modules by:
module load biocontainers
module load crispritz
Link to section 'Example job' of 'crispritz' Example job
Using #!/bin/sh -l
as shebang in the slurm job script will cause the failure of some biocontainer modules. Please use #!/bin/bash
instead.
To run Crispritz on our clusters:
#!/bin/bash
#SBATCH -A myallocation # Allocation name
#SBATCH -t 1:00:00
#SBATCH -N 1
#SBATCH -n 1
#SBATCH --job-name=crispritz
#SBATCH --mail-type=FAIL,BEGIN,END
#SBATCH --error=%x-%J-%u.err
#SBATCH --output=%x-%J-%u.out
module --force purge
ml biocontainers crispritz
crispritz.py add-variants hg38_1000genomeproject_vcf/ hg38_ref/ &> output.redirect.out
crispritz.py index-genome hg38_ref hg38_ref/ 20bp-NGG-SpCas9.txt -bMax 2 &> output.redirect.out
crispritz.py search hg38_ref/ 20bp-NGG-SpCas9.txt EMX1.sgRNA.txt emx1.hg38 -mm 4 -t -scores hg38_ref/ &> output.redirect.out
crispritz.py search genome_library/NGG_2_hg38_ref/ 20bp-NGG-SpCas9.txt EMX1.sgRNA.txt emx1.hg38.bulges -index -mm 4 -bDNA 1 -bRNA 1 -t &> output.redirect.out
crispritz.py annotate-results emx1.hg38.targets.txt hg38Annotation.bed emx1.hg38 &> output.redirect.out
cross_match
Link to section 'Introduction' of 'cross_match' Introduction
cross_match
is a general purpose utility for comparing any two DNA sequence sets using a 'banded' version of swat.
For more information, please check its home page: http://www.phrap.org/phredphrapconsed.html#block_phrap.
Link to section 'Versions' of 'cross_match' Versions
- 1.090518
Link to section 'Commands' of 'cross_match' Commands
- cross_match
Link to section 'Module' of 'cross_match' Module
You can load the modules by:
module load biocontainers
module load cross_match
Link to section 'Example job' of 'cross_match' Example job
Using #!/bin/sh -l
as shebang in the slurm job script will cause the failure of some biocontainer modules. Please use #!/bin/bash
instead.
To run cross_match on our clusters:
#!/bin/bash
#SBATCH -A myallocation # Allocation name
#SBATCH -t 1:00:00
#SBATCH -N 1
#SBATCH -n 1
#SBATCH --job-name=cross_match
#SBATCH --mail-type=FAIL,BEGIN,END
#SBATCH --error=%x-%J-%u.err
#SBATCH --output=%x-%J-%u.out
module --force purge
ml biocontainers cross_match
crossmap
Link to section 'Introduction' of 'crossmap' Introduction
Crossmap
is a program for genome coordinates conversion between different assemblies.
For more information, please check its website: https://biocontainers.pro/tools/crossmap and its home page: https://crossmap.readthedocs.io/en/latest/#convert-maf-format-files.
Link to section 'Versions' of 'crossmap' Versions
- 0.6.3
Link to section 'Commands' of 'crossmap' Commands
- CrossMap.py
Link to section 'Module' of 'crossmap' Module
You can load the modules by:
module load biocontainers
module load crossmap
Link to section 'Example job' of 'crossmap' Example job
Using #!/bin/sh -l
as shebang in the slurm job script will cause the failure of some biocontainer modules. Please use #!/bin/bash
instead.
To run Crossmap on our clusters:
#!/bin/bash
#SBATCH -A myallocation # Allocation name
#SBATCH -t 1:00:00
#SBATCH -N 1
#SBATCH -n 1
#SBATCH --job-name=crossmap
#SBATCH --mail-type=FAIL,BEGIN,END
#SBATCH --error=%x-%J-%u.err
#SBATCH --output=%x-%J-%u.out
module --force purge
ml biocontainers crossmap
CrossMap.py bed GRCh37_to_GRCh38.chain.gz test.bed
csvtk
Link to section 'Introduction' of 'csvtk' Introduction
Csvtk
is a cross-platform, efficient and practical CSV/TSV toolkit.
For more information, please check its website: https://biocontainers.pro/tools/csvtk and its home page on Github.
Link to section 'Versions' of 'csvtk' Versions
- 0.23.0
- 0.25.0
Link to section 'Commands' of 'csvtk' Commands
- csvtk
Link to section 'Module' of 'csvtk' Module
You can load the modules by:
module load biocontainers
module load csvtk
Link to section 'Example job' of 'csvtk' Example job
Using #!/bin/sh -l
as shebang in the slurm job script will cause the failure of some biocontainer modules. Please use #!/bin/bash
instead.
To run Csvtk on our clusters:
#!/bin/bash
#SBATCH -A myallocation # Allocation name
#SBATCH -t 1:00:00
#SBATCH -N 1
#SBATCH -n 1
#SBATCH --job-name=csvtk
#SBATCH --mail-type=FAIL,BEGIN,END
#SBATCH --error=%x-%J-%u.err
#SBATCH --output=%x-%J-%u.out
module --force purge
ml biocontainers csvtk
cat data.csv \
| csvtk summary --ignore-non-digits --fields f4:sum,f5:sum --groups f1,f2 \
| csvtk pretty
cufflinks
Link to section 'Introduction' of 'cufflinks' Introduction
Cufflinks
assembles transcripts, estimates their abundances, and tests for differential expression and regulation in RNA-Seq samples. It accepts aligned RNA-Seq reads and assembles the alignments into a parsimonious set of transcripts. Cufflinks then estimates the relative abundances of these transcripts based on how many reads support each one, taking into account biases in library preparation protocols.
For more information, please check its website: https://biocontainers.pro/tools/cufflinks and its home page on Github.
Link to section 'Versions' of 'cufflinks' Versions
- 2.2.1
Link to section 'Commands' of 'cufflinks' Commands
- cuffcompare
- cuffdiff
- cufflinks
- cuffmerge
- cuffnorm
- cuffquant
- gffread
- gtf_to_sam
Link to section 'Module' of 'cufflinks' Module
You can load the modules by:
module load biocontainers
module load cufflinks
Link to section 'Example job' of 'cufflinks' Example job
Using #!/bin/sh -l
as shebang in the slurm job script will cause the failure of some biocontainer modules. Please use #!/bin/bash
instead.
To run Cufflinks on our clusters:
#!/bin/bash
#SBATCH -A myallocation # Allocation name
#SBATCH -t 1:00:00
#SBATCH -N 1
#SBATCH -n 8
#SBATCH --job-name=cufflinks
#SBATCH --mail-type=FAIL,BEGIN,END
#SBATCH --error=%x-%J-%u.err
#SBATCH --output=%x-%J-%u.out
module --force purge
ml biocontainers cufflinks
cufflinks -p 8 -G transcript.gtf --library-type fr-unstranded -o cufflinks_output tophat_out/accepted_hits.bam
cutadapt
Link to section 'Introduction' of 'cutadapt' Introduction
Cutadapt
finds and removes adapter sequences, primers, poly-A tails and other types of unwanted sequence from your high-throughput sequencing reads.
For more information, please check its website: https://biocontainers.pro/tools/cutadapt and its home page: https://cutadapt.readthedocs.io/en/stable/.
Link to section 'Versions' of 'cutadapt' Versions
- 3.4
- 3.7
Link to section 'Commands' of 'cutadapt' Commands
- cutadapt
Link to section 'Module' of 'cutadapt' Module
You can load the modules by:
module load biocontainers
module load cutadapt
Link to section 'Example job' of 'cutadapt' Example job
Using #!/bin/sh -l
as shebang in the slurm job script will cause the failure of some biocontainer modules. Please use #!/bin/bash
instead.
To run Cutadapt on our clusters:
#!/bin/bash
#SBATCH -A myallocation # Allocation name
#SBATCH -t 1:00:00
#SBATCH -N 1
#SBATCH -n 1
#SBATCH --job-name=cutadapt
#SBATCH --mail-type=FAIL,BEGIN,END
#SBATCH --error=%x-%J-%u.err
#SBATCH --output=%x-%J-%u.out
module --force purge
ml biocontainers cutadapt
cutadapt -a AACCGGTT -o output.fastq input.fastq
cuttlefish
Link to section 'Introduction' of 'cuttlefish' Introduction
Cuttlefish is a fast, parallel, and very lightweight memory tool to construct the compacted de Bruijn graph from sequencing reads or reference sequences. It is highly scalable in terms of the size of the input data.
BioContainers: https://biocontainers.pro/tools/cuttlefish
Home page: https://github.com/COMBINE-lab/cuttlefish
Link to section 'Versions' of 'cuttlefish' Versions
- 2.1.1
Link to section 'Commands' of 'cuttlefish' Commands
- cuttlefish
Link to section 'Module' of 'cuttlefish' Module
You can load the modules by:
module load biocontainers
module load cuttlefish
Link to section 'Example job' of 'cuttlefish' Example job
Using #!/bin/sh -l
as shebang in the slurm job script will cause the failure of some biocontainer modules. Please use #!/bin/bash
instead.
To run cuttlefish on our clusters:
#!/bin/bash
#SBATCH -A myallocation # Allocation name
#SBATCH -t 1:00:00
#SBATCH -N 1
#SBATCH -n 1
#SBATCH --job-name=cuttlefish
#SBATCH --mail-type=FAIL,BEGIN,END
#SBATCH --error=%x-%J-%u.err
#SBATCH --output=%x-%J-%u.out
module --force purge
ml biocontainers cuttlefish
cyvcf2
Link to section 'Introduction' of 'cyvcf2' Introduction
Cyvcf2
is a cython wrapper around htslib built for fast parsing of Variant Call Format (VCF) files.
For more information, please check its website: https://biocontainers.pro/tools/cyvcf2 and its home page on Github.
Link to section 'Versions' of 'cyvcf2' Versions
- 0.30.14
Link to section 'Commands' of 'cyvcf2' Commands
- cyvcf2
- python
- python3
Link to section 'Module' of 'cyvcf2' Module
You can load the modules by:
module load biocontainers
module load cyvcf2
Link to section 'Interactive job' of 'cyvcf2' Interactive job
To run Cyvcf2 interactively on our clusters:
(base) UserID@bell-fe00:~ $ sinteractive -N1 -n1 -t1:00:00 -A myallocation
salloc: Granted job allocation 12345869
salloc: Waiting for resource configuration
salloc: Nodes bell-a008 are ready for job
(base) UserID@bell-a008:~ $ module load biocontainers scanpy/1.8.2
(base) UserID@bell-a008:~ $ python
Python 3.7.12 | packaged by conda-forge | (default, Oct 26 2021, 06:08:53)
[GCC 9.4.0] on linux
Type "help", "copyright", "credits" or "license" for more information.
>>> from cyvcf2 import VCF
Link to section 'Batch job' of 'cyvcf2' Batch job
Using #!/bin/sh -l
as shebang in the slurm job script will cause the failure of some biocontainer modules. Please use #!/bin/bash
instead.
To run Cyvcf2 on our clusters:
#!/bin/bash
#SBATCH -A myallocation # Allocation name
#SBATCH -t 1:00:00
#SBATCH -N 1
#SBATCH -n 1
#SBATCH --job-name=cyvcf2
#SBATCH --mail-type=FAIL,BEGIN,END
#SBATCH --error=%x-%J-%u.err
#SBATCH --output=%x-%J-%u.out
module --force purge
ml biocontainers cyvcf2
cyvcf2 --help
cyvcf2 [OPTIONS] <vcf_file>
das_tool
Link to section 'Introduction' of 'das_tool' Introduction
DAS Tool is an automated method that integrates the results of a flexible number of binning algorithms to calculate an optimized, non-redundant set of bins from a single assembly.
BioContainers: https://biocontainers.pro/tools/das_tool
Home page: https://github.com/cmks/DAS_Tool
Link to section 'Versions' of 'das_tool' Versions
- 1.1.6
Link to section 'Commands' of 'das_tool' Commands
- DAS_Tool
- Contigs2Bin_to_Fasta.sh
- Fasta_to_Contig2Bin.sh
- get_species_taxids.sh
Link to section 'Module' of 'das_tool' Module
You can load the modules by:
module load biocontainers
module load das_tool
Link to section 'Example job' of 'das_tool' Example job
Using #!/bin/sh -l
as shebang in the slurm job script will cause the failure of some biocontainer modules. Please use #!/bin/bash
instead.
To run das_tool on our clusters:
#!/bin/bash
#SBATCH -A myallocation # Allocation name
#SBATCH -t 1:00:00
#SBATCH -N 1
#SBATCH -n 4
#SBATCH --job-name=das_tool
#SBATCH --mail-type=FAIL,BEGIN,END
#SBATCH --error=%x-%J-%u.err
#SBATCH --output=%x-%J-%u.out
module --force purge
ml biocontainers das_tool
DAS_Tool -i sample.human.gut_concoct_contigs2bin.tsv,\
sample.human.gut_maxbin2_contigs2bin.tsv,\
sample.human.gut_metabat_contigs2bin.tsv,\
sample.human.gut_tetraESOM_contigs2bin.tsv \
-l concoct,maxbin,metabat,tetraESOM \
-c sample.human.gut_contigs.fa \
-o DASToolRun2 \
--proteins DASToolRun1_proteins.faa \
--write_bin_evals \
--threads 4 \
--score_threshold 0.6
dbg2olc
Link to section 'Introduction' of 'dbg2olc' Introduction
Dbg2olc
is used for efficient assembly of large genomes using long erroneous reads of the third generation sequencing technologies.
For more information, please check its website: https://biocontainers.pro/tools/dbg2olc and its home page on Github.
Link to section 'Versions' of 'dbg2olc' Versions
- 20180222
- 20200723
Link to section 'Commands' of 'dbg2olc' Commands
- AssemblyStatistics
- DBG2OLC
- RunSparcConsensus.txt
- SelectLongestReads
- SeqIO.py
- Sparc
- SparseAssembler
- split_and_run_sparc.sh
- split_and_run_sparc.sh.bak
- split_reads_by_backbone.py
Link to section 'Module' of 'dbg2olc' Module
You can load the modules by:
module load biocontainers
module load dbg2olc
Link to section 'Example job' of 'dbg2olc' Example job
Using #!/bin/sh -l
as shebang in the slurm job script will cause the failure of some biocontainer modules. Please use #!/bin/bash
instead.
To run Dbg2olc on our clusters:
#!/bin/bash
#SBATCH -A myallocation # Allocation name
#SBATCH -t 1:00:00
#SBATCH -N 1
#SBATCH -n 1
#SBATCH --job-name=dbg2olc
#SBATCH --mail-type=FAIL,BEGIN,END
#SBATCH --error=%x-%J-%u.err
#SBATCH --output=%x-%J-%u.out
module --force purge
ml biocontainers dbg2olc
SelectLongestReads sum 600000000 longest 0 o TEST.fq f SRR1976948.abundtrim.subset.pe.fq
deconseq
Link to section 'Introduction' of 'deconseq' Introduction
DeconSeq: DECONtamination of SEQuence data using a modified version of BWA-SW. The DeconSeq tool can be used to automatically detect and efficiently remove sequence contamination from genomic and metagenomic datasets. It is easily configurable and provides a user-friendly interface.
Home page: http://deconseq.sourceforge.net/
Link to section 'Versions' of 'deconseq' Versions
- 0.4.3
Link to section 'Commands' of 'deconseq' Commands
- bwa64
- deconseq.pl
- splitFasta.pl
Link to section 'Module' of 'deconseq' Module
You can load the modules by:
module load biocontainers
module load deconseq
Helper command
Users need to use DeconSeqConfig.pm
to specify the database information. Besides, for the current deconseq
module in `biocontainers`, users need to copy the executables to your current directory, including bwa64
, deconseq.pl
, and splitFasta.pl
. This step is only needed to run once.
A helper command copy_DeconSeqConfig
is provided to copy the configuration file DeconSeqConfig.pm
and executables to your current directory. You just need to run the command copy_DeconSeqConfig
and modify DeconSeqConfig.pm
as needed:
copy_DeconSeqConfig
nano DeconSeqConfig.pm # modify database information as needed
For detailed information about how to config DeconSeqConfig.pm
, please check its online manual (https://sourceforge.net/projects/deconseq/files/).
Link to section 'Example job' of 'deconseq' Example job
Using #!/bin/sh -l
as shebang in the slurm job script will cause the failure of some biocontainer modules. Please use #!/bin/bash
instead.
To run deconseq on our clusters:
#!/bin/bash
#SBATCH -A myallocation # Allocation name
#SBATCH -t 1:00:00
#SBATCH -N 1
#SBATCH -n 1
#SBATCH --job-name=deconseq
#SBATCH --mail-type=FAIL,BEGIN,END
#SBATCH --error=%x-%J-%u.err
#SBATCH --output=%x-%J-%u.out
module --force purge
ml biocontainers deconseq
bwa64 index -p hg38_db -a bwtsw Homo_sapiens.GRCh38.dna.fa
bwa64 index -p m39_db -a bwtsw GRCm38.p4.genome.fa
deconseq.pl -f input.fastq -dbs hg38_db -dbs_retain m39_db
deepbgc
Link to section 'Introduction' of 'deepbgc' Introduction
Deepbgc
is a tool for BGC detection and classification using deep learning.
For more information, please check its website: https://biocontainers.pro/tools/deepbgc and its home page on Github.
Link to section 'Versions' of 'deepbgc' Versions
- 0.1.26
- 0.1.30
Link to section 'Commands' of 'deepbgc' Commands
- deepbgc
Link to section 'Module' of 'deepbgc' Module
You can load the modules by:
module load biocontainers
module load deepbgc
Link to section 'Example job' of 'deepbgc' Example job
Using #!/bin/sh -l
as shebang in the slurm job script will cause the failure of some biocontainer modules. Please use #!/bin/bash
instead.
To run Deepbgc on our clusters:
#!/bin/bash
#SBATCH -A myallocation # Allocation name
#SBATCH -t 1:00:00
#SBATCH -N 1
#SBATCH -n 1
#SBATCH --job-name=deepbgc
#SBATCH --mail-type=FAIL,BEGIN,END
#SBATCH --error=%x-%J-%u.err
#SBATCH --output=%x-%J-%u.out
module --force purge
ml biocontainers deepbgc
export DEEPBGC_DOWNLOADS_DIR=$PWD
deepbgc download
deepbgc pipeline genome.fa -o output
deepconsensus
Link to section 'Introduction' of 'deepconsensus' Introduction
DeepConsensus uses gap-aware sequence transformers to correct errors in Pacific Biosciences (PacBio) Circular Consensus Sequencing (CCS) data.
Docker hub: https://hub.docker.com/r/google/deepconsensus
Home page: https://github.com/google/deepconsensus
Link to section 'Versions' of 'deepconsensus' Versions
- 0.2.0
Link to section 'Commands' of 'deepconsensus' Commands
- deepconsensus
- ccs
- actc
Link to section 'Module' of 'deepconsensus' Module
You can load the modules by:
module load biocontainers
module load deepconsensus
Link to section 'Example job' of 'deepconsensus' Example job
Using #!/bin/sh -l
as shebang in the slurm job script will cause the failure of some biocontainer modules. Please use #!/bin/bash
instead.
To run deepconsensus on our clusters:
#!/bin/bash
#SBATCH -A myallocation # Allocation name
#SBATCH -t 1:00:00
#SBATCH -N 1
#SBATCH -n 1
#SBATCH --job-name=deepconsensus
#SBATCH --mail-type=FAIL,BEGIN,END
#SBATCH --error=%x-%J-%u.err
#SBATCH --output=%x-%J-%u.out
module --force purge
ml biocontainers deepconsensus
deepconsensus run \
--subreads_to_ccs=subreads_to_ccs.bam \
--ccs_fasta=ccs.fasta \
--checkpoint=checkpoint-50 \
--output=output.fastq \
--batch_zmws=100
deepsignal2
Link to section 'Introduction' of 'deepsignal2' Introduction
Deepsignal2
is a deep-learning method for detecting DNA methylation state from Oxford Nanopore sequencing reads.
For more information, please check its home page on Github.
Link to section 'Versions' of 'deepsignal2' Versions
- 0.1.2
Link to section 'Commands' of 'deepsignal2' Commands
- deepsignal2
- call_modification_frequency.py
- combine_call_mods_freq_files.py
- combine_two_strands_frequency.py
- concat_two_files.py
- evaluate_mods_call.py
- filter_samples_by_label.py
- filter_samples_by_positions.py
- gff_reader.py
- randsel_file_rows.py
- shuffle_a_big_file.py
- split_freq_file_by_5mC_motif.py
- txt_formater.py
Link to section 'Module' of 'deepsignal2' Module
You can load the modules by:
module load biocontainers
module load deepsignal2
Link to section 'Example job' of 'deepsignal2' Example job
Using #!/bin/sh -l
as shebang in the slurm job script will cause the failure of some biocontainer modules. Please use #!/bin/bash
instead.
To run Deepsignal2 on our clusters:
#!/bin/bash
#SBATCH -A myallocation # Allocation name
#SBATCH -t 1:00:00
#SBATCH -N 1
#SBATCH -n 1
#SBATCH --job-name=deepsignal2
#SBATCH --mail-type=FAIL,BEGIN,END
#SBATCH --error=%x-%J-%u.err
#SBATCH --output=%x-%J-%u.out
module --force purge
ml biocontainers deepsignal2
deeptools
Link to section 'Introduction' of 'deeptools' Introduction
DeepTools
is a collection of user-friendly tools for normalization and visualization of deep-sequencing data.
For more information, please check its website: https://biocontainers.pro/tools/deeptools and its home page on Github.
Link to section 'Versions' of 'deeptools' Versions
- 3.5.1-py
Link to section 'Commands' of 'deeptools' Commands
- alignmentSieve
- bamCompare
- bamCoverage
- bamPEFragmentSize
- bigwigCompare
- computeGCBias
- computeMatrix
- computeMatrixOperations
- correctGCBias
- deeptools
- estimateReadFiltering
- estimateScaleFactor
- multiBamSummary
- multiBigwigSummary
- plotCorrelation
- plotCoverage
- plotEnrichment
- plotFingerprint
- plotHeatmap
- plotPCA
- plotProfile
Link to section 'Module' of 'deeptools' Module
You can load the modules by:
module load biocontainers
module load deeptools
Link to section 'Example job' of 'deeptools' Example job
Using #!/bin/sh -l
as shebang in the slurm job script will cause the failure of some biocontainer modules. Please use #!/bin/bash
instead.
To run DeepTools on our clusters:
#!/bin/bash
#SBATCH -A myallocation # Allocation name
#SBATCH -t 1:00:00
#SBATCH -N 1
#SBATCH -n 4
#SBATCH --job-name=deeptools
#SBATCH --mail-type=FAIL,BEGIN,END
#SBATCH --error=%x-%J-%u.err
#SBATCH --output=%x-%J-%u.out
module --force purge
ml biocontainers deeptools
bamCoverage --normalizeUsing CPM -p 32 \
--effectiveGenomeSize 11000000 \
-b WT_coord_sorted.bam \
-o WT_coord_sorted.bw
deepvariant
Link to section 'Introduction' of 'deepvariant' Introduction
DeepVariant is a deep learning-based variant caller that takes aligned reads (in BAM or CRAM format), produces pileup image tensors from them, classifies each tensor using a convolutional neural network, and finally reports the results in a standard VCF or gVCF file.
Home page: https://github.com/google/deepvariant
Link to section 'Versions' of 'deepvariant' Versions
- 1.0.0
- 1.1.0
Link to section 'Commands' of 'deepvariant' Commands
- call_variants
- get-pip.py
- make_examples
- model_eval
- model_train
- postprocess_variants
- run-prereq.sh
- run_deepvariant
- run_deepvariant.py
- settings.sh
- show_examples
- vcf_stats_report
Link to section 'Module' of 'deepvariant' Module
You can load the modules by:
module load biocontainers
module load deepvariant
Link to section 'Example job' of 'deepvariant' Example job
Using #!/bin/sh -l
as shebang in the slurm job script will cause the failure of some biocontainer modules. Please use #!/bin/bash
instead.
To run deepvariant on our clusters:
#!/bin/bash
#SBATCH -A myallocation # Allocation name
#SBATCH -t 1:00:00
#SBATCH -N 1
#SBATCH -n 4
#SBATCH --job-name=deepvariant
#SBATCH --mail-type=FAIL,BEGIN,END
#SBATCH --error=%x-%J-%u.err
#SBATCH --output=%x-%J-%u.out
module --force purge
ml biocontainers deepvariant
INPUT_DIR="${PWD}/quickstart-testdata"
DATA_HTTP_DIR="https://storage.googleapis.com/deepvariant/quickstart-testdata"
mkdir -p ${INPUT_DIR}
wget -P ${INPUT_DIR} "${DATA_HTTP_DIR}"/NA12878_S1.chr20.10_10p1mb.bam
wget -P ${INPUT_DIR} "${DATA_HTTP_DIR}"/NA12878_S1.chr20.10_10p1mb.bam.bai
wget -P ${INPUT_DIR} "${DATA_HTTP_DIR}"/test_nist.b37_chr20_100kbp_at_10mb.bed
wget -P ${INPUT_DIR} "${DATA_HTTP_DIR}"/test_nist.b37_chr20_100kbp_at_10mb.vcf.gz
wget -P ${INPUT_DIR} "${DATA_HTTP_DIR}"/test_nist.b37_chr20_100kbp_at_10mb.vcf.gz.tbi
wget -P ${INPUT_DIR} "${DATA_HTTP_DIR}"/ucsc.hg19.chr20.unittest.fasta
wget -P ${INPUT_DIR} "${DATA_HTTP_DIR}"/ucsc.hg19.chr20.unittest.fasta.fai
wget -P ${INPUT_DIR} "${DATA_HTTP_DIR}"/ucsc.hg19.chr20.unittest.fasta.gz
wget -P ${INPUT_DIR} "${DATA_HTTP_DIR}"/ucsc.hg19.chr20.unittest.fasta.gz.fai
wget -P ${INPUT_DIR} "${DATA_HTTP_DIR}"/ucsc.hg19.chr20.unittest.fasta.gz.gzi
run_deepvariant --model_type=WGS --ref="${INPUT_DIR}"/ucsc.hg19.chr20.unittest.fasta --reads="${INPUT_DIR}"/NA12878_S1.chr20.10_10p1mb.bam --regions "chr20:10,000,000-10,010,000" --output_vcf="output/output.vcf.gz" --output_gvcf="output/output.g.vcf.gz" --intermediate_results_dir "output/intermediate_results_dir" --num_shards=4
delly
Link to section 'Introduction' of 'delly' Introduction
Delly
is an integrated structural variant (SV) prediction method that can discover, genotype and visualize deletions, tandem duplications, inversions and translocations at single-nucleotide resolution in short-read massively parallel sequencing data.
For more information, please check its website: https://biocontainers.pro/tools/delly and its home page on Github.
Link to section 'Versions' of 'delly' Versions
- 0.9.1
- 1.0.3
- 1.1.3
- 1.1.5
- 1.1.6
Link to section 'Commands' of 'delly' Commands
- delly
Link to section 'Module' of 'delly' Module
You can load the modules by:
module load biocontainers
module load delly
Link to section 'Example job' of 'delly' Example job
Using #!/bin/sh -l
as shebang in the slurm job script will cause the failure of some biocontainer modules. Please use #!/bin/bash
instead.
To run Delly on our clusters:
#!/bin/bash
#SBATCH -A myallocation # Allocation name
#SBATCH -t 1:00:00
#SBATCH -N 1
#SBATCH -n 1
#SBATCH --job-name=delly
#SBATCH --mail-type=FAIL,BEGIN,END
#SBATCH --error=%x-%J-%u.err
#SBATCH --output=%x-%J-%u.out
module --force purge
ml biocontainers delly
delly call -x hg19.excl -o delly.bcf -g hg19.fa input.bam
delly filter -f somatic -o t1.pre.bcf -s samples.tsv t1.bcf
dendropy
Link to section 'Introduction' of 'dendropy' Introduction
DendroPy is a Python library for phylogenetic computing. It provides classes and functions for the simulation, processing, and manipulation of phylogenetic trees and character matrices, and supports the reading and writing of phylogenetic data in a range of formats, such as NEXUS, NEWICK, NeXML, Phylip, FASTA, etc. Application scripts for performing some useful phylogenetic operations, such as data conversion and tree posterior distribution summarization, are also distributed and installed as part of the library. DendroPy can thus function as a stand-alone library for phylogenetics, a component of more complex multi-library phyloinformatic pipelines, or as a scripting "glue" that assembles and drives such pipelines.
BioContainers: https://biocontainers.pro/tools/dendropy
Home page: https://github.com/jeetsukumaran/DendroPy
Link to section 'Versions' of 'dendropy' Versions
- 4.5.2
Link to section 'Commands' of 'dendropy' Commands
- python
- python3
-
- - sumtrees.py
Link to section 'Module' of 'dendropy' Module
You can load the modules by:
module load biocontainers
module load dendropy
Link to section 'Example job' of 'dendropy' Example job
Using #!/bin/sh -l
as shebang in the slurm job script will cause the failure of some biocontainer modules. Please use #!/bin/bash
instead.
To run dendropy on our clusters:
#!/bin/bash
#SBATCH -A myallocation # Allocation name
#SBATCH -t 1:00:00
#SBATCH -N 1
#SBATCH -n 1
#SBATCH --job-name=dendropy
#SBATCH --mail-type=FAIL,BEGIN,END
#SBATCH --error=%x-%J-%u.err
#SBATCH --output=%x-%J-%u.out
module --force purge
ml biocontainers dendropy
diamond
Diamond
is a sequence aligner for protein and translated DNA searches, designed for high performance analysis of big sequence data. The key features are:
- Pairwise alignment of proteins and translated DNA at 100x-10,000x speed of BLAST.
- Frameshift alignments for long read analysis.
- Low resource requirements and suitable for running on standard desktops or laptops.
- Various output formats, including BLAST pairwise, tabular and XML, as well as taxonomic classification.
Detailed about its usage can be found here: https://github.com/bbuchfink/diamond
Link to section 'Versions' of 'diamond' Versions
- 2.0.13
- 2.0.14
- 2.0.15
Link to section 'Commands' of 'diamond' Commands
- diamond makedb
- diamond prepdb
- diamond blastp
- diamond blastx
- diamond view
- diamond version
- diamond dbinfo
- diamond help
- diamond test
Link to section 'Module' of 'diamond' Module
You can load the modules by:
module load biocontainers
module load diamond/2.0.14
Link to section 'Example job' of 'diamond' Example job
Using #!/bin/sh -l
as shebang in the slurm job script will cause the failure of some biocontainer modules. Please use #!/bin/bash
instead.
To run diamond on our our clusters:
#!/bin/bash
#SBATCH -A myallocation # Allocation name
#SBATCH -t 1:00:00
#SBATCH -N 1
#SBATCH -n 24
#SBATCH --job-name=diamond
#SBATCH --mail-type=FAIL,BEGIN,END
#SBATCH --error=%x-%J-%u.err
#SBATCH --output=%x-%J-%u.out
module --force purge
ml biocontainers diamond/2.0.14
diamond makedb --in uniprot_sprot.fasta -d uniprot_sprot
diamond blastp -p 24 -q test.faa -d uniprot_sprot --very-sensitive -o blastp_output.txt
dnaio
Link to section 'Introduction' of 'dnaio' Introduction
Dnaio
is a Python 3.7+ library for very efficient parsing and writing of FASTQ and also FASTA files.
For more information, please check its website: https://biocontainers.pro/tools/dnaio and its home page on Github.
Link to section 'Versions' of 'dnaio' Versions
- 0.8.1
Link to section 'Commands' of 'dnaio' Commands
- python
- python3
Link to section 'Module' of 'dnaio' Module
You can load the modules by:
module load biocontainers
module load dnaio
Link to section 'Example job' of 'dnaio' Example job
Using #!/bin/sh -l
as shebang in the slurm job script will cause the failure of some biocontainer modules. Please use #!/bin/bash
instead.
To run Dnaio on our clusters:
#!/bin/bash
#SBATCH -A myallocation # Allocation name
#SBATCH -t 1:00:00
#SBATCH -N 1
#SBATCH -n 1
#SBATCH --job-name=dnaio
#SBATCH --mail-type=FAIL,BEGIN,END
#SBATCH --error=%x-%J-%u.err
#SBATCH --output=%x-%J-%u.out
module --force purge
ml biocontainers dnaio
python dnaio_test.py
dragonflye
Link to section 'Introduction' of 'dragonflye' Introduction
Dragonflye is a pipeline that aims to make assembling Oxford Nanopore reads quick and easy.
BioContainers: https://biocontainers.pro/tools/dragonflye
Home page: https://github.com/rpetit3/dragonflye
Link to section 'Versions' of 'dragonflye' Versions
- 1.0.13
- 1.0.14
Link to section 'Commands' of 'dragonflye' Commands
- dragonflye
Link to section 'Module' of 'dragonflye' Module
You can load the modules by:
module load biocontainers
module load dragonflye
Link to section 'Example job' of 'dragonflye' Example job
Using #!/bin/sh -l
as shebang in the slurm job script will cause the failure of some biocontainer modules. Please use #!/bin/bash
instead.
To run dragonflye on our clusters:
#!/bin/bash
#SBATCH -A myallocation # Allocation name
#SBATCH -t 1:00:00
#SBATCH -N 1
#SBATCH -n 8
#SBATCH --job-name=dragonflye
#SBATCH --mail-type=FAIL,BEGIN,END
#SBATCH --error=%x-%J-%u.err
#SBATCH --output=%x-%J-%u.out
module --force purge
ml biocontainers dragonflye
dragonflye --cpus 8 \
--outdir output \
--reads SRR18498195.fastq
drep
Link to section 'Introduction' of 'drep' Introduction
Drep
is a python program for rapidly comparing large numbers of genomes.
For more information, please check its website: https://biocontainers.pro/tools/drep and its home page on Github.
Link to section 'Versions' of 'drep' Versions
- 3.2.2
Link to section 'Commands' of 'drep' Commands
- dRep
Link to section 'Module' of 'drep' Module
You can load the modules by:
module load biocontainers
module load drep
Link to section 'Example job' of 'drep' Example job
Using #!/bin/sh -l
as shebang in the slurm job script will cause the failure of some biocontainer modules. Please use #!/bin/bash
instead.
To run Drep on our clusters:
#!/bin/bash
#SBATCH -A myallocation # Allocation name
#SBATCH -t 1:00:00
#SBATCH -N 1
#SBATCH -n 1
#SBATCH --job-name=drep
#SBATCH --mail-type=FAIL,BEGIN,END
#SBATCH --error=%x-%J-%u.err
#SBATCH --output=%x-%J-%u.out
module --force purge
ml biocontainers drep
dRep compare compare_out -g tests/genomes/*
dRep dereplicate dereplicate_out -g tests/genomes/*
drop-seq
Link to section 'Introduction' of 'drop-seq' Introduction
Drop-seq are java tools for analyzing Drop-seq data.
Home page: https://github.com/broadinstitute/Drop-seq
Link to section 'Versions' of 'drop-seq' Versions
- 2.5.2
Link to section 'Commands' of 'drop-seq' Commands
- AssignCellsToSamples
- BamTagHistogram
- BamTagOfTagCounts
- BaseDistributionAtReadPosition
- BipartiteRabiesVirusCollapse
- CensusSeq
- CollapseBarcodesInPlace
- CollapseTagWithContext
- CompareDropSeqAlignments
- ComputeUMISharing
- ConvertTagToReadGroup
- ConvertToRefFlat
- CountUnmatchedSampleIndices
- CreateIntervalsFiles
- CreateMetaCells
- CreateSnpIntervalFromVcf
- CsiAnalysis
- DetectBeadSubstitutionErrors
- DetectBeadSynthesisErrors
- DetectDoublets
- DigitalExpression
- DownsampleBamByTag
- DownsampleTranscriptsAndQuantiles
- Drop-seq_Alignment_Cookbook.pdf
- Drop-seq_alignment.sh
- FilterBam
- FilterBamByGeneFunction
- FilterBamByTag
- FilterDge
- FilterGtf
- FilterValidRabiesBarcodes
- GatherGeneGCLength
- GatherMolecularBarcodeDistributionByGene
- GatherReadQualityMetrics
- GenotypeSperm
- MaskReferenceSequence
- MergeDgeSparse
- PolyATrimmer
- ReduceGtf
- RollCall
- SelectCellsByNumTranscripts
- SignTest
- SingleCellRnaSeqMetricsCollector
- SpermSeqMarkDuplicates
- SplitBamByCell
- TagBam
- TagBamWithReadSequenceExtended
- TagReadWithGeneExonFunction
- TagReadWithGeneFunction
- TagReadWithInterval
- TagReadWithRabiesBarcodes
- TrimStartingSequence
- ValidateAlignedSam
- ValidateReference
- create_Drop-seq_reference_metadata.sh
Link to section 'Module' of 'drop-seq' Module
You can load the modules by:
module load biocontainers
module load drop-seq
Link to section 'Example job' of 'drop-seq' Example job
Using #!/bin/sh -l
as shebang in the slurm job script will cause the failure of some biocontainer modules. Please use #!/bin/bash
instead.
To run drop-seq on our clusters:
#!/bin/bash
#SBATCH -A myallocation # Allocation name
#SBATCH -t 1:00:00
#SBATCH -N 1
#SBATCH -n 1
#SBATCH --job-name=drop-seq
#SBATCH --mail-type=FAIL,BEGIN,END
#SBATCH --error=%x-%J-%u.err
#SBATCH --output=%x-%J-%u.out
module --force purge
ml biocontainers drop-seq
dropest
Link to section 'Introduction' of 'dropest' Introduction
Dropest
is a pipeline for initial analysis of droplet-based single-cell RNA-seq data.
For more information, please check its website: https://biocontainers.pro/tools/dropest and its home page on Github.
Link to section 'Versions' of 'dropest' Versions
- 0.8.6
Link to section 'Commands' of 'dropest' Commands
- dropest
- droptag
- dropReport.Rsc
- R
- Rscript
Link to section 'Module' of 'dropest' Module
You can load the modules by:
module load biocontainers
module load dropest
Link to section 'Example job' of 'dropest' Example job
Using #!/bin/sh -l
as shebang in the slurm job script will cause the failure of some biocontainer modules. Please use #!/bin/bash
instead.
To run Dropest on our clusters:
#!/bin/bash
#SBATCH -A myallocation # Allocation name
#SBATCH -t 1:00:00
#SBATCH -N 1
#SBATCH -n 1
#SBATCH --job-name=dropest
#SBATCH --mail-type=FAIL,BEGIN,END
#SBATCH --error=%x-%J-%u.err
#SBATCH --output=%x-%J-%u.out
module --force purge
ml biocontainers dropest
dropest -f -c 10x.xml -C 1200 neurons_900_possorted_genome_bam.bam
dsuite
Link to section 'Introduction' of 'dsuite' Introduction
Dsuite
is a fast C++ implementation, allowing genome scale calculations of the D and f4-ratio statistics across all combinations of tens or hundreds of populations or species directly from a variant call format (VCF) file.
For more information, please check its home page on Github.
Link to section 'Versions' of 'dsuite' Versions
- 0.4.r43
- 0.5.r44
Link to section 'Commands' of 'dsuite' Commands
- Dsuite
- dtools.py
- DtriosParallel
Link to section 'Module' of 'dsuite' Module
You can load the modules by:
module load biocontainers
module load dsuite
Link to section 'Example job' of 'dsuite' Example job
Using #!/bin/sh -l
as shebang in the slurm job script will cause the failure of some biocontainer modules. Please use #!/bin/bash
instead.
To run Dsuite on our clusters:
#!/bin/bash
#SBATCH -A myallocation # Allocation name
#SBATCH -t 1:00:00
#SBATCH -N 1
#SBATCH -n 4
#SBATCH --job-name=dsuite
#SBATCH --mail-type=FAIL,BEGIN,END
#SBATCH --error=%x-%J-%u.err
#SBATCH --output=%x-%J-%u.out
module --force purge
ml biocontainers dsuite
Dsuite Dtrios -c -n no_geneflow -t simulated_tree_no_geneflow.nwk chr1_no_geneflow.vcf.gz species_sets.txt
easysfs
Link to section 'Introduction' of 'easysfs' Introduction
easySFS
is a tool for the effective selection of population size projection for construction of the site frequency spectrum.
For more information, please check its home page on Github.
Link to section 'Versions' of 'easysfs' Versions
- 1.0
Link to section 'Commands' of 'easysfs' Commands
- easySFS.py
Link to section 'Module' of 'easysfs' Module
You can load the modules by:
module load biocontainers
module load easysfs
Link to section 'Example job' of 'easysfs' Example job
Using #!/bin/sh -l
as shebang in the slurm job script will cause the failure of some biocontainer modules. Please use #!/bin/bash
instead.
To run easySFS on our clusters:
#!/bin/bash
#SBATCH -A myallocation # Allocation name
#SBATCH -t 1:00:00
#SBATCH -N 1
#SBATCH -n 1
#SBATCH --job-name=easysfs
#SBATCH --mail-type=FAIL,BEGIN,END
#SBATCH --error=%x-%J-%u.err
#SBATCH --output=%x-%J-%u.out
module --force purge
ml biocontainers easysfs
easySFS.py -i example_files/wcs_1200.vcf -p example_files/wcs_pops.txt --preview -a
easySFS.py -i example_files/wcs_1200.vcf -p example_files/wcs_pops.txt -a --proj=7,7
edta
Link to section 'Introduction' of 'edta' Introduction
Edta
is is developed for automated whole-genome de-novo TE annotation and benchmarking the annotation performance of TE libraries.
For more information, please check its website: https://biocontainers.pro/tools/edta and its home page on Github.
Note: Running EDTA, please use the command like this: EDTA.pl [OPTIONS] DO NOT call it 'perl EDTA.pl'
Link to section 'Versions' of 'edta' Versions
- 1.9.6
- 2.0.0
Link to section 'Commands' of 'edta' Commands
- EDTA.pl
- EDTA_processI.pl
- EDTA_raw.pl
- FET.pl
- bdf2gdfont.pl
- buildRMLibFromEMBL.pl
- buildSummary.pl
- calcDivergenceFromAlign.pl
- cd-hit-2d-para.pl
- cd-hit-clstr_2_blm8.pl
- cd-hit-div.pl
- cd-hit-para.pl
- check_result.pl
- clstr2tree.pl
- clstr2txt.pl
- clstr2xml.pl
- clstr_cut.pl
- clstr_list.pl
- clstr_list_sort.pl
- clstr_merge.pl
- clstr_merge_noorder.pl
- clstr_quality_eval.pl
- clstr_quality_eval_by_link.pl
- clstr_reduce.pl
- clstr_renumber.pl
- clstr_rep.pl
- clstr_reps_faa_rev.pl
- clstr_rev.pl
- clstr_select.pl
- clstr_select_rep.pl
- clstr_size_histogram.pl
- clstr_size_stat.pl
- clstr_sort_by.pl
- clstr_sort_prot_by.pl
- clstr_sql_tbl.pl
- clstr_sql_tbl_sort.pl
- convert_MGEScan3.0.pl
- convert_ltr_struc.pl
- convert_ltrdetector.pl
- createRepeatLandscape.pl
- down_tRNA.pl
- dupliconToSVG.pl
- filter_rt.pl
- genome_plot.pl
- genome_plot2.pl
- genome_plot_svg.pl
- getRepeatMaskerBatch.pl
- legacy_blast.pl
- lib-test.pl
- make_multi_seq.pl
- maskFile.pl
- plot_2d.pl
- plot_len1.pl
- rmOut2Fasta.pl
- rmOutToGFF3.pl
- rmToUCSCTables.pl
- update_blastdb.pl
- viewMSA.pl
- wublastToCrossmatch.pl
Link to section 'Module' of 'edta' Module
You can load the modules by:
module load biocontainers
module load edta
Link to section 'Example job' of 'edta' Example job
Using #!/bin/sh -l
as shebang in the slurm job script will cause the failure of some biocontainer modules. Please use #!/bin/bash
instead.
To run Edta on our clusters:
#!/bin/bash
#SBATCH -A myallocation # Allocation name
#SBATCH -t 1:00:00
#SBATCH -N 1
#SBATCH -n 10
#SBATCH --job-name=edta
#SBATCH --mail-type=FAIL,BEGIN,END
#SBATCH --error=%x-%J-%u.err
#SBATCH --output=%x-%J-%u.out
module --force purge
ml biocontainers edta
EDTA.pl --genome genome.fa --cds genome.cds.fa --curatedlib EDTA/database/rice6.9.5.liban --exclude genome.exclude.bed --overwrite 1 --sensitive 1 --anno 1 --evaluate 1 --threads 10
eggnog-mapper
Link to section 'Introduction' of 'eggnog-mapper' Introduction
Eggnog-mapper
is a tool for fast functional annotation of novel sequences.
For more information, please check its website: https://biocontainers.pro/tools/eggnog-mapper and its home page on Github.
Link to section 'Versions' of 'eggnog-mapper' Versions
- 2.1.7
Link to section 'Commands' of 'eggnog-mapper' Commands
- create_dbs.py
- download_eggnog_data.py
- emapper.py
- hmm_mapper.py
- hmm_server.py
- hmm_worker.py
- vba_extract.py
Link to section 'Module' of 'eggnog-mapper' Module
You can load the modules by:
module load biocontainers
module load eggnog-mapper
Link to section 'Example job' of 'eggnog-mapper' Example job
Using #!/bin/sh -l
as shebang in the slurm job script will cause the failure of some biocontainer modules. Please use #!/bin/bash
instead.
To run Eggnog-mapper on our clusters:
#!/bin/bash
#SBATCH -A myallocation # Allocation name
#SBATCH -t 1:00:00
#SBATCH -N 1
#SBATCH -n 24
#SBATCH --job-name=eggnog-mapper
#SBATCH --mail-type=FAIL,BEGIN,END
#SBATCH --error=%x-%J-%u.err
#SBATCH --output=%x-%J-%u.out
module --force purge
ml biocontainers eggnog-mapper
emapper.py -i proteins.faa --cpu 24 -o protein.out
emapper.py -m diamond --itype CDS -i cDNA.fasta -o cdna.out --cpu 24
emboss
Link to section 'Introduction' of 'emboss' Introduction
Emboss
is "The European Molecular Biology Open Software Suite".
For more information, please check its website: https://biocontainers.pro/tools/emboss and its home page: http://emboss.open-bio.org.
Link to section 'Versions' of 'emboss' Versions
- 6.6.0
Link to section 'Commands' of 'emboss' Commands
- aaindexextract
- abiview
- acdc
- acdgalaxy
- acdlog
- acdpretty
- acdtable
- acdtrace
- acdvalid
- aligncopy
- aligncopypair
- antigenic
- assemblyget
- backtranambig
- backtranseq
- banana
- biosed
- btwisted
- cachedas
- cachedbfetch
- cacheebeyesearch
- cacheensembl
- cai
- chaos
- charge
- checktrans
- chips
- cirdna
- codcmp
- codcopy
- coderet
- compseq
- cons
- consambig
- cpgplot
- cpgreport
- cusp
- cutgextract
- cutseq
- dan
- dbiblast
- dbifasta
- dbiflat
- dbigcg
- dbtell
- dbxcompress
- dbxedam
- dbxfasta
- dbxflat
- dbxgcg
- dbxobo
- dbxreport
- dbxresource
- dbxstat
- dbxtax
- dbxuncompress
- degapseq
- density
- descseq
- diffseq
- distmat
- dotmatcher
- dotpath
- dottup
- dreg
- drfinddata
- drfindformat
- drfindid
- drfindresource
- drget
- drtext
- edamdef
- edamhasinput
- edamhasoutput
- edamisformat
- edamisid
- edamname
- edialign
- einverted
- embossdata
- embossupdate
- embossversion
- emma
- emowse
- entret
- epestfind
- eprimer3
- eprimer32
- equicktandem
- est2genome
- etandem
- extractalign
- extractfeat
- extractseq
- featcopy
- featmerge
- featreport
- feattext
- findkm
- freak
- fuzznuc
- fuzzpro
- fuzztran
- garnier
- geecee
- getorf
- godef
- goname
- helixturnhelix
- hmoment
- iep
- infoalign
- infoassembly
- infobase
- inforesidue
- infoseq
- isochore
- jaspextract
- jaspscan
- jembossctl
- lindna
- listor
- makenucseq
- makeprotseq
- marscan
- maskambignuc
- maskambigprot
- maskfeat
- maskseq
- matcher
- megamerger
- merger
- msbar
- mwcontam
- mwfilter
- needle
- needleall
- newcpgreport
- newcpgseek
- newseq
- nohtml
- noreturn
- nospace
- notab
- notseq
- nthseq
- nthseqset
- octanol
- oddcomp
- ontocount
- ontoget
- ontogetcommon
- ontogetdown
- ontogetobsolete
- ontogetroot
- ontogetsibs
- ontogetup
- ontoisobsolete
- ontotext
- palindrome
- pasteseq
- patmatdb
- patmatmotifs
- pepcoil
- pepdigest
- pepinfo
- pepnet
- pepstats
- pepwheel
- pepwindow
- pepwindowall
- plotcon
- plotorf
- polydot
- preg
- prettyplot
- prettyseq
- primersearch
- printsextract
- profit
- prophecy
- prophet
- prosextract
- pscan
- psiphi
- rebaseextract
- recoder
- redata
- refseqget
- remap
- restover
- restrict
- revseq
- runJemboss.sh
- seealso
- seqcount
- seqmatchall
- seqret
- seqretsetall
- seqretsplit
- seqxref
- seqxrefget
- servertell
- showalign
- showdb
- showfeat
- showorf
- showpep
- showseq
- showserver
- shuffleseq
- sigcleave
- silent
- sirna
- sixpack
- sizeseq
- skipredundant
- skipseq
- splitsource
- splitter
- stretcher
- stssearch
- supermatcher
- syco
- taxget
- taxgetdown
- taxgetrank
- taxgetspecies
- taxgetup
- tcode
- textget
- textsearch
- tfextract
- tfm
- tfscan
- tmap
- tranalign
- transeq
- trimest
- trimseq
- trimspace
- twofeat
- union
- urlget
- variationget
- vectorstrip
- water
- whichdb
- wobble
- wordcount
- wordfinder
- wordmatch
- wossdata
- wossinput
- wossname
- wossoperation
- wossoutput
- wossparam
- wosstopic
- xmlget
- xmltext
- yank
Link to section 'Module' of 'emboss' Module
You can load the modules by:
module load biocontainers
module load emboss
Link to section 'Example job' of 'emboss' Example job
Using #!/bin/sh -l
as shebang in the slurm job script will cause the failure of some biocontainer modules. Please use #!/bin/bash
instead.
To run Emboss on our clusters:
#!/bin/bash
#SBATCH -A myallocation # Allocation name
#SBATCH -t 1:00:00
#SBATCH -N 1
#SBATCH -n 1
#SBATCH --job-name=emboss
#SBATCH --mail-type=FAIL,BEGIN,END
#SBATCH --error=%x-%J-%u.err
#SBATCH --output=%x-%J-%u.out
module --force purge
ml biocontainers emboss
ensembl-vep
Link to section 'Introduction' of 'ensembl-vep' Introduction
Ensembl-vep(Ensembl Variant Effect Predictor) predicts the functional effects of genomic variants.
Docker hub: https://hub.docker.com/r/ensemblorg/ensembl-vep
Home page: https://github.com/Ensembl/ensembl-vep
Link to section 'Versions' of 'ensembl-vep' Versions
- 106.1
- 107.0
- 108.2
Link to section 'Commands' of 'ensembl-vep' Commands
- vep
- haplo
- variant_recoder
Link to section 'Module' of 'ensembl-vep' Module
You can load the modules by:
module load biocontainers
module load ensembl-vep
Link to section 'Example job' of 'ensembl-vep' Example job
Using #!/bin/sh -l
as shebang in the slurm job script will cause the failure of some biocontainer modules. Please use #!/bin/bash
instead.
To run ensembl-vep on our clusters:
#!/bin/bash
#SBATCH -A myallocation # Allocation name
#SBATCH -t 1:00:00
#SBATCH -N 1
#SBATCH -n 1
#SBATCH --job-name=ensembl-vep
#SBATCH --mail-type=FAIL,BEGIN,END
#SBATCH --error=%x-%J-%u.err
#SBATCH --output=%x-%J-%u.out
module --force purge
ml biocontainers ensembl-vep
haplo -i bos_taurus_UMD3.1.vcf -o out.txt
epic2
Link to section 'Introduction' of 'epic2' Introduction
Epic2
is an ultraperformant Chip-Seq broad domain finder based on SICER.
For more information, please check its website: https://biocontainers.pro/tools/epic2 and its home page on Github.
Link to section 'Versions' of 'epic2' Versions
- 0.0.51
- 0.0.52
Link to section 'Commands' of 'epic2' Commands
- epic2
- epic2-bw
- epic2-df
Link to section 'Module' of 'epic2' Module
You can load the modules by:
module load biocontainers
module load epic2
Link to section 'Example job' of 'epic2' Example job
Using #!/bin/sh -l
as shebang in the slurm job script will cause the failure of some biocontainer modules. Please use #!/bin/bash
instead.
To run Epic2 on our clusters:
#!/bin/bash
#SBATCH -A myallocation # Allocation name
#SBATCH -t 1:00:00
#SBATCH -N 1
#SBATCH -n 1
#SBATCH --job-name=epic2
#SBATCH --mail-type=FAIL,BEGIN,END
#SBATCH --error=%x-%J-%u.err
#SBATCH --output=%x-%J-%u.out
module --force purge
ml biocontainers epic2
epic2 -t /examples/test.bed.gz \
-c /examples/control.bed.gz \
> deleteme.txt
evidencemodeler
Link to section 'Introduction' of 'evidencemodeler' Introduction
Evidencemodeler
is a software combines ab intio gene predictions and protein and transcript alignments into weighted consensus gene structures.
For more information, please check its website: https://biocontainers.pro/tools/evidencemodeler and its home page on Github.
Link to section 'Versions' of 'evidencemodeler' Versions
- 1.1.1
Link to section 'Commands' of 'evidencemodeler' Commands
- evidence_modeler.pl
- BPbtab.pl
- EVMLite.pl
- EVM_to_GFF3.pl
- convert_EVM_outputs_to_GFF3.pl
- create_weights_file.pl
- execute_EVM_commands.pl
- extract_complete_proteins.pl
- gff3_file_to_proteins.pl
- gff3_gene_prediction_file_validator.pl
- gff_range_retriever.pl
- partition_EVM_inputs.pl
- recombine_EVM_partial_outputs.pl
- summarize_btab_tophits.pl
- write_EVM_commands.pl
Link to section 'Module' of 'evidencemodeler' Module
You can load the modules by:
module load biocontainers
module load evidencemodeler
Link to section 'Example job' of 'evidencemodeler' Example job
Using #!/bin/sh -l
as shebang in the slurm job script will cause the failure of some biocontainer modules. Please use #!/bin/bash
instead.
To run Evidencemodeler on our clusters:
#!/bin/bash
#SBATCH -A myallocation # Allocation name
#SBATCH -t 1:00:00
#SBATCH -N 1
#SBATCH -n 1
#SBATCH --job-name=evidencemodeler
#SBATCH --mail-type=FAIL,BEGIN,END
#SBATCH --error=%x-%J-%u.err
#SBATCH --output=%x-%J-%u.out
module --force purge
ml biocontainers evidencemodeler
evidence_modeler.pl --genome genome.fasta \
--weights weights.txt \
--gene_predictions gene_predictions.gff3 \
--protein_alignments protein_alignments.gff3 \
--transcript_alignments transcript_alignments.gff3 \
> evm.out
exonerate
Link to section 'Introduction' of 'exonerate' Introduction
Exonerate
is a generic tool for pairwise sequence comparison/alignment.
For more information, please check its home page: https://www.ebi.ac.uk/about/vertebrate-genomics/software/exonerate.
Link to section 'Versions' of 'exonerate' Versions
- 2.4.0
Link to section 'Commands' of 'exonerate' Commands
- exonerate
Link to section 'Module' of 'exonerate' Module
You can load the modules by:
module load biocontainers
module load exonerate
Link to section 'Example job' of 'exonerate' Example job
Using #!/bin/sh -l
as shebang in the slurm job script will cause the failure of some biocontainer modules. Please use #!/bin/bash
instead.
To run Exonerate on our clusters:
#!/bin/bash
#SBATCH -A myallocation # Allocation name
#SBATCH -t 1:00:00
#SBATCH -N 1
#SBATCH -n 1
#SBATCH --job-name=exonerate
#SBATCH --mail-type=FAIL,BEGIN,END
#SBATCH --error=%x-%J-%u.err
#SBATCH --output=%x-%J-%u.out
module --force purge
ml biocontainers exonerate
exonerate -m genome2genome cms.fasta cmm.fasta > cm_vs_cs.out
expansionhunter
Link to section 'Introduction' of 'expansionhunter' Introduction
Expansion Hunter: a tool for estimating repeat sizes.
BioContainers: https://biocontainers.pro/tools/expansionhunter
Home page: https://github.com/Illumina/ExpansionHunter
Link to section 'Versions' of 'expansionhunter' Versions
- 4.0.2
Link to section 'Commands' of 'expansionhunter' Commands
- ExpansionHunter
Link to section 'Module' of 'expansionhunter' Module
You can load the modules by:
module load biocontainers
module load expansionhunter
Link to section 'Example job' of 'expansionhunter' Example job
Using #!/bin/sh -l
as shebang in the slurm job script will cause the failure of some biocontainer modules. Please use #!/bin/bash
instead.
To run expansionhunter on our clusters:
#!/bin/bash
#SBATCH -A myallocation # Allocation name
#SBATCH -t 1:00:00
#SBATCH -N 1
#SBATCH -n 1
#SBATCH --job-name=expansionhunter
#SBATCH --mail-type=FAIL,BEGIN,END
#SBATCH --error=%x-%J-%u.err
#SBATCH --output=%x-%J-%u.out
module --force purge
ml biocontainers expansionhunter
fasta3
Link to section 'Introduction' of 'fasta3' Introduction
Fasta3
is a suite of programs for searching nucleotide or protein databases with a query sequence.
For more information, please check its website: https://biocontainers.pro/tools/fasta3 and its home page on Github.
Link to section 'Versions' of 'fasta3' Versions
- 36.3.8
Link to section 'Commands' of 'fasta3' Commands
- fasta36
- fastf36
- fastm36
- fasts36
- fastx36
- fasty36
- ggsearch36
- glsearch36
- lalign36
- ssearch36
- tfastf36
- tfastm36
- tfasts36
- tfastx36
- tfasty36
Link to section 'Module' of 'fasta3' Module
You can load the modules by:
module load biocontainers
module load fasta3
Link to section 'Example job' of 'fasta3' Example job
Using #!/bin/sh -l
as shebang in the slurm job script will cause the failure of some biocontainer modules. Please use #!/bin/bash
instead.
To run Fasta3 on our clusters:
#!/bin/bash
#SBATCH -A myallocation # Allocation name
#SBATCH -t 1:00:00
#SBATCH -N 1
#SBATCH -n 1
#SBATCH --job-name=fasta3
#SBATCH --mail-type=FAIL,BEGIN,END
#SBATCH --error=%x-%J-%u.err
#SBATCH --output=%x-%J-%u.out
module --force purge
ml biocontainers fasta3
fasta36 input.fasta genome.fasta
fastani
Link to section 'Introduction' of 'fastani' Introduction
FastANI
is developed for fast alignment-free computation of whole-genome Average Nucleotide Identity (ANI).
For more information, please check its website: https://biocontainers.pro/tools/fastani and its home page on Github.
Link to section 'Versions' of 'fastani' Versions
- 1.32
- 1.33
Link to section 'Commands' of 'fastani' Commands
- fastANI
Link to section 'Module' of 'fastani' Module
You can load the modules by:
module load biocontainers
module load fastani
Link to section 'Example job' of 'fastani' Example job
Using #!/bin/sh -l
as shebang in the slurm job script will cause the failure of some biocontainer modules. Please use #!/bin/bash
instead.
To run FastANI on our clusters:
#!/bin/bash
#SBATCH -A myallocation # Allocation name
#SBATCH -t 1:00:00
#SBATCH -N 1
#SBATCH -n 1
#SBATCH --job-name=fastani
#SBATCH --mail-type=FAIL,BEGIN,END
#SBATCH --error=%x-%J-%u.err
#SBATCH --output=%x-%J-%u.out
module --force purge
ml biocontainers fastani
fastANI -q cmm.fasta -r cms.fasta -o cm_cs_out
fastANI -q cmm.fasta -r cms.fasta --visualize -o cm_cs_visualize_out
fastp
Link to section 'Introduction' of 'fastp' Introduction
Fastp
is an ultra-fast all-in-one FASTQ preprocessor (QC/adapters/trimming/filtering/splitting/merging, etc).
For more information, please check its website: https://biocontainers.pro/tools/fastp and its home page on Github.
Link to section 'Versions' of 'fastp' Versions
- 0.20.1
- 0.23.2
Link to section 'Commands' of 'fastp' Commands
- fastp
Link to section 'Module' of 'fastp' Module
You can load the modules by:
module load biocontainers
module load fastp
Link to section 'Example job' of 'fastp' Example job
Using #!/bin/sh -l
as shebang in the slurm job script will cause the failure of some biocontainer modules. Please use #!/bin/bash
instead.
To run Fastp on our clusters:
#!/bin/bash
#SBATCH -A myallocation # Allocation name
#SBATCH -t 1:00:00
#SBATCH -N 1
#SBATCH -n 1
#SBATCH --job-name=fastp
#SBATCH --mail-type=FAIL,BEGIN,END
#SBATCH --error=%x-%J-%u.err
#SBATCH --output=%x-%J-%u.out
module --force purge
ml biocontainers fastp
fastp -i input_1.fastq -I input_2.fastq -o out.R1.fq.gz -O out.R2.fq.gz
fastq-scan
Link to section 'Introduction' of 'fastq-scan' Introduction
Fastq-scan reads a FASTQ from STDIN and outputs summary statistics (read lengths, per-read qualities, per-base qualities) in JSON format.
Docker hub: https://hub.docker.com/r/staphb/fastq-scan
Home page: https://github.com/rpetit3/fastq-scan
Link to section 'Versions' of 'fastq-scan' Versions
- 1.0.0
Link to section 'Commands' of 'fastq-scan' Commands
- fastq-scan
Link to section 'Module' of 'fastq-scan' Module
You can load the modules by:
module load biocontainers
module load fastq-scan
Link to section 'Example job' of 'fastq-scan' Example job
Using #!/bin/sh -l
as shebang in the slurm job script will cause the failure of some biocontainer modules. Please use #!/bin/bash
instead.
To run fastq-scan on our clusters:
#!/bin/bash
#SBATCH -A myallocation # Allocation name
#SBATCH -t 1:00:00
#SBATCH -N 1
#SBATCH -n 1
#SBATCH --job-name=fastq-scan
#SBATCH --mail-type=FAIL,BEGIN,END
#SBATCH --error=%x-%J-%u.err
#SBATCH --output=%x-%J-%u.out
module --force purge
ml biocontainers fastq-scan
cat example-q33.fq | fastq-scan -g 150000
fastq_pair
Link to section 'Introduction' of 'fastq_pair' Introduction
Fastq_pair
is used to match up paired end fastq files quickly and efficiently.
For more information, please check its website: https://biocontainers.pro/tools/fastq_pair and its home page on Github.
Link to section 'Versions' of 'fastq_pair' Versions
- 1.0
Link to section 'Commands' of 'fastq_pair' Commands
- fastq_pair
Link to section 'Module' of 'fastq_pair' Module
You can load the modules by:
module load biocontainers
module load fastq_pair
Link to section 'Example job' of 'fastq_pair' Example job
Using #!/bin/sh -l
as shebang in the slurm job script will cause the failure of some biocontainer modules. Please use #!/bin/bash
instead.
To run Fastq_pair on our clusters:
#!/bin/bash
#SBATCH -A myallocation # Allocation name
#SBATCH -t 1:00:00
#SBATCH -N 1
#SBATCH -n 1
#SBATCH --job-name=fastq_pair
#SBATCH --mail-type=FAIL,BEGIN,END
#SBATCH --error=%x-%J-%u.err
#SBATCH --output=%x-%J-%u.out
module --force purge
ml biocontainers fastq_pair
fastq_pair seq_1.fastq seq_2.fastq
fastqc
Link to section 'Introduction' of 'fastqc' Introduction
FastQC
aims to provide a simple way to do some quality control checks on raw sequence data coming from high throughput sequencing pipelines. It provides a modular set of analyses which you can use to give a quick impression of whether your data has any problems of which you should be aware before doing any further analysis.
For more information, please check its website: https://biocontainers.pro/tools/fastqc and its home page: https://www.bioinformatics.babraham.ac.uk/projects/fastqc/.
Link to section 'Versions' of 'fastqc' Versions
- 0.11.9
Link to section 'Commands' of 'fastqc' Commands
- fastqc
Link to section 'Module' of 'fastqc' Module
You can load the modules by:
module load biocontainers
module load fastqc
Link to section 'Example job' of 'fastqc' Example job
Using #!/bin/sh -l
as shebang in the slurm job script will cause the failure of some biocontainer modules. Please use #!/bin/bash
instead.
To run Fastqc on our clusters:
#!/bin/bash
#SBATCH -A myallocation # Allocation name
#SBATCH -t 1:00:00
#SBATCH -N 1
#SBATCH -n 4
#SBATCH --job-name=fastqc
#SBATCH --mail-type=FAIL,BEGIN,END
#SBATCH --error=%x-%J-%u.err
#SBATCH --output=%x-%J-%u.out
module --force purge
ml biocontainers fastqc
fastqc -o fastqc_out -t 4 FASTQ1 FASTQ2
fastspar
Link to section 'Introduction' of 'fastspar' Introduction
Fastspar
is a tool for rapid and scalable correlation estimation for compositional data.
For more information, please check its website: https://biocontainers.pro/tools/fastspar and its home page on Github.
Link to section 'Versions' of 'fastspar' Versions
- 1.0.0
Link to section 'Commands' of 'fastspar' Commands
- fastspar
- fastspar_bootstrap
- fastspar_pvalues
- fastspar_reduce
Link to section 'Module' of 'fastspar' Module
You can load the modules by:
module load biocontainers
module load fastspar
Link to section 'Example job' of 'fastspar' Example job
Using #!/bin/sh -l
as shebang in the slurm job script will cause the failure of some biocontainer modules. Please use #!/bin/bash
instead.
To run Fastspar on our clusters:
#!/bin/bash
#SBATCH -A myallocation # Allocation name
#SBATCH -t 1:00:00
#SBATCH -N 1
#SBATCH -n 1
#SBATCH --job-name=fastspar
#SBATCH --mail-type=FAIL,BEGIN,END
#SBATCH --error=%x-%J-%u.err
#SBATCH --output=%x-%J-%u.out
module --force purge
ml biocontainers fastspar
faststructure
Link to section 'Introduction' of 'faststructure' Introduction
fastStructure
is an algorithm for inferring population structure from large SNP genotype data. It is based on a variational Bayesian framework for posterior inference and is written in Python2.x.
Note: programs "structure.py", "chooseK.py" and "distruct.py" are standalone executable and should be called by name directly ("structure.py", etc). DO NOT invoke them as "python structure.py", or as "python /usr/local/bin/structure.py", this will not work!
Note: This containers lacks X11 libraries, so GUI plots with 'distruct.py' do not work. Instead, we need to tell the underlying Matplotlib to use a non-interactive plotting backend (to file). The easiest and most flexible way is to use the MPLBACKEND environment variable: env MPLBACKEND="svg" distruct.py --output myplot.svg .......
Available backends in this container: Backend Filetypes Description agg png raster graphics – high quality PNG output ps ps eps vector graphics – Postscript output pdf pdf vector graphics – Portable Document Format svg svg vector graphics – Scalable Vector Graphics Default MPLBACKEND="agg" (for PNG format output).
For more information, please check its website: https://biocontainers.pro/tools/faststructure and its home page on Github.
Link to section 'Versions' of 'faststructure' Versions
- 1.0-py27
Link to section 'Commands' of 'faststructure' Commands
- structure.py
- chooseK.py
- distruct.py
Link to section 'Module' of 'faststructure' Module
You can load the modules by:
module load biocontainers
module load faststructure
Link to section 'Example job' of 'faststructure' Example job
Using #!/bin/sh -l
as shebang in the slurm job script will cause the failure of some biocontainer modules. Please use #!/bin/bash
instead.
To run fastStructure on our clusters:
#!/bin/bash
#SBATCH -A myallocation # Allocation name
#SBATCH -t 1:00:00
#SBATCH -N 1
#SBATCH -n 1
#SBATCH --job-name=faststructure
#SBATCH --mail-type=FAIL,BEGIN,END
#SBATCH --error=%x-%J-%u.err
#SBATCH --output=%x-%J-%u.out
module --force purge
ml biocontainers faststructure
fasttree
Link to section 'Introduction' of 'fasttree' Introduction
FastTree
infers approximately-maximum-likelihood phylogenetic trees from alignments of nucleotide or protein sequences. FastTree can handle alignments with up to a million of sequences in a reasonable amount of time and memory.
Detailed usage can be found here: http://www.microbesonline.org/fasttree/
- 2.1.10
- 2.1.11
Link to section 'Commands' of 'fasttree' Commands
- fasttree
- FastTree
- FastTreeMP
fasttree
and FastTree
are the same program, and they only support one CPU. If you want to use multiple CPUs, please use FastTreeMP
and also set the OMP_NUM_THREADS
to the number of cores you requested.
Link to section 'Module' of 'fasttree' Module
You can load the modules by:
module load biocontainers
module load fasttree
Link to section 'Example job using single CPU' of 'fasttree' Example job using single CPU
Using #!/bin/sh -l
as shebang in the slurm job script will cause the failure of some biocontainer modules. Please use #!/bin/bash
instead.
To run FastTree on our our clusters:
#!/bin/bash
#SBATCH -A myallocation # Allocation name
#SBATCH -t 20:00:00
#SBATCH -N 1
#SBATCH -n 1
#SBATCH --job-name=fasttree
#SBATCH --mail-type=FAIL,BEGIN,END
#SBATCH --error=%x-%J-%u.err
#SBATCH --output=%x-%J-%u.out
module --force purge
ml biocontainers fasttree
FastTree alignmentfile > treefile
Link to section 'Example job using multiple CPUs' of 'fasttree' Example job using multiple CPUs
Using #!/bin/sh -l
as shebang in the slurm job script will cause the failure of some biocontainer modules. Please use #!/bin/bash
instead.
To run FastTree on our our clusters:
#!/bin/bash
#SBATCH -A myallocation # Allocation name
#SBATCH -t 20:00:00
#SBATCH -N 1
#SBATCH -n 24
#SBATCH --job-name=FastTreeMP
#SBATCH --mail-type=FAIL,BEGIN,END
#SBATCH --error=%x-%J-%u.err
#SBATCH --output=%x-%J-%u.out
module --force purge
ml biocontainers fasttree
export OMP_NUM_THREADS=24
FastTreeMP alignmentfile > treefile
fastx_toolkit
Link to section 'Introduction' of 'fastx_toolkit' Introduction
FASTX-Toolkit
is a collection of command line tools for Short-Reads FASTA/FASTQ files preprocessing.
For more information, please check its website: https://biocontainers.pro/tools/fastx_toolkit and its home page on Github.
Link to section 'Versions' of 'fastx_toolkit' Versions
- 0.0.14
Link to section 'Commands' of 'fastx_toolkit' Commands
- fasta_clipping_histogram.pl
- fasta_formatter
- fasta_nucleotide_changer
- fastq_masker
- fastq_quality_boxplot_graph.sh
- fastq_quality_converter
- fastq_quality_filter
- fastq_quality_trimmer
- fastq_to_fasta
- fastx_artifacts_filter
- fastx_barcode_splitter.pl
- fastx_clipper
- fastx_collapser
- fastx_nucleotide_distribution_graph.sh
- fastx_nucleotide_distribution_line_graph.sh
- fastx_quality_stats
- fastx_renamer
- fastx_reverse_complement
- fastx_trimmer
- fastx_uncollapser
Link to section 'Module' of 'fastx_toolkit' Module
You can load the modules by:
module load biocontainers
module load fastx_toolkit
Link to section 'Example job' of 'fastx_toolkit' Example job
Using #!/bin/sh -l
as shebang in the slurm job script will cause the failure of some biocontainer modules. Please use #!/bin/bash
instead.
To run FASTX-Toolkit on our clusters:
#!/bin/bash
#SBATCH -A myallocation # Allocation name
#SBATCH -t 1:00:00
#SBATCH -N 1
#SBATCH -n 1
#SBATCH --job-name=fastx_toolkit
#SBATCH --mail-type=FAIL,BEGIN,END
#SBATCH --error=%x-%J-%u.err
#SBATCH --output=%x-%J-%u.out
module --force purge
ml biocontainers fastx_toolkit
filtlong
Link to section 'Introduction' of 'filtlong' Introduction
Filtlong
is a tool for filtering long reads by quality. It can take a set of long reads and produce a smaller, better subset. It uses both read length (longer is better) and read identity (higher is better) when choosing which reads pass the filter.
For more information, please check its website: https://biocontainers.pro/tools/filtlong and its home page on Github.
Link to section 'Versions' of 'filtlong' Versions
- 0.2.1
Link to section 'Commands' of 'filtlong' Commands
- filtlong
Link to section 'Module' of 'filtlong' Module
You can load the modules by:
module load biocontainers
module load filtlong
Link to section 'Example job' of 'filtlong' Example job
Using #!/bin/sh -l
as shebang in the slurm job script will cause the failure of some biocontainer modules. Please use #!/bin/bash
instead.
To run Filtlong on our clusters:
#!/bin/bash
#SBATCH -A myallocation # Allocation name
#SBATCH -t 1:00:00
#SBATCH -N 1
#SBATCH -n 1
#SBATCH --job-name=filtlong
#SBATCH --mail-type=FAIL,BEGIN,END
#SBATCH --error=%x-%J-%u.err
#SBATCH --output=%x-%J-%u.out
module --force purge
ml biocontainers filtlong
flye
Link to section 'Introduction' of 'flye' Introduction
Flye
: Fast and accurate de novo assembler for single molecule sequencing reads.
For more information, please check its website: https://biocontainers.pro/tools/flye and its home page on Github.
Link to section 'Versions' of 'flye' Versions
- 2.9.1
- 2.9
Link to section 'Commands' of 'flye' Commands
- flye
Link to section 'Module' of 'flye' Module
You can load the modules by:
module load biocontainers
module load flye
Link to section 'Example job' of 'flye' Example job
Using #!/bin/sh -l
as shebang in the slurm job script will cause the failure of some biocontainer modules. Please use #!/bin/bash
instead.
To run Flye on our clusters:
#!/bin/bash
#SBATCH -A myallocation # Allocation name
#SBATCH -t 1:00:00
#SBATCH -N 1
#SBATCH -n 12
#SBATCH --job-name=flye
#SBATCH --mail-type=FAIL,BEGIN,END
#SBATCH --error=%x-%J-%u.err
#SBATCH --output=%x-%J-%u.out
module --force purge
ml biocontainers flye
flye --pacbio-raw E.coli_PacBio_40x.fasta --out-dir out_pacbio --threads 12
flye --nano-raw Loman_E.coli_MAP006-1_2D_50x.fasta --out-dir out_nano --threads 12
fraggenescan
Link to section 'Introduction' of 'fraggenescan' Introduction
Fraggenescan
is an application for finding (fragmented) genes in short reads. It can also be applied to predict prokaryotic genes in incomplete assemblies or complete genomes.
For more information, please check its website: https://biocontainers.pro/tools/fraggenescan and its home page on Github.
Link to section 'Versions' of 'fraggenescan' Versions
- 1.31
Link to section 'Commands' of 'fraggenescan' Commands
- FragGeneScan
- run_FragGeneScan.pl
Link to section 'Module' of 'fraggenescan' Module
You can load the modules by:
module load biocontainers
module load fraggenescan
Link to section 'Example job' of 'fraggenescan' Example job
Using #!/bin/sh -l
as shebang in the slurm job script will cause the failure of some biocontainer modules. Please use #!/bin/bash
instead.
To run Fraggenescan on our clusters:
#!/bin/bash
#SBATCH -A myallocation # Allocation name
#SBATCH -t 1:00:00
#SBATCH -N 1
#SBATCH -n 1
#SBATCH --job-name=fraggenescan
#SBATCH --mail-type=FAIL,BEGIN,END
#SBATCH --error=%x-%J-%u.err
#SBATCH --output=%x-%J-%u.out
module --force purge
ml biocontainers fraggenescan
FragGeneScanRs -t 454_10 < example/NC_000913-454.fna > example/NC_000913-454.faa
fraggenescanrs
Link to section 'Introduction' of 'fraggenescanrs' Introduction
FragGeneScanRs is a better and faster Rust implementation of the FragGeneScan gene prediction model for short and error-prone reads. Its command line interface is backward compatible and adds extra features for more flexible usage. Compared to the original C implementation, shotgun metagenomic reads are processed up to 22 times faster using a single thread, with better scaling for multithreaded execution.
Home page: https://github.com/unipept/FragGeneScanRs
Link to section 'Versions' of 'fraggenescanrs' Versions
- 1.1.0
Link to section 'Commands' of 'fraggenescanrs' Commands
- FragGeneScanRs
Link to section 'Module' of 'fraggenescanrs' Module
You can load the modules by:
module load biocontainers
module load fraggenescanrs
Link to section 'Example job' of 'fraggenescanrs' Example job
Using #!/bin/sh -l
as shebang in the slurm job script will cause the failure of some biocontainer modules. Please use #!/bin/bash
instead.
To run fraggenescanrs on our clusters:
#!/bin/bash
#SBATCH -A myallocation # Allocation name
#SBATCH -t 1:00:00
#SBATCH -N 1
#SBATCH -n 1
#SBATCH --job-name=fraggenescanrs
#SBATCH --mail-type=FAIL,BEGIN,END
#SBATCH --error=%x-%J-%u.err
#SBATCH --output=%x-%J-%u.out
module --force purge
ml biocontainers fraggenescanrs
freebayes
Link to section 'Introduction' of 'freebayes' Introduction
Freebayes
is a Bayesian genetic variant detector designed to find small polymorphisms, specifically SNPs (single-nucleotide polymorphisms), indels (insertions and deletions), MNPs (multi-nucleotide polymorphisms), and complex events (composite insertion and substitution events) smaller than the length of a short-read sequencing alignment.
For more information, please check its website: https://biocontainers.pro/tools/freebayes and its home page on Github.
Link to section 'Versions' of 'freebayes' Versions
- 1.3.5
- 1.3.6
Link to section 'Commands' of 'freebayes' Commands
- freebayes
- freebayes-parallel
Link to section 'Module' of 'freebayes' Module
You can load the modules by:
module load biocontainers
module load freebayes
Link to section 'Example job' of 'freebayes' Example job
Using #!/bin/sh -l
as shebang in the slurm job script will cause the failure of some biocontainer modules. Please use #!/bin/bash
instead.
To run Freebayes on our clusters:
#!/bin/bash
#SBATCH -A myallocation # Allocation name
#SBATCH -t 1:00:00
#SBATCH -N 1
#SBATCH -n 1
#SBATCH --job-name=freebayes
#SBATCH --mail-type=FAIL,BEGIN,END
#SBATCH --error=%x-%J-%u.err
#SBATCH --output=%x-%J-%u.out
module --force purge
ml biocontainers freebayes
freebayes -f ref.fa aln.cram >var.vcf
freyja
Link to section 'Introduction' of 'freyja' Introduction
Freyja is a tool to recover relative lineage abundances from mixed SARS-CoV-2 samples from a sequencing dataset (BAM aligned to the Hu-1 reference). The method uses lineage-determining mutational "barcodes" derived from the UShER global phylogenetic tree as a basis set to solve the constrained (unit sum, non-negative) de-mixing problem.
Docker hub: https://hub.docker.com/r/staphb/freyja
Home page: https://github.com/andersen-lab/Freyja
Link to section 'Versions' of 'freyja' Versions
- 1.3.11
Link to section 'Commands' of 'freyja' Commands
- freyja
Link to section 'Module' of 'freyja' Module
You can load the modules by:
module load biocontainers
module load freyja
Link to section 'Example job' of 'freyja' Example job
Using #!/bin/sh -l
as shebang in the slurm job script will cause the failure of some biocontainer modules. Please use #!/bin/bash
instead.
To run freyja on our clusters:
#!/bin/bash
#SBATCH -A myallocation # Allocation name
#SBATCH -t 1:00:00
#SBATCH -N 1
#SBATCH -n 1
#SBATCH --job-name=freyja
#SBATCH --mail-type=FAIL,BEGIN,END
#SBATCH --error=%x-%J-%u.err
#SBATCH --output=%x-%J-%u.out
module --force purge
ml biocontainers freyja
fseq
Link to section 'Introduction' of 'fseq' Introduction
Fseq
is a feature density estimator for high-throughput sequence tags.
For more information, please check its home page: https://fureylab.web.unc.edu/software/fseq/.
Link to section 'Versions' of 'fseq' Versions
- 2.0.3
Link to section 'Commands' of 'fseq' Commands
- fseq2
Link to section 'Module' of 'fseq' Module
You can load the modules by:
module load biocontainers
module load fseq
Link to section 'Example job' of 'fseq' Example job
Using #!/bin/sh -l
as shebang in the slurm job script will cause the failure of some biocontainer modules. Please use #!/bin/bash
instead.
To run Fseq on our clusters:
#!/bin/bash
#SBATCH -A myallocation # Allocation name
#SBATCH -t 1:00:00
#SBATCH -N 1
#SBATCH -n 1
#SBATCH --job-name=fseq
#SBATCH --mail-type=FAIL,BEGIN,END
#SBATCH --error=%x-%J-%u.err
#SBATCH --output=%x-%J-%u.out
module --force purge
ml biocontainers fseq
funannotate
Link to section 'Introduction' of 'funannotate' Introduction
Funannotate
is a genome prediction, annotation, and comparison software package.
Docker hub: https://hub.docker.com/r/nextgenusfs/funannotate and its home page on Github.
Link to section 'Versions' of 'funannotate' Versions
- 1.8.10
Link to section 'Commands' of 'funannotate' Commands
- funannotate
Link to section 'Module' of 'funannotate' Module
You can load the modules by:
module load biocontainers
module load funannotate
Link to section 'Example job' of 'funannotate' Example job
Using #!/bin/sh -l
as shebang in the slurm job script will cause the failure of some biocontainer modules. Please use #!/bin/bash
instead.
To run Funannotate on our clusters:
#!/bin/bash
#SBATCH -A myallocation # Allocation name
#SBATCH -t 1:00:00
#SBATCH -N 1
#SBATCH -n 12
#SBATCH --job-name=funannotate
#SBATCH --mail-type=FAIL,BEGIN,END
#SBATCH --error=%x-%J-%u.err
#SBATCH --output=%x-%J-%u.out
module --force purge
ml biocontainers funannotate
funannotate clean -i genome.fa -o genome_cleaned.fa
funannotate sort -i genome_cleaned.fa -o genome_cleaned_sorted.fa
funannotate predict -i genome_cleaned_sorted.fa -o predict_out --species "arabidopsis" --rna_bam RNAseq.bam --cpus 12
fwdpy11
Link to section 'Introduction' of 'fwdpy11' Introduction
Fwdpy11 is a Python package for forward-time population genetic simulation.
Docker hub: https://hub.docker.com/r/molpopgen/fwdpy11
Home page: https://github.com/molpopgen/fwdpy11
Link to section 'Versions' of 'fwdpy11' Versions
- 0.18.1
Link to section 'Commands' of 'fwdpy11' Commands
- python3
- python
Link to section 'Module' of 'fwdpy11' Module
You can load the modules by:
module load biocontainers
module load fwdpy11
Link to section 'Interactive job' of 'fwdpy11' Interactive job
To run fwdpy11 interactively on our clusters:
(base) UserID@bell-fe00:~ $ sinteractive -N1 -n12 -t4:00:00 -A myallocation
salloc: Granted job allocation 12345869
salloc: Waiting for resource configuration
salloc: Nodes bell-a008 are ready for job
(base) UserID@bell-a008:~ $ module load biocontainers fwdpy11
(base) UserID@bell-a008:~ $ python
Python 3.8.10 (default, Mar 15 2022, 12:22:08)
[GCC 9.4.0] on linux
Type "help", "copyright", "credits" or "license" for more information.
>>> import fwdpy11
>>> pop = fwdpy11.DiploidPopulation(100, 1000.0)
>>> print(f"N = {pop.N}, L = {pop.tables.genome_length}")
Link to section 'Batch job' of 'fwdpy11' Batch job
Using #!/bin/sh -l
as shebang in the slurm job script will cause the failure of some biocontainer modules. Please use #!/bin/bash
instead.
To run fwdpy11 on our clusters:
#!/bin/bash
#SBATCH -A myallocation # Allocation name
#SBATCH -t 1:00:00
#SBATCH -N 1
#SBATCH -n 1
#SBATCH --job-name=fwdpy11
#SBATCH --mail-type=FAIL,BEGIN,END
#SBATCH --error=%x-%J-%u.err
#SBATCH --output=%x-%J-%u.out
module --force purge
ml biocontainers fwdpy11
python script.py
gadma
Link to section 'Introduction' of 'gadma' Introduction
GADMA is a command-line tool. Basic pipeline presents a series of launches of the genetic algorithm followed by local search optimization and infers demographic history from the Allele Frequency Spectrum of multiple populations (up to three).
BioContainers: https://biocontainers.pro/tools/gadma
Home page: https://github.com/ctlab/GADMA
Link to section 'Versions' of 'gadma' Versions
- 2.0.0rc21
Link to section 'Commands' of 'gadma' Commands
- gadma
- python
- python3
Link to section 'Module' of 'gadma' Module
You can load the modules by:
module load biocontainers
module load gadma
Link to section 'Interactive job' of 'gadma' Interactive job
To run GADMA interactively on our clusters:
(base) UserID@bell-fe00:~ $ sinteractive -N1 -n12 -t4:00:00 -A myallocation
salloc: Granted job allocation 12345869
salloc: Waiting for resource configuration
salloc: Nodes bell-a008 are ready for job
(base) UserID@bell-a008:~ $ module load biocontainers gadma
(base) UserID@bell-a008:~ $ python
Python 3.8.13 | packaged by conda-forge | (default, Mar 25 2022, 06:04:10)
[GCC 10.3.0] on linux
Type "help", "copyright", "credits" or "license" for more information.
>>> from gadma import *
Link to section 'Batch job' of 'gadma' Batch job
Using #!/bin/sh -l
as shebang in the slurm job script will cause the failure of some biocontainer modules. Please use #!/bin/bash
instead.
To run gadma on our clusters:
#!/bin/bash
#SBATCH -A myallocation # Allocation name
#SBATCH -t 1:00:00
#SBATCH -N 1
#SBATCH -n 1
#SBATCH --job-name=gadma
#SBATCH --mail-type=FAIL,BEGIN,END
#SBATCH --error=%x-%J-%u.err
#SBATCH --output=%x-%J-%u.out
module --force purge
ml biocontainers gadma
gadma -p params_file
gambit
Link to section 'Introduction' of 'gambit' Introduction
GAMBIT (Genomic Approximation Method for Bacterial Identification and Tracking) is a tool for rapid taxonomic identification of microbial pathogens.
Docker hub: https://hub.docker.com/r/staphb/gambit
Home page: https://github.com/jlumpe/gambit
Link to section 'Versions' of 'gambit' Versions
- 0.5.0
Link to section 'Commands' of 'gambit' Commands
- gambit
Link to section 'Module' of 'gambit' Module
You can load the modules by:
module load biocontainers
module load gambit
Link to section 'Example job' of 'gambit' Example job
Using #!/bin/sh -l
as shebang in the slurm job script will cause the failure of some biocontainer modules. Please use #!/bin/bash
instead.
To run gambit on our clusters:
#!/bin/bash
#SBATCH -A myallocation # Allocation name
#SBATCH -t 1:00:00
#SBATCH -N 1
#SBATCH -n 1
#SBATCH --job-name=gambit
#SBATCH --mail-type=FAIL,BEGIN,END
#SBATCH --error=%x-%J-%u.err
#SBATCH --output=%x-%J-%u.out
module --force purge
ml biocontainers gambit
gambit -d database query -o results.csv *.fasta
gamma
Link to section 'Introduction' of 'gamma' Introduction
GAMMA (Gene Allele Mutation Microbial Assessment) is a command line tool that finds gene matches in microbial genomic data using protein coding (rather than nucleotide) identity, and then translates and annotates the match by providing the type (i.e., mutant, truncation, etc.) and a translated description (i.e., Y190S mutant, truncation at residue 110, etc.). Because microbial gene families often have multiple alleles and existing databases are rarely exhaustive, GAMMA is helpful in both identifying and explaining how unique alleles differ from their closest known matches.
Docker hub: https://hub.docker.com/r/staphb/gamma
Home page: https://github.com/rastanton/GAMMA
Link to section 'Versions' of 'gamma' Versions
- 1.4
- 2.2
Link to section 'Commands' of 'gamma' Commands
- GAMMA-S.py
- GAMMA.py
Link to section 'Module' of 'gamma' Module
You can load the modules by:
module load biocontainers
module load gamma
Link to section 'Example job' of 'gamma' Example job
Using #!/bin/sh -l
as shebang in the slurm job script will cause the failure of some biocontainer modules. Please use #!/bin/bash
instead.
To run gamma on our clusters:
#!/bin/bash
#SBATCH -A myallocation # Allocation name
#SBATCH -t 1:00:00
#SBATCH -N 1
#SBATCH -n 1
#SBATCH --job-name=gamma
#SBATCH --mail-type=FAIL,BEGIN,END
#SBATCH --error=%x-%J-%u.err
#SBATCH --output=%x-%J-%u.out
module --force purge
ml biocontainers gamma
GAMMA.py DHQP1701672_complete_genome.fasta ResFinderDB_Combined_05-06-20.fsa GAMMA_Test
gangstr
Link to section 'Introduction' of 'gangstr' Introduction
GangSTR is a tool for genome-wide profiling tandem repeats from short reads. A key advantage of GangSTR over existing genome-wide TR tools (e.g. lobSTR or hipSTR) is that it can handle repeats that are longer than the read length. GangSTR takes aligned reads (BAM) and a set of repeats in the reference genome as input and outputs a VCF file containing genotypes for each locus.
BioContainers: https://biocontainers.pro/tools/gangstr
Home page: https://github.com/gymreklab/GangSTR
Link to section 'Versions' of 'gangstr' Versions
- 2.5.0
Link to section 'Commands' of 'gangstr' Commands
- GangSTR
Link to section 'Module' of 'gangstr' Module
You can load the modules by:
module load biocontainers
module load gangstr
Link to section 'Example job' of 'gangstr' Example job
Using #!/bin/sh -l
as shebang in the slurm job script will cause the failure of some biocontainer modules. Please use #!/bin/bash
instead.
To run gangstr on our clusters:
#!/bin/bash
#SBATCH -A myallocation # Allocation name
#SBATCH -t 1:00:00
#SBATCH -N 1
#SBATCH -n 1
#SBATCH --job-name=gangstr
#SBATCH --mail-type=FAIL,BEGIN,END
#SBATCH --error=%x-%J-%u.err
#SBATCH --output=%x-%J-%u.out
module --force purge
ml biocontainers gangstr
gapfiller
Link to section 'Introduction' of 'gapfiller' Introduction
GapFiller is a seed-and-extend local assembler to fill the gap within paired reads. It can be used for both DNA and RNA and it has been tested on Illumina data. GapFiller can be used whenever a sequence is to be assembled starting from reads lying on its ends, provided a loose estimate of sequence length.
BioContainers: https://biocontainers.pro/tools/gapfiller
Home page: https://sourceforge.net/projects/gapfiller/
Link to section 'Versions' of 'gapfiller' Versions
- 2.1.2
Link to section 'Commands' of 'gapfiller' Commands
- GapFiller
Link to section 'Module' of 'gapfiller' Module
You can load the modules by:
module load biocontainers
module load gapfiller
Link to section 'Example job' of 'gapfiller' Example job
Using #!/bin/sh -l
as shebang in the slurm job script will cause the failure of some biocontainer modules. Please use #!/bin/bash
instead.
To run gapfiller on our clusters:
#!/bin/bash
#SBATCH -A myallocation # Allocation name
#SBATCH -t 1:00:00
#SBATCH -N 1
#SBATCH -n 1
#SBATCH --job-name=gapfiller
#SBATCH --mail-type=FAIL,BEGIN,END
#SBATCH --error=%x-%J-%u.err
#SBATCH --output=%x-%J-%u.out
module --force purge
ml biocontainers gapfiller
gatk
Link to section 'Introduction' of 'gatk' Introduction
GATK
(Genome Analysis Toolkit) is a collection of command-line tools for analyzing high-throughput sequencing data with a primary focus on variant discovery.
For more information, please check its website: https://biocontainers.pro/tools/gatk and its home page: https://www.broadinstitute.org/gatk/.
Link to section 'Versions' of 'gatk' Versions
- 3.8
Link to section 'Commands' of 'gatk' Commands
- gatk3
Link to section 'Module' of 'gatk' Module
You can load the modules by:
module load biocontainers
module load gatk
Link to section 'Example job' of 'gatk' Example job
Using #!/bin/sh -l
as shebang in the slurm job script will cause the failure of some biocontainer modules. Please use #!/bin/bash
instead.
To run GATK on our clusters:
#!/bin/bash
#SBATCH -A myallocation # Allocation name
#SBATCH -t 1:00:00
#SBATCH -N 1
#SBATCH -n 24
#SBATCH --job-name=gatk
#SBATCH --mail-type=FAIL,BEGIN,END
#SBATCH --error=%x-%J-%u.err
#SBATCH --output=%x-%J-%u.out
module --force purge
ml biocontainers gatk
gatk3 -T HaplotypeCaller \
-nct 24 -R hg38.fa \
-I 19P0126636WES.sorted.bam \
-o 19P0126636WES.HC.vcf
gatk4
GATK (Genome Analysis Toolkit)
is a collection of command-line tools for analyzing high-throughput sequencing data with a primary focus on variant discovery. Detailed usage can be found here: https://www.broadinstitute.org/gatk/.
Link to section 'Versions' of 'gatk4' Versions
- 4.2.0
- 4.2.5.0
- 4.2.6.1
- 4.3.0.0
Link to section 'Commands' of 'gatk4' Commands
gatk
Link to section 'Module' of 'gatk4' Module
You can load the modules by:
module load biocontainers
module load gatk4/4.2.5.0
Link to section 'Example job' of 'gatk4' Example job
Using #!/bin/sh -l
as shebang in the slurm job script will cause the failure of some biocontainer modules. Please use #!/bin/bash
instead.
To run gatk4 our our clusters:
#!/bin/bash
#SBATCH -A myallocation # Allocation name
#SBATCH -t 20:00:00
#SBATCH -N 1
#SBATCH -n 24
#SBATCH --job-name=gatk4
#SBATCH --mail-type=FAIL,BEGIN,END
#SBATCH --error=%x-%J-%u.err
#SBATCH --output=%x-%J-%u.out
module --force purge
ml biocontainers gatk4/4.2.5.0
gatk --java-options "-Xmx12G -XX:ParallelGCThreads=24" HaplotypeCaller -R hg38.fa -I 19P0126636WES.sorted.bam -O 19P0126636WES.HC.vcf --sample-name 19P0126636
gemma
Link to section 'Introduction' of 'gemma' Introduction
Gemma
is a software toolkit for fast application of linear mixed models (LMMs) and related models to genome-wide association studies (GWAS) and other large-scale data sets.
For more information, please check its website: https://biocontainers.pro/tools/gemma and its home page on Github.
Link to section 'Versions' of 'gemma' Versions
- 0.98.3
Link to section 'Commands' of 'gemma' Commands
- gemma
Link to section 'Module' of 'gemma' Module
You can load the modules by:
module load biocontainers
module load gemma
Link to section 'Example job' of 'gemma' Example job
Using #!/bin/sh -l
as shebang in the slurm job script will cause the failure of some biocontainer modules. Please use #!/bin/bash
instead.
To run Gemma on our clusters:
#!/bin/bash
#SBATCH -A myallocation # Allocation name
#SBATCH -t 1:00:00
#SBATCH -N 1
#SBATCH -n 1
#SBATCH --job-name=gemma
#SBATCH --mail-type=FAIL,BEGIN,END
#SBATCH --error=%x-%J-%u.err
#SBATCH --output=%x-%J-%u.out
module --force purge
ml biocontainers gemma
gemma -g ./example/mouse_hs1940.geno.txt.gz -p ./example/mouse_hs1940.pheno.txt \
-gk -o mouse_hs1940
gemma -g ./example/mouse_hs1940.geno.txt.gz \
-p ./example/mouse_hs1940.pheno.txt -n 1 -a ./example/mouse_hs1940.anno.txt \
-k ./output/mouse_hs1940.cXX.txt -lmm -o mouse_hs1940_CD8_lmm
gemoma
Link to section 'Introduction' of 'gemoma' Introduction
Gene Model Mapper (GeMoMa) is a homology-based gene prediction program. GeMoMa uses the annotation of protein-coding genes in a reference genome to infer the annotation of protein-coding genes in a target genome. Thereby, GeMoMa utilizes amino acid sequence and intron position conservation. In addition, GeMoMa allows to incorporate RNA-seq evidence for splice site prediction.
BioContainers: https://biocontainers.pro/tools/gemoma
Home page: http://www.jstacs.de/index.php/GeMoMa
Link to section 'Versions' of 'gemoma' Versions
- 1.7.1
Link to section 'Commands' of 'gemoma' Commands
- GeMoMa
Link to section 'Module' of 'gemoma' Module
You can load the modules by:
module load biocontainers
module load gemoma
Link to section 'Example job' of 'gemoma' Example job
Using #!/bin/sh -l
as shebang in the slurm job script will cause the failure of some biocontainer modules. Please use #!/bin/bash
instead.
To run gemoma on our clusters:
#!/bin/bash
#SBATCH -A myallocation # Allocation name
#SBATCH -t 1:00:00
#SBATCH -N 1
#SBATCH -n 1
#SBATCH --job-name=gemoma
#SBATCH --mail-type=FAIL,BEGIN,END
#SBATCH --error=%x-%J-%u.err
#SBATCH --output=%x-%J-%u.out
module --force purge
ml biocontainers gemoma
genemark
Link to section 'Introduction' of 'genemark' Introduction
GeneMark-ES/ET/EP
contains GeneMark-ES, GeneMark-ET and GeneMark-EP+ algorithms.
Link to section 'Versions' of 'genemark' Versions
- 4.68
- 4.69
Link to section 'Commands' of 'genemark' Commands
- bed_to_gff.pl
- bp_seq_select.pl
- build_mod.pl
- calc_introns_from_gtf.pl
- change_path_in_perl_scripts.pl
- compare_intervals_exact.pl
- gc_distr.pl
- get_below_gc.pl
- get_sequence_from_GTF.pl
- gmes_petap.pl
- hc_exons2hints.pl
- histogram.pl
- make_nt_freq_mat.pl
- parse_ET.pl
- parse_by_introns.pl
- parse_gibbs.pl
- parse_set.pl
- predict_genes.pl
- reformat_gff.pl
- rescale_gff.pl
- rnaseq_introns_to_gff.pl
- run_es.pl
- run_hmm_pbs.pl
- scan_for_bp.pl
- star_to_gff.pl
- verify_evidence_gmhmm.pl
Link to section 'Academic license' of 'genemark' Academic license
To use GeneMark, users need to download license files by yourself.
To use GeneMark, users need to download license files by yourself.
Go to the GeneMark web site: http://exon.gatech.edu/GeneMark/license_download.cgi. Check the boxes for GeneMark-ES/ET/EP ver 4.69_lic
and LINUX 64
next to it, fill out the form, then click "I agree". In the next page, right click and copy the link addresses for 64 bit
licenss. Paste the link addresses in the commands below:
cd $HOME
wget "replace with license URL"
zcat gm_key_64.gz > .gm_key
Link to section 'Module' of 'genemark' Module
You can load the modules by:
module load biocontainers
module load genemark/4.68
Link to section 'Example job' of 'genemark' Example job
Using #!/bin/sh -l
as shebang in the slurm job script will cause the failure of some biocontainer modules. Please use #!/bin/bash
instead.
To run GeneMark on our cluster:
#!/bin/bash
#SBATCH -A myallocation # Allocation name
#SBATCH -t 1:00:00
#SBATCH -N 1
#SBATCH -n 24
#SBATCH --job-name=genemark
#SBATCH --mail-type=FAIL,BEGIN,END
#SBATCH --error=%x-%J-%u.err
#SBATCH --output=%x-%J-%u.out
module --force purge
ml biocontainers genemark/4.68
gmes_petap.pl --ES --cores 24 --sequence scaffolds.fasta
genemarks-2
Link to section 'Introduction' of 'genemarks-2' Introduction
GeneMarkS-2 combines GeneMark.hmm (prokaryotic) and GeneMark (prokaryotic) with a self-training procedure that determines parameters of the models of both GeneMark.hmm and GeneMark.
Home page: http://opal.biology.gatech.edu/GeneMark/
Link to section 'Versions' of 'genemarks-2' Versions
- 1.14_1.25
Link to section 'Commands' of 'genemarks-2' Commands
- gms2.pl
- biogem
- compp
- gmhmmp2
Link to section 'Module' of 'genemarks-2' Module
You can load the modules by:
module load biocontainers
module load genemarks-2
Link to section 'Example job' of 'genemarks-2' Example job
Using #!/bin/sh -l
as shebang in the slurm job script will cause the failure of some biocontainer modules. Please use #!/bin/bash
instead.
To run genemarks-2 on our clusters:
#!/bin/bash
#SBATCH -A myallocation # Allocation name
#SBATCH -t 1:00:00
#SBATCH -N 1
#SBATCH -n 1
#SBATCH --job-name=genemarks-2
#SBATCH --mail-type=FAIL,BEGIN,END
#SBATCH --error=%x-%J-%u.err
#SBATCH --output=%x-%J-%u.out
module --force purge
ml biocontainers genemarks-2
genmap
Link to section 'Introduction' of 'genmap' Introduction
GenMap: Ultra-fast Computation of Genome Mappability.
BioContainers: https://biocontainers.pro/tools/genmap
Home page: https://github.com/cpockrandt/genmap
Link to section 'Versions' of 'genmap' Versions
- 1.3.0
Link to section 'Commands' of 'genmap' Commands
- genmap
Link to section 'Module' of 'genmap' Module
You can load the modules by:
module load biocontainers
module load genmap
Link to section 'Example job' of 'genmap' Example job
Using #!/bin/sh -l
as shebang in the slurm job script will cause the failure of some biocontainer modules. Please use #!/bin/bash
instead.
To run genmap on our clusters:
#!/bin/bash
#SBATCH -A myallocation # Allocation name
#SBATCH -t 1:00:00
#SBATCH -N 1
#SBATCH -n 1
#SBATCH --job-name=genmap
#SBATCH --mail-type=FAIL,BEGIN,END
#SBATCH --error=%x-%J-%u.err
#SBATCH --output=%x-%J-%u.out
module --force purge
ml biocontainers genmap
export TMPDIR=$PWD/tmp
genmap index -F ~/.local/share/genomes/hg38/hg38.fa -I hg38_index
genmap map -K 64 -E 2 -I hg38_index -O map_output_hg38 -t -w -bg
genomedata
Link to section 'Introduction' of 'genomedata' Introduction
Genomedata is a format for efficient storage of multiple tracks of numeric data anchored to a genome. The format allows fast random access to hundreds of gigabytes of data, while retaining a small disk space footprint.
BioContainers: https://biocontainers.pro/tools/genomedata
Home page: http://pmgenomics.ca/hoffmanlab/proj/genomedata/
Link to section 'Versions' of 'genomedata' Versions
- 1.5.0
Link to section 'Commands' of 'genomedata' Commands
- python
- python3
- genomeCoverageBed
- genomedata-close-data
- genomedata-erase-data
- genomedata-hardmask
- genomedata-histogram
- genomedata-info
- genomedata-load
- genomedata-load-assembly
- genomedata-load-data
- genomedata-load-seq
- genomedata-open-data
- genomedata-query
- genomedata-report
Link to section 'Module' of 'genomedata' Module
You can load the modules by:
module load biocontainers
module load genomedata
Link to section 'Example job' of 'genomedata' Example job
Using #!/bin/sh -l
as shebang in the slurm job script will cause the failure of some biocontainer modules. Please use #!/bin/bash
instead.
To run genomedata on our clusters:
#!/bin/bash
#SBATCH -A myallocation # Allocation name
#SBATCH -t 1:00:00
#SBATCH -N 1
#SBATCH -n 1
#SBATCH --job-name=genomedata
#SBATCH --mail-type=FAIL,BEGIN,END
#SBATCH --error=%x-%J-%u.err
#SBATCH --output=%x-%J-%u.out
module --force purge
ml biocontainers genomedata
genomepy
Link to section 'Introduction' of 'genomepy' Introduction
Genomepy
is designed to provide a simple and straightforward way to download and use genomic data.
For more information, please check its website: https://biocontainers.pro/tools/genomepy and its home page on Github.
Link to section 'Versions' of 'genomepy' Versions
- 0.12.0
- 0.14.0
Link to section 'Commands' of 'genomepy' Commands
- genomepy
Link to section 'Module' of 'genomepy' Module
You can load the modules by:
module load biocontainers
module load genomepy
Link to section 'Example job' of 'genomepy' Example job
Using #!/bin/sh -l
as shebang in the slurm job script will cause the failure of some biocontainer modules. Please use #!/bin/bash
instead.
To run Genomepy on our clusters:
#!/bin/bash
#SBATCH -A myallocation # Allocation name
#SBATCH -t 1:00:00
#SBATCH -N 1
#SBATCH -n 1
#SBATCH --job-name=genomepy
#SBATCH --mail-type=FAIL,BEGIN,END
#SBATCH --error=%x-%J-%u.err
#SBATCH --output=%x-%J-%u.out
module --force purge
ml biocontainers genomepy
genomescope2
Link to section 'Introduction' of 'genomescope2' Introduction
Genomescope2
: Reference-free profiling of polyploid genomes.
For more information, please check its website: https://biocontainers.pro/tools/genomescope2 and its home page on Github.
Link to section 'Versions' of 'genomescope2' Versions
- 2.0
Link to section 'Commands' of 'genomescope2' Commands
- genomescope2
Link to section 'Module' of 'genomescope2' Module
You can load the modules by:
module load biocontainers
module load genomescope2
Link to section 'Example job' of 'genomescope2' Example job
Using #!/bin/sh -l
as shebang in the slurm job script will cause the failure of some biocontainer modules. Please use #!/bin/bash
instead.
To run Genomescope2 on our clusters:
#!/bin/bash
#SBATCH -A myallocation # Allocation name
#SBATCH -t 1:00:00
#SBATCH -N 1
#SBATCH -n 1
#SBATCH --job-name=genomescope2
#SBATCH --mail-type=FAIL,BEGIN,END
#SBATCH --error=%x-%J-%u.err
#SBATCH --output=%x-%J-%u.out
module --force purge
ml biocontainers genomescope2
wget https://raw.githubusercontent.com/schatzlab/genomescope/master/analysis/real_data/ara_F1_21.hist
genomescope2 -i ara_F1_21.hist -o output -k 21
genomicconsensus
Link to section 'Introduction' of 'genomicconsensus' Introduction
Genomicconsensus
is the current PacBio consensus and variant calling suite.
For more information, please check its website: https://biocontainers.pro/tools/genomicconsensus.
Link to section 'Versions' of 'genomicconsensus' Versions
- 2.3.3
Link to section 'Commands' of 'genomicconsensus' Commands
- quiver
- arrow
- variantCaller
Link to section 'Module' of 'genomicconsensus' Module
You can load the modules by:
module load biocontainers
module load genomicconsensus
Link to section 'Example job' of 'genomicconsensus' Example job
Using #!/bin/sh -l
as shebang in the slurm job script will cause the failure of some biocontainer modules. Please use #!/bin/bash
instead.
To run Genomicconsensus on our clusters:
#!/bin/bash
#SBATCH -A myallocation # Allocation name
#SBATCH -t 1:00:00
#SBATCH -N 1
#SBATCH -n 1
#SBATCH --job-name=genomicconsensus
#SBATCH --mail-type=FAIL,BEGIN,END
#SBATCH --error=%x-%J-%u.err
#SBATCH --output=%x-%J-%u.out
module --force purge
ml biocontainers genomicconsensus
quiver -j12 out.aligned_subreads.bam \
-r All4mer.V2.01_Insert-changed.fa \
-o consensus.fasta -o consensus.fastq
genrich
Link to section 'Introduction' of 'genrich' Introduction
Genrich
is a peak-caller for genomic enrichment assays (e.g. ChIP-seq, ATAC-seq). It analyzes alignment files generated following the assay and produces a file detailing peaks of significant enrichment.
For more information, please check its website: https://biocontainers.pro/tools/genrich and its home page on Github.
Link to section 'Versions' of 'genrich' Versions
- 0.6.1
Link to section 'Commands' of 'genrich' Commands
- Genrich
Link to section 'Module' of 'genrich' Module
You can load the modules by:
module load biocontainers
module load genrich
Link to section 'Example job' of 'genrich' Example job
Using #!/bin/sh -l
as shebang in the slurm job script will cause the failure of some biocontainer modules. Please use #!/bin/bash
instead.
To run Genrich on our clusters:
#!/bin/bash
#SBATCH -A myallocation # Allocation name
#SBATCH -t 1:00:00
#SBATCH -N 1
#SBATCH -n 1
#SBATCH --job-name=genrich
#SBATCH --mail-type=FAIL,BEGIN,END
#SBATCH --error=%x-%J-%u.err
#SBATCH --output=%x-%J-%u.out
module --force purge
ml biocontainers genrich
Genrich -t sample.bam -o sample.narrowPeak -v
gfaffix
Link to section 'Introduction' of 'gfaffix' Introduction
GFAffix identifies walk-preserving shared affixes in variation graphs and collapses them into a non-redundant graph structure.
BioContainers: https://biocontainers.pro/tools/gfaffix
Home page: https://github.com/marschall-lab/GFAffix
Link to section 'Versions' of 'gfaffix' Versions
- 0.1.4
Link to section 'Commands' of 'gfaffix' Commands
- gfaffix
Link to section 'Module' of 'gfaffix' Module
You can load the modules by:
module load biocontainers
module load gfaffix
Link to section 'Example job' of 'gfaffix' Example job
Using #!/bin/sh -l
as shebang in the slurm job script will cause the failure of some biocontainer modules. Please use #!/bin/bash
instead.
To run gfaffix on our clusters:
#!/bin/bash
#SBATCH -A myallocation # Allocation name
#SBATCH -t 1:00:00
#SBATCH -N 1
#SBATCH -n 1
#SBATCH --job-name=gfaffix
#SBATCH --mail-type=FAIL,BEGIN,END
#SBATCH --error=%x-%J-%u.err
#SBATCH --output=%x-%J-%u.out
module --force purge
ml biocontainers gfaffix
gfastats
Link to section 'Introduction' of 'gfastats' Introduction
gfastats is a single fast and exhaustive tool for summary statistics and simultaneous fa (fasta, fastq, gfa [.gz]) genome assembly file manipulation. gfastats also allows seamless fasta<>fastq<>gfa[.gz] conversion. It has been tested in genomes even >100Gbp.
BioContainers: https://biocontainers.pro/tools/gfastats
Home page: https://github.com/vgl-hub/gfastats
Link to section 'Versions' of 'gfastats' Versions
- 1.2.3
Link to section 'Commands' of 'gfastats' Commands
- gfastats
Link to section 'Module' of 'gfastats' Module
You can load the modules by:
module load biocontainers
module load gfastats
Link to section 'Example job' of 'gfastats' Example job
Using #!/bin/sh -l
as shebang in the slurm job script will cause the failure of some biocontainer modules. Please use #!/bin/bash
instead.
To run gfastats on our clusters:
#!/bin/bash
#SBATCH -A myallocation # Allocation name
#SBATCH -t 1:00:00
#SBATCH -N 1
#SBATCH -n 1
#SBATCH --job-name=gfastats
#SBATCH --mail-type=FAIL,BEGIN,END
#SBATCH --error=%x-%J-%u.err
#SBATCH --output=%x-%J-%u.out
module --force purge
ml biocontainers gfastats
gfastats input.fasta -o gfa
gfatools
Link to section 'Introduction' of 'gfatools' Introduction
gfatools is a set of tools for manipulating sequence graphs in the GFA or the rGFA format. It has implemented parsing, subgraph and conversion to FASTA/BED.
BioContainers: https://biocontainers.pro/tools/gfatools
Home page: https://github.com/lh3/gfatools
Link to section 'Versions' of 'gfatools' Versions
- 0.5
Link to section 'Commands' of 'gfatools' Commands
- gfatools
Link to section 'Module' of 'gfatools' Module
You can load the modules by:
module load biocontainers
module load gfatools
Link to section 'Example job' of 'gfatools' Example job
Using #!/bin/sh -l
as shebang in the slurm job script will cause the failure of some biocontainer modules. Please use #!/bin/bash
instead.
To run gfatools on our clusters:
#!/bin/bash
#SBATCH -A myallocation # Allocation name
#SBATCH -t 1:00:00
#SBATCH -N 1
#SBATCH -n 1
#SBATCH --job-name=gfatools
#SBATCH --mail-type=FAIL,BEGIN,END
#SBATCH --error=%x-%J-%u.err
#SBATCH --output=%x-%J-%u.out
module --force purge
ml biocontainers gfatools
# Extract a subgraph
gfatools view -l MTh4502 -r 1 test/MT.gfa > sub.gfa
# Convert GFA to segment FASTA
gfatools gfa2fa test/MT.gfa > MT-seg.fa
# Convert rGFA to stable FASTA or BED
gfatools gfa2fa -s test/MT.gfa > MT.fa
gfatools gfa2bed -m test/MT.gfa > MT.bed
gffcompare
Link to section 'Introduction' of 'gffcompare' Introduction
Gffcompare
is used to compare, merge, annotate and estimate accuracy of one or more GFF files.
For more information, please check its website: https://biocontainers.pro/tools/gffcompare and its home page: https://ccb.jhu.edu/software/stringtie/gffcompare.shtml.
Link to section 'Versions' of 'gffcompare' Versions
- 0.11.2
Link to section 'Commands' of 'gffcompare' Commands
- gffcompare
Link to section 'Module' of 'gffcompare' Module
You can load the modules by:
module load biocontainers
module load gffcompare
Link to section 'Example job' of 'gffcompare' Example job
Using #!/bin/sh -l
as shebang in the slurm job script will cause the failure of some biocontainer modules. Please use #!/bin/bash
instead.
To run Gffcompare on our clusters:
#!/bin/bash
#SBATCH -A myallocation # Allocation name
#SBATCH -t 1:00:00
#SBATCH -N 1
#SBATCH -n 1
#SBATCH --job-name=gffcompare
#SBATCH --mail-type=FAIL,BEGIN,END
#SBATCH --error=%x-%J-%u.err
#SBATCH --output=%x-%J-%u.out
module --force purge
ml biocontainers gffcompare
gffcompare -r annotation.gff transcripts.gtf
gffread
Link to section 'Introduction' of 'gffread' Introduction
Gffread
is used to validate, filter, convert and perform various other operations on GFF files.
For more information, please check its website: https://biocontainers.pro/tools/gffread and its home page: http://ccb.jhu.edu/software/stringtie/gff.shtml.
Link to section 'Versions' of 'gffread' Versions
- 0.12.7
Link to section 'Commands' of 'gffread' Commands
- gffread
Link to section 'Module' of 'gffread' Module
You can load the modules by:
module load biocontainers
module load gffread
Link to section 'Example job' of 'gffread' Example job
Using #!/bin/sh -l
as shebang in the slurm job script will cause the failure of some biocontainer modules. Please use #!/bin/bash
instead.
To run Gffread on our clusters:
#!/bin/bash
#SBATCH -A myallocation # Allocation name
#SBATCH -t 1:00:00
#SBATCH -N 1
#SBATCH -n 1
#SBATCH --job-name=gffread
#SBATCH --mail-type=FAIL,BEGIN,END
#SBATCH --error=%x-%J-%u.err
#SBATCH --output=%x-%J-%u.out
module --force purge
ml biocontainers gffread
gffread -E annotation.gff -o ann_simple.gff
gffread annotation.gff -T -o annotation.gtf
gffread -w transcripts.fa -g genome.fa annotation.gff
gffutils
Link to section 'Introduction' of 'gffutils' Introduction
gffutils is a Python package for working with and manipulating the GFF and GTF format files typically used for genomic annotations.
BioContainers: https://biocontainers.pro/tools/gffutils
Home page: https://github.com/daler/gffutils
Link to section 'Versions' of 'gffutils' Versions
- 0.11.1
Link to section 'Commands' of 'gffutils' Commands
- python
- python3
Link to section 'Module' of 'gffutils' Module
You can load the modules by:
module load biocontainers
module load gffutils
Link to section 'Example job' of 'gffutils' Example job
Using #!/bin/sh -l
as shebang in the slurm job script will cause the failure of some biocontainer modules. Please use #!/bin/bash
instead.
To run gffutils on our clusters:
#!/bin/bash
#SBATCH -A myallocation # Allocation name
#SBATCH -t 1:00:00
#SBATCH -N 1
#SBATCH -n 1
#SBATCH --job-name=gffutils
#SBATCH --mail-type=FAIL,BEGIN,END
#SBATCH --error=%x-%J-%u.err
#SBATCH --output=%x-%J-%u.out
module --force purge
ml biocontainers gffutils
gimmemotifs
Link to section 'Introduction' of 'gimmemotifs' Introduction
GimmeMotifs is a suite of motif tools, including a motif prediction pipeline for ChIP-seq experiments.
BioContainers: https://biocontainers.pro/tools/gimmemotifs
Home page: https://github.com/vanheeringen-lab/gimmemotifs
Link to section 'Versions' of 'gimmemotifs' Versions
- 0.17.1
Link to section 'Commands' of 'gimmemotifs' Commands
- gimme -
Link to section 'Module' of 'gimmemotifs' Module
You can load the modules by:
module load biocontainers
module load gimmemotifs
Link to section 'Example job' of 'gimmemotifs' Example job
Using #!/bin/sh -l
as shebang in the slurm job script will cause the failure of some biocontainer modules. Please use #!/bin/bash
instead.
To run gimmemotifs on our clusters:
#!/bin/bash
#SBATCH -A myallocation # Allocation name
#SBATCH -t 1:00:00
#SBATCH -N 1
#SBATCH -n 1
#SBATCH --job-name=gimmemotifs
#SBATCH --mail-type=FAIL,BEGIN,END
#SBATCH --error=%x-%J-%u.err
#SBATCH --output=%x-%J-%u.out
module --force purge
ml biocontainers gimmemotifs
gimme motifs ENCFF407IVS.bed ENCFF407IVS_motifs \
-g ~/.local/share/genomes/hg38/hg38.fa --denovo
glimmer
Link to section 'Introduction' of 'glimmer' Introduction
Glimmer
is a system for finding genes in microbial DNA, especially the genomes of bacteria, archaea, and viruses.
For more information, please check its website: https://biocontainers.pro/tools/glimmer and its home page: http://ccb.jhu.edu/software/glimmer/index.shtml.
Link to section 'Versions' of 'glimmer' Versions
- 3.02
Link to section 'Commands' of 'glimmer' Commands
- anomaly
- build-fixed
- build-icm
- entropy-profile
- entropy-score
- extract
- g3-from-scratch.csh
- g3-from-training.csh
- g3-iterated.csh
- get-motif-counts.awk
- glim-diff.awk
- glimmer3
- long-orfs
- match-list-col.awk
- multi-extract
- not-acgt.awk
- score-fixed
- start-codon-distrib
- test
- uncovered
- upstream-coords.awk
- window-acgt
Link to section 'Module' of 'glimmer' Module
You can load the modules by:
module load biocontainers
module load glimmer
Link to section 'Example job' of 'glimmer' Example job
Using #!/bin/sh -l
as shebang in the slurm job script will cause the failure of some biocontainer modules. Please use #!/bin/bash
instead.
To run Glimmer on our clusters:
#!/bin/bash
#SBATCH -A myallocation # Allocation name
#SBATCH -t 1:00:00
#SBATCH -N 1
#SBATCH -n 1
#SBATCH --job-name=glimmer
#SBATCH --mail-type=FAIL,BEGIN,END
#SBATCH --error=%x-%J-%u.err
#SBATCH --output=%x-%J-%u.out
module --force purge
ml biocontainers glimmer
long-orfs -n -t 1.15 scaffolds.fasta run1.longorfs
extract -t scaffolds.fasta run1.longorfs > run1.train
build-icm -r run1.icm < run1.train
glimmer3 scaffolds.fasta run1.icm cm
glimmerhmm
Link to section 'Introduction' of 'glimmerhmm' Introduction
Glimmerhmm
is a new gene finder based on a Generalized Hidden Markov Model (GHMM).
For more information, please check its website: https://biocontainers.pro/tools/glimmerhmm and its home page: https://ccb.jhu.edu/software/glimmerhmm/.
Link to section 'Versions' of 'glimmerhmm' Versions
- 3.0.4
Link to section 'Commands' of 'glimmerhmm' Commands
- glimmerhmm
- glimmhmm.pl
- trainGlimmerHMM
Link to section 'Module' of 'glimmerhmm' Module
You can load the modules by:
module load biocontainers
module load glimmerhmm
Link to section 'Example job' of 'glimmerhmm' Example job
Using #!/bin/sh -l
as shebang in the slurm job script will cause the failure of some biocontainer modules. Please use #!/bin/bash
instead.
To run Glimmerhmm on our clusters:
#!/bin/bash
#SBATCH -A myallocation # Allocation name
#SBATCH -t 1:00:00
#SBATCH -N 1
#SBATCH -n 1
#SBATCH --job-name=glimmerhmm
#SBATCH --mail-type=FAIL,BEGIN,END
#SBATCH --error=%x-%J-%u.err
#SBATCH --output=%x-%J-%u.out
module --force purge
ml biocontainers glimmerhmm
trainGlimmerHMM Asperg.fasta Asperg.cds -d Asperg
glimmerhmm Asperg.fasta -d Asperg -o Asperg_glimmerhmm_out
glnexus
Link to section 'Introduction' of 'glnexus' Introduction
Glnexus: Scalable gVCF merging and joint variant calling for population sequencing projects.
BioContainers: https://biocontainers.pro/tools/glnexus
Home page: https://github.com/dnanexus-rnd/GLnexus
Link to section 'Versions' of 'glnexus' Versions
- 1.4.1
Link to section 'Commands' of 'glnexus' Commands
- glnexus_cli
Link to section 'Module' of 'glnexus' Module
You can load the modules by:
module load biocontainers
module load glnexus
Link to section 'Example job' of 'glnexus' Example job
Using #!/bin/sh -l
as shebang in the slurm job script will cause the failure of some biocontainer modules. Please use #!/bin/bash
instead.
To run glnexus on our clusters:
#!/bin/bash
#SBATCH -A myallocation # Allocation name
#SBATCH -t 1:00:00
#SBATCH -N 1
#SBATCH -n 1
#SBATCH --job-name=glnexus
#SBATCH --mail-type=FAIL,BEGIN,END
#SBATCH --error=%x-%J-%u.err
#SBATCH --output=%x-%J-%u.out
module --force purge
ml biocontainers glnexus
glnexus_cli --config DeepVariant \
--bed ALDH2.bed \
dv_1000G_ALDH2_gvcf/*.g.vcf.gz \
> dv_1000G_ALDH2.bcf
gmap
Link to section 'Introduction' of 'gmap' Introduction
Gmap
is a genomic mapping and alignment program for mRNA and EST sequences.
For more information, please check its website: https://biocontainers.pro/tools/gmap and its home page: http://research-pub.gene.com/gmap/.
Link to section 'Versions' of 'gmap' Versions
- 2021.05.27
- 2021.08.25
Link to section 'Commands' of 'gmap' Commands
- atoiindex
- cmetindex
- cpuid
- dbsnp_iit
- ensembl_genes
- fa_coords
- get-genome
- gff3_genes
- gff3_introns
- gff3_splicesites
- gmap
- gmap.avx2
- gmap_build
- gmap_cat
- gmapindex
- gmapl
- gmapl.avx2
- gmapl.nosimd
- gmap.nosimd
- gmap_process
- gsnap
- gsnap.avx2
- gsnapl
- gsnapl.avx2
- gsnapl.nosimd
- gsnap.nosimd
- gtf_genes
- gtf_introns
- gtf_splicesites
- gtf_transcript_splicesites
- gvf_iit
- iit_dump
- iit_get
- iit_store
- indexdb_cat
- md_coords
- psl_genes
- psl_introns
- psl_splicesites
- sam_sort
- snpindex
- trindex
- vcf_iit
Link to section 'Module' of 'gmap' Module
You can load the modules by:
module load biocontainers
module load gmap
Link to section 'Example job' of 'gmap' Example job
Using #!/bin/sh -l
as shebang in the slurm job script will cause the failure of some biocontainer modules. Please use #!/bin/bash
instead.
To run Gmap on our clusters:
#!/bin/bash
#SBATCH -A myallocation # Allocation name
#SBATCH -t 1:00:00
#SBATCH -N 1
#SBATCH -n 4
#SBATCH --job-name=gmap
#SBATCH --mail-type=FAIL,BEGIN,END
#SBATCH --error=%x-%J-%u.err
#SBATCH --output=%x-%J-%u.out
module --force purge
ml biocontainers gmap
gmap_build -d Cmm -D Cmm genome.fasta
gmap -d Cmm -t 4 -D ./Cmm cdna.fasta > gmap_out.txt
gmap_build -d GRCh38 -D GRCh38 Homo_sapiens.GRCh38.dna.primary_assembly.fa
gsnap -d GRCh38 -D ./GRCh38 --nthreads=4 SRR16956239_1.fastq SRR16956239_2.fastq > gsnap_out.txt
goatools
Goatools
is a python library for gene ontology analyses. Detailed information about its usage can be found here: https://github.com/tanghaibao/goatools
Link to section 'Versions' of 'goatools' Versions
- 1.1.12
- 1.2.3
Link to section 'Commands' of 'goatools' Commands
- python
- python3
- compare_gos.py
- fetch_associations.py
- find_enrichment.py
- go_plot.py
- map_to_slim.py
- ncbi_gene_results_to_python.py
- plot_go_term.py
- prt_terms.py
- runxlrd.py
- vba_extract.py
- wr_hier.py
- wr_sections.py
Link to section 'Module' of 'goatools' Module
You can load the modules by:
module load biocontainers
module load goatools/1.1.12
Link to section 'Interactive job' of 'goatools' Interactive job
To run goatools interactively on our clusters:
(base) UserID@bell-fe00:~ $ sinteractive -N1 -n12 -t4:00:00 -A myallocation
salloc: Granted job allocation 12345869
salloc: Waiting for resource configuration
salloc: Nodes bell-a008 are ready for job
(base) UserID@bell-a008:~ $ module load biocontainers goatools/1.1.12
(base) UserID@bell-a008:~ $ python
Python 3.8.10 (default, Nov 26 2021, 20:14:08)
[GCC 9.3.0] on linux
Type "help", "copyright", "credits" or "license" for more information.
>>> from goatools.base import download_go_basic_obo
>>> obo_fname = download_go_basic_obo()
Link to section 'Batch job' of 'goatools' Batch job
Using #!/bin/sh -l
as shebang in the slurm job script will cause the failure of some biocontainer modules. Please use #!/bin/bash
instead.
To submit a sbatch job on our clusters:
#!/bin/bash
#SBATCH -A myallocation # Allocation name
#SBATCH -t 10:00:00
#SBATCH -N 1
#SBATCH -n 24
#SBATCH --job-name=goatools
#SBATCH --mail-type=FAIL,BEGIN,END
#SBATCH --error=%x-%J-%u.err
#SBATCH --output=%x-%J-%u.out
module --force purge
ml biocontainers goatools/1.1.12
python script.py
find_enrichment.py --pval=0.05 --indent data/study data/population data/association
go_plot.py --go_file=tests/data/go_plot/go_heartjogging6.txt -r -o heartjogging6_r1.png
graphlan
Link to section 'Introduction' of 'graphlan' Introduction
Graphlan
is a software tool for producing high-quality circular representations of taxonomic and phylogenetic trees.
For more information, please check its website: https://biocontainers.pro/tools/graphlan and its home page: https://huttenhower.sph.harvard.edu/graphlan/.
Link to section 'Versions' of 'graphlan' Versions
- 1.1.3
Link to section 'Commands' of 'graphlan' Commands
- graphlan.py
- graphlan_annotate.py
Link to section 'Module' of 'graphlan' Module
You can load the modules by:
module load biocontainers
module load graphlan
Link to section 'Example job' of 'graphlan' Example job
Using #!/bin/sh -l
as shebang in the slurm job script will cause the failure of some biocontainer modules. Please use #!/bin/bash
instead.
To run Graphlan on our clusters:
#!/bin/bash
#SBATCH -A myallocation # Allocation name
#SBATCH -t 1:00:00
#SBATCH -N 1
#SBATCH -n 1
#SBATCH --job-name=graphlan
#SBATCH --mail-type=FAIL,BEGIN,END
#SBATCH --error=%x-%J-%u.err
#SBATCH --output=%x-%J-%u.out
module --force purge
ml biocontainers graphlan
graphlan_annotate.py hmptree.xml hmptree.annot.xml --annot annot.txt
graphlan.py hmptree.annot.xml hmptree.png --dpi 150 --size 14
graphmap
Link to section 'Introduction' of 'graphmap' Introduction
Graphmap
is a novel mapper targeted at aligning long, error-prone third-generation sequencing data.
For more information, please check its website: https://biocontainers.pro/tools/graphmap and its home page on Github.
Link to section 'Versions' of 'graphmap' Versions
- 0.6.3
Link to section 'Commands' of 'graphmap' Commands
- graphmap2
Link to section 'Module' of 'graphmap' Module
You can load the modules by:
module load biocontainers
module load graphmap
Link to section 'Example job' of 'graphmap' Example job
Using #!/bin/sh -l
as shebang in the slurm job script will cause the failure of some biocontainer modules. Please use #!/bin/bash
instead.
To run Graphmap on our clusters:
#!/bin/bash
#SBATCH -A myallocation # Allocation name
#SBATCH -t 1:00:00
#SBATCH -N 1
#SBATCH -n 1
#SBATCH --job-name=graphmap
#SBATCH --mail-type=FAIL,BEGIN,END
#SBATCH --error=%x-%J-%u.err
#SBATCH --output=%x-%J-%u.out
module --force purge
ml biocontainers graphmap
gridss
Link to section 'Introduction' of 'gridss' Introduction
Gridss
is a module software suite containing tools useful for the detection of genomic rearrangements.
Docker hub: https://hub.docker.com/r/gridss/gridss and its home page on Github.
Link to section 'Versions' of 'gridss' Versions
- 2.13.2
Link to section 'Commands' of 'gridss' Commands
- R
- Rscript
- gridss
- gridss_annotate_vcf_kraken2
- gridss_annotate_vcf_repeatmasker
- gridss_extract_overlapping_fragments
- gridss_somatic_filter
- gridsstools
- virusbreakend
- virusbreakend-build
Link to section 'Module' of 'gridss' Module
You can load the modules by:
module load biocontainers
module load gridss
Link to section 'Example job' of 'gridss' Example job
Using #!/bin/sh -l
as shebang in the slurm job script will cause the failure of some biocontainer modules. Please use #!/bin/bash
instead.
To run Gridss on our clusters:
#!/bin/bash
#SBATCH -A myallocation # Allocation name
#SBATCH -t 1:00:00
#SBATCH -N 1
#SBATCH -n 1
#SBATCH --job-name=gridss
#SBATCH --mail-type=FAIL,BEGIN,END
#SBATCH --error=%x-%J-%u.err
#SBATCH --output=%x-%J-%u.out
module --force purge
ml biocontainers gridss
gseapy
Link to section 'Introduction' of 'gseapy' Introduction
Gseapy
is a python wrapper for GESA and Enrichr.
For more information, please check its website: https://biocontainers.pro/tools/gseapy and its home page: https://gseapy.readthedocs.io/en/latest/introduction.html.
Link to section 'Versions' of 'gseapy' Versions
- 0.10.8
Link to section 'Commands' of 'gseapy' Commands
- gseapy
- python
- python3
Link to section 'Module' of 'gseapy' Module
You can load the modules by:
module load biocontainers
module load gseapy
Link to section 'Example job' of 'gseapy' Example job
Using #!/bin/sh -l
as shebang in the slurm job script will cause the failure of some biocontainer modules. Please use #!/bin/bash
instead.
To run Gseapy on our clusters:
#!/bin/bash
#SBATCH -A myallocation # Allocation name
#SBATCH -t 1:00:00
#SBATCH -N 1
#SBATCH -n 1
#SBATCH --job-name=gseapy
#SBATCH --mail-type=FAIL,BEGIN,END
#SBATCH --error=%x-%J-%u.err
#SBATCH --output=%x-%J-%u.out
module --force purge
ml biocontainers gseapy
gseapy ssgsea -d ./data/testSet_rand1200.gct \
-g data/temp.gmt \
-o test/ssgsea_report2 \
-p 4 --no-plot --no-scale
gseapy replot -i data -o test/replot_test
gtdbtk
Link to section 'Introduction' of 'gtdbtk' Introduction
GTDB-Tk
is a software toolkit for assigning objective taxonomic classifications to bacterial and archaeal genomes based on the Genome Database Taxonomy GTDB. It is designed to work with recent advances that allow hundreds or thousands of metagenome-assembled genomes (MAGs) to be obtained directly from environmental samples. It can also be applied to isolate and single-cell genomes.
GTDB-Tk reference data (R202) has been downloaded for users.
Link to section 'Versions' of 'gtdbtk' Versions
- 1.7.0
- 2.1.0
Link to section 'Commands' of 'gtdbtk' Commands
- gtdbtk
Link to section 'Module' of 'gtdbtk' Module
module load biocontainers module load gtdbtk/1.7.0
Link to section 'Example job' of 'gtdbtk' Example job
Using #!/bin/sh -l
as shebang in the slurm job script will cause the failure of some biocontainer modules. Please use #!/bin/bash
instead.
To run GTDB-Tk our our clusters:
#!/bin/bash
#SBATCH -A myallocation # Allocation name
#SBATCH -t 20:00:00
#SBATCH -N 1
#SBATCH -n 24
#SBATCH --job-name=gtdbtk
#SBATCH --mail-type=FAIL,BEGIN,END
#SBATCH --error=%x-%J-%u.err
#SBATCH --output=%x-%J-%u.out
module --force purge
ml biocontainers gtdbtk/1.7.0
gtdbtk identify --genome_dir genomes --out_dir identify --extension gz --cpus 8
gtdbtk align --identify_dir identify --out_dir align --cpus 8
gtdbtk classify --genome_dir genomes --align_dir align --out_dir classify --extension gz --cpus 8
gubbins
Link to section 'Introduction' of 'gubbins' Introduction
Gubbins
is an algorithm that iteratively identifies loci containing elevated densities of base substitutions while concurrently constructing a phylogeny based on the putative point mutations outside of these regions.
For more information, please check its website: https://biocontainers.pro/tools/gubbins and its home page on Github.
Link to section 'Versions' of 'gubbins' Versions
- 3.2.0
- 3.3
Link to section 'Commands' of 'gubbins' Commands
- extract_gubbins_clade.py
- generate_ska_alignment.py
- gubbins_alignment_checker.py
- mask_gubbins_aln.py
- run_gubbins.py
- sumlabels.py
- sumtrees.py
Link to section 'Module' of 'gubbins' Module
You can load the modules by:
module load biocontainers
module load gubbins
Link to section 'Example job' of 'gubbins' Example job
Using #!/bin/sh -l
as shebang in the slurm job script will cause the failure of some biocontainer modules. Please use #!/bin/bash
instead.
To run Gubbins on our clusters:
#!/bin/bash
#SBATCH -A myallocation # Allocation name
#SBATCH -t 1:00:00
#SBATCH -N 1
#SBATCH -n 1
#SBATCH --job-name=gubbins
#SBATCH --mail-type=FAIL,BEGIN,END
#SBATCH --error=%x-%J-%u.err
#SBATCH --output=%x-%J-%u.out
module --force purge
ml biocontainers gubbins
run_gubbins.py --prefix ST239 ST239.aln
guppy
Link to section 'Introduction' of 'guppy' Introduction
Guppy
is a data processing toolkit that contains the Oxford Nanopore Technologies’ basecalling algorithms, and several bioinformatic post-processing features.
Docker hub: https://hub.docker.com/r/genomicpariscentre/guppy and its home page: https://community.nanoporetech.com.
Link to section 'Versions' of 'guppy' Versions
- 6.0.1
Link to section 'Commands' of 'guppy' Commands
- guppy_aligner
- guppy_barcoder
- guppy_basecall_server
- guppy_basecaller
- guppy_basecaller_duplex
- guppy_basecaller_supervisor
- guppy_basecall_client
Link to section 'Module' of 'guppy' Module
You can load the modules by:
module load biocontainers
module load guppy
Link to section 'Example job' of 'guppy' Example job
Using #!/bin/sh -l
as shebang in the slurm job script will cause the failure of some biocontainer modules. Please use #!/bin/bash
instead.
To run Guppy on our clusters:
#!/bin/bash
#SBATCH -A myallocation # Allocation name
#SBATCH -t 1:00:00
#SBATCH -N 1
#SBATCH -n 12
#SBATCH --job-name=guppy
#SBATCH --mail-type=FAIL,BEGIN,END
#SBATCH --error=%x-%J-%u.err
#SBATCH --output=%x-%J-%u.out
module --force purge
ml biocontainers guppy
guppy_basecaller --compress_fastq -i data/fast5_tiny/ \
-s basecall_tiny/ --cpu_threads_per_caller 12 \
--num_callers 1 -c dna_r9.4.1_450bps_hac.cfg
hail
Link to section 'Introduction' of 'hail' Introduction
Hail is an open-source, general-purpose, Python-based data analysis tool with additional data types and methods for working with genomic data.
Docker hub: https://hub.docker.com/r/hailgenetics/hail
Home page: https://github.com/hail-is/hail
Link to section 'Versions' of 'hail' Versions
- 0.2.94
- 0.2.98
Link to section 'Commands' of 'hail' Commands
- python3
Link to section 'Module' of 'hail' Module
You can load the modules by:
module load biocontainers
module load hail
Link to section 'Interactive job' of 'hail' Interactive job
To run Hail interactively on our clusters:
(base) UserID@bell-fe00:~ $ sinteractive -N1 -n12 -t4:00:00 -A myallocation
salloc: Granted job allocation 12345869
salloc: Waiting for resource configuration
salloc: Nodes bell-a008 are ready for job
(base) UserID@bell-a008:~ $ module load biocontainers hail
(base) UserID@bell-a008:~ $ python3
Python 3.7.13 (default, Apr 24 2022, 01:05:22)
[GCC 9.4.0] on linux
Type "help", "copyright", "credits" or "license" for more information.
>>> import hail as hl
>>> print(hl.citation())
Hail Team. Hail 0.2.94-f0b38d6c436f. https://github.com/hail-is/hail/commit/f0b38d6c436f.
Link to section 'Batch job' of 'hail' Batch job
Using #!/bin/sh -l
as shebang in the slurm job script will cause the failure of some biocontainer modules. Please use #!/bin/bash
instead.
To run hail on our clusters:
#!/bin/bash
#SBATCH -A myallocation # Allocation name
#SBATCH -t 1:00:00
#SBATCH -N 1
#SBATCH -n 1
#SBATCH --job-name=hail
#SBATCH --mail-type=FAIL,BEGIN,END
#SBATCH --error=%x-%J-%u.err
#SBATCH --output=%x-%J-%u.out
module --force purge
ml biocontainers hail
python3 script.py
hap.py
Link to section 'Introduction' of 'hap.py' Introduction
Hap.py is a tool to compare diploid genotypes at haplotype level.
Docker hub: https://hub.docker.com/r/pkrusche/hap.py
Home page: https://github.com/Illumina/hap.py
Link to section 'Versions' of 'hap.py' Versions
- 0.3.9
Link to section 'Commands' of 'hap.py' Commands
- bamstats.py
- cnx.py
- ftx.py
- guess-ploidy.py
- hap.py
- ovc.py
- plot-roh.py
- pre.py
- qfy.py
- som.py
- varfilter.py
Link to section 'Module' of 'hap.py' Module
You can load the modules by:
module load biocontainers
module load hap.py
Link to section 'Example job' of 'hap.py' Example job
Using #!/bin/sh -l
as shebang in the slurm job script will cause the failure of some biocontainer modules. Please use #!/bin/bash
instead.
To run hap.py on our clusters:
#!/bin/bash
#SBATCH -A myallocation # Allocation name
#SBATCH -t 1:00:00
#SBATCH -N 1
#SBATCH -n 1
#SBATCH --job-name=hap.py
#SBATCH --mail-type=FAIL,BEGIN,END
#SBATCH --error=%x-%J-%u.err
#SBATCH --output=%x-%J-%u.out
module --force purge
ml biocontainers hap.py
hap.py \
example/happy/PG_NA12878_chr21.vcf.gz \
example/happy/NA12878_chr21.vcf.gz \
-f example/happy/PG_Conf_chr21.bed.gz \
-r example/chr21.fa \
-o test
helen
Link to section 'Introduction' of 'helen' Introduction
HELEN is a multi-task RNN polisher which operates on images produced by MarginPolish.
Docker hub: https://hub.docker.com/r/kishwars/helen
Home page: https://github.com/kishwarshafin/helen
Link to section 'Versions' of 'helen' Versions
- 1.0
Link to section 'Commands' of 'helen' Commands
- helen
Link to section 'Module' of 'helen' Module
You can load the modules by:
module load biocontainers
module load helen
Link to section 'Example job' of 'helen' Example job
Using #!/bin/sh -l
as shebang in the slurm job script will cause the failure of some biocontainer modules. Please use #!/bin/bash
instead.
To run helen on our clusters:
#!/bin/bash
#SBATCH -A myallocation # Allocation name
#SBATCH -t 1:00:00
#SBATCH -N 1
#SBATCH -n 32
#SBATCH --job-name=helen
#SBATCH --mail-type=FAIL,BEGIN,END
#SBATCH --error=%x-%J-%u.err
#SBATCH --output=%x-%J-%u.out
module --force purge
ml biocontainers helen
helen polish \
--image_dir mp_output \
--model_path "helen_modles/HELEN_r941_guppy344_microbial.pkl" \
--threads 32 \
--output_dir "helen_output/" \
--output_prefix Staph_Aur_draft_helen
hic-pro
Link to section 'Introduction' of 'hic-pro' Introduction
Hicpro is an optimized and flexible pipeline for Hi-C data processing.
Docker hub: https://hub.docker.com/r/nservant/hicpro
Home page: https://github.com/nservant/HiC-Pro
Link to section 'Versions' of 'hic-pro' Versions
- 3.0.0
- 3.1.0
Link to section 'Commands' of 'hic-pro' Commands
- HiC-Pro
- digest_genome.py
- extract_snps.py
- hicpro2fithic.py
- hicpro2higlass.sh
- hicpro2juicebox.sh
- make_viewpoints.py
- sparseToDense.py
- split_reads.py
- split_sparse.py
Link to section 'Module' of 'hic-pro' Module
You can load the modules by:
module load biocontainers
module load hic-pro
Link to section 'Example job' of 'hic-pro' Example job
Using #!/bin/sh -l
as shebang in the slurm job script will cause the failure of some biocontainer modules. Please use #!/bin/bash
instead.
To run hic-pro on our clusters:
#!/bin/bash
#SBATCH -A myallocation # Allocation name
#SBATCH -t 1:00:00
#SBATCH -N 1
#SBATCH -n 1
#SBATCH --job-name=hic-pro
#SBATCH --mail-type=FAIL,BEGIN,END
#SBATCH --error=%x-%J-%u.err
#SBATCH --output=%x-%J-%u.out
module --force purge
ml biocontainers hic-pro
hicexplorer
Link to section 'Introduction' of 'hicexplorer' Introduction
Hicexplorer
is a set of tools to process, normalize and visualize Hi-C data.
For more information, please check its website: https://biocontainers.pro/tools/hicexplorer and its home page: https://hicexplorer.readthedocs.io/en/latest/#.
Link to section 'Versions' of 'hicexplorer' Versions
- 3.7.2
Link to section 'Commands' of 'hicexplorer' Commands
- chicAggregateStatistic
- chicDifferentialTest
- chicExportData
- chicPlotViewpoint
- chicQualityControl
- chicSignificantInteractions
- chicViewpoint
- chicViewpointBackgroundModel
- hicAdjustMatrix
- hicAggregateContacts
- hicAverageRegions
- hicBuildMatrix
- hicCompareMatrices
- hicCompartmentalization
- hicConvertFormat
- hicCorrectMatrix
- hicCorrelate
- hicCreateThresholdFile
- hicDetectLoops
- hicDifferentialTAD
- hicexplorer
- hicFindEnrichedContacts
- hicFindRestSite
- hicFindTADs
- hicHyperoptDetectLoops
- hicHyperoptDetectLoopsHiCCUPS
- hicInfo
- hicInterIntraTAD
- hicMergeDomains
- hicMergeLoops
- hicMergeMatrixBins
- hicMergeTADbins
- hicNormalize
- hicPCA
- hicPlotAverageRegions
- hicPlotDistVsCounts
- hicPlotMatrix
- hicPlotSVL
- hicPlotTADs
- hicPlotViewpoint
- hicQC
- hicQuickQC
- hicSumMatrices
- hicTADClassifier
- hicTrainTADClassifier
- hicTransform
- hicValidateLocations
Link to section 'Module' of 'hicexplorer' Module
You can load the modules by:
module load biocontainers
module load hicexplorer
Link to section 'Example job' of 'hicexplorer' Example job
Using #!/bin/sh -l
as shebang in the slurm job script will cause the failure of some biocontainer modules. Please use #!/bin/bash
instead.
To run Hicexplorer on our clusters:
#!/bin/bash
#SBATCH -A myallocation # Allocation name
#SBATCH -t 1:00:00
#SBATCH -N 1
#SBATCH -n 1
#SBATCH --job-name=hicexplorer
#SBATCH --mail-type=FAIL,BEGIN,END
#SBATCH --error=%x-%J-%u.err
#SBATCH --output=%x-%J-%u.out
module --force purge
ml biocontainers hicexplorer
hifiasm
Link to section 'Introduction' of 'hifiasm' Introduction
Hifiasm
is a fast haplotype-resolved de novo assembler for PacBio HiFi reads.
For more information, please check its website: https://biocontainers.pro/tools/hifiasm and its home page on Github.
Link to section 'Versions' of 'hifiasm' Versions
- 0.16.0
- 0.18.5
Link to section 'Commands' of 'hifiasm' Commands
- hifiasm
Link to section 'Module' of 'hifiasm' Module
You can load the modules by:
module load biocontainers
module load hifiasm
Link to section 'Example job' of 'hifiasm' Example job
Using #!/bin/sh -l
as shebang in the slurm job script will cause the failure of some biocontainer modules. Please use #!/bin/bash
instead.
To run Hifiasm on our clusters:
#!/bin/bash
#SBATCH -A myallocation # Allocation name
#SBATCH -t 1:00:00
#SBATCH -N 1
#SBATCH -n 1
#SBATCH --job-name=hifiasm
#SBATCH --mail-type=FAIL,BEGIN,END
#SBATCH --error=%x-%J-%u.err
#SBATCH --output=%x-%J-%u.out
module --force purge
ml biocontainers hifiasm
hisat2
Link to section 'Introduction' of 'hisat2' Introduction
HISAT2
is a fast and sensitive alignment program for mapping next-generation sequencing reads (both DNA and RNA) to a population of human genomes as well as to a single reference genome.
For more information, please check its website: https://biocontainers.pro/tools/hisat2.
Link to section 'Versions' of 'hisat2' Versions
- 2.2.1
Link to section 'Commands' of 'hisat2' Commands
- extract_exons.py
- extract_splice_sites.py
- hisat2
- hisat2-align-l
- hisat2-align-s
- hisat2-build
- hisat2-build-l
- hisat2-build-s
- hisat2-inspect
- hisat2-inspect-l
- hisat2-inspect-s
- hisat2_extract_exons.py
- hisat2_extract_snps_haplotypes_UCSC.py
- hisat2_extract_snps_haplotypes_VCF.py
- hisat2_extract_splice_sites.py
- hisat2_read_statistics.py
- hisat2_simulate_reads.py
Link to section 'Module' of 'hisat2' Module
You can load the modules by:
module load biocontainers
module load hisat2
Link to section 'Example job' of 'hisat2' Example job
Using #!/bin/sh -l
as shebang in the slurm job script will cause the failure of some biocontainer modules. Please use #!/bin/bash
instead.
To run HISAT2 on our clusters:
#!/bin/bash
#SBATCH -A myallocation # Allocation name
#SBATCH -t 1:00:00
#SBATCH -N 1
#SBATCH -n 1
#SBATCH --job-name=hisat2
#SBATCH --mail-type=FAIL,BEGIN,END
#SBATCH --error=%x-%J-%u.err
#SBATCH --output=%x-%J-%u.out
module --force purge
ml biocontainers hisat2
hisat2-build genome.fa genome
# for single-end FASTA reads DNA alignment
hisat2 -f -x genome -U reads.fa -S output.sam --no-spliced-alignment
# for paired-end FASTQ reads alignment
hisat2 -x genome -1 reads_1.fq -2 read2_2.fq -S output.sam
hmmer
Link to section 'Introduction' of 'hmmer' Introduction
Hmmer
is used for searching sequence databases for sequence homologs, and for making sequence alignments.
For more information, please check its website: https://biocontainers.pro/tools/hmmer and its home page: http://hmmer.org.
Link to section 'Versions' of 'hmmer' Versions
- 3.3.2
Link to section 'Commands' of 'hmmer' Commands
- alimask
- easel
- esl-afetch
- esl-alimanip
- esl-alimap
- esl-alimask
- esl-alimerge
- esl-alipid
- esl-alirev
- esl-alistat
- esl-compalign
- esl-compstruct
- esl-construct
- esl-histplot
- esl-mask
- esl-mixdchlet
- esl-reformat
- esl-selectn
- esl-seqrange
- esl-seqstat
- esl-sfetch
- esl-shuffle
- esl-ssdraw
- esl-translate
- esl-weight
- hmmalign
- hmmbuild
- hmmconvert
- hmmemit
- hmmfetch
- hmmlogo
- hmmpgmd
- hmmpgmd_shard
- hmmpress
- hmmscan
- hmmsearch
- hmmsim
- hmmstat
- jackhmmer
- makehmmerdb
- nhmmer
- nhmmscan
- phmmer
Link to section 'Module' of 'hmmer' Module
You can load the modules by:
module load biocontainers
module load hmmer
Link to section 'Example job' of 'hmmer' Example job
Using #!/bin/sh -l
as shebang in the slurm job script will cause the failure of some biocontainer modules. Please use #!/bin/bash
instead.
To run Hmmer on our clusters:
#!/bin/bash
#SBATCH -A myallocation # Allocation name
#SBATCH -t 1:00:00
#SBATCH -N 1
#SBATCH -n 1
#SBATCH --job-name=hmmer
#SBATCH --mail-type=FAIL,BEGIN,END
#SBATCH --error=%x-%J-%u.err
#SBATCH --output=%x-%J-%u.out
module --force purge
ml biocontainers hmmer
hmmsearch Nramp.hmm protein.fa > out
homer
HOMER
(Hypergeometric Optimization of Motif EnRichment) is a suite of tools for Motif Discovery and next-gen sequencing analysis. Details about its usage can be found in HOMER website.
Link to section 'Versions' of 'homer' Versions
- 4.11
Link to section 'Commands' of 'homer' Commands
- addDataHeader.pl
- addData.pl
- addGeneAnnotation.pl
- addInternalData.pl
- addOligos.pl
- adjustPeakFile.pl
- adjustRedunGroupFile.pl
- analyzeChIP-Seq.pl
- analyzeRepeats.pl
- analyzeRNA.pl
- annotateInteractions.pl
- annotatePeaks.pl
- annotateRelativePosition.pl
- annotateTranscripts.pl
- assignGeneWeights.pl
- assignTSStoGene.pl
- batchAnnotatePeaksHistogram.pl
- batchFindMotifsGenome.pl
- batchFindMotifs.pl
- batchMakeHiCMatrix.pl
- batchMakeMultiWigHub.pl
- batchMakeTagDirectory.pl
- batchParallel.pl
- bed2DtoUCSCbed.pl
- bed2pos.pl
- bed2tag.pl
- blat2gtf.pl
- bridgeResult2Cytoscape.pl
- changeNewLine.pl
- checkPeakFile.pl
- checkTagBias.pl
- chopify.pl
- chopUpBackground.pl
- chopUpPeakFile.pl
- cleanUpPeakFile.pl
- cleanUpSequences.pl
- cluster2bedgraph.pl
- cluster2bed.pl
- combineGO.pl
- combineHubs.pl
- compareMotifs.pl
- condenseBedGraph.pl
- cons2fasta.pl
- conservationAverage.pl
- conservationPerLocus.pl
- convertCoordinates.pl
- convertIDs.pl
- convertOrganismID.pl
- duplicateCol.pl
- eland2tags.pl
- fasta2tab.pl
- fastq2fasta.pl
- filterListBy.pl
- filterTADsAndCPs.pl
- filterTADsAndLoops.pl
- findcsRNATSS.pl
- findGO.pl
- findGOtxt.pl
- findHiCCompartments.pl
- findHiCDomains.pl
- findHiCInteractionsByChr.pl
- findKnownMotifs.pl
- findMotifsGenome.pl
- findMotifs.pl
- findRedundantBLAT.pl
- findTADsAndLoops.pl
- findTopMotifs.pl
- flipPC1toMatch.pl
- freq2group.pl
- genericConvertIDs.pl
- GenomeOntology.pl
- getChrLengths.pl
- getConservedRegions.pl
- getDifferentialBedGraph.pl
- getDifferentialPeaksReplicates.pl
- getDiffExpression.pl
- getDistalPeaks.pl
- getFocalPeaks.pl
- getGenesInCategory.pl
- getGWASoverlap.pl
- getHiCcorrDiff.pl
- getHomerQCstats.pl
- getLikelyAdapters.pl
- getMappingStats.pl
- getPartOfPromoter.pl
- getPos.pl
- getRandomReads.pl
- getSiteConservation.pl
- getTopPeaks.pl
- gff2pos.pl
- go2cytoscape.pl
- groupSequences.pl
- joinFiles.pl
- loadGenome.pl
- loadPromoters.pl
- makeBigBedMotifTrack.pl
- makeBigWig.pl
- makeBinaryFile.pl
- makeHiCWashUfile.pl
- makeMetaGeneProfile.pl
- makeMultiWigHub.pl
- map-fastq.pl
- merge2Dbed.pl
- mergeData.pl
- motif2Jaspar.pl
- motif2Logo.pl
- parseGTF.pl
- pos2bed.pl
- preparseGenome.pl
- prepForR.pl
- profile2seq.pl
- qseq2fastq.pl
- randomizeGroupFile.pl
- randomizeMotifs.pl
- randRemoveBackground.pl
- removeAccVersion.pl
- removeBadSeq.pl
- removeOutOfBoundsReads.pl
- removePoorSeq.pl
- removeRedundantPeaks.pl
- renamePeaks.pl
- resizePosFile.pl
- revoppMotif.pl
- rotateHiCmatrix.pl
- runHiCpca.pl
- sam2spliceJunc.pl
- scanMotifGenomeWide.pl
- scrambleFasta.pl
- selectRepeatBg.pl
- seq2profile.pl
- SIMA.pl
- subtractBedGraphsDirectory.pl
- subtractBedGraphs.pl
- tab2fasta.pl
- tag2bed.pl
- tag2pos.pl
- tagDir2bed.pl
- tagDir2hicFile.pl
- tagDir2HiCsummary.pl
- zipHomerResults.pl
Link to section 'Database' of 'homer' Database
Selected database have been downloaded for users.
ORGANISMS
: yeast, worm, mouse, arabidopsis, zebrafish, rat, human and flyPROMOTERS
: yeast, worm, mouse, arabidopsis, zebrafish, rat, human and flyGENOMES
: hg19, hg38, mm10, ce11, dm6, rn6, danRer11, tair10, and sacCer3
Link to section 'Module' of 'homer' Module
You can load the modules by:
module load biocontainers
module load homer/4.11
Link to section 'Example job' of 'homer' Example job
Using #!/bin/sh -l
as shebang in the slurm job script will cause the failure of some biocontainer modules. Please use #!/bin/bash
instead.
To run HOMER on our cluster:
#!/bin/bash
#SBATCH -A myallocation # Allocation name
#SBATCH -t 10:00:00
#SBATCH -N 1
#SBATCH -n 24
#SBATCH --job-name=homer
#SBATCH --mail-type=FAIL,BEGIN,END
#SBATCH --error=%x-%J-%u.err
#SBATCH --output=%x-%J-%u.out
module --force purge
ml biocontainers homer/4.11
configureHomer.pl -list ## Check the installed database.
findMotifs.pl mouse_geneid.txt mouse motif_out_mouse
findMotifs.pl geneid.txt human motif_out
how_are_we_stranded_here
Link to section 'Introduction' of 'how_are_we_stranded_here' Introduction
How_are_we_stranded_here
is a python package for testing strandedness of RNA-Seq fastq files.
For more information, please check its website: https://biocontainers.pro/tools/how_are_we_stranded_here and its home page on Github.
Link to section 'Versions' of 'how_are_we_stranded_here' Versions
- 1.0.1
Link to section 'Commands' of 'how_are_we_stranded_here' Commands
- check_strandedness
Link to section 'Module' of 'how_are_we_stranded_here' Module
You can load the modules by:
module load biocontainers
module load how_are_we_stranded_here
Link to section 'Example job' of 'how_are_we_stranded_here' Example job
Using #!/bin/sh -l
as shebang in the slurm job script will cause the failure of some biocontainer modules. Please use #!/bin/bash
instead.
To run How_are_we_stranded_here on our clusters:
#!/bin/bash
#SBATCH -A myallocation # Allocation name
#SBATCH -t 1:00:00
#SBATCH -N 1
#SBATCH -n 1
#SBATCH --job-name=how_are_we_stranded_here
#SBATCH --mail-type=FAIL,BEGIN,END
#SBATCH --error=%x-%J-%u.err
#SBATCH --output=%x-%J-%u.out
module --force purge
ml biocontainers how_are_we_stranded_here
check_strandedness --gtf Homo_sapiens.GRCh38.105.gtf \
--transcripts Homo_sapiens.GRCh38.cds.all.fa \
--reads_1 seq_1.fastq --reads_2 seq_2.fastq
htseq
Link to section 'Introduction' of 'htseq' Introduction
HTSeq
is a Python library to facilitate processing and analysis of data from high-throughput sequencing (HTS) experiments.
For more information, please check its website: https://biocontainers.pro/tools/htseq and its home page on Github.
Link to section 'Versions' of 'htseq' Versions
- 0.13.5
- 1.99.2
- 2.0.1
- 2.0.2
- 2.0.2-py310
Link to section 'Commands' of 'htseq' Commands
- htseq-count
- htseq-count-barcodes
- htseq-qa
- python
- python3
Link to section 'Module' of 'htseq' Module
You can load the modules by:
module load biocontainers
module load htseq
Link to section 'Example job' of 'htseq' Example job
Using #!/bin/sh -l
as shebang in the slurm job script will cause the failure of some biocontainer modules. Please use #!/bin/bash
instead.
To run HTSeq on our clusters:
#!/bin/bash
#SBATCH -A myallocation # Allocation name
#SBATCH -t 1:00:00
#SBATCH -N 1
#SBATCH -n 1
#SBATCH --job-name=htseq
#SBATCH --mail-type=FAIL,BEGIN,END
#SBATCH --error=%x-%J-%u.err
#SBATCH --output=%x-%J-%u.out
module --force purge
ml biocontainers htseq
htseq-count input.bam ref.gtf > test.out
htslib
Link to section 'Introduction' of 'htslib' Introduction
Htslib
is a C library for high-throughput sequencing data formats.
For more information, please check its website: https://biocontainers.pro/tools/htslib and its home page on Github.
Link to section 'Versions' of 'htslib' Versions
- 1.14
- 1.15
- 1.16
Link to section 'Commands' of 'htslib' Commands
- bgzip
- htsfile
- tabix
Link to section 'Module' of 'htslib' Module
You can load the modules by:
module load biocontainers
module load htslib
Link to section 'Example job' of 'htslib' Example job
Using #!/bin/sh -l
as shebang in the slurm job script will cause the failure of some biocontainer modules. Please use #!/bin/bash
instead.
To run Htslib on our clusters:
#!/bin/bash
#SBATCH -A myallocation # Allocation name
#SBATCH -t 1:00:00
#SBATCH -N 1
#SBATCH -n 1
#SBATCH --job-name=htslib
#SBATCH --mail-type=FAIL,BEGIN,END
#SBATCH --error=%x-%J-%u.err
#SBATCH --output=%x-%J-%u.out
module --force purge
ml biocontainers htslib
tabix sorted.gff.gz chr1:10,000,000-20,000,000
htstream
Link to section 'Introduction' of 'htstream' Introduction
Htstream
is a quality control and processing pipeline for High Throughput Sequencing data.
For more information, please check its website: https://biocontainers.pro/tools/htstream and its home page on Github.
Link to section 'Versions' of 'htstream' Versions
- 1.3.3
Link to section 'Commands' of 'htstream' Commands
- hts_AdapterTrimmer
- hts_CutTrim
- hts_LengthFilter
- hts_NTrimmer
- hts_Overlapper
- hts_PolyATTrim
- hts_Primers
- hts_QWindowTrim
- hts_SeqScreener
- hts_Stats
- hts_SuperDeduper
Link to section 'Module' of 'htstream' Module
You can load the modules by:
module load biocontainers
module load htstream
Link to section 'Example job' of 'htstream' Example job
Using #!/bin/sh -l
as shebang in the slurm job script will cause the failure of some biocontainer modules. Please use #!/bin/bash
instead.
To run Htstream on our clusters:
#!/bin/bash
#SBATCH -A myallocation # Allocation name
#SBATCH -t 1:00:00
#SBATCH -N 1
#SBATCH -n 1
#SBATCH --job-name=htstream
#SBATCH --mail-type=FAIL,BEGIN,END
#SBATCH --error=%x-%J-%u.err
#SBATCH --output=%x-%J-%u.out
module --force purge
ml biocontainers htstream
humann
Link to section 'Introduction' of 'humann' Introduction
HUMAnN 3.0
is the next iteration of HUMAnN, the HMP Unified Metabolic Analysis Network. HUMAnN is a method for efficiently and accurately profiling the abundance of microbial metabolic pathways and other molecular functions from metagenomic or metatranscriptomic sequencing data.
For more information please check its website: https://huttenhower.sph.harvard.edu/humann/
Link to section 'Versions' of 'humann' Versions
- 3.0.0
- 3.6
Link to section 'Commands' of 'humann' Commands
- humann
- humann3
- humann3_databases
- humann_barplot
- humann_benchmark
- humann_build_custom_database
- humann_config
- humann_databases
- humann_genefamilies_genus_level
- humann_infer_taxonomy
- humann_join_tables
- humann_reduce_table
- humann_regroup_table
- humann_rename_table
- humann_renorm_table
- humann_split_stratified_table
- humann_split_table
- humann_test
- humann_unpack_pathways
Link to section 'Database ' of 'humann' Database
Full ChocoPhlAn, UniRef90, EC-filtered UniRef90, UniRef50, EC-filtered UniRef50, and utility_mapping databases have been downloaded for users.
Link to section 'Module' of 'humann' Module
You can load the modules by:
module load biocontainers
module load humann/3.0.0
Link to section 'Example job' of 'humann' Example job
Using #!/bin/sh -l
as shebang in the slurm job script will cause the failure of some biocontainer modules. Please use #!/bin/bash
instead.
To run HUMAnN3 on our cluster:
#!/bin/bash
#SBATCH -A myallocation # Allocation name
#SBATCH -t 10:00:00
#SBATCH -N 1
#SBATCH -n 24
#SBATCH --job-name=humann
#SBATCH --mail-type=FAIL,BEGIN,END
#SBATCH --error=%x-%J-%u.err
#SBATCH --output=%x-%J-%u.out
module --force purge
ml biocontainers humann/3.0.0
# Check the database and config by:
humann_config --print
humann --threads 24 --input examples/demo.fastq --output demo_output --metaphlan-options "--bowtie2db /depot/itap/datasets/metaphlan"
hyphy
Link to section 'Introduction' of 'hyphy' Introduction
Hyphy
is an open-source software package for the analysis of genetic sequences using techniques in phylogenetics, molecular evolution, and machine learning.
For more information, please check its website: https://biocontainers.pro/tools/hyphy and its home page on Github.
Link to section 'Versions' of 'hyphy' Versions
- 2.5.36
Link to section 'Commands' of 'hyphy' Commands
- hyphy
Link to section 'Module' of 'hyphy' Module
You can load the modules by:
module load biocontainers
module load hyphy
Link to section 'Example job' of 'hyphy' Example job
Using #!/bin/sh -l
as shebang in the slurm job script will cause the failure of some biocontainer modules. Please use #!/bin/bash
instead.
To run Hyphy on our clusters:
#!/bin/bash
#SBATCH -A myallocation # Allocation name
#SBATCH -t 1:00:00
#SBATCH -N 1
#SBATCH -n 1
#SBATCH --job-name=hyphy
#SBATCH --mail-type=FAIL,BEGIN,END
#SBATCH --error=%x-%J-%u.err
#SBATCH --output=%x-%J-%u.out
module --force purge
ml biocontainers hyphy
idba
Link to section 'Introduction' of 'idba' Introduction
Idba
is a practical iterative De Bruijn Graph De Novo Assembler for sequence assembly in bioinformatics.
For more information, please check its website: https://biocontainers.pro/tools/idba and its home page: https://i.cs.hku.hk/~alse/hkubrg/projects/idba/index.html.
Link to section 'Versions' of 'idba' Versions
- 1.1.3
Link to section 'Commands' of 'idba' Commands
- fa2fq
- filter_blat
- filter_contigs
- filterfa
- fq2fa
- idba
- idba_hybrid
- idba_tran
- idba_tran_test
- idba_ud
- parallel_blat
- parallel_rna_blat
- print_graph
- raw_n50
- run-unittest.py
- sample_reads
- scaffold
- scan.py
- shuffle_reads
- sim_reads
- sim_reads_tran
- sort_psl
- sort_reads
- split_fa
- split_fq
- split_scaffold
- test
- validate_blat
- validate_blat_parallel
- validate_component
- validate_contigs_blat
- validate_contigs_mummer
- validate_reads_blat
- validate_rna
Link to section 'Module' of 'idba' Module
You can load the modules by:
module load biocontainers
module load idba
Link to section 'Example job' of 'idba' Example job
Using #!/bin/sh -l
as shebang in the slurm job script will cause the failure of some biocontainer modules. Please use #!/bin/bash
instead.
To run Idba on our clusters:
#!/bin/bash
#SBATCH -A myallocation # Allocation name
#SBATCH -t 1:00:00
#SBATCH -N 1
#SBATCH -n 1
#SBATCH --job-name=idba
#SBATCH --mail-type=FAIL,BEGIN,END
#SBATCH --error=%x-%J-%u.err
#SBATCH --output=%x-%J-%u.out
module --force purge
ml biocontainers idba
fq2fa --paired --filter SRR1977249.abundtrim.subset.pe.fq SRR1977249.abundtrim.subset.pe.fa
idba_ud -r SRR1977249.abundtrim.subset.pe.fa -o output
igv
Link to section 'Introduction' of 'igv' Introduction
IGV
(Integrative Genomics Viewer) is a high-performance, easy-to-use, interactive tool for the visual exploration of genomic data.
For more information, please check its home page: https://software.broadinstitute.org/software/igv/home.
Link to section 'Versions' of 'igv' Versions
- 2.11.9
- 2.12.3
Link to section 'Commands' of 'igv' Commands
- igv_hidpi.sh
- igv.sh
Link to section 'Module' of 'igv' Module
You can load the modules by:
module load biocontainers
module load igv
Link to section 'Interactive job' of 'igv' Interactive job
Since IGV requires GUI, it is recommended to run it within ThinLinc:
(base) UserID@bell-fe00:~ $ sinteractive -N1 -n12 -t4:00:00 -A myallocation
salloc: Granted job allocation 12345869
salloc: Waiting for resource configuration
salloc: Nodes bell-a008 are ready for job
(base) UserID@bell-a008:~ $ module --force purge
(base) UserID@bell-a008:~ $ ml biocontainers igv
(base) UserID@bell-a008:~ $ igv.sh
impute2
Link to section 'Introduction' of 'impute2' Introduction
Impute2
is a genotype imputation and haplotype phasing program.
For more information, please check its website: https://biocontainers.pro/tools/impute2 and its home page: https://mathgen.stats.ox.ac.uk/impute/impute_v2.html#home.
Link to section 'Versions' of 'impute2' Versions
- 2.3.2
Link to section 'Commands' of 'impute2' Commands
- impute2
Link to section 'Module' of 'impute2' Module
You can load the modules by:
module load biocontainers
module load impute2
Link to section 'Example job' of 'impute2' Example job
Using #!/bin/sh -l
as shebang in the slurm job script will cause the failure of some biocontainer modules. Please use #!/bin/bash
instead.
To run Impute2 on our clusters:
#!/bin/bash
#SBATCH -A myallocation # Allocation name
#SBATCH -t 1:00:00
#SBATCH -N 1
#SBATCH -n 1
#SBATCH --job-name=impute2
#SBATCH --mail-type=FAIL,BEGIN,END
#SBATCH --error=%x-%J-%u.err
#SBATCH --output=%x-%J-%u.out
module --force purge
ml biocontainers impute2
impute2 \
-m Example/example.chr22.map \
-h Example/example.chr22.1kG.haps \
-l Example/example.chr22.1kG.legend \
-g Example/example.chr22.study.gens \
-strand_g Example/example.chr22.study.strand \
-int 20.4e6 20.5e6 \
-Ne 20000 \
-o example.chr22.one.phased.impute2
infernal
Link to section 'Introduction' of 'infernal' Introduction
Infernal ("INFERence of RNA ALignment") is for searching DNA sequence databases for RNA structure and sequence similarities. It is an implementation of a special case of profile stochastic context-free grammars called covariance models (CMs). A CM is like a sequence profile, but it scores a combination of sequence consensus and RNA secondary structure consensus, so in many cases, it is more capable of identifying RNA homologs that conserve their secondary structure more than their primary sequence.
For more information, please check:
BioContainers: https://biocontainers.pro/tools/infernal
Home page: http://eddylab.org/infernal/
Link to section 'Versions' of 'infernal' Versions
- 1.1.4
Link to section 'Commands' of 'infernal' Commands
- cmalign
- cmbuild
- cmcalibrate
- cmconvert
- cmemit
- cmfetch
- cmpress
- cmscan
- cmsearch
- cmstat
Link to section 'Module' of 'infernal' Module
You can load the modules by:
module load biocontainers
module load infernal
Link to section 'Example job' of 'infernal' Example job
Using #!/bin/sh -l
as shebang in the slurm job script will cause the failure of some biocontainer modules. Please use #!/bin/bash
instead.
To run infernal on our clusters:
#!/bin/bash
#SBATCH -A myallocation # Allocation name
#SBATCH -t 1:00:00
#SBATCH -N 1
#SBATCH -n 1
#SBATCH --job-name=infernal
#SBATCH --mail-type=FAIL,BEGIN,END
#SBATCH --error=%x-%J-%u.err
#SBATCH --output=%x-%J-%u.out
module --force purge
ml biocontainers infernal
instrain
Link to section 'Introduction' of 'instrain' Introduction
Instrain
is a python program for analysis of co-occurring genome populations from metagenomes that allows highly accurate genome comparisons, analysis of coverage, microdiversity, and linkage, and sensitive SNP detection with gene localization and synonymous non-synonymous identification.
For more information, please check its website: https://biocontainers.pro/tools/instrain and its home page on Github.
Link to section 'Versions' of 'instrain' Versions
- 1.5.7
- 1.6.3
Link to section 'Commands' of 'instrain' Commands
- inStrain
Link to section 'Module' of 'instrain' Module
You can load the modules by:
module load biocontainers
module load instrain
Link to section 'Example job' of 'instrain' Example job
Using #!/bin/sh -l
as shebang in the slurm job script will cause the failure of some biocontainer modules. Please use #!/bin/bash
instead.
To run Instrain on our clusters:
#!/bin/bash
#SBATCH -A myallocation # Allocation name
#SBATCH -t 1:00:00
#SBATCH -N 1
#SBATCH -n 1
#SBATCH --job-name=instrain
#SBATCH --mail-type=FAIL,BEGIN,END
#SBATCH --error=%x-%J-%u.err
#SBATCH --output=%x-%J-%u.out
module --force purge
ml biocontainers instrain
intarna
Link to section 'Introduction' of 'intarna' Introduction
Intarna
is a general and fast approach to the prediction of RNA-RNA interactions incorporating both the accessibility of interacting sites as well as the existence of a user-definable seed interaction.
For more information, please check its website: https://biocontainers.pro/tools/intarna and its home page on Github.
Link to section 'Versions' of 'intarna' Versions
- 3.3.1
Link to section 'Commands' of 'intarna' Commands
- IntaRNA
Link to section 'Module' of 'intarna' Module
You can load the modules by:
module load biocontainers
module load intarna
Link to section 'Example job' of 'intarna' Example job
Using #!/bin/sh -l
as shebang in the slurm job script will cause the failure of some biocontainer modules. Please use #!/bin/bash
instead.
To run Intarna on our clusters:
#!/bin/bash
#SBATCH -A myallocation # Allocation name
#SBATCH -t 1:00:00
#SBATCH -N 1
#SBATCH -n 1
#SBATCH --job-name=intarna
#SBATCH --mail-type=FAIL,BEGIN,END
#SBATCH --error=%x-%J-%u.err
#SBATCH --output=%x-%J-%u.out
module --force purge
ml biocontainers intarna
IntaRNA -t CCCCCCCCGGGGGGGGGGGGGG -q AAAACCCCCCCUUUU
interproscan
InterPro
is a database which integrates together predictive information about proteins’ function from a number of partner resources, giving an overview of the families that a protein belongs to and the domains and sites it contains.
Users who have novel nucleotide or protein sequences that they wish to functionally characterise can use the software package InterProScan
to run the scanning algorithms from the InterPro database in an integrated way. Sequences are submitted in FASTA format. Matches are then calculated against all of the required member database’s signatures and the results are then output in a variety of formats.
Link to section 'Versions' of 'interproscan' Versions
- 5.54_87.0
Link to section 'Commands' of 'interproscan' Commands
interproscan.sh
Link to section 'Database' of 'interproscan' Database
Latest version of database has been downloaded and setup in /depot/itap/datasets/interproscan-5.54-87.0/data.
Link to section 'Module' of 'interproscan' Module
You can load the modules by:
module load biocontainers
module load interproscan/5.54_87.0
Link to section 'Example job' of 'interproscan' Example job
Using #!/bin/sh -l
as shebang in the slurm job script will cause the failure of some biocontainer modules. Please use #!/bin/bash
instead.
To run run_dbcan on our cluster:
#!/bin/bash
#SBATCH -A myallocation # Allocation name
#SBATCH -t 10:00:00
#SBATCH -N 1
#SBATCH -n 24
#SBATCH --job-name=interproscan
#SBATCH --mail-type=FAIL,BEGIN,END
#SBATCH --error=%x-%J-%u.err
#SBATCH --output=%x-%J-%u.out
module --force purge
ml biocontainers interproscan/5.54_87.0
interproscan.sh -cpu 24 -i test_proteins.fasta
interproscan.sh -cpu 24 -t n -i test_nt_seqs.fasta
iqtree
Link to section 'Introduction' of 'iqtree' Introduction
IQ-TREE
is an efficient phylogenomic software by maximum likelihood.
For more information, please check its website: https://biocontainers.pro/tools/iqtree and its home page: http://www.iqtree.org.
Link to section 'Versions' of 'iqtree' Versions
- 1.6.12
- 2.1.2
- 2.2.0_beta
Link to section 'Commands' of 'iqtree' Commands
- iqtree
Link to section 'Module' of 'iqtree' Module
You can load the modules by:
module load biocontainers
module load iqtree
Link to section 'Example job' of 'iqtree' Example job
Using #!/bin/sh -l
as shebang in the slurm job script will cause the failure of some biocontainer modules. Please use #!/bin/bash
instead.
To run IQ-TREE on our clusters:
#!/bin/bash
#SBATCH -A myallocation # Allocation name
#SBATCH -t 1:00:00
#SBATCH -N 1
#SBATCH -n 1
#SBATCH --job-name=iqtree
#SBATCH --mail-type=FAIL,BEGIN,END
#SBATCH --error=%x-%J-%u.err
#SBATCH --output=%x-%J-%u.out
module --force purge
ml biocontainers iqtree
iqtree -s input.phy -m GTR+I+G > test.out
isoquant
Link to section 'Introduction' of 'isoquant' Introduction
IsoQuant is a tool for the genome-based analysis of long RNA reads, such as PacBio or Oxford Nanopores. IsoQuant allows to reconstruct and quantify transcript models with high precision and decent recall. If the reference annotation is given, IsoQuant also assigns reads to the annotated isoforms based on their intron and exon structure. IsoQuant further performs annotated gene, isoform, exon and intron quantification. If reads are grouped (e.g. according to cell type), counts are reported according to the provided grouping.
BioContainers: https://biocontainers.pro/tools/isoquant
Home page: https://github.com/ablab/IsoQuant
Link to section 'Versions' of 'isoquant' Versions
- 3.1.2
Link to section 'Commands' of 'isoquant' Commands
- isoquant.py
Link to section 'Module' of 'isoquant' Module
You can load the modules by:
module load biocontainers
module load isoquant
Link to section 'Example job' of 'isoquant' Example job
Using #!/bin/sh -l
as shebang in the slurm job script will cause the failure of some biocontainer modules. Please use #!/bin/bash
instead.
To run isoquant on our clusters:
#!/bin/bash
#SBATCH -A myallocation # Allocation name
#SBATCH -t 1:00:00
#SBATCH -N 1
#SBATCH -n 1
#SBATCH --job-name=isoquant
#SBATCH --mail-type=FAIL,BEGIN,END
#SBATCH --error=%x-%J-%u.err
#SBATCH --output=%x-%J-%u.out
module --force purge
ml biocontainers isoquant
isoquant.py --reference chr9.4M.fa.gz \
--genedb chr9.4M.gtf.gz \
--fastq chr9.4M.ont.sim.fq.gz \
--data_type nanopore -o test_ont
isoseq3
Link to section 'Introduction' of 'isoseq3' Introduction
Isoseq3
- Scalable De Novo Isoform Discovery.
For more information, please check its website: https://biocontainers.pro/tools/isoseq3 and its home page on Github.
Link to section 'Versions' of 'isoseq3' Versions
- 3.4.0
- 3.7.0
- 3.8.2
Link to section 'Commands' of 'isoseq3' Commands
- isoseq3
Link to section 'Module' of 'isoseq3' Module
You can load the modules by:
module load biocontainers
module load isoseq3
Link to section 'Example job' of 'isoseq3' Example job
Using #!/bin/sh -l
as shebang in the slurm job script will cause the failure of some biocontainer modules. Please use #!/bin/bash
instead.
To run Isoseq3 on our clusters:
#!/bin/bash
#SBATCH -A myallocation # Allocation name
#SBATCH -t 1:00:00
#SBATCH -N 1
#SBATCH -n 1
#SBATCH --job-name=isoseq3
#SBATCH --mail-type=FAIL,BEGIN,END
#SBATCH --error=%x-%J-%u.err
#SBATCH --output=%x-%J-%u.out
module --force purge
ml biocontainers isoseq3
isoseq3 --version
isoseq3 refine --require-polya \
alz.demult.5p--3p.bam \
primers.fasta alz.flnc.bam
isoseq3 cluster alz.flnc.bam \
alz.polished.bam --verbose --use-qvs
ivar
Link to section 'Introduction' of 'ivar' Introduction
Ivar is a computational package that contains functions broadly useful for viral amplicon-based sequencing.
Docker hub: https://hub.docker.com/r/andersenlabapps/ivar/
Home page: https://github.com/andersen-lab/ivar
Link to section 'Versions' of 'ivar' Versions
- 1.3.1
Link to section 'Commands' of 'ivar' Commands
- ivar
Link to section 'Module' of 'ivar' Module
You can load the modules by:
module load biocontainers
module load ivar
Link to section 'Example job' of 'ivar' Example job
Using #!/bin/sh -l
as shebang in the slurm job script will cause the failure of some biocontainer modules. Please use #!/bin/bash
instead.
To run ivar on our clusters:
#!/bin/bash
#SBATCH -A myallocation # Allocation name
#SBATCH -t 1:00:00
#SBATCH -N 1
#SBATCH -n 1
#SBATCH --job-name=ivar
#SBATCH --mail-type=FAIL,BEGIN,END
#SBATCH --error=%x-%J-%u.err
#SBATCH --output=%x-%J-%u.out
module --force purge
ml biocontainers ivar
jcvi
Link to section 'Introduction' of 'jcvi' Introduction
Jcvi is a collection of Python libraries to parse bioinformatics files, or perform computation related to assembly, annotation, and comparative genomics.
Home page: https://github.com/tanghaibao/jcvi
Link to section 'Versions' of 'jcvi' Versions
- 1.2.7
- 1.3.1
Link to section 'Commands' of 'jcvi' Commands
- python
- python3
Link to section 'Module' of 'jcvi' Module
You can load the modules by:
module load biocontainers
module load jcvi
Link to section 'Example job' of 'jcvi' Example job
Using #!/bin/sh -l
as shebang in the slurm job script will cause the failure of some biocontainer modules. Please use #!/bin/bash
instead.
To run jcvi on our clusters:
#!/bin/bash
#SBATCH -A myallocation # Allocation name
#SBATCH -t 1:00:00
#SBATCH -N 1
#SBATCH -n 1
#SBATCH --job-name=jcvi
#SBATCH --mail-type=FAIL,BEGIN,END
#SBATCH --error=%x-%J-%u.err
#SBATCH --output=%x-%J-%u.out
module --force purge
ml biocontainers jcvi
python -m jcvi.formats.fasta format Vvinifera_145_Genoscope.12X.cds.fa.gz grape.cds
python -m jcvi.formats.fasta format Ppersica_298_v2.1.cds.fa.gz peach.cds
python -m jcvi.formats.gff bed --type=mRNA --key=Name --primary_only Vvinifera_145_Genoscope.12X.gene.gff3.gz -o grape.bed
python -m jcvi.compara.catalog ortholog grape peach --no_strip_names
python -m jcvi.graphics.dotplot grape.peach.anchors
rm grape.peach.last.filtered
python -m jcvi.compara.catalog ortholog grape peach --cscore=.99 --no_strip_names
python -m jcvi.graphics.dotplot grape.peach.anchors
python -m jcvi.compara.synteny depth --histogram grape.peach.anchors
python -m jcvi.graphics.grabseeds seeds test-data/test.JPG
kaiju
Link to section 'Introduction' of 'kaiju' Introduction
Kaiju
is a tool for fast taxonomic classification of metagenomic sequencing reads using a protein reference database.
For more information, please check its website: https://biocontainers.pro/tools/kaiju and its home page on Github.
Link to section 'Versions' of 'kaiju' Versions
- 1.8.2
Link to section 'Commands' of 'kaiju' Commands
- kaiju
- kaiju-addTaxonNames
- kaiju-convertMAR.py
- kaiju-convertNR
- kaiju-excluded-accessions.txt
- kaiju-gbk2faa.pl
- kaiju-makedb
- kaiju-mergeOutputs
- kaiju-mkbwt
- kaiju-mkfmi
- kaiju-multi
- kaiju-taxonlistEuk.tsv
- kaiju2krona
- kaiju2table
- kaijup
- kaijux
Link to section 'Module' of 'kaiju' Module
You can load the modules by:
module load biocontainers
module load kaiju
Link to section 'Example job' of 'kaiju' Example job
Using #!/bin/sh -l
as shebang in the slurm job script will cause the failure of some biocontainer modules. Please use #!/bin/bash
instead.
To run Kaiju on our clusters:
#!/bin/bash
#SBATCH -A myallocation # Allocation name
#SBATCH -t 1:00:00
#SBATCH -N 1
#SBATCH -n 24
#SBATCH --job-name=kaiju
#SBATCH --mail-type=FAIL,BEGIN,END
#SBATCH --error=%x-%J-%u.err
#SBATCH --output=%x-%J-%u.out
module --force purge
ml biocontainers kaiju
kaiju -t kaijudb/nodes.dmp \
-f kaijudb/refseq/kaiju_db_refseq.fmi \
-i input_1.fastq -j input_2.fastq
-z 24
kakscalculator2
Link to section 'Introduction' of 'kakscalculator2' Introduction
kakscalculator2 is a toolkit of incorporating gamma series methods and sliding window strategies.
Home page: https://github.com/kullrich/kakscalculator2
Link to section 'Versions' of 'kakscalculator2' Versions
- 2.0.1
Link to section 'Commands' of 'kakscalculator2' Commands
- KaKs_Calculator
Link to section 'Module' of 'kakscalculator2' Module
You can load the modules by:
module load biocontainers
module load kakscalculator2
Link to section 'Example job' of 'kakscalculator2' Example job
Using #!/bin/sh -l
as shebang in the slurm job script will cause the failure of some biocontainer modules. Please use #!/bin/bash
instead.
To run kakscalculator2 on our clusters:
#!/bin/bash
#SBATCH -A myallocation # Allocation name
#SBATCH -t 1:00:00
#SBATCH -N 1
#SBATCH -n 1
#SBATCH --job-name=kakscalculator2
#SBATCH --mail-type=FAIL,BEGIN,END
#SBATCH --error=%x-%J-%u.err
#SBATCH --output=%x-%J-%u.out
module --force purge
ml biocontainers kakscalculator2
KaKs_Calculator -i example.axt -o example.axt.kaks -m YN
kallisto
Kallisto
is a program for quantifying abundances of transcripts from RNA-Seq data, or more generally of target sequences using high-throughput sequencing reads. It is based on the novel idea of pseudoalignment for rapidly determining the compatibility of reads with targets, without the need for alignment.
Detailed usage can be found here: https://github.com/pachterlab/kallisto
Link to section 'Versions' of 'kallisto' Versions
- 0.46.2
- 0.48.0
Link to section 'Commands' of 'kallisto' Commands
- kallisto
Link to section 'Module' of 'kallisto' Module
You can load the modules by:
module load biocontainers
module load kallisto/0.48.0
Link to section 'Example job' of 'kallisto' Example job
Using #!/bin/sh -l
as shebang in the slurm job script will cause the failure of some biocontainer modules. Please use #!/bin/bash
instead.
To run kallisto on our our clusters:
#!/bin/bash
#SBATCH -A myallocation # Allocation name
#SBATCH -t 10:00:00
#SBATCH -N 1
#SBATCH -n 24
#SBATCH --job-name=kallisto
#SBATCH --mail-type=FAIL,BEGIN,END
#SBATCH --error=%x-%J-%u.err
#SBATCH --output=%x-%J-%u.out
module --force purge
ml biocontainers kallisto/0.48.0
kallisto index -i transcripts.idx Homo_sapiens.GRCh38.cds.all.fa.gz
kallisto quant -t 24 -i transcripts.idx -o output -b 100 SRR11614709_1.fastq SRR11614709_2.fastq
khmer
Link to section 'Introduction' of 'khmer' Introduction
Khmer
is a tool for k-mer counting, filtering, and graph traversal FTW!
For more information, please check its website: https://biocontainers.pro/tools/khmer and its home page on Github.
Link to section 'Versions' of 'khmer' Versions
- 3.0.0a3
Link to section 'Commands' of 'khmer' Commands
- abundance-dist.py
- abundance-dist-single.py
- annotate-partitions.py
- count-median.py
- cygdb
- cython
- cythonize
- do-partition.py
- extract-long-sequences.py
- extract-paired-reads.py
- extract-partitions.py
- fastq-to-fasta.py
- filter-abund.py
- filter-abund-single.py
- filter-stoptags.py
- find-knots.py
- interleave-reads.py
- load-graph.py
- load-into-counting.py
- make-initial-stoptags.py
- merge-partitions.py
- normalize-by-median.py
- partition-graph.py
- readstats.py
- sample-reads-randomly.py
- screed
- split-paired-reads.py
- trim-low-abund.py
- unique-kmers.py
Link to section 'Module' of 'khmer' Module
You can load the modules by:
module load biocontainers
module load khmer
Link to section 'Example job' of 'khmer' Example job
Using #!/bin/sh -l
as shebang in the slurm job script will cause the failure of some biocontainer modules. Please use #!/bin/bash
instead.
To run Khmer on our clusters:
#!/bin/bash
#SBATCH -A myallocation # Allocation name
#SBATCH -t 1:00:00
#SBATCH -N 1
#SBATCH -n 1
#SBATCH --job-name=khmer
#SBATCH --mail-type=FAIL,BEGIN,END
#SBATCH --error=%x-%J-%u.err
#SBATCH --output=%x-%J-%u.out
module --force purge
ml biocontainers khmer
kissde
Link to section 'Introduction' of 'kissde' Introduction
kissDE is a R package, similar to DEseq, but which works on pairs of variants, and tests if a variant is enriched in one condition. It has been developed to work easily with KisSplice output. It can also work with a simple table of counts obtained by any other means. It requires at least two replicates per condition and at least two conditions.
Docker hub: https://hub.docker.com/r/dwishsan/kissplice-pipeline
Home page: https://kissplice.prabi.fr
Link to section 'Versions' of 'kissde' Versions
- 1.15.3
Link to section 'Commands' of 'kissde' Commands
- R
- Rscript
- kissDE.R
Link to section 'Module' of 'kissde' Module
You can load the modules by:
module load biocontainers
module load kissde
Link to section 'Example job' of 'kissde' Example job
Using #!/bin/sh -l
as shebang in the slurm job script will cause the failure of some biocontainer modules. Please use #!/bin/bash
instead.
To run kissde on our clusters:
#!/bin/bash
#SBATCH -A myallocation # Allocation name
#SBATCH -t 1:00:00
#SBATCH -N 1
#SBATCH -n 1
#SBATCH --job-name=kissde
#SBATCH --mail-type=FAIL,BEGIN,END
#SBATCH --error=%x-%J-%u.err
#SBATCH --output=%x-%J-%u.out
module --force purge
ml biocontainers kissde
kissplice
Link to section 'Introduction' of 'kissplice' Introduction
KisSplice is a software that enables to analyse RNA-seq data with or without a reference genome. It is an exact local transcriptome assembler that allows to identify SNPs, indels and alternative splicing events. It can deal with an arbitrary number of biological conditions, and will quantify each variant in each condition. It has been tested on Illumina datasets of up to 1G reads. Its memory consumption is around 5Gb for 100M reads.
Docker hub: https://hub.docker.com/r/dwishsan/kissplice-pipeline
Home page: https://kissplice.prabi.fr
Link to section 'Versions' of 'kissplice' Versions
- 2.6.2
Link to section 'Commands' of 'kissplice' Commands
- kissplice
Link to section 'Module' of 'kissplice' Module
You can load the modules by:
module load biocontainers
module load kissplice
Link to section 'Example job' of 'kissplice' Example job
Using #!/bin/sh -l
as shebang in the slurm job script will cause the failure of some biocontainer modules. Please use #!/bin/bash
instead.
To run kissplice on our clusters:
#!/bin/bash
#SBATCH -A myallocation # Allocation name
#SBATCH -t 1:00:00
#SBATCH -N 1
#SBATCH -n 1
#SBATCH --job-name=kissplice
#SBATCH --mail-type=FAIL,BEGIN,END
#SBATCH --error=%x-%J-%u.err
#SBATCH --output=%x-%J-%u.out
module --force purge
ml biocontainers kissplice
kissplice2refgenome
Link to section 'Introduction' of 'kissplice2refgenome' Introduction
KisSplice can also be used when a reference (annotated) genome is available, in order to annotate the variants found and help prioritize cases to validate experimentally. In this case, the results of KisSplice are mapped to the reference genome, using for instance STAR, and the mapping results are analysed using KisSplice2RefGenome.
Docker hub: https://hub.docker.com/r/dwishsan/kissplice-pipeline
Home page: https://kissplice.prabi.fr
Link to section 'Versions' of 'kissplice2refgenome' Versions
- 2.0.8
Link to section 'Commands' of 'kissplice2refgenome' Commands
- kissplice2refgenome
Link to section 'Module' of 'kissplice2refgenome' Module
You can load the modules by:
module load biocontainers
module load kissplice2refgenome
Link to section 'Example job' of 'kissplice2refgenome' Example job
Using #!/bin/sh -l
as shebang in the slurm job script will cause the failure of some biocontainer modules. Please use #!/bin/bash
instead.
To run kissplice2refgenome on our clusters:
#!/bin/bash
#SBATCH -A myallocation # Allocation name
#SBATCH -t 1:00:00
#SBATCH -N 1
#SBATCH -n 1
#SBATCH --job-name=kissplice2refgenome
#SBATCH --mail-type=FAIL,BEGIN,END
#SBATCH --error=%x-%J-%u.err
#SBATCH --output=%x-%J-%u.out
module --force purge
ml biocontainers kissplice2refgenome
kma
Link to section 'Introduction' of 'kma' Introduction
KMA is a mapping method designed to map raw reads directly against redundant databases, in an ultra-fast manner using seed and extend.
BioContainers: https://biocontainers.pro/tools/kma
Home page: https://bitbucket.org/genomicepidemiology/kma/src/master/
Link to section 'Versions' of 'kma' Versions
- 1.4.3
Link to section 'Commands' of 'kma' Commands
- kma
- kma_index
- kma_shm
- kma_update
Link to section 'Module' of 'kma' Module
You can load the modules by:
module load biocontainers
module load kma
Link to section 'Example job' of 'kma' Example job
Using #!/bin/sh -l
as shebang in the slurm job script will cause the failure of some biocontainer modules. Please use #!/bin/bash
instead.
To run kma on our clusters:
#!/bin/bash
#SBATCH -A myallocation # Allocation name
#SBATCH -t 1:00:00
#SBATCH -N 1
#SBATCH -n 1
#SBATCH --job-name=kma
#SBATCH --mail-type=FAIL,BEGIN,END
#SBATCH --error=%x-%J-%u.err
#SBATCH --output=%x-%J-%u.out
module --force purge
ml biocontainers kma
kmc
Link to section 'Introduction' of 'kmc' Introduction
Kmc
is a tool for efficient k-mer counting and filtering of reads based on k-mer content.
For more information, please check its website: https://biocontainers.pro/tools/kmc and its home page on Github.
Link to section 'Versions' of 'kmc' Versions
- 3.2.1
Link to section 'Commands' of 'kmc' Commands
- kmc
- kmc_dump
- kmc_tools
Link to section 'Module' of 'kmc' Module
You can load the modules by:
module load biocontainers
module load kmc
Link to section 'Example job' of 'kmc' Example job
Using #!/bin/sh -l
as shebang in the slurm job script will cause the failure of some biocontainer modules. Please use #!/bin/bash
instead.
To run Kmc on our clusters:
#!/bin/bash
#SBATCH -A myallocation # Allocation name
#SBATCH -t 1:00:00
#SBATCH -N 1
#SBATCH -n 1
#SBATCH --job-name=kmc
#SBATCH --mail-type=FAIL,BEGIN,END
#SBATCH --error=%x-%J-%u.err
#SBATCH --output=%x-%J-%u.out
module --force purge
ml biocontainers kmc
kmc -k27 seq.fastq 27mers .
kmer-jellyfish
Link to section 'Introduction' of 'kmer-jellyfish' Introduction
Jellyfish
is a tool for fast, memory-efficient counting of k-mers in DNA. A k-mer is a substring of length k, and counting the occurrences of all such substrings is a central step in many analyses of DNA sequence.
For more information, please check its website: https://biocontainers.pro/tools/kmer-jellyfish and its home page: http://www.genome.umd.edu/jellyfish.html.
Link to section 'Versions' of 'kmer-jellyfish' Versions
- 2.3.0
Link to section 'Commands' of 'kmer-jellyfish' Commands
- jellyfish
Link to section 'Module' of 'kmer-jellyfish' Module
You can load the modules by:
module load biocontainers
module load kmer-jellyfish
Link to section 'Example job' of 'kmer-jellyfish' Example job
Using #!/bin/sh -l
as shebang in the slurm job script will cause the failure of some biocontainer modules. Please use #!/bin/bash
instead.
To run Jellyfish on our clusters:
#!/bin/bash
#SBATCH -A myallocation # Allocation name
#SBATCH -t 1:00:00
#SBATCH -N 1
#SBATCH -n 12
#SBATCH --job-name=kmer-jellyfish
#SBATCH --mail-type=FAIL,BEGIN,END
#SBATCH --error=%x-%J-%u.err
#SBATCH --output=%x-%J-%u.out
module --force purge
ml biocontainers kmer-jellyfish
jellyfish count -m 16 -s 100M -t 12 \
-o mer_counts -c 7 input.fastq
kmergenie
Link to section 'Introduction' of 'kmergenie' Introduction
KmerGenie estimates the best k-mer length for genome de novo assembly.
BioContainers: https://biocontainers.pro/tools/kmergenie
Home page: http://kmergenie.bx.psu.edu
Link to section 'Versions' of 'kmergenie' Versions
- 1.7051
Link to section 'Commands' of 'kmergenie' Commands
- kmergenie
Link to section 'Module' of 'kmergenie' Module
You can load the modules by:
module load biocontainers
module load kmergenie
Link to section 'Example job' of 'kmergenie' Example job
Using #!/bin/sh -l
as shebang in the slurm job script will cause the failure of some biocontainer modules. Please use #!/bin/bash
instead.
To run kmergenie on our clusters:
#!/bin/bash
#SBATCH -A myallocation # Allocation name
#SBATCH -t 1:00:00
#SBATCH -N 1
#SBATCH -n 1
#SBATCH --job-name=kmergenie
#SBATCH --mail-type=FAIL,BEGIN,END
#SBATCH --error=%x-%J-%u.err
#SBATCH --output=%x-%J-%u.out
module --force purge
ml biocontainers kmergenie
kneaddata
KneadData
is a tool designed to perform quality control on metagenomic and metatranscriptomic sequencing data, especially data from microbiome experiments. In these experiments, samples are typically taken from a host in hopes of learning something about the microbial community on the host.
Detailed usage can be found here: https://huttenhower.sph.harvard.edu/kneaddata/
Link to section 'Versions' of 'kneaddata' Versions
- 0.10.0
Link to section 'Commands' of 'kneaddata' Commands
- kneaddata
- kneaddata_bowtie2_discordant_pairs
- kneaddata_build_database
- kneaddata_database
- kneaddata_read_count_table
- kneaddata_test
- kneaddata_trf_parallel
Link to section 'Module' of 'kneaddata' Module
You can load the modules by:
module load biocontainers
module load kneaddata
Link to section 'Example job' of 'kneaddata' Example job
Using #!/bin/sh -l
as shebang in the slurm job script will cause the failure of some biocontainer modules. Please use #!/bin/bash
instead.
To run kneaddata on our our clusters:
#!/bin/bash
#SBATCH -A myallocation # Allocation name
#SBATCH -t 20:00:00
#SBATCH -N 1
#SBATCH -n 24
#SBATCH --job-name=kneaddata
#SBATCH --mail-type=FAIL,BEGIN,END
#SBATCH --error=%x-%J-%u.err
#SBATCH --output=%x-%J-%u.out
module --force purge
ml biocontainers kneaddata
kneaddata --input examples/demo.fastq --reference-db examples/demo_db --output kneaddata_demo_outpu --threads 24 --processes 24
kover
Link to section 'Introduction' of 'kover' Introduction
Kover is an out-of-core implementation of rule-based machine learning algorithms that has been tailored for genomic biomarker discovery.
Docker hub: https://hub.docker.com/r/aldro61/kover
Home page: https://github.com/aldro61/kover
Link to section 'Versions' of 'kover' Versions
- 2.0.6
Link to section 'Commands' of 'kover' Commands
- kover
Link to section 'Module' of 'kover' Module
You can load the modules by:
module load biocontainers
module load kover
Link to section 'Example job' of 'kover' Example job
Using #!/bin/sh -l
as shebang in the slurm job script will cause the failure of some biocontainer modules. Please use #!/bin/bash
instead.
To run kover on our clusters:
#!/bin/bash
#SBATCH -A myallocation # Allocation name
#SBATCH -t 1:00:00
#SBATCH -N 1
#SBATCH -n 1
#SBATCH --job-name=kover
#SBATCH --mail-type=FAIL,BEGIN,END
#SBATCH --error=%x-%J-%u.err
#SBATCH --output=%x-%J-%u.out
module --force purge
ml biocontainers kover
kraken2
Kraken2
is the newest version of Kraken, a taxonomic classification system using exact k-mer matches to achieve high accuracy and fast classification speeds. This classifier matches each k-mer within a query sequence to the lowest common ancestor (LCA) of all genomes containing the given k-mer.
Detailed usage can be found here: https://ccb.jhu.edu/software/kraken2/
Link to section 'Versions' of 'kraken2' Versions
- 2.1.2_fixftp
- 2.1.2
Link to section 'Commands' of 'kraken2' Commands
- kraken2
- kraken2-build
- kraken2-inspect
Link to section 'Module' of 'kraken2' Module
You can load the modules by:
module load biocontainers
module load kraken2/2.1.2
Download database
There is a known bug in rsync_from_ncbi.pl
(https://github.com/DerrickWood/kraken2/issues/292). When users want to download and build databases by kraken2-build --download-library
, there will an error rsync_from_ncbi.pl: unexpected FTP path(new server?)
. We modified rsync_from_ncbi.pl
to fix the bug, and created a new module ending with the suffix _fixftp
. Please use this corrected module to download the library.
To download databases, please use the below command:
module load biocontainers
module load kraken2/2.1.2_fixftp
kraken2-build --download-library archaea --db archaea
Link to section 'Example job' of 'kraken2' Example job
Using #!/bin/sh -l
as shebang in the slurm job script will cause the failure of some biocontainer modules. Please use #!/bin/bash
instead.
To run kraken2 on our our clusters:
#!/bin/bash
#SBATCH -A myallocation # Allocation name
#SBATCH -t 20:00:00
#SBATCH -N 1
#SBATCH -n 24
#SBATCH --job-name=kraken2
#SBATCH --mail-type=FAIL,BEGIN,END
#SBATCH --error=%x-%J-%u.err
#SBATCH --output=%x-%J-%u.out
module --force purge
ml biocontainers kraken2/2.1.2
kraken2 --threads 24 --report kranken2.report --db minikraken2_v2_8GB_201904_UPDATE --paired --classified-out cseqs#.fq SRR5043021_1.fastq SRR5043021_2.fastq
krakentools
KrakenTools
provides individual scripts to analyze Kraken/Kraken2/Bracken/KrakenUniq output files.
Detailed usage can be found here: https://github.com/jenniferlu717/KrakenTools
Link to section 'Versions' of 'krakentools' Versions
- 1.2
Link to section 'Commands' of 'krakentools' Commands
- alpha_diversity.py
- beta_diversity.py
- combine_kreports.py
- combine_mpa.py
- extract_kraken_reads.py
- filter_bracken.out.py
- fix_unmapped.py
- kreport2krona.py
- kreport2mpa.py
- make_kreport.py
- make_ktaxonomy.py
Link to section 'Module' of 'krakentools' Module
You can load the modules by:
module load biocontainers
module load krakentools/1.2
Link to section 'Example job' of 'krakentools' Example job
Using #!/bin/sh -l
as shebang in the slurm job script will cause the failure of some biocontainer modules. Please use #!/bin/bash
instead.
To run krakentools on our our clusters:
#!/bin/bash
#SBATCH -A myallocation # Allocation name
#SBATCH -t 1:00:00
#SBATCH -N 1
#SBATCH -n 8
#SBATCH --job-name=krakentools
#SBATCH --mail-type=FAIL,BEGIN,END
#SBATCH --error=%x-%J-%u.err
#SBATCH --output=%x-%J-%u.out
module --force purge
ml biocontainers krakentools/1.2
extract_kraken_reads.py -k myfile.kraken -t 2 -s1 SRR5043021_1.fastq -s2 SRR5043021_2.fastq -o extracted1.fq -o2 extracted2.fq
lambda
Link to section 'Introduction' of 'lambda' Introduction
Lambda
is a local aligner optimized for many query sequences and searches in protein space.
For more information, please check its website: https://biocontainers.pro/tools/lambda and its home page: http://seqan.github.io/lambda/.
Link to section 'Versions' of 'lambda' Versions
- 2.0.0
Link to section 'Commands' of 'lambda' Commands
- lambda2
Link to section 'Module' of 'lambda' Module
You can load the modules by:
module load biocontainers
module load lambda
Link to section 'Example job' of 'lambda' Example job
Using #!/bin/sh -l
as shebang in the slurm job script will cause the failure of some biocontainer modules. Please use #!/bin/bash
instead.
To run Lambda on our clusters:
#!/bin/bash
#SBATCH -A myallocation # Allocation name
#SBATCH -t 1:00:00
#SBATCH -N 1
#SBATCH -n 1
#SBATCH --job-name=lambda
#SBATCH --mail-type=FAIL,BEGIN,END
#SBATCH --error=%x-%J-%u.err
#SBATCH --output=%x-%J-%u.out
module --force purge
ml biocontainers lambda
lambda2 mkindexp -d uniprot_sprot.fasta
lambda2 searchp \
-q proteins.fasta \
-i uniprot_sprot.fasta.lambda
last
Link to section 'Introduction' of 'last' Introduction
Last
is used to find & align related regions of sequences.
For more information, please check its website: https://biocontainers.pro/tools/last and its home page on Gitlab.
Link to section 'Versions' of 'last' Versions
- 1268
- 1356
- 1411
- 1418
Link to section 'Commands' of 'last' Commands
- last-dotplot
- last-map-probs
- last-merge-batches
- last-pair-probs
- last-postmask
- last-split
- last-split5
- last-train
- lastal
- lastal5
- lastdb
- lastdb5
Link to section 'Module' of 'last' Module
You can load the modules by:
module load biocontainers
module load last
Link to section 'Example job' of 'last' Example job
Using #!/bin/sh -l
as shebang in the slurm job script will cause the failure of some biocontainer modules. Please use #!/bin/bash
instead.
To run Last on our clusters:
#!/bin/bash
#SBATCH -A myallocation # Allocation name
#SBATCH -t 1:00:00
#SBATCH -N 1
#SBATCH -n 1
#SBATCH --job-name=last
#SBATCH --mail-type=FAIL,BEGIN,END
#SBATCH --error=%x-%J-%u.err
#SBATCH --output=%x-%J-%u.out
module --force purge
ml biocontainers last
lastdb humdb humanMito.fa
lastal humdb fuguMito.fa > myalns.maf
lastz
Link to section 'Introduction' of 'lastz' Introduction
LASTZ - pairwise DNA sequence aligner
BioContainers: https://biocontainers.pro/tools/lastz
Home page: https://github.com/lastz/lastz
Link to section 'Versions' of 'lastz' Versions
- 1.04.15
Link to section 'Commands' of 'lastz' Commands
- lastz
- lastz_32
- lastz_D
Link to section 'Module' of 'lastz' Module
You can load the modules by:
module load biocontainers
module load lastz
Link to section 'Example job' of 'lastz' Example job
Using #!/bin/sh -l
as shebang in the slurm job script will cause the failure of some biocontainer modules. Please use #!/bin/bash
instead.
To run lastz on our clusters:
#!/bin/bash
#SBATCH -A myallocation # Allocation name
#SBATCH -t 1:00:00
#SBATCH -N 1
#SBATCH -n 1
#SBATCH --job-name=lastz
#SBATCH --mail-type=FAIL,BEGIN,END
#SBATCH --error=%x-%J-%u.err
#SBATCH --output=%x-%J-%u.out
module --force purge
ml biocontainers lastz
lastz cmc_CFBP8216.fasta cmp_LPPA982.fasta \
--notransition --step=20 --nogapped \
--format=maf > cmc_vs_cmp.maf
ldhat
Link to section 'Introduction' of 'ldhat' Introduction
LDhat is a package written in the C and C++ languages for the analysis of recombination rates from population genetic data.
Home page: https://github.com/auton1/LDhat
Link to section 'Versions' of 'ldhat' Versions
- 2.2a
Link to section 'Commands' of 'ldhat' Commands
- convert
- pairwise
- interval
- rhomap
- fin
Link to section 'Module' of 'ldhat' Module
You can load the modules by:
module load biocontainers
module load ldhat
Link to section 'Example job' of 'ldhat' Example job
Using #!/bin/sh -l
as shebang in the slurm job script will cause the failure of some biocontainer modules. Please use #!/bin/bash
instead.
To run ldhat on our clusters:
#!/bin/bash
#SBATCH -A myallocation # Allocation name
#SBATCH -t 1:00:00
#SBATCH -N 1
#SBATCH -n 1
#SBATCH --job-name=ldhat
#SBATCH --mail-type=FAIL,BEGIN,END
#SBATCH --error=%x-%J-%u.err
#SBATCH --output=%x-%J-%u.out
module --force purge
ml biocontainers ldhat
ldjump
Link to section 'Introduction' of 'ldjump' Introduction
LDJump is an R package to estimate variable recombination rates from population genetic data.
Home page: https://github.com/PhHermann/LDJump
Link to section 'Versions' of 'ldjump' Versions
- 0.3.1
Link to section 'Commands' of 'ldjump' Commands
- R
- Rscript
Link to section 'Module' of 'ldjump' Module
You can load the modules by:
module load biocontainers
module load ldjump
A full path to the Phi file of PhiPack needs to be provided as follows pathPhi = "/opt/PhiPack/Phi"
. In order to use LDhat to quickly calculate some of the summary statistics, please set pathLDhat = "/opt/LDhat/"
.
Link to section 'Interactive job' of 'ldjump' Interactive job
To run interactively on our clusters:
(base) UserID@bell-fe00:~ $ sinteractive -N1 -n12 -t4:00:00 -A myallocation
salloc: Granted job allocation 12345869
salloc: Waiting for resource configuration
salloc: Nodes bell-a008 are ready for job
(base) UserID@bell-a008:~ $ module load biocontainers ldjump
(base) UserID@bell-a008:~ $ R
R version 4.2.1 (2022-06-23) -- "Funny-Looking Kid"
Copyright (C) 2022 The R Foundation for Statistical Computing
Platform: x86_64-pc-linux-gnu (64-bit)
R is free software and comes with ABSOLUTELY NO WARRANTY.
You are welcome to redistribute it under certain conditions.
Type 'license()' or 'licence()' for distribution details.
Natural language support but running in an English locale
R is a collaborative project with many contributors.
Type 'contributors()' for more information and
'citation()' on how to cite R or R packages in publications.
Type 'demo()' for some demos, 'help()' for on-line help, or
'help.start()' for an HTML browser interface to help.
Type 'q()' to quit R.
> library(LDJump)
> LDJump(seqFullPath, alpha = 0.05, segLength = 1000, pathLDhat = "/opt/LDhat/", pathPhi = "/opt/PhiPack/Phi", format = "fasta", refName = NULL,
start = NULL, constant = F, status = T, cores = 1, accept = F, demography = F, out = "")
Link to section 'Batch job' of 'ldjump' Batch job
Using #!/bin/sh -l
as shebang in the slurm job script will cause the failure of some biocontainer modules. Please use #!/bin/bash
instead.
To run ldjump on our clusters:
#!/bin/bash
#SBATCH -A myallocation # Allocation name
#SBATCH -t 1:00:00
#SBATCH -N 1
#SBATCH -n 1
#SBATCH --job-name=ldjump
#SBATCH --mail-type=FAIL,BEGIN,END
#SBATCH --error=%x-%J-%u.err
#SBATCH --output=%x-%J-%u.out
module --force purge
ml biocontainers ldjump
Rscript script.R
ldsc
Link to section 'Introduction' of 'ldsc' Introduction
ldsc is a command line tool for estimating heritability and genetic correlation from GWAS summary statistics.
BioContainers: https://biocontainers.pro/tools/ldsc
Home page: https://github.com/bulik/ldsc
Link to section 'Versions' of 'ldsc' Versions
- 1.0.1
Link to section 'Commands' of 'ldsc' Commands
- ldsc.py
- munge_sumstats.py
Link to section 'Module' of 'ldsc' Module
You can load the modules by:
module load biocontainers
module load ldsc
Link to section 'Example job' of 'ldsc' Example job
Using #!/bin/sh -l
as shebang in the slurm job script will cause the failure of some biocontainer modules. Please use #!/bin/bash
instead.
To run ldsc on our clusters:
#!/bin/bash
#SBATCH -A myallocation # Allocation name
#SBATCH -t 1:00:00
#SBATCH -N 1
#SBATCH -n 1
#SBATCH --job-name=ldsc
#SBATCH --mail-type=FAIL,BEGIN,END
#SBATCH --error=%x-%J-%u.err
#SBATCH --output=%x-%J-%u.out
module --force purge
ml biocontainers ldsc
liftoff
Link to section 'Introduction' of 'liftoff' Introduction
Liftoff
is an accurate GFF3/GTF lift over pipeline.
For more information, please check its website: https://biocontainers.pro/tools/liftoff and its home page on Github.
Link to section 'Versions' of 'liftoff' Versions
- 1.6.3
Link to section 'Commands' of 'liftoff' Commands
- liftoff
- python
- python3
Link to section 'Module' of 'liftoff' Module
You can load the modules by:
module load biocontainers
module load liftoff
Link to section 'Example job' of 'liftoff' Example job
Using #!/bin/sh -l
as shebang in the slurm job script will cause the failure of some biocontainer modules. Please use #!/bin/bash
instead.
To run Liftoff on our clusters:
#!/bin/bash
#SBATCH -A myallocation # Allocation name
#SBATCH -t 1:00:00
#SBATCH -N 1
#SBATCH -n 1
#SBATCH --job-name=liftoff
#SBATCH --mail-type=FAIL,BEGIN,END
#SBATCH --error=%x-%J-%u.err
#SBATCH --output=%x-%J-%u.out
module --force purge
ml biocontainers liftoff
liftoff -g reference.gff3 -o target.gff3 \
-chroms chr_pairs.txt target.fasta reference.fa
liftofftools
Link to section 'Introduction' of 'liftofftools' Introduction
LiftoffTools is a toolkit to compare genes lifted between genome assemblies. Specifically it is designed to compare genes lifted over using Liftoff although it is also compatible with other lift-over tools such as UCSC liftOver as long as the feature IDs are the same. LiftoffTools provides 3 different modules. The first identifies variants in protein-coding genes and their effects on the gene. The second compares the gene synteny, and the third clusters genes into groups of paralogs to evaluate gene copy number gain and loss. The input for all modules is the reference genome assembly (FASTA), target genome assembly (FASTA), reference annotation (GFF/GTF), and target annotation (GFF/GTF).
BioContainers: https://biocontainers.pro/tools/liftofftools
Home page: https://github.com/agshumate/LiftoffTools
Link to section 'Versions' of 'liftofftools' Versions
- 0.4.4
Link to section 'Commands' of 'liftofftools' Commands
- liftofftools
Link to section 'Module' of 'liftofftools' Module
You can load the modules by:
module load biocontainers
module load liftofftools
Link to section 'Example job' of 'liftofftools' Example job
Using #!/bin/sh -l
as shebang in the slurm job script will cause the failure of some biocontainer modules. Please use #!/bin/bash
instead.
To run liftofftools on our clusters:
#!/bin/bash
#SBATCH -A myallocation # Allocation name
#SBATCH -t 1:00:00
#SBATCH -N 1
#SBATCH -n 1
#SBATCH --job-name=liftofftools
#SBATCH --mail-type=FAIL,BEGIN,END
#SBATCH --error=%x-%J-%u.err
#SBATCH --output=%x-%J-%u.out
module --force purge
ml biocontainers liftofftools
lima
Link to section 'Introduction' of 'lima' Introduction
Lima
is the standard tool to identify barcode and primer sequences in PacBio single-molecule sequencing data.
For more information, please check its website: https://biocontainers.pro/tools/lima and its home page: https://lima.how.
Link to section 'Versions' of 'lima' Versions
- 2.2.0
Link to section 'Commands' of 'lima' Commands
- lima
Link to section 'Module' of 'lima' Module
You can load the modules by:
module load biocontainers
module load lima
Link to section 'Example job' of 'lima' Example job
Using #!/bin/sh -l
as shebang in the slurm job script will cause the failure of some biocontainer modules. Please use #!/bin/bash
instead.
To run Lima on our clusters:
#!/bin/bash
#SBATCH -A myallocation # Allocation name
#SBATCH -t 1:00:00
#SBATCH -N 1
#SBATCH -n 12
#SBATCH --job-name=lima
#SBATCH --mail-type=FAIL,BEGIN,END
#SBATCH --error=%x-%J-%u.err
#SBATCH --output=%x-%J-%u.out
module --force purge
ml biocontainers lima
lima --version
lima --isoseq --dump-clips \
--peek-guess -j 12 \
alz.ccs.bam primers.fasta \
alz.demult.bam
links
Link to section 'Introduction' of 'links' Introduction
LINKS is a genomics application for scaffolding genome assemblies with long reads, such as those produced by Oxford Nanopore Technologies Ltd. It can be used to scaffold high-quality draft genome assemblies with any long sequences (eg. ONT reads, PacBio reads, other draft genomes, etc). It is also used to scaffold contig pairs linked by ARCS/ARKS.
BioContainers: https://biocontainers.pro/tools/links
Home page: https://github.com/bcgsc/LINKS
Link to section 'Versions' of 'links' Versions
- 2.0.1
Link to section 'Commands' of 'links' Commands
- LINKS
Link to section 'Module' of 'links' Module
You can load the modules by:
module load biocontainers
module load links
Link to section 'Example job' of 'links' Example job
Using #!/bin/sh -l
as shebang in the slurm job script will cause the failure of some biocontainer modules. Please use #!/bin/bash
instead.
To run links on our clusters:
#!/bin/bash
#SBATCH -A myallocation # Allocation name
#SBATCH -t 1:00:00
#SBATCH -N 1
#SBATCH -n 1
#SBATCH --job-name=links
#SBATCH --mail-type=FAIL,BEGIN,END
#SBATCH --error=%x-%J-%u.err
#SBATCH --output=%x-%J-%u.out
module --force purge
ml biocontainers links
lofreq
Link to section 'Introduction' of 'lofreq' Introduction
Lofreq
is a fast and sensitive variant-caller for inferring SNVs and indels from next-generation sequencing data.
For more information, please check its website: https://biocontainers.pro/tools/lofreq and its home page on Github.
Link to section 'Versions' of 'lofreq' Versions
- 2.1.5
Link to section 'Commands' of 'lofreq' Commands
- lofreq
Link to section 'Module' of 'lofreq' Module
You can load the modules by:
module load biocontainers
module load lofreq
Link to section 'Example job' of 'lofreq' Example job
Using #!/bin/sh -l
as shebang in the slurm job script will cause the failure of some biocontainer modules. Please use #!/bin/bash
instead.
To run Lofreq on our clusters:
#!/bin/bash
#SBATCH -A myallocation # Allocation name
#SBATCH -t 1:00:00
#SBATCH -N 1
#SBATCH -n 8
#SBATCH --job-name=lofreq
#SBATCH --mail-type=FAIL,BEGIN,END
#SBATCH --error=%x-%J-%u.err
#SBATCH --output=%x-%J-%u.out
module --force purge
ml biocontainers lofreq
lofreq call -f ref.fa -o vars.vcf out_sorted.bam
lofreq call-parallel --pp-threads 8 \
-f ref.fa -o vars_pallel.vcf out_sorted.bam
longphase
Link to section 'Introduction' of 'longphase' Introduction
LongPhase is an ultra-fast program for simultaneously co-phasing SNPs and SVs by using Nanopore and PacBio long reads. It is capable of producing nearly chromosome-scale haplotype blocks by using Nanpore ultra-long reads without the need for additional trios, chromosome conformation, and strand-seq data. On an 8-core machine, LongPhase can finish phasing a human genome in 10-20 minutes.
Docker hub: https://hub.docker.com/r/alexanrna/longphase
Home page: https://github.com/twolinin/longphase
Link to section 'Versions' of 'longphase' Versions
- 1.4
Link to section 'Commands' of 'longphase' Commands
- longphase
Link to section 'Module' of 'longphase' Module
You can load the modules by:
module load biocontainers
module load longphase
Link to section 'Example job' of 'longphase' Example job
Using #!/bin/sh -l
as shebang in the slurm job script will cause the failure of some biocontainer modules. Please use #!/bin/bash
instead.
To run longphase on our clusters:
#!/bin/bash
#SBATCH -A myallocation # Allocation name
#SBATCH -t 1:00:00
#SBATCH -N 1
#SBATCH -n 8
#SBATCH --job-name=longphase
#SBATCH --mail-type=FAIL,BEGIN,END
#SBATCH --error=%x-%J-%u.err
#SBATCH --output=%x-%J-%u.out
module --force purge
ml biocontainers longphase
longphase phase \
-s SNP.vcf \
--sv-file SV.vcf \
-b alignment.bam \
-r reference.fasta \
-t 8 \
-o phased_prefix \
--ont # or --pb for PacBio Hifi
longqc
Link to section 'Introduction' of 'longqc' Introduction
LongQC is a tool for the data quality control of the PacBio and ONT long reads.
Docker hub: https://hub.docker.com/r/cymbopogon/longqc
Home page: https://github.com/yfukasawa/LongQC
Link to section 'Versions' of 'longqc' Versions
- 1.2.0c
Link to section 'Commands' of 'longqc' Commands
- longQC.py
Link to section 'Module' of 'longqc' Module
You can load the modules by:
module load biocontainers
module load longqc
Link to section 'Example job' of 'longqc' Example job
Using #!/bin/sh -l
as shebang in the slurm job script will cause the failure of some biocontainer modules. Please use #!/bin/bash
instead.
To run longqc on our clusters:
#!/bin/bash
#SBATCH -A myallocation # Allocation name
#SBATCH -t 1:00:00
#SBATCH -N 1
#SBATCH -n 1
#SBATCH --job-name=longqc
#SBATCH --mail-type=FAIL,BEGIN,END
#SBATCH --error=%x-%J-%u.err
#SBATCH --output=%x-%J-%u.out
module --force purge
ml biocontainers longqc
longQC.py sampleqc -x pb-rs2 -o out_dir seq.fastq
lra
Link to section 'Introduction' of 'lra' Introduction
Lra
is a sequence alignment program that aligns long reads from single-molecule sequencing (SMS) instruments, or megabase-scale contigs from SMS assemblies.
For more information, please check its website: https://biocontainers.pro/tools/lra and its home page on Github.
Link to section 'Versions' of 'lra' Versions
- 1.3.2
Link to section 'Commands' of 'lra' Commands
- lra
Link to section 'Module' of 'lra' Module
You can load the modules by:
module load biocontainers
module load lra
Link to section 'Example job' of 'lra' Example job
Using #!/bin/sh -l
as shebang in the slurm job script will cause the failure of some biocontainer modules. Please use #!/bin/bash
instead.
To run Lra on our clusters:
#!/bin/bash
#SBATCH -A myallocation # Allocation name
#SBATCH -t 1:00:00
#SBATCH -N 1
#SBATCH -n 12
#SBATCH --job-name=lra
#SBATCH --mail-type=FAIL,BEGIN,END
#SBATCH --error=%x-%J-%u.err
#SBATCH --output=%x-%J-%u.out
module --force purge
ml biocontainers lra
lra index genome.fasta
lra align genome.fasta input.fastq -t 12 -p s > output.sam
ltr_finder
Link to section 'Introduction' of 'ltr_finder' Introduction
LTR_Finder is an efficient program for finding full-length LTR retrotranspsons in genome sequences.
Home page: https://github.com/xzhub/LTR_Finder
Link to section 'Versions' of 'ltr_finder' Versions
- 1.07
Link to section 'Commands' of 'ltr_finder' Commands
- ltr_finder
- check_result.pl
- down_tRNA.pl
- filter_rt.pl
- genome_plot.pl
- genome_plot2.pl
- genome_plot_svg.pl
Link to section 'Module' of 'ltr_finder' Module
You can load the modules by:
module load biocontainers
module load ltr_finder
Link to section 'Example job' of 'ltr_finder' Example job
Using #!/bin/sh -l
as shebang in the slurm job script will cause the failure of some biocontainer modules. Please use #!/bin/bash
instead.
To run ltr_finder on our clusters:
#!/bin/bash
#SBATCH -A myallocation # Allocation name
#SBATCH -t 1:00:00
#SBATCH -N 1
#SBATCH -n 1
#SBATCH --job-name=ltr_finder
#SBATCH --mail-type=FAIL,BEGIN,END
#SBATCH --error=%x-%J-%u.err
#SBATCH --output=%x-%J-%u.out
module --force purge
ml biocontainers ltr_finder
ltr_finder 3ds_72.fa -P 3ds_72 -w2 > test/3ds_72_result.txt \
| genome_plot.pl test/
ltrpred
Link to section 'Introduction' of 'ltrpred' Introduction
LTRpred(ict): de novo annotation of young and intact retrotransposons.
Docker hub: https://hub.docker.com/r/drostlab/ltrpred
Home page: https://github.com/HajkD/LTRpred
Link to section 'Versions' of 'ltrpred' Versions
- 1.1.0
Link to section 'Commands' of 'ltrpred' Commands
- R
- Rscript
Link to section 'Module' of 'ltrpred' Module
You can load the modules by:
module load biocontainers
module load ltrpred
Link to section 'Example job' of 'ltrpred' Example job
Using #!/bin/sh -l
as shebang in the slurm job script will cause the failure of some biocontainer modules. Please use #!/bin/bash
instead.
To run ltrpred on our clusters:
#!/bin/bash
#SBATCH -A myallocation # Allocation name
#SBATCH -t 1:00:00
#SBATCH -N 1
#SBATCH -n 1
#SBATCH --job-name=ltrpred
#SBATCH --mail-type=FAIL,BEGIN,END
#SBATCH --error=%x-%J-%u.err
#SBATCH --output=%x-%J-%u.out
module --force purge
ml biocontainers ltrpred
lumpy-sv
Link to section 'Introduction' of 'lumpy-sv' Introduction
Lumpy-sv
is a general probabilistic framework for structural variant discovery.
For more information, please check its website: https://biocontainers.pro/tools/lumpy-sv and its home page on Github.
Link to section 'Versions' of 'lumpy-sv' Versions
- 0.3.1
Link to section 'Commands' of 'lumpy-sv' Commands
- lumpy
- lumpyexpress
Link to section 'Module' of 'lumpy-sv' Module
You can load the modules by:
module load biocontainers
module load lumpy-sv
Link to section 'Example job' of 'lumpy-sv' Example job
Using #!/bin/sh -l
as shebang in the slurm job script will cause the failure of some biocontainer modules. Please use #!/bin/bash
instead.
To run Lumpy-sv on our clusters:
#!/bin/bash
#SBATCH -A myallocation # Allocation name
#SBATCH -t 1:00:00
#SBATCH -N 1
#SBATCH -n 8
#SBATCH --job-name=lumpy-sv
#SBATCH --mail-type=FAIL,BEGIN,END
#SBATCH --error=%x-%J-%u.err
#SBATCH --output=%x-%J-%u.out
module --force purge
ml biocontainers lumpy-sv
lumpy -mw 4 -tt 0.0 -pe \
bam_file:AL87.discordant.sort.bam,histo_file:AL87.histo,mean:429,stdev:84,read_length:83,min_non_overlap:83,discordant_z:4,back_distance:1,weight:1,id:1,min_mapping_threshold:20 \
-sr bam_file:AL87.sr.sort.bam,back_distance:1,weight:1,id:2,min_mapping_threshold:20
lyveset
Link to section 'Introduction' of 'lyveset' Introduction
Lyveset is a method of using hqSNPs to create a phylogeny, especially for outbreak investigations.
Docker hub: https://hub.docker.com/r/staphb/lyveset
Home page: https://github.com/lskatz/lyve-SET
Link to section 'Versions' of 'lyveset' Versions
- 2.0.1
Link to section 'Commands' of 'lyveset' Commands
- applyFstToTree.pl
- cladeDistancesFromTree.pl
- clusterPairwise.pl
- convertAlignment.pl
- downloadDataset.pl
- errorProneRegions.pl
- filterMatrix.pl
- filterVcf.pl
- genomeDist.pl
- launch_bwa.pl
- launch_set.pl
- launch_smalt.pl
- launch_snap.pl
- launch_snpeff.pl
- launch_varscan.pl
- makeRegions.pl
- matrixToAlignment.pl
- pairwiseDistances.pl
- pairwiseTo2d.pl
- removeUninformativeSites.pl
- removeUninformativeSitesFromMatrix.pl
- run_assembly_isFastqPE.pl
- run_assembly_metrics.pl
- run_assembly_readMetrics.pl
- run_assembly_removeDuplicateReads.pl
- run_assembly_shuffleReads.pl
- run_assembly_trimClean.pl
- set_bayesHammer.pl
- set_diagnose.pl
- set_diagnose_msa.pl
- set_downloadTestData.pl
- set_findCliffs.pl
- set_findPhages.pl
- set_indexCase.pl
- set_manage.pl
- set_processPooledVcf.pl
- set_samtools_depth.pl
- set_test.pl
- shuffleSplitReads.pl
- snpDistribution.pl
- vcfToAlignment.pl
- vcfutils.pl
Link to section 'Module' of 'lyveset' Module
You can load the modules by:
module load biocontainers
module load lyveset
Link to section 'Example job' of 'lyveset' Example job
Using #!/bin/sh -l
as shebang in the slurm job script will cause the failure of some biocontainer modules. Please use #!/bin/bash
instead.
To run lyveset on our clusters:
#!/bin/bash
#SBATCH -A myallocation # Allocation name
#SBATCH -t 1:00:00
#SBATCH -N 1
#SBATCH -n 1
#SBATCH --job-name=lyveset
#SBATCH --mail-type=FAIL,BEGIN,END
#SBATCH --error=%x-%J-%u.err
#SBATCH --output=%x-%J-%u.out
module --force purge
ml biocontainers lyveset
set_test.pl lambda
set_manage.pl --create setTest
macrel
Link to section 'Introduction' of 'macrel' Introduction
Macrel is a pipeline to mine antimicrobial peptides (AMPs) from (meta)genomes.
BioContainers: https://biocontainers.pro/tools/macrel
Home page: https://github.com/BigDataBiology/macrel
Link to section 'Versions' of 'macrel' Versions
- 1.2.0
Link to section 'Commands' of 'macrel' Commands
- macrel
Link to section 'Module' of 'macrel' Module
You can load the modules by:
module load biocontainers
module load macrel
Link to section 'Example job' of 'macrel' Example job
Using #!/bin/sh -l
as shebang in the slurm job script will cause the failure of some biocontainer modules. Please use #!/bin/bash
instead.
To run macrel on our clusters:
#!/bin/bash
#SBATCH -A myallocation # Allocation name
#SBATCH -t 1:00:00
#SBATCH -N 1
#SBATCH -n 1
#SBATCH --job-name=macrel
#SBATCH --mail-type=FAIL,BEGIN,END
#SBATCH --error=%x-%J-%u.err
#SBATCH --output=%x-%J-%u.out
module --force purge
ml biocontainers macrel
macs2
Link to section 'Introduction' of 'macs2' Introduction
MACS2
is Model-based Analysis of ChIP-Seq for identifying transcript factor binding sites.
MACS consists of four steps:
- removing redundant reads
- adjusting read position
- calculating peak enrichment
- estimating the empirical false discovery rate (FDR).
For more information, please check its website: https://biocontainers.pro/tools/macs2 and its home page on Github.
Link to section 'Versions' of 'macs2' Versions
- 2.2.7.1
Link to section 'Commands' of 'macs2' Commands
- macs2
Link to section 'Module' of 'macs2' Module
You can load the modules by:
module load biocontainers
module load macs2
Link to section 'Example job' of 'macs2' Example job
Using #!/bin/sh -l
as shebang in the slurm job script will cause the failure of some biocontainer modules. Please use #!/bin/bash
instead.
To run MACS2 on our clusters:
#!/bin/bash
#SBATCH -A myallocation # Allocation name
#SBATCH -t 1:00:00
#SBATCH -N 1
#SBATCH -n 1
#SBATCH --job-name=macs2
#SBATCH --mail-type=FAIL,BEGIN,END
#SBATCH --error=%x-%J-%u.err
#SBATCH --output=%x-%J-%u.out
module --force purge
ml biocontainers macs2
macs2 callpeak -t ChIP.bam -c Control.bam -f BAM -g hs -n test -B -q 0.01
macs3
Link to section 'Introduction' of 'macs3' Introduction
MACS3
is Model-based Analysis of ChIP-Seq for identifying transcript factor.
Docker hub: https://hub.docker.com/r/lbmc/macs3/3.0.0a6 and its home page on Github.
Link to section 'Versions' of 'macs3' Versions
- 3.0.0a6
Link to section 'Commands' of 'macs3' Commands
- macs3
Link to section 'Module' of 'macs3' Module
You can load the modules by:
module load biocontainers
module load macs3
Link to section 'Example job' of 'macs3' Example job
Using #!/bin/sh -l
as shebang in the slurm job script will cause the failure of some biocontainer modules. Please use #!/bin/bash
instead.
To run Macs3 on our clusters:
#!/bin/bash
#SBATCH -A myallocation # Allocation name
#SBATCH -t 1:00:00
#SBATCH -N 1
#SBATCH -n 1
#SBATCH --job-name=macs3
#SBATCH --mail-type=FAIL,BEGIN,END
#SBATCH --error=%x-%J-%u.err
#SBATCH --output=%x-%J-%u.out
module --force purge
ml biocontainers macs3
macs3 callpeak -t ChIP.bam -c Control.bam -f BAM -g hs -n test -B -q 0.01
mafft
Link to section 'Introduction' of 'mafft' Introduction
MAFFT
is a multiple alignment program for amino acid or nucleotide sequences.
For more information, please check its website: https://biocontainers.pro/tools/mafft and its home page: https://mafft.cbrc.jp/alignment/software/.
Link to section 'Versions' of 'mafft' Versions
- 7.475
- 7.490
Link to section 'Commands' of 'mafft' Commands
- einsi
- fftns
- fftnsi
- ginsi
- linsi
- mafft
- mafft-distance
- mafft-einsi
- mafft-fftns
- mafft-fftnsi
- mafft-ginsi
- mafft-homologs.rb
- mafft-linsi
- mafft-nwns
- mafft-nwnsi
- mafft-profile
- mafft-qinsi
- mafft-sparsecore.rb
- mafft-xinsi
- nwns
- nwnsi
Link to section 'Module' of 'mafft' Module
You can load the modules by:
module load biocontainers
module load mafft
Link to section 'Example job' of 'mafft' Example job
Using #!/bin/sh -l
as shebang in the slurm job script will cause the failure of some biocontainer modules. Please use #!/bin/bash
instead.
To run MAFFT on our clusters:
#!/bin/bash
#SBATCH -A myallocation # Allocation name
#SBATCH -t 1:00:00
#SBATCH -N 1
#SBATCH -n 1
#SBATCH --job-name=mafft
#SBATCH --mail-type=FAIL,BEGIN,END
#SBATCH --error=%x-%J-%u.err
#SBATCH --output=%x-%J-%u.out
module --force purge
ml biocontainers mafft
mageck
Link to section 'Introduction' of 'mageck' Introduction
Model-based Analysis of Genome-wide CRISPR-Cas9 Knockout (MAGeCK) is a computational tool to identify important genes from the recent genome-scale CRISPR-Cas9 knockout screens (or GeCKO) technology.
Docker hub: https://hub.docker.com/r/davidliwei/mageck
Home page: https://bitbucket.org/liulab/mageck/src/master/
Link to section 'Versions' of 'mageck' Versions
- 0.5.9.5
Link to section 'Commands' of 'mageck' Commands
- mageck
- mageckGSEA
- RRA
Link to section 'Module' of 'mageck' Module
You can load the modules by:
module load biocontainers
module load mageck
Link to section 'Example job' of 'mageck' Example job
Using #!/bin/sh -l
as shebang in the slurm job script will cause the failure of some biocontainer modules. Please use #!/bin/bash
instead.
To run mageck on our clusters:
#!/bin/bash
#SBATCH -A myallocation # Allocation name
#SBATCH -t 1:00:00
#SBATCH -N 1
#SBATCH -n 1
#SBATCH --job-name=mageck
#SBATCH --mail-type=FAIL,BEGIN,END
#SBATCH --error=%x-%J-%u.err
#SBATCH --output=%x-%J-%u.out
module --force purge
ml biocontainers mageck
mageck count -l library.txt -n demo \
--sample-label L1,CTRL \
--fastq test1.fastq test2.fastq
mageck test -k demo.count.txt \
-t L1 -c CTRL -n demo
magicblast
Link to section 'Introduction' of 'magicblast' Introduction
Magic-BLAST is a tool for mapping large next-generation RNA or DNA sequencing runs against a whole genome or transcriptome. Each alignment optimizes a composite score, taking into account simultaneously the two reads of a pair, and in case of RNA-seq, locating the candidate introns and adding up the score of all exons. This is very different from other versions of BLAST, where each exon is scored as a separate hit and read-pairing is ignored.
Docker hub: https://hub.docker.com/r/ncbi/magicblast
Home page: https://ncbi.github.io/magicblast/
Link to section 'Versions' of 'magicblast' Versions
- 1.5.0
Link to section 'Commands' of 'magicblast' Commands
- magicblast
Link to section 'Module' of 'magicblast' Module
You can load the modules by:
module load biocontainers
module load magicblast
Link to section 'Example job' of 'magicblast' Example job
Using #!/bin/sh -l
as shebang in the slurm job script will cause the failure of some biocontainer modules. Please use #!/bin/bash
instead.
To run magicblast on our clusters:
#!/bin/bash
#SBATCH -A myallocation # Allocation name
#SBATCH -t 1:00:00
#SBATCH -N 1
#SBATCH -n 1
#SBATCH --job-name=magicblast
#SBATCH --mail-type=FAIL,BEGIN,END
#SBATCH --error=%x-%J-%u.err
#SBATCH --output=%x-%J-%u.out
module --force purge
ml biocontainers magicblast
maker
Link to section 'Introduction' of 'maker' Introduction
MAKER
is a popular genome annotation pipeline for both prokaryotic and eukaryotic genomes. This guide describes best practices for running MAKER on RCAC clusters. For detailed information about MAKER, see its official website (http://weatherby.genetics.utah.edu/MAKER/wiki/index.php/MAKER_Tutorial_for_WGS_Assembly_and_Annotation_Winter_School_2018).
Link to section 'Versions' of 'maker' Versions
- 2.31.11
- 3.01.03
Link to section 'Commands' of 'maker' Commands
- cegma2zff
- chado2gff3
- compare
- cufflinks2gff3
- evaluator
- fasta_merge
- fasta_tool
- genemark_gtf2gff3
- gff3_merge
- iprscan2gff3
- iprscan_wrap
- ipr_update_gff
- maker
- maker2chado
- maker2eval_gtf
- maker2jbrowse
- maker2wap
- maker2zff
- maker_functional
- maker_functional_fasta
- maker_functional_gff
- maker_map_ids
- map2assembly
- map_data_ids
- map_fasta_ids
- map_gff_ids
- tophat2gff3
Link to section 'Module' of 'maker' Module
You can load the modules by:
module load biocontainers
module load maker/2.31.11 # OR maker/3.01.03
Dfam release 3.5
(October 2021) downloaded from Dfam website (https://www.dfam.org/home) that required by RepeatMasker
has been set up for users. The RepeatMakser
library is stored here /depot/itap/datasets/Maker/RepeatMasker/Libraries
.
Link to section 'Prerequisites' of 'maker' Prerequisites
- After loading MAKER modules, users can create MAKER control files by the following comand: maker -CTL This will generate three files:
- maker_opts.ctl (required to be modified)
- maker_exe.ctl (do not need to modify this file)
- maker_bopts.ctl (optionally modify this file)
- maker_opts.ctl:
- If not using RepeatMasker, modify
model_org=all
tomodel_org=
- If not using RepeatMasker, modify
model_org=all
to an appropriate family/genus/species.
Link to section 'Example job non-mpi' of 'maker' Example job non-mpi
Using #!/bin/sh -l
as shebang in the slurm job script will cause the failure of some biocontainer modules. Please use #!/bin/bash
instead.
To run MAKER on our cluster:
#!/bin/bash
#SBATCH -A myallocation # Allocation name
#SBATCH -t 10:00:00
#SBATCH -N 1
#SBATCH -n 24
#SBATCH --job-name=MAKER
#SBATCH --mail-type=FAIL,BEGIN,END
#SBATCH --error=%x-%J-%u.err
#SBATCH --output=%x-%J-%u.out
module --force purge
ml biocontainers maker/2.31.11 # or maker/3.01.03
maker -c 24
Link to section 'Example job mpi' of 'maker' Example job mpi
To use MAKER in MPI mode, we cannot use the maker modules. Instead we have to use the singularity image files stored in /apps/biocontainers/images
:
#!/bin/bash
#SBATCH -A myallocation # Allocation name
#SBATCH -t 5:00:00
#SBATCH -N 2
#SBATCH -n 24
#SBATCH -c 8
#SBATCH --job-name=MAKER_mpi
#SBATCH --mail-type=FAIL,BEGIN,END
#SBATCH --mail-user=UserID@purdue.edu
#SBATCH --error=%x-%J-%u.err
#SBATCH --output=%x-%J-%u.out
## MAKER2
mpirun -n 24 singularity exec /apps/biocontainers/images/maker_2.31.11.sif maker -c 8
## MAKER3
mpirun -n 24 singularity exec /apps/biocontainers/images/maker_3.01.03.sif maker -c 8
manta
Link to section 'Introduction' of 'manta' Introduction
Manta calls structural variants (SVs) and indels from mapped paired-end sequencing reads.
BioContainers: https://biocontainers.pro/tools/manta
Home page: https://github.com/Illumina/manta
Link to section 'Versions' of 'manta' Versions
- 1.6.0
Link to section 'Commands' of 'manta' Commands
- configManta.py
- python
Link to section 'Module' of 'manta' Module
You can load the modules by:
module load biocontainers
module load manta
Link to section 'Example job' of 'manta' Example job
Using #!/bin/sh -l
as shebang in the slurm job script will cause the failure of some biocontainer modules. Please use #!/bin/bash
instead.
To run manta on our clusters:
#!/bin/bash
#SBATCH -A myallocation # Allocation name
#SBATCH -t 1:00:00
#SBATCH -N 1
#SBATCH -n 1
#SBATCH --job-name=manta
#SBATCH --mail-type=FAIL,BEGIN,END
#SBATCH --error=%x-%J-%u.err
#SBATCH --output=%x-%J-%u.out
module --force purge
ml biocontainers manta
configManta.py --normalBam=HCC1954.NORMAL.30x.compare.COST16011_region.bam \
--tumorBam=G15512.HCC1954.1.COST16011_region.bam \
--referenceFasta=Homo_sapiens_assembly19.COST16011_region.fa \
--region=8:107652000-107655000 \
--region=11:94974000-94989000 \
--exome --runDir="MantaDemoAnalysis"
python MantaDemoAnalysis/runWorkflow.py
mapcaller
Link to section 'Introduction' of 'mapcaller' Introduction
Mapcaller
is an efficient and versatile approach for short-read mapping and variant identification using high-throughput sequenced data.
For more information, please check its website: https://biocontainers.pro/tools/mapcaller and its home page on Github.
Link to section 'Versions' of 'mapcaller' Versions
- 0.9.9.41
Link to section 'Commands' of 'mapcaller' Commands
- MapCaller
Link to section 'Module' of 'mapcaller' Module
You can load the modules by:
module load biocontainers
module load mapcaller
Link to section 'Example job' of 'mapcaller' Example job
Using #!/bin/sh -l
as shebang in the slurm job script will cause the failure of some biocontainer modules. Please use #!/bin/bash
instead.
To run Mapcaller on our clusters:
#!/bin/bash
#SBATCH -A myallocation # Allocation name
#SBATCH -t 1:00:00
#SBATCH -N 1
#SBATCH -n 12
#SBATCH --job-name=mapcaller
#SBATCH --mail-type=FAIL,BEGIN,END
#SBATCH --error=%x-%J-%u.err
#SBATCH --output=%x-%J-%u.out
module --force purge
ml biocontainers mapcaller
MapCaller index ref.fasta ref
MapCaller -t 12 -i ref -f input_1.fastq -f2 input_2.fastq -vcf out.vcf
marginpolish
Link to section 'Introduction' of 'marginpolish' Introduction
MarginPolish is a graph-based assembly polisher. It iteratively finds multiple probable alignment paths for run-length-encoded reads and uses these to generate a refined sequence. It takes as input a FASTA assembly and an indexed BAM (ONT reads aligned to the assembly), and it produces a polished FASTA assembly.
Docker hub: https://hub.docker.com/r/kishwars/margin_polish
Home page: https://github.com/UCSC-nanopore-cgl/MarginPolish
Link to section 'Versions' of 'marginpolish' Versions
- 0.1.3
Link to section 'Commands' of 'marginpolish' Commands
- marginpolish
Link to section 'Module' of 'marginpolish' Module
You can load the modules by:
module load biocontainers
module load marginpolish
Link to section 'Example job' of 'marginpolish' Example job
Using #!/bin/sh -l
as shebang in the slurm job script will cause the failure of some biocontainer modules. Please use #!/bin/bash
instead.
To run marginpolish on our clusters:
#!/bin/bash
#SBATCH -A myallocation # Allocation name
#SBATCH -t 1:00:00
#SBATCH -N 1
#SBATCH -n 32
#SBATCH --job-name=marginpolish
#SBATCH --mail-type=FAIL,BEGIN,END
#SBATCH --error=%x-%J-%u.err
#SBATCH --output=%x-%J-%u.out
module --force purge
ml biocontainers marginpolish
marginpolish \
Reads_to_assembly_StaphAur.bam \
Draft_assembly_StaphAur.fasta \
helen_modles/MP_r941_guppy344_microbial.json \
-t 32 \
-o mp_output/mp_images \
-f
mash
Link to section 'Introduction' of 'mash' Introduction
Mash
is a fast sequence distance estimator that uses MinHash.
For more information, please check its website: https://biocontainers.pro/tools/mash and its home page on Github.
Link to section 'Versions' of 'mash' Versions
- 2.3
Link to section 'Commands' of 'mash' Commands
- mash
Link to section 'Module' of 'mash' Module
You can load the modules by:
module load biocontainers
module load mash
Link to section 'Example job' of 'mash' Example job
Using #!/bin/sh -l
as shebang in the slurm job script will cause the failure of some biocontainer modules. Please use #!/bin/bash
instead.
To run Mash on our clusters:
#!/bin/bash
#SBATCH -A myallocation # Allocation name
#SBATCH -t 1:00:00
#SBATCH -N 1
#SBATCH -n 1
#SBATCH --job-name=mash
#SBATCH --mail-type=FAIL,BEGIN,END
#SBATCH --error=%x-%J-%u.err
#SBATCH --output=%x-%J-%u.out
module --force purge
ml biocontainers mash
mash dist genome1.fasta genome2.fasta
mashmap
Link to section 'Introduction' of 'mashmap' Introduction
Mashmap
is a fast approximate aligner for long DNA sequences.
For more information, please check its website: https://biocontainers.pro/tools/mashmap and its home page on Github.
Link to section 'Versions' of 'mashmap' Versions
- 2.0-pl5321
Link to section 'Commands' of 'mashmap' Commands
- mashmap
Link to section 'Module' of 'mashmap' Module
You can load the modules by:
module load biocontainers
module load mashmap
Link to section 'Example job' of 'mashmap' Example job
Using #!/bin/sh -l
as shebang in the slurm job script will cause the failure of some biocontainer modules. Please use #!/bin/bash
instead.
To run Mashmap on our clusters:
#!/bin/bash
#SBATCH -A myallocation # Allocation name
#SBATCH -t 1:00:00
#SBATCH -N 1
#SBATCH -n 12
#SBATCH --job-name=mashmap
#SBATCH --mail-type=FAIL,BEGIN,END
#SBATCH --error=%x-%J-%u.err
#SBATCH --output=%x-%J-%u.out
module --force purge
ml biocontainers mashmap
mashmap -r ref.fasta -t 12 -q input.fasta
mashtree
Link to section 'Introduction' of 'mashtree' Introduction
Mashtree
is a tool to create a tree using Mash distances.
For more information, please check its website: https://biocontainers.pro/tools/mashtree and its home page on Github.
Link to section 'Versions' of 'mashtree' Versions
- 1.2.0
Link to section 'Commands' of 'mashtree' Commands
- mashtree
- mashtree_bootstrap.pl
- mashtree_cluster.pl
- mashtree_init.pl
- mashtree_jackknife.pl
- mashtree_wrapper_deprecated.pl
Link to section 'Module' of 'mashtree' Module
You can load the modules by:
module load biocontainers
module load mashtree
Link to section 'Example job' of 'mashtree' Example job
Using #!/bin/sh -l
as shebang in the slurm job script will cause the failure of some biocontainer modules. Please use #!/bin/bash
instead.
To run Mashtree on our clusters:
#!/bin/bash
#SBATCH -A myallocation # Allocation name
#SBATCH -t 1:00:00
#SBATCH -N 1
#SBATCH -n 1
#SBATCH --job-name=mashtree
#SBATCH --mail-type=FAIL,BEGIN,END
#SBATCH --error=%x-%J-%u.err
#SBATCH --output=%x-%J-%u.out
module --force purge
ml biocontainers mashtree
masurca
Link to section 'Introduction' of 'masurca' Introduction
The MaSuRCA (Maryland Super Read Cabog Assembler) genome assembly and analysis toolkit contains of MaSuRCA genome assembler, QuORUM error corrector for Illumina data, POLCA genome polishing software, Chromosome scaffolder, jellyfish mer counter, and MUMmer aligner.
Docker hub: https://hub.docker.com/r/staphb/masurca
Home page: https://github.com/alekseyzimin/masurca
Link to section 'Versions' of 'masurca' Versions
- 4.0.9
Link to section 'Commands' of 'masurca' Commands
- masurca
- build_human_reference.sh
- chromosome_scaffolder.sh
- close_gaps.sh
- close_scaffold_gaps.sh
- correct_with_k_unitigs.sh
- deduplicate_contigs.sh
- deduplicate_unitigs.sh
- eugene.sh
- extract_chrM.sh
- filter_library.sh
- final_polish.sh
- fix_unitigs.sh
- fragScaff.sh
- mega_reads_assemble_cluster.sh
- mega_reads_assemble_cluster2.sh
- mega_reads_assemble_polish.sh
- mega_reads_assemble_ref.sh
- parallel_delta-filter.sh
- polca.sh
- polish_with_illumina_assembly.sh
- recompute_astat_superreads.sh
- recompute_astat_superreads_CA8.sh
- reconcile_alignments.sh
- refine.sh
- resolve_trio.sh
- run_ECR.sh
- samba.sh
- splitScaffoldsAtNs.sh
Link to section 'Module' of 'masurca' Module
You can load the modules by:
module load biocontainers
module load masurca
Link to section 'Example job' of 'masurca' Example job
Using #!/bin/sh -l
as shebang in the slurm job script will cause the failure of some biocontainer modules. Please use #!/bin/bash
instead.
To run masurca on our clusters:
#!/bin/bash
#SBATCH -A myallocation # Allocation name
#SBATCH -t 1:00:00
#SBATCH -N 1
#SBATCH -n 1
#SBATCH --job-name=masurca
#SBATCH --mail-type=FAIL,BEGIN,END
#SBATCH --error=%x-%J-%u.err
#SBATCH --output=%x-%J-%u.out
module --force purge
ml biocontainers masurca
mauve
Link to section 'Introduction' of 'mauve' Introduction
Mauve
is a system for constructing multiple genome alignments in the presence of large-scale evolutionary events such as rearrangement and inversion.
For more information, please check its website: https://biocontainers.pro/tools/mauve.
Link to section 'Versions' of 'mauve' Versions
- 2.4.0
Link to section 'Commands' of 'mauve' Commands
- mauveAligner
- progressiveMauve
Link to section 'Module' of 'mauve' Module
You can load the modules by:
module load biocontainers
module load mauve
Link to section 'Example job' of 'mauve' Example job
Using #!/bin/sh -l
as shebang in the slurm job script will cause the failure of some biocontainer modules. Please use #!/bin/bash
instead.
To run Mauve on our clusters:
#!/bin/bash
#SBATCH -A myallocation # Allocation name
#SBATCH -t 1:00:00
#SBATCH -N 1
#SBATCH -n 1
#SBATCH --job-name=mauve
#SBATCH --mail-type=FAIL,BEGIN,END
#SBATCH --error=%x-%J-%u.err
#SBATCH --output=%x-%J-%u.out
module --force purge
ml biocontainers mauve
mauveAligner seqs.fasta --output=mauveAligner_output
progressiveMauve --output=threeway.xmfa \
--output-guide-tree=threeway.tree \
--backbone-output=threeway.backbone genome1.gbk genome2.gbk genome3.gbk
maxbin2
Link to section 'Introduction' of 'maxbin2' Introduction
Maxbin2 is a software for binning assembled metagenomic sequences based on an Expectation-Maximization algorithm.
Docker hub: https://hub.docker.com/r/nanozoo/maxbin2
Home page: https://sourceforge.net/projects/maxbin2/
Link to section 'Versions' of 'maxbin2' Versions
- 2.2.7
Link to section 'Commands' of 'maxbin2' Commands
- run_MaxBin.pl
- run_FragGeneScan.pl
Link to section 'Module' of 'maxbin2' Module
You can load the modules by:
module load biocontainers
module load maxbin2
Link to section 'Example job' of 'maxbin2' Example job
Using #!/bin/sh -l
as shebang in the slurm job script will cause the failure of some biocontainer modules. Please use #!/bin/bash
instead.
To run maxbin2 on our clusters:
#!/bin/bash
#SBATCH -A myallocation # Allocation name
#SBATCH -t 1:00:00
#SBATCH -N 1
#SBATCH -n 1
#SBATCH --job-name=maxbin2
#SBATCH --mail-type=FAIL,BEGIN,END
#SBATCH --error=%x-%J-%u.err
#SBATCH --output=%x-%J-%u.out
module --force purge
ml biocontainers maxbin2
run_MaxBin.pl -contig subset_assembly.fa \
-abund_list abundance.list -max_iteration 5 -out mbin
maxquant
Link to section 'Introduction' of 'maxquant' Introduction
Maxquant
is a quantitative proteomics software package designed for analyzing large mass-spectrometric data sets. It is specifically aimed at high-resolution MS data.
For more information, please check home page: https://www.maxquant.org.
Link to section 'Versions' of 'maxquant' Versions
- 2.1.0.0
- 2.1.3.0
- 2.1.4.0
- 2.3.1.0
Link to section 'Commands' of 'maxquant' Commands
- MaxQuantGui.exe
- MaxQuantCmd.exe
Link to section 'Module' of 'maxquant' Module
You can load the modules by:
module load biocontainers
module load maxquant
Link to section 'GUI' of 'maxquant' GUI
To run Maxquant with GUI, it is recommended to run within ThinLinc:
(base) UserID@bell-fe00:~ $ sinteractive -N1 -n12 -t4:00:00 -A myallocation
salloc: Granted job allocation 12345869
salloc: Waiting for resource configuration
salloc: Nodes bell-a008 are ready for job
(base) UserID@bell-a008:~ $ module load biocontainers maxquant
(base) UserID@bell-a008:~ $ MaxQuantGui.exe
Link to section 'CMD job' of 'maxquant' CMD job
Using #!/bin/sh -l
as shebang in the slurm job script will cause the failure of some biocontainer modules. Please use #!/bin/bash
instead.
To run Maxquant without GUI on our clusters:
#!/bin/bash
#SBATCH -A myallocation # Allocation name
#SBATCH -t 1:00:00
#SBATCH -N 1
#SBATCH -n 1
#SBATCH --job-name=maxquant
#SBATCH --mail-type=FAIL,BEGIN,END
#SBATCH --error=%x-%J-%u.err
#SBATCH --output=%x-%J-%u.out
module --force purge
ml biocontainers maxquant
MaxQuantCmd.exe mqpar.xml
mcl
Link to section 'Introduction' of 'mcl' Introduction
Mcl
is short for the Markov Cluster Algorithm, a fast and scalable unsupervised cluster algorithm for graphs.
For more information, please check its website: https://biocontainers.pro/tools/mcl and its home page: http://micans.org/mcl/.
Link to section 'Versions' of 'mcl' Versions
- 14.137-pl5262
Link to section 'Commands' of 'mcl' Commands
- clm
- clmformat
- clxdo
- mcl
- mclblastline
- mclcm
- mclpipeline
- mcx
- mcxarray
- mcxassemble
- mcxdeblast
- mcxdump
- mcxi
- mcxload
- mcxmap
- mcxrand
- mcxsubs
Link to section 'Module' of 'mcl' Module
You can load the modules by:
module load biocontainers
module load mcl
Link to section 'Example job' of 'mcl' Example job
Using #!/bin/sh -l
as shebang in the slurm job script will cause the failure of some biocontainer modules. Please use #!/bin/bash
instead.
To run Mcl on our clusters:
#!/bin/bash
#SBATCH -A myallocation # Allocation name
#SBATCH -t 1:00:00
#SBATCH -N 1
#SBATCH -n 1
#SBATCH --job-name=mcl
#SBATCH --mail-type=FAIL,BEGIN,END
#SBATCH --error=%x-%J-%u.err
#SBATCH --output=%x-%J-%u.out
module --force purge
ml biocontainers mcl
mcscanx
The MCScanX package has two major components: a modified version of MCscan algorithm allowing users to handle MCScan more conveniently and to view multiple alignment of syntenic blocks more clearly, and a variety of downstream analysis tools to conduct different biological analyses based on the synteny data generated by the modified MCScan algorithm.
Home page: https://github.com/wyp1125/MCScanX.
Link to section 'Versions' of 'mcscanx' Versions
- default
Link to section 'Commands' of 'mcscanx' Commands
- MCScanX
- MCScanX_h
- duplicate_gene_classifier
- add_ka_and_ks_to_collinearity
- add_kaks_to_synteny
- detect_collinearity_within_gene_families
- detect_synteny_within_gene_families
- group_collinear_genes
- group_syntenic_genes
- origin_enrichment_analysis
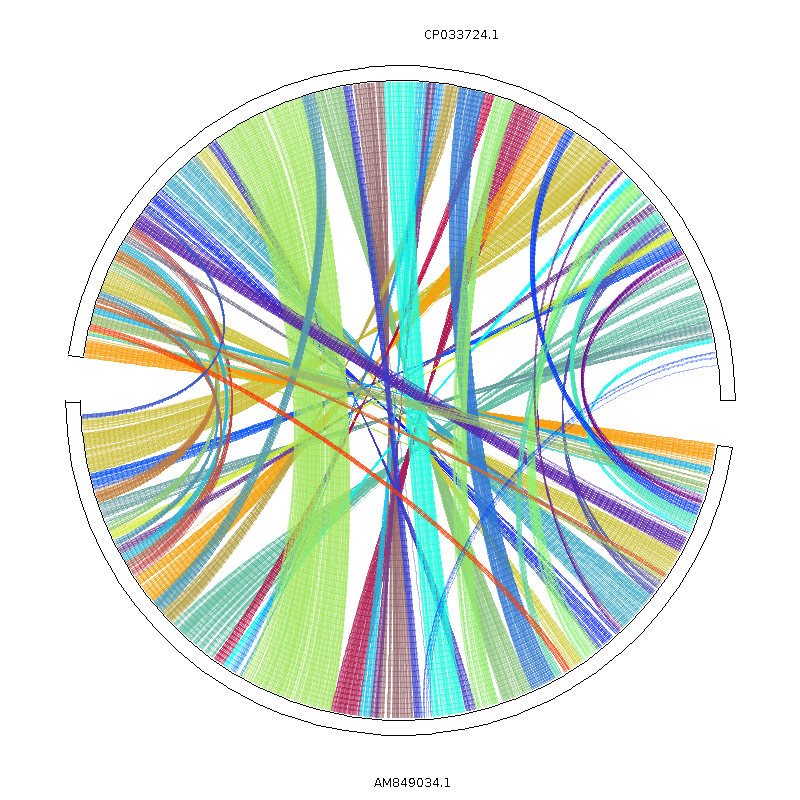
Link to section 'Introduction' of 'mcscanx' Introduction
Link to section 'Module' of 'mcscanx' Module
You can load the modules by:
module load biocontainers
module load mcscanx
Helper command
To conduct downstream analyses, users need to copy the folder downstream_analyses
from container into the host system.
A helper command copy_downstream_analyses
is provided to simplify the task. Follow the procedure below to copy downstream_analyses into target directory:
$ copy_downstream_analyses $PWD # this will copy the downstream_analyses into the current directory.
Link to section 'Example job' of 'mcscanx' Example job
Using #!/bin/sh -l
as shebang in the slurm job script will cause the failure of some biocontainer modules. Please use #!/bin/bash
instead.
To run mcscanx on our clusters:
#!/bin/bash
#SBATCH -A myallocation # Allocation name
#SBATCH -t 1:00:00
#SBATCH -N 1
#SBATCH -n 1
#SBATCH --job-name=mcscanx
#SBATCH --mail-type=FAIL,BEGIN,END
#SBATCH --error=%x-%J-%u.err
#SBATCH --output=%x-%J-%u.out
module --force purge
ml biocontainers mcscanx
## Run MCScanX
MCScanX Result/merge
## Copy downstream_analyses
copy_downstream_analyses $PWD
## Downstream analyses
java circle_plotter -g ../Result/merge.gff -s ../Result/merge.collinearity -c ../Result/merge_circ.ctl -o ../Result/merge_circle.png
java dot_plotter -g ../Result/merge.gff -s ../Result/merge.collinearity -c ../Result/merge_dot.ctl -o ../Result/merge_dot.png
java dual_synteny_plotter -g ../Result/merge.gff -s ../Result/merge.collinearity -c ../Result/merge_dot.ctl -o ../Result/merge_dual_synteny.png
medaka
Link to section 'Introduction' of 'medaka' Introduction
Medaka
is a tool to create consensus sequences and variant calls from nanopore sequencing data.
Docker hub: https://hub.docker.com/r/ontresearch/medaka and its home page on Github.
Link to section 'Versions' of 'medaka' Versions
- 1.6.0
Link to section 'Commands' of 'medaka' Commands
- medaka
- medaka_consensus
- medaka_counts
- medaka_data_path
- medaka_haploid_variant
- medaka_version_report
Link to section 'Module' of 'medaka' Module
You can load the modules by:
module load biocontainers
module load medaka
Link to section 'Example job' of 'medaka' Example job
Using #!/bin/sh -l
as shebang in the slurm job script will cause the failure of some biocontainer modules. Please use #!/bin/bash
instead.
To run Medaka on our clusters:
#!/bin/bash
#SBATCH -A myallocation # Allocation name
#SBATCH -t 1:00:00
#SBATCH -N 1
#SBATCH -n 1
#SBATCH --job-name=medaka
#SBATCH --mail-type=FAIL,BEGIN,END
#SBATCH --error=%x-%J-%u.err
#SBATCH --output=%x-%J-%u.out
module --force purge
ml biocontainers medaka
megadepth
Link to section 'Introduction' of 'megadepth' Introduction
Megadepth
is an efficient tool for extracting coverage related information from RNA and DNA-seq BAM and BigWig files.
For more information, please check its website: https://biocontainers.pro/tools/megadepth and its home page on Github.
Link to section 'Versions' of 'megadepth' Versions
- 1.2.0
Link to section 'Commands' of 'megadepth' Commands
- megadepth
Link to section 'Module' of 'megadepth' Module
You can load the modules by:
module load biocontainers
module load megadepth
Link to section 'Example job' of 'megadepth' Example job
Using #!/bin/sh -l
as shebang in the slurm job script will cause the failure of some biocontainer modules. Please use #!/bin/bash
instead.
To run Megadepth on our clusters:
#!/bin/bash
#SBATCH -A myallocation # Allocation name
#SBATCH -t 1:00:00
#SBATCH -N 1
#SBATCH -n 1
#SBATCH --job-name=megadepth
#SBATCH --mail-type=FAIL,BEGIN,END
#SBATCH --error=%x-%J-%u.err
#SBATCH --output=%x-%J-%u.out
module --force purge
ml biocontainers megadepth
megadepth sorted.bam
megahit
Link to section 'Introduction' of 'megahit' Introduction
Megahit
is a ultra-fast single-node solution for large and complex metagenomics assembly via succinct de Bruijn graph.
For more information, please check its website: https://biocontainers.pro/tools/megahit and its home page on Github.
Link to section 'Versions' of 'megahit' Versions
- 1.2.9
Link to section 'Commands' of 'megahit' Commands
- megahit
Link to section 'Module' of 'megahit' Module
You can load the modules by:
module load biocontainers
module load megahit
Link to section 'Example job' of 'megahit' Example job
Using #!/bin/sh -l
as shebang in the slurm job script will cause the failure of some biocontainer modules. Please use #!/bin/bash
instead.
To run Megahit on our clusters:
#!/bin/bash
#SBATCH -A myallocation # Allocation name
#SBATCH -t 1:00:00
#SBATCH -N 1
#SBATCH -n 12
#SBATCH --job-name=megahit
#SBATCH --mail-type=FAIL,BEGIN,END
#SBATCH --error=%x-%J-%u.err
#SBATCH --output=%x-%J-%u.out
module --force purge
ml biocontainers megahit
megahit --12 SRR1976948.abundtrim.subset.pe.fq.gz,SRR1977249.abundtrim.subset.pe.fq.gz -o combined
megan
Link to section 'Introduction' of 'megan' Introduction
Megan
is a computer program that allows optimized analysis of large metagenomic datasets. Metagenomics is the analysis of the genomic sequences from a usually uncultured environmental sample.
For more information, please check its website: https://biocontainers.pro/tools/megan and its home page: https://uni-tuebingen.de/fakultaeten/mathematisch-naturwissenschaftliche-fakultaet/fachbereiche/informatik/lehrstuehle/algorithms-in-bioinformatics/software/megan6/.
Link to section 'Versions' of 'megan' Versions
- 6.21.7
Link to section 'Commands' of 'megan' Commands
- MEGAN
- blast2lca
- blast2rma
- daa2info
- daa2rma
- daa-meganizer
- gc-assembler
- rma2info
- sam2rma
- references-annotator
Link to section 'Module' of 'megan' Module
You can load the modules by:
module load biocontainers
module load megan
Link to section 'GUI' of 'megan' GUI
To run MEGAN with GUI, it is recommended to run within ThinLinc:
(base) UserID@bell-fe00:~ $ sinteractive -N1 -n12 -t4:00:00 -A myallocation
salloc: Granted job allocation 12345869
salloc: Waiting for resource configuration
salloc: Nodes bell-a008 are ready for job
(base) UserID@bell-a008:~ $ module load biocontainers megan
(base) UserID@bell-a008:~ $ MEGAN
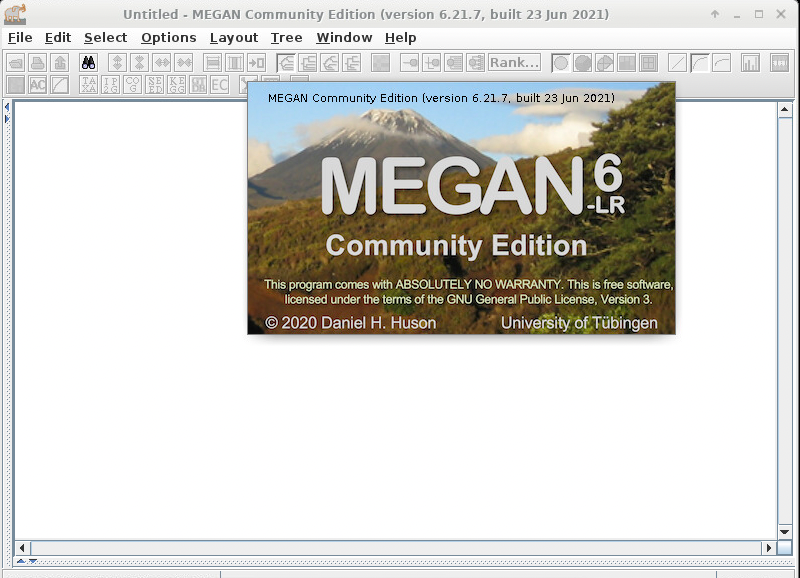
Link to section 'Example job' of 'megan' Example job
Using #!/bin/sh -l
as shebang in the slurm job script will cause the failure of some biocontainer modules. Please use #!/bin/bash
instead.
To run Megan on our clusters:
#!/bin/bash
#SBATCH -A myallocation # Allocation name
#SBATCH -t 1:00:00
#SBATCH -N 1
#SBATCH -n 1
#SBATCH --job-name=megan
#SBATCH --mail-type=FAIL,BEGIN,END
#SBATCH --error=%x-%J-%u.err
#SBATCH --output=%x-%J-%u.out
module --force purge
ml biocontainers megan
meme
Link to section 'Introduction' of 'meme' Introduction
Meme
is a collection of tools for the discovery and analysis of sequence motifs.
For more information, please check its website: https://biocontainers.pro/tools/meme and its home page: https://meme-suite.org/meme/.
Link to section 'Versions' of 'meme' Versions
- 5.3.3
- 5.4.1
- 5.5.0
Link to section 'Commands' of 'meme' Commands
- ame
- centrimo
- dreme
- dust
- fimo
- glam2
- glam2scan
- gomo
- mast
- mcast
- meme
- meme-chip
- momo
- purge
- spamo
- tomtom
Link to section 'Module' of 'meme' Module
You can load the modules by:
module load biocontainers
module load meme
Link to section 'Example job' of 'meme' Example job
Using #!/bin/sh -l
as shebang in the slurm job script will cause the failure of some biocontainer modules. Please use #!/bin/bash
instead.
To run Meme on our clusters:
#!/bin/bash
#SBATCH -A myallocation # Allocation name
#SBATCH -t 1:00:00
#SBATCH -N 1
#SBATCH -n 1
#SBATCH --job-name=meme
#SBATCH --mail-type=FAIL,BEGIN,END
#SBATCH --error=%x-%J-%u.err
#SBATCH --output=%x-%J-%u.out
module --force purge
ml biocontainers meme
meme seq.fasta -dna -mod oops -pal
meme-chip Klf1.fna -o memechip_klf1_out
memes
Link to section 'Introduction' of 'memes' Introduction
memes is an R interface to the MEME Suite family of tools, which provides several utilities for performing motif analysis on DNA, RNA, and protein sequences. memes works by detecting a local install of the MEME suite, running the commands, then importing the results directly into R.
Docker hub: https://hub.docker.com/r/snystrom/memes_docker
Home page: https://github.com/snystrom/memes
Link to section 'Versions' of 'memes' Versions
- 1.1.2
Link to section 'Commands' of 'memes' Commands
- R
Link to section 'Module' of 'memes' Module
You can load the modules by:
module load biocontainers
module load memes
Link to section 'Example job' of 'memes' Example job
Using #!/bin/sh -l
as shebang in the slurm job script will cause the failure of some biocontainer modules. Please use #!/bin/bash
instead.
To run memes on our clusters:
#!/bin/bash
#SBATCH -A myallocation # Allocation name
#SBATCH -t 1:00:00
#SBATCH -N 1
#SBATCH -n 1
#SBATCH --job-name=memes
#SBATCH --mail-type=FAIL,BEGIN,END
#SBATCH --error=%x-%J-%u.err
#SBATCH --output=%x-%J-%u.out
module --force purge
ml biocontainers memes
meraculous
Link to section 'Introduction' of 'meraculous' Introduction
Meraculous is a whole genome assembler for Next Generation Sequencing data, geared for large genomes. It is hybrid k-mer/read-based approach capitalizes on the high accuracy of Illumina sequence by eschewing an explicit error correction step which we argue to be redundant with the assembly process. Meraculous achieves high performance with large datasets by utilizing lightweight data structures and multi-threaded parallelization, allowing to assemble human-sized genomes on a high-cpu cluster in under a day. The process pipeline implements a highly transparent and portable model of job control and monitoring where different assembly stages can be executed and re-executed separately or in unison on a wide variety of architectures.
Home page: https://jgi.doe.gov/data-and-tools/software-tools/meraculous/
Link to section 'Versions' of 'meraculous' Versions
- 2.2.6
Link to section 'Commands' of 'meraculous' Commands
- run_meraculous.sh
- blastMapAnalyzer2.pl
- bmaToLinks.pl
- _bubbleFinder2.pl
- bubblePopper.pl
- bubbleScout.pl
- contigBias.pl
- divide_it.pl
- fasta_splitter.pl
- findDMin2.pl
- gapDivider.pl
- gapPlacer.pl
- haplotyper.Naive.pl
- haplotyper.pl
- histogram2.pl
- kmerHistAnalyzer.pl
- loadBalanceMers.pl
- meraculous4h.pl
- meraculous.pl
- N50.pl
- _oNo4.pl
- oNo7.pl
- optimize2.pl
- randomList2.pl
- scaffold2contig.pl
- scaffReportToFasta.pl
- screen_list2.pl
- spanner.pl
- splinter.pl
- splinter_scaffolds.pl
- split_and_validate_reads.pl
- test_dependencies.pl
- unique.pl
Link to section 'Module' of 'meraculous' Module
You can load the modules by:
module load biocontainers
module load meraculous
Link to section 'Example job' of 'meraculous' Example job
Using #!/bin/sh -l
as shebang in the slurm job script will cause the failure of some biocontainer modules. Please use #!/bin/bash
instead.
To run meraculous on our clusters:
#!/bin/bash
#SBATCH -A myallocation # Allocation name
#SBATCH -t 1:00:00
#SBATCH -N 1
#SBATCH -n 1
#SBATCH --job-name=meraculous
#SBATCH --mail-type=FAIL,BEGIN,END
#SBATCH --error=%x-%J-%u.err
#SBATCH --output=%x-%J-%u.out
module --force purge
ml biocontainers meraculous
merqury
Link to section 'Introduction' of 'merqury' Introduction
Merqury is a tool to evaluate genome assemblies with k-mers and more.
Docker hub: https://hub.docker.com/r/dovetailg/merqury
Home page: https://github.com/marbl/merqury
Link to section 'Versions' of 'merqury' Versions
- 1.3
Link to section 'Commands' of 'merqury' Commands
- merqury.sh
Link to section 'Module' of 'merqury' Module
You can load the modules by:
module load biocontainers
module load merqury
Link to section 'Example job' of 'merqury' Example job
Using #!/bin/sh -l
as shebang in the slurm job script will cause the failure of some biocontainer modules. Please use #!/bin/bash
instead.
To run merqury on our clusters:
#!/bin/bash
#SBATCH -A myallocation # Allocation name
#SBATCH -t 1:00:00
#SBATCH -N 1
#SBATCH -n 1
#SBATCH --job-name=merqury
#SBATCH --mail-type=FAIL,BEGIN,END
#SBATCH --error=%x-%J-%u.err
#SBATCH --output=%x-%J-%u.out
module --force purge
ml biocontainers merqury
merqury.sh F1.k18.meryl col0.hapmer.meryl cvi0.hapmer.meryl \
athal_COL.fasta athal_CVI.fasta test
meryl
Link to section 'Introduction' of 'meryl' Introduction
Meryl
is a genomic k-mer counter (and sequence utility) with nice features.
For more information, please check its website: https://biocontainers.pro/tools/meryl and its home page on Github.
Link to section 'Versions' of 'meryl' Versions
- 1.3
Link to section 'Commands' of 'meryl' Commands
- meryl
- meryl-analyze
- meryl-import
- meryl-lookup
- meryl-simple
Link to section 'Module' of 'meryl' Module
You can load the modules by:
module load biocontainers
module load meryl
Link to section 'Example job' of 'meryl' Example job
Using #!/bin/sh -l
as shebang in the slurm job script will cause the failure of some biocontainer modules. Please use #!/bin/bash
instead.
To run Meryl on our clusters:
#!/bin/bash
#SBATCH -A myallocation # Allocation name
#SBATCH -t 1:00:00
#SBATCH -N 1
#SBATCH -n 1
#SBATCH --job-name=meryl
#SBATCH --mail-type=FAIL,BEGIN,END
#SBATCH --error=%x-%J-%u.err
#SBATCH --output=%x-%J-%u.out
module --force purge
ml biocontainers meryl
meryl count k=42 data/ec.fna.gz output ec.meryl
metabat
Link to section 'Introduction' of 'metabat' Introduction
Metabat
is a robust statistical framework for reconstructing genomes from metagenomic data.
Docker hub: https://hub.docker.com/r/metabat/metabat and its home page: https://bitbucket.org/berkeleylab/metabat/src/master/
Link to section 'Versions' of 'metabat' Versions
- 2.15-5
Link to section 'Commands' of 'metabat' Commands
- aggregateBinDepths.pl
- aggregateContigOverlapsByBin.pl
- contigOverlaps
- jgi_summarize_bam_contig_depths
- merge_depths.pl
- metabat
- metabat1
- metabat2
- runMetaBat.sh
Link to section 'Module' of 'metabat' Module
You can load the modules by:
module load biocontainers
module load metabat
Link to section 'Example job' of 'metabat' Example job
Using #!/bin/sh -l
as shebang in the slurm job script will cause the failure of some biocontainer modules. Please use #!/bin/bash
instead.
To run Metabat on our clusters:
#!/bin/bash
#SBATCH -A myallocation # Allocation name
#SBATCH -t 1:00:00
#SBATCH -N 1
#SBATCH -n 24
#SBATCH --job-name=metabat
#SBATCH --mail-type=FAIL,BEGIN,END
#SBATCH --error=%x-%J-%u.err
#SBATCH --output=%x-%J-%u.out
module --force purge
ml biocontainers metabat
metabat2 -m 10000 \
-t 24 \
-i contig.fasta \
-o metabat2_output \
-a depth.txt
metachip
Link to section 'Introduction' of 'metachip' Introduction
Metachip is a pipeline for Horizontal gene transfer (HGT) identification.
BioContainers: https://biocontainers.pro/tools/metachip
Home page: https://github.com/songweizhi/MetaCHIP
Link to section 'Versions' of 'metachip' Versions
- 1.10.12
Link to section 'Commands' of 'metachip' Commands
- MetaCHIP
Link to section 'Module' of 'metachip' Module
You can load the modules by:
module load biocontainers
module load metachip
Link to section 'Example job' of 'metachip' Example job
Using #!/bin/sh -l
as shebang in the slurm job script will cause the failure of some biocontainer modules. Please use #!/bin/bash
instead.
To run metachip on our clusters:
#!/bin/bash
#SBATCH -A myallocation # Allocation name
#SBATCH -t 1:00:00
#SBATCH -N 1
#SBATCH -n 1
#SBATCH --job-name=metachip
#SBATCH --mail-type=FAIL,BEGIN,END
#SBATCH --error=%x-%J-%u.err
#SBATCH --output=%x-%J-%u.out
module --force purge
ml biocontainers metachip
metaphlan
Link to section 'Introduction' of 'metaphlan' Introduction
MetaPhlAn
(Metagenomic Phylogenetic Analysis) is a computational tool for profiling the composition of microbial communities from metagenomic shotgun sequencing data. MetaPhlAn relies on unique clade-specific marker genes identified from 17,000 reference genomes ( 13,500 bacterial and archaeal, 3,500 viral, and 110 eukaryotic), allowing:
- up to 25,000 reads-per-second (on one CPU) analysis speed (orders of magnitude faster compared to existing methods);
- unambiguous taxonomic assignments as the MetaPhlAn markers are clade-specific;
- accurate estimation of organismal relative abundance (in terms of number of cells rather than fraction of reads);
- species-level resolution for bacteria, archaea, eukaryotes and viruses;
- extensive validation of the profiling accuracy on several synthetic datasets and on thousands of real metagenomes.
For more information, please check its user guide at: https://huttenhower.sph.harvard.edu/metaphlan/
Link to section 'Versions' of 'metaphlan' Versions
- 3.0.14
- 3.0.9
- 4.0.2
Commands
metaphlan
Link to section 'Database' of 'metaphlan' Database
The latest version of database(mpa_v30) has been downloaded and built in /depot/itap/datasets/metaphlan/
.
Link to section 'Module' of 'metaphlan' Module
You can load the modules by:
module load biocontainers
module load metaphlan/3.0.14
Link to section 'Example job' of 'metaphlan' Example job
Using #!/bin/sh -l
as shebang in the slurm job script will cause the failure of some biocontainer modules. Please use #!/bin/bash
instead.
To run MetaPhlAn on our cluster:
#!/bin/bash
#SBATCH -A myallocation # Allocation name
#SBATCH -t 10:00:00
#SBATCH -N 1
#SBATCH -n 24
#SBATCH --job-name=MetaPhlAn
#SBATCH --mail-type=FAIL,BEGIN,END
#SBATCH --error=%x-%J-%u.err
#SBATCH --output=%x-%J-%u.out
module --force purge
ml biocontainers metaphlan/3.0.14
DATABASE=/depot/itap/datasets/metaphlan/
metaphlan SRR11234553_1.fastq,SRR11234553_2.fastq --input_type fastq --nproc 24 -o profiled_metagenome.txt --bowtie2db $DATABASE --bowtie2out metagenome.bowtie2.bz2
metaseq
Link to section 'Introduction' of 'metaseq' Introduction
Metaseq is a Python package for integrative genome-wide analysis reveals relationships between chromatin insulators and associated nuclear mRNA.
Docker hub: https://hub.docker.com/r/vsmalladi/metaseq
Home page: https://github.com/daler/metaseq
Link to section 'Versions' of 'metaseq' Versions
- 0.5.6
Link to section 'Commands' of 'metaseq' Commands
- python
- python2
Link to section 'Module' of 'metaseq' Module
You can load the modules by:
module load biocontainers
module load metaseq
Link to section 'Example job' of 'metaseq' Example job
Using #!/bin/sh -l
as shebang in the slurm job script will cause the failure of some biocontainer modules. Please use #!/bin/bash
instead.
To run metaseq on our clusters:
#!/bin/bash
#SBATCH -A myallocation # Allocation name
#SBATCH -t 1:00:00
#SBATCH -N 1
#SBATCH -n 1
#SBATCH --job-name=metaseq
#SBATCH --mail-type=FAIL,BEGIN,END
#SBATCH --error=%x-%J-%u.err
#SBATCH --output=%x-%J-%u.out
module --force purge
ml biocontainers metaseq
methyldackel
Link to section 'Introduction' of 'methyldackel' Introduction
MethylDackel (formerly named PileOMeth, which was a temporary name derived due to it using a PILEup to extract METHylation metrics) will process a coordinate-sorted and indexed BAM or CRAM file containing some form of BS-seq alignments and extract per-base methylation metrics from them. MethylDackel requires an indexed fasta file containing the reference genome as well.
BioContainers: https://biocontainers.pro/tools/methyldackel
Home page: https://github.com/dpryan79/MethylDackel
Link to section 'Versions' of 'methyldackel' Versions
- 0.6.1
Link to section 'Commands' of 'methyldackel' Commands
- MethylDackel
Link to section 'Module' of 'methyldackel' Module
You can load the modules by:
module load biocontainers
module load methyldackel
Link to section 'Example job' of 'methyldackel' Example job
Using #!/bin/sh -l
as shebang in the slurm job script will cause the failure of some biocontainer modules. Please use #!/bin/bash
instead.
To run methyldackel on our clusters:
#!/bin/bash
#SBATCH -A myallocation # Allocation name
#SBATCH -t 1:00:00
#SBATCH -N 1
#SBATCH -n 1
#SBATCH --job-name=methyldackel
#SBATCH --mail-type=FAIL,BEGIN,END
#SBATCH --error=%x-%J-%u.err
#SBATCH --output=%x-%J-%u.out
module --force purge
ml biocontainers methyldackel
MethylDackel extract chgchh.fa chgchh_aln.bam
metilene
Link to section 'Introduction' of 'metilene' Introduction
Metilene is a versatile tool to study the effect of epigenetic modifications in differentiation/development, tumorigenesis, and systems biology on a global, genome-wide level.
BioContainers: https://biocontainers.pro/tools/metilene
Home page: https://www.bioinf.uni-leipzig.de/Software/metilene/
Link to section 'Versions' of 'metilene' Versions
- 0.2.8
Link to section 'Commands' of 'metilene' Commands
- metilene
- metilene_input.pl
- metilene_output.pl
- metilene_output.R
Link to section 'Module' of 'metilene' Module
You can load the modules by:
module load biocontainers
module load metilene
Link to section 'Example job' of 'metilene' Example job
Using #!/bin/sh -l
as shebang in the slurm job script will cause the failure of some biocontainer modules. Please use #!/bin/bash
instead.
To run metilene on our clusters:
#!/bin/bash
#SBATCH -A myallocation # Allocation name
#SBATCH -t 1:00:00
#SBATCH -N 1
#SBATCH -n 1
#SBATCH --job-name=metilene
#SBATCH --mail-type=FAIL,BEGIN,END
#SBATCH --error=%x-%J-%u.err
#SBATCH --output=%x-%J-%u.out
module --force purge
ml biocontainers metilene
metilene -a g1 -b g2 methylation-file
mhm2
Link to section 'Introduction' of 'mhm2' Introduction
MetaHipMer is a de novo metagenome short-read assembler. Version 2 (MHM2) is written entirely in UPC++ and runs efficiently on both single servers and on multinode supercomputers, where it can scale up to coassemble terabase-sized metagenomes.
Home page: https://bitbucket.org/berkeleylab/mhm2/wiki/Home.md
Link to section 'Versions' of 'mhm2' Versions
- 2.0.0
Link to section 'Commands' of 'mhm2' Commands
- mhm2.py
Link to section 'Module' of 'mhm2' Module
You can load the modules by:
module load biocontainers
module load mhm2
Link to section 'Example job' of 'mhm2' Example job
Using #!/bin/sh -l
as shebang in the slurm job script will cause the failure of some biocontainer modules. Please use #!/bin/bash
instead.
To run mhm2 on our clusters:
#!/bin/bash
#SBATCH -A myallocation # Allocation name
#SBATCH -t 1:00:00
#SBATCH -N 1
#SBATCH -n 1
#SBATCH --job-name=mhm2
#SBATCH --mail-type=FAIL,BEGIN,END
#SBATCH --error=%x-%J-%u.err
#SBATCH --output=%x-%J-%u.out
module --force purge
ml biocontainers mhm2
mhm2.py -r input_1.fastq,input_2.fastq
microbedmm
Link to section 'Introduction' of 'microbedmm' Introduction
MicrobeDMM
is a suite of programs used for empirical Bayes fitting of DMM models.
For more information, please check its home page: https://code.google.com/archive/p/microbedmm.
Link to section 'Versions' of 'microbedmm' Versions
- 1.0
Link to section 'Commands' of 'microbedmm' Commands
- DirichletMixtureGHPFit
Link to section 'Module' of 'microbedmm' Module
You can load the modules by:
module load biocontainers
module load microbedmm
Link to section 'Example job' of 'microbedmm' Example job
Using #!/bin/sh -l
as shebang in the slurm job script will cause the failure of some biocontainer modules. Please use #!/bin/bash
instead.
To run MicrobeDMM on our clusters:
#!/bin/bash
#SBATCH -A myallocation # Allocation name
#SBATCH -t 1:00:00
#SBATCH -N 1
#SBATCH -n 1
#SBATCH --job-name=microbedmm
#SBATCH --mail-type=FAIL,BEGIN,END
#SBATCH --error=%x-%J-%u.err
#SBATCH --output=%x-%J-%u.out
module --force purge
ml biocontainers microbedmm
minialign
Link to section 'Introduction' of 'minialign' Introduction
Minialign
is a little bit fast and moderately accurate nucleotide sequence alignment tool designed for PacBio and Nanopore long reads.
For more information, please check its website: https://biocontainers.pro/tools/minialign and its home page on Github.
Link to section 'Versions' of 'minialign' Versions
- 0.5.3
Link to section 'Commands' of 'minialign' Commands
- minialign
Link to section 'Module' of 'minialign' Module
You can load the modules by:
module load biocontainers
module load minialign
Link to section 'Example job' of 'minialign' Example job
Using #!/bin/sh -l
as shebang in the slurm job script will cause the failure of some biocontainer modules. Please use #!/bin/bash
instead.
To run Minialign on our clusters:
#!/bin/bash
#SBATCH -A myallocation # Allocation name
#SBATCH -t 1:00:00
#SBATCH -N 1
#SBATCH -n 1
#SBATCH --job-name=minialign
#SBATCH --mail-type=FAIL,BEGIN,END
#SBATCH --error=%x-%J-%u.err
#SBATCH --output=%x-%J-%u.out
module --force purge
ml biocontainers minialign
minialign -d index.mai genome.fasta
minialign -l index.mai input.fastq > out.sam
miniasm
Link to section 'Introduction' of 'miniasm' Introduction
Miniasm
is a very fast OLC-based de novo assembler for noisy long reads.
For more information, please check its website: https://biocontainers.pro/tools/miniasm and its home page on Github.
Link to section 'Versions' of 'miniasm' Versions
- 0.3_r179
Link to section 'Commands' of 'miniasm' Commands
- miniasm
- minidot
Link to section 'Module' of 'miniasm' Module
You can load the modules by:
module load biocontainers
module load miniasm
Link to section 'Example job' of 'miniasm' Example job
Using #!/bin/sh -l
as shebang in the slurm job script will cause the failure of some biocontainer modules. Please use #!/bin/bash
instead.
To run Miniasm on our clusters:
#!/bin/bash
#SBATCH -A myallocation # Allocation name
#SBATCH -t 1:00:00
#SBATCH -N 1
#SBATCH -n 12
#SBATCH --job-name=miniasm
#SBATCH --mail-type=FAIL,BEGIN,END
#SBATCH --error=%x-%J-%u.err
#SBATCH --output=%x-%J-%u.out
module --force purge
ml biocontainers miniasm
miniasm -f Elysia_ont_test.fq Elysia_reads.paf.gz \
> Elysia_reads.gfa
minimap2
Link to section 'Introduction' of 'minimap2' Introduction
Minimap2
is a versatile pairwise aligner for genomic and spliced nucleotide sequences.
For more information, please check its website: https://biocontainers.pro/tools/minimap2 and its home page on Github.
Link to section 'Versions' of 'minimap2' Versions
- 2.22
- 2.24
- 2.26
Link to section 'Commands' of 'minimap2' Commands
- minimap2
- paftools.js
- k8
Link to section 'Module' of 'minimap2' Module
You can load the modules by:
module load biocontainers
module load minimap2
Link to section 'Example job' of 'minimap2' Example job
Using #!/bin/sh -l
as shebang in the slurm job script will cause the failure of some biocontainer modules. Please use #!/bin/bash
instead.
To run Minimap2 on our clusters:
#!/bin/bash
#SBATCH -A myallocation # Allocation name
#SBATCH -t 1:00:00
#SBATCH -N 1
#SBATCH -n 1
#SBATCH --job-name=minimap2
#SBATCH --mail-type=FAIL,BEGIN,END
#SBATCH --error=%x-%J-%u.err
#SBATCH --output=%x-%J-%u.out
module --force purge
ml biocontainers minimap2
minimap2 -ax sr Wuhan-Hu-1.fasta \
seq_1.fastq seq_2.fastq \
> aln.sam
minipolish
Link to section 'Introduction' of 'minipolish' Introduction
Minipolish is a tool for Racon polishing of miniasm assemblies.
Docker hub: https://hub.docker.com/r/staphb/minipolish
Home page: https://github.com/rrwick/Minipolish
Link to section 'Versions' of 'minipolish' Versions
- 0.1.3
Link to section 'Commands' of 'minipolish' Commands
- minipolish
Link to section 'Module' of 'minipolish' Module
You can load the modules by:
module load biocontainers
module load minipolish
Link to section 'Example job' of 'minipolish' Example job
Using #!/bin/sh -l
as shebang in the slurm job script will cause the failure of some biocontainer modules. Please use #!/bin/bash
instead.
To run minipolish on our clusters:
#!/bin/bash
#SBATCH -A myallocation # Allocation name
#SBATCH -t 1:00:00
#SBATCH -N 1
#SBATCH -n 8
#SBATCH --job-name=minipolish
#SBATCH --mail-type=FAIL,BEGIN,END
#SBATCH --error=%x-%J-%u.err
#SBATCH --output=%x-%J-%u.out
module --force purge
ml biocontainers minipolish
minipolish -t 8 long_reads.fastq.gz assembly.gfa > polished.gfa
miniprot
Link to section 'Introduction' of 'miniprot' Introduction
Miniprot aligns a protein sequence against a genome with affine gap penalty, splicing and frameshift. It is primarily intended for annotating protein-coding genes in a new species using known genes from other species. Miniprot is similar to GeneWise and Exonerate in functionality but it can map proteins to whole genomes and is much faster at the residue alignment step.
Home page: https://github.com/lh3/miniprot
Link to section 'Versions' of 'miniprot' Versions
- 0.3
- 0.7
Link to section 'Commands' of 'miniprot' Commands
- miniprot
Link to section 'Module' of 'miniprot' Module
You can load the modules by:
module load biocontainers
module load miniprot
Link to section 'Example job' of 'miniprot' Example job
Using #!/bin/sh -l
as shebang in the slurm job script will cause the failure of some biocontainer modules. Please use #!/bin/bash
instead.
To run miniprot on our clusters:
#!/bin/bash
#SBATCH -A myallocation # Allocation name
#SBATCH -t 1:00:00
#SBATCH -N 1
#SBATCH -n 1
#SBATCH --job-name=miniprot
#SBATCH --mail-type=FAIL,BEGIN,END
#SBATCH --error=%x-%J-%u.err
#SBATCH --output=%x-%J-%u.out
module --force purge
ml biocontainers miniprot
mirdeep2
Link to section 'Introduction' of 'mirdeep2' Introduction
miRDeep2
discovers active known or novel miRNAs from deep sequencing data (Solexa/Illumina, 454, ...).
For more information, please check its website: https://biocontainers.pro/tools/mirdeep2 and its home page on Github.
Link to section 'Versions' of 'mirdeep2' Versions
- 2.0.1.3
Link to section 'Commands' of 'mirdeep2' Commands
- bwa_sam_converter.pl
- clip_adapters.pl
- collapse_reads_md.pl
- convert_bowtie_output.pl
- excise_precursors_iterative_final.pl
- excise_precursors.pl
- extract_miRNAs.pl
- fastaparse.pl
- fastaselect.pl
- fastq2fasta.pl
- find_read_count.pl
- geo2fasta.pl
- get_mirdeep2_precursors.pl
- illumina_to_fasta.pl
- make_html2.pl
- make_html.pl
- mapper.pl
- mirdeep2bed.pl
- miRDeep2_core_algorithm.pl
- miRDeep2.pl
- parse_mappings.pl
- perform_controls.pl
- permute_structure.pl
- prepare_signature.pl
- quantifier.pl
- remove_white_space_in_id.pl
- rna2dna.pl
- samFLAGinfo.pl
- sam_reads_collapse.pl
- sanity_check_genome.pl
- sanity_check_mapping_file.pl
- sanity_check_mature_ref.pl
- sanity_check_reads_ready_file.pl
- select_for_randfold.pl
- survey.pl
Link to section 'Module' of 'mirdeep2' Module
You can load the modules by:
module load biocontainers
module load mirdeep2
Link to section 'Example job' of 'mirdeep2' Example job
Using #!/bin/sh -l
as shebang in the slurm job script will cause the failure of some biocontainer modules. Please use #!/bin/bash
instead.
To run miRDeep2 on our clusters:
#!/bin/bash
#SBATCH -A myallocation # Allocation name
#SBATCH -t 1:00:00
#SBATCH -N 1
#SBATCH -n 1
#SBATCH --job-name=mirdeep2
#SBATCH --mail-type=FAIL,BEGIN,END
#SBATCH --error=%x-%J-%u.err
#SBATCH --output=%x-%J-%u.out
module --force purge
ml biocontainers mirdeep2
miRDeep2.pl reads_collapsed.fa genome.fa reads_collapsed_vs_genome.arf \
miRBase_mmu_v14.fa miRBase_rno_v14.fa precursors_ref_this_species.fa \
-t Mouse 2>report.log
mirtop
Link to section 'Introduction' of 'mirtop' Introduction
Mirtop is a ommand line tool to annotate with a standard naming miRNAs e isomiRs.
BioContainers: https://biocontainers.pro/tools/mirtop
Home page: https://github.com/miRTop/mirtop
Link to section 'Versions' of 'mirtop' Versions
- 0.4.25
Link to section 'Commands' of 'mirtop' Commands
- mirtop
Link to section 'Module' of 'mirtop' Module
You can load the modules by:
module load biocontainers
module load mirtop
Link to section 'Example job' of 'mirtop' Example job
Using #!/bin/sh -l
as shebang in the slurm job script will cause the failure of some biocontainer modules. Please use #!/bin/bash
instead.
To run mirtop on our clusters:
#!/bin/bash
#SBATCH -A myallocation # Allocation name
#SBATCH -t 1:00:00
#SBATCH -N 1
#SBATCH -n 1
#SBATCH --job-name=mirtop
#SBATCH --mail-type=FAIL,BEGIN,END
#SBATCH --error=%x-%J-%u.err
#SBATCH --output=%x-%J-%u.out
module --force purge
ml biocontainers mirtop
mirtop gff --format prost --sps hsa
--hairpin examples/annotate/hairpin.fa \
--gtf examples/annotate/hsa.gff3 \
-o test_out \
examples/prost/prost.example.txt
mitofinder
Link to section 'Introduction' of 'mitofinder' Introduction
Mitofinder
is a pipeline to assemble mitochondrial genomes and annotate mitochondrial genes from trimmed read sequencing data.
For more information, please check its website: https://cloud.sylabs.io/library/remiallio/default/mitofinder and its home page on Github.
Link to section 'Versions' of 'mitofinder' Versions
- 1.4.1
Link to section 'Commands' of 'mitofinder' Commands
- mitofinder
Link to section 'Module' of 'mitofinder' Module
You can load the modules by:
module load biocontainers
module load mitofinder
Link to section 'Example job' of 'mitofinder' Example job
Using #!/bin/sh -l
as shebang in the slurm job script will cause the failure of some biocontainer modules. Please use #!/bin/bash
instead.
To run Mitofinder on our clusters:
#!/bin/bash
#SBATCH -A myallocation # Allocation name
#SBATCH -t 1:00:00
#SBATCH -N 1
#SBATCH -n 1
#SBATCH --job-name=mitofinder
#SBATCH --mail-type=FAIL,BEGIN,END
#SBATCH --error=%x-%J-%u.err
#SBATCH --output=%x-%J-%u.out
module --force purge
ml biocontainers mitofinder
mitofinder -j Aphaenogaster_megommata_SRR1303315 \
-1 Aphaenogaster_megommata_SRR1303315_R1_cleaned.fastq.gz \
-2 Aphaenogaster_megommata_SRR1303315_R2_cleaned.fastq.gz \
-r reference.gb -o 5 -p 5 -m 10
mlst
Link to section 'Introduction' of 'mlst' Introduction
Mlst is used to scan contig files against traditional PubMLST typing schemes.
Docker hub: https://hub.docker.com/r/staphb/mlst
Home page: https://github.com/tseemann/mlst
Link to section 'Versions' of 'mlst' Versions
- 2.22.0
- 2.23.0
Link to section 'Commands' of 'mlst' Commands
- mlst
Link to section 'Module' of 'mlst' Module
You can load the modules by:
module load biocontainers
module load mlst
Link to section 'Example job' of 'mlst' Example job
Using #!/bin/sh -l
as shebang in the slurm job script will cause the failure of some biocontainer modules. Please use #!/bin/bash
instead.
To run mlst on our clusters:
#!/bin/bash
#SBATCH -A myallocation # Allocation name
#SBATCH -t 1:00:00
#SBATCH -N 1
#SBATCH -n 1
#SBATCH --job-name=mlst
#SBATCH --mail-type=FAIL,BEGIN,END
#SBATCH --error=%x-%J-%u.err
#SBATCH --output=%x-%J-%u.out
module --force purge
ml biocontainers mlst
mlst contigs.fa
mlst genome.gbk.gz
mmseqs2
Link to section 'Introduction' of 'mmseqs2' Introduction
Mmseqs2
is a software suite to search and cluster huge protein and nucleotide sequence sets.
For more information, please check its website: https://biocontainers.pro/tools/mmseqs2 and its home page on Github.
Link to section 'Versions' of 'mmseqs2' Versions
- 13.45111
- 14.7e284
Link to section 'Commands' of 'mmseqs2' Commands
- mmseqs
Link to section 'Module' of 'mmseqs2' Module
You can load the modules by:
module load biocontainers
module load mmseqs2
Link to section 'Example job' of 'mmseqs2' Example job
Using #!/bin/sh -l
as shebang in the slurm job script will cause the failure of some biocontainer modules. Please use #!/bin/bash
instead.
To run Mmseqs2 on our clusters:
#!/bin/bash
#SBATCH -A myallocation # Allocation name
#SBATCH -t 1:00:00
#SBATCH -N 1
#SBATCH -n 1
#SBATCH --job-name=mmseqs2
#SBATCH --mail-type=FAIL,BEGIN,END
#SBATCH --error=%x-%J-%u.err
#SBATCH --output=%x-%J-%u.out
module --force purge
ml biocontainers mmseqs2
mmseqs createdb examples/DB.fasta targetDB
mmseqs createtaxdb targetDB tmp
mmseqs createindex targetDB tmp
mmseqs easy-taxonomy examples/QUERY.fasta targetDB alnRes tmp
mob_suite
Link to section 'Introduction' of 'mob_suite' Introduction
MOB-suite: Software tools for clustering, reconstruction and typing of plasmids from draft assemblies.
Docker hub: https://hub.docker.com/r/kbessonov/mob_suite
Home page: https://github.com/phac-nml/mob-suite
Link to section 'Versions' of 'mob_suite' Versions
- 3.0.3
Link to section 'Commands' of 'mob_suite' Commands
- mob_cluster
- mob_init
- mob_recon
- mob_typer
Link to section 'Module' of 'mob_suite' Module
You can load the modules by:
module load biocontainers
module load mob_suite
Link to section 'Example job' of 'mob_suite' Example job
Using #!/bin/sh -l
as shebang in the slurm job script will cause the failure of some biocontainer modules. Please use #!/bin/bash
instead.
To run mob_suite on our clusters:
#!/bin/bash
#SBATCH -A myallocation # Allocation name
#SBATCH -t 1:00:00
#SBATCH -N 1
#SBATCH -n 1
#SBATCH --job-name=mob_suite
#SBATCH --mail-type=FAIL,BEGIN,END
#SBATCH --error=%x-%J-%u.err
#SBATCH --output=%x-%J-%u.out
module --force purge
ml biocontainers mob_suite
modbam2bed
Link to section 'Introduction' of 'modbam2bed' Introduction
Modbam2bed is a program to aggregate modified base counts stored in a modified-base BAM file to a bedMethyl file.
Docker hub: https://hub.docker.com/r/zeunas/modbam2bed
Home page: https://github.com/epi2me-labs/modbam2bed
Link to section 'Versions' of 'modbam2bed' Versions
- 0.9.1
Link to section 'Commands' of 'modbam2bed' Commands
- modbam2bed
Link to section 'Module' of 'modbam2bed' Module
You can load the modules by:
module load biocontainers
module load modbam2bed
Link to section 'Example job' of 'modbam2bed' Example job
Using #!/bin/sh -l
as shebang in the slurm job script will cause the failure of some biocontainer modules. Please use #!/bin/bash
instead.
To run modbam2bed on our clusters:
#!/bin/bash
#SBATCH -A myallocation # Allocation name
#SBATCH -t 1:00:00
#SBATCH -N 1
#SBATCH -n 1
#SBATCH --job-name=modbam2bed
#SBATCH --mail-type=FAIL,BEGIN,END
#SBATCH --error=%x-%J-%u.err
#SBATCH --output=%x-%J-%u.out
module --force purge
ml biocontainers modbam2bed
modeltest-ng
Link to section 'Introduction' of 'modeltest-ng' Introduction
ModelTest-NG is a tool for selecting the best-fit model of evolution for DNA and protein alignments. ModelTest-NG supersedes jModelTest and ProtTest in one single tool, with graphical and command console interfaces.
BioContainers: https://biocontainers.pro/tools/modeltest-ng
Home page: https://github.com/ddarriba/modeltest
Link to section 'Versions' of 'modeltest-ng' Versions
- 0.1.7
Link to section 'Commands' of 'modeltest-ng' Commands
- modeltest-ng
- modeltest-ng-mpi
Link to section 'Module' of 'modeltest-ng' Module
You can load the modules by:
module load biocontainers
module load modeltest-ng
Link to section 'Example job' of 'modeltest-ng' Example job
Using #!/bin/sh -l
as shebang in the slurm job script will cause the failure of some biocontainer modules. Please use #!/bin/bash
instead.
To run modeltest-ng on our clusters:
#!/bin/bash
#SBATCH -A myallocation # Allocation name
#SBATCH -t 1:00:00
#SBATCH -N 1
#SBATCH -n 1
#SBATCH --job-name=modeltest-ng
#SBATCH --mail-type=FAIL,BEGIN,END
#SBATCH --error=%x-%J-%u.err
#SBATCH --output=%x-%J-%u.out
module --force purge
ml biocontainers modeltest-ng
momi
Link to section 'Introduction' of 'momi' Introduction
momi (MOran Models for Inference) is a Python package that computes the expected sample frequency spectrum (SFS), a statistic commonly used in population genetics, and uses it to fit demographic history.
Home page: https://momi2.readthedocs.io/en/latest/
Link to section 'Versions' of 'momi' Versions
- 2.1.19
Link to section 'Commands' of 'momi' Commands
- python
- python3
Link to section 'Module' of 'momi' Module
You can load the modules by:
module load biocontainers
module load momi
Link to section 'Interactive job' of 'momi' Interactive job
To run momi interactively on our clusters:
(base) UserID@bell-fe00:~ $ sinteractive -N1 -n12 -t4:00:00 -A myallocation
salloc: Granted job allocation 12345869
salloc: Waiting for resource configuration
salloc: Nodes bell-a008 are ready for job
(base) UserID@bell-a008:~ $ module load biocontainers momi
(base) UserID@bell-a008:~ $ python
Python 3.9.7 (default, Sep 16 2021, 13:09:58)
[GCC 7.5.0] :: Anaconda, Inc. on linux
Type "help", "copyright", "credits" or "license" for more information.
>>> import momi
>>> import logging
>>> logging.basicConfig(level=logging.INFO,
filename="tutorial.log")
>>> model = momi.DemographicModel(N_e=1.2e4, gen_time=29,
muts_per_gen=1.25e-8)
Link to section 'Batch job' of 'momi' Batch job
Using #!/bin/sh -l
as shebang in the slurm job script will cause the failure of some biocontainer modules. Please use #!/bin/bash
instead.
To run momi on our clusters:
#!/bin/bash
#SBATCH -A myallocation # Allocation name
#SBATCH -t 1:00:00
#SBATCH -N 1
#SBATCH -n 1
#SBATCH --job-name=momi
#SBATCH --mail-type=FAIL,BEGIN,END
#SBATCH --error=%x-%J-%u.err
#SBATCH --output=%x-%J-%u.out
module --force purge
ml biocontainers momi
python python.py
mothur
Mothur
is an open source software package for bioinformatics data processing. The package is frequently used in the analysis of DNA from uncultured microbes.
Detailed information about Mothur can be found here: https://mothur.org
Link to section 'Versions' of 'mothur' Versions
- 1.46.0
- 1.47.0
- 1.48.0
Link to section 'Commands' of 'mothur' Commands
- mothur
Link to section 'Module' of 'mothur' Module
You can load the modules by:
module load biocontainers
module load mothur/1.47.0
Link to section 'Interactive job' of 'mothur' Interactive job
To run mothur
interactively on our clusters:
(base) UserID@bell-fe00:~ $ sinteractive -N1 -n12 -t4:00:00 -A myallocation
salloc: Granted job allocation 12345869
salloc: Waiting for resource configuration
salloc: Nodes bell-a008 are ready for job
(base) UserID@bell-a008:~ $ module load biocontainers mothur/1.47.0
(base) UserID@bell-a008:~ $ mothur
Linux version
Using ReadLine,Boost,HDF5,GSL
mothur v.1.47.0
Last updated: 1/21/22
by
Patrick D. Schloss
Department of Microbiology & Immunology
University of Michigan
http://www.mothur.org
When using, please cite:
Schloss, P.D., et al., Introducing mothur: Open-source, platform-independent, community-supported software for describing and comparing microbial communities. Appl Environ Microbiol, 2009. 75(23):7537-41.
Distributed under the GNU General Public License
Type 'help()' for information on the commands that are available
For questions and analysis support, please visit our forum at https://forum.mothur.org
Type 'quit()' to exit program
[NOTE]: Setting random seed to 19760620.
Interactive Mode
mothur > align.seqs(help)
mothur > quit()
Link to section 'Batch job' of 'mothur' Batch job
Using #!/bin/sh -l
as shebang in the slurm job script will cause the failure of some biocontainer modules. Please use #!/bin/bash
instead.
To submit a sbatch job on our clusters:
#!/bin/bash
#SBATCH -A myallocation # Allocation name
#SBATCH -t 10:00:00
#SBATCH -N 1
#SBATCH -n 24
#SBATCH --job-name=mothur
#SBATCH --mail-type=FAIL,BEGIN,END
#SBATCH --error=%x-%J-%u.err
#SBATCH --output=%x-%J-%u.out
module --force purge
ml biocontainers mothur/1.47.0
mothur batch_file
motus
Link to section 'Introduction' of 'motus' Introduction
The mOTU profiler is a computational tool that estimates relative taxonomic abundance of known and currently unknown microbial community members using metagenomic shotgun sequencing data.
Home page: https://github.com/motu-tool/mOTUs
Link to section 'Versions' of 'motus' Versions
- 3.0.3
Link to section 'Commands' of 'motus' Commands
- motus
Link to section 'Module' of 'motus' Module
You can load the modules by:
module load biocontainers
module load motus
Link to section 'Example job' of 'motus' Example job
Using #!/bin/sh -l
as shebang in the slurm job script will cause the failure of some biocontainer modules. Please use #!/bin/bash
instead.
To run motus on our clusters:
#!/bin/bash
#SBATCH -A myallocation # Allocation name
#SBATCH -t 1:00:00
#SBATCH -N 1
#SBATCH -n 1
#SBATCH --job-name=motus
#SBATCH --mail-type=FAIL,BEGIN,END
#SBATCH --error=%x-%J-%u.err
#SBATCH --output=%x-%J-%u.out
module --force purge
ml biocontainers motus
mrbayes
Link to section 'Introduction' of 'mrbayes' Introduction
MrBayes
is a program for Bayesian inference and model choice across a wide range of phylogenetic and evolutionary models. MrBayes uses Markov chain Monte Carlo (MCMC) methods to estimate the posterior distribution of model parameters.
MrBayes is available both in a serial version ('mb') and in a parallel version ('mb-mpi') that uses MPI instructions to distribute computations across several processors or processor cores. The serial version does not support multi-threading, which means that you will not be able to utilize more than one core on a multi-core machine for a single MrBayes analysis. If you want to utilize all cores,you need to run the MPI version of MrBayes.
Note: 'mb-mpi' in this version of the container does not run across multiple nodes (only within a node). This is a bug in the container (upstream).
For more information, please check its website: https://biocontainers.pro/tools/mrbayes and its home page: http://mrbayes.net.
Link to section 'Versions' of 'mrbayes' Versions
- 3.2.7
Link to section 'Commands' of 'mrbayes' Commands
- mb
- mb-mpi
- mpirun
- mpiexec
Link to section 'Module' of 'mrbayes' Module
You can load the modules by:
module load biocontainers
module load mrbayes
Link to section 'Example job' of 'mrbayes' Example job
Using #!/bin/sh -l
as shebang in the slurm job script will cause the failure of some biocontainer modules. Please use #!/bin/bash
instead.
To run MrBayes on our clusters:
#!/bin/bash
#SBATCH -A myallocation # Allocation name
#SBATCH -t 1:00:00
#SBATCH -N 1
#SBATCH -n 1
#SBATCH --job-name=mrbayes
#SBATCH --mail-type=FAIL,BEGIN,END
#SBATCH --error=%x-%J-%u.err
#SBATCH --output=%x-%J-%u.out
module --force purge
ml biocontainers mrbayes
multiqc
Link to section 'Introduction' of 'multiqc' Introduction
Multiqc
is a reporting tool that parses summary statistics from results and log files generated by other bioinformatics tools.
For more information, please check its website: https://biocontainers.pro/tools/multiqc and its home page: https://multiqc.info.
Link to section 'Versions' of 'multiqc' Versions
- 1.11
Link to section 'Commands' of 'multiqc' Commands
- multiqc
Link to section 'Module' of 'multiqc' Module
You can load the modules by:
module load biocontainers
module load multiqc
Link to section 'Example job' of 'multiqc' Example job
Using #!/bin/sh -l
as shebang in the slurm job script will cause the failure of some biocontainer modules. Please use #!/bin/bash
instead.
To run Multiqc on our clusters:
#!/bin/bash
#SBATCH -A myallocation # Allocation name
#SBATCH -t 1:00:00
#SBATCH -N 1
#SBATCH -n 1
#SBATCH --job-name=multiqc
#SBATCH --mail-type=FAIL,BEGIN,END
#SBATCH --error=%x-%J-%u.err
#SBATCH --output=%x-%J-%u.out
module --force purge
ml biocontainers multiqc
multiqc fastqc_out -o multiqc_out
mummer4
Link to section 'Introduction' of 'mummer4' Introduction
Mummer4
is a versatile alignment tool for DNA and protein sequences.
For more information, please check its website: https://biocontainers.pro/tools/mummer4 and its home page on Github.
Link to section 'Versions' of 'mummer4' Versions
- 4.0.0rc1-pl5262
Link to section 'Commands' of 'mummer4' Commands
- annotate
- combineMUMs
- delta-filter
- delta2vcf
- dnadiff
- exact-tandems
- mummer
- mummerplot
- nucmer
- promer
- repeat-match
- show-aligns
- show-coords
- show-diff
- show-snps
- show-tiling
Link to section 'Module' of 'mummer4' Module
You can load the modules by:
module load biocontainers
module load mummer4
Link to section 'Example job' of 'mummer4' Example job
Using #!/bin/sh -l
as shebang in the slurm job script will cause the failure of some biocontainer modules. Please use #!/bin/bash
instead.
To run Mummer4 on our clusters:
#!/bin/bash
#SBATCH -A myallocation # Allocation name
#SBATCH -t 1:00:00
#SBATCH -N 1
#SBATCH -n 1
#SBATCH --job-name=mummer4
#SBATCH --mail-type=FAIL,BEGIN,END
#SBATCH --error=%x-%J-%u.err
#SBATCH --output=%x-%J-%u.out
module --force purge
ml biocontainers mummer4
mummer -mum -b -c H_pylori26695_Eslice.fasta H_pyloriJ99_Eslice.fasta > mummer.mums
muscle
Link to section 'Introduction' of 'muscle' Introduction
Muscle
is a modified progressive alignment algorithm which has comparable accuracy to MAFFT, but faster performance.
For more information, please check its website: https://biocontainers.pro/tools/muscle and its home page: http://www.drive5.com/muscle/muscle_userguide3.8.html.
Link to section 'Versions' of 'muscle' Versions
- 3.8.1551
- 5.1
Link to section 'Versions' of 'muscle' Versions
- 3.8.1551
- 5.1
Link to section 'Commands' of 'muscle' Commands
- muscle
Link to section 'Module' of 'muscle' Module
You can load the modules by:
module load biocontainers
module load muscle
Link to section 'Example job' of 'muscle' Example job
Using #!/bin/sh -l
as shebang in the slurm job script will cause the failure of some biocontainer modules. Please use #!/bin/bash
instead.
To run Muscle on our clusters:
#!/bin/bash
#SBATCH -A myallocation # Allocation name
#SBATCH -t 1:00:00
#SBATCH -N 1
#SBATCH -n 1
#SBATCH --job-name=muscle
#SBATCH --mail-type=FAIL,BEGIN,END
#SBATCH --error=%x-%J-%u.err
#SBATCH --output=%x-%J-%u.out
module --force purge
ml biocontainers muscle
muscle -align seqs2.fasta -output seqs.afa
mutmap
Link to section 'Introduction' of 'mutmap' Introduction
MutMap is a powerful and efficient method to identify agronomically important loci in crop plants.
BioContainers: https://biocontainers.pro/tools/mutmap
Home page: https://github.com/YuSugihara/MutMap#What-is-MutMap
Link to section 'Versions' of 'mutmap' Versions
- 2.3.3
Link to section 'Commands' of 'mutmap' Commands
- mutmap
- mutplot
Link to section 'Module' of 'mutmap' Module
You can load the modules by:
module load biocontainers
module load mutmap
Link to section 'Example job' of 'mutmap' Example job
Using #!/bin/sh -l
as shebang in the slurm job script will cause the failure of some biocontainer modules. Please use #!/bin/bash
instead.
To run mutmap on our clusters:
#!/bin/bash
#SBATCH -A myallocation # Allocation name
#SBATCH -t 1:00:00
#SBATCH -N 1
#SBATCH -n 1
#SBATCH --job-name=mutmap
#SBATCH --mail-type=FAIL,BEGIN,END
#SBATCH --error=%x-%J-%u.err
#SBATCH --output=%x-%J-%u.out
module --force purge
ml biocontainers mutmap
mykrobe
Link to section 'Introduction' of 'mykrobe' Introduction
Mykrobe analyses the whole genome of a bacterial sample, all within a couple of minutes, and predicts which drugs the infection is resistant to.
Docker hub: https://hub.docker.com/r/staphb/mykrobe
Home page: https://github.com/Mykrobe-tools/mykrobe
Link to section 'Versions' of 'mykrobe' Versions
- 0.11.0
Link to section 'Commands' of 'mykrobe' Commands
- mykrobe
Link to section 'Module' of 'mykrobe' Module
You can load the modules by:
module load biocontainers
module load mykrobe
Link to section 'Example job' of 'mykrobe' Example job
Using #!/bin/sh -l
as shebang in the slurm job script will cause the failure of some biocontainer modules. Please use #!/bin/bash
instead.
To run mykrobe on our clusters:
#!/bin/bash
#SBATCH -A myallocation # Allocation name
#SBATCH -t 1:00:00
#SBATCH -N 1
#SBATCH -n 1
#SBATCH --job-name=mykrobe
#SBATCH --mail-type=FAIL,BEGIN,END
#SBATCH --error=%x-%J-%u.err
#SBATCH --output=%x-%J-%u.out
module --force purge
ml biocontainers mykrobe
n50
Link to section 'Introduction' of 'n50' Introduction
N50 is a command line tool to calculate assembly metrices.
BioContainers: https://biocontainers.pro/tools/n50
Home page: https://github.com/quadram-institute-bioscience/seqfu/wiki/n50
Link to section 'Versions' of 'n50' Versions
- 1.5.6
Link to section 'Commands' of 'n50' Commands
- n50
Link to section 'Module' of 'n50' Module
You can load the modules by:
module load biocontainers
module load n50
Link to section 'Example job' of 'n50' Example job
Using #!/bin/sh -l
as shebang in the slurm job script will cause the failure of some biocontainer modules. Please use #!/bin/bash
instead.
To run n50 on our clusters:
#!/bin/bash
#SBATCH -A myallocation # Allocation name
#SBATCH -t 1:00:00
#SBATCH -N 1
#SBATCH -n 1
#SBATCH --job-name=n50
#SBATCH --mail-type=FAIL,BEGIN,END
#SBATCH --error=%x-%J-%u.err
#SBATCH --output=%x-%J-%u.out
module --force purge
ml biocontainers n50
nanofilt
Link to section 'Introduction' of 'nanofilt' Introduction
Nanofilt
is a tool for filtering and trimming of Oxford Nanopore Sequencing data.
For more information, please check its website: https://biocontainers.pro/tools/nanofilt and its home page on Github.
Link to section 'Versions' of 'nanofilt' Versions
- 2.8.0
Link to section 'Commands' of 'nanofilt' Commands
- NanoFilt
Link to section 'Module' of 'nanofilt' Module
You can load the modules by:
module load biocontainers
module load nanofilt
Link to section 'Example job' of 'nanofilt' Example job
Using #!/bin/sh -l
as shebang in the slurm job script will cause the failure of some biocontainer modules. Please use #!/bin/bash
instead.
To run Nanofilt on our clusters:
#!/bin/bash
#SBATCH -A myallocation # Allocation name
#SBATCH -t 1:00:00
#SBATCH -N 1
#SBATCH -n 1
#SBATCH --job-name=nanofilt
#SBATCH --mail-type=FAIL,BEGIN,END
#SBATCH --error=%x-%J-%u.err
#SBATCH --output=%x-%J-%u.out
module --force purge
ml biocontainers nanofilt
NanoFilt -q 12 --headcrop 75 reads.fastq | gzip > trimmed-reads.fastq.gz
nanolyse
Link to section 'Introduction' of 'nanolyse' Introduction
Nanolyse
is a tool to remove reads mapping to the lambda phage genome from a fastq file.
For more information, please check its website: https://biocontainers.pro/tools/nanolyse and its home page on Github.
Link to section 'Versions' of 'nanolyse' Versions
- 1.2.0
Link to section 'Commands' of 'nanolyse' Commands
- NanoLyse
Link to section 'Module' of 'nanolyse' Module
You can load the modules by:
module load biocontainers
module load nanolyse
Link to section 'Example job' of 'nanolyse' Example job
Using #!/bin/sh -l
as shebang in the slurm job script will cause the failure of some biocontainer modules. Please use #!/bin/bash
instead.
To run Nanolyse on our clusters:
#!/bin/bash
#SBATCH -A myallocation # Allocation name
#SBATCH -t 1:00:00
#SBATCH -N 1
#SBATCH -n 1
#SBATCH --job-name=nanolyse
#SBATCH --mail-type=FAIL,BEGIN,END
#SBATCH --error=%x-%J-%u.err
#SBATCH --output=%x-%J-%u.out
module --force purge
ml biocontainers nanolyse
gunzip -c reads.fastq.gz | NanoLyse | gzip > reads_without_lambda.fastq.gz
nanoplot
Link to section 'Introduction' of 'nanoplot' Introduction
Nanoplot
is a plotting tool for long read sequencing data and alignments.
For more information, please check its website: https://biocontainers.pro/tools/nanoplot and its home page on Github.
Link to section 'Versions' of 'nanoplot' Versions
- 1.39.0
Link to section 'Commands' of 'nanoplot' Commands
- NanoPlot
Link to section 'Module' of 'nanoplot' Module
You can load the modules by:
module load biocontainers
module load nanoplot
Link to section 'Example job' of 'nanoplot' Example job
Using #!/bin/sh -l
as shebang in the slurm job script will cause the failure of some biocontainer modules. Please use #!/bin/bash
instead.
To run Nanoplot on our clusters:
#!/bin/bash
#SBATCH -A myallocation # Allocation name
#SBATCH -t 1:00:00
#SBATCH -N 1
#SBATCH -n 12
#SBATCH --job-name=nanoplot
#SBATCH --mail-type=FAIL,BEGIN,END
#SBATCH --error=%x-%J-%u.err
#SBATCH --output=%x-%J-%u.out
module --force purge
ml biocontainers nanoplot
NanoPlot --summary sequencing_summary.txt --loglength -o summary-plots-log-transformed
NanoPlot -t 2 --fastq reads1.fastq.gz reads2.fastq.gz --maxlength 40000 --plots dot --legacy hex
NanoPlot -t 12 --color yellow --bam alignment1.bam alignment2.bam alignment3.bam --downsample 10000 -o bamplots_downsampled
nanopolish
Link to section 'Introduction' of 'nanopolish' Introduction
Nanopolish
is a software package for signal-level analysis of Oxford Nanopore sequencing data.
For more information, please check its website: https://biocontainers.pro/tools/nanopolish and its home page on Github.
Link to section 'Versions' of 'nanopolish' Versions
- 0.13.2
- 0.14.0
Link to section 'Commands' of 'nanopolish' Commands
- nanopolish
Link to section 'Module' of 'nanopolish' Module
You can load the modules by:
module load biocontainers
module load nanopolish
Link to section 'Example job' of 'nanopolish' Example job
Using #!/bin/sh -l
as shebang in the slurm job script will cause the failure of some biocontainer modules. Please use #!/bin/bash
instead.
To run Nanopolish on our clusters:
#!/bin/bash
#SBATCH -A myallocation # Allocation name
#SBATCH -t 1:00:00
#SBATCH -N 1
#SBATCH -n 1
#SBATCH --job-name=nanopolish
#SBATCH --mail-type=FAIL,BEGIN,END
#SBATCH --error=%x-%J-%u.err
#SBATCH --output=%x-%J-%u.out
module --force purge
ml biocontainers nanopolish
nanopolish index -d fast5_files/ reads.fasta
nanopolish variants --consensus \
-o polished.vcf -w "tig00000001:200000-202000" \
-r reads.fasta -b reads.sorted.bam -g draft.fa
ncbi-amrfinderplus
Link to section 'Introduction' of 'ncbi-amrfinderplus' Introduction
Ncbi-amrfinderplus
and the accompanying database identify acquired antimicrobial resistance genes in bacterial protein and/or assembled nucleotide sequences as well as known resistance-associated point mutations for several taxa.
BioContainers: https://biocontainers.pro/tools/ncbi-amrfinderplus
Home page: https://github.com/ncbi/amr
Link to section 'Versions' of 'ncbi-amrfinderplus' Versions
- 3.10.30
- 3.10.42
Link to section 'Commands' of 'ncbi-amrfinderplus' Commands
- amrfinder
Link to section 'Module' of 'ncbi-amrfinderplus' Module
You can load the modules by:
module load biocontainers
module load ncbi-amrfinderplus
AMRFinderPlus database has been setup for users. Users can check the database version by amrfinder -V
. RCAC will keep updating database for users. If you notice our database is out of date, you can contact us to update the database.
Link to section 'Example job' of 'ncbi-amrfinderplus' Example job
Using #!/bin/sh -l
as shebang in the slurm job script will cause the failure of some biocontainer modules. Please use #!/bin/bash
instead.
To run ncbi-amrfinderplus on our clusters:
#!/bin/bash
#SBATCH -A myallocation # Allocation name
#SBATCH -t 1:00:00
#SBATCH -N 1
#SBATCH -n 1
#SBATCH --job-name=ncbi-amrfinderplus
#SBATCH --mail-type=FAIL,BEGIN,END
#SBATCH --error=%x-%J-%u.err
#SBATCH --output=%x-%J-%u.out
module --force purge
ml biocontainers ncbi-amrfinderplus
# Protein AMRFinder with no genomic coordinates
amrfinder -p test_prot.fa
# Translated nucleotide AMRFinder (will not use HMMs)
amrfinder -n test_dna.fa
# Protein AMRFinder using GFF to get genomic coordinates and 'plus' genes
amrfinder -p test_prot.fa -g test_prot.gff --plus
# Protein AMRFinder with Escherichia protein point mutations
amrfinder -p test_prot.fa -O Escherichia
# Full AMRFinderPlus search combining results
amrfinder -p test_prot.fa -g test_prot.gff -n test_dna.fa -O Escherichia --plus
ncbi-datasets
Link to section 'Introduction' of 'ncbi-datasets' Introduction
NCBI Datasets is a new resource that lets you easily gather data from across NCBI databases. You can use it to find and download sequence, annotation, and metadata for genes and genomes using our command-line interface (CLI) tools or NCBI Datasets web interface.
Docker hub: https://hub.docker.com/r/staphb/ncbi-datasets
Home page: https://github.com/ncbi/datasets
Link to section 'Versions' of 'ncbi-datasets' Versions
- 14.3.0
Link to section 'Commands' of 'ncbi-datasets' Commands
- datasets
- dataformat
Link to section 'Module' of 'ncbi-datasets' Module
You can load the modules by:
module load biocontainers
module load ncbi-datasets
Link to section 'Example job' of 'ncbi-datasets' Example job
Using #!/bin/sh -l
as shebang in the slurm job script will cause the failure of some biocontainer modules. Please use #!/bin/bash
instead.
To run ncbi-datasets on our clusters:
#!/bin/bash
#SBATCH -A myallocation # Allocation name
#SBATCH -t 1:00:00
#SBATCH -N 1
#SBATCH -n 1
#SBATCH --job-name=ncbi-datasets
#SBATCH --mail-type=FAIL,BEGIN,END
#SBATCH --error=%x-%J-%u.err
#SBATCH --output=%x-%J-%u.out
module --force purge
ml biocontainers ncbi-datasets
ncbi-genome-download
Link to section 'Introduction' of 'ncbi-genome-download' Introduction
Ncbi-genome-download
is a script to download genomes from the NCBI FTP servers.
For more information, please check its website: https://biocontainers.pro/tools/ncbi-genome-download and its home page on Github.
Link to section 'Versions' of 'ncbi-genome-download' Versions
- 0.3.1
Link to section 'Commands' of 'ncbi-genome-download' Commands
- ncbi-genome-download
Link to section 'Module' of 'ncbi-genome-download' Module
You can load the modules by:
module load biocontainers
module load ncbi-genome-download
Link to section 'Example job' of 'ncbi-genome-download' Example job
Using #!/bin/sh -l
as shebang in the slurm job script will cause the failure of some biocontainer modules. Please use #!/bin/bash
instead.
To run Ncbi-genome-download on our clusters:
#!/bin/bash
#SBATCH -A myallocation # Allocation name
#SBATCH -t 1:00:00
#SBATCH -N 1
#SBATCH -n 4
#SBATCH --job-name=ncbi-genome-download
#SBATCH --mail-type=FAIL,BEGIN,END
#SBATCH --error=%x-%J-%u.err
#SBATCH --output=%x-%J-%u.out
module --force purge
ml biocontainers ncbi-genome-download
ncbi-genome-download bacteria,viral --parallel 4
ncbi-genome-download --genera "Streptomyces coelicolor,Escherichia coli" bacteria
ncbi-genome-download --species-taxids 562 bacteria
ncbi-table2asn
Link to section 'Introduction' of 'ncbi-table2asn' Introduction
table2asn is a command-line program that creates sequence records for submission to GenBank. It uses many of the same functions as Genome Workbench but is driven generally by data files, and the records it produces do not necessarily require additional manual editing before submission to GenBank.
Docker hub: https://hub.docker.com/r/staphb/ncbi-table2asn
Home page: https://www.ncbi.nlm.nih.gov/genbank/table2asn/
Link to section 'Versions' of 'ncbi-table2asn' Versions
- 1.26.678
Link to section 'Commands' of 'ncbi-table2asn' Commands
- table2asn
Link to section 'Module' of 'ncbi-table2asn' Module
You can load the modules by:
module load biocontainers
module load ncbi-table2asn
Link to section 'Example job' of 'ncbi-table2asn' Example job
Using #!/bin/sh -l
as shebang in the slurm job script will cause the failure of some biocontainer modules. Please use #!/bin/bash
instead.
To run ncbi-table2asn on our clusters:
#!/bin/bash
#SBATCH -A myallocation # Allocation name
#SBATCH -t 1:00:00
#SBATCH -N 1
#SBATCH -n 1
#SBATCH --job-name=ncbi-table2asn
#SBATCH --mail-type=FAIL,BEGIN,END
#SBATCH --error=%x-%J-%u.err
#SBATCH --output=%x-%J-%u.out
module --force purge
ml biocontainers ncbi-table2asn
neusomatic
Link to section 'Introduction' of 'neusomatic' Introduction
NeuSomatic is based on deep convolutional neural networks for accurate somatic mutation detection. With properly trained models, it can robustly perform across sequencing platforms, strategies, and conditions. NeuSomatic summarizes and augments sequence alignments in a novel way and incorporates multi-dimensional features to capture variant signals effectively. It is not only a universal but also accurate somatic mutation detection method.
Docker hub: https://hub.docker.com/r/msahraeian/neusomatic/
Home page: https://github.com/bioinform/neusomatic
Link to section 'Versions' of 'neusomatic' Versions
- 0.2.1
Link to section 'Commands' of 'neusomatic' Commands
- call.py
- dataloader.py
- extract_postprocess_targets.py
- filter_candidates.py
- generate_dataset.py
- long_read_indelrealign.py
- merge_post_vcfs.py
- merge_tsvs.py
- network.py
- postprocess.py
- preprocess.py
- resolve_scores.py
- resolve_variants.py
- scan_alignments.py
- split_bed.py
- train.py
- utils.py
Link to section 'Module' of 'neusomatic' Module
You can load the modules by:
module load biocontainers
module load neusomatic
Link to section 'Example job' of 'neusomatic' Example job
Using #!/bin/sh -l
as shebang in the slurm job script will cause the failure of some biocontainer modules. Please use #!/bin/bash
instead.
To run neusomatic on our clusters:
#!/bin/bash
#SBATCH -A myallocation # Allocation name
#SBATCH -t 1:00:00
#SBATCH -N 1
#SBATCH -n 1
#SBATCH --job-name=neusomatic
#SBATCH --mail-type=FAIL,BEGIN,END
#SBATCH --error=%x-%J-%u.err
#SBATCH --output=%x-%J-%u.out
module --force purge
ml biocontainers neusomatic
nextalign
Link to section 'Introduction' of 'nextalign' Introduction
Nextalign
is a viral genome sequence alignment tool for command line.
Docker hub: https://hub.docker.com/r/nextstrain/nextalign and its home page: https://docs.nextstrain.org/projects/nextclade/en/stable/user/nextalign-cli.html.
Link to section 'Versions' of 'nextalign' Versions
- 1.10.3
Link to section 'Commands' of 'nextalign' Commands
- nextalign
Link to section 'Module' of 'nextalign' Module
You can load the modules by:
module load biocontainers
module load nextalign
Link to section 'Example job' of 'nextalign' Example job
Using #!/bin/sh -l
as shebang in the slurm job script will cause the failure of some biocontainer modules. Please use #!/bin/bash
instead.
To run Nextalign on our clusters:
#!/bin/bash
#SBATCH -A myallocation # Allocation name
#SBATCH -t 1:00:00
#SBATCH -N 1
#SBATCH -n 1
#SBATCH --job-name=nextalign
#SBATCH --mail-type=FAIL,BEGIN,END
#SBATCH --error=%x-%J-%u.err
#SBATCH --output=%x-%J-%u.out
module --force purge
ml biocontainers nextalign
nextalign \
--sequences data/sars-cov-2/sequences.fasta \
--reference data/sars-cov-2/reference.fasta \
--genemap data/sars-cov-2/genemap.gff \
--genes E,M,N,ORF1a,ORF1b,ORF3a,ORF6,ORF7a,ORF7b,ORF8,ORF9b,S \
--output-dir output/ \
--output-basename nextalign
nextclade
Link to section 'Introduction' of 'nextclade' Introduction
Nextclade
is a tool that identifies differences between your sequences and a reference sequence, uses these differences to assign your sequences to clades, and reports potential sequence quality issues in your data.
Docker hub: https://hub.docker.com/r/nextstrain/nextclade and its home page: https://docs.nextstrain.org/projects/nextclade/en/stable/user/nextclade-cli.html.
Link to section 'Versions' of 'nextclade' Versions
- 1.10.3
Link to section 'Commands' of 'nextclade' Commands
- nextclade
Link to section 'Module' of 'nextclade' Module
You can load the modules by:
module load biocontainers
module load nextclade
Link to section 'Example job' of 'nextclade' Example job
Using #!/bin/sh -l
as shebang in the slurm job script will cause the failure of some biocontainer modules. Please use #!/bin/bash
instead.
To run Nextclade on our clusters:
#!/bin/bash
#SBATCH -A myallocation # Allocation name
#SBATCH -t 1:00:00
#SBATCH -N 1
#SBATCH -n 1
#SBATCH --job-name=nextclade
#SBATCH --mail-type=FAIL,BEGIN,END
#SBATCH --error=%x-%J-%u.err
#SBATCH --output=%x-%J-%u.out
module --force purge
ml biocontainers nextclade
mkdir -p data
nextclade dataset get --name 'sars-cov-2' --output-dir 'data/sars-cov-2'
nextclade \
--in-order \
--input-fasta data/sars-cov-2/sequences.fasta \
--input-dataset data/sars-cov-2 \
--output-tsv output/nextclade.tsv \
--output-tree output/nextclade.auspice.json \
--output-dir output/ \
--output-basename nextclade
nextflow
Link to section 'Introduction' of 'nextflow' Introduction
Nextflow
is a bioinformatics workflow manager that enables the development of portable and reproducible workflows.
For more information, please check its website: https://biocontainers.pro/tools/nextflow and its home page on Github.
Link to section 'Versions' of 'nextflow' Versions
- 21.10.0
Link to section 'Commands' of 'nextflow' Commands
- nextflow
Link to section 'Module' of 'nextflow' Module
You can load the modules by:
module load biocontainers
module load nextflow
Link to section 'Example job' of 'nextflow' Example job
Using #!/bin/sh -l
as shebang in the slurm job script will cause the failure of some biocontainer modules. Please use #!/bin/bash
instead.
To run Nextflow on our clusters:
#!/bin/bash
#SBATCH -A myallocation # Allocation name
#SBATCH -t 1:00:00
#SBATCH -N 1
#SBATCH -n 1
#SBATCH --job-name=nextflow
#SBATCH --mail-type=FAIL,BEGIN,END
#SBATCH --error=%x-%J-%u.err
#SBATCH --output=%x-%J-%u.out
module --force purge
ml biocontainers nextflow
ngs-bits
Link to section 'Introduction' of 'ngs-bits' Introduction
Ngs-bits
- Short-read sequencing tools.
For more information, please check its website: https://biocontainers.pro/tools/ngs-bits and its home page on Github.
Link to section 'Versions' of 'ngs-bits' Versions
- 2022_04
Link to section 'Commands' of 'ngs-bits' Commands
- SampleAncestry
- SampleDiff
- SampleGender
- SampleOverview
- SampleSimilarity
- SeqPurge
- CnvHunter
- RohHunter
- UpdHunter
- CfDnaQC
- MappingQC
- NGSDImportQC
- ReadQC
- SomaticQC
- VariantQC
- TrioMaternalContamination
- BamCleanHaloplex
- BamClipOverlap
- BamDownsample
- BamFilter
- BamToFastq
- BedAdd
- BedAnnotateFreq
- BedAnnotateFromBed
- BedAnnotateGC
- BedAnnotateGenes
- BedChunk
- BedCoverage
- BedExtend
- BedGeneOverlap
- BedHighCoverage
- BedInfo
- BedIntersect
- BedLiftOver
- BedLowCoverage
- BedMerge
- BedReadCount
- BedShrink
- BedSort
- BedSubtract
- BedToFasta
- BedpeAnnotateBreakpointDensity
- BedpeAnnotateCnvOverlap
- BedpeAnnotateCounts
- BedpeAnnotateFromBed
- BedpeFilter
- BedpeGeneAnnotation
- BedpeSort
- BedpeToBed
- FastqAddBarcode
- FastqConcat
- FastqConvert
- FastqDownsample
- FastqExtract
- FastqExtractBarcode
- FastqExtractUMI
- FastqFormat
- FastqList
- FastqMidParser
- FastqToFasta
- FastqTrim
- VcfAnnotateFromBed
- VcfAnnotateFromBigWig
- VcfAnnotateFromVcf
- VcfBreakMulti
- VcfCalculatePRS
- VcfCheck
- VcfExtractSamples
- VcfFilter
- VcfLeftNormalize
- VcfSort
- VcfStreamSort
- VcfToBedpe
- VcfToTsv
- SvFilterAnnotations
- NGSDExportGenes
- GenePrioritization
- GenesToApproved
- GenesToBed
- GraphStringDb
- PhenotypeSubtree
- PhenotypesToGenes
- PERsim
- FastaInfo
Link to section 'Module' of 'ngs-bits' Module
You can load the modules by:
module load biocontainers
module load ngs-bits
Link to section 'Example job' of 'ngs-bits' Example job
Using #!/bin/sh -l
as shebang in the slurm job script will cause the failure of some biocontainer modules. Please use #!/bin/bash
instead.
To run Ngs-bits on our clusters:
#!/bin/bash
#SBATCH -A myallocation # Allocation name
#SBATCH -t 1:00:00
#SBATCH -N 1
#SBATCH -n 1
#SBATCH --job-name=ngs-bits
#SBATCH --mail-type=FAIL,BEGIN,END
#SBATCH --error=%x-%J-%u.err
#SBATCH --output=%x-%J-%u.out
module --force purge
ml biocontainers ngs-bits
SeqPurge -in1 input1_1.fastq input2_1.fastq \
-in2 input2_2.fastq input2_2.fastq \
-out1 R1.fastq.gz -out2 R2.fastq.gz
ngsld
Link to section 'Introduction' of 'ngsld' Introduction
ngsLD is a program to estimate pairwise linkage disequilibrium (LD) taking the uncertainty of genotype's assignation into account. It does so by avoiding genotype calling and using genotype likelihoods or posterior probabilities.
Home page: https://github.com/fgvieira/ngsLD
Link to section 'Versions' of 'ngsld' Versions
- 1.1.1
Link to section 'Commands' of 'ngsld' Commands
- ngsLD
Link to section 'Module' of 'ngsld' Module
You can load the modules by:
module load biocontainers
module load ngsld
Link to section 'Example job' of 'ngsld' Example job
Using #!/bin/sh -l
as shebang in the slurm job script will cause the failure of some biocontainer modules. Please use #!/bin/bash
instead.
To run ngsld on our clusters:
#!/bin/bash
#SBATCH -A myallocation # Allocation name
#SBATCH -t 1:00:00
#SBATCH -N 1
#SBATCH -n 1
#SBATCH --job-name=ngsld
#SBATCH --mail-type=FAIL,BEGIN,END
#SBATCH --error=%x-%J-%u.err
#SBATCH --output=%x-%J-%u.out
module --force purge
ml biocontainers ngsld
ngsutils
Link to section 'Introduction' of 'ngsutils' Introduction
Ngsutils
is a suite of software tools for working with next-generation sequencing datasets.
For more information, please check its website: https://biocontainers.pro/tools/ngsutils and its home page: http://ngsutils.org.
Link to section 'Versions' of 'ngsutils' Versions
- 0.5.9
Link to section 'Commands' of 'ngsutils' Commands
- ngsutils
- bamutils
- bedutils
- fastqutils
- gtfutils
Link to section 'Module' of 'ngsutils' Module
You can load the modules by:
module load biocontainers
module load ngsutils
Link to section 'Example job' of 'ngsutils' Example job
Using #!/bin/sh -l
as shebang in the slurm job script will cause the failure of some biocontainer modules. Please use #!/bin/bash
instead.
To run Ngsutils on our clusters:
#!/bin/bash
#SBATCH -A myallocation # Allocation name
#SBATCH -t 1:00:00
#SBATCH -N 1
#SBATCH -n 1
#SBATCH --job-name=ngsutils
#SBATCH --mail-type=FAIL,BEGIN,END
#SBATCH --error=%x-%J-%u.err
#SBATCH --output=%x-%J-%u.out
module --force purge
ml biocontainers ngsutils
bamutils filter \
input.bam \
MQ10filtered.bam \
-mapped \
-noqcfail \
-gte MAPQ 10
bamutils stats \
-gtf genome.gtf MQ10filtered.bam \
> MQ10filtered_bamstats
orthofinder
OrthoFinder
: phylogenetic orthology inference for comparative genomics
Detailed usage can be found here: https://github.com/davidemms/OrthoFinder
Link to section 'Versions' of 'orthofinder' Versions
- 2.5.2
- 2.5.4
Link to section 'Commands' of 'orthofinder' Commands
- orthofinder
Link to section 'Module' of 'orthofinder' Module
You can load the modules by:
module load biocontainers
module load orthofinder/2.5.4
Link to section 'Example job' of 'orthofinder' Example job
Using #!/bin/sh -l
as shebang in the slurm job script will cause the failure of some biocontainer modules. Please use #!/bin/bash
instead.
To run orthofinder on our our clusters:
#!/bin/bash
#SBATCH -A myallocation # Allocation name
#SBATCH -t 20:00:00
#SBATCH -N 1
#SBATCH -n 24
#SBATCH --job-name=orthofinder
#SBATCH --mail-type=FAIL,BEGIN,END
#SBATCH --error=%x-%J-%u.err
#SBATCH --output=%x-%J-%u.out
module --force purge
ml biocontainers orthofinder/2.5.4
orthofinder -t 24 -f InputData -o output
paml
Link to section 'Introduction' of 'paml' Introduction
Paml
is a package of programs for phylogenetic analyses of DNA or protein sequences using maximum likelihood.
For more information, please check its website: https://biocontainers.pro/tools/paml and its home page: http://abacus.gene.ucl.ac.uk/software/paml.html.
Link to section 'Versions' of 'paml' Versions
- 4.9
Link to section 'Commands' of 'paml' Commands
- baseml
- basemlg
- chi2
- codeml
- evolver
- infinitesites
- mcmctree
- pamp
- yn00
Link to section 'Module' of 'paml' Module
You can load the modules by:
module load biocontainers
module load paml
Link to section 'Example job' of 'paml' Example job
Using #!/bin/sh -l
as shebang in the slurm job script will cause the failure of some biocontainer modules. Please use #!/bin/bash
instead.
To run Paml on our clusters:
#!/bin/bash
#SBATCH -A myallocation # Allocation name
#SBATCH -t 1:00:00
#SBATCH -N 1
#SBATCH -n 1
#SBATCH --job-name=paml
#SBATCH --mail-type=FAIL,BEGIN,END
#SBATCH --error=%x-%J-%u.err
#SBATCH --output=%x-%J-%u.out
module --force purge
ml biocontainers paml
panacota
Link to section 'Introduction' of 'panacota' Introduction
Panacota
is a software providing tools for large scale bacterial comparative genomics.
For more information, please check its website: https://biocontainers.pro/tools/panacota and its home page on Github.
Link to section 'Versions' of 'panacota' Versions
- 1.3.1
Link to section 'Commands' of 'panacota' Commands
- PanACoTA
Link to section 'Module' of 'panacota' Module
You can load the modules by:
module load biocontainers
module load panacota
Link to section 'Example job' of 'panacota' Example job
Using #!/bin/sh -l
as shebang in the slurm job script will cause the failure of some biocontainer modules. Please use #!/bin/bash
instead.
To run Panacota on our clusters:
#!/bin/bash
#SBATCH -A myallocation # Allocation name
#SBATCH -t 1:00:00
#SBATCH -N 1
#SBATCH -n 1
#SBATCH --job-name=panacota
#SBATCH --mail-type=FAIL,BEGIN,END
#SBATCH --error=%x-%J-%u.err
#SBATCH --output=%x-%J-%u.out
module --force purge
ml biocontainers panacota
PanACoTA annotate \
-d Examples/genomes_init \
-l Examples/input_files/list_genomes.lst \
-r Examples/2-res-QC -Q
panaroo
Link to section 'Introduction' of 'panaroo' Introduction
Panaroo is an updated pipeline for pangenome investigation.
BioContainers: https://biocontainers.pro/tools/panaroo
Home page: https://github.com/gtonkinhill/panaroo
Link to section 'Versions' of 'panaroo' Versions
- 1.2.10
Link to section 'Commands' of 'panaroo' Commands
- panaroo
- panaroo-extract-gene
- panaroo-filter-pa
- panaroo-fmg
- panaroo-gene-neighbourhood
- panaroo-img
- panaroo-integrate
- panaroo-merge
- panaroo-msa
- panaroo-plot-abundance
- panaroo-qc
- panaroo-spydrpick
Link to section 'Module' of 'panaroo' Module
You can load the modules by:
module load biocontainers
module load panaroo
Link to section 'Example job' of 'panaroo' Example job
Using #!/bin/sh -l
as shebang in the slurm job script will cause the failure of some biocontainer modules. Please use #!/bin/bash
instead.
To run panaroo on our clusters:
#!/bin/bash
#SBATCH -A myallocation # Allocation name
#SBATCH -t 1:00:00
#SBATCH -N 1
#SBATCH -n 1
#SBATCH --job-name=panaroo
#SBATCH --mail-type=FAIL,BEGIN,END
#SBATCH --error=%x-%J-%u.err
#SBATCH --output=%x-%J-%u.out
module --force purge
ml biocontainers panaroo
panaroo -i gff/*.gff -o results --clean-mode strict
pandaseq
Link to section 'Introduction' of 'pandaseq' Introduction
Pandaseq
is a program to align Illumina reads, optionally with PCR primers embedded in the sequence, and reconstruct an overlapping sequence.
Docker hub: https://hub.docker.com/r/pipecraft/pandaseq and its home page on Github.
Link to section 'Versions' of 'pandaseq' Versions
- 2.11
Link to section 'Commands' of 'pandaseq' Commands
- pandaseq
Link to section 'Module' of 'pandaseq' Module
You can load the modules by:
module load biocontainers
module load pandaseq
Link to section 'Example job' of 'pandaseq' Example job
Using #!/bin/sh -l
as shebang in the slurm job script will cause the failure of some biocontainer modules. Please use #!/bin/bash
instead.
To run Pandaseq on our clusters:
#!/bin/bash
#SBATCH -A myallocation # Allocation name
#SBATCH -t 1:00:00
#SBATCH -N 1
#SBATCH -n 1
#SBATCH --job-name=pandaseq
#SBATCH --mail-type=FAIL,BEGIN,END
#SBATCH --error=%x-%J-%u.err
#SBATCH --output=%x-%J-%u.out
module --force purge
ml biocontainers pandaseq
pandaseq -f SRR069027_1.fastq -r SRR069027_2.fastq
pandora
Link to section 'Introduction' of 'pandora' Introduction
Pandora is a tool for bacterial genome analysis using a pangenome reference graph (PanRG). It allows gene presence/absence detection and genotyping of SNPs, indels and longer variants in one or a number of samples.
BioContainers: https://biocontainers.pro/tools/pandora
Home page: https://github.com/rmcolq/pandora
Link to section 'Versions' of 'pandora' Versions
- 0.9.1
Link to section 'Commands' of 'pandora' Commands
- pandora
Link to section 'Module' of 'pandora' Module
You can load the modules by:
module load biocontainers
module load pandora
Link to section 'Example job' of 'pandora' Example job
Using #!/bin/sh -l
as shebang in the slurm job script will cause the failure of some biocontainer modules. Please use #!/bin/bash
instead.
To run pandora on our clusters:
#!/bin/bash
#SBATCH -A myallocation # Allocation name
#SBATCH -t 1:00:00
#SBATCH -N 1
#SBATCH -n 4
#SBATCH --job-name=pandora
#SBATCH --mail-type=FAIL,BEGIN,END
#SBATCH --error=%x-%J-%u.err
#SBATCH --output=%x-%J-%u.out
module --force purge
ml biocontainers pandora
pandora index -t 4 GC00006032.fa
pangolin
Link to section 'Introduction' of 'pangolin' Introduction
Pangolin
is a software package for assigning SARS-CoV-2 genome sequences to global lineages.
For more information, please check its website: https://biocontainers.pro/tools/pangolin and its home page on Github.
Link to section 'Versions' of 'pangolin' Versions
- 3.1.20
- 4.0.6
- 4.1.2
- 4.1.3
- 4.2
Link to section 'Commands' of 'pangolin' Commands
- pangolin
Link to section 'Module' of 'pangolin' Module
You can load the modules by:
module load biocontainers
module load pangolin
Link to section 'Example job' of 'pangolin' Example job
Using #!/bin/sh -l
as shebang in the slurm job script will cause the failure of some biocontainer modules. Please use #!/bin/bash
instead.
To run Pangolin on our clusters:
#!/bin/bash
#SBATCH -A myallocation # Allocation name
#SBATCH -t 1:00:00
#SBATCH -N 1
#SBATCH -n 1
#SBATCH --job-name=pangolin
#SBATCH --mail-type=FAIL,BEGIN,END
#SBATCH --error=%x-%J-%u.err
#SBATCH --output=%x-%J-%u.out
module --force purge
ml biocontainers pangolin
panphlan
Link to section 'Introduction' of 'panphlan' Introduction
PanPhlAn
(Pangenome-based Phylogenomic Analysis) is a strain-level metagenomic profiling tool for identifying the gene composition and in-vivo transcriptional activity of individual strains in metagenomic samples.
For more information, please check its home page: http://segatalab.cibio.unitn.it/tools/panphlan/.
Link to section 'Versions' of 'panphlan' Versions
- 3.1
Link to section 'Commands' of 'panphlan' Commands
- panphlan_download_pangenome.py
- panphlan_map.py
- panphlan_profiling.py
Link to section 'Module' of 'panphlan' Module
You can load the modules by:
module load biocontainers
module load panphlan
Link to section 'Example job' of 'panphlan' Example job
Using #!/bin/sh -l
as shebang in the slurm job script will cause the failure of some biocontainer modules. Please use #!/bin/bash
instead.
To run PanPhlAn on our clusters:
#!/bin/bash
#SBATCH -A myallocation # Allocation name
#SBATCH -t 1:00:00
#SBATCH -N 1
#SBATCH -n 1
#SBATCH --job-name=panphlan
#SBATCH --mail-type=FAIL,BEGIN,END
#SBATCH --error=%x-%J-%u.err
#SBATCH --output=%x-%J-%u.out
module --force purge
ml biocontainers panphlan
parabricks
Link to section 'Introduction' of 'parabricks' Introduction
NVIDIA's Clara Parabricks brings next generation sequencing to GPUs, accelerating an array of gold-standard tooling such as BWA-MEM, GATK4, Google's DeepVariant, and many more. Users can achieve a 30-60x acceleration and 99.99% accuracy for variant calling when comparing against CPU-only BWA-GATK4 pipelines, meaning a single server can process up to 60 whole genomes per day. These tools can be easily integrated into current pipelines with drop-in replacement commands to quickly bring speed and data-center scale to a range of applications including germline, somatic and RNA workflows.
NGC Container: https://catalog.ngc.nvidia.com/orgs/nvidia/teams/clara/containers/clara-parabricks Home page: https://docs.nvidia.com/clara/
Link to section 'Versions' of 'parabricks' Versions
- 4.0.0-1
Link to section 'Commands' of 'parabricks' Commands
- pbrun
Link to section 'Module' of 'parabricks' Module
You can load the modules by:
module load biocontainers
module load parabricks
Link to section 'Example job' of 'parabricks' Example job
Using #!/bin/sh -l
as shebang in the slurm job script will cause the failure of some biocontainer modules. Please use #!/bin/bash
instead.
As Clara Parabricks depends on Nvidia GPU, it is only deployed in Scholar, Gilbreth, and ACCESS Anvil.
To run Clara Parabricks on our clusters:
#!/bin/bash
#SBATCH -A myallocation # Allocation name
#SBATCH -t 1:00:00
#SBATCH -N 1
#SBATCH -n 1
#SBATCH --gpus=1
#SBATCH --job-name=parabricks
#SBATCH --mail-type=FAIL,BEGIN,END
#SBATCH --error=%x-%J-%u.err
#SBATCH --output=%x-%J-%u.out
module --force purge
ml biocontainers parabricks
pbrun haplotypecaller \
--ref FVZG01.1.fsa_nt \
--in-bam output.bam \
--out-variants variants.vcf
parallel-fastq-dump
Link to section 'Introduction' of 'parallel-fastq-dump' Introduction
Parallel-fastq-dump
is the parallel fastq-dump wrapper.
For more information, please check its website: https://biocontainers.pro/tools/parallel-fastq-dump and its home page on Github.
Link to section 'Versions' of 'parallel-fastq-dump' Versions
- 0.6.7
Link to section 'Commands' of 'parallel-fastq-dump' Commands
- parallel-fastq-dump
Link to section 'Module' of 'parallel-fastq-dump' Module
You can load the modules by:
module load biocontainers
module load parallel-fastq-dump
Link to section 'Example job' of 'parallel-fastq-dump' Example job
Using #!/bin/sh -l
as shebang in the slurm job script will cause the failure of some biocontainer modules. Please use #!/bin/bash
instead.
To run Parallel-fastq-dump on our clusters:
#!/bin/bash
#SBATCH -A myallocation # Allocation name
#SBATCH -t 1:00:00
#SBATCH -N 1
#SBATCH -n 4
#SBATCH --job-name=parallel-fastq-dump
#SBATCH --mail-type=FAIL,BEGIN,END
#SBATCH --error=%x-%J-%u.err
#SBATCH --output=%x-%J-%u.out
module --force purge
ml biocontainers parallel-fastq-dump
parallel-fastq-dump -s SRR11941281/SRR11941281.sra \
--split-files --threads 4 --gzip
parliament2
Link to section 'Introduction' of 'parliament2' Introduction
Parliament2 identifies structural variants in a given sample relative to a reference genome. These structural variants cover large deletion events that are called as Deletions of a region, Insertions of a sequence into a region, Duplications of a region, Inversions of a region, or Translocations between two regions in the genome.
Docker hub: https://hub.docker.com/r/dnanexus/parliament2
Home page: https://github.com/fritzsedlazeck/parliament2
Link to section 'Versions' of 'parliament2' Versions
- 0.1.11
Link to section 'Commands' of 'parliament2' Commands
- parliament2.py
Link to section 'Module' of 'parliament2' Module
You can load the modules by:
module load biocontainers
module load parliament2
Link to section 'Example job' of 'parliament2' Example job
Using #!/bin/sh -l
as shebang in the slurm job script will cause the failure of some biocontainer modules. Please use #!/bin/bash
instead.
To run parliament2 on our clusters:
#!/bin/bash
#SBATCH -A myallocation # Allocation name
#SBATCH -t 1:00:00
#SBATCH -N 1
#SBATCH -n 1
#SBATCH --job-name=parliament2
#SBATCH --mail-type=FAIL,BEGIN,END
#SBATCH --error=%x-%J-%u.err
#SBATCH --output=%x-%J-%u.out
module --force purge
ml biocontainers parliament2
parsnp
Link to section 'Introduction' of 'parsnp' Introduction
Parsnp
is used to align the core genome of hundreds to thousands of bacterial genomes within a few minutes to few hours.
For more information, please check its website: https://biocontainers.pro/tools/parsnp and its home page on Github.
Link to section 'Versions' of 'parsnp' Versions
- 1.6.2
Link to section 'Commands' of 'parsnp' Commands
- parsnp
Link to section 'Module' of 'parsnp' Module
You can load the modules by:
module load biocontainers
module load parsnp
Link to section 'Example job' of 'parsnp' Example job
Using #!/bin/sh -l
as shebang in the slurm job script will cause the failure of some biocontainer modules. Please use #!/bin/bash
instead.
To run Parsnp on our clusters:
#!/bin/bash
#SBATCH -A myallocation # Allocation name
#SBATCH -t 1:00:00
#SBATCH -N 1
#SBATCH -n 8
#SBATCH --job-name=parsnp
#SBATCH --mail-type=FAIL,BEGIN,END
#SBATCH --error=%x-%J-%u.err
#SBATCH --output=%x-%J-%u.out
module --force purge
ml biocontainers parsnp
parsnp -g examples/mers_virus/ref/England1.gbk \
-d examples/mers_virus/genomes/*.fna -c -p 8
pasta
Link to section 'Introduction' of 'pasta' Introduction
PASTA: Ultra-Large Multiple Sequence Alignment for Nucleotide and Amino-Acid Sequences.
BioContainers: https://biocontainers.pro/tools/pasta
Home page: https://github.com/smirarab/pasta
Link to section 'Versions' of 'pasta' Versions
- 1.8.7
Link to section 'Commands' of 'pasta' Commands
- run_pasta.py
- run_seqtools.py
- sumlabels.py
- sumtrees.py
Link to section 'Module' of 'pasta' Module
You can load the modules by:
module load biocontainers
module load pasta
Link to section 'Example job' of 'pasta' Example job
Using #!/bin/sh -l
as shebang in the slurm job script will cause the failure of some biocontainer modules. Please use #!/bin/bash
instead.
To run pasta on our clusters:
#!/bin/bash
#SBATCH -A myallocation # Allocation name
#SBATCH -t 1:00:00
#SBATCH -N 1
#SBATCH -n 1
#SBATCH --job-name=pasta
#SBATCH --mail-type=FAIL,BEGIN,END
#SBATCH --error=%x-%J-%u.err
#SBATCH --output=%x-%J-%u.out
module --force purge
ml biocontainers pasta
pbmm2
Link to section 'Introduction' of 'pbmm2' Introduction
Pbmm2
is a minimap2 frontend for PacBio native data formats.
For more information, please check its website: https://biocontainers.pro/tools/pbmm2 and its home page on Github.
Link to section 'Versions' of 'pbmm2' Versions
- 1.7.0
Link to section 'Commands' of 'pbmm2' Commands
- pbmm2
Link to section 'Module' of 'pbmm2' Module
You can load the modules by:
module load biocontainers
module load pbmm2
Link to section 'Example job' of 'pbmm2' Example job
Using #!/bin/sh -l
as shebang in the slurm job script will cause the failure of some biocontainer modules. Please use #!/bin/bash
instead.
To run Pbmm2 on our clusters:
#!/bin/bash
#SBATCH -A myallocation # Allocation name
#SBATCH -t 1:00:00
#SBATCH -N 1
#SBATCH -n 12
#SBATCH --job-name=pbmm2
#SBATCH --mail-type=FAIL,BEGIN,END
#SBATCH --error=%x-%J-%u.err
#SBATCH --output=%x-%J-%u.out
module --force purge
ml biocontainers pbmm2
pbmm2 --version
pbmm2 align hg38.fa \
alz.polished.hq.bam alz.aligned.bam \
-j 12 --preset ISOSEQ --sort \
--log-level INFO
pbptyper
Link to section 'Introduction' of 'pbptyper' Introduction
pbptyper is a tool to identify the Penicillin Binding Protein (PBP) of Streptococcus pneumoniae assemblies.
Docker hub: https://hub.docker.com/r/staphb/pbptyper
Home page: https://github.com/rpetit3/pbptyper
Link to section 'Versions' of 'pbptyper' Versions
- 1.0.4
Link to section 'Commands' of 'pbptyper' Commands
- pbptyper
Link to section 'Module' of 'pbptyper' Module
You can load the modules by:
module load biocontainers
module load pbptyper
Link to section 'Example job' of 'pbptyper' Example job
Using #!/bin/sh -l
as shebang in the slurm job script will cause the failure of some biocontainer modules. Please use #!/bin/bash
instead.
To run pbptyper on our clusters:
#!/bin/bash
#SBATCH -A myallocation # Allocation name
#SBATCH -t 1:00:00
#SBATCH -N 1
#SBATCH -n 1
#SBATCH --job-name=pbptyper
#SBATCH --mail-type=FAIL,BEGIN,END
#SBATCH --error=%x-%J-%u.err
#SBATCH --output=%x-%J-%u.out
module --force purge
ml biocontainers pbptyper
pbptyper --assembly test/SRR2912551.fna.gz --outdir output
pcangsd
Link to section 'Introduction' of 'pcangsd' Introduction
PCAngsd
is a program that estimates the covariance matrix and individual allele frequencies for low-depth next-generation sequencing (NGS) data in structured/heterogeneous populations using principal component analysis (PCA) to perform multiple population genetic analyses using genotype likelihoods.
For more information, please check its home page on Github.
Link to section 'Versions' of 'pcangsd' Versions
- 1.10
Link to section 'Commands' of 'pcangsd' Commands
- pcangsd
Link to section 'Module' of 'pcangsd' Module
You can load the modules by:
module load biocontainers
module load pcangsd
Link to section 'Example job' of 'pcangsd' Example job
Using #!/bin/sh -l
as shebang in the slurm job script will cause the failure of some biocontainer modules. Please use #!/bin/bash
instead.
To run PCAngsd on our clusters:
#!/bin/bash
#SBATCH -A myallocation # Allocation name
#SBATCH -t 1:00:00
#SBATCH -N 1
#SBATCH -n 12
#SBATCH --job-name=pcangsd
#SBATCH --mail-type=FAIL,BEGIN,END
#SBATCH --error=%x-%J-%u.err
#SBATCH --output=%x-%J-%u.out
module --force purge
ml biocontainers pcangsd
pcangsd -b pupfish.beagle.gz --inbreedSites \
--selection -o pup_pca2 --threads 12
peakranger
Link to section 'Introduction' of 'peakranger' Introduction
Peakranger
is a multi-purpose software suite for analyzing next-generation sequencing (NGS) data.
For more information, please check its website: https://biocontainers.pro/tools/peakranger and its home page: http://ranger.sourceforge.net.
Link to section 'Versions' of 'peakranger' Versions
- 1.18
Link to section 'Commands' of 'peakranger' Commands
- peakranger
Link to section 'Module' of 'peakranger' Module
You can load the modules by:
module load biocontainers
module load peakranger
Link to section 'Example job' of 'peakranger' Example job
Using #!/bin/sh -l
as shebang in the slurm job script will cause the failure of some biocontainer modules. Please use #!/bin/bash
instead.
To run Peakranger on our clusters:
#!/bin/bash
#SBATCH -A myallocation # Allocation name
#SBATCH -t 1:00:00
#SBATCH -N 1
#SBATCH -n 1
#SBATCH --job-name=peakranger
#SBATCH --mail-type=FAIL,BEGIN,END
#SBATCH --error=%x-%J-%u.err
#SBATCH --output=%x-%J-%u.out
module --force purge
ml biocontainers peakranger
peakranger ccat --format bam 27-1_sorted_MDRD_MQ30filtered.bam 27-4_sorted_MDRD_MQ30filtered.bam \
ccat_result_with_HTML_report_5kb_region --report \
--gene_annot_file refGene.txt --plot_region 10000
pepper_deepvariant
Link to section 'Introduction' of 'pepper_deepvariant' Introduction
PEPPER is a genome inference module based on recurrent neural networks that enables long-read variant calling and nanopore assembly polishing in the PEPPER-Margin-DeepVariant pipeline. This pipeline enables nanopore-based variant calling with DeepVariant.
Docker hub: https://hub.docker.com/r/kishwars/pepper_deepvariant
Home page: https://github.com/kishwarshafin/pepper
Link to section 'Versions' of 'pepper_deepvariant' Versions
- r0.4.1
Link to section 'Commands' of 'pepper_deepvariant' Commands
- run_pepper_margin_deepvariant
Link to section 'Module' of 'pepper_deepvariant' Module
You can load the modules by:
module load biocontainers
module load pepper_deepvariant
Link to section 'Example job' of 'pepper_deepvariant' Example job
Using #!/bin/sh -l
as shebang in the slurm job script will cause the failure of some biocontainer modules. Please use #!/bin/bash
instead.
To run pepper_deepvariant on our clusters:
#!/bin/bash
#SBATCH -A myallocation # Allocation name
#SBATCH -t 1:00:00
#SBATCH -N 1
#SBATCH -n 32
#SBATCH --job-name=pepper_deepvariant
#SBATCH --mail-type=FAIL,BEGIN,END
#SBATCH --error=%x-%J-%u.err
#SBATCH --output=%x-%J-%u.out
module --force purge
ml biocontainers pepper_deepvariant
BASE=$PWD
# Set up input data
INPUT_DIR="${BASE}/input/data"
REF="GRCh38_no_alt.chr20.fa"
BAM="HG002_ONT_2_GRCh38.chr20.quickstart.bam"
# Set the number of CPUs to use
THREADS=32
# Set up output directory
OUTPUT_DIR="${BASE}/output"
OUTPUT_PREFIX="HG002_ONT_2_GRCh38_PEPPER_Margin_DeepVariant.chr20"
OUTPUT_VCF="HG002_ONT_2_GRCh38_PEPPER_Margin_DeepVariant.chr20.vcf.gz"
TRUTH_VCF="HG002_GRCh38_1_22_v4.2.1_benchmark.quickstart.vcf.gz"
TRUTH_BED="HG002_GRCh38_1_22_v4.2.1_benchmark_noinconsistent.quickstart.bed"
# Create local directory structure
mkdir -p "${OUTPUT_DIR}"
mkdir -p "${INPUT_DIR}"
# Download the data to input directory
wget -P ${INPUT_DIR} https://storage.googleapis.com/pepper-deepvariant-public/quickstart_data/HG002_ONT_2_GRCh38.chr20.quickstart.bam
wget -P ${INPUT_DIR} https://storage.googleapis.com/pepper-deepvariant-public/quickstart_data/HG002_ONT_2_GRCh38.chr20.quickstart.bam.bai
wget -P ${INPUT_DIR} https://storage.googleapis.com/pepper-deepvariant-public/quickstart_data/GRCh38_no_alt.chr20.fa
wget -P ${INPUT_DIR} https://storage.googleapis.com/pepper-deepvariant-public/quickstart_data/GRCh38_no_alt.chr20.fa.fai
wget -P ${INPUT_DIR} https://storage.googleapis.com/pepper-deepvariant-public/quickstart_data/HG002_GRCh38_1_22_v4.2.1_benchmark.quickstart.vcf.gz
wget -P ${INPUT_DIR} https://storage.googleapis.com/pepper-deepvariant-public/quickstart_data/HG002_GRCh38_1_22_v4.2.1_benchmark_noinconsistent.quickstart.bed
run_pepper_margin_deepvariant call_variant \
-b input/data/HG002_ONT_2_GRCh38.chr20.quickstart.bam \
-f input/data/GRCh38_no_alt.chr20.fa -o output \
-p HG002_ONT_2_GRCh38_PEPPER_Margin_DeepVariant.chr20 \
-t 32 -r chr20:1000000-1020000 \
--ont_r9_guppy5_sup --ont
perl-bioperl
Link to section 'Introduction' of 'perl-bioperl' Introduction
BioPerl
is a collection of Perl modules that facilitate the development of Perl scripts for bioinformatics applications. It provides software modules for many of the typical tasks of bioinformatics programming.
For more information, please check its website: https://biocontainers.pro/tools/perl-bioperl.
Link to section 'Versions' of 'perl-bioperl' Versions
- 1.7.2-pl526
Link to section 'Commands' of 'perl-bioperl' Commands
- SOAPsh.pl
- ace.pl
- bam2bedgraph
- bamToGBrowse.pl
- bdf2gdfont.pl
- bdftogd
- binhex.pl
- bp_aacomp.pl
- bp_biofetch_genbank_proxy.pl
- bp_bioflat_index.pl
- bp_biogetseq.pl
- bp_blast2tree.pl
- bp_bulk_load_gff.pl
- bp_chaos_plot.pl
- bp_classify_hits_kingdom.pl
- bp_composite_LD.pl
- bp_das_server.pl
- bp_dbsplit.pl
- bp_download_query_genbank.pl
- bp_extract_feature_seq.pl
- bp_fast_load_gff.pl
- bp_fastam9_to_table.pl
- bp_fetch.pl
- bp_filter_search.pl
- bp_find-blast-matches.pl
- bp_flanks.pl
- bp_gccalc.pl
- bp_genbank2gff.pl
- bp_genbank2gff3.pl
- bp_generate_histogram.pl
- bp_heterogeneity_test.pl
- bp_hivq.pl
- bp_hmmer_to_table.pl
- bp_index.pl
- bp_load_gff.pl
- bp_local_taxonomydb_query.pl
- bp_make_mrna_protein.pl
- bp_mask_by_search.pl
- bp_meta_gff.pl
- bp_mrtrans.pl
- bp_mutate.pl
- bp_netinstall.pl
- bp_nexus2nh.pl
- bp_nrdb.pl
- bp_oligo_count.pl
- bp_pairwise_kaks
- bp_parse_hmmsearch.pl
- bp_process_gadfly.pl
- bp_process_sgd.pl
- bp_process_wormbase.pl
- bp_query_entrez_taxa.pl
- bp_remote_blast.pl
- bp_revtrans-motif.pl
- bp_search2alnblocks.pl
- bp_search2gff.pl
- bp_search2table.pl
- bp_search2tribe.pl
- bp_seq_length.pl
- bp_seqconvert.pl
- bp_seqcut.pl
- bp_seqfeature_delete.pl
- bp_seqfeature_gff3.pl
- bp_seqfeature_load.pl
- bp_seqpart.pl
- bp_seqret.pl
- bp_seqretsplit.pl
- bp_split_seq.pl
- bp_sreformat.pl
- bp_taxid4species.pl
- bp_taxonomy2tree.pl
- bp_translate_seq.pl
- bp_tree2pag.pl
- bp_unflatten_seq.pl
- ccconfig
- chartex
- chi2
- chrom_sizes.pl
- circo
- clustalw
- clustalw2
- corelist
- cpan
- cpanm
- dbilogstrip
- dbiprof
- dbiproxy
- debinhex.pl
- enc2xs
- encguess
- genomeCoverageBed.pl
- h2ph
- h2xs
- htmltree
- instmodsh
- json_pp
- json_xs
- lwp-download
- lwp-dump
- lwp-mirror
- lwp-request
- perl
- perl5.26.2
- perlbug
- perldoc
- perlivp
- perlthanks
- piconv
- pl2pm
- pod2html
- pod2man
- pod2text
- pod2usage
- podchecker
- podselect
- prove
- ptar
- ptardiff
- ptargrep
- shasum
- splain
- stag-autoschema.pl
- stag-db.pl
- stag-diff.pl
- stag-drawtree.pl
- stag-filter.pl
- stag-findsubtree.pl
- stag-flatten.pl
- stag-grep.pl
- stag-handle.pl
- stag-itext2simple.pl
- stag-itext2sxpr.pl
- stag-itext2xml.pl
- stag-join.pl
- stag-merge.pl
- stag-mogrify.pl
- stag-parse.pl
- stag-query.pl
- stag-splitter.pl
- stag-view.pl
- stag-xml2itext.pl
- stubmaker.pl
- t_coffee
- tpage
- ttree
- unflatten
- webtidy
- xml_grep
- xml_merge
- xml_pp
- xml_spellcheck
- xml_split
- xpath
- xsubpp
- zipdetails
Link to section 'Module' of 'perl-bioperl' Module
You can load the modules by:
module load biocontainers
module load perl-bioperl
Link to section 'Example job' of 'perl-bioperl' Example job
Using #!/bin/sh -l
as shebang in the slurm job script will cause the failure of some biocontainer modules. Please use #!/bin/bash
instead.
To run BioPerl on our clusters:
#!/bin/bash
#SBATCH -A myallocation # Allocation name
#SBATCH -t 1:00:00
#SBATCH -N 1
#SBATCH -n 1
#SBATCH --job-name=perl-bioperl
#SBATCH --mail-type=FAIL,BEGIN,END
#SBATCH --error=%x-%J-%u.err
#SBATCH --output=%x-%J-%u.out
module --force purge
ml biocontainers perl-bioperl
phast
Link to section 'Introduction' of 'phast' Introduction
PHAST is a freely available software package for comparative and evolutionary genomics.
For more information, please check:
BioContainers: https://biocontainers.pro/tools/phast
Home page: http://compgen.cshl.edu/phast/
Link to section 'Versions' of 'phast' Versions
- 1.5
Link to section 'Commands' of 'phast' Commands
- all_dists
- base_evolve
- chooseLines
- clean_genes
- consEntropy
- convert_coords
- display_rate_matrix
- dless
- dlessP
- draw_tree
- eval_predictions
- exoniphy
- hmm_train
- hmm_tweak
- hmm_view
- indelFit
- indelHistory
- maf_parse
- makeHKY
- modFreqs
- msa_diff
- msa_split
- msa_view
- pbsDecode
- pbsEncode
- pbsScoreMatrix
- pbsTrain
- phast
- phastBias
- phastCons
- phastMotif
- phastOdds
- phyloBoot
- phyloFit
- phyloP
- prequel
- refeature
- stringiphy
- treeGen
- tree_doctor
Link to section 'Module' of 'phast' Module
You can load the modules by:
module load biocontainers
module load phast
Link to section 'Example job' of 'phast' Example job
Using #!/bin/sh -l
as shebang in the slurm job script will cause the failure of some biocontainer modules. Please use #!/bin/bash
instead.
To run phast on our clusters:
#!/bin/bash
#SBATCH -A myallocation # Allocation name
#SBATCH -t 1:00:00
#SBATCH -N 1
#SBATCH -n 1
#SBATCH --job-name=phast
#SBATCH --mail-type=FAIL,BEGIN,END
#SBATCH --error=%x-%J-%u.err
#SBATCH --output=%x-%J-%u.out
module --force purge
ml biocontainers phast
phd2fasta
Link to section 'Introduction' of 'phd2fasta' Introduction
Phd2fasta
is a tool to convert Phred ‘phd’ format files to ‘fasta’ format.
For more information, please check its home page: http://www.phrap.org/phredphrapconsed.html.
Link to section 'Versions' of 'phd2fasta' Versions
- 0.990622
Link to section 'Commands' of 'phd2fasta' Commands
- phd2fasta
Link to section 'Module' of 'phd2fasta' Module
You can load the modules by:
module load biocontainers
module load phd2fasta
Link to section 'Example job' of 'phd2fasta' Example job
Using #!/bin/sh -l
as shebang in the slurm job script will cause the failure of some biocontainer modules. Please use #!/bin/bash
instead.
To run Phd2fasta on our clusters:
#!/bin/bash
#SBATCH -A myallocation # Allocation name
#SBATCH -t 1:00:00
#SBATCH -N 1
#SBATCH -n 1
#SBATCH --job-name=phd2fasta
#SBATCH --mail-type=FAIL,BEGIN,END
#SBATCH --error=%x-%J-%u.err
#SBATCH --output=%x-%J-%u.out
module --force purge
ml biocontainers phd2fasta
phg
Link to section 'Introduction' of 'phg' Introduction
Practical Haplotype Graph (PHG) is a general, graph-based, computational framework that can be used with a variety of skim sequencing methods to infer high-density genotypes directly from low-coverage sequence.
Docker hub: https://hub.docker.com/r/maizegenetics/phg
Home page: https://www.maizegenetics.net/phg
Link to section 'Versions' of 'phg' Versions
- 1.0
Link to section 'Commands' of 'phg' Commands
- CreateConsensi.sh
- CreateHaplotypes.sh
- CreateReferenceIntervals.sh
- CreateSmallDataSet.sh
- CreateValidIntervalsFile.sh
- IndexPangenome.sh
- LoadAssemblyAnchors.sh
- LoadGenomeIntervals.sh
- ParallelAssemblyAnchorsLoad.sh
- RunLiquibaseUpdates.sh
- CreateHaplotypesFromBAM.groovy
- CreateHaplotypesFromFastq.groovy
- CreateHaplotypesFromGVCF.groovy
Link to section 'Module' of 'phg' Module
You can load the modules by:
module load biocontainers
module load phg
Link to section 'Example job' of 'phg' Example job
Using #!/bin/sh -l
as shebang in the slurm job script will cause the failure of some biocontainer modules. Please use #!/bin/bash
instead.
To run phg on our clusters:
#!/bin/bash
#SBATCH -A myallocation # Allocation name
#SBATCH -t 1:00:00
#SBATCH -N 1
#SBATCH -n 1
#SBATCH --job-name=phg
#SBATCH --mail-type=FAIL,BEGIN,END
#SBATCH --error=%x-%J-%u.err
#SBATCH --output=%x-%J-%u.out
module --force purge
ml biocontainers phg
phipack
Link to section 'Introduction' of 'phipack' Introduction
PhiPack: PHI test and other tests of recombination
BioContainers: https://biocontainers.pro/tools/phipack
Home page: http://www.maths.otago.ac.nz/~dbryant/software.html
Link to section 'Versions' of 'phipack' Versions
- 1.1
Link to section 'Commands' of 'phipack' Commands
- Phi
- Profile
Link to section 'Module' of 'phipack' Module
You can load the modules by:
module load biocontainers
module load phipack
Link to section 'Example job' of 'phipack' Example job
Using #!/bin/sh -l
as shebang in the slurm job script will cause the failure of some biocontainer modules. Please use #!/bin/bash
instead.
To run phipack on our clusters:
#!/bin/bash
#SBATCH -A myallocation # Allocation name
#SBATCH -t 1:00:00
#SBATCH -N 1
#SBATCH -n 1
#SBATCH --job-name=phipack
#SBATCH --mail-type=FAIL,BEGIN,END
#SBATCH --error=%x-%J-%u.err
#SBATCH --output=%x-%J-%u.out
module --force purge
ml biocontainers phipack
phrap
Link to section 'Introduction' of 'phrap' Introduction
phrap
is a program for assembling shotgun DNA sequence data.
For more information, please check its home page: http://www.phrap.org/phredphrapconsed.html#block_phrap.
Link to section 'Versions' of 'phrap' Versions
- 1.090518
Link to section 'Commands' of 'phrap' Commands
- phrap
Link to section 'Module' of 'phrap' Module
You can load the modules by:
module load biocontainers
module load phrap
Link to section 'Example job' of 'phrap' Example job
Using #!/bin/sh -l
as shebang in the slurm job script will cause the failure of some biocontainer modules. Please use #!/bin/bash
instead.
To run phrap on our clusters:
#!/bin/bash
#SBATCH -A myallocation # Allocation name
#SBATCH -t 1:00:00
#SBATCH -N 1
#SBATCH -n 1
#SBATCH --job-name=phrap
#SBATCH --mail-type=FAIL,BEGIN,END
#SBATCH --error=%x-%J-%u.err
#SBATCH --output=%x-%J-%u.out
module --force purge
ml biocontainers phrap
phred
Link to section 'Introduction' of 'phred' Introduction
phred
software reads DNA sequencing trace files, calls bases, and assigns a quality value to each called base.
For more information, please check its home page: http://www.phrap.org/phredphrapconsed.html#block_phred.
Link to section 'Versions' of 'phred' Versions
- 0.071220.c
Link to section 'Commands' of 'phred' Commands
- phred
Link to section 'Module' of 'phred' Module
You can load the modules by:
module load biocontainers
module load phred
Link to section 'Example job' of 'phred' Example job
Using #!/bin/sh -l
as shebang in the slurm job script will cause the failure of some biocontainer modules. Please use #!/bin/bash
instead.
To run phred on our clusters:
#!/bin/bash
#SBATCH -A myallocation # Allocation name
#SBATCH -t 1:00:00
#SBATCH -N 1
#SBATCH -n 1
#SBATCH --job-name=phred
#SBATCH --mail-type=FAIL,BEGIN,END
#SBATCH --error=%x-%J-%u.err
#SBATCH --output=%x-%J-%u.out
module --force purge
ml biocontainers phred
phylosuite
Link to section 'Introduction' of 'phylosuite' Introduction
PhyloSuite is an integrated and scalable desktop platform for streamlined molecular sequence data management and evolutionary phylogenetics studies.
Docker hub: https://hub.docker.com/r/dongzhang0725/phylosuite
Home page: https://github.com/dongzhang0725/PhyloSuite
Link to section 'Versions' of 'phylosuite' Versions
- 1.2.3
Link to section 'Commands' of 'phylosuite' Commands
- PhyloSuite.sh
Link to section 'Module' of 'phylosuite' Module
You can load the modules by:
module load biocontainers
module load phylosuite
Link to section 'Example job' of 'phylosuite' Example job
Using #!/bin/sh -l
as shebang in the slurm job script will cause the failure of some biocontainer modules. Please use #!/bin/bash
instead.
To run phylosuite on our clusters:
#!/bin/bash
#SBATCH -A myallocation # Allocation name
#SBATCH -t 1:00:00
#SBATCH -N 1
#SBATCH -n 1
#SBATCH --job-name=phylosuite
#SBATCH --mail-type=FAIL,BEGIN,END
#SBATCH --error=%x-%J-%u.err
#SBATCH --output=%x-%J-%u.out
module --force purge
ml biocontainers phylosuite
picard
Picard
is a set of command line tools for manipulating high-throughput sequencing (HTS) data and formats such as SAM/BAM/CRAM and VCF.
Detailed usage can be found here: https://broadinstitute.github.io/picard/
Link to section 'Versions' of 'picard' Versions
- 2.25.1
- 2.26.10
Link to section 'Commands' of 'picard' Commands
picard
Link to section 'Module' of 'picard' Module
You can load the modules by:
module load biocontainers
module load picard/2.26.10
Link to section 'Example job' of 'picard' Example job
Using #!/bin/sh -l
as shebang in the slurm job script will cause the failure of some biocontainer modules. Please use #!/bin/bash
instead.
To run picard our our clusters:
#!/bin/bash
#SBATCH -A myallocation # Allocation name
#SBATCH -t 20:00:00
#SBATCH -N 1
#SBATCH -n 24
#SBATCH --job-name=picard
#SBATCH --mail-type=FAIL,BEGIN,END
#SBATCH --error=%x-%J-%u.err
#SBATCH --output=%x-%J-%u.out
module --force purge
ml biocontainers picard/2.26.10
picard MarkDuplicates -Xmx64g I=19P0126636WES_sorted.bam O=19P0126636WES_sorted_md.bam M=19P0126636WES.sorted.markdup.txt REMOVE_DUPLICATES=true
picard BuildBamIndex -Xmx64g I=19P0126636WES_sorted_md.bam
picard CreateSequenceDictionary -R hg38.fa -O hg38.dict
picrust2
Link to section 'Introduction' of 'picrust2' Introduction
Picrust2
is a software for predicting functional abundances based only on marker gene sequences.
For more information, please check its website: https://biocontainers.pro/tools/picrust2 and its home page on Github.
Link to section 'Versions' of 'picrust2' Versions
- 2.4.2
- 2.5.0
Link to section 'Commands' of 'picrust2' Commands
- add_descriptions.py
- convert_table.py
- hsp.py
- metagenome_pipeline.py
- pathway_pipeline.py
- picrust2_pipeline.py
- place_seqs.py
- print_picrust2_config.py
- run_abundance.py
- run_sepp.py
- run_tipp.py
- run_tipp_tool.py
- run_upp.py
- shuffle_predictions.py
- split_sequences.py
- sumlabels.py
- sumtrees.py
Link to section 'Module' of 'picrust2' Module
You can load the modules by:
module load biocontainers
module load picrust2
Link to section 'Example job' of 'picrust2' Example job
Using #!/bin/sh -l
as shebang in the slurm job script will cause the failure of some biocontainer modules. Please use #!/bin/bash
instead.
To run Picrust2 on our clusters:
#!/bin/bash
#SBATCH -A myallocation # Allocation name
#SBATCH -t 1:00:00
#SBATCH -N 1
#SBATCH -n 10
#SBATCH --job-name=picrust2
#SBATCH --mail-type=FAIL,BEGIN,END
#SBATCH --error=%x-%J-%u.err
#SBATCH --output=%x-%J-%u.out
module --force purge
ml biocontainers picrust2
place_seqs.py -s ../seqs.fna -o out.tre -p 10 \
--intermediate intermediate/place_seqs
hsp.py -i 16S -t out.tre -o marker_predicted_and_nsti.tsv.gz -p 10 -n
hsp.py -i EC -t out.tre -o EC_predicted.tsv.gz -p 10
metagenome_pipeline.py -i ../table.biom -m marker_predicted_and_nsti.tsv.gz -f EC_predicted.tsv.gz -o EC_metagenome_out --strat_out
convert_table.py EC_metagenome_out/pred_metagenome_contrib.tsv.gz \
-c contrib_to_legacy \
-o EC_metagenome_out/pred_metagenome_contrib.legacy.tsv.gz
pathway_pipeline.py -i EC_metagenome_out/pred_metagenome_contrib.tsv.gz \
-o pathways_out -p 10
add_descriptions.py -i EC_metagenome_out/pred_metagenome_unstrat.tsv.gz -m EC \
-o EC_metagenome_out/pred_metagenome_unstrat_descrip.tsv.gz
add_descriptions.py -i pathways_out/path_abun_unstrat.tsv.gz -m METACYC \
-o pathways_out/path_abun_unstrat_descrip.tsv.gz
picrust2_pipeline.py -s chemerin_16S/seqs.fna -i chemerin_16S/table.biom \
-o picrust2_out_pipeline -p 10
pilon
Link to section 'Introduction' of 'pilon' Introduction
Pilon
is an automated genome assembly improvement and variant detection tool.
For more information, please check its website: https://biocontainers.pro/tools/pilon and its home page on Github.
Link to section 'Versions' of 'pilon' Versions
- 1.24
Link to section 'Commands' of 'pilon' Commands
- pilon.jar
Link to section 'Module' of 'pilon' Module
You can load the modules by:
module load biocontainers
module load pilon
Link to section 'Example job' of 'pilon' Example job
Using #!/bin/sh -l
as shebang in the slurm job script will cause the failure of some biocontainer modules. Please use #!/bin/bash
instead.
To run Pilon on our clusters:
#!/bin/bash
#SBATCH -A myallocation # Allocation name
#SBATCH -t 1:00:00
#SBATCH -N 1
#SBATCH -n 12
#SBATCH --job-name=pilon
#SBATCH --mail-type=FAIL,BEGIN,END
#SBATCH --error=%x-%J-%u.err
#SBATCH --output=%x-%J-%u.out
module --force purge
ml biocontainers pilon
pilon.jar --nostrays \
--genome scaffolds.fasta \
--frags out_sorted.bam \
--vcf --verbose --threads 12 \
--output pilon_corrected \
--outdir pilon_outdir
pindel
Link to section 'Introduction' of 'pindel' Introduction
Pindel
is used to detect breakpoints of large deletions, medium sized insertions, inversions, tandem duplications and other structural variants at single-based resolution from next-gen sequence data.
For more information, please check its website: https://biocontainers.pro/tools/pindel and its home page: http://gmt.genome.wustl.edu/packages/pindel/index.html.
Link to section 'Versions' of 'pindel' Versions
- 0.2.5b9
Link to section 'Commands' of 'pindel' Commands
- pindel
- pindel2cvf
Link to section 'Module' of 'pindel' Module
You can load the modules by:
module load biocontainers
module load pindel
Link to section 'Example job' of 'pindel' Example job
Using #!/bin/sh -l
as shebang in the slurm job script will cause the failure of some biocontainer modules. Please use #!/bin/bash
instead.
To run Pindel on our clusters:
#!/bin/bash
#SBATCH -A myallocation # Allocation name
#SBATCH -t 1:00:00
#SBATCH -N 1
#SBATCH -n 1
#SBATCH --job-name=pindel
#SBATCH --mail-type=FAIL,BEGIN,END
#SBATCH --error=%x-%J-%u.err
#SBATCH --output=%x-%J-%u.out
module --force purge
ml biocontainers pindel
pindel -i simulated_config.txt -f simulated_reference.fa -o bamtest -c ALL
pindel -p COLO-829_20-p_ok.txt -f hs_ref_chr20.fa -o colontumor -c 20
pindel2vcf -r hs_ref_chr20.fa -R HUMAN_G1K_V2 -d 20100101 -p colontumor_D -e 5
pirate
Link to section 'Introduction' of 'pirate' Introduction
Pirate
is a pangenome analysis and threshold evaluation toolbox.
For more information, please check its website: https://biocontainers.pro/tools/pirate and its home page on Github.
Link to section 'Versions' of 'pirate' Versions
- 1.0.4
Link to section 'Commands' of 'pirate' Commands
- PIRATE
- FET.pl
- PIRATE_to_Rtab.pl
- PIRATE_to_roary.pl
- SOAPsh.pl
- ace.pl
- analyse_blast_outputs.pl
- analyse_loci_list.pl
- annotate_treeWAS_output.pl
- bamToGBrowse.pl
- bdf2gdfont.pl
- binhex.pl
- bp_aacomp.pl
- bp_biofetch_genbank_proxy.pl
- bp_bioflat_index.pl
- bp_biogetseq.pl
- bp_blast2tree.pl
- bp_bulk_load_gff.pl
- bp_chaos_plot.pl
- bp_classify_hits_kingdom.pl
- bp_composite_LD.pl
- bp_das_server.pl
- bp_dbsplit.pl
- bp_download_query_genbank.pl
- bp_extract_feature_seq.pl
- bp_fast_load_gff.pl
- bp_fastam9_to_table.pl
- bp_fetch.pl
- bp_filter_search.pl
- bp_find-blast-matches.pl
- bp_flanks.pl
- bp_gccalc.pl
- bp_genbank2gff.pl
- bp_genbank2gff3.pl
- bp_generate_histogram.pl
- bp_heterogeneity_test.pl
- bp_hivq.pl
- bp_hmmer_to_table.pl
- bp_index.pl
- bp_load_gff.pl
- bp_local_taxonomydb_query.pl
- bp_make_mrna_protein.pl
- bp_mask_by_search.pl
- bp_meta_gff.pl
- bp_mrtrans.pl
- bp_mutate.pl
- bp_netinstall.pl
- bp_nexus2nh.pl
- bp_nrdb.pl
- bp_oligo_count.pl
- bp_parse_hmmsearch.pl
- bp_process_gadfly.pl
- bp_process_sgd.pl
- bp_process_wormbase.pl
- bp_query_entrez_taxa.pl
- bp_remote_blast.pl
- bp_revtrans-motif.pl
- bp_search2alnblocks.pl
- bp_search2gff.pl
- bp_search2table.pl
- bp_search2tribe.pl
- bp_seq_length.pl
- bp_seqconvert.pl
- bp_seqcut.pl
- bp_seqfeature_delete.pl
- bp_seqfeature_gff3.pl
- bp_seqfeature_load.pl
- bp_seqpart.pl
- bp_seqret.pl
- bp_seqretsplit.pl
- bp_split_seq.pl
- bp_sreformat.pl
- bp_taxid4species.pl
- bp_taxonomy2tree.pl
- bp_translate_seq.pl
- bp_tree2pag.pl
- bp_unflatten_seq.pl
- cd-hit-2d-para.pl
- cd-hit-clstr_2_blm8.pl
- cd-hit-div.pl
- cd-hit-para.pl
- chrom_sizes.pl
- clstr2tree.pl
- clstr2txt.pl
- clstr2xml.pl
- clstr_cut.pl
- clstr_list.pl
- clstr_list_sort.pl
- clstr_merge.pl
- clstr_merge_noorder.pl
- clstr_quality_eval.pl
- clstr_quality_eval_by_link.pl
- clstr_reduce.pl
- clstr_renumber.pl
- clstr_rep.pl
- clstr_reps_faa_rev.pl
- clstr_rev.pl
- clstr_select.pl
- clstr_select_rep.pl
- clstr_size_histogram.pl
- clstr_size_stat.pl
- clstr_sort_by.pl
- clstr_sort_prot_by.pl
- clstr_sql_tbl.pl
- clstr_sql_tbl_sort.pl
- convert_to_distmat.pl
- convert_to_treeWAS.pl
- debinhex.pl
- genomeCoverageBed.pl
- legacy_blast.pl
- make_multi_seq.pl
- pangenome_variants_to_treeWAS.pl
- paralogs_to_Rtab.pl
- plot_2d.pl
- plot_len1.pl
- stag-autoschema.pl
- stag-db.pl
- stag-diff.pl
- stag-drawtree.pl
- stag-filter.pl
- stag-findsubtree.pl
- stag-flatten.pl
- stag-grep.pl
- stag-handle.pl
- stag-itext2simple.pl
- stag-itext2sxpr.pl
- stag-itext2xml.pl
- stag-join.pl
- stag-merge.pl
- stag-mogrify.pl
- stag-parse.pl
- stag-query.pl
- stag-splitter.pl
- stag-view.pl
- stag-xml2itext.pl
- stubmaker.pl
- subsample_outputs.pl
- subset_alignments.pl
- unique_sequences.pl
- update_blastdb.pl
Link to section 'Module' of 'pirate' Module
You can load the modules by:
module load biocontainers
module load pirate
Link to section 'Example job' of 'pirate' Example job
Using #!/bin/sh -l
as shebang in the slurm job script will cause the failure of some biocontainer modules. Please use #!/bin/bash
instead.
To run Pirate on our clusters:
#!/bin/bash
#SBATCH -A myallocation # Allocation name
#SBATCH -t 1:00:00
#SBATCH -N 1
#SBATCH -n 1
#SBATCH --job-name=pirate
#SBATCH --mail-type=FAIL,BEGIN,END
#SBATCH --error=%x-%J-%u.err
#SBATCH --output=%x-%J-%u.out
module --force purge
ml biocontainers pirate
piscem
Link to section 'Introduction' of 'piscem' Introduction
piscem is a rust wrapper for a next-generation index + mapper tool (still currently written in C++17).
BioContainers: https://biocontainers.pro/tools/piscem
Home page: https://github.com/COMBINE-lab/piscem
Link to section 'Versions' of 'piscem' Versions
- 0.4.3
Link to section 'Commands' of 'piscem' Commands
- piscem
Link to section 'Module' of 'piscem' Module
You can load the modules by:
module load biocontainers
module load piscem
Link to section 'Example job' of 'piscem' Example job
Using #!/bin/sh -l
as shebang in the slurm job script will cause the failure of some biocontainer modules. Please use #!/bin/bash
instead.
To run piscem on our clusters:
#!/bin/bash
#SBATCH -A myallocation # Allocation name
#SBATCH -t 1:00:00
#SBATCH -N 1
#SBATCH -n 1
#SBATCH --job-name=piscem
#SBATCH --mail-type=FAIL,BEGIN,END
#SBATCH --error=%x-%J-%u.err
#SBATCH --output=%x-%J-%u.out
module --force purge
ml biocontainers piscem
pixy
Link to section 'Introduction' of 'pixy' Introduction
pixy is a command-line tool for painlessly estimating average nucleotide diversity within (π) and between (dxy) populations from a VCF.
Home page: https://github.com/ksamuk/pixy
Link to section 'Versions' of 'pixy' Versions
- 1.2.7
Link to section 'Commands' of 'pixy' Commands
- pixy
Link to section 'Module' of 'pixy' Module
You can load the modules by:
module load biocontainers
module load pixy
Link to section 'Example job' of 'pixy' Example job
Using #!/bin/sh -l
as shebang in the slurm job script will cause the failure of some biocontainer modules. Please use #!/bin/bash
instead.
To run pixy on our clusters:
#!/bin/bash
#SBATCH -A myallocation # Allocation name
#SBATCH -t 1:00:00
#SBATCH -N 1
#SBATCH -n 1
#SBATCH --job-name=pixy
#SBATCH --mail-type=FAIL,BEGIN,END
#SBATCH --error=%x-%J-%u.err
#SBATCH --output=%x-%J-%u.out
module --force purge
ml biocontainers pixy
plasmidfinder
Link to section 'Introduction' of 'plasmidfinder' Introduction
PlasmidFinder identifies plasmids in total or partial sequenced isolates of bacteria.
Docker hub: https://hub.docker.com/r/staphb/plasmidfinder
Home page: https://bitbucket.org/genomicepidemiology/plasmidfinder/src/master/
Link to section 'Versions' of 'plasmidfinder' Versions
- 2.1.6
Link to section 'Commands' of 'plasmidfinder' Commands
- plasmidfinder.py
Link to section 'Module' of 'plasmidfinder' Module
You can load the modules by:
module load biocontainers
module load plasmidfinder
Link to section 'Example job' of 'plasmidfinder' Example job
Using #!/bin/sh -l
as shebang in the slurm job script will cause the failure of some biocontainer modules. Please use #!/bin/bash
instead.
To run plasmidfinder on our clusters:
#!/bin/bash
#SBATCH -A myallocation # Allocation name
#SBATCH -t 1:00:00
#SBATCH -N 1
#SBATCH -n 1
#SBATCH --job-name=plasmidfinder
#SBATCH --mail-type=FAIL,BEGIN,END
#SBATCH --error=%x-%J-%u.err
#SBATCH --output=%x-%J-%u.out
module --force purge
ml biocontainers plasmidfinder
plasmidfinder.py -p test/database \
-i test/test.fsa -o output -mp blastn -x -q
platon
Link to section 'Introduction' of 'platon' Introduction
Platon: identification and characterization of bacterial plasmid contigs from short-read draft assemblies.
BioContainers: https://biocontainers.pro/tools/platon
Home page: https://github.com/oschwengers/platon
Link to section 'Versions' of 'platon' Versions
- 1.6
Link to section 'Commands' of 'platon' Commands
- platon
Link to section 'Module' of 'platon' Module
You can load the modules by:
module load biocontainers
module load platon
The environment variable PLATON_DB
is set as /depot/itap/datasets/platon/db
. This directory contains the required database.
Link to section 'Example job' of 'platon' Example job
Using #!/bin/sh -l
as shebang in the slurm job script will cause the failure of some biocontainer modules. Please use #!/bin/bash
instead.
To run platon on our clusters:
#!/bin/bash
#SBATCH -A myallocation # Allocation name
#SBATCH -t 1:00:00
#SBATCH -N 1
#SBATCH -n 4
#SBATCH --job-name=platon
#SBATCH --mail-type=FAIL,BEGIN,END
#SBATCH --error=%x-%J-%u.err
#SBATCH --output=%x-%J-%u.out
module --force purge
ml biocontainers platon
platon --verbose --threads 4 contigs.fasta
getorganelle
Link to section 'Introduction' of 'getorganelle' Introduction
GetOrganelle is a fast and versatile toolkit for accurate de novo assembly of organelle genomes.
For more information, please check its website: https://biocontainers.pro/tools/getorganelle and its home page on https://github.com/Kinggerm/GetOrganelle.
Link to section 'Versions' of 'getorganelle' Versions
-
1.7.7.0
Link to section 'Commands' of 'getorganelle' Commands
- get_organelle_config.py
- get_organelle_from_assembly.py
- get_organelle_from_reads.py
- slim_graph.py
- summary_get_organelle_output.py
Link to section 'Module' of 'getorganelle' Module
You can load the modules by:
module load biocontainers
module load getorganelle
Link to section 'Example job' of 'getorganelle' Example job
Using #!/bin/sh -l
as shebang in the slurm job script will cause the failure of some biocontainer modules. Please use #!/bin/bash
instead.
To run GetOrganelle on our clusters:
#!/bin/bash
#SBATCH -A myallocation # Allocation name
#SBATCH -t 1:00:00
#SBATCH -N 1
#SBATCH -n 1
#SBATCH --job-name=getorganelle
#SBATCH --mail-type=FAIL,BEGIN,END
#SBATCH --error=%x-%J-%u.err
#SBATCH --output=%x-%J-%u.out
module --force purge
ml biocontainers getorganelle
get_organelle_config.py --add embplant_pt,embplant_mt
get_organelle_from_reads.py -1 Arabidopsis_simulated.1.fq.gz \
-2 Arabidopsis_simulated.2.fq.gz -t 1 -o Arabidopsis_simulated.plastome \
-F embplant_pt -R 10
platypus
Link to section 'Introduction' of 'platypus' Introduction
Platypus
is a tool designed for efficient and accurate variant-detection in high-throughput sequencing data.
For more information, please check its website: https://biocontainers.pro/tools/platypus
Link to section 'Versions' of 'platypus' Versions
- 0.8.1
Link to section 'Commands' of 'platypus' Commands
- platypus
Link to section 'Module' of 'platypus' Module
You can load the modules by:
module load biocontainers
module load platypus
Link to section 'Example job' of 'platypus' Example job
Using #!/bin/sh -l
as shebang in the slurm job script will cause the failure of some biocontainer modules. Please use #!/bin/bash
instead.
To run Platypus on our clusters:
#!/bin/bash
#SBATCH -A myallocation # Allocation name
#SBATCH -t 1:00:00
#SBATCH -N 1
#SBATCH -n 1
#SBATCH --job-name=platypus
#SBATCH --mail-type=FAIL,BEGIN,END
#SBATCH --error=%x-%J-%u.err
#SBATCH --output=%x-%J-%u.out
module --force purge
ml biocontainers platypus
plink
Link to section 'Introduction' of 'plink' Introduction
Plink
is a free, open-source whole genome association analysis toolset, designed to perform a range of basic, large-scale analyses in a computationally efficient manner.
For more information, please check its website: https://biocontainers.pro/tools/plink and its home page: https://zzz.bwh.harvard.edu/plink/.
Link to section 'Versions' of 'plink' Versions
- 1.90b6.21
Link to section 'Commands' of 'plink' Commands
- plink
- prettify
Link to section 'Module' of 'plink' Module
You can load the modules by:
module load biocontainers
module load plink
Link to section 'Example job' of 'plink' Example job
Using #!/bin/sh -l
as shebang in the slurm job script will cause the failure of some biocontainer modules. Please use #!/bin/bash
instead.
To run Plink on our clusters:
#!/bin/bash
#SBATCH -A myallocation # Allocation name
#SBATCH -t 1:00:00
#SBATCH -N 1
#SBATCH -n 1
#SBATCH --job-name=plink
#SBATCH --mail-type=FAIL,BEGIN,END
#SBATCH --error=%x-%J-%u.err
#SBATCH --output=%x-%J-%u.out
module --force purge
ml biocontainers plink
plink --file toy --freq --out toy_analysis
plink2
Link to section 'Introduction' of 'plink2' Introduction
Plink2
is a whole genome association analysis toolset.
For more information, please check its website: https://biocontainers.pro/tools/plink2 and its home page on Github.
Link to section 'Versions' of 'plink2' Versions
- 2.00a2.3
Link to section 'Commands' of 'plink2' Commands
- plink2
Link to section 'Module' of 'plink2' Module
You can load the modules by:
module load biocontainers
module load plink2
Link to section 'Example job' of 'plink2' Example job
Using #!/bin/sh -l
as shebang in the slurm job script will cause the failure of some biocontainer modules. Please use #!/bin/bash
instead.
To run Plink2 on our clusters:
#!/bin/bash
#SBATCH -A myallocation # Allocation name
#SBATCH -t 1:00:00
#SBATCH -N 1
#SBATCH -n 1
#SBATCH --job-name=plink2
#SBATCH --mail-type=FAIL,BEGIN,END
#SBATCH --error=%x-%J-%u.err
#SBATCH --output=%x-%J-%u.out
module --force purge
ml biocontainers plink2
plink2 --bfile HapMap_3_r3_1 --freq --out HapMap_3_r3_1_out
plotsr
Link to section 'Introduction' of 'plotsr' Introduction
Plotsr generates high-quality visualisation of synteny and structural rearrangements between multiple genomes. For this, it uses the genomic structural annotations between multiple chromosome-level assemblies.
Home page: https://github.com/schneebergerlab/plotsr
Link to section 'Versions' of 'plotsr' Versions
- 0.5.4
Link to section 'Commands' of 'plotsr' Commands
- plotsr
Link to section 'Module' of 'plotsr' Module
You can load the modules by:
module load biocontainers
module load plotsr
Link to section 'Example job' of 'plotsr' Example job
Using #!/bin/sh -l
as shebang in the slurm job script will cause the failure of some biocontainer modules. Please use #!/bin/bash
instead.
To run plotsr on our clusters:
#!/bin/bash
#SBATCH -A myallocation # Allocation name
#SBATCH -t 1:00:00
#SBATCH -N 1
#SBATCH -n 1
#SBATCH --job-name=plotsr
#SBATCH --mail-type=FAIL,BEGIN,END
#SBATCH --error=%x-%J-%u.err
#SBATCH --output=%x-%J-%u.out
module --force purge
ml biocontainers plotsr
plotsr syri.out refgenome qrygenome -H 8 -W 5
pomoxis
Link to section 'Introduction' of 'pomoxis' Introduction
Pomoxis comprises a set of basic bioinformatic tools tailored to nanopore sequencing. Notably tools are included for generating and analyzing draft assemblies. Many of these tools are used by the research data analysis group at Oxford Nanopore Technologies.
Docker hub: https://hub.docker.com/r/zeunas/pomoxis
Home page: https://github.com/nanoporetech/pomoxis
Link to section 'Versions' of 'pomoxis' Versions
- 0.3.9
Link to section 'Commands' of 'pomoxis' Commands
- assess_assembly
- catalogue_errors
- common_errors_from_bam
- coverage_from_bam
- coverage_from_fastx
- fast_convert
- find_indels
- intersect_assembly_errors
- long_fastx
- mini_align
- mini_assemble
- pomoxis_path
- qscores_from_summary
- ref_seqs_from_bam
- reverse_bed
- split_fastx
- stats_from_bam
- subsample_bam
- summary_from_stats
- tag_bam
- trim_alignments
Link to section 'Module' of 'pomoxis' Module
You can load the modules by:
module load biocontainers
module load pomoxis
Link to section 'Example job' of 'pomoxis' Example job
Using #!/bin/sh -l
as shebang in the slurm job script will cause the failure of some biocontainer modules. Please use #!/bin/bash
instead.
To run pomoxis on our clusters:
#!/bin/bash
#SBATCH -A myallocation # Allocation name
#SBATCH -t 1:00:00
#SBATCH -N 1
#SBATCH -n 4
#SBATCH --job-name=pomoxis
#SBATCH --mail-type=FAIL,BEGIN,END
#SBATCH --error=%x-%J-%u.err
#SBATCH --output=%x-%J-%u.out
module --force purge
ml biocontainers pomoxis
assess_assembly \
-i helen_output/Staph_Aur_draft_helen.fa \
-r truth_assembly_staph_aur.fasta \
-p polished_assembly_quality \
-l 50 \
-t 4 \
-e \
-T
poppunk
Link to section 'Introduction' of 'poppunk' Introduction
PopPUNK is a tool for clustering genomes. We refer to the clusters as variable-length-k-mer clusters, or VLKCs. Biologically, these clusters typically represent distinct strains. We refer to subclusters of strains as lineages.
Docker hub: https://hub.docker.com/r/staphb/poppunk
Home page: https://github.com/bacpop/PopPUNK
Link to section 'Versions' of 'poppunk' Versions
- 2.5.0
- 2.6.0
Link to section 'Commands' of 'poppunk' Commands
- poppunk
- poppunk_add_weights.py
- poppunk_assign
- poppunk_batch_mst.py
- poppunk_calculate_rand_indices.py
- poppunk_calculate_silhouette.py
- poppunk_easy_run.py
- poppunk_extract_components.py
- poppunk_extract_distances.py
- poppunk_info
- poppunk_iterate.py
- poppunk_mandrake
- poppunk_mst
- poppunk_references
- poppunk_visualise
Link to section 'Module' of 'poppunk' Module
You can load the modules by:
module load biocontainers
module load poppunk
Link to section 'Example job' of 'poppunk' Example job
Using #!/bin/sh -l
as shebang in the slurm job script will cause the failure of some biocontainer modules. Please use #!/bin/bash
instead.
To run poppunk on our clusters:
#!/bin/bash
#SBATCH -A myallocation # Allocation name
#SBATCH -t 1:00:00
#SBATCH -N 1
#SBATCH -n 1
#SBATCH --job-name=poppunk
#SBATCH --mail-type=FAIL,BEGIN,END
#SBATCH --error=%x-%J-%u.err
#SBATCH --output=%x-%J-%u.out
module --force purge
ml biocontainers poppunk
popscle
Link to section 'Introduction' of 'popscle' Introduction
Popscle
is a suite of population scale analysis tools for single-cell genomics data.
Docker hub: https://hub.docker.com/r/cumulusprod/popscle and its home page on Github.
Link to section 'Versions' of 'popscle' Versions
- 0.1b
Link to section 'Commands' of 'popscle' Commands
- popscle
Link to section 'Module' of 'popscle' Module
You can load the modules by:
module load biocontainers
module load popscle
Link to section 'Example job' of 'popscle' Example job
Using #!/bin/sh -l
as shebang in the slurm job script will cause the failure of some biocontainer modules. Please use #!/bin/bash
instead.
To run Popscle on our clusters:
#!/bin/bash
#SBATCH -A myallocation # Allocation name
#SBATCH -t 1:00:00
#SBATCH -N 1
#SBATCH -n 1
#SBATCH --job-name=popscle
#SBATCH --mail-type=FAIL,BEGIN,END
#SBATCH --error=%x-%J-%u.err
#SBATCH --output=%x-%J-%u.out
module --force purge
ml biocontainers popscle
popscle dsc-pileup --sam data/$bam --vcf data/$ref_vcf --out data/$pileup
pplacer
Link to section 'Introduction' of 'pplacer' Introduction
Pplacer places query sequences on a fixed reference phylogenetic tree to maximize phylogenetic likelihood or posterior probability according to a reference alignment, guppy does all of the downstream analysis of placements, and rppr does useful things having to do with reference packages.
For more information, please check:
BioContainers: https://biocontainers.pro/tools/pplacer
Home page: https://matsen.fhcrc.org/pplacer/
Link to section 'Versions' of 'pplacer' Versions
- 1.1.alpha19
Link to section 'Commands' of 'pplacer' Commands
- pplacer
- guppy
- rppr
Link to section 'Module' of 'pplacer' Module
You can load the modules by:
module load biocontainers
module load pplacer
Link to section 'Example job' of 'pplacer' Example job
Using #!/bin/sh -l
as shebang in the slurm job script will cause the failure of some biocontainer modules. Please use #!/bin/bash
instead.
To run pplacer on our clusters:
#!/bin/bash
#SBATCH -A myallocation # Allocation name
#SBATCH -t 1:00:00
#SBATCH -N 1
#SBATCH -n 1
#SBATCH --job-name=pplacer
#SBATCH --mail-type=FAIL,BEGIN,END
#SBATCH --error=%x-%J-%u.err
#SBATCH --output=%x-%J-%u.out
module --force purge
ml biocontainers pplacer
prinseq
Link to section 'Introduction' of 'prinseq' Introduction
Prinseq
is a tool that generates summary statistics of sequence and quality data and that is used to filter, reformat and trim next-generation sequence data.
For more information, please check its website: https://biocontainers.pro/tools/prinseq and its home page: http://prinseq.sourceforge.net.
Link to section 'Versions' of 'prinseq' Versions
- 0.20.4
Link to section 'Commands' of 'prinseq' Commands
- prinseq-graphs-noPCA.pl
- prinseq-graphs.pl
- prinseq-lite.pl
Link to section 'Module' of 'prinseq' Module
You can load the modules by:
module load biocontainers
module load prinseq
Link to section 'Example job' of 'prinseq' Example job
Using #!/bin/sh -l
as shebang in the slurm job script will cause the failure of some biocontainer modules. Please use #!/bin/bash
instead.
To run Prinseq on our clusters:
#!/bin/bash
#SBATCH -A myallocation # Allocation name
#SBATCH -t 1:00:00
#SBATCH -N 1
#SBATCH -n 1
#SBATCH --job-name=prinseq
#SBATCH --mail-type=FAIL,BEGIN,END
#SBATCH --error=%x-%J-%u.err
#SBATCH --output=%x-%J-%u.out
module --force purge
ml biocontainers prinseq
prinseq-lite.pl -verbose -fastq SRR5043021_1.fastq -fastq2 SRR5043021_2.fastq -graph_data test.gd -out_good null -out_bad null
prinseq-graphs.pl -i test.gd -png_all -o test
prinseq-graphs-noPCA.pl -i test.gd -png_all -o test_noPCA
prodigal
Link to section 'Introduction' of 'prodigal' Introduction
Prodigal
is a tool for fast, reliable protein-coding gene prediction for prokaryotic genome.
For more information, please check its website: https://biocontainers.pro/tools/prodigal and its home page on Github.
Link to section 'Versions' of 'prodigal' Versions
- 2.6.3
Link to section 'Commands' of 'prodigal' Commands
- prodigal
Link to section 'Module' of 'prodigal' Module
You can load the modules by:
module load biocontainers
module load prodigal
Link to section 'Example job' of 'prodigal' Example job
Using #!/bin/sh -l
as shebang in the slurm job script will cause the failure of some biocontainer modules. Please use #!/bin/bash
instead.
To run Prodigal on our clusters:
#!/bin/bash
#SBATCH -A myallocation # Allocation name
#SBATCH -t 1:00:00
#SBATCH -N 1
#SBATCH -n 1
#SBATCH --job-name=prodigal
#SBATCH --mail-type=FAIL,BEGIN,END
#SBATCH --error=%x-%J-%u.err
#SBATCH --output=%x-%J-%u.out
module --force purge
ml biocontainers prodigal
prodigal -i genome.fasta -o output.genes -a proteins.faa
prokka
Prokka
is a pipeline for rapidly annotating prokaryotic genomes. It produces GFF3, GBK and SQN files that are ready for editing in Sequin and ultimately submitted to Genbank/DDJB/ENA.
Detailed usage can be found here: https://github.com/tseemann/prokka
Link to section 'Versions' of 'prokka' Versions
- 1.14.6
Link to section 'Commands' of 'prokka' Commands
- prokka
- prokka-abricate_to_fasta_db
- prokka-biocyc_to_fasta_db
- prokka-build_kingdom_dbs
- prokka-cdd_to_hmm
- prokka-clusters_to_hmm
- prokka-genbank_to_fasta_db
- prokka-genpept_to_fasta_db
- prokka-hamap_to_hmm
- prokka-tigrfams_to_hmm
- prokka-uniprot_to_fasta_db
Link to section 'Module' of 'prokka' Module
You can load the modules by:
module load biocontainers
module load prokka
Link to section 'Example job' of 'prokka' Example job
Using #!/bin/sh -l
as shebang in the slurm job script will cause the failure of some biocontainer modules. Please use #!/bin/bash
instead.
To run prokka on our our clusters:
#!/bin/bash
#SBATCH -A myallocation # Allocation name
#SBATCH -t 20:00:00
#SBATCH -N 1
#SBATCH -n 24
#SBATCH --job-name=prokka
#SBATCH --mail-type=FAIL,BEGIN,END
#SBATCH --error=%x-%J-%u.err
#SBATCH --output=%x-%J-%u.out
module --force purge
ml biocontainers prokka
prokka --compliant --centre UoN --outdir PRJEB12345 --locustag EHEC --prefix EHEC-Chr1 contigs.fa --cpus 24
prokka-genbank_to_fasta_db Coccus1.gbk Coccus2.gbk Coccus3.gbk Coccus4.gbk > Coccus.faa
proteinortho
Link to section 'Introduction' of 'proteinortho' Introduction
Proteinortho
is a tool to detect orthologous genes within different species.
For more information, please check its website: https://biocontainers.pro/tools/proteinortho and its home page on Gitlab.
Link to section 'Versions' of 'proteinortho' Versions
- 6.0.33
Link to section 'Commands' of 'proteinortho' Commands
- proteinortho
- proteinortho2html.pl
- proteinortho2tree.pl
- proteinortho2xml.pl
- proteinortho6.pl
- proteinortho_cleanupblastgraph
- proteinortho_clustering
- proteinortho_compareProteinorthoGraphs.pl
- proteinortho_do_mcl.pl
- proteinortho_extract_from_graph.pl
- proteinortho_ffadj_mcs.py
- proteinortho_formatUsearch.pl
- proteinortho_grab_proteins.pl
- proteinortho_graphMinusRemovegraph
- proteinortho_history.pl
- proteinortho_singletons.pl
- proteinortho_summary.pl
- proteinortho_treeBuilderCore
Link to section 'Module' of 'proteinortho' Module
You can load the modules by:
module load biocontainers
module load proteinortho
Link to section 'Example job' of 'proteinortho' Example job
Using #!/bin/sh -l
as shebang in the slurm job script will cause the failure of some biocontainer modules. Please use #!/bin/bash
instead.
To run Proteinortho on our clusters:
#!/bin/bash
#SBATCH -A myallocation # Allocation name
#SBATCH -t 1:00:00
#SBATCH -N 1
#SBATCH -n 1
#SBATCH --job-name=proteinortho
#SBATCH --mail-type=FAIL,BEGIN,END
#SBATCH --error=%x-%J-%u.err
#SBATCH --output=%x-%J-%u.out
module --force purge
ml biocontainers proteinortho
proteinortho6.pl test/C.faa test/E.faa test/L.faa test/M.faa
prothint
Link to section 'Introduction' of 'prothint' Introduction
ProtHint
is a pipeline for predicting and scoring hints (in the form of introns, start and stop codons) in the genome of interest by mapping and spliced aligning predicted genes to a database of reference protein sequences.
Link to section 'Versions' of 'prothint' Versions
- 2.6.0
Commands
- cds_with_upstream_support.py
- combine_gff_records.pl
- count_cds_overlaps.py
- flag_top_proteins.py
- gff_from_region_to_contig.pl
- make_chains.py
- nucseq_for_selected_genes.pl
- print_high_confidence.py
- print_longest_isoform.py
- proteins_from_gtf.pl
- prothint.py
- prothint2augustus.py
- run_spliced_alignment.pl
- run_spliced_alignment_pbs.pl
- select_best_proteins.py
- select_for_next_iteration.py
- spalnBatch.sh
- spaln_to_gff.py
Academic license
ProtHint depends on GenMark. To use GeneMark, users need to download license files by yourself.
Go to the GeneMark web site: http://exon.gatech.edu/GeneMark/license_download.cgi. Check the boxes for GeneMark-ES/ET/EP ver 4.69_lic
and LINUX 64
next to it, fill out the form, then click "I agree". In the next page, right click and copy the link addresses for 64 bit
licenss. Paste the link addresses in the commands below:
cd $HOME
wget "replace with license URL"
zcat gm_key_64.gz > .gm_key
Link to section 'Module' of 'prothint' Module
You can load the modules by:
module load biocontainers
module load prothint
Link to section 'Example job' of 'prothint' Example job
Using #!/bin/sh -l
as shebang in the slurm job script will cause the failure of some biocontainer modules. Please use #!/bin/bash
instead.
To run ProtHint on our cluster:
#!/bin/bash
#SBATCH -A myallocation # Allocation name
#SBATCH -t 1:00:00
#SBATCH -N 1
#SBATCH -n 4
#SBATCH --job-name=prothint
#SBATCH --mail-type=FAIL,BEGIN,END
#SBATCH --error=%x-%J-%u.err
#SBATCH --output=%x-%J-%u.out
module --force purge
ml biocontainers prothint
prothint.py --threads 4 input/genome.fasta input/proteins.fasta --geneSeeds input/genemark.gtf --workdir test
pullseq
Link to section 'Introduction' of 'pullseq' Introduction
Pullseq is an utility program for extracting sequences from a fasta/fastq file.
BioContainers: https://biocontainers.pro/tools/pullseq
Home page: https://github.com/bcthomas/pullseq
Link to section 'Versions' of 'pullseq' Versions
- 1.0.2
Link to section 'Commands' of 'pullseq' Commands
- pcre-config
- pcregrep
- pcretest
- pullseq
- seqdiff
Link to section 'Module' of 'pullseq' Module
You can load the modules by:
module load biocontainers
module load pullseq
Link to section 'Example job' of 'pullseq' Example job
Using #!/bin/sh -l
as shebang in the slurm job script will cause the failure of some biocontainer modules. Please use #!/bin/bash
instead.
To run pullseq on our clusters:
#!/bin/bash
#SBATCH -A myallocation # Allocation name
#SBATCH -t 1:00:00
#SBATCH -N 1
#SBATCH -n 1
#SBATCH --job-name=pullseq
#SBATCH --mail-type=FAIL,BEGIN,END
#SBATCH --error=%x-%J-%u.err
#SBATCH --output=%x-%J-%u.out
module --force purge
ml biocontainers pullseq
purge_dups
Link to section 'Introduction' of 'purge_dups' Introduction
purge_dups is designed to remove haplotigs and contig overlaps in a de novo assembly based on read depth.
BioContainers: https://biocontainers.pro/tools/purge_dups
Home page: https://github.com/dfguan/purge_dups
Link to section 'Versions' of 'purge_dups' Versions
- 1.2.6
Link to section 'Commands' of 'purge_dups' Commands
- augustify.py
- bamToWig.py
- cleanup-blastdb-volumes.py
- edirect.py
- executeTestCGP.py
- extractAnno.py
- findRepetitiveProtSeqs.py
- fix_in_frame_stop_codon_genes.py
- generate_plot.py
- getAnnoFastaFromJoingenes.py
- hist_plot.py
- pd_config.py
- run_abundance.py
- run_purge_dups.py
- run_sepp.py
- run_tipp.py
- run_tipp_tool.py
- run_upp.py
- split_sequences.py
- stringtie2fa.py
- sumlabels.py
- sumtrees.py
Link to section 'Module' of 'purge_dups' Module
You can load the modules by:
module load biocontainers
module load purge_dups
Link to section 'Example job' of 'purge_dups' Example job
Using #!/bin/sh -l
as shebang in the slurm job script will cause the failure of some biocontainer modules. Please use #!/bin/bash
instead.
To run purge_dups on our clusters:
#!/bin/bash
#SBATCH -A myallocation # Allocation name
#SBATCH -t 1:00:00
#SBATCH -N 1
#SBATCH -n 1
#SBATCH --job-name=purge_dups
#SBATCH --mail-type=FAIL,BEGIN,END
#SBATCH --error=%x-%J-%u.err
#SBATCH --output=%x-%J-%u.out
module --force purge
ml biocontainers purge_dups
pvactools
Link to section 'Introduction' of 'pvactools' Introduction
pVACtools is a cancer immunotherapy tools suite consisting of pVACseq, pVACbind, pVACfuse, pVACvector, and pVACview.
Docker hub: https://hub.docker.com/r/griffithlab/pvactools/
Home page: https://pvactools.readthedocs.io/en/latest/
Link to section 'Versions' of 'pvactools' Versions
- 3.0.1
Link to section 'Commands' of 'pvactools' Commands
- pvacbind
- pvacfuse
- pvacseq
- pvactools
- pvacvector
- pvacview
Link to section 'Module' of 'pvactools' Module
You can load the modules by:
module load biocontainers
module load pvactools
Link to section 'Example job' of 'pvactools' Example job
Using #!/bin/sh -l
as shebang in the slurm job script will cause the failure of some biocontainer modules. Please use #!/bin/bash
instead.
To run pvactools on our clusters:
#!/bin/bash
#SBATCH -A myallocation # Allocation name
#SBATCH -t 1:00:00
#SBATCH -N 1
#SBATCH -n 1
#SBATCH --job-name=pvactools
#SBATCH --mail-type=FAIL,BEGIN,END
#SBATCH --error=%x-%J-%u.err
#SBATCH --output=%x-%J-%u.out
module --force purge
ml biocontainers pvactools
pvacseq download_example_data .
pvacseq run \
pvacseq_example_data/input.vcf \
Test \
HLA-A*02:01,HLA-B*35:01,DRB1*11:01 \
MHCflurry MHCnuggetsI MHCnuggetsII NNalign NetMHC PickPocket SMM SMMPMBEC SMMalign \
pvacseq_output_data \
-e1 8,9,10 \
-e2 15 \
--iedb-install-directory /opt/iedb
pyani
Link to section 'Introduction' of 'pyani' Introduction
Pyani
is an application and Python module for whole-genome classification of microbes using Average Nucleotide Identity.
For more information, please check its website: https://biocontainers.pro/tools/pyani and its home page on Github.
Link to section 'Versions' of 'pyani' Versions
- 0.2.11
- 0.2.12
Link to section 'Commands' of 'pyani' Commands
- average_nucleotide_identity.py
- genbank_get_genomes_by_taxon.py
- delta_filter_wrapper.py
Link to section 'Module' of 'pyani' Module
You can load the modules by:
module load biocontainers
module load pyani
Link to section 'Example job' of 'pyani' Example job
Using #!/bin/sh -l
as shebang in the slurm job script will cause the failure of some biocontainer modules. Please use #!/bin/bash
instead.
To run Pyani on our clusters:
#!/bin/bash
#SBATCH -A myallocation # Allocation name
#SBATCH -t 1:00:00
#SBATCH -N 1
#SBATCH -n 1
#SBATCH --job-name=pyani
#SBATCH --mail-type=FAIL,BEGIN,END
#SBATCH --error=%x-%J-%u.err
#SBATCH --output=%x-%J-%u.out
module --force purge
ml biocontainers pyani
average_nucleotide_identity.py -i tests/ -o tests/test_ANIm_output -m ANIm -g
average_nucleotide_identity.py -i tests/ -o tests/test_ANIb_output -m ANIb -g
average_nucleotide_identity.py -i tests/ -o tests/test_ANIblastall_output -m ANIblastall -g
average_nucleotide_identity.py -i tests/ -o tests/test_TETRA_output -m TETRA -g
pybedtools
Link to section 'Introduction' of 'pybedtools' Introduction
Pybedtools
wraps and extends BEDTools and offers feature-level manipulations from within Python.
For more information, please check its website: https://biocontainers.pro/tools/pybedtools and its home page on Github.
Link to section 'Versions' of 'pybedtools' Versions
- 0.9.0
Link to section 'Commands' of 'pybedtools' Commands
- python
- python3
Link to section 'Module' of 'pybedtools' Module
You can load the modules by:
module load biocontainers
module load pybedtools
Link to section 'Example job' of 'pybedtools' Example job
Using #!/bin/sh -l
as shebang in the slurm job script will cause the failure of some biocontainer modules. Please use #!/bin/bash
instead.
To run Pybedtools on our clusters:
#!/bin/bash
#SBATCH -A myallocation # Allocation name
#SBATCH -t 1:00:00
#SBATCH -N 1
#SBATCH -n 1
#SBATCH --job-name=pybedtools
#SBATCH --mail-type=FAIL,BEGIN,END
#SBATCH --error=%x-%J-%u.err
#SBATCH --output=%x-%J-%u.out
module --force purge
ml biocontainers pybedtools
pybigwig
Link to section 'Introduction' of 'pybigwig' Introduction
Pybigwig
is a python extension, written in C, for quick access to bigBed files and access to and creation of bigWig files.
For more information, please check its website: https://biocontainers.pro/tools/pybigwig and its home page on Github.
Link to section 'Versions' of 'pybigwig' Versions
- 0.3.18
Link to section 'Commands' of 'pybigwig' Commands
- python
- python3
Link to section 'Module' of 'pybigwig' Module
You can load the modules by:
module load biocontainers
module load pybigwig
Link to section 'Interactive job' of 'pybigwig' Interactive job
To run pybigwig interactively on our clusters:
(base) UserID@bell-fe00:~ $ sinteractive -N1 -n12 -t4:00:00 -A myallocation
salloc: Granted job allocation 12345869
salloc: Waiting for resource configuration
salloc: Nodes bell-a008 are ready for job
(base) UserID@bell-a008:~ $ module load biocontainers pybigwig
(base) UserID@bell-a008:~ $ python
Python 3.6.15 | packaged by conda-forge | (default, Dec 3 2021, 18:49:41)
[GCC 9.4.0] on linux
Type "help", "copyright", "credits" or "license" for more information.
>>> import pyBigWig
>>> bw = pyBigWig.open("test/test.bw")
Link to section 'Batch job' of 'pybigwig' Batch job
Using #!/bin/sh -l
as shebang in the slurm job script will cause the failure of some biocontainer modules. Please use #!/bin/bash
instead.
To run batch jobs on our clusters:
#!/bin/bash
#SBATCH -A myallocation # Allocation name
#SBATCH -t 1:00:00
#SBATCH -N 1
#SBATCH -n 1
#SBATCH --job-name=pybigwig
#SBATCH --mail-type=FAIL,BEGIN,END
#SBATCH --error=%x-%J-%u.err
#SBATCH --output=%x-%J-%u.out
module --force purge
ml biocontainers pybigwig
python script.py
pychopper
Link to section 'Introduction' of 'pychopper' Introduction
Pychopper is a tool to identify, orient and trim full-length Nanopore cDNA reads. The tool is also able to rescue fused reads.
BioContainers: https://biocontainers.pro/tools/pychopper
Home page: https://github.com/nanoporetech/pychopper
Link to section 'Versions' of 'pychopper' Versions
- 2.5.0
Link to section 'Commands' of 'pychopper' Commands
- cdna_classifier.py
Link to section 'Module' of 'pychopper' Module
You can load the modules by:
module load biocontainers
module load pychopper
Link to section 'Example job' of 'pychopper' Example job
Using #!/bin/sh -l
as shebang in the slurm job script will cause the failure of some biocontainer modules. Please use #!/bin/bash
instead.
To run pychopper on our clusters:
#!/bin/bash
#SBATCH -A myallocation # Allocation name
#SBATCH -t 1:00:00
#SBATCH -N 1
#SBATCH -n 1
#SBATCH --job-name=pychopper
#SBATCH --mail-type=FAIL,BEGIN,END
#SBATCH --error=%x-%J-%u.err
#SBATCH --output=%x-%J-%u.out
module --force purge
ml biocontainers pychopper
pycoqc
Link to section 'Introduction' of 'pycoqc' Introduction
Pycoqc
is a tool that computes metrics and generates interactive QC plots for Oxford Nanopore technologies sequencing data.
For more information, please check its website: https://biocontainers.pro/tools/pycoqc and its home page on Github.
Link to section 'Versions' of 'pycoqc' Versions
- 2.5.2
Link to section 'Commands' of 'pycoqc' Commands
- pycoQC
- python
- python3
Link to section 'Module' of 'pycoqc' Module
You can load the modules by:
module load biocontainers
module load pycoqc
Link to section 'Example job' of 'pycoqc' Example job
Using #!/bin/sh -l
as shebang in the slurm job script will cause the failure of some biocontainer modules. Please use #!/bin/bash
instead.
To run Pycoqc on our clusters:
#!/bin/bash
#SBATCH -A myallocation # Allocation name
#SBATCH -t 1:00:00
#SBATCH -N 1
#SBATCH -n 1
#SBATCH --job-name=pycoqc
#SBATCH --mail-type=FAIL,BEGIN,END
#SBATCH --error=%x-%J-%u.err
#SBATCH --output=%x-%J-%u.out
module --force purge
ml biocontainers pycoqc
pycoQC \
-f Albacore-1.2.1_basecall-1D-DNA_sequencing_summary.txt\
-o Albacore-1.2.1_basecall-1D-DNA.html \
--quiet
pyensembl
Link to section 'Introduction' of 'pyensembl' Introduction
Pyensembl
is a Python interface to Ensembl reference genome metadata such as exons and transcripts.
For more information, please check its website: https://biocontainers.pro/tools/pyensembl and its home page on Github.
Link to section 'Versions' of 'pyensembl' Versions
- 1.9.4
Link to section 'Commands' of 'pyensembl' Commands
- pyensembl
- python
- python3
Link to section 'Module' of 'pyensembl' Module
You can load the modules by:
module load biocontainers
module load pyensembl
Link to section 'Example job' of 'pyensembl' Example job
Using #!/bin/sh -l
as shebang in the slurm job script will cause the failure of some biocontainer modules. Please use #!/bin/bash
instead.
To run Pyensembl on our clusters:
#!/bin/bash
#SBATCH -A myallocation # Allocation name
#SBATCH -t 1:00:00
#SBATCH -N 1
#SBATCH -n 1
#SBATCH --job-name=pyensembl
#SBATCH --mail-type=FAIL,BEGIN,END
#SBATCH --error=%x-%J-%u.err
#SBATCH --output=%x-%J-%u.out
module --force purge
ml biocontainers pyensembl
pyfaidx
Link to section 'Introduction' of 'pyfaidx' Introduction
Pyfaidx
is a Python package for random access and indexing of fasta files.
For more information, please check its website: https://biocontainers.pro/tools/pyfaidx and its home page on Github.
Link to section 'Versions' of 'pyfaidx' Versions
- 0.6.4
Link to section 'Commands' of 'pyfaidx' Commands
- python
- python3
Link to section 'Module' of 'pyfaidx' Module
You can load the modules by:
module load biocontainers
module load pyfaidx
Link to section 'Example job' of 'pyfaidx' Example job
Using #!/bin/sh -l
as shebang in the slurm job script will cause the failure of some biocontainer modules. Please use #!/bin/bash
instead.
To run Pyfaidx on our clusters:
#!/bin/bash
#SBATCH -A myallocation # Allocation name
#SBATCH -t 1:00:00
#SBATCH -N 1
#SBATCH -n 1
#SBATCH --job-name=pyfaidx
#SBATCH --mail-type=FAIL,BEGIN,END
#SBATCH --error=%x-%J-%u.err
#SBATCH --output=%x-%J-%u.out
module --force purge
ml biocontainers pyfaidx
pygenometracks
Link to section 'Introduction' of 'pygenometracks' Introduction
pyGenomeTracks aims to produce high-quality genome browser tracks that are highly customizable.
BioContainers: https://biocontainers.pro/tools/pygenometracks
Home page: https://github.com/deeptools/pyGenomeTracks
Link to section 'Versions' of 'pygenometracks' Versions
- 3.7
Link to section 'Commands' of 'pygenometracks' Commands
- make_tracks_file
- pyGenomeTracks
Link to section 'Module' of 'pygenometracks' Module
You can load the modules by:
module load biocontainers
module load pygenometracks
Link to section 'Example job' of 'pygenometracks' Example job
Using #!/bin/sh -l
as shebang in the slurm job script will cause the failure of some biocontainer modules. Please use #!/bin/bash
instead.
To run pygenometracks on our clusters:
#!/bin/bash
#SBATCH -A myallocation # Allocation name
#SBATCH -t 1:00:00
#SBATCH -N 1
#SBATCH -n 1
#SBATCH --job-name=pygenometracks
#SBATCH --mail-type=FAIL,BEGIN,END
#SBATCH --error=%x-%J-%u.err
#SBATCH --output=%x-%J-%u.out
module --force purge
ml biocontainers pygenometracks
make_tracks_file --trackFiles domains.bed bigwig.bw -o tracks.ini
pyGenomeTracks --tracks tracks.ini \
--region chr2:10,000,000-11,000,000 --outFileName nice_image.pdf
pygenomeviz
Link to section 'Introduction' of 'pygenomeviz' Introduction
pyGenomeViz is a genome visualization python package for comparative genomics implemented based on matplotlib.
Docker hub: https://hub.docker.com/r/staphb/pygenomeviz
Home page: https://github.com/moshi4/pyGenomeViz#cli-examples
Link to section 'Versions' of 'pygenomeviz' Versions
- 0.2.2
Link to section 'Commands' of 'pygenomeviz' Commands
- pgv-download-dataset
- pgv-mmseqs
- pgv-mummer
- pgv-pmauve
- python
- python3
Link to section 'Module' of 'pygenomeviz' Module
You can load the modules by:
module load biocontainers
module load pygenomeviz
Link to section 'Example job' of 'pygenomeviz' Example job
Using #!/bin/sh -l
as shebang in the slurm job script will cause the failure of some biocontainer modules. Please use #!/bin/bash
instead.
To run pygenomeviz on our clusters:
#!/bin/bash
#SBATCH -A myallocation # Allocation name
#SBATCH -t 1:00:00
#SBATCH -N 1
#SBATCH -n 1
#SBATCH --job-name=pygenomeviz
#SBATCH --mail-type=FAIL,BEGIN,END
#SBATCH --error=%x-%J-%u.err
#SBATCH --output=%x-%J-%u.out
module --force purge
ml biocontainers pygenomeviz
pyranges
Link to section 'Introduction' of 'pyranges' Introduction
Pyranges
are collections of intervals that support comparison operations (like overlap and intersect) and other methods that are useful for genomic analyses.
For more information, please check its website: https://biocontainers.pro/tools/pyranges and its home page on Github.
Link to section 'Versions' of 'pyranges' Versions
- 0.0.115
Link to section 'Commands' of 'pyranges' Commands
- python
- python3
Link to section 'Module' of 'pyranges' Module
You can load the modules by:
module load biocontainers
module load pyranges
Link to section 'Example job' of 'pyranges' Example job
Using #!/bin/sh -l
as shebang in the slurm job script will cause the failure of some biocontainer modules. Please use #!/bin/bash
instead.
To run Pyranges on our clusters:
#!/bin/bash
#SBATCH -A myallocation # Allocation name
#SBATCH -t 1:00:00
#SBATCH -N 1
#SBATCH -n 1
#SBATCH --job-name=pyranges
#SBATCH --mail-type=FAIL,BEGIN,END
#SBATCH --error=%x-%J-%u.err
#SBATCH --output=%x-%J-%u.out
module --force purge
ml biocontainers pyranges
pysam
Link to section 'Introduction' of 'pysam' Introduction
Pysam
is a python module that makes it easy to read and manipulate mapped short read sequence data stored in SAM/BAM files.
For more information, please check its website: https://biocontainers.pro/tools/pysam and its home page on Github.
Link to section 'Versions' of 'pysam' Versions
- 0.18.0
Link to section 'Commands' of 'pysam' Commands
- python
- python3
Link to section 'Module' of 'pysam' Module
You can load the modules by:
module load biocontainers
module load pysam
Link to section 'Example job' of 'pysam' Example job
Using #!/bin/sh -l
as shebang in the slurm job script will cause the failure of some biocontainer modules. Please use #!/bin/bash
instead.
To run Pysam on our clusters:
#!/bin/bash
#SBATCH -A myallocation # Allocation name
#SBATCH -t 1:00:00
#SBATCH -N 1
#SBATCH -n 1
#SBATCH --job-name=pysam
#SBATCH --mail-type=FAIL,BEGIN,END
#SBATCH --error=%x-%J-%u.err
#SBATCH --output=%x-%J-%u.out
module --force purge
ml biocontainers pysam
pyvcf3
Link to section 'Introduction' of 'pyvcf3' Introduction
PyVCF3 has been created because the Official PyVCF repository is no longer maintained and do not accept any pull requests.
BioContainers: https://biocontainers.pro/tools/pyvcf3
Home page: https://github.com/dridk/PyVCF3
Link to section 'Versions' of 'pyvcf3' Versions
- 1.0.3
Link to section 'Commands' of 'pyvcf3' Commands
- python
- python3
Link to section 'Module' of 'pyvcf3' Module
You can load the modules by:
module load biocontainers
module load pyvcf3
Link to section 'Example job' of 'pyvcf3' Example job
Using #!/bin/sh -l
as shebang in the slurm job script will cause the failure of some biocontainer modules. Please use #!/bin/bash
instead.
To run pyvcf3 on our clusters:
#!/bin/bash
#SBATCH -A myallocation # Allocation name
#SBATCH -t 1:00:00
#SBATCH -N 1
#SBATCH -n 1
#SBATCH --job-name=pyvcf3
#SBATCH --mail-type=FAIL,BEGIN,END
#SBATCH --error=%x-%J-%u.err
#SBATCH --output=%x-%J-%u.out
module --force purge
ml biocontainers pyvcf3
qiime2
Link to section 'Introduction' of 'qiime2' Introduction
QIIME 2
is a is a powerful, extensible, and decentralized microbiome analysis package with a focus on data and analysis transparency. QIIME 2 enables researchers to start an analysis with raw DNA sequence data and finish with publication-quality figures and statistical results.
For more information, please check its website: https://quay.io/repository/qiime2/core and its home page: https://qiime2.org/.
Link to section 'Versions' of 'qiime2' Versions
- 2021.2
- 2022.11
- 2022.2
- 2022.8
- 2023.2
- 2023.5
- 2023.7
Link to section 'Commands' of 'qiime2' Commands
- biom
- qiime
- python
- python3
Link to section 'Module' of 'qiime2' Module
You can load the modules by:
module load biocontainers
module load qiime2
Link to section 'Example job' of 'qiime2' Example job
Using #!/bin/sh -l
as shebang in the slurm job script will cause the failure of some biocontainer modules. Please use #!/bin/bash
instead.
To run QIIME 2 on our clusters:
#!/bin/bash
#SBATCH -A myallocation # Allocation name
#SBATCH -t 1:00:00
#SBATCH -N 1
#SBATCH -n 1
#SBATCH --job-name=qiime2
#SBATCH --mail-type=FAIL,BEGIN,END
#SBATCH --error=%x-%J-%u.err
#SBATCH --output=%x-%J-%u.out
module --force purge
ml biocontainers qiime2
qiime metadata tabulate \
--m-input-file rep-seqs.qza \
--m-input-file taxonomy.qza \
--o-visualization tabulated-feature-metadata.qzv
qtlseq
Link to section 'Introduction' of 'qtlseq' Introduction
Bulked segregant analysis, as implemented in QTL-seq (Takagi et al., 2013), is a powerful and efficient method to identify agronomically important loci in crop plants. QTL-seq was adapted from MutMap to identify quantitative trait loci. It utilizes sequences pooled from two segregating progeny populations with extreme opposite traits (e.g. resistant vs susceptible) and a single whole-genome resequencing of either of the parental cultivars.
BioContainers: https://biocontainers.pro/tools/qtlseq
Home page: https://github.com/YuSugihara/QTL-seq#What-is-QTL-seq
Link to section 'Versions' of 'qtlseq' Versions
- 2.2.3
Link to section 'Commands' of 'qtlseq' Commands
- qtlseq
Link to section 'Module' of 'qtlseq' Module
You can load the modules by:
module load biocontainers
module load qtlseq
Link to section 'Example job' of 'qtlseq' Example job
Using #!/bin/sh -l
as shebang in the slurm job script will cause the failure of some biocontainer modules. Please use #!/bin/bash
instead.
To run qtlseq on our clusters:
#!/bin/bash
#SBATCH -A myallocation # Allocation name
#SBATCH -t 1:00:00
#SBATCH -N 1
#SBATCH -n 1
#SBATCH --job-name=qtlseq
#SBATCH --mail-type=FAIL,BEGIN,END
#SBATCH --error=%x-%J-%u.err
#SBATCH --output=%x-%J-%u.out
module --force purge
ml biocontainers qtlseq
qualimap
Link to section 'Introduction' of 'qualimap' Introduction
Qualimap
is a platform-independent application written in Java and R that provides both a Graphical User Interface (GUI) and a command-line interface to facilitate the quality control of alignment sequencing data and its derivatives like feature counts.
For more information, please check its website: https://biocontainers.pro/tools/qualimap and its home page: http://qualimap.conesalab.org.
Link to section 'Versions' of 'qualimap' Versions
- 2.2.1
Link to section 'Commands' of 'qualimap' Commands
- qualimap
Link to section 'Module' of 'qualimap' Module
You can load the modules by:
module load biocontainers
module load qualimap
Link to section 'Example job' of 'qualimap' Example job
Using #!/bin/sh -l
as shebang in the slurm job script will cause the failure of some biocontainer modules. Please use #!/bin/bash
instead.
To run Qualimap on our clusters:
#!/bin/bash
#SBATCH -A myallocation # Allocation name
#SBATCH -t 1:00:00
#SBATCH -N 1
#SBATCH -n 1
#SBATCH --job-name=qualimap
#SBATCH --mail-type=FAIL,BEGIN,END
#SBATCH --error=%x-%J-%u.err
#SBATCH --output=%x-%J-%u.out
module --force purge
ml biocontainers qualimap
quast
Link to section 'Introduction' of 'quast' Introduction
Quast
is Quality Assessment Tool for Genome Assemblies.
Note: Running QUAST, please use the command: quast.py metaquast.py'
For more information, please check its website: https://biocontainers.pro/tools/quast and its home page on Github.
Link to section 'Versions' of 'quast' Versions
- 5.0.2
- 5.2.0
Link to section 'Commands' of 'quast' Commands
- quast.py
- metaquast.py
Link to section 'Module' of 'quast' Module
You can load the modules by:
module load biocontainers
module load quast
Link to section 'Example job' of 'quast' Example job
Using #!/bin/sh -l
as shebang in the slurm job script will cause the failure of some biocontainer modules. Please use #!/bin/bash
instead.
To run Quast on our clusters:
#!/bin/bash
#SBATCH -A myallocation # Allocation name
#SBATCH -t 1:00:00
#SBATCH -N 1
#SBATCH -n 8
#SBATCH --job-name=quast
#SBATCH --mail-type=FAIL,BEGIN,END
#SBATCH --error=%x-%J-%u.err
#SBATCH --output=%x-%J-%u.out
module --force purge
ml biocontainers quast
metaquast.py --gene-finding --threads 8 \
meta_contigs_1.fasta meta_contigs_2.fasta \
-r meta_ref_1.fasta,meta_ref_2.fasta,meta_ref_3.fasta \
-o quast_out_genefinding
quickmirseq
Link to section 'Introduction' of 'quickmirseq' Introduction
QuickMIRSeq
is an integrated pipeline for quick and accurate quantification of known miRNAs and isomiRs by jointly processing multiple samples.
Docker hub: https://hub.docker.com/r/gcfntnu/quickmirseq and its home page on Github.
Link to section 'Versions' of 'quickmirseq' Versions
- 1.0
Link to section 'Commands' of 'quickmirseq' Commands
- perl
- QuickMIRSeq-report.sh
Link to section 'Module' of 'quickmirseq' Module
You can load the modules by:
module load biocontainers
module load quickmirseq
This module defines program installation directory (note: inside the container!) as environment variable $QuickMIRSeq
. Once again, this is not a host path, this path is only available from inside the container.
With the way this module is organized, you should be able to use the variable freely for both the perl $QuickMIRSeq/QuickMIRSeq.pl allIDs.txt run.config
and the $QuickMIRSeq/QuickMIRSeq-report.sh
steps as directed by the user guide.
A simple QuickMIRSeq.pl
and QuickMIRSeq-report.sh
will also work (and can be a backup if the variable expansion somehow does not work for you).
You will also need a run configuration file. You can copy from an existing one, or take from the user guide, or as a last resort, use Singularity to copy the template (in $QuickMIRSeq/run.config.template
) from inside the container image. singularity shell
may be an easiest way for the latter.
Link to section 'Example job' of 'quickmirseq' Example job
Using #!/bin/sh -l
as shebang in the slurm job script will cause the failure of some biocontainer modules. Please use #!/bin/bash
instead.
To run QuickMIRSeq on our clusters:
#!/bin/bash
#SBATCH -A myallocation # Allocation name
#SBATCH -t 1:00:00
#SBATCH -N 1
#SBATCH -n 1
#SBATCH --job-name=quickmirseq
#SBATCH --mail-type=FAIL,BEGIN,END
#SBATCH --error=%x-%J-%u.err
#SBATCH --output=%x-%J-%u.out
module --force purge
ml biocontainers quickmirseq
quickmerge -d out.rq.delta -q q.fasta -r scab8722.fasta -hco 5.0 -c 1.5 -l n -ml m -p prefix
r
Link to section 'Introduction' of 'r' Introduction
R
is a system for statistical computation and graphics.
This is a plain R-base installation (see https://github.com/rocker-org/rocker/) repackaged by RCAC with an addition of a handful prerequisite libraries (libcurl, libopenssl, libxml2, libcairo2 and libXt) and their header files.
Docker hub: https://hub.docker.com/_/r-base and its home page: https://www.r-project.org/.
Link to section 'Versions' of 'r' Versions
- 4.1.1
Link to section 'Commands' of 'r' Commands
- R
- Rscript
Link to section 'Module' of 'r' Module
You can load the modules by:
module load biocontainers
module load r
Link to section 'Example job' of 'r' Example job
Using #!/bin/sh -l
as shebang in the slurm job script will cause the failure of some biocontainer modules. Please use #!/bin/bash
instead.
To run R on our clusters:
#!/bin/bash
#SBATCH -A myallocation # Allocation name
#SBATCH -t 1:00:00
#SBATCH -N 1
#SBATCH -n 1
#SBATCH --job-name=r
#SBATCH --mail-type=FAIL,BEGIN,END
#SBATCH --error=%x-%J-%u.err
#SBATCH --output=%x-%J-%u.out
module --force purge
ml biocontainers r
r-rnaseq
r-rnaseq
is a customerized R module based on R/4.1.1
used for RNAseq analysis.
In the module, we have some packages installed:
- BiocManager 1.30.16
- ComplexHeatmap 2.9.4
- DESeq2 1.34.0
- edgeR 3.36.0
- pheatmap 1.0.12
- limma 3.48.3
- tibble 3.1.5
- tidyr 1.1.4
- readr 2.0.2
- readxl 1.3.1
- purrr 0.3.4
- dplyr 1.0.7
- stringr 1.4.0
- forcats 0.5.1
- ggplot2 3.3.5
- openxlsx 4.2.5
Link to section 'Versions' of 'r-rnaseq' Versions
- 4.1.1-1
- 4.1.1-1-rstudio
Link to section 'Commands' of 'r-rnaseq' Commands
- R
- Rscript
- rstudio (only for the rstudio version)
Link to section 'Module' of 'r-rnaseq' Module
You can load the modules by:
module load biocontainers
module load r-rnaseq/4.1.1-1
# If you want to use Rstudio, load the rstudio version
module load r-rnaseq/4.1.1-1-rstudio
Install packages
Users can also install packages they need. The installed location depends on the setting in your ~/.Rprofile
.
Detailed guide about installing R packages can be found here: https://www.rcac.purdue.edu/knowledge/bell/run/examples/apps/r/package.
Link to section 'Interactive job' of 'r-rnaseq' Interactive job
To run interactively on our clusters:
(base) UserID@bell-fe00:~ $ sinteractive -N1 -n12 -t4:00:00 -A myallocation
salloc: Granted job allocation 12345869
salloc: Waiting for resource configuration
salloc: Nodes bell-a008 are ready for job
(base) UserID@bell-a008:~ $ module load biocontainers r-rnaseq/4.1.1-1 # or r-rnaseq/4.1.1-1-rstudio
(base) UserID@bell-a008:~ $ R
R version 4.1.1 (2021-08-10) -- "Kick Things"
Copyright (C) 2021 The R Foundation for Statistical Computing
Platform: x86_64-pc-linux-gnu (64-bit)
R is free software and comes with ABSOLUTELY NO WARRANTY.
You are welcome to redistribute it under certain conditions.
Type 'license()' or 'licence()' for distribution details.
Natural language support but running in an English locale
R is a collaborative project with many contributors.
Type 'contributors()' for more information and
'citation()' on how to cite R or R packages in publications.
Type 'demo()' for some demos, 'help()' for on-line help, or
'help.start()' for an HTML browser interface to help.
Type 'q()' to quit R.
> library(edgeR)
> library(pheatmap)
Link to section 'Batch job' of 'r-rnaseq' Batch job
Using #!/bin/sh -l
as shebang in the slurm job script will cause the failure of some biocontainer modules. Please use #!/bin/bash
instead.
To submit a sbatch job on our clusters:
#!/bin/bash
#SBATCH -A myallocation # Allocation name
#SBATCH -t 10:00:00
#SBATCH -N 1
#SBATCH -n 24
#SBATCH --job-name=r_RNAseq
#SBATCH --mail-type=FAIL,BEGIN,END
#SBATCH --error=%x-%J-%u.err
#SBATCH --output=%x-%J-%u.out
module --force purge
ml biocontainers r-rnaseq
Rscript RNAseq.R
r-rstudio
Link to section 'Introduction' of 'r-rstudio' Introduction
RStudio
is an integrated development environment (IDE) for the R statistical computation and graphics system.
This is an RStudio IDE together with a plain R-base installation (see https://github.com/rocker-org/rocker/), repackaged by RCAC with an addition of a handful prerequisite libraries (libcurl, libopenssl, libxml2, libcairo2 and libXt) and their header files. It is intentionally separate from the biocontainers' 'r' module for reasons of image size (700MB vs 360MB).
Docker hub: https://hub.docker.com/_/r-base and its home page: https://www.rstudio.com/products/rstudio/ and https://www.r-project.org/.
Link to section 'Versions' of 'r-rstudio' Versions
- 4.1.1
Link to section 'Commands' of 'r-rstudio' Commands
- R
- Rscript
- rstudio
Link to section 'Module' of 'r-rstudio' Module
You can load the modules by:
module load biocontainers
module load r-studio
Link to section 'Example job' of 'r-rstudio' Example job
Using #!/bin/sh -l
as shebang in the slurm job script will cause the failure of some biocontainer modules. Please use #!/bin/bash
instead.
To run RStudio on our clusters:
#!/bin/bash
#SBATCH -A myallocation # Allocation name
#SBATCH -t 1:00:00
#SBATCH -N 1
#SBATCH -n 1
#SBATCH --job-name=r-studio
#SBATCH --mail-type=FAIL,BEGIN,END
#SBATCH --error=%x-%J-%u.err
#SBATCH --output=%x-%J-%u.out
module --force purge
ml biocontainers r-studio
r-scrnaseq
r-scrnaseq
is a customerized R module based on R/4.1.1
or R/4.2.0
used for scRNAseq analysis.
In the module, we have some packages installed:
- BiocManager 1.30.16
- CellChat 1.6.1
- ProjecTILs 3.0
- Seurat 4.1.0
- SeuratObject 4.0.4
- SeuratWrappers 0.3.0
- monocle3 1.0.0
- SnapATAC 1.0.0
- SingleCellExperiment 1.14.1, 1.16.0
- scDblFinder 1.8.0
- SingleR 1.8.1
- scCATCH 3.0
- scMappR 1.0.7
- rliger 1.0.0
- schex 1.8.0
- CoGAPS 3.14.0
- celldex 1.4.0
- dittoSeq 1.6.0
- DropletUtils 1.14.2
- miQC 1.2.0
- Nebulosa 1.4.0
- tricycle 1.2.0
- pheatmap 1.0.12
- limma 3.48.3, 3.50.0
- tibble 3.1.5
- tidyr 1.1.4
- readr 2.0.2
- readxl 1.3.1
- purrr 0.3.4
- dplyr 1.0.7
- stringr 1.4.0
- forcats 0.5.1
- ggplot2 3.3.5
- openxlsx 4.2.5
Link to section 'Versions' of 'r-scrnaseq' Versions
- 4.1.1-1
- 4.1.1-1-rstudio
- 4.2.0
- 4.2.0-rstudio
- r-scrnaseq/4.2.3-rstudio
Link to section 'Commands' of 'r-scrnaseq' Commands
- R
- Rscript
- rstudio (only for the rstudio version)
Link to section 'Module' of 'r-scrnaseq' Module
You can load the modules by:
module load biocontainers
module load r-scrnaseq
# or module load r-scrnaseq/4.2.0
# If you want to use Rstudio, load the rstudio version
module load r-scrnaseq/4.1.1-1-rstudio
# or module load r-scrnaseq/4.2.0-rstudio
Install packages
Users can also install packages they need. The installed location depends on the setting in your ~/.Rprofile
.
Detailed guide about installing R packages can be found here: https://www.rcac.purdue.edu/knowledge/bell/run/examples/apps/r/package.
Link to section 'Interactive job' of 'r-scrnaseq' Interactive job
To run interactively on our clusters:
(base) UserID@bell-fe00:~ $ sinteractive -N1 -n12 -t4:00:00 -A myallocation
salloc: Granted job allocation 12345869
salloc: Waiting for resource configuration
salloc: Nodes bell-a008 are ready for job
(base) UserID@bell-a008:~ $ module load biocontainers r-scrnaseq/4.2.0 # or r-scrnaseq/4.2.0-rstudio
(base) UserID@bell-a008:~ $ R
R version 4.2.0 (2022-04-22) -- "Vigorous Calisthenics"
Copyright (C) 2022 The R Foundation for Statistical Computing
Platform: x86_64-pc-linux-gnu (64-bit)
R is free software and comes with ABSOLUTELY NO WARRANTY.
You are welcome to redistribute it under certain conditions.
Type 'license()' or 'licence()' for distribution details.
Natural language support but running in an English locale
R is a collaborative project with many contributors.
Type 'contributors()' for more information and
'citation()' on how to cite R or R packages in publications.
Type 'demo()' for some demos, 'help()' for on-line help, or
'help.start()' for an HTML browser interface to help.
Type 'q()' to quit R.
> library(Seurat)
> library(monocle3)
Link to section 'Batch job' of 'r-scrnaseq' Batch job
Using #!/bin/sh -l
as shebang in the slurm job script will cause the failure of some biocontainer modules. Please use #!/bin/bash
instead.
To submit a sbatch job on our clusters:
#!/bin/bash
#SBATCH -A myallocation # Allocation name
#SBATCH -t 10:00:00
#SBATCH -N 1
#SBATCH -n 24
#SBATCH --job-name=r_scRNAseq
#SBATCH --mail-type=FAIL,BEGIN,END
#SBATCH --error=%x-%J-%u.err
#SBATCH --output=%x-%J-%u.out
module --force purge
ml biocontainers r-scrnaseq
Rscript scRNAseq.R
racon
Link to section 'Introduction' of 'racon' Introduction
Racon
is a consensus module for raw de novo DNA assembly of long uncorrected reads.
For more information, please check its website: https://biocontainers.pro/tools/racon and its home page on Github.
Link to section 'Versions' of 'racon' Versions
- 1.4.20
- 1.5.0
Link to section 'Commands' of 'racon' Commands
- racon
Link to section 'Module' of 'racon' Module
You can load the modules by:
module load biocontainers
module load racon
Link to section 'Example job' of 'racon' Example job
Using #!/bin/sh -l
as shebang in the slurm job script will cause the failure of some biocontainer modules. Please use #!/bin/bash
instead.
To run Racon on our clusters:
#!/bin/bash
#SBATCH -A myallocation # Allocation name
#SBATCH -t 1:00:00
#SBATCH -N 1
#SBATCH -n 1
#SBATCH --job-name=racon
#SBATCH --mail-type=FAIL,BEGIN,END
#SBATCH --error=%x-%J-%u.err
#SBATCH --output=%x-%J-%u.out
module --force purge
ml biocontainers racon
ragout
Link to section 'Introduction' of 'ragout' Introduction
Ragout
is a tool for chromosome-level scaffolding using multiple references.
For more information, please check its website: https://biocontainers.pro/tools/ragout and its home page on Github.
Link to section 'Versions' of 'ragout' Versions
- 2.3
Link to section 'Commands' of 'ragout' Commands
- ragout
Link to section 'Module' of 'ragout' Module
You can load the modules by:
module load biocontainers
module load ragout
Link to section 'Example job' of 'ragout' Example job
Using #!/bin/sh -l
as shebang in the slurm job script will cause the failure of some biocontainer modules. Please use #!/bin/bash
instead.
To run Ragout on our clusters:
#!/bin/bash
#SBATCH -A myallocation # Allocation name
#SBATCH -t 1:00:00
#SBATCH -N 1
#SBATCH -n 1
#SBATCH --job-name=ragout
#SBATCH --mail-type=FAIL,BEGIN,END
#SBATCH --error=%x-%J-%u.err
#SBATCH --output=%x-%J-%u.out
module --force purge
ml biocontainers ragout
ragtag
Link to section 'Introduction' of 'ragtag' Introduction
Ragtag
is a tool for fast reference-guided genome assembly scaffolding.
For more information, please check its website: https://biocontainers.pro/tools/ragtag and its home page on Github.
Link to section 'Versions' of 'ragtag' Versions
- 2.1.0
Link to section 'Commands' of 'ragtag' Commands
- ragtag.py
Link to section 'Module' of 'ragtag' Module
You can load the modules by:
module load biocontainers
module load ragtag
Link to section 'Example job' of 'ragtag' Example job
Using #!/bin/sh -l
as shebang in the slurm job script will cause the failure of some biocontainer modules. Please use #!/bin/bash
instead.
To run Ragtag on our clusters:
#!/bin/bash
#SBATCH -A myallocation # Allocation name
#SBATCH -t 1:00:00
#SBATCH -N 1
#SBATCH -n 1
#SBATCH --job-name=ragtag
#SBATCH --mail-type=FAIL,BEGIN,END
#SBATCH --error=%x-%J-%u.err
#SBATCH --output=%x-%J-%u.out
module --force purge
ml biocontainers ragtag
ragtag.py correct ref.fasta query.fasta
ragtag.py patch target.fa query.fa
rapmap
Link to section 'Introduction' of 'rapmap' Introduction
RapMap is a testing ground for ideas in quasi-mapping and selective alignment.
BioContainers: https://biocontainers.pro/tools/rapmap
Home page: https://github.com/COMBINE-lab/RapMap
Link to section 'Versions' of 'rapmap' Versions
- 0.6.0
Link to section 'Commands' of 'rapmap' Commands
- rapmap
Link to section 'Module' of 'rapmap' Module
You can load the modules by:
module load biocontainers
module load rapmap
Link to section 'Example job' of 'rapmap' Example job
Using #!/bin/sh -l
as shebang in the slurm job script will cause the failure of some biocontainer modules. Please use #!/bin/bash
instead.
To run rapmap on our clusters:
#!/bin/bash
#SBATCH -A myallocation # Allocation name
#SBATCH -t 1:00:00
#SBATCH -N 1
#SBATCH -n 1
#SBATCH --job-name=rapmap
#SBATCH --mail-type=FAIL,BEGIN,END
#SBATCH --error=%x-%J-%u.err
#SBATCH --output=%x-%J-%u.out
module --force purge
ml biocontainers rapmap
rasusa
Link to section 'Introduction' of 'rasusa' Introduction
Rasusa: Randomly subsample sequencing reads to a specified coverage.
Docker hub: https://hub.docker.com/r/staphb/rasusa
Home page: https://github.com/mbhall88/rasusa
Link to section 'Versions' of 'rasusa' Versions
- 0.6.0
- 0.7.0
Link to section 'Commands' of 'rasusa' Commands
- rasusa
Link to section 'Module' of 'rasusa' Module
You can load the modules by:
module load biocontainers
module load rasusa
Link to section 'Example job' of 'rasusa' Example job
Using #!/bin/sh -l
as shebang in the slurm job script will cause the failure of some biocontainer modules. Please use #!/bin/bash
instead.
To run rasusa on our clusters:
#!/bin/bash
#SBATCH -A myallocation # Allocation name
#SBATCH -t 1:00:00
#SBATCH -N 1
#SBATCH -n 1
#SBATCH --job-name=rasusa
#SBATCH --mail-type=FAIL,BEGIN,END
#SBATCH --error=%x-%J-%u.err
#SBATCH --output=%x-%J-%u.out
module --force purge
ml biocontainers rasusa
rasusa -i seq_1.fq -i seq_2.fq \
--coverage 100 --genome-size 35mb \
-o out.r1.fq -o out.r2.fq
raven-assembler
Link to section 'Introduction' of 'raven-assembler' Introduction
Raven-assembler
is a de novo genome assembler for long uncorrected reads.
For more information, please check its website: https://biocontainers.pro/tools/raven-assembler and its home page on Github.
Link to section 'Versions' of 'raven-assembler' Versions
- 1.8.1
Link to section 'Commands' of 'raven-assembler' Commands
- raven
Link to section 'Module' of 'raven-assembler' Module
You can load the modules by:
module load biocontainers
module load raven-assembler
Link to section 'Example job' of 'raven-assembler' Example job
Using #!/bin/sh -l
as shebang in the slurm job script will cause the failure of some biocontainer modules. Please use #!/bin/bash
instead.
To run Raven-assembler on our clusters:
#!/bin/bash
#SBATCH -A myallocation # Allocation name
#SBATCH -t 1:00:00
#SBATCH -N 1
#SBATCH -n 12
#SBATCH --job-name=raven-assembler
#SBATCH --mail-type=FAIL,BEGIN,END
#SBATCH --error=%x-%J-%u.err
#SBATCH --output=%x-%J-%u.out
module --force purge
ml biocontainers raven-assembler
raven -t 12 input.fastq
raxml
Link to section 'Introduction' of 'raxml' Introduction
Raxml
(Randomized Axelerated Maximum Likelihood) is a program for the Maximum Likelihood-based inference of large phylogenetic trees.
For more information, please check its website: https://biocontainers.pro/tools/raxml and its home page: https://cme.h-its.org/exelixis/web/software/raxml/.
Link to section 'Versions' of 'raxml' Versions
- 8.2.12
Link to section 'Commands' of 'raxml' Commands
- raxmlHPC
- raxmlHPC-AVX2
- raxmlHPC-PTHREADS
- raxmlHPC-PTHREADS-AVX2
- raxmlHPC-PTHREADS-SSE3
- raxmlHPC-SSE3
Link to section 'Module' of 'raxml' Module
You can load the modules by:
module load biocontainers
module load raxml
Link to section 'Example job' of 'raxml' Example job
Using #!/bin/sh -l
as shebang in the slurm job script will cause the failure of some biocontainer modules. Please use #!/bin/bash
instead.
To run Raxml on our clusters:
#!/bin/bash
#SBATCH -A myallocation # Allocation name
#SBATCH -t 1:00:00
#SBATCH -N 1
#SBATCH -n 36
#SBATCH --job-name=raxml
#SBATCH --mail-type=FAIL,BEGIN,END
#SBATCH --error=%x-%J-%u.err
#SBATCH --output=%x-%J-%u.out
module --force purge
ml biocontainers raxml
raxmlHPC-SSE3 -m GTRGAMMA -p 12345 -s input.fasta -n HPC-SSE3_out -# 20 -T 36
raxmlHPC -m GTRGAMMA -p 12345 -s input.fasta -n HPC_out -# 20 -T 36
raxmlHPC-AVX2 -m GTRGAMMA -p 12345 -s input.fasta -n HPC-AVX2_out -# 20 -T 36
raxmlHPC-PTHREADS -m GTRGAMMA -p 12345 -s input.fasta -n HPC-PTHREADS_out -# 20 -T 36
raxmlHPC-PTHREADS-AVX2 -m GTRGAMMA -p 12345 -s input.fasta -n HPC-PTHREADS-AVX2_out -# 20 -T 36
raxmlHPC-PTHREADS-SSE3 -m GTRGAMMA -p 12345 -s input.fasta -n HPC-PTHREADS-SSE3_out -# 20 -T 36
raxml-ng
Link to section 'Introduction' of 'raxml-ng' Introduction
Raxml-ng
is a phylogenetic tree inference tool which uses maximum-likelihood (ML) optimality criterion.
For more information, please check its website: https://biocontainers.pro/tools/raxml-ng and its home page on Github.
Link to section 'Versions' of 'raxml-ng' Versions
- 1.1.0
Link to section 'Commands' of 'raxml-ng' Commands
- raxml-ng
- raxml-ng-mpi
- mpirun
- mpiexec
Link to section 'Module' of 'raxml-ng' Module
You can load the modules by:
module load biocontainers
module load raxml-ng
Link to section 'Example job' of 'raxml-ng' Example job
Using #!/bin/sh -l
as shebang in the slurm job script will cause the failure of some biocontainer modules. Please use #!/bin/bash
instead.
To run Raxml-ng on our clusters:
#!/bin/bash
#SBATCH -A myallocation # Allocation name
#SBATCH -t 1:00:00
#SBATCH -N 1
#SBATCH -n 1
#SBATCH --job-name=raxml-ng
#SBATCH --mail-type=FAIL,BEGIN,END
#SBATCH --error=%x-%J-%u.err
#SBATCH --output=%x-%J-%u.out
module --force purge
ml biocontainers raxml-ng
raxml-ng --bootstrap --msa alignment.phy \
--model GTR+G --threads 12 --bs-trees 1000
reapr
Link to section 'Introduction' of 'reapr' Introduction
Reapr is a tool that evaluates the accuracy of a genome assembly using mapped paired end reads.
BioContainers: https://biocontainers.pro/tools/reapr
Home page: https://www.sanger.ac.uk/tool/reapr/
Link to section 'Notes provided by Neelam Jha' of 'reapr' Notes provided by Neelam Jha
https://bioinformaticsonline.com/bookmarks/view/26925/reapr-a-universal-tool-for-genome-assembly-evaluation
Reapr is a tool trying to find explicit errors in the assembly based on incongruently mapped reads. It is heavily based on too low span coverage, or reads mapping too far or too close to each other. The program will also break up contigs/scaffolds at spurious sites to form smaller (but hopefully correct) contigs. Reapr runs pretty slowly, sadly,
Reapr is a bit fuzzy with contig names, but luckily it’s given us a tool to check if things are ok before we proceed! The command reapr facheck <assembly.fasta>
will tell you if everything’s ok! in this case, no output is good output, since the only output from the command is the potential problems with the contig names. If you run into any problems, run reapr facheck <assembly.fasta> <renamed_assembly.fasta>
, and you will get an assembly file with renamed contigs.
Once the names are ok, we continue:
The first thing we reapr needs, is a list of all “perfect” reads. This is reads that have a perfect map to the reference. Reapr is finicky though, and can’t use libraries with different read lengths, so you’ll have to use assemblies based on the raw data for this. Run the command reapr perfectmap
to get information on how to create a perfect mapping file, and create a perfect mapping called <assembler>_perfect
.
The next tool we need is reapr smaltmap
which creates a bam file of read-pair mappings. Do the same thing you did with perfectmap and create an output file called <assembler>_smalt.bam
.
Finally we can use the smalt mapping, and the perfect mapping to run the reapr pipeline
. Run reapr pipeline
to get help on how to run, and then run the pipeline. Store the results in reapr_<assembler>
.
Link to section 'Versions' of 'reapr' Versions
- 1.0.18
Link to section 'Commands' of 'reapr' Commands
- reapr
Link to section 'Module' of 'reapr' Module
You can load the modules by:
module load biocontainers
module load reapr
Link to section 'Example job' of 'reapr' Example job
Using #!/bin/sh -l
as shebang in the slurm job script will cause the failure of some biocontainer modules. Please use #!/bin/bash
instead.
To run reapr on our clusters:
#!/bin/bash
#SBATCH -A myallocation # Allocation name
#SBATCH -t 1:00:00
#SBATCH -N 1
#SBATCH -n 1
#SBATCH --job-name=reapr
#SBATCH --mail-type=FAIL,BEGIN,END
#SBATCH --error=%x-%J-%u.err
#SBATCH --output=%x-%J-%u.out
module --force purge
ml biocontainers reapr
reapr facheck Assembly.fasta renamedAssembly.fasta
reapr perfectmap renamedAssembly.fasta reads_1.fastq reads_2.fastq 100 outputPrefix
reapr smaltmap renamedAssembly.fasta reads_1.fastq reads_2.fastq mapped.bam
reapr pipeline renamedAssembly.fasta mapped.bam pipeoutdir outputPrefix
rebaler
Link to section 'Introduction' of 'rebaler' Introduction
Rebaler
is a program for conducting reference-based assemblies using long reads.
For more information, please check its website: https://biocontainers.pro/tools/rebaler and its home page on Github.
Link to section 'Versions' of 'rebaler' Versions
- 0.2.0
Link to section 'Commands' of 'rebaler' Commands
- rebaler
Link to section 'Module' of 'rebaler' Module
You can load the modules by:
module load biocontainers
module load rebaler
Link to section 'Example job' of 'rebaler' Example job
Using #!/bin/sh -l
as shebang in the slurm job script will cause the failure of some biocontainer modules. Please use #!/bin/bash
instead.
To run Rebaler on our clusters:
#!/bin/bash
#SBATCH -A myallocation # Allocation name
#SBATCH -t 1:00:00
#SBATCH -N 1
#SBATCH -n 1
#SBATCH --job-name=rebaler
#SBATCH --mail-type=FAIL,BEGIN,END
#SBATCH --error=%x-%J-%u.err
#SBATCH --output=%x-%J-%u.out
module --force purge
ml biocontainers rebaler
reciprocal_smallest_distance
Link to section 'Introduction' of 'reciprocal_smallest_distance' Introduction
The reciprocal smallest distance
(RSD) algorithm accurately infers orthologs between pairs of genomes by considering global sequence alignment and maximum likelihood evolutionary distance between sequences.
For more information, please check its home page on Github.
Link to section 'Versions' of 'reciprocal_smallest_distance' Versions
- 1.1.7
Link to section 'Commands' of 'reciprocal_smallest_distance' Commands
- rsd_search
- rsd_blast
- rsd_format
Link to section 'Module' of 'reciprocal_smallest_distance' Module
You can load the modules by:
module load biocontainers
module load reciprocal_smallest_distance
Link to section 'Example job' of 'reciprocal_smallest_distance' Example job
Using #!/bin/sh -l
as shebang in the slurm job script will cause the failure of some biocontainer modules. Please use #!/bin/bash
instead.
To run Reciprocal Smallest Distance on our clusters:
#!/bin/bash
#SBATCH -A myallocation # Allocation name
#SBATCH -t 1:00:00
#SBATCH -N 1
#SBATCH -n 1
#SBATCH --job-name=reciprocal_smallest_distance
#SBATCH --mail-type=FAIL,BEGIN,END
#SBATCH --error=%x-%J-%u.err
#SBATCH --output=%x-%J-%u.out
module --force purge
ml biocontainers reciprocal_smallest_distance
rsd_search
-q Mycoplasma_genitalium.aa \
--subject-genome=Mycobacterium_leprae.aa \
-o Mycoplasma_genitalium.aa_Mycobacterium_leprae.aa_0.8_1e-5.orthologs.txt
rsd_format -g Mycoplasma_genitalium.aa
rsd_blast -v -q Mycoplasma_genitalium.aa \
--subject-genome=Mycobacterium_leprae.aa \
--forward-hits q_s.hits --reverse-hits s_q.hits \
--no-format --evalue 0.1
recycler
Link to section 'Introduction' of 'recycler' Introduction
Recycler
is a tool designed for extracting circular sequences from de novo assembly graphs.
For more information, please check its website: https://biocontainers.pro/tools/recycler and its home page on Github.
Link to section 'Versions' of 'recycler' Versions
- 0.7
Link to section 'Commands' of 'recycler' Commands
- make_fasta_from_fastg.py
- get_simple_cycs.py
- recycle.py
Link to section 'Module' of 'recycler' Module
You can load the modules by:
module load biocontainers
module load recycler
Link to section 'Example job' of 'recycler' Example job
Using #!/bin/sh -l
as shebang in the slurm job script will cause the failure of some biocontainer modules. Please use #!/bin/bash
instead.
To run Recycler on our clusters:
#!/bin/bash
#SBATCH -A myallocation # Allocation name
#SBATCH -t 1:00:00
#SBATCH -N 1
#SBATCH -n 1
#SBATCH --job-name=recycler
#SBATCH --mail-type=FAIL,BEGIN,END
#SBATCH --error=%x-%J-%u.err
#SBATCH --output=%x-%J-%u.out
module --force purge
ml biocontainers recycler
recycle.py -g test/assembly_graph.fastg \
-k 55 -b test/test.sort.bam -i True
regtools
Link to section 'Introduction' of 'regtools' Introduction
Regtools are tools that integrate DNA-seq and RNA-seq data to help interpret mutations in a regulatory and splicing context.
Docker hub: https://hub.docker.com/r/griffithlab/regtools/
Home page: https://github.com/griffithlab/regtools
Link to section 'Versions' of 'regtools' Versions
- 1.0.0
Link to section 'Commands' of 'regtools' Commands
- regtools
Link to section 'Module' of 'regtools' Module
You can load the modules by:
module load biocontainers
module load regtools
Link to section 'Example job' of 'regtools' Example job
Using #!/bin/sh -l
as shebang in the slurm job script will cause the failure of some biocontainer modules. Please use #!/bin/bash
instead.
To run regtools on our clusters:
#!/bin/bash
#SBATCH -A myallocation # Allocation name
#SBATCH -t 1:00:00
#SBATCH -N 1
#SBATCH -n 1
#SBATCH --job-name=regtools
#SBATCH --mail-type=FAIL,BEGIN,END
#SBATCH --error=%x-%J-%u.err
#SBATCH --output=%x-%J-%u.out
module --force purge
ml biocontainers regtools
repeatmasker
Link to section 'Introduction' of 'repeatmasker' Introduction
RepeatMakser
is a program that screens DNA sequences for interspersed repeats and low complexity DNA sequences.
Detailed usage can be found here: http://www.repeatmasker.org.
Link to section 'Versions' of 'repeatmasker' Versions
- 4.1.2
Commands
- RepeatMasker
Database
As of May 20, 2019 GIRI has rescinded the working agreement allowing the www.repeatmasker.org website to offer a repeatmasking service utilizing the RepBase RepeatMasker Edition library. As a result, repeatmasker can only offer masking using the open database Dfam, which starting in 3.0 includes consensus sequences in addition to profile hidden Markov models for many transposable element families. Users requiring RepBase will need to purchase a commercial or academic license from GIRI and run RepeatMasker localy.
In our cluster, we set up the Dfam release 3.5 (October 2021) that include 285,580 repetitive DNA families.
Species name
Since v4.1.1, RepeatMakser has switched to the FamDB format for the Dfam database. Due to this change, RepeatMasker becomes more strict with regards to what is acceptable for the -species
flag. The commonly used names such as "mammal" and "mouse" will not be accepted. To check for valid names, you can query the database using the python script famdb.py
(https://github.com/Dfam-consortium/FamDB).
See famdb.py --help
for usage information and below for an example the check the valid name for "mammal" using our copy of the Dfam database:
/depot/itap/datasets/Maker/RepeatMasker/Libraries/famdb.py -i /depot/itap/datasets/Maker/RepeatMasker/Libraries/Dfam.h5 names mammal
Link to section 'Module' of 'repeatmasker' Module
You can load the modules by:
module load biocontainers
module load repeatmasker/4.1.2
Link to section 'Example job' of 'repeatmasker' Example job
Using #!/bin/sh -l
as shebang in the slurm job script will cause the failure of some biocontainer modules. Please use #!/bin/bash
instead.
To run RepeatMasker on our cluster:
#!/bin/bash
#SBATCH -A myallocation # Allocation name
#SBATCH -t 2:00:00
#SBATCH -N 1
#SBATCH -n 24
#SBATCH --job-name=repeatmsker
#SBATCH --mail-type=FAIL,BEGIN,END
#SBATCH --error=%x-%J-%u.err
#SBATCH --output=%x-%J-%u.out
module --force purge
ml biocontainers repeatmasker/4.1.2
RepeatMasker -pa 24 -species mammals genome.fasta
repeatmodeler
Link to section 'Introduction' of 'repeatmodeler' Introduction
RepeatModeler
is a de novo transposable element (TE) family identification and modeling package.
For more information, please check its website: https://biocontainers.pro/tools/repeatmodeler and its home page: http://www.repeatmasker.org/RepeatModeler/.
Link to section 'Versions' of 'repeatmodeler' Versions
- 2.0.2
- 2.0.3
Link to section 'Commands' of 'repeatmodeler' Commands
- RepeatModeler
- BuildDatabase
- RepeatClassifier
Link to section 'Module' of 'repeatmodeler' Module
You can load the modules by:
module load biocontainers
module load repeatmodeler
Link to section 'Example job' of 'repeatmodeler' Example job
Using #!/bin/sh -l
as shebang in the slurm job script will cause the failure of some biocontainer modules. Please use #!/bin/bash
instead.
To run RepeatModeler on our clusters:
#!/bin/bash
#SBATCH -A myallocation # Allocation name
#SBATCH -t 1:00:00
#SBATCH -N 1
#SBATCH -n 1
#SBATCH --job-name=repeatmodeler
#SBATCH --mail-type=FAIL,BEGIN,END
#SBATCH --error=%x-%J-%u.err
#SBATCH --output=%x-%J-%u.out
module --force purge
ml biocontainers repeatmodeler
repeatscout
Link to section 'Introduction' of 'repeatscout' Introduction
RepeatScout
is a tool to discover repetitive substrings in DNA.
For more information, please check its website: https://biocontainers.pro/tools/repeatscout and its home page on Github.
Link to section 'Versions' of 'repeatscout' Versions
- 1.0.6
Link to section 'Commands' of 'repeatscout' Commands
- RepeatScout
- build_lmer_table
- compare-out-to-gff.prl
- filter-stage-1.prl
- filter-stage-2.prl
- merge-lmer-tables.prl
Link to section 'Module' of 'repeatscout' Module
You can load the modules by:
module load biocontainers
module load repeatscout
Link to section 'Example job' of 'repeatscout' Example job
Using #!/bin/sh -l
as shebang in the slurm job script will cause the failure of some biocontainer modules. Please use #!/bin/bash
instead.
To run RepeatScout on our clusters:
#!/bin/bash
#SBATCH -A myallocation # Allocation name
#SBATCH -t 1:00:00
#SBATCH -N 1
#SBATCH -n 1
#SBATCH --job-name=repeatscout
#SBATCH --mail-type=FAIL,BEGIN,END
#SBATCH --error=%x-%J-%u.err
#SBATCH --output=%x-%J-%u.out
module --force purge
ml biocontainers repeatscout
build_lmer_table -l 14 -sequence genome.fasta -freq Final_assembly.freq
RepeatScout -sequence genome.fasta -output Final_assembly_repeats.fasta -freq Final_assembly.freq -l 14
resfinder
Link to section 'Introduction' of 'resfinder' Introduction
ResFinder identifies acquired antimicrobial resistance genes in total or partial sequenced isolates of bacteria.
Home page: https://github.com/cadms/resfinder
Link to section 'Versions' of 'resfinder' Versions
- 4.1.5
Link to section 'Commands' of 'resfinder' Commands
- run_resfinder.py
- run_batch_resfinder.py
Link to section 'Module' of 'resfinder' Module
You can load the modules by:
module load biocontainers
module load resfinder
Link to section 'Example job' of 'resfinder' Example job
Using #!/bin/sh -l
as shebang in the slurm job script will cause the failure of some biocontainer modules. Please use #!/bin/bash
instead.
To run resfinder on our clusters:
#!/bin/bash
#SBATCH -A myallocation # Allocation name
#SBATCH -t 1:00:00
#SBATCH -N 1
#SBATCH -n 1
#SBATCH --job-name=resfinder
#SBATCH --mail-type=FAIL,BEGIN,END
#SBATCH --error=%x-%J-%u.err
#SBATCH --output=%x-%J-%u.out
module --force purge
ml biocontainers resfinder
run_resfinder.py -o output -db_res db_resfinder/ \
-db_res_kma db_resfinder/kma_indexing -db_point db_pointfinder/ \
-s "Escherichia coli" --acquired --point -ifq data/test_isolate_01_*
revbayes
Link to section 'Introduction' of 'revbayes' Introduction
RevBayes -- Bayesian phylogenetic inference using probabilistic graphical models and an interactive language.
Home page: https://github.com/revbayes/revbayes
Link to section 'Versions' of 'revbayes' Versions
- 1.1.1
Link to section 'Commands' of 'revbayes' Commands
- rb
- rb-mpi
Link to section 'Module' of 'revbayes' Module
You can load the modules by:
module load biocontainers
module load revbayes
Link to section 'Example job' of 'revbayes' Example job
Using #!/bin/sh -l
as shebang in the slurm job script will cause the failure of some biocontainer modules. Please use #!/bin/bash
instead.
To run revbayes on our clusters:
#!/bin/bash
#SBATCH -A myallocation # Allocation name
#SBATCH -t 1:00:00
#SBATCH -N 1
#SBATCH -n 1
#SBATCH --job-name=revbayes
#SBATCH --mail-type=FAIL,BEGIN,END
#SBATCH --error=%x-%J-%u.err
#SBATCH --output=%x-%J-%u.out
module --force purge
ml biocontainers revbayes
rmats
MATS
is a computational tool to detect differential alternative splicing events from RNA-Seq data. The statistical model of MATS calculates the P-value and false discovery rate that the difference in the isoform ratio of a gene between two conditions exceeds a given user-defined threshold. From the RNA-Seq data, MATS can automatically detect and analyze alternative splicing events corresponding to all major types of alternative splicing patterns. MATS handles replicate RNA-Seq data from both paired and unpaired study design.
Detailed usage can be found here: http://rnaseq-mats.sourceforge.net
Link to section 'Versions' of 'rmats' Versions
- 4.1.1
Link to section 'Commands' of 'rmats' Commands
- rmats.py
Link to section 'Module' of 'rmats' Module
You can load the modules by:
module load biocontainers
module load rmats
Link to section 'Example job' of 'rmats' Example job
Using #!/bin/sh -l
as shebang in the slurm job script will cause the failure of some biocontainer modules. Please use #!/bin/bash
instead.
To run rmats on our our clusters:
#!/bin/bash
#SBATCH -A myallocation # Allocation name
#SBATCH -t 10:00:00
#SBATCH -N 1
#SBATCH -n 24
#SBATCH --job-name=rmats
#SBATCH --mail-type=FAIL,BEGIN,END
#SBATCH --error=%x-%J-%u.err
#SBATCH --output=%x-%J-%u.out
module --force purge
ml biocontainers rmats
rmats.py --b1 SR_b1.txt --b2 SR_b2.txt --gtf Homo_sapiens.GRCh38.105.gtf --od rmats_out_homo --tmp rmats_tmp -t paired --nthread 10 --readLength 150
rmats2sashimiplot
rmats2sashimiplot
produces a sashimiplot visualization of rMATS output. rmats2sashimiplot can also produce plots using an annotation file and genomic coordinates. The plotting backend is MISO.
Detailed usage can be found here: https://github.com/Xinglab/rmats2sashimiplot
Link to section 'Versions' of 'rmats2sashimiplot' Versions
- 2.0.4
Link to section 'Commands' of 'rmats2sashimiplot' Commands
- rmats2sashimiplot
Link to section 'Module' of 'rmats2sashimiplot' Module
You can load the modules by:
module load biocontainers
module load rmats2sashimiplot
Link to section 'Example job' of 'rmats2sashimiplot' Example job
Using #!/bin/sh -l
as shebang in the slurm job script will cause the failure of some biocontainer modules. Please use #!/bin/bash
instead.
To run rmats on our our clusters:
#!/bin/bash
#SBATCH -A myallocation # Allocation name
#SBATCH -t 1:00:00
#SBATCH -N 1
#SBATCH -n 8
#SBATCH --job-name=rmats2sashimiplot
#SBATCH --mail-type=FAIL,BEGIN,END
#SBATCH --error=%x-%J-%u.err
#SBATCH --output=%x-%J-%u.out
module --force purge
ml biocontainers rmats2sashimiplot
rmats2sashimiplot --s1 sample_1_replicate_1.sam,sample_1_replicate_2.sam,sample_1_replicate_3.sam \
--s2 sample_2_replicate_1.sam,sample_2_replicate_2.sam,sample_2_replicate_3.sam \
-t SE -e SE.MATS.JC.txt --l1 SampleOne --l2 SampleTwo --exon_s 1 --intron_s 5 \
-o test_events_output
rnaindel
Link to section 'Introduction' of 'rnaindel' Introduction
RNAIndel
calls coding indels from tumor RNA-Seq data and classifies them as somatic, germline, and artifactual. RNAIndel supports GRCh38 and 37.
For more information, please check its Github package: https://github.com/stjude/RNAIndel/pkgs/container/rnaindel and its home page on Github.
Link to section 'Versions' of 'rnaindel' Versions
- 3.0.9
Link to section 'Commands' of 'rnaindel' Commands
- rnaindel
Link to section 'Module' of 'rnaindel' Module
You can load the modules by:
module load biocontainers
module load rnaindel
Link to section 'Example job' of 'rnaindel' Example job
Using #!/bin/sh -l
as shebang in the slurm job script will cause the failure of some biocontainer modules. Please use #!/bin/bash
instead.
To run RNAIndel on our clusters:
#!/bin/bash
#SBATCH -A myallocation # Allocation name
#SBATCH -t 1:00:00
#SBATCH -N 1
#SBATCH -n 1
#SBATCH --job-name=rnaindel
#SBATCH --mail-type=FAIL,BEGIN,END
#SBATCH --error=%x-%J-%u.err
#SBATCH --output=%x-%J-%u.out
module --force purge
ml biocontainers rnaindel
rnapeg
Link to section 'Introduction' of 'rnapeg' Introduction
RNApeg
is an RNA junction calling, correction, and quality-control package. RNAIndel supports GRCh38 and 37.
For more information, please check its Github package: https://github.com/stjude/RNApeg/pkgs/container/rnapeg and its home page on Github.
Link to section 'Versions' of 'rnapeg' Versions
- 2.7.1
Link to section 'Commands' of 'rnapeg' Commands
- RNApeg.sh
Link to section 'Module' of 'rnapeg' Module
You can load the modules by:
module load biocontainers
module load rnapeg
Link to section 'Example job' of 'rnapeg' Example job
Using #!/bin/sh -l
as shebang in the slurm job script will cause the failure of some biocontainer modules. Please use #!/bin/bash
instead.
To run RNApeg on our clusters:
#!/bin/bash
#SBATCH -A myallocation # Allocation name
#SBATCH -t 1:00:00
#SBATCH -N 1
#SBATCH -n 1
#SBATCH --job-name=rnapeg
#SBATCH --mail-type=FAIL,BEGIN,END
#SBATCH --error=%x-%J-%u.err
#SBATCH --output=%x-%J-%u.out
module --force purge
ml biocontainers rnapeg
rnaquast
Link to section 'Introduction' of 'rnaquast' Introduction
Rnaquast
is a quality assessment tool for de novo transcriptome assemblies.
For more information, please check its website: https://biocontainers.pro/tools/rnaquast and its home page: http://cab.spbu.ru/software/rnaquast/.
Link to section 'Versions' of 'rnaquast' Versions
- 2.2.1
Link to section 'Commands' of 'rnaquast' Commands
- rnaQUAST.py
Link to section 'Dependencies de novo quality assessment and read alignment' of 'rnaquast' Dependencies de novo quality assessment and read alignment
When reference genome and gene database are unavailable, users can also use BUSCO
and GeneMarkS-T
in rnaQUAST pipeline. Since GeneMarkS-T
requires the license key, users may need to download your own key, and put it in your $HOME.
rnaQUAST is also capable of calculating various statistics using raw reads (e.g. database coverage by reads). To use this, you will need use STAR
in the pipeline.
BUSCO
, GeneMarkS-T
, and STAR
have been installed, and the directories of their executables have been added to $PATH. Users do not need to load these modules. The only module required is rnaquast
itself.
Link to section 'Module' of 'rnaquast' Module
When reference genome and gene database are unavailable, users can also use BUSCO
and GeneMarkS-T
in rnaQUAST pipeline. Since GeneMarkS-T
requires the license key, users may need to download your own key, and put it in your $HOME.
rnaQUAST is also capable of calculating various statistics using raw reads (e.g. database coverage by reads). To use this, you will need use STAR
in the pipeline.
BUSCO
, GeneMarkS-T
, and STAR
have been installed, and the directories of their executables have been added to $PATH. Users do not need to load these modules. The only module required is rnaquast
itself.
You can load the modules by:
module load biocontainers
module load rnaquast
Link to section 'Example job' of 'rnaquast' Example job
Using #!/bin/sh -l
as shebang in the slurm job script will cause the failure of some biocontainer modules. Please use #!/bin/bash
instead.
To run Rnaquast on our clusters:
#!/bin/bash
#SBATCH -A myallocation # Allocation name
#SBATCH -t 1:00:00
#SBATCH -N 1
#SBATCH -n 12
#SBATCH --job-name=rnaquast
#SBATCH --mail-type=FAIL,BEGIN,END
#SBATCH --error=%x-%J-%u.err
#SBATCH --output=%x-%J-%u.out
module --force purge
ml biocontainers rnaquast
rnaQUAST.py -t 12 -o output \
--transcripts Trinity.fasta idba.fasta \
--reference Saccharomyces_cerevisiae.R64-1-1.75.dna.toplevel.fa \
--gtf Saccharomyces_cerevisiae.R64-1-1.75.gtf
rnaQUAST.py -t 12 -o output2 \
--reference reference.fasta \
--transcripts transcripts.fasta \
--left_reads lef.fastq \
--right_reads right.fastq \
--busco fungi_odb10
roary
Link to section 'Introduction' of 'roary' Introduction
Roary is a high speed stand alone pan genome pipeline, which takes annotated assemblies in GFF3 format (produced by Prokka) and calculates the pan genome.
Docker hub: https://hub.docker.com/r/staphb/roary
Home page: https://github.com/sanger-pathogens/Roary
Link to section 'Versions' of 'roary' Versions
- 3.13.0
Link to section 'Commands' of 'roary' Commands
- roary
Link to section 'Module' of 'roary' Module
You can load the modules by:
module load biocontainers
module load roary
Link to section 'Example job' of 'roary' Example job
Using #!/bin/sh -l
as shebang in the slurm job script will cause the failure of some biocontainer modules. Please use #!/bin/bash
instead.
To run roary on our clusters:
#!/bin/bash
#SBATCH -A myallocation # Allocation name
#SBATCH -t 1:00:00
#SBATCH -N 1
#SBATCH -n 1
#SBATCH --job-name=roary
#SBATCH --mail-type=FAIL,BEGIN,END
#SBATCH --error=%x-%J-%u.err
#SBATCH --output=%x-%J-%u.out
module --force purge
ml biocontainers roary
roary -f demo -e -n -v gff/*.gff
rsem
RSEM
is a software package for estimating gene and isoform expression levels from RNA-Seq data. Further information can be found here: https://deweylab.github.io/RSEM/.
Link to section 'Versions' of 'rsem' Versions
- 1.3.3
Commands
- rsem-bam2readdepth
- rsem-bam2wig
- rsem-build-read-index
- rsem-calculate-credibility-intervals
- rsem-calculate-expression
- rsem-control-fdr
- rsem-extract-reference-transcripts
- rsem-generate-data-matrix
- rsem-generate-ngvector
- rsem-gen-transcript-plots
- rsem-get-unique
- rsem-gff3-to-gtf
- rsem-parse-alignments
- rsem-plot-model
- rsem-plot-transcript-wiggles
- rsem-prepare-reference
- rsem-preref
- rsem-refseq-extract-primary-assembly
- rsem-run-ebseq
- rsem-run-em
- rsem-run-gibbs
- rsem-run-prsem-testing-procedure
- rsem-sam-validator
- rsem-scan-for-paired-end-reads
- rsem-simulate-reads
- rsem-synthesis-reference-transcripts
- rsem-tbam2gbam
Link to section 'Dependencies' of 'rsem' Dependencies
STAR v2.7.9a
, Bowtie v1.2.3
, Bowtie2 v2.3.5.1
, HISAT2 v2.2.1
were included in the container image. So users do not need to provide the dependency path in the RSEM parameter.
Link to section 'Module' of 'rsem' Module
You can load the modules by:
module load biocontainers
module load rsem/1.3.3
Link to section 'Example job' of 'rsem' Example job
Using #!/bin/sh -l
as shebang in the slurm job script will cause the failure of some biocontainer modules. Please use #!/bin/bash
instead.
To run RSEM on our cluster:
#!/bin/bash
#SBATCH -A myallocation # Allocation name
#SBATCH -t 10:00:00
#SBATCH -N 1
#SBATCH -n 24
#SBATCH --job-name=rsem
#SBATCH --mail-type=FAIL,BEGIN,END
#SBATCH --error=%x-%J-%u.err
#SBATCH --output=%x-%J-%u.out
module --force purge
ml biocontainers rsem/1.3.3
rsem-prepare-reference --gtf Homo_sapiens.GRCh38.105.gtf --bowtie Homo_sapiens.GRCh38.dna.primary_assembly.fa Gh38_bowtie -p 24
rsem-prepare-reference --gtf Homo_sapiens.GRCh38.105.gtf --bowtie2 Homo_sapiens.GRCh38.dna.primary_assembly.fa Gh38_bowtie2 -p 24
rsem-prepare-reference --gtf Homo_sapiens.GRCh38.105.gtf --hisat2-hca Homo_sapiens.GRCh38.dna.primary_assembly.fa Gh38_hisat2 -p 24
rsem-prepare-reference --gtf Homo_sapiens.GRCh38.105.gtf --star Homo_sapiens.GRCh38.dna.primary_assembly.fa Gh38_star -p 24
rsem-calculate-expression --paired-end --star -p 24 SRR12095148_1.fastq SRR12095148_2.fastq Gh38_star SRR12095148_rsem_expression
rseqc
Link to section 'Introduction' of 'rseqc' Introduction
Rseqc
is a package provides a number of useful modules that can comprehensively evaluate high throughput sequence data especially RNA-seq data.
For more information, please check its website: https://biocontainers.pro/tools/rseqc and its home page: http://rseqc.sourceforge.net.
Link to section 'Versions' of 'rseqc' Versions
- 4.0.0
Link to section 'Commands' of 'rseqc' Commands
- FPKM-UQ.py
- FPKM_count.py
- RNA_fragment_size.py
- RPKM_saturation.py
- aggregate_scores_in_intervals.py
- align_print_template.py
- axt_extract_ranges.py
- axt_to_fasta.py
- axt_to_lav.py
- axt_to_maf.py
- bam2fq.py
- bam2wig.py
- bam_stat.py
- bed_bigwig_profile.py
- bed_build_windows.py
- bed_complement.py
- bed_count_by_interval.py
- bed_count_overlapping.py
- bed_coverage.py
- bed_coverage_by_interval.py
- bed_diff_basewise_summary.py
- bed_extend_to.py
- bed_intersect.py
- bed_intersect_basewise.py
- bed_merge_overlapping.py
- bed_rand_intersect.py
- bed_subtract_basewise.py
- bnMapper.py
- clipping_profile.py
- deletion_profile.py
- div_snp_table_chr.py
- divide_bam.py
- find_in_sorted_file.py
- geneBody_coverage.py
- geneBody_coverage2.py
- gene_fourfold_sites.py
- get_scores_in_intervals.py
- infer_experiment.py
- inner_distance.py
- insertion_profile.py
- int_seqs_to_char_strings.py
- interval_count_intersections.py
- interval_join.py
- junction_annotation.py
- junction_saturation.py
- lav_to_axt.py
- lav_to_maf.py
- line_select.py
- lzop_build_offset_table.py
- mMK_bitset.py
- maf_build_index.py
- maf_chop.py
- maf_chunk.py
- maf_col_counts.py
- maf_col_counts_all.py
- maf_count.py
- maf_covered_ranges.py
- maf_covered_regions.py
- maf_div_sites.py
- maf_drop_overlapping.py
- maf_extract_chrom_ranges.py
- maf_extract_ranges.py
- maf_extract_ranges_indexed.py
- maf_filter.py
- maf_filter_max_wc.py
- maf_gap_frequency.py
- maf_gc_content.py
- maf_interval_alignibility.py
- maf_limit_to_species.py
- maf_mapping_word_frequency.py
- maf_mask_cpg.py
- maf_mean_length_ungapped_piece.py
- maf_percent_columns_matching.py
- maf_percent_identity.py
- maf_print_chroms.py
- maf_print_scores.py
- maf_randomize.py
- maf_region_coverage_by_src.py
- maf_select.py
- maf_shuffle_columns.py
- maf_species_in_all_files.py
- maf_split_by_src.py
- maf_thread_for_species.py
- maf_tile.py
- maf_tile_2.py
- maf_tile_2bit.py
- maf_to_axt.py
- maf_to_concat_fasta.py
- maf_to_fasta.py
- maf_to_int_seqs.py
- maf_translate_chars.py
- maf_truncate.py
- maf_word_frequency.py
- mask_quality.py
- mismatch_profile.py
- nib_chrom_intervals_to_fasta.py
- nib_intervals_to_fasta.py
- nib_length.py
- normalize_bigwig.py
- one_field_per_line.py
- out_to_chain.py
- overlay_bigwig.py
- prefix_lines.py
- pretty_table.py
- qv_to_bqv.py
- random_lines.py
- read_GC.py
- read_NVC.py
- read_distribution.py
- read_duplication.py
- read_hexamer.py
- read_quality.py
- split_bam.py
- split_paired_bam.py
- table_add_column.py
- table_filter.py
- tfloc_summary.py
- tin.py
- ucsc_gene_table_to_intervals.py
- wiggle_to_array_tree.py
- wiggle_to_binned_array.py
- wiggle_to_chr_binned_array.py
- wiggle_to_simple.py
Link to section 'Module' of 'rseqc' Module
You can load the modules by:
module load biocontainers
module load rseqc
Link to section 'Example job' of 'rseqc' Example job
Using #!/bin/sh -l
as shebang in the slurm job script will cause the failure of some biocontainer modules. Please use #!/bin/bash
instead.
To run Rseqc on our clusters:
#!/bin/bash
#SBATCH -A myallocation # Allocation name
#SBATCH -t 1:00:00
#SBATCH -N 1
#SBATCH -n 1
#SBATCH --job-name=rseqc
#SBATCH --mail-type=FAIL,BEGIN,END
#SBATCH --error=%x-%J-%u.err
#SBATCH --output=%x-%J-%u.out
module --force purge
ml biocontainers rseqc
bam_stat.py -i *.bam -q 30
run_dbcan
run_dbCAN
using genomes/metagenomes/proteomes of any assembled organisms (prokaryotes, fungi, plants, animals, viruses) to search for CAZymes. This is a standalone tool of http://bcb.unl.edu/dbCAN2/. Details about its usage can be found in its Github repository.
Link to section 'Versions' of 'run_dbcan' Versions
- 3.0.2
- 3.0.6
Commands
run_dbcan
Link to section 'Database' of 'run_dbcan' Database
Latest version of database has been downloaded and setup, including CAZyDB.09242021.fa, dbCAN-HMMdb-V10.txt, tcdb.fa, tf-1.hmm, tf-2.hmm, and stp.hmm.
Link to section 'Module' of 'run_dbcan' Module
You can load the modules by:
module load biocontainers
module load run_dbcan/3.0.2
Link to section 'Example job' of 'run_dbcan' Example job
Using #!/bin/sh -l
as shebang in the slurm job script will cause the failure of some biocontainer modules. Please use #!/bin/bash
instead.
To run run_dbcan on our cluster:
#!/bin/bash
#SBATCH -A myallocation # Allocation name
#SBATCH -t 10:00:00
#SBATCH -N 1
#SBATCH -n 24
#SBATCH --job-name=run_dbcan
#SBATCH --mail-type=FAIL,BEGIN,END
#SBATCH --error=%x-%J-%u.err
#SBATCH --output=%x-%J-%u.out
module --force purge
ml biocontainers run_dbcan/3.0.2
run_dbcan protein.faa protein --out_dir test1_dbcan
run_dbcan genome.fasta prok --out_dir test2_dbcan
rush
Link to section 'Introduction' of 'rush' Introduction
rush
is a tool similar to GNU parallel and gargs. rush borrows some idea from them and has some unique features, e.g., supporting custom defined variables, resuming multi-line commands, more advanced embedded replacement strings.
For more information, please check its home page on Github.
Link to section 'Versions' of 'rush' Versions
- 0.4.2
Link to section 'Commands' of 'rush' Commands
- rush
Link to section 'Module' of 'rush' Module
You can load the modules by:
module load biocontainers
module load rush
Link to section 'Example job' of 'rush' Example job
Using #!/bin/sh -l
as shebang in the slurm job script will cause the failure of some biocontainer modules. Please use #!/bin/bash
instead.
To run rush on our clusters:
#!/bin/bash
#SBATCH -A myallocation # Allocation name
#SBATCH -t 1:00:00
#SBATCH -N 1
#SBATCH -n 1
#SBATCH --job-name=rush
#SBATCH --mail-type=FAIL,BEGIN,END
#SBATCH --error=%x-%J-%u.err
#SBATCH --output=%x-%J-%u.out
module --force purge
ml biocontainers rush
sage
Link to section 'Introduction' of 'sage' Introduction
Sage is a proteomics search engine - a tool that transforms raw mass spectra from proteomics experiments into peptide identifications via database searching & spectral matching. But, it's also more than just a search engine - Sage includes a variety of advanced features that make it a one-stop shop: retention time prediction, quantification (both isobaric & LFQ), peptide-spectrum match rescoring, and FDR control.
GitHub Packages: https://github.com/lazear/sage/pkgs/container/sage
Home page: https://github.com/lazear/sage
Link to section 'Versions' of 'sage' Versions
- 0.8.1
Link to section 'Commands' of 'sage' Commands
- sage
Link to section 'Module' of 'sage' Module
You can load the modules by:
module load biocontainers
module load sage
Link to section 'Example job' of 'sage' Example job
Using #!/bin/sh -l
as shebang in the slurm job script will cause the failure of some biocontainer modules. Please use #!/bin/bash
instead.
To run sage on our clusters:
#!/bin/bash
#SBATCH -A myallocation # Allocation name
#SBATCH -t 1:00:00
#SBATCH -N 1
#SBATCH -n 1
#SBATCH --job-name=sage
#SBATCH --mail-type=FAIL,BEGIN,END
#SBATCH --error=%x-%J-%u.err
#SBATCH --output=%x-%J-%u.out
module --force purge
ml biocontainers sage
salmon
Salmon
is a wicked-fast program to produce a highly-accurate, transcript-level quantification estimates from RNA-seq data.
Detailed usage can be found here: https://github.com/COMBINE-lab/salmon
Link to section 'Versions' of 'salmon' Versions
- 1.5.2
- 1.6.0
- 1.7.0
- 1.8.0
- 1.9.0
Link to section 'Commands' of 'salmon' Commands
- salmon index
- salmon quant
- salmon alevin
- salmon swim
- salmon quantmerge
Link to section 'Module' of 'salmon' Module
You can load the modules by:
module load biocontainers
module load salmon
Link to section 'Example job' of 'salmon' Example job
Using #!/bin/sh -l
as shebang in the slurm job script will cause the failure of some biocontainer modules. Please use #!/bin/bash
instead.
To run Salmon on our our clusters:
#!/bin/bash
#SBATCH -A myallocation # Allocation name
#SBATCH -t 10:00:00
#SBATCH -N 1
#SBATCH -n 24
#SBATCH --job-name=salmon
#SBATCH --mail-type=FAIL,BEGIN,END
#SBATCH --error=%x-%J-%u.err
#SBATCH --output=%x-%J-%u.out
module --force purge
ml biocontainers salmon
salmon index -t Homo_sapiens.GRCh38.cds.all.fa -i salmon_index
salmon quant -i salmon_index -l A -p 24 -1 SRR16956239_1.fastq -2 SRR16956239_2.fastq --validateMappings -o transcripts_quan
sambamba
Link to section 'Introduction' of 'sambamba' Introduction
Sambamba
is a high performance highly parallel robust and fast tool (and library), written in the D programming language, for working with SAM and BAM files.
For more information, please check its website: https://biocontainers.pro/tools/sambamba and its home page on Github.
Link to section 'Versions' of 'sambamba' Versions
- 0.8.2
Link to section 'Commands' of 'sambamba' Commands
- sambamba
Link to section 'Module' of 'sambamba' Module
You can load the modules by:
module load biocontainers
module load sambamba
Link to section 'Example job' of 'sambamba' Example job
Using #!/bin/sh -l
as shebang in the slurm job script will cause the failure of some biocontainer modules. Please use #!/bin/bash
instead.
To run Sambamba on our clusters:
#!/bin/bash
#SBATCH -A myallocation # Allocation name
#SBATCH -t 1:00:00
#SBATCH -N 1
#SBATCH -n 1
#SBATCH --job-name=sambamba
#SBATCH --mail-type=FAIL,BEGIN,END
#SBATCH --error=%x-%J-%u.err
#SBATCH --output=%x-%J-%u.out
module --force purge
ml biocontainers sambamba
sambamba view --reference-info input.bam
sambamba view -c -F "mapping_quality >= 40" input.bam
samblaster
Link to section 'Introduction' of 'samblaster' Introduction
Samblaster
is a tool to mark duplicates and extract discordant and split reads from sam files.
For more information, please check its website: https://biocontainers.pro/tools/samblaster and its home page on Github.
Link to section 'Versions' of 'samblaster' Versions
- 0.1.26
Link to section 'Commands' of 'samblaster' Commands
- samblaster
Link to section 'Module' of 'samblaster' Module
You can load the modules by:
module load biocontainers
module load samblaster
Link to section 'Example job' of 'samblaster' Example job
Using #!/bin/sh -l
as shebang in the slurm job script will cause the failure of some biocontainer modules. Please use #!/bin/bash
instead.
To run Samblaster on our clusters:
#!/bin/bash
#SBATCH -A myallocation # Allocation name
#SBATCH -t 1:00:00
#SBATCH -N 1
#SBATCH -n 1
#SBATCH --job-name=samblaster
#SBATCH --mail-type=FAIL,BEGIN,END
#SBATCH --error=%x-%J-%u.err
#SBATCH --output=%x-%J-%u.out
module --force purge
ml biocontainers samblaster
samclip
Link to section 'Introduction' of 'samclip' Introduction
Samclip is a tool to filter SAM file for soft and hard clipped alignments.
BioContainers: https://biocontainers.pro/tools/samclip
Home page: https://github.com/tseemann/samclip
Link to section 'Versions' of 'samclip' Versions
- 0.4.0
Link to section 'Commands' of 'samclip' Commands
- samclip
Link to section 'Module' of 'samclip' Module
You can load the modules by:
module load biocontainers
module load samclip
Link to section 'Example job' of 'samclip' Example job
Using #!/bin/sh -l
as shebang in the slurm job script will cause the failure of some biocontainer modules. Please use #!/bin/bash
instead.
To run samclip on our clusters:
#!/bin/bash
#SBATCH -A myallocation # Allocation name
#SBATCH -t 1:00:00
#SBATCH -N 1
#SBATCH -n 1
#SBATCH --job-name=samclip
#SBATCH --mail-type=FAIL,BEGIN,END
#SBATCH --error=%x-%J-%u.err
#SBATCH --output=%x-%J-%u.out
module --force purge
ml biocontainers samclip
samclip --ref test.fna < test.sam > out.sam
samplot
Link to section 'Introduction' of 'samplot' Introduction
Samplot
is a command line tool for rapid, multi-sample structural variant visualization.
For more information, please check its website: https://biocontainers.pro/tools/samplot and its home page on Github.
Link to section 'Versions' of 'samplot' Versions
- 1.3.0
Link to section 'Commands' of 'samplot' Commands
- samplot
Link to section 'Module' of 'samplot' Module
You can load the modules by:
module load biocontainers
module load samplot
Link to section 'Example job' of 'samplot' Example job
Using #!/bin/sh -l
as shebang in the slurm job script will cause the failure of some biocontainer modules. Please use #!/bin/bash
instead.
To run Samplot on our clusters:
#!/bin/bash
#SBATCH -A myallocation # Allocation name
#SBATCH -t 1:00:00
#SBATCH -N 1
#SBATCH -n 1
#SBATCH --job-name=samplot
#SBATCH --mail-type=FAIL,BEGIN,END
#SBATCH --error=%x-%J-%u.err
#SBATCH --output=%x-%J-%u.out
module --force purge
ml biocontainers samplot
samplot plot \
-n NA12878 NA12889 NA12890 \
-b samplot/test/data/NA12878_restricted.bam \
samplot/test/data/NA12889_restricted.bam \
samplot/test/data/NA12890_restricted.bam \
-o 4_115928726_115931880.png \
-c chr4 \
-s 115928726 \
-e 115931880 \
-t DEL
samtools
Link to section 'Introduction' of 'samtools' Introduction
Samtools
is a set of utilities for the Sequence Alignment/Map (SAM) format.
For more information, please check its website: https://biocontainers.pro/tools/samtools and its home page on Github.
Link to section 'Versions' of 'samtools' Versions
- 1.15
- 1.16
- 1.17
- 1.9
Link to section 'Commands' of 'samtools' Commands
- samtools
- ace2sam
- htsfile
- maq2sam-long
- maq2sam-short
- tabix
- wgsim
Link to section 'Module' of 'samtools' Module
You can load the modules by:
module load biocontainers
module load samtools
Link to section 'Example job' of 'samtools' Example job
Using #!/bin/sh -l
as shebang in the slurm job script will cause the failure of some biocontainer modules. Please use #!/bin/bash
instead.
To run Samtools on our clusters:
#!/bin/bash
#SBATCH -A myallocation # Allocation name
#SBATCH -t 1:00:00
#SBATCH -N 1
#SBATCH -n 1
#SBATCH --job-name=samtools
#SBATCH --mail-type=FAIL,BEGIN,END
#SBATCH --error=%x-%J-%u.err
#SBATCH --output=%x-%J-%u.out
module --force purge
ml biocontainers samtools
samtools sort my.sam > my_sorted.bam
samtools index my_sorted.bam
scanpy
Scanpy
is scalable toolkit for analyzing single-cell gene expression data. It includes preprocessing, visualization, clustering, pseudotime and trajectory inference and differential expression testing. The Python-based implementation efficiently deals with datasets of more than one million cells. Details about its usage can be found here (https://scanpy.readthedocs.io/en/stable/)
Link to section 'Versions' of 'scanpy' Versions
- 1.8.2
- 1.9.1
Link to section 'Commands' of 'scanpy' Commands
- python
- python3
Link to section 'Module' of 'scanpy' Module
You can load the modules by:
module load biocontainers
module load scanpy/1.8.2
Link to section 'Interactive job' of 'scanpy' Interactive job
To run scanpy interactively on our clusters:
(base) UserID@bell-fe00:~ $ sinteractive -N1 -n12 -t4:00:00 -A myallocation
salloc: Granted job allocation 12345869
salloc: Waiting for resource configuration
salloc: Nodes bell-a008 are ready for job
(base) UserID@bell-a008:~ $ module load biocontainers scanpy/1.8.2
(base) UserID@bell-a008:~ $ python
Python 3.9.5 (default, Jun 4 2021, 12:28:51)
[GCC 7.5.0] :: Anaconda, Inc. on linux
Type "help", "copyright", "credits" or "license" for more information.
>>> import scanpy as sc
>>> sc.tl.umap(adata, **tool_params)
Link to section 'Batch job' of 'scanpy' Batch job
Using #!/bin/sh -l
as shebang in the slurm job script will cause the failure of some biocontainer modules. Please use #!/bin/bash
instead.
To submit a sbatch job on our clusters:
#!/bin/bash
#SBATCH -A myallocation # Allocation name
#SBATCH -t 10:00:00
#SBATCH -N 1
#SBATCH -n 24
#SBATCH --job-name=scanpy
#SBATCH --mail-type=FAIL,BEGIN,END
#SBATCH --error=%x-%J-%u.err
#SBATCH --output=%x-%J-%u.out
module --force purge
ml biocontainers scanpy/1.8.2
python script.py
scarches
Link to section 'Introduction' of 'scarches' Introduction
scArches is a package to integrate newly produced single-cell datasets into integrated reference atlases.
Home page: https://github.com/theislab/scarches
Link to section 'Versions' of 'scarches' Versions
- 0.5.3
Link to section 'Commands' of 'scarches' Commands
- python
- python3
Link to section 'Module' of 'scarches' Module
You can load the modules by:
module load biocontainers
module load scarches
Link to section 'Example job' of 'scarches' Example job
Using #!/bin/sh -l
as shebang in the slurm job script will cause the failure of some biocontainer modules. Please use #!/bin/bash
instead.
To run scarches on our clusters:
#!/bin/bash
#SBATCH -A myallocation # Allocation name
#SBATCH -t 1:00:00
#SBATCH -N 1
#SBATCH -n 1
#SBATCH --job-name=scarches
#SBATCH --mail-type=FAIL,BEGIN,END
#SBATCH --error=%x-%J-%u.err
#SBATCH --output=%x-%J-%u.out
module --force purge
ml biocontainers scarches
scgen
Link to section 'Introduction' of 'scgen' Introduction
scGen is a generative model to predict single-cell perturbation response across cell types, studies and species.
Home page: https://github.com/theislab/scgen
Link to section 'Versions' of 'scgen' Versions
- 2.1.0
Link to section 'Commands' of 'scgen' Commands
- python
- python3
Link to section 'Module' of 'scgen' Module
You can load the modules by:
module load biocontainers
module load scgen
Link to section 'Example job' of 'scgen' Example job
Using #!/bin/sh -l
as shebang in the slurm job script will cause the failure of some biocontainer modules. Please use #!/bin/bash
instead.
To run scgen on our clusters:
#!/bin/bash
#SBATCH -A myallocation # Allocation name
#SBATCH -t 1:00:00
#SBATCH -N 1
#SBATCH -n 1
#SBATCH --job-name=scgen
#SBATCH --mail-type=FAIL,BEGIN,END
#SBATCH --error=%x-%J-%u.err
#SBATCH --output=%x-%J-%u.out
module --force purge
ml biocontainers scgen
scirpy
Link to section 'Introduction' of 'scirpy' Introduction
Scirpy is a scalable python-toolkit to analyse T cell receptor (TCR) or B cell receptor (BCR) repertoires from single-cell RNA sequencing (scRNA-seq) data. It seamlessly integrates with the popular scanpy library and provides various modules for data import, analysis and visualization.
Home page: https://github.com/scverse/scirpy
Link to section 'Versions' of 'scirpy' Versions
- 0.10.1
Link to section 'Commands' of 'scirpy' Commands
- python
- python3
Link to section 'Module' of 'scirpy' Module
You can load the modules by:
module load biocontainers
module load scirpy
Link to section 'Example job' of 'scirpy' Example job
Using #!/bin/sh -l
as shebang in the slurm job script will cause the failure of some biocontainer modules. Please use #!/bin/bash
instead.
To run scirpy on our clusters:
#!/bin/bash
#SBATCH -A myallocation # Allocation name
#SBATCH -t 1:00:00
#SBATCH -N 1
#SBATCH -n 1
#SBATCH --job-name=scirpy
#SBATCH --mail-type=FAIL,BEGIN,END
#SBATCH --error=%x-%J-%u.err
#SBATCH --output=%x-%J-%u.out
module --force purge
ml biocontainers scirpy
scvelo
scVelo
is a scalable toolkit for RNA velocity analysis in single cells, based on https://doi.org/10.1038/s41587-020-0591-3. Its detailed usage can be found here: https://scvelo.readthedocs.io.
Link to section 'Versions' of 'scvelo' Versions
- 0.2.4
Link to section 'Commands' of 'scvelo' Commands
- python
- python3
Link to section 'Module' of 'scvelo' Module
You can load the modules by:
module load biocontainers
module load scvelo/0.2.4
Link to section 'Interactive job' of 'scvelo' Interactive job
To run scVelo interactively on our clusters:
(base) UserID@bell-fe00:~ $ sinteractive -N1 -n12 -t4:00:00 -A myallocation
salloc: Granted job allocation 12345869
salloc: Waiting for resource configuration
salloc: Nodes bell-a008 are ready for job
(base) UserID@bell-a008:~ $ module load biocontainers scvelo/0.2.4
(base) UserID@bell-a008:~ $ python
Python 3.9.5 (default, Jun 4 2021, 12:28:51)
[GCC 7.5.0] :: Anaconda, Inc. on linux
Type "help", "copyright", "credits" or "license" for more information.
>>> import scvelo as scv
>>> scv.set_figure_params()
Link to section 'Batch job' of 'scvelo' Batch job
Using #!/bin/sh -l
as shebang in the slurm job script will cause the failure of some biocontainer modules. Please use #!/bin/bash
instead.
To submit a sbatch job on our clusters:
#!/bin/bash
#SBATCH -A myallocation # Allocation name
#SBATCH -t 10:00:00
#SBATCH -N 1
#SBATCH -n 24
#SBATCH --job-name=scvelo
#SBATCH --mail-type=FAIL,BEGIN,END
#SBATCH --error=%x-%J-%u.err
#SBATCH --output=%x-%J-%u.out
module --force purge
ml biocontainers scvelo/0.2.4
python script.py
scvi-tools
Link to section 'Introduction' of 'scvi-tools' Introduction
scvi-tools (single-cell variational inference tools) is a package for end-to-end analysis of single-cell omics data primarily developed and maintained by the Yosef Lab at UC Berkeley.
Home page: https://scvi-tools.org
Link to section 'Versions' of 'scvi-tools' Versions
- 0.16.2
Link to section 'Commands' of 'scvi-tools' Commands
- python
- python3
- R
- Rscript
Link to section 'Module' of 'scvi-tools' Module
You can load the modules by:
module load biocontainers
module load scvi-tools
Link to section 'Example job' of 'scvi-tools' Example job
Using #!/bin/sh -l
as shebang in the slurm job script will cause the failure of some biocontainer modules. Please use #!/bin/bash
instead.
To run scvi-tools on our clusters:
#!/bin/bash
#SBATCH -A myallocation # Allocation name
#SBATCH -t 1:00:00
#SBATCH -N 1
#SBATCH -n 1
#SBATCH --job-name=scvi-tools
#SBATCH --mail-type=FAIL,BEGIN,END
#SBATCH --error=%x-%J-%u.err
#SBATCH --output=%x-%J-%u.out
module --force purge
ml biocontainers scvi-tools
segalign
Link to section 'Introduction' of 'segalign' Introduction
Segalign is a scalable GPU system for pairwise whole genome alignments based on LASTZ's seed-filter-extend paradigm.
Docker hub: https://hub.docker.com/r/gsneha/segalign
Home page: https://github.com/gsneha26/SegAlign
Link to section 'Versions' of 'segalign' Versions
- 0.1.2
Link to section 'Commands' of 'segalign' Commands
- faToTwoBit
- run_segalign
- run_segalign_repeat_masker
- segalign
- segalign_repeat_masker
- twoBitToFa
Link to section 'Module' of 'segalign' Module
You can load the modules by:
module load biocontainers
module load segalign
Link to section 'Example job' of 'segalign' Example job
Using #!/bin/sh -l
as shebang in the slurm job script will cause the failure of some biocontainer modules. Please use #!/bin/bash
instead.
To run segalign on our clusters:
#!/bin/bash
#SBATCH -A myallocation # Allocation name
#SBATCH -t 1:00:00
#SBATCH -N 1
#SBATCH -n 1
#SBATCH --job-name=segalign
#SBATCH --mail-type=FAIL,BEGIN,END
#SBATCH --error=%x-%J-%u.err
#SBATCH --output=%x-%J-%u.out
module --force purge
ml biocontainers segalign
seidr
Link to section 'Introduction' of 'seidr' Introduction
Seidr
is a community gene network inference and exploration toolkit.
For more information, please check its website: https://biocontainers.pro/tools/seidr and its home page on Github.
Link to section 'Versions' of 'seidr' Versions
- 0.14.2
Link to section 'Commands' of 'seidr' Commands
- correlation
- seidr
- mi
- pcor
- narromi
- plsnet
- llr-ensemble
- svm-ensemble
- genie3
- tigress
- el-ensemble
- makeconv
- genrb
- gencfu
- gencnval
- gendict
- tomsimilarity
Link to section 'Module' of 'seidr' Module
You can load the modules by:
module load biocontainers
module load seidr
Link to section 'Example job' of 'seidr' Example job
Using #!/bin/sh -l
as shebang in the slurm job script will cause the failure of some biocontainer modules. Please use #!/bin/bash
instead.
To run Seidr on our clusters:
#!/bin/bash
#SBATCH -A myallocation # Allocation name
#SBATCH -t 1:00:00
#SBATCH -N 1
#SBATCH -n 1
#SBATCH --job-name=seidr
#SBATCH --mail-type=FAIL,BEGIN,END
#SBATCH --error=%x-%J-%u.err
#SBATCH --output=%x-%J-%u.out
module --force purge
ml biocontainers seidr
sepp
Link to section 'Introduction' of 'sepp' Introduction
Sepp
stands for SATé-Enabled Phylogenetic Placement and addresses the problem of phylogenetic placement for meta-genomic short reads.
For more information, please check its website: https://biocontainers.pro/tools/sepp and its home page on Github.
Link to section 'Versions' of 'sepp' Versions
- 4.5.1
Link to section 'Commands' of 'sepp' Commands
- run_sepp.py
- run_upp.py
- split_sequences.py
- sumlabels.py
- sumtrees.py
Link to section 'Module' of 'sepp' Module
You can load the modules by:
module load biocontainers
module load sepp
Link to section 'Example job' of 'sepp' Example job
Using #!/bin/sh -l
as shebang in the slurm job script will cause the failure of some biocontainer modules. Please use #!/bin/bash
instead.
To run Sepp on our clusters:
#!/bin/bash
#SBATCH -A myallocation # Allocation name
#SBATCH -t 1:00:00
#SBATCH -N 1
#SBATCH -n 1
#SBATCH --job-name=sepp
#SBATCH --mail-type=FAIL,BEGIN,END
#SBATCH --error=%x-%J-%u.err
#SBATCH --output=%x-%J-%u.out
module --force purge
ml biocontainers sepp
run_sepp.py -t mock/rpsS/sate.tre \
-r mock/rpsS/sate.tre.RAxML_info \
-a mock/rpsS/sate.fasta \
-f mock/rpsS/rpsS.even.fas \
-o rpsS.out.default
seqcode
Link to section 'Introduction' of 'seqcode' Introduction
SeqCode is a family of applications designed to develop high-quality images and perform genome-wide calculations from high-throughput sequencing experiments. This software is presented into two distinct modes: web tools and command line. The website of SeqCode offers most functions to users with no previous expertise in bioinformatics, including operations on a selection of published ChIP-seq samples and applications to generate multiple classes of graphics from data files of the user. On the contrary, the standalone version of SeqCode allows bioinformaticians to run each command on any type of sequencing data locally in their computer. The architecture of the source code is modular and the input/output interface of the commands is suitable to be integrated into existing pipelines of genome analysis. SeqCode has been written in ANSI C, which favors the compatibility in every UNIX platform and grants a high performance and speed when analyzing sequencing data. Meta-plots, heatmaps, boxplots and the rest of images produced by SeqCode are internally generated using R. SeqCode relies on the RefSeq reference annotations and is able to deal with the genome and assembly release of every organism that is available from this consortium.
Docker hub: https://hub.docker.com/r/eblancocrg/seqcode
Home page: https://github.com/eblancoga/seqcode
Link to section 'Versions' of 'seqcode' Versions
- 1.0
Link to section 'Commands' of 'seqcode' Commands
- buildChIPprofile
- combineChIPprofiles
- combineTSSmaps
- combineTSSplots
- computemaxsignal
- findPeaks
- genomeDistribution
- matchpeaks
- matchpeaksgenes
- processmacs
- produceGENEmaps
- produceGENEplots
- producePEAKmaps
- producePEAKplots
- produceTESmaps
- produceTESplots
- produceTSSmaps
- produceTSSplots
- recoverChIPlevels
- scorePhastCons
Link to section 'Module' of 'seqcode' Module
You can load the modules by:
module load biocontainers
module load seqcode
Link to section 'Example job' of 'seqcode' Example job
Using #!/bin/sh -l
as shebang in the slurm job script will cause the failure of some biocontainer modules. Please use #!/bin/bash
instead.
To run seqcode on our clusters:
#!/bin/bash
#SBATCH -A myallocation # Allocation name
#SBATCH -t 1:00:00
#SBATCH -N 1
#SBATCH -n 1
#SBATCH --job-name=seqcode
#SBATCH --mail-type=FAIL,BEGIN,END
#SBATCH --error=%x-%J-%u.err
#SBATCH --output=%x-%J-%u.out
module --force purge
ml biocontainers seqcode
buildChIPprofile -vd ChromInfo.txt \
H3K4me3_sample.bam test_buildChIPprofile
seqkit
Link to section 'Introduction' of 'seqkit' Introduction
Seqkit
is a rapid tool for manipulating fasta and fastq files.
For more information, please check its website: https://biocontainers.pro/tools/seqkit and its home page on Github.
Link to section 'Versions' of 'seqkit' Versions
- 2.0.0
- 2.1.0
- 2.3.1
Link to section 'Commands' of 'seqkit' Commands
- seqkit
Link to section 'Module' of 'seqkit' Module
You can load the modules by:
module load biocontainers
module load seqkit
Link to section 'Example job' of 'seqkit' Example job
Using #!/bin/sh -l
as shebang in the slurm job script will cause the failure of some biocontainer modules. Please use #!/bin/bash
instead.
To run Seqkit on our clusters:
#!/bin/bash
#SBATCH -A myallocation # Allocation name
#SBATCH -t 1:00:00
#SBATCH -N 1
#SBATCH -n 1
#SBATCH --job-name=seqkit
#SBATCH --mail-type=FAIL,BEGIN,END
#SBATCH --error=%x-%J-%u.err
#SBATCH --output=%x-%J-%u.out
module --force purge
ml biocontainers seqkit
seqkit stats configs.fasta > contigs_statistics.txt
seqyclean
Link to section 'Introduction' of 'seqyclean' Introduction
Seqyclean is used to pre-process NGS data in order to prepare for downstream analysis.
For more information, please check:
Docker hub: https://hub.docker.com/r/staphb/seqyclean
Home page: https://github.com/ibest/seqyclean
Link to section 'Versions' of 'seqyclean' Versions
- 1.10.09
Link to section 'Commands' of 'seqyclean' Commands
- seqyclean
Link to section 'Module' of 'seqyclean' Module
You can load the modules by:
module load biocontainers
module load seqyclean
Link to section 'Example job' of 'seqyclean' Example job
Using #!/bin/sh -l
as shebang in the slurm job script will cause the failure of some biocontainer modules. Please use #!/bin/bash
instead.
To run seqyclean on our clusters:
#!/bin/bash
#SBATCH -A myallocation # Allocation name
#SBATCH -t 1:00:00
#SBATCH -N 1
#SBATCH -n 1
#SBATCH --job-name=seqyclean
#SBATCH --mail-type=FAIL,BEGIN,END
#SBATCH --error=%x-%J-%u.err
#SBATCH --output=%x-%J-%u.out
module --force purge
ml biocontainers seqyclean
shapeit4
Link to section 'Introduction' of 'shapeit4' Introduction
SHAPEIT4 is a fast and accurate method for estimation of haplotypes (aka phasing) for SNP array and high coverage sequencing data.
BioContainers: https://biocontainers.pro/tools/shapeit4
Home page: https://odelaneau.github.io/shapeit4/
Link to section 'Versions' of 'shapeit4' Versions
- 4.2.2
Link to section 'Commands' of 'shapeit4' Commands
- shapeit4
Link to section 'Module' of 'shapeit4' Module
You can load the modules by:
module load biocontainers
module load shapeit4
Link to section 'Example job' of 'shapeit4' Example job
Using #!/bin/sh -l
as shebang in the slurm job script will cause the failure of some biocontainer modules. Please use #!/bin/bash
instead.
To run shapeit4 on our clusters:
#!/bin/bash
#SBATCH -A myallocation # Allocation name
#SBATCH -t 1:00:00
#SBATCH -N 1
#SBATCH -n 1
#SBATCH --job-name=shapeit4
#SBATCH --mail-type=FAIL,BEGIN,END
#SBATCH --error=%x-%J-%u.err
#SBATCH --output=%x-%J-%u.out
module --force purge
ml biocontainers shapeit4
shapeit5
Link to section 'Introduction' of 'shapeit5' Introduction
SHAPEIT5 is a software package to estimate haplotypes in large genotype datasets (WGS and SNP array).
Home page: https://github.com/odelaneau/shapeit5
Link to section 'Versions' of 'shapeit5' Versions
- 5.1.1
Link to section 'Commands' of 'shapeit5' Commands
-
phase_common
-
ligate
-
phase_rare
-
simulate
-
switch
-
xcftools
Link to section 'Module' of 'shapeit5' Module
You can load the modules by:
module load biocontainers
module load shapeit5
Link to section 'Example job' of 'shapeit5' Example job
Using #!/bin/sh -l
as shebang in the slurm job script will cause the failure of some biocontainer modules. Please use #!/bin/bash
instead.
To run shapeit5 on our clusters:
#!/bin/bash
#SBATCH -A myallocation # Allocation name
#SBATCH -t 1:00:00
#SBATCH -N 1
#SBATCH -n 1
#SBATCH --job-name=shapeit5
#SBATCH --mail-type=FAIL,BEGIN,END
#SBATCH --error=%x-%J-%u.err
#SBATCH --output=%x-%J-%u.out
module --force purge
ml biocontainers shapeit5
phase_common --input wgs/target.unrelated.bcf --filter-maf 0.001 --region 1 --map info/chr1.gmap.gz --output tmp/target.scaffold.bcf --thread 8
shasta
Link to section 'Introduction' of 'shasta' Introduction
Shasta is a software for de novo assembly from Oxford Nanopore reads.
Home page: https://github.com/chanzuckerberg/shasta
Link to section 'Versions' of 'shasta' Versions
- 0.10.0
Link to section 'Commands' of 'shasta' Commands
- shasta
Link to section 'Module' of 'shasta' Module
You can load the modules by:
module load biocontainers
module load shasta
Link to section 'Example job' of 'shasta' Example job
Using #!/bin/sh -l
as shebang in the slurm job script will cause the failure of some biocontainer modules. Please use #!/bin/bash
instead.
To run shasta on our clusters:
#!/bin/bash
#SBATCH -A myallocation # Allocation name
#SBATCH -t 1:00:00
#SBATCH -N 1
#SBATCH -n 1
#SBATCH --job-name=shasta
#SBATCH --mail-type=FAIL,BEGIN,END
#SBATCH --error=%x-%J-%u.err
#SBATCH --output=%x-%J-%u.out
module --force purge
ml biocontainers shasta
shasta --input r94_ec_rad2.181119.60x-10kb.fasta \
--config Nanopore-May2022
shigeifinder
Link to section 'Introduction' of 'shigeifinder' Introduction
Shigeifinder is a tool that is used to identify differentiate Shigella/EIEC using cluster-specific genes and identify the serotype using O-antigen/H-antigen genes.
For more information, please check:
Docker hub: https://hub.docker.com/r/staphb/shigeifinder
Home page: https://github.com/LanLab/ShigEiFinder
Link to section 'Versions' of 'shigeifinder' Versions
- 1.3.2
Link to section 'Commands' of 'shigeifinder' Commands
- shigeifinder
Link to section 'Module' of 'shigeifinder' Module
You can load the modules by:
module load biocontainers
module load shigeifinder
Link to section 'Example job' of 'shigeifinder' Example job
Using #!/bin/sh -l
as shebang in the slurm job script will cause the failure of some biocontainer modules. Please use #!/bin/bash
instead.
To run shigeifinder on our clusters:
#!/bin/bash
#SBATCH -A myallocation # Allocation name
#SBATCH -t 1:00:00
#SBATCH -N 1
#SBATCH -n 1
#SBATCH --job-name=shigeifinder
#SBATCH --mail-type=FAIL,BEGIN,END
#SBATCH --error=%x-%J-%u.err
#SBATCH --output=%x-%J-%u.out
module --force purge
ml biocontainers shigeifinder
shorah
Link to section 'Introduction' of 'shorah' Introduction
Shorah
is an open source project for the analysis of next generation sequencing data.
For more information, please check its website: https://biocontainers.pro/tools/shorah and its home page on Github.
Link to section 'Versions' of 'shorah' Versions
- 1.99.2
Link to section 'Commands' of 'shorah' Commands
- shorah
- b2w
- diri_sampler
- fil
Link to section 'Module' of 'shorah' Module
You can load the modules by:
module load biocontainers
module load shorah
Link to section 'Example job' of 'shorah' Example job
Using #!/bin/sh -l
as shebang in the slurm job script will cause the failure of some biocontainer modules. Please use #!/bin/bash
instead.
To run Shorah on our clusters:
#!/bin/bash
#SBATCH -A myallocation # Allocation name
#SBATCH -t 1:00:00
#SBATCH -N 1
#SBATCH -n 1
#SBATCH --job-name=shorah
#SBATCH --mail-type=FAIL,BEGIN,END
#SBATCH --error=%x-%J-%u.err
#SBATCH --output=%x-%J-%u.out
module --force purge
ml biocontainers shorah
shorah amplicon -b ampli_sorted.bam -f reference.fasta
shorah shotgun -b test_aln.cram -f test_ref.fasta
shorah shotgun -a 0.1 -w 42 -x 100000 -p 0.9 -c 0 -r REF:42-272 -R 42 -b test_aln.cram -f ref.fasta
shortstack
Link to section 'Introduction' of 'shortstack' Introduction
Shortstack
is a tool for comprehensive annotation and quantification of small RNA genes.
For more information, please check its website: https://biocontainers.pro/tools/shortstack and its home page on Github.
Link to section 'Versions' of 'shortstack' Versions
- 3.8.5
Link to section 'Commands' of 'shortstack' Commands
- ShortStack
Link to section 'Module' of 'shortstack' Module
You can load the modules by:
module load biocontainers
module load shortstack
Link to section 'Example job' of 'shortstack' Example job
Using #!/bin/sh -l
as shebang in the slurm job script will cause the failure of some biocontainer modules. Please use #!/bin/bash
instead.
To run Shortstack on our clusters:
#!/bin/bash
#SBATCH -A myallocation # Allocation name
#SBATCH -t 1:00:00
#SBATCH -N 1
#SBATCH -n 1
#SBATCH --job-name=shortstack
#SBATCH --mail-type=FAIL,BEGIN,END
#SBATCH --error=%x-%J-%u.err
#SBATCH --output=%x-%J-%u.out
module --force purge
ml biocontainers shortstack
shovill
Link to section 'Introduction' of 'shovill' Introduction
Shovill is a tool to assemble bacterial isolate genomes from Illumina paired-end reads.
Docker hub: https://hub.docker.com/r/staphb/shovill
Home page: https://github.com/tseemann/shovill
Link to section 'Versions' of 'shovill' Versions
- 1.1.0
Link to section 'Commands' of 'shovill' Commands
- shovill
Link to section 'Module' of 'shovill' Module
You can load the modules by:
module load biocontainers
module load shovill
Link to section 'Example job' of 'shovill' Example job
Using #!/bin/sh -l
as shebang in the slurm job script will cause the failure of some biocontainer modules. Please use #!/bin/bash
instead.
To run shovill on our clusters:
#!/bin/bash
#SBATCH -A myallocation # Allocation name
#SBATCH -t 1:00:00
#SBATCH -N 1
#SBATCH -n 1
#SBATCH --job-name=shovill
#SBATCH --mail-type=FAIL,BEGIN,END
#SBATCH --error=%x-%J-%u.err
#SBATCH --output=%x-%J-%u.out
module --force purge
ml biocontainers shovill
shovill --outdir out \
--R1 test/R1.fq.gz \
--R2 test/R2.fq.gz
sicer
Link to section 'Introduction' of 'sicer' Introduction
Sicer
is a clustering approach for identification of enriched domains from histone modification ChIP-Seq data.
For more information, please check its website: https://biocontainers.pro/tools/sicer and its home page: http://home.gwu.edu/~wpeng/Software.htm.
Link to section 'Versions' of 'sicer' Versions
- 1.1
Link to section 'Commands' of 'sicer' Commands
- SICER-df-rb.sh
- SICER-df.sh
- SICER-rb.sh
- SICER.sh
Link to section 'Module' of 'sicer' Module
You can load the modules by:
module load biocontainers
module load sicer
Link to section 'Example job' of 'sicer' Example job
Using #!/bin/sh -l
as shebang in the slurm job script will cause the failure of some biocontainer modules. Please use #!/bin/bash
instead.
To run Sicer on our clusters:
#!/bin/bash
#SBATCH -A myallocation # Allocation name
#SBATCH -t 1:00:00
#SBATCH -N 1
#SBATCH -n 1
#SBATCH --job-name=sicer
#SBATCH --mail-type=FAIL,BEGIN,END
#SBATCH --error=%x-%J-%u.err
#SBATCH --output=%x-%J-%u.out
module --force purge
ml biocontainers sicer
SICER.sh ./ test.bed control.bed . hg18 1 200 150 0.74 600 .01
SICER-rb.sh ./ test.bed . hg18 1 200 150 0.74 400 100
sicer2
Link to section 'Introduction' of 'sicer2' Introduction
Sicer2
is the redesigned and improved ChIP-seq broad peak calling tool SICER.
For more information, please check its website: https://biocontainers.pro/tools/sicer2 and its home page on Github.
Link to section 'Versions' of 'sicer2' Versions
- 1.0.3
- 1.2.0
Link to section 'Commands' of 'sicer2' Commands
- sicer
- sicer_df
- recognicer
- recognicer_df
Link to section 'Module' of 'sicer2' Module
You can load the modules by:
module load biocontainers
module load sicer2
Link to section 'Example job' of 'sicer2' Example job
Using #!/bin/sh -l
as shebang in the slurm job script will cause the failure of some biocontainer modules. Please use #!/bin/bash
instead.
To run Sicer2 on our clusters:
#!/bin/bash
#SBATCH -A myallocation # Allocation name
#SBATCH -t 1:00:00
#SBATCH -N 1
#SBATCH -n 1
#SBATCH --job-name=sicer2
#SBATCH --mail-type=FAIL,BEGIN,END
#SBATCH --error=%x-%J-%u.err
#SBATCH --output=%x-%J-%u.out
module --force purge
ml biocontainers sicer2
sicer_df -t ./test/treatment_1.bed ./test/treatment_2.bed \
-c ./test/control_1.bed ./test/control_2.bed \
-s hg38 --significant_reads
recognicer_df -t ./test/treatment_1.bed ./test/treatment_2.bed \
-c ./test/control_1.bed ./test/control_2.bed \
-s hg38 --significant_reads
signalp4
Link to section 'Introduction' of 'signalp4' Introduction
SignalP
predicts the presence and location of signal peptide cleavage sites in amino acid sequences from different organisms: Gram-positive prokaryotes, Gram-negative prokaryotes, and eukaryotes.
For more information, please check its home page: https://services.healthtech.dtu.dk/service.php?SignalP-4.1.
Link to section 'Versions' of 'signalp4' Versions
- 4.1
Link to section 'Commands' of 'signalp4' Commands
- signalp
Link to section 'Module' of 'signalp4' Module
You can load the modules by:
module load biocontainers
module load signalp
Link to section 'Example job' of 'signalp4' Example job
Using #!/bin/sh -l
as shebang in the slurm job script will cause the failure of some biocontainer modules. Please use #!/bin/bash
instead.
To run SignalP on our clusters:
#!/bin/bash
#SBATCH -A myallocation # Allocation name
#SBATCH -t 1:00:00
#SBATCH -N 1
#SBATCH -n 1
#SBATCH --job-name=signalp
#SBATCH --mail-type=FAIL,BEGIN,END
#SBATCH --error=%x-%J-%u.err
#SBATCH --output=%x-%J-%u.out
module --force purge
ml biocontainers signalp
signalp -t gram+ -f all proka.fasta > proka_out
signalp -t euk -f all euk.fasta > euk.out
signalp6
Link to section 'Introduction' of 'signalp6' Introduction
SignalP predicts the presence and location of signal peptide cleavage sites in amino acid sequences from different organisms: Gram-positive prokaryotes, Gram-negative prokaryotes, and eukaryotes.
Home page: https://services.healthtech.dtu.dk/service.php?SignalP
Link to section 'Versions' of 'signalp6' Versions
- 6.0-fast
- 6.0-slow
Link to section 'Commands' of 'signalp6' Commands
- signalp6
Link to section 'Module' of 'signalp6' Module
You can load the modules by:
module load biocontainers
module load signalp6
Link to section 'Example job for fast mode' of 'signalp6' Example job for fast mode
Using #!/bin/sh -l
as shebang in the slurm job script will cause the failure of some biocontainer modules. Please use #!/bin/bash
instead.
Using #!/bin/sh -l
as shebang in the slurm job script will cause the failure of some biocontainer modules. Please use #!/bin/bash
instead.
To run signalp6 on our clusters:
#!/bin/bash
#SBATCH -A myallocation # Allocation name
#SBATCH -t 2:00:00
#SBATCH -N 1
#SBATCH -n 24
#SBATCH --job-name=signalp6-fast
#SBATCH --mail-type=FAIL,BEGIN,END
#SBATCH --error=%x-%J-%u.err
#SBATCH --output=%x-%J-%u.out
module --force purge
ml biocontainers signalp6/6.0-fast
signalp6 --write_procs 24 --fastafile proteins_clean.fasta \
--organism euk --output_dir output_fast \
--format txt --mode fast
Link to section 'Example job for slow mode' of 'signalp6' Example job for slow mode
Using #!/bin/sh -l
as shebang in the slurm job script will cause the failure of some biocontainer modules. Please use #!/bin/bash
instead.
To run signalp6 on our clusters:
#!/bin/bash
#SBATCH -A myallocation # Allocation name
#SBATCH -t 12:00:00
#SBATCH -N 1
#SBATCH -n 24
#SBATCH --job-name=signalp6-slow
#SBATCH --mail-type=FAIL,BEGIN,END
#SBATCH --error=%x-%J-%u.err
#SBATCH --output=%x-%J-%u.out
module --force purge
ml biocontainers signalp6/6.0-slow
signalp6 --write_procs 24 --fastafile proteins_clean.fasta \
--organism euk --output_dir output_slow \
--format txt --mode slow
signalp6 --write_procs 24 --fastafile proteins_clean.fasta \
--organism euk --output_dir output_slow-sequential \
--format txt --mode slow-sequential
simug
Link to section 'Introduction' of 'simug' Introduction
Simug
is a general-purpose genome simulator.
For more information, please check its website: https://biocontainers.pro/tools/simug and its home page on Github.
Link to section 'Versions' of 'simug' Versions
- 1.0.0
Link to section 'Commands' of 'simug' Commands
- simuG
- vcf2model
Link to section 'Module' of 'simug' Module
You can load the modules by:
module load biocontainers
module load simug
Link to section 'Example job' of 'simug' Example job
Using #!/bin/sh -l
as shebang in the slurm job script will cause the failure of some biocontainer modules. Please use #!/bin/bash
instead.
To run Simug on our clusters:
#!/bin/bash
#SBATCH -A myallocation # Allocation name
#SBATCH -t 1:00:00
#SBATCH -N 1
#SBATCH -n 1
#SBATCH --job-name=simug
#SBATCH --mail-type=FAIL,BEGIN,END
#SBATCH --error=%x-%J-%u.err
#SBATCH --output=%x-%J-%u.out
module --force purge
ml biocontainers simug
singlem
Link to section 'Introduction' of 'singlem' Introduction
SingleM is a tool for profiling shotgun metagenomes. It has a particular strength in detecting microbial lineages which are not in reference databases. The method it uses also makes it suitable for some related tasks, such as assessing eukaryotic contamination, finding bias in genome recovery, computing ecological diversity metrics, and lineage-targeted MAG recovery.
Docker hub: https://hub.docker.com/r/wwood/singlem
Home page: https://github.com/wwood/singlem
Link to section 'Versions' of 'singlem' Versions
- 0.13.2
Link to section 'Commands' of 'singlem' Commands
- singlem
Link to section 'Module' of 'singlem' Module
You can load the modules by:
module load biocontainers
module load singlem
Link to section 'Example job' of 'singlem' Example job
Using #!/bin/sh -l
as shebang in the slurm job script will cause the failure of some biocontainer modules. Please use #!/bin/bash
instead.
To run singlem on our clusters:
#!/bin/bash
#SBATCH -A myallocation # Allocation name
#SBATCH -t 1:00:00
#SBATCH -N 1
#SBATCH -n 1
#SBATCH --job-name=singlem
#SBATCH --mail-type=FAIL,BEGIN,END
#SBATCH --error=%x-%J-%u.err
#SBATCH --output=%x-%J-%u.out
module --force purge
ml biocontainers singlem
ska
Link to section 'Introduction' of 'ska' Introduction
SKA (Split Kmer Analysis) is a toolkit for prokaryotic (and any other small, haploid) DNA sequence analysis using split kmers. A split kmer is a pair of kmers in a DNA sequence that are separated by a single base. Split kmers allow rapid comparison and alignment of small genomes, and is particularly suited for surveillance or outbreak investigation. SKA can produce split kmer files from fasta format assemblies or directly from fastq format read sequences, cluster them, align them with or without a reference sequence and provide various comparison and summary statistics. Currently all testing has been carried out on high-quality Illumina read data, so results for other platforms may vary.
Docker hub: https://hub.docker.com/r/staphb/ska
Home page: https://github.com/simonrharris/SKA
Link to section 'Versions' of 'ska' Versions
- 1.0
Link to section 'Commands' of 'ska' Commands
- ska
Link to section 'Module' of 'ska' Module
You can load the modules by:
module load biocontainers
module load ska
Link to section 'Example job' of 'ska' Example job
Using #!/bin/sh -l
as shebang in the slurm job script will cause the failure of some biocontainer modules. Please use #!/bin/bash
instead.
To run ska on our clusters:
#!/bin/bash
#SBATCH -A myallocation # Allocation name
#SBATCH -t 1:00:00
#SBATCH -N 1
#SBATCH -n 1
#SBATCH --job-name=ska
#SBATCH --mail-type=FAIL,BEGIN,END
#SBATCH --error=%x-%J-%u.err
#SBATCH --output=%x-%J-%u.out
module --force purge
ml biocontainers ska
skewer
Link to section 'Introduction' of 'skewer' Introduction
Skewer
is a fast and accurate adapter trimmer for paired-end reads.
For more information, please check its website: https://biocontainers.pro/tools/skewer and its home page on Github.
Link to section 'Versions' of 'skewer' Versions
- 0.2.2
Link to section 'Commands' of 'skewer' Commands
- skewer
Link to section 'Module' of 'skewer' Module
You can load the modules by:
module load biocontainers
module load skewer
Link to section 'Example job' of 'skewer' Example job
Using #!/bin/sh -l
as shebang in the slurm job script will cause the failure of some biocontainer modules. Please use #!/bin/bash
instead.
To run Skewer on our clusters:
#!/bin/bash
#SBATCH -A myallocation # Allocation name
#SBATCH -t 1:00:00
#SBATCH -N 1
#SBATCH -n 1
#SBATCH --job-name=skewer
#SBATCH --mail-type=FAIL,BEGIN,END
#SBATCH --error=%x-%J-%u.err
#SBATCH --output=%x-%J-%u.out
module --force purge
ml biocontainers skewer
skewer -l 50 -m pe -o skewerQ30 --mean-quality 30 \
--end-quality 30 -t 10 -x TruSeq3-PE.fa \
input_1.fastq input_2.fastq
slamdunk
Link to section 'Introduction' of 'slamdunk' Introduction
Slamdunk is a novel, fully automated software tool for automated, robust, scalable and reproducible SLAMseq data analysis.
Docker hub: https://hub.docker.com/r/tobneu/slamdunk
Home page: http://t-neumann.github.io/slamdunk/
Link to section 'Versions' of 'slamdunk' Versions
- 0.4.3
Link to section 'Commands' of 'slamdunk' Commands
- slamdunk
- alleyoop
Link to section 'Module' of 'slamdunk' Module
You can load the modules by:
module load biocontainers
module load slamdunk
Link to section 'Example job' of 'slamdunk' Example job
Using #!/bin/sh -l
as shebang in the slurm job script will cause the failure of some biocontainer modules. Please use #!/bin/bash
instead.
To run slamdunk on our clusters:
#!/bin/bash
#SBATCH -A myallocation # Allocation name
#SBATCH -t 1:00:00
#SBATCH -N 1
#SBATCH -n 1
#SBATCH --job-name=slamdunk
#SBATCH --mail-type=FAIL,BEGIN,END
#SBATCH --error=%x-%J-%u.err
#SBATCH --output=%x-%J-%u.out
module --force purge
ml biocontainers slamdunk
smoove
Link to section 'Introduction' of 'smoove' Introduction
Smoove
simplifies and speeds calling and genotyping SVs for short reads.
For more information, please check its website: https://biocontainers.pro/tools/smoove and its home page on Github.
Link to section 'Versions' of 'smoove' Versions
- 0.2.7
Link to section 'Commands' of 'smoove' Commands
- smoove
Link to section 'Module' of 'smoove' Module
You can load the modules by:
module load biocontainers
module load smoove
Link to section 'Example job' of 'smoove' Example job
Using #!/bin/sh -l
as shebang in the slurm job script will cause the failure of some biocontainer modules. Please use #!/bin/bash
instead.
To run Smoove on our clusters:
#!/bin/bash
#SBATCH -A myallocation # Allocation name
#SBATCH -t 1:00:00
#SBATCH -N 1
#SBATCH -n 24
#SBATCH --job-name=smoove
#SBATCH --mail-type=FAIL,BEGIN,END
#SBATCH --error=%x-%J-%u.err
#SBATCH --output=%x-%J-%u.out
module --force purge
ml biocontainers smoove
smoove call \
-x --name my-cohort \
--exclude hg38_blacklist.bed \
--fasta Homo_sapiens.GRCh38.dna.primary_assembly.fa \
-p 24 \
--genotype input_bams/*.bam
snakemake
Link to section 'Introduction' of 'snakemake' Introduction
Snakemake
is a workflow engine that provides a readable Python-based workflow definition language and a powerful execution environment that scales from single-core workstations to compute clusters without modifying the workflow.
For more information, please check its website: https://biocontainers.pro/tools/snakemake and its home page: https://snakemake.readthedocs.io/en/stable/.
Link to section 'Versions' of 'snakemake' Versions
- 6.8.0
Link to section 'Commands' of 'snakemake' Commands
- snakemake
Link to section 'Module' of 'snakemake' Module
You can load the modules by:
module load biocontainers
module load snakemake
Link to section 'Example job' of 'snakemake' Example job
Using #!/bin/sh -l
as shebang in the slurm job script will cause the failure of some biocontainer modules. Please use #!/bin/bash
instead.
To run Snakemake on our clusters:
#!/bin/bash
#SBATCH -A myallocation # Allocation name
#SBATCH -t 1:00:00
#SBATCH -N 1
#SBATCH -n 1
#SBATCH --job-name=snakemake
#SBATCH --mail-type=FAIL,BEGIN,END
#SBATCH --error=%x-%J-%u.err
#SBATCH --output=%x-%J-%u.out
module --force purge
ml biocontainers snakemake
snap
Link to section 'Introduction' of 'snap' Introduction
Snap
is a semi-HMM-based Nucleic Acid Parser -- gene prediction tool.
For more information, please check its website: https://biocontainers.pro/tools/snap and its home page: http://korflab.ucdavis.edu/software.html.
Link to section 'Versions' of 'snap' Versions
- 2013_11_29
Link to section 'Commands' of 'snap' Commands
- snap
Link to section 'Module' of 'snap' Module
You can load the modules by:
module load biocontainers
module load snap
Link to section 'Example job' of 'snap' Example job
Using #!/bin/sh -l
as shebang in the slurm job script will cause the failure of some biocontainer modules. Please use #!/bin/bash
instead.
To run Snap on our clusters:
#!/bin/bash
#SBATCH -A myallocation # Allocation name
#SBATCH -t 1:00:00
#SBATCH -N 1
#SBATCH -n 1
#SBATCH --job-name=snap
#SBATCH --mail-type=FAIL,BEGIN,END
#SBATCH --error=%x-%J-%u.err
#SBATCH --output=%x-%J-%u.out
module --force purge
ml biocontainers snap
snap-aligner
Link to section 'Introduction' of 'snap-aligner' Introduction
Snap-aligner
(Scalable Nucleotide Alignment Program) is a fast and accurate read aligner for high-throughput sequencing data.
For more information, please check its website: https://biocontainers.pro/tools/snap-aligner and its home page: http://snap.cs.berkeley.edu/.
Link to section 'Versions' of 'snap-aligner' Versions
- 2.0.0
Link to section 'Commands' of 'snap-aligner' Commands
- snap-aligner
Link to section 'Module' of 'snap-aligner' Module
You can load the modules by:
module load biocontainers
module load snap-aligner
Link to section 'Example job' of 'snap-aligner' Example job
Using #!/bin/sh -l
as shebang in the slurm job script will cause the failure of some biocontainer modules. Please use #!/bin/bash
instead.
To run Snap-aligner on our clusters:
#!/bin/bash
#SBATCH -A myallocation # Allocation name
#SBATCH -t 1:00:00
#SBATCH -N 1
#SBATCH -n 1
#SBATCH --job-name=snap-aligner
#SBATCH --mail-type=FAIL,BEGIN,END
#SBATCH --error=%x-%J-%u.err
#SBATCH --output=%x-%J-%u.out
module --force purge
ml biocontainers snap-aligner
snaptools
Link to section 'Introduction' of 'snaptools' Introduction
Snaptools
is a python module for pre-processing and working with snap file.
For more information, please check its website: https://biocontainers.pro/tools/snaptools and its home page on Github.
Link to section 'Versions' of 'snaptools' Versions
- 1.4.8
Link to section 'Commands' of 'snaptools' Commands
- snaptools
Link to section 'Module' of 'snaptools' Module
You can load the modules by:
module load biocontainers
module load snaptools
Link to section 'Example job' of 'snaptools' Example job
Using #!/bin/sh -l
as shebang in the slurm job script will cause the failure of some biocontainer modules. Please use #!/bin/bash
instead.
To run Snaptools on our clusters:
#!/bin/bash
#SBATCH -A myallocation # Allocation name
#SBATCH -t 1:00:00
#SBATCH -N 1
#SBATCH -n 1
#SBATCH --job-name=snaptools
#SBATCH --mail-type=FAIL,BEGIN,END
#SBATCH --error=%x-%J-%u.err
#SBATCH --output=%x-%J-%u.out
module --force purge
ml biocontainers snaptools
snippy
Link to section 'Introduction' of 'snippy' Introduction
Snippy
is a tool for rapid haploid variant calling and core genome alignment.
Docker hub: https://hub.docker.com/r/staphb/snippy and its home page on Github.
Link to section 'Versions' of 'snippy' Versions
- 4.6.0
Link to section 'Commands' of 'snippy' Commands
- snippy
- snippy-clean_full_aln
- snippy-core
- snippy-multi
- snippy-vcf_extract_subs
- snippy-vcf_report
- snippy-vcf_to_tab
Link to section 'Module' of 'snippy' Module
You can load the modules by:
module load biocontainers
module load snippy
Link to section 'Example job' of 'snippy' Example job
Using #!/bin/sh -l
as shebang in the slurm job script will cause the failure of some biocontainer modules. Please use #!/bin/bash
instead.
To run Snippy on our clusters:
#!/bin/bash
#SBATCH -A myallocation # Allocation name
#SBATCH -t 1:00:00
#SBATCH -N 1
#SBATCH -n 1
#SBATCH --job-name=snippy
#SBATCH --mail-type=FAIL,BEGIN,END
#SBATCH --error=%x-%J-%u.err
#SBATCH --output=%x-%J-%u.out
module --force purge
ml biocontainers snippy
snp-dists
Link to section 'Introduction' of 'snp-dists' Introduction
Snp-dists is a tool to convert a FASTA alignment to SNP distance matrix.
Docker hub: https://hub.docker.com/r/staphb/snp-dists
Home page: https://github.com/tseemann/snp-dists
Link to section 'Versions' of 'snp-dists' Versions
- 0.8.2
Link to section 'Commands' of 'snp-dists' Commands
- snp-dists
Link to section 'Module' of 'snp-dists' Module
You can load the modules by:
module load biocontainers
module load snp-dists
Link to section 'Example job' of 'snp-dists' Example job
Using #!/bin/sh -l
as shebang in the slurm job script will cause the failure of some biocontainer modules. Please use #!/bin/bash
instead.
To run snp-dists on our clusters:
#!/bin/bash
#SBATCH -A myallocation # Allocation name
#SBATCH -t 1:00:00
#SBATCH -N 1
#SBATCH -n 1
#SBATCH --job-name=snp-dists
#SBATCH --mail-type=FAIL,BEGIN,END
#SBATCH --error=%x-%J-%u.err
#SBATCH --output=%x-%J-%u.out
module --force purge
ml biocontainers snp-dists
snp-dists test/good.aln > distances.tab
snp-sites
Link to section 'Introduction' of 'snp-sites' Introduction
SNP-sites is a tool that apidly extracts SNPs from a multi-FASTA alignment.
Docker hub: https://hub.docker.com/r/staphb/snp-sites
Home page: https://github.com/sanger-pathogens/snp-sites
Link to section 'Versions' of 'snp-sites' Versions
- 2.5.1
Link to section 'Commands' of 'snp-sites' Commands
- snp-sites
Link to section 'Module' of 'snp-sites' Module
You can load the modules by:
module load biocontainers
module load snp-sites
Link to section 'Example job' of 'snp-sites' Example job
Using #!/bin/sh -l
as shebang in the slurm job script will cause the failure of some biocontainer modules. Please use #!/bin/bash
instead.
To run snp-sites on our clusters:
#!/bin/bash
#SBATCH -A myallocation # Allocation name
#SBATCH -t 1:00:00
#SBATCH -N 1
#SBATCH -n 1
#SBATCH --job-name=snp-sites
#SBATCH --mail-type=FAIL,BEGIN,END
#SBATCH --error=%x-%J-%u.err
#SBATCH --output=%x-%J-%u.out
module --force purge
ml biocontainers snp-sites
snp-sites salmonella_serovars_core_genes.aln
snpeff
Link to section 'Introduction' of 'snpeff' Introduction
Snpeff
is an open source tool that annotates variants and predicts their effects on genes by using an interval forest approach.
For more information, please check its website: https://biocontainers.pro/tools/snpeff and its home page on Github.
Link to section 'Versions' of 'snpeff' Versions
- 5.1d
- 5.1
Link to section 'Commands' of 'snpeff' Commands
- snpEff
Link to section 'Module' of 'snpeff' Module
You can load the modules by:
module load biocontainers
module load snpeff
Link to section 'Example job' of 'snpeff' Example job
Using #!/bin/sh -l
as shebang in the slurm job script will cause the failure of some biocontainer modules. Please use #!/bin/bash
instead.
By default, snpEff only uses 1gb
of memory. To allocate larger memory, add -Xmx
flag in your command.:
snpeff -Xmx10g ## To allocate 10gb of memory.
To run Snpeff on our clusters:
#!/bin/bash
#SBATCH -A myallocation # Allocation name
#SBATCH -t 1:00:00
#SBATCH -N 1
#SBATCH -n 1
#SBATCH --job-name=snpeff
#SBATCH --mail-type=FAIL,BEGIN,END
#SBATCH --error=%x-%J-%u.err
#SBATCH --output=%x-%J-%u.out
module --force purge
ml biocontainers snpeff
snpEff GRCh37.75 examples/test.chr22.vcf > test.chr22.ann.vcf
snpgenie
Link to section 'Introduction' of 'snpgenie' Introduction
Snpgenie
is a collection of Perl scripts for estimating πN/πS, dN/dS, and gene diversity from next-generation sequencing (NGS) single-nucleotide polymorphism (SNP) variant data.
For more information, please check its website: https://biocontainers.pro/tools/snpgenie and its home page on Github.
Link to section 'Versions' of 'snpgenie' Versions
- 1.0
Link to section 'Commands' of 'snpgenie' Commands
- fasta2revcom.pl
- gtf2revcom.pl
- snpgenie.pl
- snpgenie_between_group.pl
- snpgenie_between_group_processor.pl
- snpgenie_within_group.pl
- snpgenie_within_group_processor.pl
- vcf2revcom.pl
Link to section 'Module' of 'snpgenie' Module
You can load the modules by:
module load biocontainers
module load snpgenie
Link to section 'Example job' of 'snpgenie' Example job
Using #!/bin/sh -l
as shebang in the slurm job script will cause the failure of some biocontainer modules. Please use #!/bin/bash
instead.
To run Snpgenie on our clusters:
#!/bin/bash
#SBATCH -A myallocation # Allocation name
#SBATCH -t 1:00:00
#SBATCH -N 1
#SBATCH -n 1
#SBATCH --job-name=snpgenie
#SBATCH --mail-type=FAIL,BEGIN,END
#SBATCH --error=%x-%J-%u.err
#SBATCH --output=%x-%J-%u.out
module --force purge
ml biocontainers snpgenie
snpgenie.pl --minfreq=0.01 --snpreport=CLC_SNP_EXAMPLE.txt \
--fastafile=REFERENCE_EXAMPLE.fasta --gtffile=CDS_EXAMPLE.gtf
snphylo
Link to section 'Introduction' of 'snphylo' Introduction
Snphylo is a pipeline to generate a phylogenetic tree from huge SNP data.
Docker hub: https://hub.docker.com/r/finchnsnps/snphylo
Home page: https://github.com/thlee/SNPhylo
Link to section 'Versions' of 'snphylo' Versions
- 20180901
Link to section 'Commands' of 'snphylo' Commands
- Rscript
- snphylo.sh
- convert_fasta_to_phylip.py
- convert_simple_to_hapmap.py
- determine_bs_tree.R
- draw_unrooted_tree.R
- generate_snp_sequence.R
- remove_low_depth_genotype_data.py
- remove_no_genotype_data.py
Link to section 'Module' of 'snphylo' Module
You can load the modules by:
module load biocontainers
module load snphylo
Link to section 'Example job' of 'snphylo' Example job
Using #!/bin/sh -l
as shebang in the slurm job script will cause the failure of some biocontainer modules. Please use #!/bin/bash
instead.
To run snphylo on our clusters:
#!/bin/bash
#SBATCH -A myallocation # Allocation name
#SBATCH -t 1:00:00
#SBATCH -N 1
#SBATCH -n 1
#SBATCH --job-name=snphylo
#SBATCH --mail-type=FAIL,BEGIN,END
#SBATCH --error=%x-%J-%u.err
#SBATCH --output=%x-%J-%u.out
module --force purge
ml biocontainers snphylo
snpsift
Link to section 'Introduction' of 'snpsift' Introduction
Snpsift
is a tool used to annotate genomic variants using databases, filters, and manipulates genomic annotated variants.
For more information, please check its website: https://biocontainers.pro/tools/snpsift and its home page on Github.
Link to section 'Versions' of 'snpsift' Versions
- 4.3.1t
Link to section 'Commands' of 'snpsift' Commands
- SnpSift
Link to section 'Module' of 'snpsift' Module
You can load the modules by:
module load biocontainers
module load snpsift
Link to section 'Example job' of 'snpsift' Example job
Using #!/bin/sh -l
as shebang in the slurm job script will cause the failure of some biocontainer modules. Please use #!/bin/bash
instead.
To run Snpsift on our clusters:
#!/bin/bash
#SBATCH -A myallocation # Allocation name
#SBATCH -t 1:00:00
#SBATCH -N 1
#SBATCH -n 1
#SBATCH --job-name=snpsift
#SBATCH --mail-type=FAIL,BEGIN,END
#SBATCH --error=%x-%J-%u.err
#SBATCH --output=%x-%J-%u.out
module --force purge
ml biocontainers snpsift
SnpSift annotate -id dbSnp132.vcf \
variants.vcf > variants_annotated.vcf
soapdenovo2
Link to section 'Introduction' of 'soapdenovo2' Introduction
Soapdenovo2
is a short-read assembly method to build de novo draft assembly.
For more information, please check its website: https://biocontainers.pro/tools/soapdenovo2.
Link to section 'Versions' of 'soapdenovo2' Versions
- 2.40
Link to section 'Commands' of 'soapdenovo2' Commands
- SOAPdenovo-127mer
- SOAPdenovo-63mer
Link to section 'Module' of 'soapdenovo2' Module
You can load the modules by:
module load biocontainers
module load soapdenovo2
Link to section 'Example job' of 'soapdenovo2' Example job
Using #!/bin/sh -l
as shebang in the slurm job script will cause the failure of some biocontainer modules. Please use #!/bin/bash
instead.
To run Soapdenovo2 on our clusters:
#!/bin/bash
#SBATCH -A myallocation # Allocation name
#SBATCH -t 1:00:00
#SBATCH -N 1
#SBATCH -n 1
#SBATCH --job-name=soapdenovo2
#SBATCH --mail-type=FAIL,BEGIN,END
#SBATCH --error=%x-%J-%u.err
#SBATCH --output=%x-%J-%u.out
module --force purge
ml biocontainers soapdenovo2
SOAPdenovo-127mer all -s config_file -K 63 -R -o graph_prefix 1>ass.log 2>ass.err
sortmerna
Link to section 'Introduction' of 'sortmerna' Introduction
SortMeRNA
is a local sequence alignment tool for filtering, mapping and clustering.
For more information, please check its website: https://biocontainers.pro/tools/sortmerna and its home page on Github.
Link to section 'Versions' of 'sortmerna' Versions
- 2.1b
- 4.3.4
Link to section 'Commands' of 'sortmerna' Commands
- sortmerna
Link to section 'Module' of 'sortmerna' Module
You can load the modules by:
module load biocontainers
module load sortmerna
Link to section 'Example job' of 'sortmerna' Example job
Using #!/bin/sh -l
as shebang in the slurm job script will cause the failure of some biocontainer modules. Please use #!/bin/bash
instead.
To run SortMeRNA on our clusters:
#!/bin/bash
#SBATCH -A myallocation # Allocation name
#SBATCH -t 1:00:00
#SBATCH -N 1
#SBATCH -n 1
#SBATCH --job-name=sortmerna
#SBATCH --mail-type=FAIL,BEGIN,END
#SBATCH --error=%x-%J-%u.err
#SBATCH --output=%x-%J-%u.out
module --force purge
ml biocontainers sortmerna
sortmerna --ref silva-bac-16s-id90.fasta,silva-bac-16s-db \
--reads set2_environmental_study_550_amplicon.fasta \
--fastx --aligned Test
souporcell
Link to section 'Introduction' of 'souporcell' Introduction
souporcell is a method for clustering mixed-genotype scRNAseq experiments by individual.
Home page: https://github.com/wheaton5/souporcell
Link to section 'Versions' of 'souporcell' Versions
- 2.0
Link to section 'Commands' of 'souporcell' Commands
- check_modules.py
- compile_stan_model.py
- consensus.py
- renamer.py
- retag.py
- shared_samples.py
- souporcell.py
- souporcell_pipeline.py
Link to section 'Module' of 'souporcell' Module
You can load the modules by:
module load biocontainers
module load souporcell
Link to section 'Example job' of 'souporcell' Example job
Using #!/bin/sh -l
as shebang in the slurm job script will cause the failure of some biocontainer modules. Please use #!/bin/bash
instead.
To run souporcell on our clusters:
#!/bin/bash
#SBATCH -A myallocation # Allocation name
#SBATCH -t 1:00:00
#SBATCH -N 1
#SBATCH -n 8
#SBATCH --job-name=souporcell
#SBATCH --mail-type=FAIL,BEGIN,END
#SBATCH --error=%x-%J-%u.err
#SBATCH --output=%x-%J-%u.out
module --force purge
ml biocontainers souporcell
souporcell_pipeline.py -i A.merged.bam \
-b GSM2560245_barcodes.tsv \
-f refdata-cellranger-GRCh38-3.0.0/fasta/genome.fa \
-t 8 -o demux_data_test -k 4
sourmash
Link to section 'Introduction' of 'sourmash' Introduction
Sourmash
is a tool for quickly search, compare, and analyze genomic and metagenomic data sets.
For more information, please check its website: https://biocontainers.pro/tools/sourmash and its home page on Github.
Link to section 'Versions' of 'sourmash' Versions
- 4.3.0
- 4.5.0
Link to section 'Commands' of 'sourmash' Commands
- sourmash
Link to section 'Module' of 'sourmash' Module
You can load the modules by:
module load biocontainers
module load sourmash
Link to section 'Example job' of 'sourmash' Example job
Using #!/bin/sh -l
as shebang in the slurm job script will cause the failure of some biocontainer modules. Please use #!/bin/bash
instead.
To run Sourmash on our clusters:
#!/bin/bash
#SBATCH -A myallocation # Allocation name
#SBATCH -t 1:00:00
#SBATCH -N 1
#SBATCH -n 1
#SBATCH --job-name=sourmash
#SBATCH --mail-type=FAIL,BEGIN,END
#SBATCH --error=%x-%J-%u.err
#SBATCH --output=%x-%J-%u.out
module --force purge
ml biocontainers sourmash
sourmash sketch dna -p k=31 *.fna.gz
sourmash compare *.sig -o cmp.dist
sourmash plot cmp.dist --labels
spaceranger
Link to section 'Introduction' of 'spaceranger' Introduction
Spaceranger
is a set of analysis pipelines that process Visium Spatial Gene Expression data with brightfield and fluorescence microscope images.
Docker hub: https://hub.docker.com/r/cumulusprod/spaceranger/tags and its home page: https://support.10xgenomics.com/spatial-gene-expression/software/pipelines/latest/what-is-space-ranger.
Link to section 'Versions' of 'spaceranger' Versions
- 1.3.0
- 1.3.1
- 2.0.0
Link to section 'Commands' of 'spaceranger' Commands
- spaceranger
Link to section 'Module' of 'spaceranger' Module
You can load the modules by:
module load biocontainers
module load spaceranger
Link to section 'Example job' of 'spaceranger' Example job
Using #!/bin/sh -l
as shebang in the slurm job script will cause the failure of some biocontainer modules. Please use #!/bin/bash
instead.
To run Spaceranger on our clusters:
#!/bin/bash
#SBATCH -A myallocation # Allocation name
#SBATCH -t 1:00:00
#SBATCH -N 1
#SBATCH -n 1
#SBATCH --job-name=spaceranger
#SBATCH --mail-type=FAIL,BEGIN,END
#SBATCH --error=%x-%J-%u.err
#SBATCH --output=%x-%J-%u.out
module --force purge
ml biocontainers spaceranger
spaceranger count --id=sample345 \ #Output directory
--transcriptome=/opt/refdata/GRCh38-2020-A \ #Path to Reference
--fastqs=/home/jdoe/runs/HAWT7ADXX/outs/fastq_path \ #Path to FASTQs
--sample=mysample \ #Sample name from FASTQ filename
--image=/home/jdoe/runs/images/sample345.tiff \ #Path to brightfield image
--slide=V19J01-123 \ #Slide ID
--area=A1 \ #Capture area
--localcores=8 \ #Allowed cores in localmode
--localmem=64 #Allowed memory (GB) in localmode
spades
SPAdes
- St. Petersburg genome assembler - is an assembly toolkit containing various assembly pipelines.
Detailed usage can be found here: https://github.com/ablab/spades
Link to section 'Versions' of 'spades' Versions
- 3.15.3
- 3.15.4
- 3.15.5
Link to section 'Commands' of 'spades' Commands
- coronaspades.py
- metaplasmidspades.py
- metaspades.py
- metaviralspades.py
- plasmidspades.py
- rnaspades.py
- rnaviralspades.py
- spades.py
- spades_init.py
- truspades.py
- spades-bwa
- spades-convert-bin-to-fasta
- spades-core
- spades-corrector-core
- spades-gbuilder
- spades-gmapper
- spades-gsimplifier
- spades-hammer
- spades-ionhammer
- spades-kmer-estimating
- spades-kmercount
- spades-read-filter
- spades-truseq-scfcorrection
Link to section 'Module' of 'spades' Module
You can load the modules by:
module load biocontainers
module load spades
Link to section 'Example job' of 'spades' Example job
Using #!/bin/sh -l
as shebang in the slurm job script will cause the failure of some biocontainer modules. Please use #!/bin/bash
instead.
To run spades on our our clusters:
#!/bin/bash
#SBATCH -A myallocation # Allocation name
#SBATCH -t 20:00:00
#SBATCH -N 1
#SBATCH -n 24
#SBATCH --job-name=spades
#SBATCH --mail-type=FAIL,BEGIN,END
#SBATCH --error=%x-%J-%u.err
#SBATCH --output=%x-%J-%u.out
module --force purge
ml biocontainers spades
spades.py --pe1-1 SRR11234553_1.fastq --pe1-2 SRR11234553_2.fastq -o spades_out -t 24
sprod
Link to section 'Introduction' of 'sprod' Introduction
Sprod: De-noising Spatially Resolved Transcriptomics Data Based on Position and Image Information.
Home page: https://github.com/yunguan-wang/SPROD
Link to section 'Versions' of 'sprod' Versions
- 1.0
Link to section 'Commands' of 'sprod' Commands
- python
- python3
- sprod.py
Link to section 'Module' of 'sprod' Module
You can load the modules by:
module load biocontainers
module load sprod
Link to section 'Example job' of 'sprod' Example job
Using #!/bin/sh -l
as shebang in the slurm job script will cause the failure of some biocontainer modules. Please use #!/bin/bash
instead.
To run sprod on our clusters:
#!/bin/bash
#SBATCH -A myallocation # Allocation name
#SBATCH -t 1:00:00
#SBATCH -N 1
#SBATCH -n 1
#SBATCH --job-name=sprod
#SBATCH --mail-type=FAIL,BEGIN,END
#SBATCH --error=%x-%J-%u.err
#SBATCH --output=%x-%J-%u.out
module --force purge
ml biocontainers sprod
python3 test_examples.py
squeezemeta
Link to section 'Introduction' of 'squeezemeta' Introduction
SqueezeMeta is a fully automated metagenomics pipeline, from reads to bins.
Home page: https://github.com/jtamames/SqueezeMeta
Link to section 'Versions' of 'squeezemeta' Versions
- 1.5.1
Link to section 'Commands' of 'squeezemeta' Commands
- 01.merge_assemblies.pl
- 01.merge_sequential.pl
- 01.remap.pl
- 01.run_assembly.pl
- 01.run_assembly_merged.pl
- 02.rnas.pl
- 03.run_prodigal.pl
- 04.rundiamond.pl
- 05.run_hmmer.pl
- 06.lca.pl
- 07.fun3assign.pl
- 08.blastx.pl
- 09.summarycontigs3.pl
- 10.mapsamples.pl
- 11.mcount.pl
- 12.funcover.pl
- 13.mergeannot2.pl
- 14.runbinning.pl
- 15.dastool.pl
- 16.addtax2.pl
- 17.checkM_batch.pl
- 18.getbins.pl
- 19.getcontigs.pl
- 20.minpath.pl
- 21.stats.pl
- SqueezeMeta.pl
- SqueezeMeta_conf.pl
- SqueezeMeta_conf_original.pl
- parameters.pl
- restart.pl
- add_database.pl
- cover.pl
- sqm2ipath.pl
- sqm2itol.pl
- sqm2keggplots.pl
- sqm2pavian.pl
- sqm_annot.pl
- sqm_hmm_reads.pl
- sqm_longreads.pl
- sqm_mapper.pl
- sqm_reads.pl
- versionchange.pl
- find_missing_markers.pl
- remove_duplicate_markers.pl
- anvi-filter-sqm.py
- anvi-load-sqm.py
- sqm2anvio.pl
- configure_nodb.pl
- configure_nodb_alt.pl
- download_databases.pl
- make_databases.pl
- make_databases_alt.pl
- test_install.pl
Link to section 'Module' of 'squeezemeta' Module
You can load the modules by:
module load biocontainers
module load squeezemeta
Link to section 'Example job' of 'squeezemeta' Example job
Using #!/bin/sh -l
as shebang in the slurm job script will cause the failure of some biocontainer modules. Please use #!/bin/bash
instead.
To run squeezemeta on our clusters:
#!/bin/bash
#SBATCH -A myallocation # Allocation name
#SBATCH -t 1:00:00
#SBATCH -N 1
#SBATCH -n 1
#SBATCH --job-name=squeezemeta
#SBATCH --mail-type=FAIL,BEGIN,END
#SBATCH --error=%x-%J-%u.err
#SBATCH --output=%x-%J-%u.out
module --force purge
ml biocontainers squeezemeta
SqueezeMeta.pl -m coassembly -p Hadza -s test.samples -f raw
squid
Link to section 'Introduction' of 'squid' Introduction
SQUID is designed to detect both fusion-gene and non-fusion-gene transcriptomic structural variations from RNA-seq alignment.
Home page: https://github.com/Kingsford-Group/squid
Link to section 'Versions' of 'squid' Versions
- 1.5
Link to section 'Commands' of 'squid' Commands
- squid
- AnnotateSQUIDOutput.py
Link to section 'Module' of 'squid' Module
You can load the modules by:
module load biocontainers
module load squid
Link to section 'Example job' of 'squid' Example job
Using #!/bin/sh -l
as shebang in the slurm job script will cause the failure of some biocontainer modules. Please use #!/bin/bash
instead.
To run squid on our clusters:
#!/bin/bash
#SBATCH -A myallocation # Allocation name
#SBATCH -t 1:00:00
#SBATCH -N 1
#SBATCH -n 1
#SBATCH --job-name=squid
#SBATCH --mail-type=FAIL,BEGIN,END
#SBATCH --error=%x-%J-%u.err
#SBATCH --output=%x-%J-%u.out
module --force purge
ml biocontainers squid
sra-tools
SRA-Toolkit
is a collection of tools and libraries for using data in the INSDC Sequence Read Archives. Its detailed documentation can be found in https://trace.ncbi.nlm.nih.gov/Traces/sra/sra.cgi?view=toolkit_doc.
Link to section 'Versions' of 'sra-tools' Versions
- 2.11.0-pl5262
Link to section 'Commands' of 'sra-tools' Commands
- abi-dump
- align-cache
- align-info
- bam-load
- cache-mgr
- cg-load
- fasterq-dump
- fasterq-dump-orig
- fastq-dump
- fastq-dump-orig
- illumina-dump
- kar
- kdbmeta
- kget
- latf-load
- md5cp
- prefetch
- prefetch-orig
- rcexplain
- read-filter-redact
- sam-dump
- sam-dump-orig
- sff-dump
- sra-pileup
- sra-pileup-orig
- sra-sort
- sra-sort-cg
- sra-stat
- srapath
- srapath-orig
- sratools
- test-sra
- vdb-config
- vdb-copy
- vdb-diff
- vdb-dump
- vdb-encrypt
- vdb-lock
- vdb-passwd
- vdb-unlock
- vdb-validate
Link to section 'Module' of 'sra-tools' Module
You can load the modules by:
module load sra-tools
Link to section 'Configuring SRA-Toolkit' of 'sra-tools' Configuring SRA-Toolkit
Users can config SRA-Toolkit by the command vdb-config
. For example, the below command set up the current working directory for downloading:
vdb-config --prefetch-to-cwd
Link to section 'Example job' of 'sra-tools' Example job
To run SRA-Toolkit on our cluster:
#!/bin/bash
#SBATCH -A myallocation # Allocation name
#SBATCH -t 1:00:00
#SBATCH -N 1
#SBATCH -n 8
#SBATCH --job-name=SRA-Toolkit
#SBATCH --mail-type=FAIL,BEGIN,END
#SBATCH --error=%x-%J-%u.err
#SBATCH --output=%x-%J-%u.out
module --force purge
ml sra-tools
vdb-config --prefetch-to-cwd # The data will be downloaded to the current working directory.
prefetch SRR11941281
fastq-dump --split-3 SRR11941281/SRR11941281.sra
srst2
Link to section 'Introduction' of 'srst2' Introduction
Srst2 is designed to take Illumina sequence data, a MLST database and/or a database of gene sequences (e.g. resistance genes, virulence genes, etc) and report the presence of STs and/or reference genes.
For more information, please check:
Docker hub: https://hub.docker.com/r/staphb/srst2
Home page: https://github.com/katholt/srst2
Link to section 'Versions' of 'srst2' Versions
- 0.2.0
Link to section 'Commands' of 'srst2' Commands
- getmlst.py
- srst2
- slurm_srst2.py
Link to section 'Module' of 'srst2' Module
You can load the modules by:
module load biocontainers
module load srst2
Link to section 'Example job' of 'srst2' Example job
Using #!/bin/sh -l
as shebang in the slurm job script will cause the failure of some biocontainer modules. Please use #!/bin/bash
instead.
To run srst2 on our clusters:
#!/bin/bash
#SBATCH -A myallocation # Allocation name
#SBATCH -t 1:00:00
#SBATCH -N 1
#SBATCH -n 1
#SBATCH --job-name=srst2
#SBATCH --mail-type=FAIL,BEGIN,END
#SBATCH --error=%x-%J-%u.err
#SBATCH --output=%x-%J-%u.out
module --force purge
ml biocontainers srst2
stacks
Link to section 'Introduction' of 'stacks' Introduction
Stacks
is a software pipeline for building loci from RAD-seq.
For more information, please check its website: https://biocontainers.pro/tools/stacks.
Link to section 'Versions' of 'stacks' Versions
- 2.60
Link to section 'Commands' of 'stacks' Commands
- clone_filter
- count_fixed_catalog_snps.py
- cstacks
- denovo_map.pl
- gstacks
- integrate_alignments.py
- kmer_filter
- phasedstacks
- populations
- process_radtags
- process_shortreads
- ref_map.pl
- sstacks
- stacks-dist-extract
- stacks-gdb
- stacks-integrate-alignments
- tsv2bam
- ustacks
Link to section 'Module' of 'stacks' Module
You can load the modules by:
module load biocontainers
module load stacks
Link to section 'Example job' of 'stacks' Example job
Using #!/bin/sh -l
as shebang in the slurm job script will cause the failure of some biocontainer modules. Please use #!/bin/bash
instead.
To run Stacks on our clusters:
#!/bin/bash
#SBATCH -A myallocation # Allocation name
#SBATCH -t 1:00:00
#SBATCH -N 1
#SBATCH -n 8
#SBATCH --job-name=stacks
#SBATCH --mail-type=FAIL,BEGIN,END
#SBATCH --error=%x-%J-%u.err
#SBATCH --output=%x-%J-%u.out
module --force purge
ml biocontainers stacks
denovo_map.pl -T 8 -M 4 -o ./stacks/ \
--samples ./samples --popmap ./popmaps/popmap
star
STAR
: ultrafast universal RNA-seq aligner.
Detailed usage can be found here: https://github.com/alexdobin/STAR
Link to section 'Versions' of 'star' Versions
- 2.7.10a
- 2.7.10b
- 2.7.9a
Link to section 'Commands' of 'star' Commands
- STAR
- STARlong
Link to section 'Module' of 'star' Module
You can load the modules by:
module load biocontainers
module load star/2.7.10a
Link to section 'Example job' of 'star' Example job
Using #!/bin/sh -l
as shebang in the slurm job script will cause the failure of some biocontainer modules. Please use #!/bin/bash
instead.
To run STAR on our our clusters:
#!/bin/bash
#SBATCH -A myallocation # Allocation name
#SBATCH -t 20:00:00
#SBATCH -N 1
#SBATCH -n 24
#SBATCH --job-name=star
#SBATCH --mail-type=FAIL,BEGIN,END
#SBATCH --error=%x-%J-%u.err
#SBATCH --output=%x-%J-%u.out
module --force purge
ml biocontainers star/2.7.10a
STAR --runThreadN 24 --runMode genomeGenerate --genomeDir ref_genome --genomeFastaFiles ref_genome.fasta
STAR --runThreadN 24 --genomeDir ref_genome --readFilesIn seq_1.fastq seq_2.fastq --outSAMtype BAM SortedByCoordinate --outWigType wiggle read2
staramr
Link to section 'Introduction' of 'staramr' Introduction
staramr scans bacterial genome contigs against the ResFinder, PointFinder, and PlasmidFinder databases (used by the ResFinder webservice and other webservices offered by the Center for Genomic Epidemiology) and compiles a summary report of detected antimicrobial resistance genes.
Docker hub: https://hub.docker.com/r/staphb/staramr
Home page: https://github.com/phac-nml/staramr
Link to section 'Versions' of 'staramr' Versions
- 0.7.1
Link to section 'Commands' of 'staramr' Commands
- staramr
Link to section 'Module' of 'staramr' Module
You can load the modules by:
module load biocontainers
module load staramr
Link to section 'Example job' of 'staramr' Example job
Using #!/bin/sh -l
as shebang in the slurm job script will cause the failure of some biocontainer modules. Please use #!/bin/bash
instead.
To run staramr on our clusters:
#!/bin/bash
#SBATCH -A myallocation # Allocation name
#SBATCH -t 1:00:00
#SBATCH -N 1
#SBATCH -n 1
#SBATCH --job-name=staramr
#SBATCH --mail-type=FAIL,BEGIN,END
#SBATCH --error=%x-%J-%u.err
#SBATCH --output=%x-%J-%u.out
module --force purge
ml biocontainers staramr
staramr db info
staramr search \
--pointfinder-organism salmonella \
-o out *.fasta
starfusion
Link to section 'Introduction' of 'starfusion' Introduction
STAR-Fusion
is a component of the Trinity Cancer Transcriptome Analysis Toolkit (CTAT).
Docker hub: https://hub.docker.com/r/trinityctat/starfusion and its home page on Github.
Link to section 'Versions' of 'starfusion' Versions
- 1.11b
Link to section 'Commands' of 'starfusion' Commands
- STAR-Fusion
Link to section 'Module' of 'starfusion' Module
You can load the modules by:
module load biocontainers
module load starfusion
Link to section 'Example job' of 'starfusion' Example job
Using #!/bin/sh -l
as shebang in the slurm job script will cause the failure of some biocontainer modules. Please use #!/bin/bash
instead.
To run STAR-Fusion on our clusters:
#!/bin/bash
#SBATCH -A myallocation # Allocation name
#SBATCH -t 1:00:00
#SBATCH -N 1
#SBATCH -n 24
#SBATCH --job-name=starfusion
#SBATCH --mail-type=FAIL,BEGIN,END
#SBATCH --error=%x-%J-%u.err
#SBATCH --output=%x-%J-%u.out
module --force purge
ml biocontainers starfusion
STAR-Fusion --CPU 24 --left_fq ../star/SRR12095148_1.fastq --right_fq ../star/SRR12095148_2.fastq\
--genome_lib_dir GRCh38_gencode_v33_CTAT_lib_Apr062020.plug-n-play/ctat_genome_lib_build_dir \
--FusionInspector validate \
--denovo_reconstruct \
--examine_coding_effect \
--output_dir STAR-Fusion-output
stream
Link to section 'Introduction' of 'stream' Introduction
STREAM
(Single-cell Trajectories Reconstruction, Exploration And Mapping) is an interactive pipeline capable of disentangling and visualizing complex branching trajectories from both single-cell transcriptomic and epigenomic data.
Docker hub: https://hub.docker.com/r/pinellolab/stream and its home page on Github.
Link to section 'Versions' of 'stream' Versions
- 1.0
Link to section 'Commands' of 'stream' Commands
- python
- python3
Link to section 'Module' of 'stream' Module
You can load the modules by:
module load biocontainers
module load stream
Link to section 'Example job' of 'stream' Example job
Using #!/bin/sh -l
as shebang in the slurm job script will cause the failure of some biocontainer modules. Please use #!/bin/bash
instead.
To run STREAM on our clusters:
#!/bin/bash
#SBATCH -A myallocation # Allocation name
#SBATCH -t 1:00:00
#SBATCH -N 1
#SBATCH -n 1
#SBATCH --job-name=stream
#SBATCH --mail-type=FAIL,BEGIN,END
#SBATCH --error=%x-%J-%u.err
#SBATCH --output=%x-%J-%u.out
module --force purge
ml biocontainers stream
stringdecomposer
Link to section 'Introduction' of 'stringdecomposer' Introduction
Stringdecomposer is a tool for decomposition centromeric assemblies and long reads into monomers.
BioContainers: https://biocontainers.pro/tools/stringdecomposer
Home page: https://github.com/ablab/stringdecomposer
Link to section 'Versions' of 'stringdecomposer' Versions
- 1.1.2
Link to section 'Commands' of 'stringdecomposer' Commands
- stringdecomposer
Link to section 'Module' of 'stringdecomposer' Module
You can load the modules by:
module load biocontainers
module load stringdecomposer
Link to section 'Example job' of 'stringdecomposer' Example job
Using #!/bin/sh -l
as shebang in the slurm job script will cause the failure of some biocontainer modules. Please use #!/bin/bash
instead.
To run stringdecomposer on our clusters:
#!/bin/bash
#SBATCH -A myallocation # Allocation name
#SBATCH -t 1:00:00
#SBATCH -N 1
#SBATCH -n 1
#SBATCH --job-name=stringdecomposer
#SBATCH --mail-type=FAIL,BEGIN,END
#SBATCH --error=%x-%J-%u.err
#SBATCH --output=%x-%J-%u.out
module --force purge
ml biocontainers stringdecomposer
stringtie
StringTie
: efficient transcript assembly and quantitation of RNA-Seq data.
Stringtie employs efficient algorithms for transcript structure recovery and abundance estimation from bulk RNA-Seq reads aligned to a reference genome. It takes as input spliced alignments in coordinate-sorted SAM/BAM/CRAM format and produces a GTF output which consists of assembled transcript structures and their estimated expression levels (FPKM/TPM and base coverage values).
Detailed usage can be found here: https://github.com/gpertea/stringtie
Link to section 'Versions' of 'stringtie' Versions
- 2.1.7
- 2.2.1
Link to section 'Commands' of 'stringtie' Commands
- stringtie
Link to section 'Module' of 'stringtie' Module
You can load the modules by:
module load biocontainers
module load stringtie
Link to section 'Example job' of 'stringtie' Example job
Using #!/bin/sh -l
as shebang in the slurm job script will cause the failure of some biocontainer modules. Please use #!/bin/bash
instead.
To run stringtie on our our clusters:
#!/bin/bash
#SBATCH -A myallocation # Allocation name
#SBATCH -t 20:00:00
#SBATCH -N 1
#SBATCH -n 24
#SBATCH --job-name=stringtie
#SBATCH --mail-type=FAIL,BEGIN,END
#SBATCH --error=%x-%J-%u.err
#SBATCH --output=%x-%J-%u.out
module --force purge
ml biocontainers stringtie
stringtie -o SRR11614710.gtf -G Homo_sapiens.GRCh38.105.gtf SRR11614710Aligned.sortedByCoord.out.bam
strique
Link to section 'Introduction' of 'strique' Introduction
STRique is a python package to analyze repeat expansion and methylation states of short tandem repeats (STR) in Oxford Nanopore Technology (ONT) long read sequencing data.
Docker hub: https://hub.docker.com/r/giesselmann/strique
Home page: https://github.com/giesselmann/STRique
Link to section 'Versions' of 'strique' Versions
- 0.4.2
Link to section 'Commands' of 'strique' Commands
- STRique.py
- STRique_test.py
- fast5Masker.py
Link to section 'Module' of 'strique' Module
You can load the modules by:
module load biocontainers
module load strique
Link to section 'Example job' of 'strique' Example job
Using #!/bin/sh -l
as shebang in the slurm job script will cause the failure of some biocontainer modules. Please use #!/bin/bash
instead.
To run strique on our clusters:
#!/bin/bash
#SBATCH -A myallocation # Allocation name
#SBATCH -t 1:00:00
#SBATCH -N 1
#SBATCH -n 1
#SBATCH --job-name=strique
#SBATCH --mail-type=FAIL,BEGIN,END
#SBATCH --error=%x-%J-%u.err
#SBATCH --output=%x-%J-%u.out
module --force purge
ml biocontainers strique
STRique_test.py
STRique.py index data/ > data/reads.fofn
cat data/c9orf72.sam | STRique.py count ./data/reads.fofn ./models/r9_4_450bps.model ./configs/repeat_config.tsv --config ./configs/STRique.json
structure
Link to section 'Introduction' of 'structure' Introduction
Structure is a software package for using multi-locus genotype data to investigate population structure.
Home page: https://web.stanford.edu/group/pritchardlab/structure.html
Link to section 'Versions' of 'structure' Versions
- 2.3.4
Link to section 'Commands' of 'structure' Commands
- structure
Link to section 'Module' of 'structure' Module
You can load the modules by:
module load biocontainers
module load structure
Link to section 'Example job' of 'structure' Example job
Using #!/bin/sh -l
as shebang in the slurm job script will cause the failure of some biocontainer modules. Please use #!/bin/bash
instead.
To run structure on our clusters:
#!/bin/bash
#SBATCH -A myallocation # Allocation name
#SBATCH -t 1:00:00
#SBATCH -N 1
#SBATCH -n 1
#SBATCH --job-name=structure
#SBATCH --mail-type=FAIL,BEGIN,END
#SBATCH --error=%x-%J-%u.err
#SBATCH --output=%x-%J-%u.out
module --force purge
ml biocontainers structure
subread
Link to section 'Introduction' of 'subread' Introduction
Subread
carries out high-performance read alignment, quantification and mutation discovery. It is a general-purpose read aligner which can be used to map both genomic DNA-seq reads and DNA-seq reads. It uses a new mapping paradigm called seed-and-vote to achieve fast, accurate and scalable read mapping. Subread automatically determines if a read should be globally or locally aligned, therefore particularly powerful in mapping RNA-seq reads. It supports INDEL detection and can map reads with both fixed and variable lengths.
For more information, please check its website: https://biocontainers.pro/tools/subread and its home page: http://subread.sourceforge.net.
Link to section 'Versions' of 'subread' Versions
- 1.6.4
- 2.0.1
Link to section 'Commands' of 'subread' Commands
- detectionCall
- exactSNP
- featureCounts
- flattenGTF
- genRandomReads
- propmapped
- qualityScores
- removeDup
- repair
- subindel
- subjunc
- sublong
- subread-align
- subread-buildindex
- subread-fullscan
- txUnique
Link to section 'Module' of 'subread' Module
You can load the modules by:
module load biocontainers
module load subread
Link to section 'Example job' of 'subread' Example job
Using #!/bin/sh -l
as shebang in the slurm job script will cause the failure of some biocontainer modules. Please use #!/bin/bash
instead.
To run Subread on our clusters:
#!/bin/bash
#SBATCH -A myallocation # Allocation name
#SBATCH -t 1:00:00
#SBATCH -N 1
#SBATCH -n 4
#SBATCH --job-name=subread
#SBATCH --mail-type=FAIL,BEGIN,END
#SBATCH --error=%x-%J-%u.err
#SBATCH --output=%x-%J-%u.out
module --force purge
ml biocontainers subread
featureCounts -s 2 -p -Q 10 -T 4 -a genome.gtf -o featurecounts.txt mapped.bam
survivor
Link to section 'Introduction' of 'survivor' Introduction
SURVIVOR is a tool set for simulating/evaluating SVs, merging and comparing SVs within and among samples, and includes various methods to reformat or summarize SVs.
BioContainers: https://biocontainers.pro/tools/survivor
Home page: https://github.com/fritzsedlazeck/SURVIVOR
Link to section 'Versions' of 'survivor' Versions
- 1.0.7
Link to section 'Commands' of 'survivor' Commands
- SURVIVOR
Link to section 'Module' of 'survivor' Module
You can load the modules by:
module load biocontainers
module load survivor
Link to section 'Example job' of 'survivor' Example job
Using #!/bin/sh -l
as shebang in the slurm job script will cause the failure of some biocontainer modules. Please use #!/bin/bash
instead.
To run survivor on our clusters:
#!/bin/bash
#SBATCH -A myallocation # Allocation name
#SBATCH -t 1:00:00
#SBATCH -N 1
#SBATCH -n 1
#SBATCH --job-name=survivor
#SBATCH --mail-type=FAIL,BEGIN,END
#SBATCH --error=%x-%J-%u.err
#SBATCH --output=%x-%J-%u.out
module --force purge
ml biocontainers survivor
SURVIVOR simSV parameter_file
SURVIVOR simSV ref.fa parameter_file 0.1 0 simulated
SURVIVOR eval caller.vcf simulated.bed 10 eval_res
svaba
Link to section 'Introduction' of 'svaba' Introduction
SvABA is a method for detecting structural variants in sequencing data using genome-wide local assembly.
BioContainers: https://biocontainers.pro/tools/svaba
Home page: https://github.com/walaj/svaba
Link to section 'Versions' of 'svaba' Versions
- 1.1.0
Link to section 'Commands' of 'svaba' Commands
- svaba
Link to section 'Module' of 'svaba' Module
You can load the modules by:
module load biocontainers
module load svaba
Link to section 'Example job' of 'svaba' Example job
Using #!/bin/sh -l
as shebang in the slurm job script will cause the failure of some biocontainer modules. Please use #!/bin/bash
instead.
To run svaba on our clusters:
#!/bin/bash
#SBATCH -A myallocation # Allocation name
#SBATCH -t 1:00:00
#SBATCH -N 1
#SBATCH -n 8
#SBATCH --job-name=svaba
#SBATCH --mail-type=FAIL,BEGIN,END
#SBATCH --error=%x-%J-%u.err
#SBATCH --output=%x-%J-%u.out
module --force purge
ml biocontainers svaba
DBSNP=dbsnp_indel.vcf
TUM_BAM=G15512.HCC1954.1.COST16011_region.bam
NORM_BAM=HCC1954.NORMAL.30x.compare.COST16011_region.bam
CORES=8 ## set any number of cores
REF=Homo_sapiens_assembly19.COST16011_region.fa
svaba run -t $TUM_BAM -n $NORM_BAM \
-p $CORES -D $DBSNP \
-a somatic_run -G $REF
svtools
Link to section 'Introduction' of 'svtools' Introduction
Svtools is a suite of utilities designed to help bioinformaticians construct and explore cohort-level structural variation calls.
Docker hub: https://hub.docker.com/r/halllab/svtools
Home page: https://github.com/hall-lab/svtools
Link to section 'Versions' of 'svtools' Versions
- 0.5.1
Link to section 'Commands' of 'svtools' Commands
- svtools
Link to section 'Module' of 'svtools' Module
You can load the modules by:
module load biocontainers
module load svtools
Link to section 'Example job' of 'svtools' Example job
Using #!/bin/sh -l
as shebang in the slurm job script will cause the failure of some biocontainer modules. Please use #!/bin/bash
instead.
To run svtools on our clusters:
#!/bin/bash
#SBATCH -A myallocation # Allocation name
#SBATCH -t 1:00:00
#SBATCH -N 1
#SBATCH -n 1
#SBATCH --job-name=svtools
#SBATCH --mail-type=FAIL,BEGIN,END
#SBATCH --error=%x-%J-%u.err
#SBATCH --output=%x-%J-%u.out
module --force purge
ml biocontainers svtools
svtyper
Link to section 'Introduction' of 'svtyper' Introduction
SVTyper performs breakpoint genotyping of structural variants (SVs) using whole genome sequencing data. svtyper is the original implementation of the genotyping algorithm, and works with multiple samples. svtyper-sso is an alternative implementation of svtyper that is optimized for genotyping a single sample. svtyper-sso is a parallelized implementation of svtyper that takes advantage of multiple CPU cores via the multiprocessing module. svtyper-sso can offer a 2x or more speedup (depending on how many CPU cores used) in genotyping a single sample. NOTE: svtyper-sso is not yet stable. There are minor logging differences between the two and svtyper-sso may exit with an error prematurely when processing CRAM files.
BioContainers: https://biocontainers.pro/tools/svtyper
Home page: https://github.com/hall-lab/svtyper
Link to section 'Versions' of 'svtyper' Versions
- 0.7.1
Link to section 'Commands' of 'svtyper' Commands
- svtyper
- svtyper-sso
- python
- python2
Link to section 'Module' of 'svtyper' Module
You can load the modules by:
module load biocontainers
module load svtyper
Link to section 'Example job' of 'svtyper' Example job
Using #!/bin/sh -l
as shebang in the slurm job script will cause the failure of some biocontainer modules. Please use #!/bin/bash
instead.
To run svtyper on our clusters:
#!/bin/bash
#SBATCH -A myallocation # Allocation name
#SBATCH -t 1:00:00
#SBATCH -N 1
#SBATCH -n 1
#SBATCH --job-name=svtyper
#SBATCH --mail-type=FAIL,BEGIN,END
#SBATCH --error=%x-%J-%u.err
#SBATCH --output=%x-%J-%u.out
module --force purge
ml biocontainers svtyper
svtyper \
-i data/example.vcf \
-B data/NA12878.target_loci.sorted.bam \
-l data/NA12878.bam.json \
> out.vcf
swat
Link to section 'Introduction' of 'swat' Introduction
swat
is a program for searching one or more DNA or protein query sequences, or a query profile, against a sequence database, using an efficient implementation of the Smith-Waterman or Needleman-Wunsch algorithms with linear (affine) gap penalties.
For more information, please check its home page: http://www.phrap.org/phredphrapconsed.html#block_phrap.
Link to section 'Versions' of 'swat' Versions
- 1.090518
Link to section 'Commands' of 'swat' Commands
- swat
Link to section 'Module' of 'swat' Module
You can load the modules by:
module load biocontainers
module load swat
Link to section 'Example job' of 'swat' Example job
Using #!/bin/sh -l
as shebang in the slurm job script will cause the failure of some biocontainer modules. Please use #!/bin/bash
instead.
To run swat on our clusters:
#!/bin/bash
#SBATCH -A myallocation # Allocation name
#SBATCH -t 1:00:00
#SBATCH -N 1
#SBATCH -n 1
#SBATCH --job-name=swat
#SBATCH --mail-type=FAIL,BEGIN,END
#SBATCH --error=%x-%J-%u.err
#SBATCH --output=%x-%J-%u.out
module --force purge
ml biocontainers swat
syri
Link to section 'Introduction' of 'syri' Introduction
Syri compares alignments between two chromosome-level assemblies and identifies synteny and structural rearrangements.
Home page: https://github.com/schneebergerlab/syri
Link to section 'Versions' of 'syri' Versions
- 1.6
Link to section 'Commands' of 'syri' Commands
- syri
Link to section 'Module' of 'syri' Module
You can load the modules by:
module load biocontainers
module load syri
Link to section 'Example job' of 'syri' Example job
Using #!/bin/sh -l
as shebang in the slurm job script will cause the failure of some biocontainer modules. Please use #!/bin/bash
instead.
To run syri on our clusters:
#!/bin/bash
#SBATCH -A myallocation # Allocation name
#SBATCH -t 1:00:00
#SBATCH -N 1
#SBATCH -n 1
#SBATCH --job-name=syri
#SBATCH --mail-type=FAIL,BEGIN,END
#SBATCH --error=%x-%J-%u.err
#SBATCH --output=%x-%J-%u.out
module --force purge
ml biocontainers syri
syri -c out.sam -r refgenome -q qrygenome -k -F S
t-coffee
Link to section 'Introduction' of 't-coffee' Introduction
T-coffee
is a multiple sequence alignment software using a progressive approach.
For more information, please check its website: https://biocontainers.pro/tools/t-coffee and its home page on Github.
Link to section 'Versions' of 't-coffee' Versions
- 13.45.0.4846264
Link to section 'Commands' of 't-coffee' Commands
- t_coffee
Link to section 'Module' of 't-coffee' Module
You can load the modules by:
module load biocontainers
module load t-coffee
Link to section 'Example job' of 't-coffee' Example job
Using #!/bin/sh -l
as shebang in the slurm job script will cause the failure of some biocontainer modules. Please use #!/bin/bash
instead.
To run T-coffee on our clusters:
#!/bin/bash
#SBATCH -A myallocation # Allocation name
#SBATCH -t 1:00:00
#SBATCH -N 1
#SBATCH -n 1
#SBATCH --job-name=t-coffee
#SBATCH --mail-type=FAIL,BEGIN,END
#SBATCH --error=%x-%J-%u.err
#SBATCH --output=%x-%J-%u.out
module --force purge
ml biocontainers t-coffee
t_coffee OG0002077.fa -mode expresso
talon
Link to section 'Introduction' of 'talon' Introduction
Talon
is a Python package for identifying and quantifying known and novel genes/isoforms in long-read transcriptome data sets.
For more information, please check its website: https://biocontainers.pro/tools/talon and its home page on Github.
Link to section 'Versions' of 'talon' Versions
- 5.0
Link to section 'Commands' of 'talon' Commands
- talon
- talon_abundance
- talon_create_GTF
- talon_fetch_reads
- talon_filter_transcripts
- talon_generate_report
- talon_get_sjs
- talon_initialize_database
- talon_label_reads
- talon_reformat_gtf
- talon_summarize
Link to section 'Module' of 'talon' Module
You can load the modules by:
module load biocontainers
module load talon
Link to section 'Example job' of 'talon' Example job
Using #!/bin/sh -l
as shebang in the slurm job script will cause the failure of some biocontainer modules. Please use #!/bin/bash
instead.
To run Talon on our clusters:
#!/bin/bash
#SBATCH -A myallocation # Allocation name
#SBATCH -t 1:00:00
#SBATCH -N 1
#SBATCH -n 1
#SBATCH --job-name=talon
#SBATCH --mail-type=FAIL,BEGIN,END
#SBATCH --error=%x-%J-%u.err
#SBATCH --output=%x-%J-%u.out
module --force purge
ml biocontainers talon
targetp
Link to section 'Introduction' of 'targetp' Introduction
TargetP-2.0 tool predicts the presence of N-terminal presequences: signal peptide (SP), mitochondrial transit peptide (mTP), chloroplast transit peptide (cTP) or thylakoid luminal transit peptide (luTP). For the sequences predicted to contain an N-terminal presequence a potential cleavage site is also predicted.
Home page: https://services.healthtech.dtu.dk/service.php?TargetP-2.0
Link to section 'Versions' of 'targetp' Versions
- 2.0
Link to section 'Commands' of 'targetp' Commands
- targetp
Link to section 'Module' of 'targetp' Module
You can load the modules by:
module load biocontainers
module load targetp
Link to section 'Example job' of 'targetp' Example job
Using #!/bin/sh -l
as shebang in the slurm job script will cause the failure of some biocontainer modules. Please use #!/bin/bash
instead.
To run targetp on our clusters:
#!/bin/bash
#SBATCH -A myallocation # Allocation name
#SBATCH -t 1:00:00
#SBATCH -N 1
#SBATCH -n 1
#SBATCH --job-name=targetp
#SBATCH --mail-type=FAIL,BEGIN,END
#SBATCH --error=%x-%J-%u.err
#SBATCH --output=%x-%J-%u.out
module --force purge
ml biocontainers targetp
tassel
Link to section 'Introduction' of 'tassel' Introduction
TASSEL is a software package used to evaluate traits associations, evolutionary patterns, and linkage disequilibrium.
Home page: https://www.maizegenetics.net/tassel
Link to section 'Versions' of 'tassel' Versions
- 5.0
Link to section 'Commands' of 'tassel' Commands
- run_pipeline.pl
- start_tassel.pl
- Tassel5
Link to section 'Module' of 'tassel' Module
You can load the modules by:
module load biocontainers
module load tassel
Link to section 'Example job' of 'tassel' Example job
Using #!/bin/sh -l
as shebang in the slurm job script will cause the failure of some biocontainer modules. Please use #!/bin/bash
instead.
To run tassel on our clusters:
#!/bin/bash
#SBATCH -A myallocation # Allocation name
#SBATCH -t 1:00:00
#SBATCH -N 1
#SBATCH -n 1
#SBATCH --job-name=tassel
#SBATCH --mail-type=FAIL,BEGIN,END
#SBATCH --error=%x-%J-%u.err
#SBATCH --output=%x-%J-%u.out
module --force purge
ml biocontainers tassel
taxonkit
Link to section 'Introduction' of 'taxonkit' Introduction
Taxonkit
is a practical and efficient NCBI taxonomy toolkit.
For more information, please check its website: https://biocontainers.pro/tools/taxonkit and its home page on Github.
Link to section 'Versions' of 'taxonkit' Versions
- 0.9.0
Link to section 'Commands' of 'taxonkit' Commands
- taxonkit
Link to section 'Module' of 'taxonkit' Module
You can load the modules by:
module load biocontainers
module load taxonkit
Link to section 'Example job' of 'taxonkit' Example job
Using #!/bin/sh -l
as shebang in the slurm job script will cause the failure of some biocontainer modules. Please use #!/bin/bash
instead.
To run Taxonkit on our clusters:
#!/bin/bash
#SBATCH -A myallocation # Allocation name
#SBATCH -t 1:00:00
#SBATCH -N 1
#SBATCH -n 1
#SBATCH --job-name=taxonkit
#SBATCH --mail-type=FAIL,BEGIN,END
#SBATCH --error=%x-%J-%u.err
#SBATCH --output=%x-%J-%u.out
module --force purge
ml biocontainers taxonkit
taxonkit list --show-rank --show-name --indent " " --ids 9605,239934
tetranscripts
Link to section 'Introduction' of 'tetranscripts' Introduction
Tetranscripts
is a package for including transposable elements in differential enrichment analysis of sequencing datasets.
For more information, please check its website: https://biocontainers.pro/tools/tetranscripts and its home page on Github.
Link to section 'Versions' of 'tetranscripts' Versions
- 2.2.1
Link to section 'Commands' of 'tetranscripts' Commands
- TEtranscripts
- TEcount
Link to section 'Module' of 'tetranscripts' Module
You can load the modules by:
module load biocontainers
module load tetranscripts
Link to section 'Example job' of 'tetranscripts' Example job
Using #!/bin/sh -l
as shebang in the slurm job script will cause the failure of some biocontainer modules. Please use #!/bin/bash
instead.
To run Tetranscripts on our clusters:
#!/bin/bash
#SBATCH -A myallocation # Allocation name
#SBATCH -t 1:00:00
#SBATCH -N 1
#SBATCH -n 1
#SBATCH --job-name=tetranscripts
#SBATCH --mail-type=FAIL,BEGIN,END
#SBATCH --error=%x-%J-%u.err
#SBATCH --output=%x-%J-%u.out
module --force purge
ml biocontainers tetranscripts
TEtranscripts --format BAM --mode multi \
-t treatment_sample1.bam treatment_sample2.bam treatment_sample3.bam \
-c control_sample1.bam control_sample2.bam control_sample3.bam \
--GTF genic-GTF-file \
--GTF genic-GTF-file \
--project sample_nosort_test
tiara
Link to section 'Introduction' of 'tiara' Introduction
Tiara
is a deep-learning-based approach for identification of eukaryotic sequences in the metagenomic data powered by PyTorch.
Docker hub: https://hub.docker.com/r/zhan4429/tiara and its home page on Github.
Link to section 'Versions' of 'tiara' Versions
- 1.0.2
Link to section 'Commands' of 'tiara' Commands
- tiara
Link to section 'Module' of 'tiara' Module
You can load the modules by:
module load biocontainers
module load tiara
Link to section 'Example job' of 'tiara' Example job
Using #!/bin/sh -l
as shebang in the slurm job script will cause the failure of some biocontainer modules. Please use #!/bin/bash
instead.
To run Tiara on our clusters:
#!/bin/bash
#SBATCH -A myallocation # Allocation name
#SBATCH -t 1:00:00
#SBATCH -N 1
#SBATCH -n 24
#SBATCH --job-name=tiara
#SBATCH --mail-type=FAIL,BEGIN,END
#SBATCH --error=%x-%J-%u.err
#SBATCH --output=%x-%J-%u.out
module --force purge
ml biocontainers tiara
tiara -t 24 -i archaea_fr.fasta -o archaea_out.txt
tiara -t 24 -i bacteria_fr.fasta -o bacteria_out.txt
tiara -t 24 -i eukarya_fr.fasta -o eukarya_out.txt
tiara -t 24 -i mitochondria_fr.fasta -o mitochondria_out.txt
tiara -t 24 -i plast_fr.fasta -o plast_out.txt
tiara -t 24 -i total.fasta -o mix_out.txt --tf all -p 0.65 0.60 --probabilities
tigmint
Link to section 'Introduction' of 'tigmint' Introduction
Tigmint identifies and corrects misassemblies using linked (e.g. MGI's stLFR, 10x Genomics Chromium) or long (e.g. Oxford Nanopore Technologies long reads) DNA sequencing reads. The reads are first aligned to the assembly, and the extents of the large DNA molecules are inferred from the alignments of the reads. The physical coverage of the large molecules is more consistent and less prone to coverage dropouts than that of the short read sequencing data. The sequences are cut at positions that have insufficient spanning molecules. Tigmint outputs a BED file of these cut points, and a FASTA file of the cut sequences. For more information, please check: Home page: https://github.com/bcgsc/tigmint
Link to section 'Versions' of 'tigmint' Versions
- 1.2.6
Link to section 'Commands' of 'tigmint' Commands
- tigmint
- tigmint-arcs-tsv
- tigmint-cut
- tigmint-make
- tigmint_estimate_dist.py
- tigmint_molecule.py
- tigmint_molecule_paf.py
Link to section 'Module' of 'tigmint' Module
You can load the modules by:
module load biocontainers
module load tigmint
Link to section 'Example job' of 'tigmint' Example job
Using #!/bin/sh -l
as shebang in the slurm job script will cause the failure of some biocontainer modules. Please use #!/bin/bash
instead.
To run tigmint on our clusters:
#!/bin/bash
#SBATCH -A myallocation # Allocation name
#SBATCH -t 1:00:00
#SBATCH -N 1
#SBATCH -n 1
#SBATCH --job-name=tigmint
#SBATCH --mail-type=FAIL,BEGIN,END
#SBATCH --error=%x-%J-%u.err
#SBATCH --output=%x-%J-%u.out
module --force purge
ml biocontainers tigmint
tobias
Link to section 'Introduction' of 'tobias' Introduction
Tobias
is a collection of command-line bioinformatics tools for performing footprinting analysis on ATAC-seq data.
For more information, please check its website: https://biocontainers.pro/tools/tobias and its home page on Github.
Link to section 'Versions' of 'tobias' Versions
- 0.13.3
Link to section 'Commands' of 'tobias' Commands
- TOBIAS
Link to section 'Module' of 'tobias' Module
You can load the modules by:
module load biocontainers
module load tobias
Link to section 'Example job' of 'tobias' Example job
Using #!/bin/sh -l
as shebang in the slurm job script will cause the failure of some biocontainer modules. Please use #!/bin/bash
instead.
To run Tobias on our clusters:
#!/bin/bash
#SBATCH -A myallocation # Allocation name
#SBATCH -t 1:00:00
#SBATCH -N 1
#SBATCH -n 8
#SBATCH --job-name=tobias
#SBATCH --mail-type=FAIL,BEGIN,END
#SBATCH --error=%x-%J-%u.err
#SBATCH --output=%x-%J-%u.out
module --force purge
ml biocontainers tobias
TOBIAS DownloadData --bucket data-tobias-2020
mv data-tobias-2020/ test_data/
TOBIAS PlotAggregate --TFBS test_data/BATF_all.bed \
--signals test_data/Bcell_corrected.bw test_data/Tcell_corrected.bw \
--output BATFJUN_footprint_comparison_all.pdf \
--share_y both --plot_boundaries --signal-on-x
TOBIAS BINDetect --motifs test_data/motifs.jaspar \
--signals test_data/Bcell_footprints.bw test_data/Tcell_footprints.bw \
--genome test_data/genome.fa.gz \
--peaks test_data/merged_peaks_annotated.bed \
--peak_header test_data/merged_peaks_annotated_header.txt \
--outdir BINDetect_output --cond_names Bcell Tcell --cores 8
TOBIAS ATACorrect --bam test_data/Bcell.bam \
--genome test_data/genome.fa.gz \
--peaks test_data/merged_peaks.bed \
--blacklist test_data/blacklist.bed \
--outdir ATACorrect_test --cores 8
TOBIAS FootprintScores --signal test_data/Bcell_corrected.bw \
--regions test_data/merged_peaks.bed \
--output Bcell_footprints.bw --cores 8
tombo
Link to section 'Introduction' of 'tombo' Introduction
Tombo
is a suite of tools primarily for the identification of modified nucleotides from nanopore sequencing data. Tombo also provides tools for the analysis and visualization of raw nanopore signal.
For more information, please check its website: https://biocontainers.pro/tools/ont-tombo and its home page on Github.
Link to section 'Versions' of 'tombo' Versions
- 1.5.1
Link to section 'Commands' of 'tombo' Commands
- tombo
Link to section 'Module' of 'tombo' Module
You can load the modules by:
module load biocontainers
module load tombo
Link to section 'Example job' of 'tombo' Example job
Using #!/bin/sh -l
as shebang in the slurm job script will cause the failure of some biocontainer modules. Please use #!/bin/bash
instead.
To run Tombo on our clusters:
#!/bin/bash
#SBATCH -A myallocation # Allocation name
#SBATCH -t 1:00:00
#SBATCH -N 1
#SBATCH -n 4
#SBATCH --job-name=tombo
#SBATCH --mail-type=FAIL,BEGIN,END
#SBATCH --error=%x-%J-%u.err
#SBATCH --output=%x-%J-%u.out
module --force purge
ml biocontainers tombo
tombo resquiggle path/to/fast5s/ genome.fasta --processes 4 --num-most-common-errors 5
tombo detect_modifications alternative_model --fast5-basedirs path/to/fast5s/ \
--statistics-file-basename native.e_coli_sample \
--alternate-bases dam dcm --processes 4
# plot raw signal at most significant dcm locations
tombo plot most_significant --fast5-basedirs path/to/fast5s/ \
--statistics-filename native.e_coli_sample.dcm.tombo.stats \
--plot-standard-model --plot-alternate-model dcm \
--pdf-filename sample.most_significant_dcm_sites.pdf
# produces wig file with estimated fraction of modified reads at each valid reference site
tombo text_output browser_files --statistics-filename native.e_coli_sample.dam.tombo.stats \
--file-types dampened_fraction --browser-file-basename native.e_coli_sample.dam
# also produce successfully processed reads coverage file for reference
tombo text_output browser_files --fast5-basedirs path/to/fast5s/ \
--file-types coverage --browser-file-basename native.e_coli_sample
tophat
Link to section 'Introduction' of 'tophat' Introduction
TopHat
is a fast splice junction mapper for RNA-Seq reads. It aligns RNA-Seq reads to mammalian-sized genomes using the ultra high-throughput short read aligner Bowtie, and then analyzes the mapping results to identify splice junctions between exons.
For more information, please check its website: https://biocontainers.pro/tools/tophat and its home page: https://ccb.jhu.edu/software/tophat/index.shtml.
Link to section 'Versions' of 'tophat' Versions
- 2.1.1-py27
Link to section 'Commands' of 'tophat' Commands
- tophat
- tophat2
Link to section 'Module' of 'tophat' Module
You can load the modules by:
module load biocontainers
module load tophat
Link to section 'Example job' of 'tophat' Example job
Using #!/bin/sh -l
as shebang in the slurm job script will cause the failure of some biocontainer modules. Please use #!/bin/bash
instead.
To run TopHat on our clusters:
#!/bin/bash
#SBATCH -A myallocation # Allocation name
#SBATCH -t 1:00:00
#SBATCH -N 1
#SBATCH -n 1
#SBATCH --job-name=tophat
#SBATCH --mail-type=FAIL,BEGIN,END
#SBATCH --error=%x-%J-%u.err
#SBATCH --output=%x-%J-%u.out
module --force purge
ml biocontainers tophat
tophat -r 20 test_ref reads_1.fq reads_2.fq
tpmcalculator
TPMCalculator
quantifies mRNA abundance directly from the alignments by parsing BAM files.
Detailed usage can be found here: https://github.com/ncbi/TPMCalculator
Link to section 'Versions' of 'tpmcalculator' Versions
- 0.0.3
- 0.0.4
Link to section 'Commands' of 'tpmcalculator' Commands
- TPMCalculator
Link to section 'Module' of 'tpmcalculator' Module
You can load the modules by:
module load biocontainers
module load tpmcalculator
Link to section 'Example job' of 'tpmcalculator' Example job
Using #!/bin/sh -l
as shebang in the slurm job script will cause the failure of some biocontainer modules. Please use #!/bin/bash
instead.
To run tpmcalculator on our our clusters:
#!/bin/bash
#SBATCH -A myallocation # Allocation name
#SBATCH -t 10:00:00
#SBATCH -N 1
#SBATCH -n 12
#SBATCH --job-name=tpmcalculator
#SBATCH --mail-type=FAIL,BEGIN,END
#SBATCH --error=%x-%J-%u.err
#SBATCH --output=%x-%J-%u.out
module --force purge
ml biocontainers transdecoder
TPMCalculator -g Homo_sapiens.GRCh38.105.chr.gtf -b SRR12095148Aligned.sortedByCoord.out.bam
transabyss
Link to section 'Introduction' of 'transabyss' Introduction
Transabyss
is a tool for De novo assembly of RNAseq data using ABySS.
For more information, please check its home page on Github.
Link to section 'Versions' of 'transabyss' Versions
- 2.0.1
Link to section 'Commands' of 'transabyss' Commands
- transabyss
- transabyss-merge
Link to section 'Module' of 'transabyss' Module
You can load the modules by:
module load biocontainers
module load transabyss
Link to section 'Example job' of 'transabyss' Example job
Using #!/bin/sh -l
as shebang in the slurm job script will cause the failure of some biocontainer modules. Please use #!/bin/bash
instead.
To run Transabyss on our clusters:
#!/bin/bash
#SBATCH -A myallocation # Allocation name
#SBATCH -t 1:00:00
#SBATCH -N 1
#SBATCH -n 12
#SBATCH --job-name=transabyss
#SBATCH --mail-type=FAIL,BEGIN,END
#SBATCH --error=%x-%J-%u.err
#SBATCH --output=%x-%J-%u.out
module --force purge
ml biocontainers transabyss
transabyss --name SRR12095148 \
--pe SRR12095148_1.fastq SRR12095148_2.fastq \
--outdir SRR12095148_assembly --threads 12
transdecoder
TransDecoder
identifies candidate coding regions within transcript sequences, such as those generated by de novo RNA-Seq transcript assembly using Trinity, or constructed based on RNA-Seq alignments to the genome using Tophat and Cufflinks.
- TransDecoder identifies likely coding sequences based on the following criteria:
- a minimum length open reading frame (ORF) is found in a transcript sequence
- a log-likelihood score similar to what is computed by the GeneID software is > 0.
- the above coding score is greatest when the ORF is scored in the 1st reading frame as compared to scores in the other 2 forward reading frames.
- if a candidate ORF is found fully encapsulated by the coordinates of another candidate ORF, the longer one is reported. However, a single transcript can report multiple ORFs (allowing for operons, chimeras, etc).
- a PSSM is built/trained/used to refine the start codon prediction.
- optional the putative peptide has a match to a Pfam domain above the noise cutoff score.
Detailed usage can be found here: https://github.com/TransDecoder/TransDecoder/wiki#running-transdecoder
Link to section 'Versions' of 'transdecoder' Versions
- 5.5.0
Link to section 'Commands' of 'transdecoder' Commands
- TransDecoder.LongOrfs
- TransDecoder.Predict
- cdna_alignment_orf_to_genome_orf.pl
- compute_base_probs.pl
- exclude_similar_proteins.pl
- fasta_prot_checker.pl
- ffindex_resume.pl
- gene_list_to_gff.pl
- get_FL_accs.pl
- get_longest_ORF_per_transcript.pl
- get_top_longest_fasta_entries.pl
- gff3_file_to_bed.pl
- gff3_file_to_proteins.pl
- gff3_gene_to_gtf_format.pl
- gtf_genome_to_cdna_fasta.pl
- gtf_to_alignment_gff3.pl
- gtf_to_bed.pl
- nr_ORFs_gff3.pl
- pfam_runner.pl
- refine_gff3_group_iso_strip_utrs.pl
- refine_hexamer_scores.pl
- remove_eclipsed_ORFs.pl
- score_CDS_likelihood_all_6_frames.pl
- select_best_ORFs_per_transcript.pl
- seq_n_baseprobs_to_loglikelihood_vals.pl
- start_codon_refinement.pl
- train_start_PWM.pl
- uri_unescape.pl
Link to section 'Module' of 'transdecoder' Module
You can load the modules by:
module load biocontainers
module load transdecoder
Link to section 'Example job' of 'transdecoder' Example job
Using #!/bin/sh -l
as shebang in the slurm job script will cause the failure of some biocontainer modules. Please use #!/bin/bash
instead.
To run transdecoder on our our clusters:
#!/bin/bash
#SBATCH -A myallocation # Allocation name
#SBATCH -t 20:00:00
#SBATCH -N 1
#SBATCH -n 24
#SBATCH --job-name=transdecoder
#SBATCH --mail-type=FAIL,BEGIN,END
#SBATCH --error=%x-%J-%u.err
#SBATCH --output=%x-%J-%u.out
module --force purge
ml biocontainers transdecoder
gtf_genome_to_cdna_fasta.pl transcripts.gtf test.genome.fasta > transcripts.fasta
gtf_to_alignment_gff3.pl transcripts.gtf > transcripts.gff3
TransDecoder.LongOrfs -t transcripts.fasta
TransDecoder.Predict -t transcripts.fasta
transrate
Link to section 'Introduction' of 'transrate' Introduction
Transrate is software for de-novo transcriptome assembly quality analysis.
Docker hub: https://hub.docker.com/r/arnaudmeng/transrate
Home page: http://hibberdlab.com/transrate/
Link to section 'Versions' of 'transrate' Versions
- 1.0.3
Link to section 'Commands' of 'transrate' Commands
- transrate
Link to section 'Module' of 'transrate' Module
You can load the modules by:
module load biocontainers
module load transrate
Link to section 'Example job' of 'transrate' Example job
Using #!/bin/sh -l
as shebang in the slurm job script will cause the failure of some biocontainer modules. Please use #!/bin/bash
instead.
To run transrate on our clusters:
#!/bin/bash
#SBATCH -A myallocation # Allocation name
#SBATCH -t 1:00:00
#SBATCH -N 1
#SBATCH -n 12
#SBATCH --job-name=transrate
#SBATCH --mail-type=FAIL,BEGIN,END
#SBATCH --error=%x-%J-%u.err
#SBATCH --output=%x-%J-%u.out
module --force purge
ml biocontainers transrate
transrate --assembly mm10/Mus_musculus.GRCm38.cds.all.fa \
--left seq_1.fq.gz \
--right seq_2.fq.gz \
--threads 12
transvar
Link to section 'Introduction' of 'transvar' Introduction
Transvar
is a multi-way annotator for genetic elements and genetic variations.
Docker hub: https://hub.docker.com/r/zhouwanding/transvar and its home page: https://bioinformatics.mdanderson.org/public-software/transvar/.
Link to section 'Versions' of 'transvar' Versions
- 2.5.9
Link to section 'Commands' of 'transvar' Commands
- transvar
Link to section 'Module' of 'transvar' Module
You can load the modules by:
module load biocontainers
module load transvar
Link to section 'Example job' of 'transvar' Example job
Using #!/bin/sh -l
as shebang in the slurm job script will cause the failure of some biocontainer modules. Please use #!/bin/bash
instead.
To run Transvar on our clusters:
#!/bin/bash
#SBATCH -A myallocation # Allocation name
#SBATCH -t 1:00:00
#SBATCH -N 1
#SBATCH -n 1
#SBATCH --job-name=transvar
#SBATCH --mail-type=FAIL,BEGIN,END
#SBATCH --error=%x-%J-%u.err
#SBATCH --output=%x-%J-%u.out
module --force purge
ml biocontainers transvar
# set up databases
transvar config --download_anno --refversion hg19
# in case you don't have a reference
transvar config --download_ref --refversion hg19
transvar panno -i 'PIK3CA:p.E545K' --ucsc --ccds
trax
Link to section 'Introduction' of 'trax' Introduction
tRAX
(tRNA Analysis of eXpression) is a software package built for in-depth analyses of tRNA-derived small RNAs (tDRs), mature tRNAs, and inference of RNA modifications from high-throughput small RNA sequencing data.
Docker hub: https://hub.docker.com/r/ucsclowelab/trax and its home page on Github.
Link to section 'Versions' of 'trax' Versions
- 1.0.0
Link to section 'Commands' of 'trax' Commands
- TestRun.bash
- quickdb.bash
- maketrnadb.py
- trimadapters.py
- processamples.py
Link to section 'Module' of 'trax' Module
You can load the modules by:
module load biocontainers
module load trax
Link to section 'Example job' of 'trax' Example job
Using #!/bin/sh -l
as shebang in the slurm job script will cause the failure of some biocontainer modules. Please use #!/bin/bash
instead.
To run tRAX on our clusters:
#!/bin/bash
#SBATCH -A myallocation # Allocation name
#SBATCH -t 1:00:00
#SBATCH -N 1
#SBATCH -n 1
#SBATCH --job-name=trax
#SBATCH --mail-type=FAIL,BEGIN,END
#SBATCH --error=%x-%J-%u.err
#SBATCH --output=%x-%J-%u.out
module --force purge
ml biocontainers trax
treetime
Link to section 'Introduction' of 'treetime' Introduction
Treetime
is a tool for maximum likelihood dating and ancestral sequence inference.
For more information, please check its website: https://biocontainers.pro/tools/treetime and its home page on Github.
Link to section 'Versions' of 'treetime' Versions
- 0.8.6
- 0.9.4
Link to section 'Commands' of 'treetime' Commands
- treetime
Link to section 'Module' of 'treetime' Module
You can load the modules by:
module load biocontainers
module load treetime
Link to section 'Example job' of 'treetime' Example job
Using #!/bin/sh -l
as shebang in the slurm job script will cause the failure of some biocontainer modules. Please use #!/bin/bash
instead.
To run Treetime on our clusters:
#!/bin/bash
#SBATCH -A myallocation # Allocation name
#SBATCH -t 1:00:00
#SBATCH -N 1
#SBATCH -n 1
#SBATCH --job-name=treetime
#SBATCH --mail-type=FAIL,BEGIN,END
#SBATCH --error=%x-%J-%u.err
#SBATCH --output=%x-%J-%u.out
module --force purge
ml biocontainers treetime
treetime ancestral --aln input.fasta --tree input.nwk
trim-galore
Link to section 'Introduction' of 'trim-galore' Introduction
Trim-galore
is a wrapper tool that automates quality and adapter trimming to FastQ files.
For more information, please check its website: https://biocontainers.pro/tools/trim-galore and its home page: https://www.bioinformatics.babraham.ac.uk/projects/trim_galore/.
Link to section 'Versions' of 'trim-galore' Versions
- 0.6.7
Link to section 'Commands' of 'trim-galore' Commands
- trim_galore
Link to section 'Module' of 'trim-galore' Module
You can load the modules by:
module load biocontainers
module load trim-galore
Link to section 'Example job' of 'trim-galore' Example job
Using #!/bin/sh -l
as shebang in the slurm job script will cause the failure of some biocontainer modules. Please use #!/bin/bash
instead.
To run Trim-galore on our clusters:
#!/bin/bash
#SBATCH -A myallocation # Allocation name
#SBATCH -t 1:00:00
#SBATCH -N 1
#SBATCH -n 4
#SBATCH --job-name=trim-galore
#SBATCH --mail-type=FAIL,BEGIN,END
#SBATCH --error=%x-%J-%u.err
#SBATCH --output=%x-%J-%u.out
module --force purge
ml biocontainers trim-galore
trim_galore --paired --fastqc --length 20 -o sample1_trimmed Sample1_1.fq Sample1_2.fq
trimal
Link to section 'Introduction' of 'trimal' Introduction
Trimal
is a tool for the automated removal of spurious sequences or poorly aligned regions from a multiple sequence alignment.
For more information, please check its website: https://biocontainers.pro/tools/trimal and its home page: http://trimal.cgenomics.org.
Link to section 'Versions' of 'trimal' Versions
- 1.4.1
Link to section 'Commands' of 'trimal' Commands
- trimal
- readal
- statal
Link to section 'Module' of 'trimal' Module
You can load the modules by:
module load biocontainers
module load trimal
Link to section 'Example job' of 'trimal' Example job
Using #!/bin/sh -l
as shebang in the slurm job script will cause the failure of some biocontainer modules. Please use #!/bin/bash
instead.
To run Trimal on our clusters:
#!/bin/bash
#SBATCH -A myallocation # Allocation name
#SBATCH -t 1:00:00
#SBATCH -N 1
#SBATCH -n 1
#SBATCH --job-name=trimal
#SBATCH --mail-type=FAIL,BEGIN,END
#SBATCH --error=%x-%J-%u.err
#SBATCH --output=%x-%J-%u.out
module --force purge
ml biocontainers trimal
trimal -in input.fasta -out output1 -htmlout output1.html -gt 1
trimmomatic
Link to section 'Introduction' of 'trimmomatic' Introduction
Trimmomatic
is a flexible read trimming tool for Illumina NGS data.
For more information, please check its website: https://biocontainers.pro/tools/trimmomatic.
Link to section 'Versions' of 'trimmomatic' Versions
- 0.39
Link to section 'Commands' of 'trimmomatic' Commands
- trimmomatic
Link to section 'Module' of 'trimmomatic' Module
You can load the modules by:
module load biocontainers
module load trimmomatic
Link to section 'Example job' of 'trimmomatic' Example job
Using #!/bin/sh -l
as shebang in the slurm job script will cause the failure of some biocontainer modules. Please use #!/bin/bash
instead.
To run Trimmomatic on our clusters:
#!/bin/bash
#SBATCH -A myallocation # Allocation name
#SBATCH -t 1:00:00
#SBATCH -N 1
#SBATCH -n 8
#SBATCH --job-name=trimmomatic
#SBATCH --mail-type=FAIL,BEGIN,END
#SBATCH --error=%x-%J-%u.err
#SBATCH --output=%x-%J-%u.out
module --force purge
ml biocontainers trimmomatic
trimmomatic PE -threads 8 \
input_forward.fq.gz input_reverse.fq.gz \
output_forward_paired.fq.gz output_forward_unpaired.fq.gz \
output_reverse_paired.fq.gz output_reverse_unpaired.fq.gz \
ILLUMINACLIP:TruSeq3-PE.fa:2:30:10:2:True LEADING:3 TRAILING:3 MINLEN:36
trinity
Link to section 'Introduction' of 'trinity' Introduction
Trinity
assembles transcript sequences from Illumina RNA-Seq data.
For more information, please check its website: https://biocontainers.pro/tools/trinity and its home page on Github.
Link to section 'Versions' of 'trinity' Versions
- 2.12.0
- 2.13.2
- 2.14.0
- 2.15.0
Link to section 'Commands' of 'trinity' Commands
- Trinity
- TrinityStats.pl
- Trinity_gene_splice_modeler.py
- ace2sam
- align_and_estimate_abundance.pl
- analyze_blastPlus_topHit_coverage.pl
- analyze_diff_expr.pl
- blast2sam.pl
- bowtie
- bowtie2
- bowtie2-build
- bowtie2-inspect
- bowtie2sam.pl
- contig_ExN50_statistic.pl
- define_clusters_by_cutting_tree.pl
- export2sam.pl
- extract_supertranscript_from_reference.py
- filter_low_expr_transcripts.pl
- get_Trinity_gene_to_trans_map.pl
- insilico_read_normalization.pl
- interpolate_sam.pl
- jellyfish
- novo2sam.pl
- retrieve_sequences_from_fasta.pl
- run_DE_analysis.pl
- sam2vcf.pl
- samtools
- samtools.pl
- seq_cache_populate.pl
- seqtk-trinity
- sift_bam_max_cov.pl
- soap2sam.pl
- tabix
- trimmomatic
- wgsim
- wgsim_eval.pl
- zoom2sam.pl
Link to section 'Module' of 'trinity' Module
You can load the modules by:
module load biocontainers
module load trinity
Link to section 'Example job' of 'trinity' Example job
Using #!/bin/sh -l
as shebang in the slurm job script will cause the failure of some biocontainer modules. Please use #!/bin/bash
instead.
To run Trinity on our clusters:
#!/bin/bash
#SBATCH -A myallocation # Allocation name
#SBATCH -t 1:00:00
#SBATCH -N 1
#SBATCH -n 6
#SBATCH --job-name=trinity
#SBATCH --mail-type=FAIL,BEGIN,END
#SBATCH --error=%x-%J-%u.err
#SBATCH --output=%x-%J-%u.out
module --force purge
ml biocontainers trinity
Trinity --seqType fq --left reads_1.fq --right reads_2.fq \
--CPU 6 --max_memory 20G
trinotate
Link to section 'Introduction' of 'trinotate' Introduction
Trinotate
is a comprehensive annotation suite designed for automatic functional annotation of transcriptomes, particularly de novo assembled transcriptomes, from model or non-model organisms.
For more information, please check its website: https://biocontainers.pro/tools/trinotate.
Link to section 'Versions' of 'trinotate' Versions
- 3.2.2
Link to section 'Commands' of 'trinotate' Commands
- Trinotate
- Build_Trinotate_Boilerplate_SQLite_db.pl
- EMBL_dat_to_Trinotate_sqlite_resourceDB.pl
- EMBL_swissprot_parser.pl
- PFAM_dat_parser.pl
- PFAMtoGoParser.pl
- RnammerTranscriptome.pl
- TrinotateSeqLoader.pl
- Trinotate_BLAST_loader.pl
- Trinotate_GO_to_SLIM.pl
- Trinotate_GTF_loader.pl
- Trinotate_GTF_or_GFF3_annot_prep.pl
- Trinotate_PFAM_loader.pl
- Trinotate_RNAMMER_loader.pl
- Trinotate_SIGNALP_loader.pl
- Trinotate_TMHMM_loader.pl
- Trinotate_get_feature_name_encoding_attributes.pl
- Trinotate_report_writer.pl
- assign_eggnog_funccats.pl
- autoTrinotate.pl
- build_DE_cache_tables.pl
- cleanMe.pl
- cleanme.pl
- count_table_fields.pl
- create_clusters_tables.pl
- extract_GO_assignments_from_Trinotate_xls.pl
- extract_GO_for_BiNGO.pl
- extract_specific_genes_from_all_matrices.pl
- import_DE_results.pl
- import_Trinotate_xls_as_annot.pl
- import_expression_and_DE_results.pl
- import_expression_matrix.pl
- import_samples_n_expression_matrix.pl
- import_samples_only.pl
- import_transcript_annotations.pl
- import_transcript_clusters.pl
- import_transcript_names.pl
- init_Trinotate_sqlite_db.pl
- legacy_blast.pl
- make_cXp_html.pl
- obo_tab_to_sqlite_db.pl
- obo_to_tab.pl
- prep_nuc_prot_set_for_trinotate_loading.pl
- print.pl
- rnammer_supperscaffold_gff_to_indiv_transcripts.pl
- runMe.pl
- run_TrinotateWebserver.pl
- run_cluster_functional_enrichment_analysis.pl
- shrink_db.pl
- sqlite.pl
- superScaffoldGenerator.pl
- test_Barplot.pl
- test_GO_DAG.pl
- test_GenomeBrowser.pl
- test_Heatmap.pl
- test_Lineplot.pl
- test_Piechart.pl
- test_Scatter2D.pl
- test_Sunburst.pl
- trinotate_report_summary.pl
- update_blastdb.pl
- update_seq_n_annotation_fields.pl
Link to section 'Module' of 'trinotate' Module
You can load the modules by:
module load biocontainers
module load trinotate
Link to section 'Example job' of 'trinotate' Example job
Using #!/bin/sh -l
as shebang in the slurm job script will cause the failure of some biocontainer modules. Please use #!/bin/bash
instead.
To run Trinotate on our clusters:
#!/bin/bash
#SBATCH -A myallocation # Allocation name
#SBATCH -t 1:00:00
#SBATCH -N 1
#SBATCH -n 1
#SBATCH --job-name=trinotate
#SBATCH --mail-type=FAIL,BEGIN,END
#SBATCH --error=%x-%J-%u.err
#SBATCH --output=%x-%J-%u.out
module --force purge
ml biocontainers trinotate
sqlite_db="myTrinotate.sqlite"
Trinotate ${sqlite_db} init \
--gene_trans_map data/Trinity.fasta.gene_to_trans_map \
--transcript_fasta data/Trinity.fasta \
--transdecoder_pep \
data/Trinity.fasta.transdecoder.pep
Trinotate ${sqlite_db} LOAD_swissprot_blastp data/swissprot.blastp.outfmt6
Trinotate ${sqlite_db} LOAD_pfam data/TrinotatePFAM.out
trnascan-se
Link to section 'Introduction' of 'trnascan-se' Introduction
Trnascan-se
is a convenient, ready-for-use means to identify tRNA genes in one or more query sequences.
For more information, please check its website: https://biocontainers.pro/tools/trnascan-se and its home page: http://lowelab.ucsc.edu/tRNAscan-SE/.
Link to section 'Versions' of 'trnascan-se' Versions
- 2.0.9
Link to section 'Commands' of 'trnascan-se' Commands
- tRNAscan-SE
Link to section 'Module' of 'trnascan-se' Module
You can load the modules by:
module load biocontainers
module load trnascan-se
Link to section 'Example job' of 'trnascan-se' Example job
Using #!/bin/sh -l
as shebang in the slurm job script will cause the failure of some biocontainer modules. Please use #!/bin/bash
instead.
To run Trnascan-se on our clusters:
#!/bin/bash
#SBATCH -A myallocation # Allocation name
#SBATCH -t 1:00:00
#SBATCH -N 1
#SBATCH -n 12
#SBATCH --job-name=trnascan-se
#SBATCH --mail-type=FAIL,BEGIN,END
#SBATCH --error=%x-%J-%u.err
#SBATCH --output=%x-%J-%u.out
module --force purge
ml biocontainers trnascan-se
tRNAscan-SE --thread 12 -o tRNA.out \
-f rRNA.ss -m tRNA.stats genome.fasta
trtools
Link to section 'Introduction' of 'trtools' Introduction
TRTools includes a variety of utilities for filtering, quality control and analysis of tandem repeats downstream of genotyping them from next-generation sequencing.
BioContainers: https://biocontainers.pro/tools/trtools
Home page: https://github.com/gymreklab/TRTools
Link to section 'Versions' of 'trtools' Versions
- 5.0.1
Link to section 'Commands' of 'trtools' Commands
- associaTR
- compareSTR
- dumpSTR
- mergeSTR
- qcSTR
- statSTR
Link to section 'Module' of 'trtools' Module
You can load the modules by:
module load biocontainers
module load trtools
Link to section 'Example job' of 'trtools' Example job
Using #!/bin/sh -l
as shebang in the slurm job script will cause the failure of some biocontainer modules. Please use #!/bin/bash
instead.
We noticed that xalt
module can cause the failure of certain commands including statSTR
. Please unload all loaded modules by module --force purge
before loading required modules.
To run trtools on our clusters:
#!/bin/bash
#SBATCH -A myallocation # Allocation name
#SBATCH -t 1:00:00
#SBATCH -N 1
#SBATCH -n 1
#SBATCH --job-name=trtools
#SBATCH --mail-type=FAIL,BEGIN,END
#SBATCH --error=%x-%J-%u.err
#SBATCH --output=%x-%J-%u.out
module --force purge
ml biocontainers trtools htslib bcftools
mergeSTR --vcfs ceu_ex.vcf.gz,yri_ex.vcf.gz --out merged
bgzip merged.vcf
tabix -p vcf merged.vcf.gz
# Get the CEU and YRI sample lists
bcftools query -l yri_ex.vcf.gz > yri_samples.txt
bcftools query -l ceu_ex.vcf.gz > ceu_samples.txt
# Run statSTR on region chr21:35348646-35348646 (hg38)
statSTR \
--vcf merged.vcf.gz \
--samples yri_samples.txt,ceu_samples.txt \
--sample-prefixes YRI,CEU \
--out stdout \
--mean --het --acount \
--use-length \
--region chr21:34351482-34363028
trust4
Link to section 'Introduction' of 'trust4' Introduction
Tcr Receptor Utilities for Solid Tissue (TRUST) is a computational tool to analyze TCR and BCR sequences using unselected RNA sequencing data, profiled from solid tissues, including tumors.
BioContainers: https://biocontainers.pro/tools/trust4
Home page: https://github.com/liulab-dfci/TRUST4
Link to section 'Versions' of 'trust4' Versions
- 1.0.7
Link to section 'Commands' of 'trust4' Commands
- run-trust4
- BuildDatabaseFa.pl
- BuildImgtAnnot.pl
- trust-airr.pl
- trust-barcoderep.pl
- trust-simplerep.pl
- trust-smartseq.pl
Link to section 'Module' of 'trust4' Module
You can load the modules by:
module load biocontainers
module load trust4
Link to section 'Example job' of 'trust4' Example job
Using #!/bin/sh -l
as shebang in the slurm job script will cause the failure of some biocontainer modules. Please use #!/bin/bash
instead.
To run trust4 on our clusters:
#!/bin/bash
#SBATCH -A myallocation # Allocation name
#SBATCH -t 1:00:00
#SBATCH -N 1
#SBATCH -n 1
#SBATCH --job-name=trust4
#SBATCH --mail-type=FAIL,BEGIN,END
#SBATCH --error=%x-%J-%u.err
#SBATCH --output=%x-%J-%u.out
module --force purge
ml biocontainers trust4
run-trust4 -b mapped.bam -f hg38_bcrtcr.fa --ref human_IMGT+C.fa
trycycler
Link to section 'Introduction' of 'trycycler' Introduction
Trycycler is a tool for generating consensus long-read assemblies for bacterial genomes. I.e. if you have multiple long-read assemblies for the same isolate, Trycycler can combine them into a single assembly that is better than any of your inputs.
Docker hub: https://hub.docker.com/r/staphb/trycycler
Home page: https://github.com/rrwick/Trycycler
Link to section 'Versions' of 'trycycler' Versions
- 0.5.0
- 0.5.3
Link to section 'Commands' of 'trycycler' Commands
- trycycler
Link to section 'Module' of 'trycycler' Module
You can load the modules by:
module load biocontainers
module load trycycler
Link to section 'Example job' of 'trycycler' Example job
Using #!/bin/sh -l
as shebang in the slurm job script will cause the failure of some biocontainer modules. Please use #!/bin/bash
instead.
To run trycycler on our clusters:
#!/bin/bash
#SBATCH -A myallocation # Allocation name
#SBATCH -t 1:00:00
#SBATCH -N 1
#SBATCH -n 1
#SBATCH --job-name=trycycler
#SBATCH --mail-type=FAIL,BEGIN,END
#SBATCH --error=%x-%J-%u.err
#SBATCH --output=%x-%J-%u.out
module --force purge
ml biocontainers trycycler
trycycler cluster --assemblies \
test/test_cluster/assembly_*.fasta \
--read test/test_cluster/reads.fastq \
--out_dir trycycler_out
ucsc_genome_toolkit
UCSC Executables
is a variety of executables that perform functions ranging from sequence analysis and format conversion, to basic number crunching and statistics, to complex database generation and manipulation.
These executables have been downloaded from http://hgdownload.soe.ucsc.edu/admin/exe/linux.x86_64.v369/ and made available on RCAC clusters.
Link to section 'Versions' of 'ucsc_genome_toolkit' Versions
- 369
Link to section 'Commands' of 'ucsc_genome_toolkit' Commands
- addCols
- ameme
- autoDtd
- autoSql
- autoXml
- ave
- aveCols
- axtChain
- axtSort
- axtSwap
- axtToMaf
- axtToPsl
- bamToPsl
- barChartMaxLimit
- bedClip
- bedCommonRegions
- bedCoverage
- bedExtendRanges
- bedGeneParts
- bedGraphPack
- bedGraphToBigWig
- bedIntersect
- bedItemOverlapCount
- bedJoinTabOffset
- bedJoinTabOffset.py
- bedMergeAdjacent
- bedPartition
- bedPileUps
- bedRemoveOverlap
- bedRestrictToPositions
- bedSingleCover.pl
- bedSort
- bedToBigBed
- bedToExons
- bedToGenePred
- bedToPsl
- bedWeedOverlapping
- bigBedInfo
- bigBedNamedItems
- bigBedSummary
- bigBedToBed
- bigGenePredToGenePred
- bigHeat
- bigMafToMaf
- bigPslToPsl
- bigWigAverageOverBed
- bigWigCat
- bigWigCluster
- bigWigCorrelate
- bigWigInfo
- bigWigMerge
- bigWigSummary
- bigWigToBedGraph
- bigWigToWig
- binFromRange
- blastToPsl
- blastXmlToPsl
- blat
- calc
- catDir
- catUncomment
- chainAntiRepeat
- chainBridge
- chainCleaner
- chainFilter
- chainMergeSort
- chainNet
- chainPreNet
- chainScore
- chainSort
- chainSplit
- chainStitchId
- chainSwap
- chainToAxt
- chainToPsl
- chainToPslBasic
- checkAgpAndFa
- checkCoverageGaps
- checkHgFindSpec
- checkTableCoords
- chopFaLines
- chromGraphFromBin
- chromGraphToBin
- chromToUcsc
- clusterGenes
- clusterMatrixToBarChartBed
- colTransform
- countChars
- cpg_lh
- crTreeIndexBed
- crTreeSearchBed
- dbSnoop
- dbTrash
- endsInLf
- estOrient
- expMatrixToBarchartBed
- faAlign
- faCmp
- faCount
- faFilter
- faFilterN
- faFrag
- faNoise
- faOneRecord
- faPolyASizes
- faRandomize
- faRc
- faSize
- faSomeRecords
- faSplit
- faToFastq
- faToTab
- faToTwoBit
- faToVcf
- faTrans
- fastqStatsAndSubsample
- fastqToFa
- featureBits
- fetchChromSizes
- findMotif
- fixStepToBedGraph.pl
- gapToLift
- genePredCheck
- genePredFilter
- genePredHisto
- genePredSingleCover
- genePredToBed
- genePredToBigGenePred
- genePredToFakePsl
- genePredToGtf
- genePredToMafFrames
- genePredToProt
- gensub2
- getRna
- getRnaPred
- gff3ToGenePred
- gff3ToPsl
- gmtime
- gtfToGenePred
- headRest
- hgBbiDbLink
- hgFakeAgp
- hgFindSpec
- hgGcPercent
- hgGoldGapGl
- hgLoadBed
- hgLoadChain
- hgLoadGap
- hgLoadMaf
- hgLoadMafSummary
- hgLoadNet
- hgLoadOut
- hgLoadOutJoined
- hgLoadSqlTab
- hgLoadWiggle
- hgSpeciesRna
- hgTrackDb
- hgWiggle
- hgsql
- hgsqldump
- hgvsToVcf
- hicInfo
- htmlCheck
- hubCheck
- hubClone
- hubPublicCheck
- ixIxx
- lastz-1.04.00
- lastz_D-1.04.00
- lavToAxt
- lavToPsl
- ldHgGene
- liftOver
- liftOverMerge
- liftUp
- linesToRa
- localtime
- mafAddIRows
- mafAddQRows
- mafCoverage
- mafFetch
- mafFilter
- mafFrag
- mafFrags
- mafGene
- mafMeFirst
- mafNoAlign
- mafOrder
- mafRanges
- mafSpeciesList
- mafSpeciesSubset
- mafSplit
- mafSplitPos
- mafToAxt
- mafToBigMaf
- mafToPsl
- mafToSnpBed
- mafsInRegion
- makeTableList
- maskOutFa
- matrixClusterColumns
- matrixMarketToTsv
- matrixNormalize
- mktime
- mrnaToGene
- netChainSubset
- netClass
- netFilter
- netSplit
- netSyntenic
- netToAxt
- netToBed
- newProg
- newPythonProg
- nibFrag
- nibSize
- oligoMatch
- overlapSelect
- para
- paraFetch
- paraHub
- paraHubStop
- paraNode
- paraNodeStart
- paraNodeStatus
- paraNodeStop
- paraSync
- paraTestJob
- parasol
- positionalTblCheck
- pslCDnaFilter
- pslCat
- pslCheck
- pslDropOverlap
- pslFilter
- pslHisto
- pslLiftSubrangeBlat
- pslMap
- pslMapPostChain
- pslMrnaCover
- pslPairs
- pslPartition
- pslPosTarget
- pslPretty
- pslRc
- pslRecalcMatch
- pslRemoveFrameShifts
- pslReps
- pslScore
- pslSelect
- pslSomeRecords
- pslSort
- pslSortAcc
- pslStats
- pslSwap
- pslToBed
- pslToBigPsl
- pslToChain
- pslToPslx
- pslxToFa
- qaToQac
- qacAgpLift
- qacToQa
- qacToWig
- raSqlQuery
- raToLines
- raToTab
- randomLines
- rmFaDups
- rowsToCols
- sizeof
- spacedToTab
- splitFile
- splitFileByColumn
- sqlToXml
- strexCalc
- stringify
- subChar
- subColumn
- tabQuery
- tailLines
- tdbQuery
- tdbRename
- tdbSort
- textHistogram
- tickToDate
- toLower
- toUpper
- trackDbIndexBb
- transMapPslToGenePred
- trfBig
- twoBitDup
- twoBitInfo
- twoBitMask
- twoBitToFa
- ucscApiClient
- udr
- vai.pl
- validateFiles
- validateManifest
- varStepToBedGraph.pl
- webSync
- wigCorrelate
- wigEncode
- wigToBigWig
- wordLine
- xmlCat
- xmlToSql
Link to section 'Module' of 'ucsc_genome_toolkit' Module
You can load the modules by:
module load biocontainers
module load ucsc_genome_toolkit/369
Link to section 'Example job' of 'ucsc_genome_toolkit' Example job
Using #!/bin/sh -l
as shebang in the slurm job script will cause the failure of some biocontainer modules. Please use #!/bin/bash
instead.
To run UCSC executables on our our clusters:
#!/bin/bash
#SBATCH -A myallocation # Allocation name
#SBATCH -t 1:00:00
#SBATCH -N 1
#SBATCH -n 12
#SBATCH --job-name=UCSC
#SBATCH --mail-type=FAIL,BEGIN,END
#SBATCH --error=%x-%J-%u.err
#SBATCH --output=%x-%J-%u.out
module --force purge
ml biocontainers ucsc_genome_toolkit/369
blat genome.fasta input.fasta blat.out
fastqToFa input.fastq output.fasta
unicycler
Link to section 'Introduction' of 'unicycler' Introduction
Unicycler
is an assembly pipeline for bacterial genomes.
For more information, please check its website: https://biocontainers.pro/tools/unicycler and its home page on Github.
Link to section 'Versions' of 'unicycler' Versions
- 0.5.0
Link to section 'Commands' of 'unicycler' Commands
- unicycler
Link to section 'Module' of 'unicycler' Module
You can load the modules by:
module load biocontainers
module load unicycler
Link to section 'Example job' of 'unicycler' Example job
Using #!/bin/sh -l
as shebang in the slurm job script will cause the failure of some biocontainer modules. Please use #!/bin/bash
instead.
To run Unicycler on our clusters:
#!/bin/bash
#SBATCH -A myallocation # Allocation name
#SBATCH -t 1:00:00
#SBATCH -N 1
#SBATCH -n 12
#SBATCH --job-name=unicycler
#SBATCH --mail-type=FAIL,BEGIN,END
#SBATCH --error=%x-%J-%u.err
#SBATCH --output=%x-%J-%u.out
module --force purge
ml biocontainers unicycler
unicycler -t 12 -1 SRR11234553_1.fastq -2 SRR11234553_2.fastq -o shortout
unicycler -t 12 -l SRR3982487.fastq -o longout
vadr
Link to section 'Introduction' of 'vadr' Introduction
VADR is a suite of tools for classifying and analyzing sequences homologous to a set of reference models of viral genomes or gene families. It has been mainly tested for analysis of Norovirus, Dengue, and SARS-CoV-2 virus sequences in preparation for submission to the GenBank database.
Docker hub: https://hub.docker.com/r/staphb/vadr
Home page: https://github.com/ncbi/vadr
Link to section 'Versions' of 'vadr' Versions
- 1.4.1
- 1.4.2
- 1.5
Link to section 'Commands' of 'vadr' Commands
- parse_blast.pl
- v-annotate.pl
- v-build.pl
- v-test.pl
Link to section 'Module' of 'vadr' Module
You can load the modules by:
module load biocontainers
module load vadr
Link to section 'Example job' of 'vadr' Example job
Using #!/bin/sh -l
as shebang in the slurm job script will cause the failure of some biocontainer modules. Please use #!/bin/bash
instead.
To run vadr on our clusters:
#!/bin/bash
#SBATCH -A myallocation # Allocation name
#SBATCH -t 1:00:00
#SBATCH -N 1
#SBATCH -n 1
#SBATCH --job-name=vadr
#SBATCH --mail-type=FAIL,BEGIN,END
#SBATCH --error=%x-%J-%u.err
#SBATCH --output=%x-%J-%u.out
module --force purge
ml biocontainers vadr
v-annotate.pl noro.9.fa va-noro.9
usefulaf
Link to section 'Introduction' of 'usefulaf' Introduction
Usefulaf is an all-in-one Docker/Singularity image for single-cell processing with Alevin-fry(paper). It includes the all tools you need to turn your FASTQ files into a count matrix and then load it into your favorite analysis environment.
Docker hub: https://hub.docker.com/r/combinelab/usefulaf
Home page: https://github.com/COMBINE-lab/usefulaf
Link to section 'Versions' of 'usefulaf' Versions
- 0.9.2
Link to section 'Commands' of 'usefulaf' Commands
- simpleaf
- R
- Rscript
- python
- python3
Link to section 'Module' of 'usefulaf' Module
You can load the modules by:
module load biocontainers
module load usefulaf
Link to section 'Example job' of 'usefulaf' Example job
Using #!/bin/sh -l
as shebang in the slurm job script will cause the failure of some biocontainer modules. Please use #!/bin/bash
instead.
To run usefulaf on our clusters:
#!/bin/bash
#SBATCH -A myallocation # Allocation name
#SBATCH -t 1:00:00
#SBATCH -N 1
#SBATCH -n 1
#SBATCH --job-name=usefulaf
#SBATCH --mail-type=FAIL,BEGIN,END
#SBATCH --error=%x-%J-%u.err
#SBATCH --output=%x-%J-%u.out
module --force purge
ml biocontainers usefulaf
vardict-java
Link to section 'Introduction' of 'vardict-java' Introduction
VarDictJava is a variant discovery program written in Java and Perl. It is a Java port of VarDict variant caller.
Docker hub: https://hub.docker.com/r/hydragenetics/vardict
Home page: https://github.com/AstraZeneca-NGS/VarDictJava
Link to section 'Versions' of 'vardict-java' Versions
- 1.8.3
Link to section 'Commands' of 'vardict-java' Commands
- vardict-java
- var2vcf_paired.pl
- var2vcf_valid.pl
- testsomatic.R
- teststrandbias.R
Link to section 'Module' of 'vardict-java' Module
You can load the modules by:
module load biocontainers
module load vardict-java
Link to section 'Example job' of 'vardict-java' Example job
Using #!/bin/sh -l
as shebang in the slurm job script will cause the failure of some biocontainer modules. Please use #!/bin/bash
instead.
To run vardict-java on our clusters:
#!/bin/bash
#SBATCH -A myallocation # Allocation name
#SBATCH -t 1:00:00
#SBATCH -N 1
#SBATCH -n 1
#SBATCH --job-name=vardict-java
#SBATCH --mail-type=FAIL,BEGIN,END
#SBATCH --error=%x-%J-%u.err
#SBATCH --output=%x-%J-%u.out
module --force purge
ml biocontainers vardict-java
AF_THR="0.01" # minimum allele frequency
vardict-java -G genome.fasta \
-f $AF_THR -N genome \
-b input.bam \
-c 1 -S 2 -E 3 -g 4 output.bed \
| teststrandbias.R \
| var2vcf_valid.pl \
-N genome -E -f $AF_THR \
> vars.vcf
varlociraptor
Link to section 'Introduction' of 'varlociraptor' Introduction
Varlociraptor
implements a novel, unified fully uncertainty-aware approach to genomic variant calling in arbitrary scenarios.
For more information, please check its website: https://biocontainers.pro/tools/varlociraptor and its home page on Github.
Link to section 'Versions' of 'varlociraptor' Versions
- 4.11.4
Link to section 'Commands' of 'varlociraptor' Commands
- varlociraptor
Link to section 'Module' of 'varlociraptor' Module
You can load the modules by:
module load biocontainers
module load varlociraptor
Link to section 'Example job' of 'varlociraptor' Example job
Using #!/bin/sh -l
as shebang in the slurm job script will cause the failure of some biocontainer modules. Please use #!/bin/bash
instead.
To run Varlociraptor on our clusters:
#!/bin/bash
#SBATCH -A myallocation # Allocation name
#SBATCH -t 1:00:00
#SBATCH -N 1
#SBATCH -n 1
#SBATCH --job-name=varlociraptor
#SBATCH --mail-type=FAIL,BEGIN,END
#SBATCH --error=%x-%J-%u.err
#SBATCH --output=%x-%J-%u.out
module --force purge
ml biocontainers varlociraptor
varlociraptor call variants tumor-normal --purity 0.75 --tumor
varscan
Link to section 'Introduction' of 'varscan' Introduction
Varscan
is a tool used for variant detection in massively parallel sequencing data.
For more information, please check its home page: http://varscan.sourceforge.net/index.html.
Link to section 'Versions' of 'varscan' Versions
- 2.4.2
- 2.4.4
Link to section 'Commands' of 'varscan' Commands
- VarScan.v2.4.4.jar
Link to section 'Module' of 'varscan' Module
You can load the modules by:
module load biocontainers
module load varscan
Link to section 'Example job' of 'varscan' Example job
Using #!/bin/sh -l
as shebang in the slurm job script will cause the failure of some biocontainer modules. Please use #!/bin/bash
instead.
To run Varscan on our clusters:
#!/bin/bash
#SBATCH -A myallocation # Allocation name
#SBATCH -t 1:00:00
#SBATCH -N 1
#SBATCH -n 1
#SBATCH --job-name=varscan
#SBATCH --mail-type=FAIL,BEGIN,END
#SBATCH --error=%x-%J-%u.err
#SBATCH --output=%x-%J-%u.out
module --force purge
ml biocontainers varscan
vartrix
Link to section 'Introduction' of 'vartrix' Introduction
Vartrix
is a software tool for extracting single cell variant information from 10x Genomics single cell data.
For more information, please check its website: https://biocontainers.pro/tools/vartrix and its home page on Github.
Link to section 'Versions' of 'vartrix' Versions
- 1.1.22
Link to section 'Commands' of 'vartrix' Commands
- vartrix
Link to section 'Module' of 'vartrix' Module
You can load the modules by:
module load biocontainers
module load vartrix
Link to section 'Example job' of 'vartrix' Example job
Using #!/bin/sh -l
as shebang in the slurm job script will cause the failure of some biocontainer modules. Please use #!/bin/bash
instead.
To run Vartrix on our clusters:
#!/bin/bash
#SBATCH -A myallocation # Allocation name
#SBATCH -t 1:00:00
#SBATCH -N 1
#SBATCH -n 1
#SBATCH --job-name=vartrix
#SBATCH --mail-type=FAIL,BEGIN,END
#SBATCH --error=%x-%J-%u.err
#SBATCH --output=%x-%J-%u.out
module --force purge
ml biocontainers vartrix
vartrix -v test/test.vcf -b test/test.bam \
-f test/test.fa -c test/barcodes.tsv \
-o output.matrix
vatools
Link to section 'Introduction' of 'vatools' Introduction
VAtools is a python package that includes several tools to annotate VCF files with data from other tools.
Docker hub: https://hub.docker.com/r/griffithlab/vatools
Home page: https://vatools.readthedocs.io/en/latest/
Link to section 'Versions' of 'vatools' Versions
- 5.0.1
Link to section 'Commands' of 'vatools' Commands
- ref-transcript-mismatch-reporter
- transform-split-values
- vcf-expression-annotator
- vcf-genotype-annotator
- vcf-info-annotator
- vcf-readcount-annotator
- vep-annotation-reporter
Link to section 'Module' of 'vatools' Module
You can load the modules by:
module load biocontainers
module load vatools
Link to section 'Example job' of 'vatools' Example job
Using #!/bin/sh -l
as shebang in the slurm job script will cause the failure of some biocontainer modules. Please use #!/bin/bash
instead.
To run vatools on our clusters:
#!/bin/bash
#SBATCH -A myallocation # Allocation name
#SBATCH -t 1:00:00
#SBATCH -N 1
#SBATCH -n 1
#SBATCH --job-name=vatools
#SBATCH --mail-type=FAIL,BEGIN,END
#SBATCH --error=%x-%J-%u.err
#SBATCH --output=%x-%J-%u.out
module --force purge
ml biocontainers vatools
vcf-readcount-annotator <input_vcf> <snv_bam_readcount_file> <DNA| RNA> \
-s <sample_name> -t snv -o <snv_annotated_vcf>
vcf-kit
Link to section 'Introduction' of 'vcf-kit' Introduction
VCF-kit is a command-line based collection of utilities for performing analysis on Variant Call Format (VCF) files.
BioContainers: https://biocontainers.pro/tools/vcf-kit
Home page: https://github.com/AndersenLab/VCF-kit
Link to section 'Versions' of 'vcf-kit' Versions
- 0.2.6
- 0.2.9
Link to section 'Commands' of 'vcf-kit' Commands
- vk
Link to section 'Module' of 'vcf-kit' Module
You can load the modules by:
module load biocontainers
module load vcf-kit
Link to section 'Example job' of 'vcf-kit' Example job
Using #!/bin/sh -l
as shebang in the slurm job script will cause the failure of some biocontainer modules. Please use #!/bin/bash
instead.
To run vcf-kit on our clusters:
#!/bin/bash
#SBATCH -A myallocation # Allocation name
#SBATCH -t 1:00:00
#SBATCH -N 1
#SBATCH -n 1
#SBATCH --job-name=vcf-kit
#SBATCH --mail-type=FAIL,BEGIN,END
#SBATCH --error=%x-%J-%u.err
#SBATCH --output=%x-%J-%u.out
module --force purge
ml biocontainers vcf-kit
vcf2maf
Link to section 'Introduction' of 'vcf2maf' Introduction
To convert a VCF into a MAF, each variant must be mapped to only one of all possible gene transcripts/isoforms that it might affect. This selection of a single effect per variant, is often subjective. So this project is an attempt to make the selection criteria smarter, reproducible, and more configurable. And the default criteria must lean towards best practices.
Home page: https://github.com/mskcc/vcf2maf
Link to section 'Versions' of 'vcf2maf' Versions
- 1.6.21
Link to section 'Commands' of 'vcf2maf' Commands
- maf2maf.pl
- maf2vcf.pl
- vcf2maf.pl
- vcf2vcf.pl
Link to section 'Module' of 'vcf2maf' Module
You can load the modules by:
module load biocontainers
module load vcf2maf
Link to section 'Example job' of 'vcf2maf' Example job
Using #!/bin/sh -l
as shebang in the slurm job script will cause the failure of some biocontainer modules. Please use #!/bin/bash
instead.
If users need to use vep
, please add --vep-path /opt/conda/bin
.
To run vcf2maf on our clusters:
#!/bin/bash
#SBATCH -A myallocation # Allocation name
#SBATCH -t 1:00:00
#SBATCH -N 1
#SBATCH -n 1
#SBATCH --job-name=vcf2maf
#SBATCH --mail-type=FAIL,BEGIN,END
#SBATCH --error=%x-%J-%u.err
#SBATCH --output=%x-%J-%u.out
module --force purge
ml biocontainers vcf2maf
vcf2maf.pl --vep-path /opt/conda/bin \
--ref-fasta Homo_sapiens.GRCh37.dna.toplevel.fa.gz \
--input-vcf tests/test.vcf --output-maf test.vep.maf
vcf2phylip
Link to section 'Introduction' of 'vcf2phylip' Introduction
vcf2phylip is a tool to convert SNPs in VCF format to PHYLIP, NEXUS, binary NEXUS, or FASTA alignments for phylogenetic analysis.
Home page: https://github.com/edgardomortiz/vcf2phylip
Link to section 'Versions' of 'vcf2phylip' Versions
- 2.8
Link to section 'Commands' of 'vcf2phylip' Commands
- vcf2phylip.py
Link to section 'Module' of 'vcf2phylip' Module
You can load the modules by:
module load biocontainers
module load vcf2phylip
Link to section 'Example job' of 'vcf2phylip' Example job
Using #!/bin/sh -l
as shebang in the slurm job script will cause the failure of some biocontainer modules. Please use #!/bin/bash
instead.
To run vcf2phylip on our clusters:
#!/bin/bash
#SBATCH -A myallocation # Allocation name
#SBATCH -t 1:00:00
#SBATCH -N 1
#SBATCH -n 1
#SBATCH --job-name=vcf2phylip
#SBATCH --mail-type=FAIL,BEGIN,END
#SBATCH --error=%x-%J-%u.err
#SBATCH --output=%x-%J-%u.out
module --force purge
ml biocontainers vcf2phylip
vcf2phylip --input myfile.vcf
vcf2tsvpy
Link to section 'Introduction' of 'vcf2tsvpy' Introduction
Vcf2tsvpy is a small Python program that converts genomic variant data encoded in VCF format into a tab-separated values (TSV) file.
BioContainers: https://biocontainers.pro/tools/vcf2tsvpy
Home page: https://github.com/sigven/vcf2tsvpy
Link to section 'Versions' of 'vcf2tsvpy' Versions
- 0.6.0
Link to section 'Commands' of 'vcf2tsvpy' Commands
- vcf2tsvpy
Link to section 'Module' of 'vcf2tsvpy' Module
You can load the modules by:
module load biocontainers
module load vcf2tsvpy
Link to section 'Example job' of 'vcf2tsvpy' Example job
Using #!/bin/sh -l
as shebang in the slurm job script will cause the failure of some biocontainer modules. Please use #!/bin/bash
instead.
To run vcf2tsvpy on our clusters:
#!/bin/bash
#SBATCH -A myallocation # Allocation name
#SBATCH -t 1:00:00
#SBATCH -N 1
#SBATCH -n 1
#SBATCH --job-name=vcf2tsvpy
#SBATCH --mail-type=FAIL,BEGIN,END
#SBATCH --error=%x-%J-%u.err
#SBATCH --output=%x-%J-%u.out
module --force purge
ml biocontainers vcf2tsvpy
vcftools
Link to section 'Introduction' of 'vcftools' Introduction
VCFtools
is a program package designed for working with VCF files, such as those generated by the 1000 Genomes Project. The aim of VCFtools is to provide easily accessible methods for working with complex genetic variation data in the form of VCF files.
For more information, please check its website: https://biocontainers.pro/tools/vcftools and its home page on Github.
Link to section 'Versions' of 'vcftools' Versions
- 0.1.16
Link to section 'Commands' of 'vcftools' Commands
- vcftools
Link to section 'Module' of 'vcftools' Module
You can load the modules by:
module load biocontainers
module load vartrix
Link to section 'Example job' of 'vcftools' Example job
Using #!/bin/sh -l
as shebang in the slurm job script will cause the failure of some biocontainer modules. Please use #!/bin/bash
instead.
To run VCFtools on our clusters:
#!/bin/bash
#SBATCH -A myallocation # Allocation name
#SBATCH -t 1:00:00
#SBATCH -N 1
#SBATCH -n 1
#SBATCH --job-name=vcftools
#SBATCH --mail-type=FAIL,BEGIN,END
#SBATCH --error=%x-%J-%u.err
#SBATCH --output=%x-%J-%u.out
module --force purge
ml biocontainers vcftools
vcftools --vcf input_data.vcf --chr 1 \
--from-bp 1000000 --to-bp 2000000
velocyto.py
Velocyto.py
a library for the analysis of RNA velocity.
Detailed information about velocyto.py can be found here: https://github.com/velocyto-team/velocyto.py.
Link to section 'Versions' of 'velocyto.py' Versions
- 0.17.17
Link to section 'Commands' of 'velocyto.py' Commands
- python
- python3
- velocyto
Link to section 'Module' of 'velocyto.py' Module
You can load the modules by:
module load biocontainers
module load velocyto.py/0.17.17-py39
Link to section 'Interactive job' of 'velocyto.py' Interactive job
To run Velocyto.py
interactively on our clusters:
(base) UserID@bell-fe00:~ $ sinteractive -N1 -n12 -t4:00:00 -A myallocation
salloc: Granted job allocation 12345869
salloc: Waiting for resource configuration
salloc: Nodes bell-a008 are ready for job
(base) UserID@bell-a008:~ $ module load biocontainers cellrank/1.5.1
(base) UserID@bell-a008:~ $ python
Python 3.9.10 | packaged by conda-forge | (main, Feb 1 2022, 21:24:11)
[GCC 9.4.0] :: Anaconda, Inc. on linux
Type "help", "copyright", "credits" or "license" for more information.
>>> import velocyto as vcy
>>> vlm = vcy.VelocytoLoom("YourData.loom")
>>> vlm.normalize("S", size=True, log=True)
>>> vlm.S_norm # contains log normalized
Link to section 'Batch job' of 'velocyto.py' Batch job
Using #!/bin/sh -l
as shebang in the slurm job script will cause the failure of some biocontainer modules. Please use #!/bin/bash
instead.
To submit a sbatch job on our clusters:
#!/bin/bash
#SBATCH -A myallocation # Allocation name
#SBATCH -t 10:00:00
#SBATCH -N 1
#SBATCH -n 24
#SBATCH --job-name=Velocyto
#SBATCH --mail-type=FAIL,BEGIN,END
#SBATCH --error=%x-%J-%u.err
#SBATCH --output=%x-%J-%u.out
module --force purge
ml biocontainers velocyto.py/0.17.17-py39
velocyto run10x cellranger_count_1kpbmcs_out refdata-gex-GRCh38-2020-A/genes/genes.gtf
velvet
Link to section 'Introduction' of 'velvet' Introduction
Velvet
is a sequence assembler for very short reads.
For more information, please check its website: https://biocontainers.pro/tools/velvet.
Link to section 'Versions' of 'velvet' Versions
- 1.2.10
Link to section 'Commands' of 'velvet' Commands
- velveth
- velvetg
Link to section 'Module' of 'velvet' Module
You can load the modules by:
module load biocontainers
module load trimmomatic
Link to section 'Example job' of 'velvet' Example job
Using #!/bin/sh -l
as shebang in the slurm job script will cause the failure of some biocontainer modules. Please use #!/bin/bash
instead.
To run Velvet on our clusters:
#!/bin/bash
#SBATCH -A myallocation # Allocation name
#SBATCH -t 1:00:00
#SBATCH -N 1
#SBATCH -n 1
#SBATCH --job-name=velvet
#SBATCH --mail-type=FAIL,BEGIN,END
#SBATCH --error=%x-%J-%u.err
#SBATCH --output=%x-%J-%u.out
module --force purge
ml biocontainers velvet
velveth output_directory 21 -fasta -short solexa1.fa solexa2.fa solexa3.fa -long capillary.fa
velvetg output_directory -cov_cutoff 4
veryfasttree
Link to section 'Introduction' of 'veryfasttree' Introduction
VeryFastTree is a highly-tuned implementation of the FastTree-2 tool that takes advantage of parallelization and vectorization strategies to speed up the inference of phylogenies for huge alignments. It is important to highlight that VeryFastTree keeps unchanged the phases, methods and heuristics used by FastTree-2 to estimate the phylogenetic tree. In this way, it produces trees with the same topological accuracy than FastTree-2. In addition, unlike the parallel version of FastTree-2, VeryFastTree is deterministic.
BioContainers: https://biocontainers.pro/tools/veryfasttree
Home page: https://github.com/citiususc/veryfasttree
Link to section 'Versions' of 'veryfasttree' Versions
- 3.2.1
Link to section 'Commands' of 'veryfasttree' Commands
- VeryFastTree
Link to section 'Module' of 'veryfasttree' Module
You can load the modules by:
module load biocontainers
module load veryfasttree
Link to section 'Example job' of 'veryfasttree' Example job
Using #!/bin/sh -l
as shebang in the slurm job script will cause the failure of some biocontainer modules. Please use #!/bin/bash
instead.
To run veryfasttree on our clusters:
#!/bin/bash
#SBATCH -A myallocation # Allocation name
#SBATCH -t 1:00:00
#SBATCH -N 1
#SBATCH -n 1
#SBATCH --job-name=veryfasttree
#SBATCH --mail-type=FAIL,BEGIN,END
#SBATCH --error=%x-%J-%u.err
#SBATCH --output=%x-%J-%u.out
module --force purge
ml biocontainers veryfasttree
vg
Link to section 'Introduction' of 'vg' Introduction
Variation graphs (vg) provides tools for working with genome variation graphs.
Home page: https://github.com/vgteam/vg
Link to section 'Versions' of 'vg' Versions
- 1.40.0
Link to section 'Commands' of 'vg' Commands
- vg
Link to section 'Module' of 'vg' Module
You can load the modules by:
module load biocontainers
module load vg
Link to section 'Example job' of 'vg' Example job
Using #!/bin/sh -l
as shebang in the slurm job script will cause the failure of some biocontainer modules. Please use #!/bin/bash
instead.
To run vg on our clusters:
#!/bin/bash
#SBATCH -A myallocation # Allocation name
#SBATCH -t 1:00:00
#SBATCH -N 1
#SBATCH -n 1
#SBATCH --job-name=vg
#SBATCH --mail-type=FAIL,BEGIN,END
#SBATCH --error=%x-%J-%u.err
#SBATCH --output=%x-%J-%u.out
module --force purge
ml biocontainers vg
vg construct -r test/small/x.fa -v test/small/x.vcf.gz >x.vg
# GFA output
vg view x.vg >x.gfa
# dot output suitable for graphviz
vg view -d x.vg >x.dot
# And if you have a GAM file
cp small/x-s1337-n1.gam x.gam
# json version of binary alignments
vg view -a x.gam >x.json
vg align -s CTACTGACAGCAGAAGTTTGCTGTGAAGATTAAATTAGGTGATGCTTG x.vg
viennarna
Link to section 'Introduction' of 'viennarna' Introduction
Viennarna
is a set of standalone programs and libraries used for prediction and analysis of RNA secondary structures.
For more information, please check its website: https://biocontainers.pro/tools/viennarna and its home page: https://www.tbi.univie.ac.at/RNA/.
Link to section 'Versions' of 'viennarna' Versions
- 2.5.0
Link to section 'Commands' of 'viennarna' Commands
- RNA2Dfold
- RNALalifold
- RNALfold
- RNAPKplex
- RNAaliduplex
- RNAalifold
- RNAcofold
- RNAdistance
- RNAdos
- RNAduplex
- RNAeval
- RNAfold
- RNAforester
- RNAheat
- RNAinverse
- RNAlocmin
- RNAmultifold
- RNApaln
- RNAparconv
- RNApdist
- RNAplex
- RNAplfold
- RNAplot
- RNApvmin
- RNAsnoop
- RNAsubopt
- RNAup
- Kinfold
- b2ct
- popt
Link to section 'Module' of 'viennarna' Module
You can load the modules by:
module load biocontainers
module load viennarna
Link to section 'Example job' of 'viennarna' Example job
Using #!/bin/sh -l
as shebang in the slurm job script will cause the failure of some biocontainer modules. Please use #!/bin/bash
instead.
To run Viennarna on our clusters:
#!/bin/bash
#SBATCH -A myallocation # Allocation name
#SBATCH -t 1:00:00
#SBATCH -N 1
#SBATCH -n 1
#SBATCH --job-name=viennarna
#SBATCH --mail-type=FAIL,BEGIN,END
#SBATCH --error=%x-%J-%u.err
#SBATCH --output=%x-%J-%u.out
module --force purge
ml biocontainers viennarna
RNAfold < test.seq
RNAfold -p --MEA < test.seq
weblogo
Link to section 'Introduction' of 'weblogo' Introduction
Weblogo
is a web based application designed to make the generation of sequence logos as easy and painless as possible.
For more information, please check its website: https://biocontainers.pro/tools/weblogo and its home page: http://weblogo.threeplusone.com.
Link to section 'Versions' of 'weblogo' Versions
- 3.7.8
Link to section 'Commands' of 'weblogo' Commands
- weblogo
Link to section 'Module' of 'weblogo' Module
You can load the modules by:
module load biocontainers
module load weblogo
Link to section 'Example job' of 'weblogo' Example job
Using #!/bin/sh -l
as shebang in the slurm job script will cause the failure of some biocontainer modules. Please use #!/bin/bash
instead.
To run Weblogo on our clusters:
#!/bin/bash
#SBATCH -A myallocation # Allocation name
#SBATCH -t 1:00:00
#SBATCH -N 1
#SBATCH -n 1
#SBATCH --job-name=weblogo
#SBATCH --mail-type=FAIL,BEGIN,END
#SBATCH --error=%x-%J-%u.err
#SBATCH --output=%x-%J-%u.out
module --force purge
ml biocontainers weblogo
weblogo --resolution 600 --format PNG \
<seq.fasta >logo.png
vsearch
Link to section 'Introduction' of 'vsearch' Introduction
Vsearch
is a versatile open source tool for metagenomics.
For more information, please check its website: https://biocontainers.pro/tools/vsearch and its home page on Github.
Link to section 'Versions' of 'vsearch' Versions
- 2.19.0
- 2.21.1
- 2.22.1
Link to section 'Commands' of 'vsearch' Commands
- vsearch
Link to section 'Module' of 'vsearch' Module
You can load the modules by:
module load biocontainers
module load vsearch
Link to section 'Example job' of 'vsearch' Example job
Using #!/bin/sh -l
as shebang in the slurm job script will cause the failure of some biocontainer modules. Please use #!/bin/bash
instead.
To run Vsearch on our clusters:
#!/bin/bash
#SBATCH -A myallocation # Allocation name
#SBATCH -t 1:00:00
#SBATCH -N 1
#SBATCH -n 1
#SBATCH --job-name=vsearch
#SBATCH --mail-type=FAIL,BEGIN,END
#SBATCH --error=%x-%J-%u.err
#SBATCH --output=%x-%J-%u.out
module --force purge
ml biocontainers vsearch
vsearch -sintax SRR8723605_merged.fasta -db rdp_16s_v16_sp.fa \
-tabbedout SRR8723605_out.txt -strand both -sintax_cutoff 0.5
whatshap
Link to section 'Introduction' of 'whatshap' Introduction
Whatshap is a software for phasing genomic variants using DNA sequencing reads, also called read-based phasing or haplotype assembly. It is especially suitable for long reads, but works also well with short reads.
BioContainers: https://biocontainers.pro/tools/whatshap
Home page: https://github.com/whatshap/whatshap
Link to section 'Versions' of 'whatshap' Versions
- 1.4
Link to section 'Commands' of 'whatshap' Commands
- whatshap
Link to section 'Module' of 'whatshap' Module
You can load the modules by:
module load biocontainers
module load whatshap
Link to section 'Example job' of 'whatshap' Example job
Using #!/bin/sh -l
as shebang in the slurm job script will cause the failure of some biocontainer modules. Please use #!/bin/bash
instead.
To run whatshap on our clusters:
#!/bin/bash
#SBATCH -A myallocation # Allocation name
#SBATCH -t 1:00:00
#SBATCH -N 1
#SBATCH -n 1
#SBATCH --job-name=whatshap
#SBATCH --mail-type=FAIL,BEGIN,END
#SBATCH --error=%x-%J-%u.err
#SBATCH --output=%x-%J-%u.out
module --force purge
ml biocontainers whatshap
whatshap phase --indels \
--reference=reference.fasta \
variants.vcf pacbio.bam
wiggletools
Link to section 'Introduction' of 'wiggletools' Introduction
The WiggleTools package allows genomewide data files to be manipulated as numerical functions, equipped with all the standard functional analysis operators (sum, product, product by a scalar, comparators), and derived statistics (mean, median, variance, stddev, t-test, Wilcoxon's rank sum test, etc).
Docker hub: https://hub.docker.com/r/ensemblorg/wiggletools
Home page: https://github.com/Ensembl/WiggleTools
Link to section 'Versions' of 'wiggletools' Versions
- 1.2.11
Link to section 'Commands' of 'wiggletools' Commands
- wiggletools
Link to section 'Module' of 'wiggletools' Module
You can load the modules by:
module load biocontainers
module load wiggletools
Link to section 'Example job' of 'wiggletools' Example job
Using #!/bin/sh -l
as shebang in the slurm job script will cause the failure of some biocontainer modules. Please use #!/bin/bash
instead.
To run wiggletools on our clusters:
#!/bin/bash
#SBATCH -A myallocation # Allocation name
#SBATCH -t 1:00:00
#SBATCH -N 1
#SBATCH -n 1
#SBATCH --job-name=wiggletools
#SBATCH --mail-type=FAIL,BEGIN,END
#SBATCH --error=%x-%J-%u.err
#SBATCH --output=%x-%J-%u.out
module --force purge
ml biocontainers wiggletools
wiggletools test/fixedStep.wig
wiggletools test/fixedStep.bw
wiggletools test/bedfile.bg
wiggletools test/overlapping.bed
wiggletools test/bam.bam
wiggletools test/cram.cram
wiggletools test/vcf.vcf
wiggletools test/bcf.bcf
winnowmap
Link to section 'Introduction' of 'winnowmap' Introduction
Winnowmap is a long-read mapping algorithm optimized for mapping ONT and PacBio reads to repetitive reference sequences.
BioContainers: https://biocontainers.pro/tools/winnowmap
Home page: https://github.com/marbl/Winnowmap
Link to section 'Versions' of 'winnowmap' Versions
- 2.03
Link to section 'Commands' of 'winnowmap' Commands
- winnowmap
Link to section 'Module' of 'winnowmap' Module
You can load the modules by:
module load biocontainers
module load winnowmap
Link to section 'Example job' of 'winnowmap' Example job
Using #!/bin/sh -l
as shebang in the slurm job script will cause the failure of some biocontainer modules. Please use #!/bin/bash
instead.
To run winnowmap on our clusters:
#!/bin/bash
#SBATCH -A myallocation # Allocation name
#SBATCH -t 1:00:00
#SBATCH -N 1
#SBATCH -n 1
#SBATCH --job-name=winnowmap
#SBATCH --mail-type=FAIL,BEGIN,END
#SBATCH --error=%x-%J-%u.err
#SBATCH --output=%x-%J-%u.out
module --force purge
ml biocontainers winnowmap
winnowmap -W repetitive_k15.txt \
-ax map-pb Cm.contigs.fasta \
SRR3982487.fastq > output.sam
wtdbg
Link to section 'Introduction' of 'wtdbg' Introduction
Wtdbg2
is a de novo sequence assembler for long noisy reads produced by PacBio or Oxford Nanopore Technologies (ONT).
For more information, please check its website: https://biocontainers.pro/tools/wtdbg and its home page on Github.
Link to section 'Versions' of 'wtdbg' Versions
- 2.5
Link to section 'Commands' of 'wtdbg' Commands
- wtdbg-cns
- wtdbg2
- wtpoa-cns
Link to section 'Module' of 'wtdbg' Module
You can load the modules by:
module load biocontainers
module load wtdbg
Link to section 'Example job' of 'wtdbg' Example job
Using #!/bin/sh -l
as shebang in the slurm job script will cause the failure of some biocontainer modules. Please use #!/bin/bash
instead.
To run Wtdbg2 on our clusters:
#!/bin/bash
#SBATCH -A myallocation # Allocation name
#SBATCH -t 1:00:00
#SBATCH -N 1
#SBATCH -n 24
#SBATCH --job-name=wtdbg
#SBATCH --mail-type=FAIL,BEGIN,END
#SBATCH --error=%x-%J-%u.err
#SBATCH --output=%x-%J-%u.out
module --force purge
ml biocontainers wtdbg
wtpoa-cns -t 24 -i dbg.ctg.lay.gz -fo dbg.ctg.fa
bayescan
Link to section 'Introduction' of 'bayescan' Introduction
BayeScan aims at identifying candidate loci under natural selection from genetic data, using differences in allele frequencies between populations.
For more information, please check its home page on http://cmpg.unibe.ch/software/BayeScan/.
Link to section 'Versions' of 'bayescan' Versions
-
2.1
Link to section 'Commands' of 'bayescan' Commands
-
bayescan
Link to section 'Module' of 'bayescan' Module
You can load the modules by:
module load biocontainers
module load bayescan
Link to section 'Example job' of 'bayescan' Example job
Using #!/bin/sh -l
as shebang in the slurm job script will cause the failure of some biocontainer modules. Please use #!/bin/bash
instead.
To run bayescan on our clusters:
#!/bin/bash
#SBATCH -A myallocation # Allocation name
#SBATCH -t 1:00:00
#SBATCH -N 1
#SBATCH -n 1
#SBATCH --job-name=bayescan
#SBATCH --mail-type=FAIL,BEGIN,END
#SBATCH --error=%x-%J-%u.err
#SBATCH --output=%x-%J-%u.out
module --force purge
ml biocontainers bayescan
aspera-connect
Link to section 'Introduction' of 'aspera-connect' Introduction
Aspera connect
is software that allows download and upload data. The software includes a command line tool (ascp) that allows scripted data transfer.
Link to section 'Versions' of 'aspera-connect' Versions
- 4.2.6
Link to section 'Commands' of 'aspera-connect' Commands
- ascp
- ascp4
- asperaconnect
- asperaconnect.bin
- asperaconnect-nmh
- asperacrypt
- asunprotect
Link to section 'Module' of 'aspera-connect' Module
You can load the modules by:
module load biocontainers
module load aspera-connect
Link to section 'Example job' of 'aspera-connect' Example job
Using #!/bin/sh -l
as shebang in the slurm job script will cause the failure of some biocontainer modules. Please use #!/bin/bash
instead.
To run Abacas on our clusters:
#!/bin/bash
#SBATCH -A myallocation # Allocation name
#SBATCH -t 1:00:00
#SBATCH -N 1
#SBATCH -n 1
#SBATCH --job-name=abacas
#SBATCH --mail-type=FAIL,BEGIN,END
#SBATCH --error=%x-%J-%u.err
#SBATCH --output=%x-%J-%u.out
module --force purge
ml biocontainers aspera-connect
ascp -i ~/aspera.openssh -QT -l100m -k1 -d SRC DEST
NVIDIA NGC containers
Link to section 'What is NGC?' of 'NVIDIA NGC containers' What is NGC?
Nvidia GPU cloud (NGC) is a GPU-accelerated cloud platform optimized for deep learning and scientific computing. NGC offers a comprehensive catalogue of GPU-accelerated containers, so the application runs quickly and reliably on the high performance computing environment. NGC was deployed to extend the cluster capabilities and to enable powerful software and deliver the fastest results. By utilizing Singularity and NGC, users can focus on building lean models, producing optimal solutions and gathering faster insights. For more information, please visit https://www.nvidia.com/en-us/gpu-cloud and NGC software catalog.
Link to section 'Getting Started' of 'NVIDIA NGC containers' Getting Started
Users can download containers from the NGC software catalog and run them directly using Singularity instructions from the corresponding container’s catalog page.
In addition, a subset of pre-downloaded NGC containers wrapped into convenient software modules are provided. These modules wrap underlying complexity and provide the same commands that are expected from non-containerized versions of each application.
On clusters equipped with NVIDIA GPUs, type the command below to see the lists of NGC containers we deployed.
$ module load ngc
$ module avail
Link to section 'Deployed Applications' of 'NVIDIA NGC containers' Deployed Applications
autodock
Link to section 'Description' of 'autodock' Description
The AutoDock Suite is a growing collection of methods for computational docking and virtual screening, for use in structure-based drug discovery and exploration of the basic mechanisms of biomolecular structure and function.
Link to section 'Versions' of 'autodock' Versions
- Scholar: 2020.06
- Gilbreth: 2020.06
- Anvil: 2020.06
Link to section 'Module' of 'autodock' Module
You can load the modules by:
module load ngc
module load autodock
Link to section 'Example job' of 'autodock' Example job
Using #!/bin/sh -l
as shebang in the slurm job script will cause the failure of some biocontainer modules. Please use #!/bin/bash
instead.
To run autodock on our clusters:
#!/bin/bash
#SBATCH -A myallocation # Allocation name
#SBATCH -t 1:00:00
#SBATCH -N 1
#SBATCH -n 1
#SBATCH -c 8
#SBATCH --gpus-per-node=1
#SBATCH --job-name=autodock
#SBATCH --mail-type=FAIL,BEGIN,END
#SBATCH --error=%x-%J-%u.err
#SBATCH --output=%x-%J-%u.out
module --force purge
ml ngc autodock
chroma
Link to section 'Description' of 'chroma' Description
The Chroma package provides a toolbox and executables to carry out calculation of lattice Quantum Chromodynamics LQCD. It is built on top of the QDP++ QCD Data Parallel Layer which provides an abstract data parallel view of the lattice and provides lattice wide types and expressions, using expression templates, to allow straightforward encoding of LQCD equations.
Link to section 'Versions' of 'chroma' Versions
- Scholar: 2018-cuda9.0-ubuntu16.04-volta-openmpi, 2020.06, 2021.04
- Gilbreth: 2018-cuda9.0-ubuntu16.04-volta-openmpi, 2020.06, 2021.04
Link to section 'Module' of 'chroma' Module
You can load the modules by:
module load ngc
module load chroma
Link to section 'Example job' of 'chroma' Example job
Using #!/bin/sh -l
as shebang in the slurm job script will cause the failure of some biocontainer modules. Please use #!/bin/bash
instead.
To run chroma on our clusters:
#!/bin/bash
#SBATCH -A myallocation # Allocation name
#SBATCH -t 1:00:00
#SBATCH -N 1
#SBATCH -n 1
#SBATCH -c 8
#SBATCH --gpus-per-node=1
#SBATCH --job-name=chroma
#SBATCH --mail-type=FAIL,BEGIN,END
#SBATCH --error=%x-%J-%u.err
#SBATCH --output=%x-%J-%u.out
module --force purge
ml ngc chroma
gamess
Link to section 'Description' of 'gamess' Description
The General Atomic and Molecular Electronic Structure Systems GAMESS program simulates molecular quantum chemistry, allowing users to calculate various molecular properties and dynamics.
Link to section 'Versions' of 'gamess' Versions
- Scholar: 17.09-r2-libcchem
- Gilbreth: 17.09-r2-libcchem
- Anvil: 17.09-r2-libcchem
Link to section 'Module' of 'gamess' Module
You can load the modules by:
module load ngc
module load gamess
Link to section 'Example job' of 'gamess' Example job
Using #!/bin/sh -l
as shebang in the slurm job script will cause the failure of some biocontainer modules. Please use #!/bin/bash
instead.
To run gamess on our clusters:
#!/bin/bash
#SBATCH -A myallocation # Allocation name
#SBATCH -t 1:00:00
#SBATCH -N 1
#SBATCH -n 1
#SBATCH -c 8
#SBATCH --gpus-per-node=1
#SBATCH --job-name=gamess
#SBATCH --mail-type=FAIL,BEGIN,END
#SBATCH --error=%x-%J-%u.err
#SBATCH --output=%x-%J-%u.out
module --force purge
ml ngc gamess
gromacs
Link to section 'Description' of 'gromacs' Description
GROMACS GROningen MAchine for Chemical Simulations is a molecular dynamics package primarily designed for simulations of proteins, lipids and nucleic acids. It was originally developed in the Biophysical Chemistry department of University of Groningen, and is now maintained by contributors in universities and research centers across the world.
Link to section 'Versions' of 'gromacs' Versions
- Scholar: 2018.2, 2020.2, 2021, 2021.3
- Gilbreth: 2018.2, 2020.2, 2021, 2021.3
- Anvil: 2018.2, 2020.2, 2021, 2021.3
Link to section 'Module' of 'gromacs' Module
You can load the modules by:
module load ngc
module load gromacs
Link to section 'Example job' of 'gromacs' Example job
Using #!/bin/sh -l
as shebang in the slurm job script will cause the failure of some biocontainer modules. Please use #!/bin/bash
instead.
To run gromacs on our clusters:
#!/bin/bash
#SBATCH -A myallocation # Allocation name
#SBATCH -t 1:00:00
#SBATCH -N 1
#SBATCH -n 1
#SBATCH -c 8
#SBATCH --gpus-per-node=1
#SBATCH --job-name=gromacs
#SBATCH --mail-type=FAIL,BEGIN,END
#SBATCH --error=%x-%J-%u.err
#SBATCH --output=%x-%J-%u.out
module --force purge
ml ngc gromacs
julia
Link to section 'Description' of 'julia' Description
The Julia programming language is a flexible dynamic language, appropriate for scientific and numerical computing, with performance comparable to traditional statically-typed languages.
Link to section 'Versions' of 'julia' Versions
- Scholar: v1.5.0, v2.4.2
- Gilbreth: v1.5.0, v2.4.2
- Anvil: v1.5.0, v2.4.2
Link to section 'Module' of 'julia' Module
You can load the modules by:
module load ngc
module load julia
Link to section 'Example job' of 'julia' Example job
#!/bin/sh -l
as shebang in the slurm job script will cause the failure of some biocontainer modules. Please use #!/bin/bash
instead.
To run julia on our clusters:
#!/bin/bash
#SBATCH -A myallocation # Allocation name
#SBATCH -t 1:00:00
#SBATCH -N 1
#SBATCH -n 1
#SBATCH -c 8
#SBATCH --gpus-per-node=1
#SBATCH --job-name=julia
#SBATCH --mail-type=FAIL,BEGIN,END
#SBATCH --error=%x-%J-%u.err
#SBATCH --output=%x-%J-%u.out
module --force purge
ml ngc julia
lammps
Link to section 'Description' of 'lammps' Description
Large-scale Atomic/Molecular Massively Parallel Simulator LAMMPS is a software application designed for molecular dynamics simulations. It has potentials for solid-state materials metals, semiconductor, soft matter biomolecules, polymers and coarse-grained or mesoscopic systems. It can be used to model atoms or, more generically, as a parallel particle simulator at the atomic, meso, or continuum scale.
Link to section 'Versions' of 'lammps' Versions
- Scholar: 10Feb2021, 15Jun2020, 24Oct2018, 29Oct2020
- Gilbreth: 10Feb2021, 15Jun2020, 24Oct2018, 29Oct2020
- Anvil: 10Feb2021, 15Jun2020, 24Oct2018, 29Oct2020
Link to section 'Module' of 'lammps' Module
You can load the modules by:
module load ngc
module load lammps
Link to section 'Example job' of 'lammps' Example job
#!/bin/sh -l
as shebang in the slurm job script will cause the failure of some biocontainer modules. Please use #!/bin/bash
instead.
To run lammps on our clusters:
#!/bin/bash
#SBATCH -A myallocation # Allocation name
#SBATCH -t 1:00:00
#SBATCH -N 1
#SBATCH -n 1
#SBATCH -c 8
#SBATCH --gpus-per-node=1
#SBATCH --job-name=lammps
#SBATCH --mail-type=FAIL,BEGIN,END
#SBATCH --error=%x-%J-%u.err
#SBATCH --output=%x-%J-%u.out
module --force purge
ml ngc lammps
milc
Link to section 'Description' of 'milc' Description
MILC represents part of a set of codes written by the MIMD Lattice Computation MILC collaboration used to study quantum chromodynamics QCD, the theory of the strong interactions of subatomic physics. It performs simulations of four dimensional SU3 lattice gauge theory on MIMD parallel machines. \Strong interactions\ are responsible for binding quarks into protons and neutrons and holding them all together in the atomic nucleus.
Link to section 'Versions' of 'milc' Versions
- Scholar: quda0.8-patch4Oct2017
- Gilbreth: quda0.8-patch4Oct2017
Link to section 'Module' of 'milc' Module
You can load the modules by:
module load ngc
module load milc
Link to section 'Example job' of 'milc' Example job
Using #!/bin/sh -l
as shebang in the slurm job script will cause the failure of some biocontainer modules. Please use #!/bin/bash
instead.
To run milc on our clusters:
#!/bin/bash
#SBATCH -A myallocation # Allocation name
#SBATCH -t 1:00:00
#SBATCH -N 1
#SBATCH -n 1
#SBATCH -c 8
#SBATCH --gpus-per-node=1
#SBATCH --job-name=milc
#SBATCH --mail-type=FAIL,BEGIN,END
#SBATCH --error=%x-%J-%u.err
#SBATCH --output=%x-%J-%u.out
module --force purge
ml ngc milc
namd
Link to section 'Description' of 'namd' Description
NAMD is a parallel molecular dynamics code designed for high-performance simulation of large biomolecular systems. NAMD uses the popular molecular graphics program VMD for simulation setup and trajectory analysis, but is also file-compatible with AMBER, CHARMM, and X-PLOR.
Link to section 'Versions' of 'namd' Versions
- Scholar: 2.13-multinode, 2.13-singlenode, 3.0-alpha3-singlenode
- Gilbreth: 2.13-multinode, 2.13-singlenode, 3.0-alpha3-singlenode
- Anvil: 2.13-multinode, 2.13-singlenode, 3.0-alpha3-singlenode
Link to section 'Module' of 'namd' Module
You can load the modules by:
module load ngc
module load namd
Link to section 'Example job' of 'namd' Example job
Using #!/bin/sh -l
as shebang in the slurm job script will cause the failure of some biocontainer modules. Please use #!/bin/bash
instead.
To run namd on our clusters:
#!/bin/bash
#SBATCH -A myallocation # Allocation name
#SBATCH -t 1:00:00
#SBATCH -N 1
#SBATCH -n 1
#SBATCH -c 8
#SBATCH --gpus-per-node=1
#SBATCH --job-name=namd
#SBATCH --mail-type=FAIL,BEGIN,END
#SBATCH --error=%x-%J-%u.err
#SBATCH --output=%x-%J-%u.out
module --force purge
ml ngc namd
nvhpc
Link to section 'Description' of 'nvhpc' Description
The NVIDIA HPC SDK C, C++, and Fortran compilers support GPU acceleration of HPC modeling and simulation applications with standard C++ and Fortran, OpenACC® directives, and CUDA®. GPU-accelerated math libraries maximize performance on common HPC algorithms, and optimized communications libraries enable standards-based multi-GPU and scalable systems programming.
Link to section 'Versions' of 'nvhpc' Versions
- Scholar: 20.7, 20.9, 20.11, 21.5, 21.9
- Gilbreth: 20.7, 20.9, 20.11, 21.5, 21.9
- Anvil: 20.7, 20.9, 20.11, 21.5, 21.9
Link to section 'Module' of 'nvhpc' Module
You can load the modules by:
module load ngc
module load nvhpc
Link to section 'Example job' of 'nvhpc' Example job
Using #!/bin/sh -l
as shebang in the slurm job script will cause the failure of some biocontainer modules. Please use #!/bin/bash
instead.
To run nvhpc on our clusters:
#!/bin/bash
#SBATCH -A myallocation # Allocation name
#SBATCH -t 1:00:00
#SBATCH -N 1
#SBATCH -n 1
#SBATCH -c 8
#SBATCH --gpus-per-node=1
#SBATCH --job-name=nvhpc
#SBATCH --mail-type=FAIL,BEGIN,END
#SBATCH --error=%x-%J-%u.err
#SBATCH --output=%x-%J-%u.out
module --force purge
ml ngc nvhpc
parabricks
Link to section 'Description' of 'parabricks' Description
NVIDIAs Clara Parabricks brings next generation sequencing to GPUs, accelerating an array of gold-standard tooling such as BWA-MEM, GATK4, Googles DeepVariant, and many more. Users can achieve a 30-60x acceleration and 99.99% accuracy for variant calling when comparing against CPU-only BWA-GATK4 pipelines, meaning a single server can process up to 60 whole genomes per day. These tools can be easily integrated into current pipelines with drop-in replacement commands to quickly bring speed and data-center scale to a range of applications including germline, somatic and RNA workflows.
Link to section 'Versions' of 'parabricks' Versions
- Scholar: 4.0.0-1
- Gilbreth: 4.0.0-1
- Anvil: 4.0.0-1
Link to section 'Module' of 'parabricks' Module
You can load the modules by:
module load ngc
module load parabricks
Link to section 'Example job' of 'parabricks' Example job
Using #!/bin/sh -l
as shebang in the slurm job script will cause the failure of some biocontainer modules. Please use #!/bin/bash
instead.
To run parabricks on our clusters:
#!/bin/bash
#SBATCH -A myallocation # Allocation name
#SBATCH -t 1:00:00
#SBATCH -N 1
#SBATCH -n 1
#SBATCH -c 8
#SBATCH --gpus-per-node=1
#SBATCH --job-name=parabricks
#SBATCH --mail-type=FAIL,BEGIN,END
#SBATCH --error=%x-%J-%u.err
#SBATCH --output=%x-%J-%u.out
module --force purge
ml ngc parabricks
paraview
Link to section 'Description' of 'paraview' Description
no ParaView client GUI in this container, but ParaView Web application is included.
Link to section 'Versions' of 'paraview' Versions
- Scholar: 5.9.0
- Gilbreth: 5.9.0
- Anvil: 5.9.0
Link to section 'Module' of 'paraview' Module
You can load the modules by:
module load ngc
module load paraview
Link to section 'Example job' of 'paraview' Example job
Using #!/bin/sh -l
as shebang in the slurm job script will cause the failure of some biocontainer modules. Please use #!/bin/bash
instead.
To run paraview on our clusters:
#!/bin/bash
#SBATCH -A myallocation # Allocation name
#SBATCH -t 1:00:00
#SBATCH -N 1
#SBATCH -n 1
#SBATCH -c 8
#SBATCH --gpus-per-node=1
#SBATCH --job-name=paraview
#SBATCH --mail-type=FAIL,BEGIN,END
#SBATCH --error=%x-%J-%u.err
#SBATCH --output=%x-%J-%u.out
module --force purge
ml ngc paraview
pytorch
Link to section 'Description' of 'pytorch' Description
PyTorch is a GPU accelerated tensor computational framework with a Python front end. Functionality can be easily extended with common Python libraries such as NumPy, SciPy, and Cython. Automatic differentiation is done with a tape-based system at both a functional and neural network layer level. This functionality brings a high level of flexibility and speed as a deep learning framework and provides accelerated NumPy-like functionality.
Link to section 'Versions' of 'pytorch' Versions
- Scholar: 20.02-py3, 20.03-py3, 20.06-py3, 20.11-py3, 20.12-py3, 21.06-py3, 21.09-py3
- Gilbreth: 20.02-py3, 20.03-py3, 20.06-py3, 20.11-py3, 20.12-py3, 21.06-py3, 21.09-py3
- Anvil: 20.02-py3, 20.03-py3, 20.06-py3, 20.11-py3, 20.12-py3, 21.06-py3, 21.09-py3
Link to section 'Module' of 'pytorch' Module
You can load the modules by:
module load ngc
module load pytorch
Link to section 'Example job' of 'pytorch' Example job
Using #!/bin/sh -l
as shebang in the slurm job script will cause the failure of some biocontainer modules. Please use #!/bin/bash
instead.
To run pytorch on our clusters:
#!/bin/bash
#SBATCH -A myallocation # Allocation name
#SBATCH -t 1:00:00
#SBATCH -N 1
#SBATCH -n 1
#SBATCH -c 8
#SBATCH --gpus-per-node=1
#SBATCH --job-name=pytorch
#SBATCH --mail-type=FAIL,BEGIN,END
#SBATCH --error=%x-%J-%u.err
#SBATCH --output=%x-%J-%u.out
module --force purge
ml ngc pytorch
qmcpack
Link to section 'Description' of 'qmcpack' Description
QMCPACK is an open-source, high-performance electronic structure code that implements numerous Quantum Monte Carlo algorithms. Its main applications are electronic structure calculations of molecular, periodic 2D and periodic 3D solid-state systems. Variational Monte Carlo VMC, diffusion Monte Carlo DMC and a number of other advanced QMC algorithms are implemented. By directly solving the Schrodinger equation, QMC methods offer greater accuracy than methods such as density functional theory, but at a trade-off of much greater computational expense. Distinct from many other correlated many-body methods, QMC methods are readily applicable to both bulk periodic and isolated molecular systems.
Link to section 'Versions' of 'qmcpack' Versions
- Scholar: v3.5.0
- Gilbreth: v3.5.0
- Anvil: v3.5.0
Link to section 'Module' of 'qmcpack' Module
You can load the modules by:
module load ngc
module load qmcpack
Link to section 'Example job' of 'qmcpack' Example job
Using #!/bin/sh -l
as shebang in the slurm job script will cause the failure of some biocontainer modules. Please use #!/bin/bash
instead.
To run qmcpack on our clusters:
#!/bin/bash
#SBATCH -A myallocation # Allocation name
#SBATCH -t 1:00:00
#SBATCH -N 1
#SBATCH -n 1
#SBATCH -c 8
#SBATCH --gpus-per-node=1
#SBATCH --job-name=qmcpack
#SBATCH --mail-type=FAIL,BEGIN,END
#SBATCH --error=%x-%J-%u.err
#SBATCH --output=%x-%J-%u.out
module --force purge
ml ngc qmcpack
quantum_espresso
Link to section 'Description' of 'quantum_espresso' Description
Quantum ESPRESSO is an integrated suite of Open-Source computer codes for electronic-structure calculations and materials modeling at the nanoscale based on density-functional theory, plane waves, and pseudopotentials.
Link to section 'Versions' of 'quantum_espresso' Versions
- Scholar: v6.6a1, v6.7
- Gilbreth: v6.6a1, v6.7
- Anvil: v6.6a1, v6.7
Link to section 'Module' of 'quantum_espresso' Module
You can load the modules by:
module load ngc
module load quantum_espresso
Link to section 'Example job' of 'quantum_espresso' Example job
Using #!/bin/sh -l
as shebang in the slurm job script will cause the failure of some biocontainer modules. Please use #!/bin/bash
instead.
To run quantum_espresso on our clusters:
#!/bin/bash
#SBATCH -A myallocation # Allocation name
#SBATCH -t 1:00:00
#SBATCH -N 1
#SBATCH -n 1
#SBATCH -c 8
#SBATCH --gpus-per-node=1
#SBATCH --job-name=quantum_espresso
#SBATCH --mail-type=FAIL,BEGIN,END
#SBATCH --error=%x-%J-%u.err
#SBATCH --output=%x-%J-%u.out
module --force purge
ml ngc quantum_espresso
rapidsai
Link to section 'Description' of 'rapidsai' Description
The RAPIDS suite of software libraries gives you the freedom to execute end-to-end data science and analytics pipelines entirely on GPUs. It relies on NVIDIA® CUDA® primitives for low-level compute optimization, but exposes that GPU parallelism and high-bandwidth memory speed through user-friendly Python interfaces.
Link to section 'Versions' of 'rapidsai' Versions
- Scholar: 0.12, 0.13, 0.14, 0.15, 0.16, 0.17, 21.06, 21.10
- Gilbreth: 0.12, 0.13, 0.14, 0.15, 0.16, 0.17, 21.06, 21.10
- Anvil: 0.12, 0.13, 0.14, 0.15, 0.16, 0.17, 21.06, 21.10
Link to section 'Module' of 'rapidsai' Module
You can load the modules by:
module load ngc
module load rapidsai
Link to section 'Example job' of 'rapidsai' Example job
Using #!/bin/sh -l
as shebang in the slurm job script will cause the failure of some biocontainer modules. Please use #!/bin/bash
instead.
To run rapidsai on our clusters:
#!/bin/bash
#SBATCH -A myallocation # Allocation name
#SBATCH -t 1:00:00
#SBATCH -N 1
#SBATCH -n 1
#SBATCH -c 8
#SBATCH --gpus-per-node=1
#SBATCH --job-name=rapidsai
#SBATCH --mail-type=FAIL,BEGIN,END
#SBATCH --error=%x-%J-%u.err
#SBATCH --output=%x-%J-%u.out
module --force purge
ml ngc rapidsai
relion
Link to section 'Description' of 'relion' Description
RELION for REgularized LIkelihood OptimizatioN implements an empirical Bayesian approach for analysis of electron cryo-microscopy Cryo-EM. Specifically it provides methods of refinement of singular or multiple 3D reconstructions as well as 2D class averages. RELION is an important tool in the study of living cells.
Link to section 'Versions' of 'relion' Versions
- Scholar: 2.1.b1, 3.0.8, 3.1.0, 3.1.2, 3.1.3
- Gilbreth: 2.1.b1, 3.0.8, 3.1.0, 3.1.2, 3.1.3
- Anvil: 2.1.b1, 3.1.0, 3.1.2, 3.1.3
Link to section 'Module' of 'relion' Module
You can load the modules by:
module load ngc
module load relion
Link to section 'Example job' of 'relion' Example job
Using #!/bin/sh -l
as shebang in the slurm job script will cause the failure of some biocontainer modules. Please use #!/bin/bash
instead.
To run relion on our clusters:
#!/bin/bash
#SBATCH -A myallocation # Allocation name
#SBATCH -t 1:00:00
#SBATCH -N 1
#SBATCH -n 1
#SBATCH -c 8
#SBATCH --gpus-per-node=1
#SBATCH --job-name=relion
#SBATCH --mail-type=FAIL,BEGIN,END
#SBATCH --error=%x-%J-%u.err
#SBATCH --output=%x-%J-%u.out
module --force purge
ml ngc relion
tensorflow
Link to section 'Description' of 'tensorflow' Description
TensorFlow is an open-source software library for numerical computation using data flow graphs. Nodes in the graph represent mathematical operations, while the graph edges represent the multidimensional data arrays tensors that flow between them. This flexible architecture lets you deploy computation to one or more CPUs or GPUs in a desktop, server, or mobile device without rewriting code.
Link to section 'Versions' of 'tensorflow' Versions
- Scholar: 20.02-tf1-py3, 20.02-tf2-py3, 20.03-tf1-py3, 20.03-tf2-py3, 20.06-tf1-py3, 20.06-tf2-py3, 20.11-tf1-py3, 20.11-tf2-py3, 20.12-tf1-py3, 20.12-tf2-py3, 21.06-tf1-py3, 21.06-tf2-py3, 21.09-tf1-py3, 21.09-tf2-py3
- Gilbreth: 20.02-tf1-py3, 20.02-tf2-py3, 20.03-tf1-py3, 20.03-tf2-py3, 20.06-tf1-py3, 20.06-tf2-py3, 20.11-tf1-py3, 20.11-tf2-py3, 20.12-tf1-py3, 20.12-tf2-py3, 21.06-tf1-py3, 21.06-tf2-py3, 21.09-tf1-py3, 21.09-tf2-py3
- Anvil: 20.02-tf1-py3, 20.02-tf2-py3, 20.03-tf1-py3, 20.03-tf2-py3, 20.06-tf1-py3, 20.06-tf2-py3, 20.11-tf1-py3, 20.11-tf2-py3, 20.12-tf1-py3, 20.12-tf2-py3, 21.06-tf1-py3, 21.06-tf2-py3, 21.09-tf1-py3, 21.09-tf2-py3
Link to section 'Module' of 'tensorflow' Module
You can load the modules by:
module load ngc
module load tensorflow
Link to section 'Example job' of 'tensorflow' Example job
Using #!/bin/sh -l
as shebang in the slurm job script will cause the failure of some biocontainer modules. Please use #!/bin/bash
instead.
To run tensorflow on our clusters:
#!/bin/bash
#SBATCH -A myallocation # Allocation name
#SBATCH -t 1:00:00
#SBATCH -N 1
#SBATCH -n 1
#SBATCH -c 8
#SBATCH --gpus-per-node=1
#SBATCH --job-name=tensorflow
#SBATCH --mail-type=FAIL,BEGIN,END
#SBATCH --error=%x-%J-%u.err
#SBATCH --output=%x-%J-%u.out
module --force purge
ml ngc tensorflow
torchani
Link to section 'Description' of 'torchani' Description
TorchANI is a PyTorch-based program for training/inference of ANI (ANAKIN-ME) deep learning models to obtain potential energy surfaces and other physical properties of molecular systems.
Link to section 'Versions' of 'torchani' Versions
- Scholar: 2021.04
- Gilbreth: 2021.04
- Anvil: 2021.04
Link to section 'Module' of 'torchani' Module
You can load the modules by:
module load ngc
module load torchani
Link to section 'Example job' of 'torchani' Example job
Using #!/bin/sh -l
as shebang in the slurm job script will cause the failure of some biocontainer modules. Please use #!/bin/bash
instead.
To run torchani on our clusters:
#!/bin/bash
#SBATCH -A myallocation # Allocation name
#SBATCH -t 1:00:00
#SBATCH -N 1
#SBATCH -n 1
#SBATCH -c 8
#SBATCH --gpus-per-node=1
#SBATCH --job-name=torchani
#SBATCH --mail-type=FAIL,BEGIN,END
#SBATCH --error=%x-%J-%u.err
#SBATCH --output=%x-%J-%u.out
module --force purge
ml ngc torchani
AMD ROCm containers
Link to section 'What is AMD ROCm' of 'AMD ROCm containers' What is AMD ROCm
The AMD Infinity Hub contains a collection of advanced AMD GPU software containers and deployment guides for HPC, AI & Machine Learning applications, enabling researchers to speed up their time to science. Containerized applications run quickly and reliably in the high performance computing environment with full support of AMD GPUs. A collection of Infinity Hub tools were deployed to extend cluster capabilities and to enable powerful software and deliver the fastest results. By utilizing Singularity and Infinity Hub ROCm-enabled containers, users can focus on building lean models, producing optimal solutions and gathering faster insights. For more information, please visit AMD Infinity Hub.
Link to section 'Getting Started' of 'AMD ROCm containers' Getting Started
Users can download ROCm containers from the AMD Infinity Hub and run them directly using Singularity instructions from the corresponding container’s catalog page.
In addition, a subset of pre-downloaded ROCm containers wrapped into convenient software modules are provided. These modules wrap underlying complexity and provide the same commands that are expected from non-containerized versions of each application.
On clusters equipped with AMD GPUs, type the command below to see the lists of ROCm containers we deployed.
module load rocmcontainers
module avail
------------ ROCm-based application container modules for AMD GPUs -------------
cp2k/20210311--h87ec1599
deepspeed/rocm4.2_ubuntu18.04_py3.6_pytorch_1.8.1
gromacs/2020.3 (D)
namd/2.15a2
openmm/7.4.2
pytorch/1.8.1-rocm4.2-ubuntu18.04-py3.6
pytorch/1.9.0-rocm4.2-ubuntu18.04-py3.6 (D)
specfem3d/20201122--h9c0626d1
specfem3d_globe/20210322--h1ee10977
tensorflow/2.5-rocm4.2-dev
[....]
Link to section 'Deployed Applications' of 'AMD ROCm containers' Deployed Applications
cp2k
Link to section 'Description' of 'cp2k' Description
CP2K is a quantum chemistry and solid state physics software package that can perform atomistic simulations of solid state, liquid, molecular, periodic, material, crystal, and biological systems. CP2K provides a general framework for different modeling methods such as DFT using the mixed Gaussian and plane waves approaches GPW and GAPW. Supported theory levels include DFTB, LDA, GGA, MP2, RPA, semi-empirical methods AM1, PM3, PM6, RM1, MNDO, ..., and classical force fields AMBER, CHARMM, .... CP2K can do simulations of molecular dynamics, metadynamics, Monte Carlo, Ehrenfest dynamics, vibrational analysis, core level spectroscopy, energy minimization, and transition state optimization using NEB or dimer method. CP2K is written in Fortran 2008 and can be run efficiently in parallel using a combination of multi-threading, MPI, and HIP/CUDA.
Link to section 'Versions' of 'cp2k' Versions
- Bell: 8.2, 20210311--h87ec1599
- Negishi: 8.2, 20210311--h87ec1599
Link to section 'Module' of 'cp2k' Module
You can load the modules by:
module load rocmcontainers
module load cp2k
Link to section 'Example job' of 'cp2k' Example job
Using #!/bin/sh -l
as shebang in the slurm job script will cause the failure of some biocontainer modules. Please use #!/bin/bash
instead.
To run cp2k on our clusters:
#!/bin/bash
#SBATCH -A gpu
#SBATCH -t 1:00:00
#SBATCH -N 1
#SBATCH -n 1
#SBATCH -c 8
#SBATCH --gpus-per-node=1
#SBATCH --job-name=cp2k
#SBATCH --mail-type=FAIL,BEGIN,END
#SBATCH --error=%x-%J-%u.err
#SBATCH --output=%x-%J-%u.out
module --force purge
ml rocmcontainers cp2k
deepspeed
Link to section 'Description' of 'deepspeed' Description
DeepSpeed is a deep learning optimization library that makes distributed training easy, efficient, and effective.
Link to section 'Versions' of 'deepspeed' Versions
- Bell: rocm4.2_ubuntu18.04_py3.6_pytorch_1.8.1
- Negishi: rocm4.2_ubuntu18.04_py3.6_pytorch_1.8.1
Link to section 'Module' of 'deepspeed' Module
You can load the modules by:
module load rocmcontainers
module load deepspeed
Link to section 'Example job' of 'deepspeed' Example job
Using #!/bin/sh -l
as shebang in the slurm job script will cause the failure of some biocontainer modules. Please use #!/bin/bash
instead.
To run deepspeed on our clusters:
#!/bin/bash
#SBATCH -A gpu
#SBATCH -t 1:00:00
#SBATCH -N 1
#SBATCH -n 1
#SBATCH -c 8
#SBATCH --gpus-per-node=1
#SBATCH --job-name=deepspeed
#SBATCH --mail-type=FAIL,BEGIN,END
#SBATCH --error=%x-%J-%u.err
#SBATCH --output=%x-%J-%u.out
module --force purge
ml rocmcontainers deepspeed
gromacs
Link to section 'Description' of 'gromacs' Description
GROMACS is a molecular dynamics application designed to simulate Newtonian equations of motion for systems with hundreds to millions of particles. GROMACS is designed to simulate biochemical molecules like proteins, lipids, and nucleic acids that have a lot of complicated bonded interactions.
Link to section 'Versions' of 'gromacs' Versions
- Bell: 2020.3, 2022.3.amd1
- Negishi: 2020.3, 2022.3.amd1
Link to section 'Module' of 'gromacs' Module
You can load the modules by:
module load rocmcontainers
module load gromacs
Link to section 'Example job' of 'gromacs' Example job
Using #!/bin/sh -l
as shebang in the slurm job script will cause the failure of some biocontainer modules. Please use #!/bin/bash
instead.
To run gromacs on our clusters:
#!/bin/bash
#SBATCH -A gpu
#SBATCH -t 1:00:00
#SBATCH -N 1
#SBATCH -n 1
#SBATCH -c 8
#SBATCH --gpus-per-node=1
#SBATCH --job-name=gromacs
#SBATCH --mail-type=FAIL,BEGIN,END
#SBATCH --error=%x-%J-%u.err
#SBATCH --output=%x-%J-%u.out
module --force purge
ml rocmcontainers gromacs
lammps
Link to section 'Description' of 'lammps' Description
LAMMPS stands for Large-scale Atomic/Molecular Massively Parallel Simulator and is a classical molecular dynamics MD code.
Link to section 'Versions' of 'lammps' Versions
- Bell: 2022.5.04
- Negishi: 2022.5.04
Link to section 'Module' of 'lammps' Module
You can load the modules by:
module load rocmcontainers
module load lammps
Link to section 'Example job' of 'lammps' Example job
Using #!/bin/sh -l
as shebang in the slurm job script will cause the failure of some biocontainer modules. Please use #!/bin/bash
instead.
To run lammps on our clusters:
#!/bin/bash
#SBATCH -A gpu
#SBATCH -t 1:00:00
#SBATCH -N 1
#SBATCH -n 1
#SBATCH -c 8
#SBATCH --gpus-per-node=1
#SBATCH --job-name=lammps
#SBATCH --mail-type=FAIL,BEGIN,END
#SBATCH --error=%x-%J-%u.err
#SBATCH --output=%x-%J-%u.out
module --force purge
ml rocmcontainers lammps
namd
Link to section 'Description' of 'namd' Description
NAMD is a parallel molecular dynamics code designed for high-performance simulation of large biomolecular systems. NAMD uses the popular molecular graphics program VMD for simulation setup and trajectory analysis, but is also file-compatible with AMBER, CHARMM, and X-PLOR.
Link to section 'Versions' of 'namd' Versions
- Bell: 2.15a2, 3.0a9
- Negishi: 2.15a2, 3.0a9
Link to section 'Module' of 'namd' Module
You can load the modules by:
module load rocmcontainers
module load namd
Link to section 'Example job' of 'namd' Example job
Using #!/bin/sh -l
as shebang in the slurm job script will cause the failure of some biocontainer modules. Please use #!/bin/bash
instead.
To run namd on our clusters:
#!/bin/bash
#SBATCH -A gpu
#SBATCH -t 1:00:00
#SBATCH -N 1
#SBATCH -n 1
#SBATCH -c 8
#SBATCH --gpus-per-node=1
#SBATCH --job-name=namd
#SBATCH --mail-type=FAIL,BEGIN,END
#SBATCH --error=%x-%J-%u.err
#SBATCH --output=%x-%J-%u.out
module --force purge
ml rocmcontainers namd
openmm
Link to section 'Description' of 'openmm' Description
OpenMM is a high-performance toolkit for molecular simulation. It can be used as an application, a library, or a flexible programming environment. OpenMM includes extensive language bindings for Python, C, C++, and even Fortran. The code is open source and developed on GitHub, licensed under MIT and LGPL.
Link to section 'Versions' of 'openmm' Versions
- Bell: 7.4.2, 7.7.0
- Negishi: 7.4.2, 7.7.0
Link to section 'Module' of 'openmm' Module
You can load the modules by:
module load rocmcontainers
module load openmm
Link to section 'Example job' of 'openmm' Example job
Using #!/bin/sh -l
as shebang in the slurm job script will cause the failure of some biocontainer modules. Please use #!/bin/bash
instead.
To run openmm on our clusters:
#!/bin/bash
#SBATCH -A gpu
#SBATCH -t 1:00:00
#SBATCH -N 1
#SBATCH -n 1
#SBATCH -c 8
#SBATCH --gpus-per-node=1
#SBATCH --job-name=openmm
#SBATCH --mail-type=FAIL,BEGIN,END
#SBATCH --error=%x-%J-%u.err
#SBATCH --output=%x-%J-%u.out
module --force purge
ml rocmcontainers openmm
pytorch
Link to section 'Description' of 'pytorch' Description
PyTorch is an optimized tensor library for deep learning using GPUs and CPUs.
Link to section 'Versions' of 'pytorch' Versions
- Bell: 1.8.1-rocm4.2-ubuntu18.04-py3.6, 1.9.0-rocm4.2-ubuntu18.04-py3.6, 1.10.0-rocm5.0-ubuntu18.04-py3.7
- Negishi: 1.8.1-rocm4.2-ubuntu18.04-py3.6, 1.9.0-rocm4.2-ubuntu18.04-py3.6, 1.10.0-rocm5.0-ubuntu18.04-py3.7
Link to section 'Module' of 'pytorch' Module
You can load the modules by:
module load rocmcontainers
module load pytorch
Link to section 'Example job' of 'pytorch' Example job
Using #!/bin/sh -l
as shebang in the slurm job script will cause the failure of some biocontainer modules. Please use #!/bin/bash
instead.
To run pytorch on our clusters:
#!/bin/bash
#SBATCH -A gpu
#SBATCH -t 1:00:00
#SBATCH -N 1
#SBATCH -n 1
#SBATCH -c 8
#SBATCH --gpus-per-node=1
#SBATCH --job-name=pytorch
#SBATCH --mail-type=FAIL,BEGIN,END
#SBATCH --error=%x-%J-%u.err
#SBATCH --output=%x-%J-%u.out
module --force purge
ml rocmcontainers pytorch
rochpcg
Link to section 'Description' of 'rochpcg' Description
HPCG is a HPC benchmark intended to better represent computational and data access patterns that closely match a broad set of scientific workloads. This container implements the HPCG benchmark on top of AMDs ROCm platform.
Link to section 'Versions' of 'rochpcg' Versions
- Bell: 3.1.0
- Negishi: 3.1.0
Link to section 'Module' of 'rochpcg' Module
You can load the modules by:
module load rocmcontainers
module load rochpcg
Link to section 'Example job' of 'rochpcg' Example job
Using #!/bin/sh -l
as shebang in the slurm job script will cause the failure of some biocontainer modules. Please use #!/bin/bash
instead.
To run rochpcg on our clusters:
#!/bin/bash
#SBATCH -A gpu
#SBATCH -t 1:00:00
#SBATCH -N 1
#SBATCH -n 1
#SBATCH -c 8
#SBATCH --gpus-per-node=1
#SBATCH --job-name=rochpcg
#SBATCH --mail-type=FAIL,BEGIN,END
#SBATCH --error=%x-%J-%u.err
#SBATCH --output=%x-%J-%u.out
module --force purge
ml rocmcontainers rochpcg
rochpl
Link to section 'Description' of 'rochpl' Description
HPL, or High-Performance Linpack, is a benchmark which solves a uniformly random system of linear equations and reports floating-point execution rate. This container implements the HPL benchmark on top of AMDs ROCm platform.
Link to section 'Versions' of 'rochpl' Versions
- Bell: 5.0.5
- Negishi: 5.0.5
Link to section 'Module' of 'rochpl' Module
You can load the modules by:
module load rocmcontainers
module load rochpl
Link to section 'Example job' of 'rochpl' Example job
Using #!/bin/sh -l
as shebang in the slurm job script will cause the failure of some biocontainer modules. Please use #!/bin/bash
instead.
To run rochpl on our clusters:
#!/bin/bash
#SBATCH -A gpu
#SBATCH -t 1:00:00
#SBATCH -N 1
#SBATCH -n 1
#SBATCH -c 8
#SBATCH --gpus-per-node=1
#SBATCH --job-name=rochpl
#SBATCH --mail-type=FAIL,BEGIN,END
#SBATCH --error=%x-%J-%u.err
#SBATCH --output=%x-%J-%u.out
module --force purge
ml rocmcontainers rochpl
specfem3d
Link to section 'Description' of 'specfem3d' Description
SPECFEM3D Cartesian simulates acoustic fluid, elastic solid, coupled acoustic/elastic, poroelastic or seismic wave propagation in any type of conforming mesh of hexahedra structured or not. It can, for instance, model seismic waves propagating in sedimentary basins or any other regional geological model following earthquakes. It can also be used for non-destructive testing or for ocean acoustics.
Link to section 'Versions' of 'specfem3d' Versions
- Bell: 20201122--h9c0626d1
- Negishi: 20201122--h9c0626d1
Link to section 'Module' of 'specfem3d' Module
You can load the modules by:
module load rocmcontainers
module load specfem3d
Link to section 'Example job' of 'specfem3d' Example job
Using #!/bin/sh -l
as shebang in the slurm job script will cause the failure of some biocontainer modules. Please use #!/bin/bash
instead.
To run specfem3d on our clusters:
#!/bin/bash
#SBATCH -A gpu
#SBATCH -t 1:00:00
#SBATCH -N 1
#SBATCH -n 1
#SBATCH -c 8
#SBATCH --gpus-per-node=1
#SBATCH --job-name=specfem3d
#SBATCH --mail-type=FAIL,BEGIN,END
#SBATCH --error=%x-%J-%u.err
#SBATCH --output=%x-%J-%u.out
module --force purge
ml rocmcontainers specfem3d
specfem3d_globe
Link to section 'Description' of 'specfem3d_globe' Description
SPECFEM3D Globe simulates global and regional continental-scale seismic wave propagation.
Link to section 'Versions' of 'specfem3d_globe' Versions
- Bell: 20210322--h1ee10977
- Negishi: 20210322--h1ee10977
Link to section 'Module' of 'specfem3d_globe' Module
You can load the modules by:
module load rocmcontainers
module load specfem3d_globe
Link to section 'Example job' of 'specfem3d_globe' Example job
Using #!/bin/sh -l
as shebang in the slurm job script will cause the failure of some biocontainer modules. Please use #!/bin/bash
instead.
To run specfem3d_globe on our clusters:
#!/bin/bash
#SBATCH -A gpu
#SBATCH -t 1:00:00
#SBATCH -N 1
#SBATCH -n 1
#SBATCH -c 8
#SBATCH --gpus-per-node=1
#SBATCH --job-name=specfem3d_globe
#SBATCH --mail-type=FAIL,BEGIN,END
#SBATCH --error=%x-%J-%u.err
#SBATCH --output=%x-%J-%u.out
module --force purge
ml rocmcontainers specfem3d_globe
tensorflow
Link to section 'Description' of 'tensorflow' Description
TensorFlow is an end-to-end open source platform for machine learning.
Link to section 'Versions' of 'tensorflow' Versions
- Bell: 2.5-rocm4.2-dev, 2.7-rocm5.0-dev
- Negishi: 2.5-rocm4.2-dev, 2.7-rocm5.0-dev
Link to section 'Module' of 'tensorflow' Module
You can load the modules by:
module load rocmcontainers
module load tensorflow
Link to section 'Example job' of 'tensorflow' Example job
Using #!/bin/sh -l
as shebang in the slurm job script will cause the failure of some biocontainer modules. Please use #!/bin/bash
instead.
To run tensorflow on our clusters:
#!/bin/bash
#SBATCH -A gpu
#SBATCH -t 1:00:00
#SBATCH -N 1
#SBATCH -n 1
#SBATCH -c 8
#SBATCH --gpus-per-node=1
#SBATCH --job-name=tensorflow
#SBATCH --mail-type=FAIL,BEGIN,END
#SBATCH --error=%x-%J-%u.err
#SBATCH --output=%x-%J-%u.out
module --force purge
ml rocmcontainers tensorflow
This example demonstrates how to run Tensorflow on AMD GPUs with rocmcontainers modules.
First, prepare the matrix multiplication example from Tensorflow documentation:
# filename: matrixmult.py
import tensorflow as tf
# Log device placement
print("Num GPUs Available: ", len(tf.config.list_physical_devices('GPU')))
tf.debugging.set_log_device_placement(True)
# Create some tensors
a = tf.constant([[1.0, 2.0, 3.0], [4.0, 5.0, 6.0]])
b = tf.constant([[1.0, 2.0], [3.0, 4.0], [5.0, 6.0]])
c = tf.matmul(a, b)
print(c)
Submit a Slurm job, making sure to request GPU-enabled queue and desired number of GPUs. For illustration purpose, the following example shows an interactive job submission, asking for one node (${resource.nodecores} cores) in the "gpu" account with and two GPUs for 6 hours, but the same applies to your production batch jobs as well:
sinteractive -A gpu -N 1 -n ${resource.nodecores} -t 6:00:00 --gres=gpu:2
salloc: Granted job allocation 5401130
salloc: Waiting for resource configuration
salloc: Nodes ${resource.hostname}-g000 are ready for job
Inside the job, load necessary modules:
module load rocmcontainers
module load tensorflow/2.5-rocm4.2-dev
And run the application as usual:
python matrixmult.py
Num GPUs Available: 2
[...]
2021-09-02 21:07:34.087607: I tensorflow/core/common_runtime/gpu/gpu_device.cc:1418] Created TensorFlow device (/job:localhost/replica:0/task:0/device:GPU:0 with 32252 MB memory) -> physical GPU (device: 0, name: Vega 20, pci bus id: 0000:83:00.0)
[...]
2021-09-02 21:07:36.265167: I tensorflow/core/common_runtime/eager/execute.cc:733] Executing op MatMul in device /job:localhost/replica:0/task:0/device:GPU:0
2021-09-02 21:07:36.266755: I tensorflow/stream_executor/platform/default/dso_loader.cc:53] Successfully opened dynamic library librocblas.so
tf.Tensor(
[[22. 28.]
[49. 64.]], shape=(2, 2), dtype=float32)
FAQs
Frequently Asked Questions about applications.
AMD GPUs in Bell and Negishi
AMD presents a serious rival for Nvidia when it comes to HPC, but Nvidia still maintains the edge for AI acceleration. Nvidia has a more mature programming framework in CUDA. But with AMD's accelerated computing framework (ROCm), AMD is catching up.
Several nodes of Bell and Negishi are equipped with AMD GPUs. To take advantage of AMD GPU acceleration, applications need to be compatible with AMD GPUs, and built with ROCm. Below are a few usage of AMD GPUs in Bell/Negishi.
Link to section 'PyTorch' of 'AMD GPUs in Bell and Negishi' PyTorch
Users can need to follow PyTorch installation guide(https://pytorch.org/get-started/locally/) to install PyTorch with AMD GPU support:
module purge
module load rocm conda
conda create -n torch-rocm
conda activate torch-rocm
conda install pytorch torchvision torchaudio -c pytorch
Once the environment is created, you may add the following commands in your job script to activate the environment:
module purge
module load rocm conda
conda activate torch-rocm
Using constraint to request specific GPUs
Gilbreth has heterogeneous hardware comprising of Nvidia V100, A100, A10, and A30 GPUs in separate sub-clusters. You can run sfeatures
to check the specifications of different Gilbreth nodes:
NODELIST CPUS MEMORY AVAIL_FEATURES GRES
gilbreth-b[000-015] 24 190000 B,b,A30,a30 gpu:3
gilbreth-c[000-002] 20 760000 C,c,V100,v100 gpu:4
gilbreth-d[000-007] 16 190000 D,d,A30,a30 gpu:3
gilbreth-e[000-015] 16 190000 E,e,V100,v100 gpu:2
gilbreth-f[000-004] 40 190000 F,f,V100,v100 gpu:2
gilbreth-g[000-011] 128 510000 G,g,A100,a100,A100-40GB,a100-40gb gpu:2
gilbreth-h[000-015] 32 512000 H,h,A10,a10 gpu:3
gilbreth-i[000-004] 32 512000 I,i,A100,a100,A100-80GB,a100-80gb gpu:2
gilbreth-j[000-001] 128 1020000 J,j,A100,a100,A100-80GB,a100-80gb gpu:4
To run your jobs in specific nodes, you can use -C, --constraint
to specify the features. Below are a few examples:
#SBATCH --constraint 'E|F' ## request E or F nodes
#SBATCH --constraint A100 ## request A100 GPU
#SBATCH -C "v100|a100|a30" ## request v100, a100 or a30
MPI
SLURM can run an MPI program with the srun
command. The number of processes is requested with the -n
option. If you do not specify the -n
option, it will default to the total number of processor cores you request from SLURM.
If the code is built with OpenMPI, it can be run with a simple srun -n
command. If it is built with Intel IMPI, then you also need to add the --mpi=pmi2
option: srun --mpi=pmi2 -n 32 ./mpi_hello
.
R
Link to section 'Setting Up R Preferences with .Rprofile' of 'R' Setting Up R Preferences with .Rprofile
Different clusters have different hardware and softwares. So, if you have access to multiple clusters, you must install your R packages separately for each cluster. Each cluster has multiple versions of R and packages installed with one version of R may not work with another version of R. So, libraries for each R version must be installed in a separate directory. You can define the directory where your R packages will be installed using the environment variable R_LIBS_USER
.
For your convenience, a sample .Rprofile example file is provided that can be downloaded to your cluster account and renamed into /.Rprofile (or appended to one) to customize your installation preferences. Detailed instructions:
curl -#LO https://www.rcac.purdue.edu/files/knowledge/run/examples/apps/r/Rprofile_example
mv -ib Rprofile_example ~/.Rprofile
The above installation step needs to be done only once on each of the clusters you have access to. Now load the R module and run R to confirm the unique libPaths:
module load r/4.2.2
R
R> .libPaths()
[1] "/home/zhan4429/R/bell/4.2.2-gcc-9.3.0-xxbnk6s"
[2] "/apps/spack/bell/apps/r/4.2.2-gcc-9.3.0-xxbnk6s/rlib/R/library"
Link to section 'Challenging packages' of 'R' Challenging packages
Below are packages users may have difficulty in installation.
Link to section 'nloptr' of 'R' nloptr
In Bell, the installation may fail due to the default cmake
version is too old. The solution is easy, users just need to load the newer versions of cmake
:
module load cmake/3.20.6
module load r
Rscript -e 'install.packages("nloptr")'
In Brown or other older clusters, because our system's cmake
and gcc
compilers are old, we may not be able to install the latest version of nloptr
. The walkaround is that users can install the older versions of nloptr
:
module load r
R
> myrepos = c("https://cran.case.edu")
> install.packages("devtools", repos = myrepos)
> library(devtools)
> install_version("nloptr", version = "> 1.2.2, < 2.0.0", repos = myrepos)
Link to section 'Error: C++17 standard requested but CXX17 is not defined' of 'R' Error: C++17 standard requested but CXX17 is not defined
When users want to install some packages, such as colourvalues
, the installation may fail due to Error: C++17 standard requested but CXX17 is not defined
. Please follow the below command to fix it:
module load r
module spider gcc
module load gcc/xxx ## the lateste gcc is recommended
mkdir -p ~/.R
echo 'CXX17 = g++ -std=gnu++17 -fPIC' > ~/.R/Makevars
R
> install.packages("xxxx")
Link to section 'RCurl' of 'R' RCurl
Some R packages rely on curl. When you install these packages such as RCurl, you may see such error: checking for curl-config... no Cannot find curl-config
To install such packages, you need to load the curl module:
module load curl
module load r
R
> install.packages("RCurl")
Link to section 'raster, stars and sf' of 'R' raster, stars and sf
These R packages have some dependencies. To install them, users will need to load several modules. Note that these modules have multiple versions, and the latest version is recommended. However, the default version may not be the latest version. To check the latest version, please run module spider XX
.
module spider gdal
module spider geos
module spider proj
module spider sqlite
module load gdal/XXX geos/XXX proj/XXX sqlite/XXX ## XXX is the version to use. The latest version is recommended.
module load r/XXX
R
> install.packages("raster")
install.packages("stars")
install.packages("sf")
Many-Task Computing using HyperShell
HyperShell is an elegant, cross-platform, high-throughput computing utility for processing shell commands over a distributed, asynchronous queue. It is a highly scalable workflow automation tool for many-task scenarios.
Several tools offer similar functionality but not all together in a single tool with the user ergonomics we provide. Novel design elements include but are not limited to (1) cross-platform, (2) client-server design, (3) staggered launch for large scales, (4) persistent hosting of the server, and optionally (5) a database in-the-loop for persisting task metadata and automated retries.
HyperShell is pure Python and is tested on Linux, macOS, and Windows 10 in Python 3.9 and 3.10 environments. The server and client don’t even need to use the same platform simultaneously.
Detailed usage can be found here: https://hypershell.readthedocs.io/en/latest/
Link to section 'Cluster' of 'Many-Task Computing using HyperShell' Cluster
Start the cluster either locally or with remote clients over ssh or a custom launcher. This mode should be the most common entry-point for general usage. It fully encompasses all of the different agents in the system in a concise workflow.
The input source for tasks is file-like, either a local path, or from stdin if no argument is given. The command-line tasks are pulled in and either directly published to a distributed queue (see --no-db) or committed to a database first before being scheduled later.
For large, long running workflows, it might be a good idea to configure a database and run an initial submit job to populate the database, and then run the cluster with --restart and no input FILE. If the cluster is interrupted for whatever reason it can gracefully restart where it left off.
A simple user case is that users just need to privde a taskfile containing commands/tasks. Each line is one command/task. Below is a batch jobscript that can used in ACCESS Anvil cluster:
#!/bin/bash
#SBATCH -A AllocationName
#SBATCH -N 1
#SBATCH -n 1
#SBATCH -c 64
#SBATCH -p shared
#SBATCH --time=4:00:00
#SBATCH --job-name=trim-galore
#SBATCH --error=%x-%J-%u.err
#SBATCH --output=%x-%J-%u.out
#SBATCH --mail-type=ALL
module load hyper-shell
hs cluster Taskfile.txt \
-o trim_Taskfile.output \
-f trim_Taskfile.failed \
-N 16 ## Number of tasks to run simultaneously
Below are contents of Taskfile.txt that I want to run a bioinformatics application called Trim-galore
:
trim_galore ---fastqc -j 4 -q 25 --paired seq1_1.fastq seq1_2.fastq -o trim_out && echo task1 success
trim_galore ---fastqc -j 4 -q 25 --paired seq2_1.fastq seq2_2.fastq -o trim_out && echo task2 success
trim_galore ---fastqc -j 4 -q 25 --paired seq3_1.fastq seq3_1.fastq -o trim_out && echo task3 success
trim_galore ---fastqc -j 4 -q 25 --paired seq4_1.fastq seq4_2.fastq -o trim_out && echo task4 success
trim_galore ---fastqc -j 4 -q 25 --paired seq5_1.fastq seq5_2.fastq -o trim_out && echo task5 success
trim-galore ---fastqc -j 4 -q 25 --paired seq6_1.fastq seq6_2.fastq -o trim_out && echo task6 success
In the slurm jobscript, we request 64 CPUs and 16 tasks (-N 3) to run simultaneously. Each trim_galore
task will use 4 CPUs (-j 4). So that we can efficiently use all 64 CPUs. The task1-16 will run when the hypershell job starts. If any of the first 16 tasks completes, task17 will start, and so on until all tasks complete.
You may notice that in task6, there is a typo. The command should be trim_galore
instead of trim-galore
. So this taks will fail. Since we used -f trim_Taskfile.failed
in the hypershell command, task6 will be saved to trim_Taskfile.failed
. This can help you track which tasks are successful and which ones fail.
Julia package installation
Users do not have write permission to the default julia package installation destination. However, users can install packages into home directory under ~/.julia
.
Users can side step this by explicitly defining where to put julia packages:
$ export JULIA_DEPOT_PATH=$HOME/.julia
$ julia -e 'using Pkg; Pkg.add("PackageName")'
Jupyter kernel creation
JupyterLab is the latest web-based interactive development environment for notebooks, code, and data. Its flexible interface allows users to configure and arrange workflows in data science, scientific computing, computational journalism, and machine learning. The Jupyter Notebook is the original web application for creating and sharing computational documents.
Both JupyterLab and Jupyter Notebook are supported on Open OnDemand of RCAC clusters. This tutorial will introduce how to create a personal kernal using the terminal, then run python codes on Open OnDemand Jupyter with the newly created kernel.
To facilitate the process, we provide a script conda-env-mod
that generates a module file for an environment, as well as an optional Jupyter kernel to use this environment in Jupyter.
Link to section 'Step1: Load the anaconda module' of 'Jupyter kernel creation' Step1: Load the anaconda module
You must load one of the anaconda modules in order to use this script:
module spider anaconda
module load anaconda/xxxx ## choose the anaconda and python version you want to use
Link to section 'Step 2: Create a conda environment' of 'Jupyter kernel creation' Step 2: Create a conda environment
By default, conda-env-mod
will only create the environment and a module file(no Jupyter kernel). If you plan to use your environment in a Jupyter, you need to append a --jupyter
flag:
conda-env-mod create -n mypackages --jupyter
Link to section 'Step 3: Load the conda environment' of 'Jupyter kernel creation' Step 3: Load the conda environment
The following instructions assume that you have used conda-env-mod
script to create an environment named mypackages
:
module load use.own
module load conda-env/mypackages-py3.8.5 # py3.8.5 is associated with the python in the loaded anaconda module.
Link to section 'Step 4: Install packages' of 'Jupyter kernel creation' Step 4: Install packages
Now you can install custom packages in the environment using either conda install
or pip install
:
conda install Package1
pip install Package2
Link to section 'Step 5: Open OnDemand Jupyter' of 'Jupyter kernel creation' Step 5: Open OnDemand Jupyter
In Jupyter Lab or Jupter Notebook of Open OnDemand, you can create a new notebook with the newly created kernel.
Link to section 'Example: CellRank' of 'Jupyter kernel creation' Example: CellRank
CellRank is a toolkit to uncover cellular dynamics based on Markov state modeling of single-cell data (https://github.com/theislab/cellrank).
Create a conda environment with Jupyter kernel:
module load anaconda/2020.11-py38
conda-env-mod create -n cellrank --jupyter
Next we can load the module, and install CellRank:
module load use.own
module load conda-env/cellrank-py3.8.5
conda install -c conda-forge -c bioconda cellrank
Select the newly created kernel Python(My cellrank Kernel)
in Jupyter Notebook or Jupyter Lab.
Now we can use Python(My cellrank Kernel)
to run scRNAseq analysis.
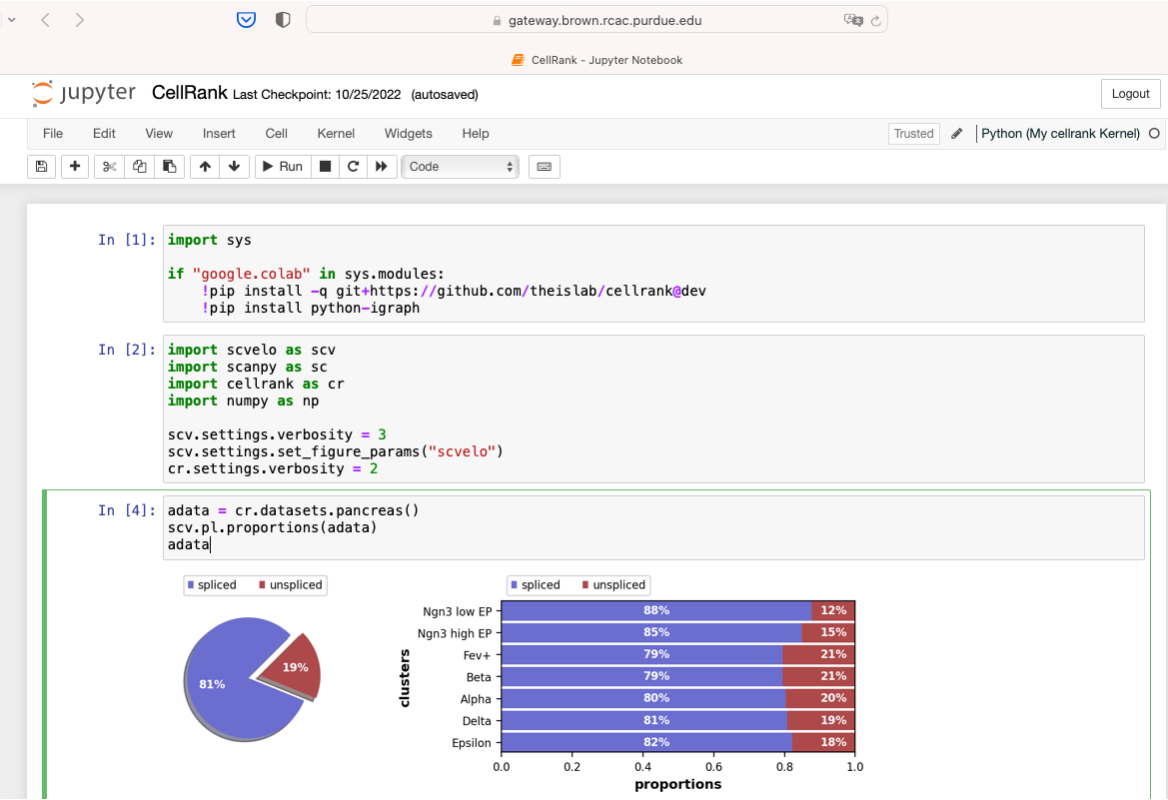
Disk quota exhausted
On RCAC clusters, each user's $HOME
only has a quota of 25GB. $HOME
is the ideal place to store important scripts, executables, but it should not be used to run jobs and store data of large size. If the quota of $HOME
is exhausted, it will have a big affect and users even cannot submit or run jobs.
Link to section 'ncdu' of 'Disk quota exhausted' ncdu
RCAC has deployed a nice and easy tool called ncdu
to help users check sizes of files and subdirectories in a specific directory. For example, if users want to check which files/folders occupy how much disk quota of $HOME
, you can easily run ncdu
like this:
$ ncdu $HOME
You will see results similar to this:
ncdu 1.17 ~ Use the arrow keys to navigate, press ? for help
--- /home/zhan4429 -------------------------------------------------------------
3.3 GiB [###########] /.singularity
1.4 GiB [#### ] /myapps
1.1 GiB [### ] /Fidelity
776.8 MiB [## ] /projects
240.9 MiB [ ] /.local
177.9 MiB [ ] /R
174.9 MiB [ ] /git
113.8 MiB [ ] /Downloads
107.4 MiB [ ] /.vscode-server
101.2 MiB [ ] /svn
72.4 MiB [ ] /spack
35.4 MiB [ ] cpu-percent.log
35.0 MiB [ ] /.celltypist
33.9 MiB [ ] /Desktop
32.9 MiB [ ] /alphafold
There are a few hidden directory can occpuy a lot of disk space, including ~/.cache
, ~/.conda/pkgs
, and .singularity/cache
. Since these folders just contain cache files, it is safe to delete them.
glibc
When you try to install some software on clusters, the installation may fail due to reasons similar to this: "XX requires libc version 2.27 or at least 2.23, but the current version is 2.17". Unfortunately, glibc is bundled with the operation system, and we cannot update it for you.
To check the version of glibc on our cluster, you can run the below command:
$ ldd --version
ldd (GNU libc) 2.17
Copyright (C) 2012 Free Software Foundation, Inc.
This is free software; see the source for copying conditions. There is NO
warranty; not even for MERCHANTABILITY or FITNESS FOR A PARTICULAR PURPOSE.
Written by Roland McGrath and Ulrich Drepper.
Link to section 'Workaround' of 'glibc' Workaround
- Singularity containers
- Install an older version of your software
Large memory jobs
One bonus for purchasing regular nodes of HPC clusters is that users will have access to large memory nodes of 1TB. As memory is evenly distributed among all the available cores, so each core is tied with about 8GB(1024 GB/128 cores) memory. This can be very useful for jobs with a large data set. Using the command slist
, users can see that the queue name of large memory nodes is highmem
and the max walltime is 24 hours
. Note that the large memory nodes are shared by all users, so we will not extend walltime for users.
user@cluster-fe04:~ $ slist
Current Number of Cores Node
Account Total Queue Run Free Max Walltime Type
============== ================================= ============== ======
debug 256 249 0 256 00:30:00 A
gpu 512 128 256 256 G
highmem 1024 368 590 434 1-00:00:00 B
multigpu 48 0 0 48 G
rcac-b 1024 0 0 1024 14-00:00:00 B
standby 46976 1600 4563 11552 04:00:00 A
Link to section 'Example script for large memory jobs' of 'Large memory jobs' Example script for large memory jobs
#!/bin/bash
#SBATCH -A highmem
#SBATCH -t 12:00:00
#SBATCH -N 1
#SBATCH -n 12
#SBATCH --job-name=alphafold
#SBATCH --mail-type=FAIL,BEGIN,END
#SBATCH --error=%x-%J-%u.err
#SBATCH --output=%x-%J-%u.out
module --force purge
ml biocontainers alphafold/2.3.1
run_alphafold.sh --flagfile=full_db_20230311.ff \
--fasta_paths=sample.fasta --max_template_date=2022-02-01 \
--output_dir=af2_full_out --model_preset=monomer \
--use_gpu_relax=False
Custom Software Installation
Please review Software Installation 101 for assistance installing software that isn't included in our standard module portfolioStorage
-Data Depot User Guide
The Data Depot is a high-capacity, fast, reliable and secure data storage service designed, configured and operated for the needs of Purdue researchers in any field and shareable with both on-campus and off-campus collaborators.
Data Depot Overview
As with the community clusters, research labs will be able to easily purchase capacity in the Data Depot through the Data Depot Purchase page on this site. For more information, please contact us.
Link to section 'Data Depot Features' of 'Data Depot Overview' Data Depot Features
The Data Depot offers research groups in need of centralized data storage unique features and benefits. View or download our detailed features guide below:
-
Available
To any Purdue research group as a purchase in increments of 1 TB at a competitive annual price or you may request a 100 GB trial space free of charge. Participation in the Community Cluster program is not required.
-
Accessible
- As a Windows or Mac OS X network drive on personal and lab computers on campus.
- Directly on Community Cluster nodes.
- From other universities or labs through Globus.
-
Capable
The Data Depot facilitates joint work on shared files across your research group, avoiding the need for numerous copies of datasets across individuals' home or scratch directories. It is an ideal place to store group applications, tools, scripts, and documents.
-
Controllable Access
Access management is under your direct control. Unix groups can be created for your group and staff can assist you in setting appropriate permissions to allow exactly the access you want and prevent any you do not. Easily manage who has access through a simple web application — the same application used to manage access to Community Cluster queues.
-
Data Retention
All data kept in the Data Depot remains owned by the research group's lead faculty. When researchers or students leave your group, any files left in their home directories may become difficult to recover. Files kept in Data Depot remain with the research group, unaffected by turnover, and could head off potentially difficult disputes.
-
Never Purged
The Data Depot is never subject to purging.
-
Reliable
The Data Depot is redundant and protected against hardware failures and accidental deletion. All data is mirrored at two different sites on campus to provide for greater reliability and to protect against physical disasters.
-
Restricted Data
The Data Depot is suitable for non-HIPAA human subjects data. See the Data Depot FAQ for a data security statement for your IRB documentation. The Data Depot is not approved for regulated data, including HIPAA, ePHI, FISMA, or ITAR data.
-
Available
To any Purdue research group as a purchase in increments of 1 TB at a competitive annual price or you may request a 100 GB trial space free of charge. Participation in the Community Cluster program is not required.
-
Accessible
- As a Windows or Mac OS X network drive on personal and lab computers on campus.
- Directly on Community Cluster nodes.
- From other universities or labs through Globus.
-
Capable
The Data Depot facilitates joint work on shared files across your research group, avoiding the need for numerous copies of datasets across individuals' home or scratch directories. It is an ideal place to store group applications, tools, scripts, and documents.
-
Controllable Access
Access management is under your direct control. Unix groups can be created for your group and staff can assist you in setting appropriate permissions to allow exactly the access you want and prevent any you do not. Easily manage who has access through a simple web application — the same application used to manage access to Community Cluster queues.
-
Data Retention
All data kept in the Data Depot remains owned by the research group's lead faculty. When researchers or students leave your group, any files left in their home directories may become difficult to recover. Files kept in Data Depot remain with the research group, unaffected by turnover, and could head off potentially difficult disputes.
-
Never Purged
The Data Depot is never subject to purging.
-
Reliable
The Data Depot is redundant and protected against hardware failures and accidental deletion. All data is mirrored at two different sites on campus to provide for greater reliability and to protect against physical disasters.
-
Restricted Data
The Data Depot is suitable for non-HIPAA human subjects data. See the Data Depot FAQ for a data security statement for your IRB documentation. The Data Depot is not approved for regulated data, including HIPAA, ePHI, FISMA, or ITAR data.
Link to section 'Data Depot Hardware Details' of 'Data Depot Overview' Data Depot Hardware Details
The Data Depot uses an enterprise-class GPFS storage solution with an initial total capacity of over 2 PB. This storage is redundant and reliable, features regular snapshots, and is globally available on all RCAC systems. The Data Depot is non-purged space suitable for tasks such as sharing data, editing files, developing and building software, and many other uses. Built on Data Direct Networks' SFA12k storage platform, the Data Depot has redundant storage arrays in multiple campus datacenters for maximum availability.
While the Data Depot will scale well for most uses, it is recommended to continue using each cluster's parallel scratch filesystem for use as high-performance working space (scratch) for running jobs.
File Storage and Transfer
Learn more about file storage transfer for Data Depot.
Link to section 'Archive and Compression' of 'Archive and Compression' Archive and Compression
There are several options for archiving and compressing groups of files or directories. The mostly commonly used options are:
Link to section 'tar' of 'Archive and Compression' tar
See the official documentation for tar for more information.
Saves many files together into a single archive file, and restores individual files from the archive. Includes automatic archive compression/decompression options and special features for incremental and full backups.
Examples:
(list contents of archive somefile.tar)
$ tar tvf somefile.tar
(extract contents of somefile.tar)
$ tar xvf somefile.tar
(extract contents of gzipped archive somefile.tar.gz)
$ tar xzvf somefile.tar.gz
(extract contents of bzip2 archive somefile.tar.bz2)
$ tar xjvf somefile.tar.bz2
(archive all ".c" files in current directory into one archive file)
$ tar cvf somefile.tar *.c
(archive and gzip-compress all files in a directory into one archive file)
$ tar czvf somefile.tar.gz somedirectory/
(archive and bzip2-compress all files in a directory into one archive file)
$ tar cjvf somefile.tar.bz2 somedirectory/
Other arguments for tar can be explored by using the man tar command.
Link to section 'gzip' of 'Archive and Compression' gzip
The standard compression system for all GNU software.
Examples:
(compress file somefile - also removes uncompressed file)
$ gzip somefile
(uncompress file somefile.gz - also removes compressed file)
$ gunzip somefile.gz
Link to section 'bzip2' of 'Archive and Compression' bzip2
See the official documentation for bzip for more information.
Strong, lossless data compressor based on the Burrows-Wheeler transform. Stronger compression than gzip.
Examples:
(compress file somefile - also removes uncompressed file)
$ bzip2 somefile
(uncompress file somefile.bz2 - also removes compressed file)
$ bunzip2 somefile.bz2
There are several other, less commonly used, options available as well:
- zip
- 7zip
- xz
Link to section 'Sharing Files from Data Depot' of 'Sharing' Sharing Files from Data Depot
Data Depot supports several methods for file sharing. Use the links below to learn more about these methods.
Link to section 'Sharing Data with Globus' of 'Globus' Sharing Data with Globus
Data on any RCAC resource can be shared with other users within Purdue or with collaborators at other institutions. Globus allows convenient sharing of data with outside collaborators. Data can be shared with collaborators' personal computers or directly with many other computing resources at other institutions.
To share files, login to https://transfer.rcac.purdue.edu, navigate to the endpoint (collection) of your choice, and follow instructions as described in Globus documentation on how to share data:
See also RCAC Globus presentation.
Link to section 'Sharing static content from your Data Depot space via the WWW' of 'WWW' Sharing static content from your Data Depot space via the WWW
Your research group can easily share static files (images, data, HTML) from your depot space via the WWW.
- Contact support to set up a "www" folder in your Data Depot space.
- Copy any files that you wish to share via the WWW into your Data Depot space's "www" folder.
- For example, cp /path/to/image.jpg /depot/mylab/www/, where mylab is your research group name.
- or upload to smb://datadepot.rcac.purdue.edu/depot/mylab/www, where mylab is your research group name.
- Your file is now accessible via your web browser at the URL https://www.datadepot.rcac.purdue.edu/mylab/image.jpg
Note that it is not possible to run web sites, dynamic content, interpreters (PHP, Perl, Python), or CGI scripts from this web site.
File Transfer
Data Depot supports several methods for file transfer. Use the links below to learn more about these methods.
Globus
Link to section 'Globus' of 'Globus' Globus
Globus, previously known as Globus Online, is a powerful and easy to use file transfer service for transferring files virtually anywhere. It works within RCAC's various research storage systems; it connects between RCAC and remote research sites running Globus; and it connects research systems to personal systems. You may use Globus to connect to your home, scratch, and Fortress storage directories. Since Globus is web-based, it works on any operating system that is connected to the internet. The Globus Personal client is available on Windows, Linux, and Mac OS X. It is primarily used as a graphical means of transfer but it can also be used over the command line.
Link to section 'Link to section 'Globus Web:' of 'Globus' Globus Web:' of 'Globus' Link to section 'Globus Web:' of 'Globus' Globus Web:
- Navigate to http://transfer.rcac.purdue.edu
- Click "Proceed" to log in with your Purdue Career Account.
- On your first login it will ask to make a connection to a Globus account. Accept the conditions.
- Now you are at the main screen. Click "File Transfer" which will bring you to a two-panel interface (if you only see one panel, you can use selector in the top-right corner to switch the view).
- You will need to select one collection and file path on one side as the source, and the second collection on the other as the destination. This can be one of several Purdue endpoints, or another University, or even your personal computer (see Personal Client section below).
The RCAC collections are as follows. A search for "Purdue" will give you several suggested results you can choose from, or you can give a more specific search.
- Home Directory storage: "Purdue Research Computing - Home Directories", however, you can start typing "Purdue" and "Home Directories" and it will suggest appropriate matches.
- Weber scratch storage: "Purdue Weber Cluster", however, you can start typing "Purdue" and "Weber and it will suggest appropriate matches. From here you will need to navigate into the first letter of your username, and then into your username.
- Research Data Depot: "Purdue Research Computing - Data Depot", a search for "Depot" should provide appropriate matches to choose from.
- Fortress: "Purdue Fortress HPSS Archive", a search for "Fortress" should provide appropriate matches to choose from.
From here, select a file or folder in either side of the two-pane window, and then use the arrows in the top-middle of the interface to instruct Globus to move files from one side to the other. You can transfer files in either direction. You will receive an email once the transfer is completed.
Link to section 'Link to section 'Globus Personal Client setup:' of 'Globus' Globus Personal Client setup:' of 'Globus' Link to section 'Globus Personal Client setup:' of 'Globus' Globus Personal Client setup:
Globus Connect Personal is a small software tool you can install to make your own computer a Globus endpoint on its own. It is useful if you need to transfer files via Globus to and from your computer directly.
- On the "Collections" page from earlier, click "Get Globus Connect Personal" or download a version for your operating system it from here: Globus Connect Personal
- Name this particular personal system and follow the setup prompts to create your Globus Connect Personal endpoint.
- Your personal system is now available as a collection within the Globus transfer interface.
Link to section 'Link to section 'Globus Command Line:' of 'Globus' Globus Command Line:' of 'Globus' Link to section 'Globus Command Line:' of 'Globus' Globus Command Line:
Globus supports command line interface, allowing advanced automation of your transfers.
To use the recommended standalone Globus CLI application (the globus command):
- First time use: issue the globus login command and follow instructions for initial login.
- Commands for interfacing with the CLI can be found via Using the Command Line Interface, as well as the Globus CLI Examples pages.
Link to section 'Link to section 'Sharing Data with Outside Collaborators' of 'Globus' Sharing Data with Outside Collaborators' of 'Globus' Link to section 'Sharing Data with Outside Collaborators' of 'Globus' Sharing Data with Outside Collaborators
Globus allows convenient sharing of data with outside collaborators. Data can be shared with collaborators' personal computers or directly with many other computing resources at other institutions. See the Globus documentation on how to share data:
For links to more information, please see Globus Support page and RCAC Globus presentation.
Windows Network Drive / SMB
SMB (Server Message Block), also known as CIFS, is an easy to use file transfer protocol that is useful for transferring files between RCAC systems and a desktop or laptop. You may use SMB to connect to your home, scratch, and Fortress storage directories. The SMB protocol is available on Windows, Linux, and Mac OS X. It is primarily used as a graphical means of transfer but it can also be used over the command line.
Note: to access Data Depot through SMB file sharing, you must be on a Purdue campus network or connected through VPN.
Link to section 'Windows:' of 'Windows Network Drive / SMB' Windows:
- Windows 7: Click Windows menu > Computer, then click Map Network Drive in the top bar
- Windows 8 & 10: Tap the Windows key, type computer, select This PC, click Computer > Map Network Drive in the top bar
- Windows 11: Tap the Windows key, type File Explorer, select This PC, click Computer > Map Network Drive in the top bar
- In the folder location enter the following information and click Finish:
- To access your Data Depot directory, enter \\datadepot.rcac.purdue.edu\depot\mylab where mylab is your research group name. Use your career account login name,
sername@purdue.edu
, and password when prompted. (You will not need to add ",push
" nor use your Purdue Duo client.)
- To access your Data Depot directory, enter \\datadepot.rcac.purdue.edu\depot\mylab where mylab is your research group name. Use your career account login name,
- Note: Use your career account login name and password when prompted. (You will not need to add "
,push
" nor use your Purdue Duo client.) - Your home or scratch directory should now be mounted as a drive in the Computer window.
Link to section 'Mac OS X:' of 'Windows Network Drive / SMB' Mac OS X:
- In the Finder, click Go > Connect to Server
- In the Server Address enter the following information and click Connect:
- To access your Data Depot directory, enter smb://datadepot.rcac.purdue.edu/depot/mylab where mylab is your research group name. Use your career account login name and password when prompted. (You will not need to add "
,push
" nor use your Purdue Duo client.)
- To access your Data Depot directory, enter smb://datadepot.rcac.purdue.edu/depot/mylab where mylab is your research group name. Use your career account login name and password when prompted. (You will not need to add "
- Note: Use your career account login name and password when prompted. (You will not need to add "
,push
" nor use your Purdue Duo client.) - Your home or scratch directory should now be mounted as a drive in the Computer window.
Link to section 'Linux:' of 'Windows Network Drive / SMB' Linux:
- There are several graphical methods to connect in Linux depending on your desktop environment. Once you find out how to connect to a network server on your desktop environment, choose the Samba/SMB protocol and adapt the information from the Mac OS X section to connect.
- If you would like access via samba on the command line you may install smbclient which will give you FTP-like access and can be used as shown below. For all the possible ways to connect look at the Mac OS X instructions.
smbclient //datadepot.rcac.purdue.edu/depot/ -U myusername cd mylab
- Note: Use your career account login name and password when prompted. (You will not need to add "
,push
" nor use your Purdue Duo client.)
Lost File Recovery
Data Depot is protected against accidental file deletion through a series of snapshots taken every night just after midnight. Each snapshot provides the state of your files at the time the snapshot was taken. It does so by storing only the files which have changed between snapshots. A file that has not changed between snapshots is only stored once but will appear in every snapshot. This is an efficient method of providing snapshots because the snapshot system does not have to store multiple copies of every file.
These snapshots are kept for a limited time at various intervals. RCAC keeps nightly snapshots for 7 days, weekly snapshots for 4 weeks, and monthly snapshots for 3 months. This means you will find snapshots from the last 7 nights, the last 4 Sundays, and the last 3 first of the months. Files are available going back between two and three months, depending on how long ago the last first of the month was. Snapshots beyond this are not kept.
Only files which have been saved during an overnight snapshot are recoverable. If you lose a file the same day you created it, the file is not recoverable because the snapshot system has not had a chance to save the file.
Snapshots are not a substitute for regular backups. It is the responsibility of the researchers to back up any important data to the Fortress Archive. Data Depot does protect against hardware failures or physical disasters through other means however these other means are also not substitutes for backups.
Files in scratch directories are not recoverable. Files in scratch directories are not backed up. If you accidentally delete a file, a disk crashes, or old files are purged, they cannot be restored.
Data Depot offers several ways for researchers to access snapshots of their files.
flost
If you know when you lost the file, the easiest way is to use the flost command. This tool is available from any RCAC resource. If you do not have access to a compute cluster, any Data Depot user may use an SSH client to connect to data.rcac.purdue.edu and run this command.
To run the tool you will need to specify the location where the lost file was with the -w argument:
$ flost -w /depot/mylab
Replace mylab with the name of your lab's Data Depot directory. If you know more specifically where the lost file was you may provide the full path to that directory.
This tool will prompt you for the date on which you lost the file or would like to recover the file from. If the tool finds an appropriate snapshot it will provide instructions on how to search for and recover the file.
If you are not sure what date you lost the file you may try entering different dates into the flost to try to find the file or you may also manually browse the snapshots as described below.
Manual Browsing
You may also search through the snapshots by hand on the Data Depot filesystem if you are not sure what date you lost the file or would like to browse by hand. Snapshots can be browsed from any RCAC resource. If you do not have access to a compute cluster, any Data Depot user may use an SSH client to connect to data.rcac.purdue.edu and browse from there. The snapshots are located at /depot/.snapshots
on these resources.
You can also mount the snapshot directory over Samba (or SMB, CIFS) on Windows or Mac OS X. Mount (or map) the snapshot directory in the same way as you did for your main Data Depot space substituting the server name and path for \\datadepot.rcac.purdue.edu\depot\.winsnaps
(Windows) or smb://datadepot.rcac.purdue.edu/depot/.winsnaps
(Mac OS X).
Once connected to the snapshot directory through SSH or Samba, you will see something similar to this:
SSH to data.rcac.purdue.edu | Samba mount on datadepot.rcac.purdue.edu |
---|---|
|
![]() |
Each of these directories is a snapshot of the entire Data Depot filesystem at the timestamp encoded into the directory name. The format for this timestamp is year, two digits for month, two digits for day, followed by the time of the day.
You may cd into any of these directories where you will find the entire Data Depot filesystem. Use cd to continue into your lab's Data Depot space and then you may browse the snapshot as normal.
If you are browsing these directories over a Samba network drive you can simply drag and drop the files over into your live Data Depot folder.
Once you find the file you are looking for, use cp to copy the file back into your lab's live Data Depot space. Do not attempt to modify files directly in the snapshot directories.
Windows
If you use Data Depot through "network drives" on Windows you may recover lost files directly from within Windows:
- Open the folder that contained the lost file.
- Right click inside the window and select "Properties".
- Click on the "Previous Versions" tab.
- A list of snapshots will be displayed.
- Select the snapshot from which you wish to restore.
- In the new window, locate the file you wish to restore.
- Simply drag the file or folder to their correct locations.
In the "Previous Versions" window the list contains two columns. The first column is the timestamp on which the snapshot was taken. The second column is the date on which the selected file or folder was last modified in that snapshot. This may give you some extra clues to which snapshot contains the version of the file you are looking for.
Mac OS X
Mac OS X does not provide any way to access the Data Depot snapshots directly. To access the snapshots there are two options: browse the snapshots by hand through a network drive mount or use an automated command-line based tool.
To browse the snapshots by hand, follow the directions outlined in the Manual Browsing section.
To use the automated command-line tool, log into a compute cluster or into the host data.rcac.purdue.edu (which is available to all Data Depot users) and use the flost tool. On Mac OS X you can use the built-in SSH terminal application to connect.
- Open the Applications folder from Finder.
- Navigate to the Utilities folder.
- Double click the Terminal application to open it.
- Type the following command when the terminal opens.
Replace myusername with your Purdue career account username and provide your password when prompted.$ ssh myusername@data.rcac.purdue.edu
Once logged in use the flost tool as described above. The tool will guide you through the process and show you the commands necessary to retrieve your lost file.
Access Permissions & Directories
Data Depot is very flexible in the access permission models and directory structures it can support. New spaces on Data Depot are given a default access model and directory structure at setup time. This can be modified as needed to support your workflows.
Information follows about the default structure and common access models.
Default Configuration
This is what a default configuration looks like for a research group called "mylab":
/depot/mylab/
+--apps/
|
+--data/
|
+--etc/
| +--bashrc
| +--cshrc
|
(other subdirectories)
The /depot/mylab/ directory is the main top-level directory for all your research group storage. All files are to be kept within one of the subdirectories of this, based on your specific access requirements. These subdirectories will be created after consulting with you as to exactly what you need.
By default,the following subdirectories, with the following access and use models, will be created. All of these details can be changed to suit the particular needs of your research group.
- data/
Intended for read and write use by a limited set of people chosen by the research group's managers.
Restricted to not be readable or writable by anyone else.
This is frequently used as an open space for storage of shared research data. - apps/
Intended for write use by a limited set of people chosen by the research group's managers.
Restricted to not be writable by anyone else.
Allows read and execute by anyone who has access to any cluster queues owned by the research group and anyone who has other file permissions granted by the research group (such as "data" access above).
This is frequently used as a space for central management of shared research applications. - etc/
Intended for write use by a limited set of people chosen by the research group's managers (by default, the same as for "apps" above).
Restricted to not be writable by anyone else.
Allows read and execute by anyone who has access to any cluster queues owned by the research group and anyone who has other file permissions granted by the research group (such as "data" access above).
This is frequently used as a space for central management of shared startup/login scripts, environment settings, aliases, etc. - etc/bashrc
etc/cshrc
Examples of group-wide shell startup files. Group members can source these from their own $HOME/.bashrc or $HOME/.cshrc files so they would automatically pick up changes to their environment needed to work with applications and data for the research group. There are more detailed instructions in these files on how to use them. - Additional subdirectories can be created as needed in the top and/or any of the lower levels. Just contact support and we will be happy to figure out what will work best for your needs.
Common Access Permission Scenarios
Depending on your research group's specific needs and preferred way of sharing, there are various permission models your Data Depot can be designed to reflect. Here are some common scenarios for access:
- "We have privately shared data within the group and some software for use only by us and a few collaborators."
Suggested implementation:Keep data in the data/ subdirectory and limit read and write access to select approved researchers.
Keep applications (if any) in the apps/ subdirectory and limit write access to your developers and/or application stewards.
Allow read/execute to apps/ by anyone in the larger research group with cluster queue access and approved collaborators. - "We have privately shared data within the group and some software which is needed by all cluster users (not just our group or known collaborators)."
Suggested implementation:Keep data in the data/ subdirectory and limit read and write access to select approved researchers.
Keep applications (if any) in the apps/ subdirectory and limit write access to your developers and/or application stewards.
Allow read/execute to apps/ by anyone at all by opening read/execute permissions on your base Data Depot directory. - "We have a few different projects and only the PI and respective project members should have any access to files for each project."
Suggested implementation:Create distinct subdirectories within your Data Depot base directory for each project and corresponding Unix groups for read/write access to each.
Approve specific researchers for read and write access to only the projects they are working on.
Many variants and combinations of the above are also possible covering the range from "very restrictive" to "mostly open" in terms of both read and write access to each subdirectory within your Data Depot space. Your lab can sit down with our staff and explain your specific needs in human terms, and then we can help you implement those requirements in actual permissions and groups. Once the initial configuration is done, you will then be able to easily add or remove access for your people. If your needs change, just let us know and we can accommodate your new requirements as well.
Storage Access Unix Groups
To enable a wide variety of access permissions, users are assigned one or more auxiliary Unix groups. It is the combination of this Unix group membership and the r/w/x permission bits on subdirectories that allow fine-tuning who can and can not do what within specific areas of your Data Depot. These Unix groups will generally closely match the name of your Data Depot root directory and the name of the subdirectory to which write access is being given. For example, write access to /depot/mylab/data/ is controlled by membership in the mylab-data Unix group.
There is also one Unix group which has the name of the base directory of your Data Depot, mylab. This group serves to limit read/execute access to your base /depot/mylab/ directory and also helps to define the read/execute permissions of some of the subdirectories within. This Unix group is composed of the union of the following:
- all members of your more specific Unix groups
- all users authorized to access any of your research group's cluster queues
- any other specific individuals you may have approved
Research group faculty and their designees may directly manage membership in these Unix groups, and by extension, the storage access permissions they grant, through the online web application.
Link to section 'Checking Your Group Membership' of 'Storage Access Unix Groups' Checking Your Group Membership
As a user you can check which groups you are a member of by issuing the groups
command while logged into any RCAC resource. You can also look on the website at https://www.rcac.purdue.edu/account/groups.
$ groups
mylab mylab-apps mylab-data
If you have recently been added to a group you need to log out and then back in again before the permissions changes take effect.
Frequently Asked Questions
Some common questions, errors, and problems are categorized below. Click the Expand Topics link in the upper right to see all entries at once. You can also use the search box above to search the user guide for any issues you are seeing.
About Data Depot
Frequently asked questions about Data Depot.
Can you remove me from the Data Depot mailing list?
Your subscription in the Data Depot mailing list is tied to your account on Data Depot. If you are no longer using your account on Data Depot, your account can be deleted from the My Accounts page. Hover over the resource you wish to remove yourself from and click the red 'X' button. Your account and mailing list subscription will be removed overnight. Be sure to make a copy of any data you wish to keep first.
What sort of performance should I expect to and from the Data Depot?
Access type | Large file, reading | Large file, writing | Many small files, reading | Many small files, writing |
---|---|---|---|---|
CIFS access, single client (GigE) | 102.1 MB/sec | 71.64 MB/sec | 12.43 MB/sec | 11.57 MB/sec |
Is the Data Depot just a file server?
The Data Depot is a suite of file service tools, specifically targeted at the needs of an academic research lab. More than just the file service infrastructure and hardware, the Data Depot also encompasses self-service access management, permissions control, and file sharing with Globus.
Do I need to do anything to my firewall to access Data Depot?
No firewall changes are needed to access Data Depot. However, to access data through Network Drives (i.e., CIFS, "Z: Drive"), you must be on a Purdue campus network or connected through VPN.
What is the best way to mount Data Depot in my lab?
You can mount your Data Depot space via Network Drives / CIFS using your Purdue Career Account. NFS access may also be possible depending on your lab's environment. If you require NFS access, contact support.
How do Data Depot, Fortress, and PURR relate to each other?
The Data Depot, Fortress, and PURR, are complementary parts of Purdue's infrastructure for working with research data. The Data Depot is designed for large, actively-used, persistent research data; Fortress is intended for long-term, archival storage of data and results; and PURR is for management, curation, and long-term preservation of research data.
Data
Frequently asked questions about data and data management.
Can I store Export-controlled data on Data Depot?
The Data Depot is not approved for storing data requiring Export control including ITAR, FISMA, DFAR-7012, NIST 800-171. Please contact the Export Control Office to discuss technology control plans and data storage appropriate for export controlled projects.
Can I store HIPAA data on Data Depot?
The Data Depot is not approved for storing data covered by HIPAA. Please contact the HIPAA Compliance Office to discuss HIPAA-compliant data storage.
What do I need to do in order to store non-HIPAA human subjects data in the Data Depot?
Use the following IRB-approved text in your IRB documentation when describing your data safeguards, substituting the PI's name for "PROFESSORNAME":
Only individuals specifically approved by PROFESSORNAME may access project data in the Research Data Depot. All membership in the PROFESSORNAME group is authorized by the project PI(s) and/or designees. Purdue University has network firewalls and other security devices to protect the Research Data Depot infrastructure from outside the campus.
Purdue Career accounts have password security policies that enforce age and quality requirements.
Auditing is enabled on Research Data Depot fileservers to track login attempts, maintain logs, and generate reports of access attempts.
Can I share data with outside collaborators?
Yes! Globus allows convenient sharing of data with outside collaborators. Data can be shared with collaborators' personal computers or directly with many other computing resources at other institutions. See the Globus documentation on how to share data:
Fortress User Guide
The Fortress system is a large, long-term, multi-tiered file caching and storage system utilizing both online disk and robotic tape drives.
Fortress Overview
Fortress uses a Spectra Logic TFinity robotic tape library with a capacity of over 200 PB.
Storage Subsystem | Current Capacity | Hardware |
---|---|---|
Disk Cache | Over 800 TB | 2 Seagate 5U84 Storage arrays |
Long-Term Storage | Over 200 PB | LTO-9 Robotic Tape Library |
All files stored on Fortress appear in at least two separate storage devices:
- One copy is permanently on tape.
- Recently used or files smaller than 100MB have their primary copy stored on a conventional spinning disk storage array (disk cache). Disk cache provides a rapid restore time.
Both primary and secondary copies of larger files reside on separate tape cartridges in the robotic tape library. After a period of inactivity, HPSS will migrate files from disk cache to tape.
Fortress writes two copies of every file either to two tapes, or to disk and a tape, to protect against medium errors. Unfortunately, Fortress does not automatically switch to the alternate copy when it has trouble accessing the primary. If it seems to be taking an extraordinary amount of time to retrieve a file (hours), please contact support. We can then investigate why it is taking so long. If it is an error on the primary copy, we will instruct Fortress to switch to the alternate copy as the primary and recreate a new alternate copy.
Link to section 'Fortress Storage Quota' of 'Fortress Overview' Fortress Storage Quota
There is currently no quota on Fortress disk use. Fortress users will receive a monthly email report showing your current Fortress usage.
Files belonging to deleted accounts will also be retained, but inaccessible except by special request after the accounts have been terminated. The files will be kept for no more than ten years or the usability of the media on which they are stored, whichever comes first.
Link to section 'Fortress File Recovery' of 'Fortress Overview' Fortress File Recovery
Data on Fortress is not backed up elsewhere in a traditional sense. New and modified files in the disk cache are migrated to tape within 30 minutes, and Fortress maintains two copies of every file on different media to protect against media failures, but there is no backup protecting against accidental deletions.
Link to section 'Fortress Regular Maintenance' of 'Fortress Overview' Fortress Regular Maintenance
Regular planned maintenance on Fortress is scheduled for the first Wednesday of every month, 8:00am to 12:00pm.
Accounts on Fortress
Link to section 'Obtaining an Account' of 'Accounts on Fortress' Obtaining an Account
All Purdue faculty, staff, and students participating in the Community Cluster program have access to Fortress along with their cluster nodes and scratch space. Your home directory on Fortress is the default directory that in which your archive files are stored and will appear as /home/myusername
, but this is not the same directory as your home directory on any other Purdue IT systems. Your home directory on Fortress is your long-term storage directory for all Purdue IT systems.
Research groups are assigned a group data storage space within Fortress with each Data Depot group space. Faculty should request a Data Depot trial to create a shared Fortress space for their research group. The actual location for the group space on Fortress is /group/mylab/ (replace mylab with your group name).
- Particularly in the case of group storage, please keep in mind that such spaces are, by design, accessible by others and should not be used to store private information such as grades, login credentials, or personal data.
Fortress sets no limits on the amount or number of files that you may store. However, there are several restrictions on the nature of files you may store:
- Many small files: Fortress is a tape archive and works best with a few, large files. Large sets of small files should be compressed into archives with utilities such as htar. Other technical limitations are detailed on the Fortress FAQs.
- Backing up individual or departmental computers. Fortress is intended to be a research data store and not a personal or enterprise backup solution.
Additionally, while Fortress access is included with RCAC services, storing more than 1 PB of data may incur a cost recovery charge.
Link to section 'Outside Collaborators' of 'Accounts on Fortress' Outside Collaborators
Your Departmental Business Office can submit a Request for Privileges (R4P) to provide access to collaborators outside Purdue, including recent graduates.
Link to section 'Login & Keytabs' of 'Accounts on Fortress' Login & Keytabs
It is not possible to login directly to Fortress via SSH or SCP. You may access your files there efficiently using HSI, HTAR, or SFTP. Windows Network Drive/SMB access is possible, though with significant performance loss.
A keytab file is required to log into Fortress via HSI or HTAR. However, all RCAC systems may access Fortress without any keytab preparation. If for some reason you lose your keytab, you may easily regenerate one on any RCAC system by running the command fortresskey.
However, to access Fortress from a personal or departmental computer, you will need to first copy your keytab file to the computer you wish to use. This keytab can be found in your research home directory, within the hidden subdirectory named ".private" as the file "hpss.unix.keytab" (~/.private/hpss.unix.keytab). This keytab will allow you to access HPSS services without needing to type a password and will remain valid for 90 days. Your keytab on RCAC systems will automatically be regenerated after this time, and you will need to re-copy the new keytab file to any other computers you use to directly access Fortress then.
If you do not have an account on any RCAC systems other than Fortress, you will need to generate a keytab file using the web interface:
- Web Form to Generate New Fortress Keytab
Warning: Using this invalidates your existing keytab on RCAC systems in the
~/.private/hpss.unix.keytab
file.
File Storage and Transfer
Learn more about file storage transfer for Fortress.
Your home directory on Fortress is the default directory that in which your archive files are stored.
On Fortress, your home directory will appear as /home/myusername
, but this is not the same directory as your home directory on any other Purdue IT systems. Your home directory on Fortress is your long-term storage directory for all Purdue IT systems.
The following link will take you to more information about transferring files in and out of Fortress.
Link to section 'Sharing Files from Fortress' of 'Sharing' Sharing Files from Fortress
Fortress supports several methods for file sharing. Use the links below to learn more about these methods.
Link to section 'Sharing Data with Globus' of 'Globus' Sharing Data with Globus
Data on any RCAC resource can be shared with other users within Purdue or with collaborators at other institutions. Globus allows convenient sharing of data with outside collaborators. Data can be shared with collaborators' personal computers or directly with many other computing resources at other institutions.
To share files, login to https://transfer.rcac.purdue.edu, navigate to the endpoint (collection) of your choice, and follow instructions as described in Globus documentation on how to share data:
See also RCAC Globus presentation.
File Transfer
Fortress supports several methods for file transfer. Use the links below to learn more about these methods.
FTP / SFTP
FTP is not supported because it does not allow for secure transmission of data. Use SFTP instead, as described below.
SFTP (Secure File Transfer Protocol) is a reliable way of transferring files between two machines. SFTP is available as a protocol choice in some graphical file transfer programs and also as a command-line program on most Linux, Unix, and Mac OS X systems. SFTP has more features than SCP and allows for other operations on remote files, remote directory listing, and resuming interrupted transfers. Command-line SFTP cannot recursively copy directory contents; to do so, try using a graphical SFTP client.
Note: to access Fortress through SFTP, you must be on a Purdue campus network or connected through VPN.
To transfer files to or from Fortress, your client should connect to the server name 'sftp.fortress.rcac.purdue.edu'.
Command-line usage:
$ sftp myusername@sftp.fortress.rcac.purdue.edu
(to the Fortress system from a local computer)
sftp> put sourcefile somedir/destinationfile
sftp> put -P sourcefile somedir/
(from the Fortress system to a local computer)
sftp> get sourcefile somedir/destinationfile
sftp> get -P sourcefile somedir/
sftp> exit
The -P flag is optional. When used, it will cause the transfer to preserve file attributes and permissions.
Linux / Solaris / AIX / HP-UX / Unix:
- The "sftp" command-line program should already be installed.
Microsoft Windows:
- MobaXterm
Free, full-featured, graphical Windows SSH, SCP, and SFTP client.
Mac OS X:
- The "sftp" command-line program should already be installed. You may start a local terminal window from "Applications->Utilities".
- Cyberduck is a full-featured and free graphical SFTP and SCP client.
Globus
Link to section 'Globus' of 'Globus' Globus
Globus, previously known as Globus Online, is a powerful and easy to use file transfer service for transferring files virtually anywhere. It works within RCAC's various research storage systems; it connects between RCAC and remote research sites running Globus; and it connects research systems to personal systems. You may use Globus to connect to your home, scratch, and Fortress storage directories. Since Globus is web-based, it works on any operating system that is connected to the internet. The Globus Personal client is available on Windows, Linux, and Mac OS X. It is primarily used as a graphical means of transfer but it can also be used over the command line.
Link to section 'Link to section 'Globus Web:' of 'Globus' Globus Web:' of 'Globus' Link to section 'Globus Web:' of 'Globus' Globus Web:
- Navigate to http://transfer.rcac.purdue.edu
- Click "Proceed" to log in with your Purdue Career Account.
- On your first login it will ask to make a connection to a Globus account. Accept the conditions.
- Now you are at the main screen. Click "File Transfer" which will bring you to a two-panel interface (if you only see one panel, you can use selector in the top-right corner to switch the view).
- You will need to select one collection and file path on one side as the source, and the second collection on the other as the destination. This can be one of several Purdue endpoints, or another University, or even your personal computer (see Personal Client section below).
The RCAC collections are as follows. A search for "Purdue" will give you several suggested results you can choose from, or you can give a more specific search.
- Home Directory storage: "Purdue Research Computing - Home Directories", however, you can start typing "Purdue" and "Home Directories" and it will suggest appropriate matches.
- Weber scratch storage: "Purdue Weber Cluster", however, you can start typing "Purdue" and "Weber and it will suggest appropriate matches. From here you will need to navigate into the first letter of your username, and then into your username.
- Research Data Depot: "Purdue Research Computing - Data Depot", a search for "Depot" should provide appropriate matches to choose from.
- Fortress: "Purdue Fortress HPSS Archive", a search for "Fortress" should provide appropriate matches to choose from.
From here, select a file or folder in either side of the two-pane window, and then use the arrows in the top-middle of the interface to instruct Globus to move files from one side to the other. You can transfer files in either direction. You will receive an email once the transfer is completed.
Link to section 'Link to section 'Globus Personal Client setup:' of 'Globus' Globus Personal Client setup:' of 'Globus' Link to section 'Globus Personal Client setup:' of 'Globus' Globus Personal Client setup:
Globus Connect Personal is a small software tool you can install to make your own computer a Globus endpoint on its own. It is useful if you need to transfer files via Globus to and from your computer directly.
- On the "Collections" page from earlier, click "Get Globus Connect Personal" or download a version for your operating system it from here: Globus Connect Personal
- Name this particular personal system and follow the setup prompts to create your Globus Connect Personal endpoint.
- Your personal system is now available as a collection within the Globus transfer interface.
Link to section 'Link to section 'Globus Command Line:' of 'Globus' Globus Command Line:' of 'Globus' Link to section 'Globus Command Line:' of 'Globus' Globus Command Line:
Globus supports command line interface, allowing advanced automation of your transfers.
To use the recommended standalone Globus CLI application (the globus command):
- First time use: issue the globus login command and follow instructions for initial login.
- Commands for interfacing with the CLI can be found via Using the Command Line Interface, as well as the Globus CLI Examples pages.
Link to section 'Link to section 'Sharing Data with Outside Collaborators' of 'Globus' Sharing Data with Outside Collaborators' of 'Globus' Link to section 'Sharing Data with Outside Collaborators' of 'Globus' Sharing Data with Outside Collaborators
Globus allows convenient sharing of data with outside collaborators. Data can be shared with collaborators' personal computers or directly with many other computing resources at other institutions. See the Globus documentation on how to share data:
For links to more information, please see Globus Support page and RCAC Globus presentation.
HSI
HSI, the Hierarchical Storage Interface, is the preferred method of transferring files to and from Fortress. HSI is designed to be a friendly interface for users of the High Performance Storage System (HPSS). It provides a familiar Unix-style environment for working within HPSS while automatically taking advantage of high-speed, parallel file transfers without requiring any special user knowledge.
HSI is provided on all research systems as the command hsi. HSI is also available for download for many operating systems.
Interactive usage:
$ hsi
*************************************************************************
* Purdue University
* High Performance Storage System (HPSS)
*************************************************************************
* This is the Purdue Data Archive, Fortress. For further information
* see http://www.rcac.purdue.edu/storage/fortress/
*
* If you are having problems with HPSS, please call IT/Operational
* Services at 49-44000 or send E-mail to rcac-help@purdue.edu.
*
*************************************************************************
Username: myusername UID: 12345 Acct: 12345(12345) Copies: 1 Firewall: off [hsi.3.5.8 Wed Sep 21 17:31:14 EDT 2011]
[Fortress HSI]/home/myusername->put data1.fits
put 'test' : '/home/myusername/test' ( 1024000000 bytes, 250138.1 KBS (cos=11))
[Fortress HSI]/home/myusername->lcd /tmp
[Fortress HSI]/home/myusername->get data1.fits
get '/tmp/data1.fits' : '/home/myusername/data1.fits' (2011/10/04 16:28:50 1024000000 bytes, 325844.9 KBS )
[Fortress HSI]/home/myusername->quit
Batch transfer file:
put data1.fits
put data2.fits
put data3.fits
put data4.fits
put data5.fits
put data6.fits
put data7.fits
put data8.fits
put data9.fits
Batch usage:
$ hsi < my_batch_transfer_file
*************************************************************************
* Purdue University
* High Performance Storage System (HPSS)
*************************************************************************
* This is the Purdue Data Archive, Fortress. For further information
* see http://www.rcac.purdue.edu/storage/fortress/
*
* If you are having problems with HPSS, please call IT/Operational
* Services at 49-44000 or send E-mail to rcac-help@purdue.edu.
*
*************************************************************************
Username: myusername UID: 12345 Acct: 12345(12345) Copies: 1 Firewall: off [hsi.3.5.8 Wed Sep 21 17:31:14 EDT 2011]
put 'data1.fits' : '/home/myusername/data1.fits' ( 1024000000 bytes, 250200.7 KBS (cos=11))
put 'data2.fits' : '/home/myusername/data2.fits' ( 1024000000 bytes, 258893.4 KBS (cos=11))
put 'data3.fits' : '/home/myusername/data3.fits' ( 1024000000 bytes, 222819.7 KBS (cos=11))
put 'data4.fits' : '/home/myusername/data4.fits' ( 1024000000 bytes, 224311.9 KBS (cos=11))
put 'data5.fits' : '/home/myusername/data5.fits' ( 1024000000 bytes, 323707.3 KBS (cos=11))
put 'data6.fits' : '/home/myusername/data6.fits' ( 1024000000 bytes, 320322.9 KBS (cos=11))
put 'data7.fits' : '/home/myusername/data7.fits' ( 1024000000 bytes, 253192.6 KBS (cos=11))
put 'data8.fits' : '/home/myusername/data8.fits' ( 1024000000 bytes, 253056.2 KBS (cos=11))
put 'data9.fits' : '/home/myusername/data9.fits' ( 1024000000 bytes, 323218.9 KBS (cos=11))
EOF detected on TTY - ending HSI session
For more information about HSI:
HTAR
HTAR (short for "HPSS TAR") is a utility program that writes TAR-compatible archive files directly onto Fortress, without having to first create a local file. Its command line was originally based on tar, with a number of extensions added to provide extra features.
HTAR is provided on all research systems as the command htar. HTAR is also available for download for many operating systems.
Link to section 'Usage:' of 'HTAR' Usage:
Create a tar archive on Fortress named data.tar including all files with the extension ".fits":
$ htar -cvf data.tar *.fits
HTAR: a data1.fits
HTAR: a data2.fits
HTAR: a data3.fits
HTAR: a data4.fits
HTAR: a data5.fits
HTAR: a /tmp/HTAR_CF_CHK_17953_1317760775
HTAR Create complete for data.tar. 5,120,006,144 bytes written for 5 member files, max threads: 3 Transfer time: 16.457 seconds (311.121 MB/s)
HTAR: HTAR SUCCESSFUL
Unpack a tar archive on Fortress named data.tar into a scratch directory for use in a batch job:
$ cd $RCAC_SCRATCH/job_dir
$ htar -xvf data.tar
HTAR: x data1.fits, 1024000000 bytes, 2000001 media blocks
HTAR: x data2.fits, 1024000000 bytes, 2000001 media blocks
HTAR: x data3.fits, 1024000000 bytes, 2000001 media blocks
HTAR: x data4.fits, 1024000000 bytes, 2000001 media blocks
HTAR: x data5.fits, 1024000000 bytes, 2000001 media blocks
HTAR: Extract complete for data.tar, 5 files. total bytes read: 5,120,004,608 in 18.841 seconds (271.749 MB/s )
HTAR: HTAR SUCCESSFUL
Look at the contents of the data.tar HTAR archive on Fortress:
$ htar -tvf data.tar
HTAR: -rw-r--r-- myusername/pucc 1024000000 2011-10-04 16:30 data1.fits
HTAR: -rw-r--r-- myusername/pucc 1024000000 2011-10-04 16:35 data2.fits
HTAR: -rw-r--r-- myusername/pucc 1024000000 2011-10-04 16:35 data3.fits
HTAR: -rw-r--r-- myusername/pucc 1024000000 2011-10-04 16:35 data4.fits
HTAR: -rw-r--r-- myusername/pucc 1024000000 2011-10-04 16:35 data5.fits
HTAR: -rw------- myusername/pucc 256 2011-10-04 16:39 /tmp/HTAR_CF_CHK_17953_1317760775
HTAR: Listing complete for data.tar, 6 files 6 total objects
HTAR: HTAR SUCCESSFUL
Unpack a single file, "data5.fits", from the tar archive on Fortress named data.tar into a scratch directory.:
$ htar -xvf data.tar data5.fits
HTAR: x data5.fits, 1024000000 bytes, 2000001 media blocks
HTAR: Extract complete for data.tar, 1 files. total bytes read: 1,024,000,512 in 3.642 seconds (281.166 MB/s )
HTAR: HTAR SUCCESSFUL
Link to section 'HTAR Archive Verification' of 'HTAR' HTAR Archive Verification
HTAR allows different types of content verification while creating archives. Users can ask HTAR to verify the contents of an archive during (or after) creation using the '-Hverify' switch. The syntax of this option is:
$ htar -Hverify=option[,option...] ... other arguments ...
Option | Explanation |
---|---|
info |
Compares tar header info with the corresponding values in the index. |
crc |
Enables CRC checking of archive files for which a CRC was generated when the file is added to the archive. |
compare |
Enables a byte-by-byte comparison of archive member files and their local file counterparts. |
nocrc |
Disables CRC checking of archive files. |
nocompare |
Disables a byte-by-byte comparison of archive member files and their local file counterparts. |
Users can use a comma-separated list of options shown above, or a numeric value, or the wildcard all to specify the degree of verification. The numeric values for Hverify can be interpreted as follows:
0: Enables "info" verification.
1: Enables level 0 + "crc" verification.
2: Enables level 1 + "compare" verification.
all: Enables all comparison options.
An example to verify an archive during creation using checksums (crc):
htar -Hverify=1 -cvf abc.tar ./abc
An example to verify a previously created archive using checksums (crc):
htar -Hverify=1 -Kvf abc.tar
Please note that the time for verifying an archive increases as you increase the verification level. Carefully choose the option that suits your dataset best.
For details please see the HTAR Man Page.
For more information about HTAR:
HTAR has an individual file size limit of 64GB. If any files you are trying to archive with HTAR are greater than 64GB, then HTAR will immediately fail. This does not limit the number of files in the archive or the total overall size of the archive. To get around this limitation, try using the htar_large
command. It is slower than using htar
but it will work around the 64GB file size limit. This does not limit the number of files in the archive or the total overall size of the archive.
To get around this limitation, try using the htar_large
command. It is slower than using HTAR but it will work around the 64GB file size limit. The usage of htar_large
is almost the same as htar
except that htar_large
will not generate the tar index file. Thus, the -Hverify=1
option cannot be used since it's based on index file.
Frequently Asked Questions
Some common questions, errors, and problems are categorized below. Click the Expand Topics link in the upper right to see all entries at once. You can also use the search box above to search the user guide for any issues you are seeing.
About Fortress
Frequently asked questions about Fortress.
Can you remove me from the Fortress mailing list?
Your subscription in the Fortress mailing list is tied to your account on Fortress. If you are no longer using your account on Fortress, your account can be deleted from the My Accounts page. Hover over the resource you wish to remove yourself from and click the red 'X' button. Your account and mailing list subscription will be removed overnight. Be sure to make a copy of any data you wish to keep first.
Do I need to do anything to my firewall to access Fortress?
Yes, any machines using HSI or HTAR must have all firewalls (local and departmental) configured to allow open access from the following IP addresses:
128.211.138.154
128.211.138.155
128.211.138.156
128.211.138.157
128.211.138.158
128.211.138.159
128.211.138.160
128.211.138.161
128.211.138.162
128.211.138.163
Firewall issues may manifest with error messages like "put: Error -50 on transfer." If you are unsure of how to modify your firewall settings, please consult with your department's IT support or the documentation for your operating system. Access to Fortress is restricted to on-campus networks. If you need to directly access Fortress from off-campus, please use the Purdue VPN service before connecting.
Note: The list of IP addresses changes occasionally as machines are added or retired. The list above is current, so if you have other IP addresses in your firewall, they can be safely removed. In particular, any IPs in the range 128.211.138.40-128.211.138.48 can be removed.
Can I download HSI or HTAR binaries for my OS platform?
Yes, visit the Downloads page to download HSI or HTAR packages for your operating system.
Note: If your username on your desktop does not match your career account username, HSI and HTAR require configuration to connect using your career account username:
- For HSI, use the -l careeraccount option on the hsi command line.
- For HTAR, set the HPSS_PRINCIPAL environment variable to your career account username:
bash: export HPSS_PRINCIPAL=careeraccount
csh/tcsh: setenv HPSS_PRINCIPAL=careeraccount
Can I set up a shared space for my research group to share data?
Research groups are assigned a group data storage space within Fortress with each Data Depot group space. Faculty should request a Data Depot trial to create a shared Fortress space for their research group.
RCAC resources are not intended to store data protected by Federal privacy and security laws (e.g., HIPAA, ITAR, classified, etc.). It is the responsibility of the faculty partner to ensure that no protected data is stored on the systems.
Please keep in mind that such spaces are, by design, accessible by others and should not be used to store private information such as grades, login credentials, or personal data. Contact us to create a group space for your group.
What limitations does Fortress have?
Fortress has a few limitations that you should keep in mind:
- Fortress does not support direct FTP or SCP transfers. SFTP connections are supported.
- Fortress does not support Unicode filenames. All filenames must contain only ASCII characters.
- Fortress does not support sparse files.
- Fortress is a tape archive. While it can handle use case of "multitude of small files", performance may be severely decreased (compared to a much preferred case of "fewer files of much larger size"). If you need to store a large number of small files, we strongly recommend that you bundle them up first (with zip, tar, htar, etc) before placing resulting archive into Fortress. Note: a "small file" on Fortress scale is typically considered something under 30-50MB per file.
- HTAR has an individual file size limit of 64GB. If any files you are trying to archive with HTAR are greater than 64GB, then HTAR will immediately fail. This does not limit the number of files in the archive or the total overall size of the archive. To get around this limitation, try using the htar_large command. It is slower than using HTAR but it will work around the 64GB file size limit.
Data
Frequently asked questions about data and data management.
What is the best way to access my data?
HSI and HTAR: HSI provides a FTP-style interface taking advantage of the power of HPSS without requiring any special user knowledge. HTAR is a utility to aggregate a set of files into a single tar archive directly into Fortress, without requiring space to first create an archive.
Can I set up a shared space for my research group to share data?
Research groups are assigned a group data storage space within Fortress with each Data Depot group space. Faculty should request a Data Depot trial to create a shared Fortress space for their research group.
RCAC resources are not intended to store data protected by Federal privacy and security laws (e.g., HIPAA, ITAR, classified, etc.). It is the responsibility of the faculty partner to ensure that no protected data is stored on the systems.
Please keep in mind that such spaces are, by design, accessible by others and should not be used to store private information such as grades, login credentials, or personal data. Contact us to create a group space for your group.
How can I verify the contents of my archives while using HTAR?
You can ask HTAR to verify the contents of an archive during/after creation using the '-Hverify' switch. Please see the Fortress User Guide for details.
HSI/HTAR: put: Error -5 on transfer
First, check your firewall settings, and ensure that there are no firewall rules interfering with connecting to Fortress. For firewall configuration, please see "Do I need to do anything to my firewall to access Fortress?" If firewalls are not responsible:
Open the file named /etc/hosts on your workstation, especially if you run a Debian or Ubuntu Linux distribution. Look for a line like:
127.0.1.1 hostname.dept.purdue.edu hostname
Replace the IP address 127.0.1.1 with the real IP address for your system. If you don't know your IP address, you can find it with the command:
host `hostname --fqdn`
Can I share data with outside collaborators?
Yes! Globus allows convenient sharing of data with outside collaborators. Data can be shared with collaborators' personal computers or directly with many other computing resources at other institutions. See the Globus documentation on how to share data:
HSI/HTAR: Unable to authenticate user with remote gateway (error 2 or 9)
There could be a variety of such errors, with wordings along the lines of
Could not initialize keytab on remote server.
result = -2, errno = 2rver connection
*** hpssex_OpenConnection: Unable to authenticate user with remote gateway at 128.211.138.40.1217result = -2, errno = 9
Unable to setup communication to HPSS...
ERROR (main) unable to open remote gateway server connection
HTAR: HTAR FAILED
and
*** hpssex_OpenConnection: Unable to authenticate user with remote gateway at 128.211.138.40.1217result = -11000, errno = 9
Unable to setup communication to HPSS...
*** HSI: error opening logging
Error - authentication/initialization failed
The root cause for these errors is an expired or non-existent keytab file (a special authentication token stored in your home directory). These keytabs are valid for 90 days and on most RCAC resources they are usually automatically checked and regenerated when you execute hsi
or htar
commands. However, if the keytab is invalid, or fails to generate, Fortress may be unable to authenticate you and you would see the above errors. This is especially common on those RCAC clusters that have their own dedicated home directories (such as Bell), or on standalone installations (such as if you downloaded and installed HSI and HTAR on your non-RCAC computer).
This is a temporary problem and a permanent system-wide solution is being developed. In the interim, the recommended workaround is to generate a new valid keytab file in your main research computing home directory, and then copy it to your home directory on Fortress. The fortresskey
command is used to generate the keytab and can be executed on another cluster or a dedicated data management host data.rcac.purdue.edu
:
$ ssh myusername@data.rcac.purdue.edu fortresskey
$ scp -pr myusername@data.rcac.purdue.edu:~/.private $HOME
With a valid keytab in place, you should then be able to use hsi
and htar
commands to access Fortress from Fortress. Note that only one keytab can be valid at any given time (i.e. if you regenerated it, you may have to copy the new keytab to all systems that you intend to use hsi
or htar
from if they do not share the main research computing home directory).
REED Folder User Guide
A REED Folder is a managed storage solution built on top of the Box.com cloud platform, for research projects requiring compliance with regulations or heightened security.
REED Folder Overview
As with the community clusters or Research Data Depot, research labs requiring data storage for regulated research will be able to easily purchase REED Folders through the Purchase page on this site. For more information, please contact us.
Link to section 'REED Folder Features' of 'REED Folder Overview' REED Folder Features
A REED Folder offers research groups in need of centralized data storage for regulated research unique features and benefits:
-
Available
To any Purdue research group requiring data storage space for regulated research.
-
Accessible
Easily accessible through your web browser, and facilitates easy sharing with collaborators within Purdue and without.
-
Capable
A REED folder facilitates joint work on shared files across your research group, avoiding the need for numerous copies of datasets across individuals' private storage. It is an ideal place to store a project's data and documents. A REED Folder can store unlimited data, with a maximum size of a single file up to 15 GB.
-
Controllable Access
Access management is under your direct control, within the bounds of the appropriate data use agreements, IRB protocols, or technology control plans.
-
Data Retention
All data kept in the REED Folder remains owned by the research group's lead faculty. Files kept in the project's REED folder remain with the research group, unaffected by turnover, and could head off potentially difficult disputes. Data is also retained and protected in alignment with the appropriate data security standards governing your research projects.
-
Never Purged
A REED Folder is never subject to purging.
-
Reliable
REED Folders are built on Purdue's centrally-managed Box.com service, which is a highly-available, secure cloud storage platform.
-
Restricted Data
REED Folders are designed to align with the NIST SP 800-171 standard, and are approved for storing L3 projects requiring HIPAA-aligned storage. Compliance with additional regulations such as CUI, ITAR, EAR, or FERPA are under review.
Link to section 'REED Folder Hardware Details' of 'REED Folder Overview' REED Folder Hardware Details
REED Folders are built on Box.com, an enterprise-grade cloud platform for file storage and collaboration.
Frequently Asked Questions
Some common questions, errors, and problems are categorized below. Click the Expand Topics link in the upper right to see all entries at once. You can also use the search box above to search the user guide for any issues you are seeing.
About REED Folder
Frequently asked questions about REED Folder.
What do I need to do before I access my REED Folder?
- Anyone with access to data in scope of HIPAA must complete the required HIPAA training annually. A PI is responsible to ensure the training is completed annually.
- Ensure you are using Purdue Login via the Duo client.
- For limited data sets, a Data Security Plan and Data Use Agreement will be required. This process should be started by Sponsored Program Services.
- Ensure you understand the following policies and procedures specific to HIPAA.
- If you are working with the IRB, ensure the process is completed prior to requesting access to REED Folder.
Does Purdue have a Business Associate Agreement with Box?
Box supports the HIPAA and HITECH regulations, as well as the ability to sign HIPAA Business Associate Agreements (BAAs) with customers. Customers who are required by law to comply with HIPAA, such as HIPAA Covered Entities and HIPAA Business Associates, must have an Enterprise or Elite account with Box and sign a HIPAA Business Associate Agreement (BAA).
- Purdue University signed a Business Associate Agreement with Box, and Box accounts are Enterprise.
- Box provides the ability to use O365 online office application to open and work with content. Purdue University has signed a Business Associate Agreement with Microsoft.
- All other third-party service providers with our instance of Box Enterprise are not covered by a Business Associate Agreement (BAA).
Do not use third party party applications to process data considered Restricted via your Purdue managed Box account. Purdue University does not maintain a contractual agreement with the vendor, and the required security controls are not in place.
A Box Managed account is your Purdue managed Box account. A REED folder is a project folder created in secure folder structure within Box.
Who should I contact for an IT incident?
- The incident should be reported as soon as it is discovered. Submit an incident request to abuse@purdue.edu
- If you lost a device, your account can be logged out using a Box admin feature. Submit an incident request to abuse@purdue.edu
What is a Box Managed Account and REED Folder?
A Box Managed account is your Purdue managed Box account. A REED folder is a project folder created in secure folder structured within Box.
Logging In & Accounts
Frequently asked questions about logging in & accounts.
Can I manage my Login Activity in Box?
In Box under your account settings, click the "Security" tab. You can review and remove sessions.
Data
Frequently asked questions about data and data management.
Can I integrate 3rd-Party Apps with my Box folder?
Third-Party Apps for Box
While official Box add-on applications are approved for use with Purdue Box.com folders, third-party apps have not been reviewed for impact to security and are not approved for use until a security review has been completed by IT Security and Policy. If the official Box apps cannot provide the functionality you need, you may request a security review of the third-party app you are considering by submitting an email including the app name and the functionality you are addressing with the app to itpolicyreq@purdue.edu.
How should I monitor access to my REED Folder?
The PI or Project Owner’s should follow the recommendations below:
- Review Sharing tab monthly, to ensure only authorized people have access to the data
- Review the Access Stats on files deemed restricted periodically to ensure the actions taken are appropriate.
- Maintain a log of your actions, the log can be a spreadsheet or word document. The log should be stored outside of Box.
Can I use Unmanaged Box accounts, and other Cloud Storage Options?
Unmanaged (free and commercial) Box accounts and all other Cloud Storage options are not approved for storing or sharing sensitive or restricted data. Purdue University does not maintain a contractual agreement with the vendor, and the required security controls are not in place. Personal use of cloud storage can continue, however sensitive and restricted data must not be stored in the account. The table below will help you determine where to store your data.
Classification | Personal Box Folder | REED Folder |
---|---|---|
Individually Identifiable Health Information | No | Determined by Review |
Limited Data Set | No | Yes |
De-Identified Data | No | Yes |
De-Identified Data with Contractual Requirements | No | Yes |
What is De-identified Data?
De-identified data is created by removing all 18 elements that could be used to identify the individual or the individual's relatives, employers, or household members; these elements are enumerated in the Privacy Rule. The covered entity also must have no actual knowledge that the remaining information could be used alone or in combination with other information to identify the individual who is the subject of the information. De-identified health information, as described in the Privacy Rule, is not PHI, and thus is not protected by the Privacy Rule however it could be subject to contractual requirements. Requests to store additional data types within scope of HIPAA should be sent to ITSP for review prior to uploading to Box Enterprise.
Should I use Box Drive if I work with Restricted Data?
Currently, Box Drive is not recommended for use to work with restricted data. However, if you have a business need for Box Drive, contact IT Security and Policy so we can help you understand the risk and ensure you have the appropriate controls in place to protect the data.
Will Box Drive or Box Edit cache files on the local machine?
Box Drive does locally cache files that you have opened. Box Drive's cache size limit is based on your free disk space (50% of available space) and has a maximum limit of 25 GB. If you reach this limit, Box Drive begins removing files, starting with those files files that have gone the longest without your accessing them. Also, if a cached file has a new version created on Box, Box Drive discards the locally cached version. Do not open data in Box Drive containing restricted data. More details: Technical Information for Box Drive Administrators
Box Edit does download the file to a cache when you are modifying a file. The cache can be cleared. See: Clear Your Box Edit Cache
Can I use File Level Encryption with a REED Folder?
The data stored in REED folder is encrypted at rest, however file level encryption is a responsibility of the individual. Files can be encrypted prior to upload for added security measures. Do not use Windows EFS, the data will be uploaded without the encryption. Currently, we recommend using VeraCrypt.
Can I use FTP to access Box?
No, FTP is disabled.
What are the requirements for a laptop or desktop if I am working with Restricted data?
- Encrypt hard drive using Whole Disk Encryption for all drives in the system
- Must be a Purdue managed laptop or desktop
- Must have software security updates applied every 30 days
- Install anti-virus, anti-spyware software and ensure definitions are up to date and run regular scans; utilize an endpoint protection solution.
- Require re-authentication after 15 minutes of inactivity
- Enable host based firewall
- Ensure only authorized software on systems is accessing, transmitting, storing sensitive or restricted information
- Require Authentication from remote devices
- Disable bluetooth on machines unless otherwise required.
- Avoid the use of removable media unless required, in which case removable media must be encrypted.
- Require Administrator privileges to install applications.
What is a Limited Data Set?
A limited data set is a separate legal concept under the privacy rule and is considered identifiable data. Specifically, a limited data set refers to PHI that has had the following 16 unique identifiers removed. A data use agreement is how covered entities obtain satisfactory assurances that the recipient of the limited data set will use or disclose the PHI in the data set only for specified purposes. A Data Use Agreement will continue to be required when working with limited data sets in Box.
Should I move restricted data to other folders outside of a REED Folder?
When storing data within scope of HIPAA, if must be stored in a REED Folder:
- Do not move and or store the data in a folder hosted directly in your personal Box account.
- Do not store the data outside of REED Folder unless you moving the data to a HIPAA aligned system and you have received approval from Purdue's IT Security and Policy group (ITSP).
- Moving data may also require approval from campus offices and committees responsible for contractual compliance and research regulatory affairs.
What are the folder naming conventions and folder description requirements for a REED Folder?
All folders and subfolders must follow a naming convention. The text must be added manually. The folder name must begin with [L3 HIPAA]-Folder Name. The naming convention should be used on any folder hosted in REED Folder. This is important, to allow for auditing of the service. All folders containing restricted data, must add a banner to the folder by using the Box folder description.
"The folder contains restricted data in scope of HIPAA. Exercise caution when sharing restricted or sensitive data, individuals must be authorized to access the data. Never sync restricted or sensitive data to an unauthorized system."
It best that you project team work from root folder of the project. If you create a collaboration in a sub folder, be certain you add the banner text and naming convention to ensure the project team knows the folder contains restricted data.
As a best practice, you can add [P1 Public] to folder names that don’t contain restricted or sensitive data, this will help you managed the various data types you generate.
Can I delete my files permanently from Box?
Individuals can delete a file or folder, and the item will be placed in the Trash. Individuals will not be able to permanently delete the items. An automated process is in place to remove data older than 90 days. Once the item is purged, it can't be recovered using the built in Box tools.
What are the recommended permission levels?
Role | Permission Level |
---|---|
Project Team member who needs full control | Editor |
Project Team members who just needs to work within Box | Viewer Uploader |
Project Team member who needs Read/Download access | Viewer |
Partner who needs Read access | Previewer |
Partner who need to upload data | Uploader |
How do I host sensitive or restricted data in Box?
Storage of restricted data in Box is subject to review from Purdue's IT Security and Policy group (ITSP). Access may also require approval from campus offices and committees responsible for contractual compliance and research regulatory affairs.
Purdue’s managed Box environment can be used to store, de-identified data and limited data sets in scope of HIPAA for research. Fully identified data is subject to a security review and approval process.
All restricted data must be stored in a REED Folder. Do not use Box for an actual medical practice.
See the data map below for more details:
Box Research Lab Folder User Guide
A Box Research Lab Folder is a managed storage solution built on top of the Box.com cloud platform, for research labs to share and collaborate within the lab and with outside collaborators.
Box Research Lab Folder Overview
As with the community clusters or Research Data Depot, research labs requiring a lab collaboration space will be able to easily request Box Research Lab Folders through the Purchase page on this site. For more information, please contact us.
Link to section 'Box Research Lab Folder Features' of 'Box Research Lab Folder Overview' Box Research Lab Folder Features
A Box Research Lab Folder offers research groups in need of centralized data storage for research collaboration many unique features and benefits:
-
Available
To any Purdue research group requiring data storage space for research collaboration.
-
Accessible
Easily accessible through your web browser, and facilitates easy sharing with collaborators within Purdue and without.
-
Capable
A Box Research Lab folder facilitates joint work on shared files across your research group, avoiding the need for numerous copies of datasets across individuals' private storage. It is an ideal place to store a project's data and documents. A Lab Folder can store unlimited data, with a maximum size of a single file up to 15 GB.
-
Controllable Access
Access management is under your direct control, within the bounds of the appropriate data use agreements, IRB protocols, or technology control plans.
-
Data Retention
All data kept in the Box Research Lab Folder remains owned by the research group's lead faculty. Files kept in the project's lab folder remain with the research group, unaffected by turnover, and could head off potentially difficult disputes. Data is also retained and protected in alignment with the appropriate data security standards governing your research projects.
-
Never Purged
A Box Research Lab Folder is never subject to purging.
-
Reliable
Box Research Lab Folders are built on Purdue's centrally-managed Box.com service, which is a highly-available, secure cloud storage platform.
-
Restricted Data
Box Research Lab Folders are not designed to align with the NIST SP 800-171 standard, and are not approved for storing L3 projects requiring HIPAA-aligned storage. Please request a REED Folder for sensitive or restricted research data.
Link to section 'Box Research Lab Folder Hardware Details' of 'Box Research Lab Folder Overview' Box Research Lab Folder Hardware Details
Box Research Lab Folders are built on Box.com, an enterprise-grade cloud platform for file storage and collaboration.
Link to section 'Access to Box Research Lab Folder' of 'Accounts on Box Research Lab Folders' Access to Box Research Lab Folder
Link to section 'Obtaining an Account' of 'Accounts on Box Research Lab Folders' Obtaining an Account
All Purdue faculty, staff, and students have a Box.com account for personal files.
Research projects requiring regulated storage are assigned group data storage space within Box Research Lab Folder. Project PIs may authorize additional users to collaborate within the folder.
Box research lab Folders are not intended to store data protected by Federal privacy and security laws. REED Folders are designed to align with the NIST SP 800-171 standard, and are approved for storing L3 projects requiring HIPAA-aligned storage. Compliance with additional regulations such as CUI, ITAR, EAR, or FERPA are under review.
Neither Purdue or Box set any limits on the total amount or number of files that you may store within your Box Research Lab Folder. However, there are several restrictions on the nature of files you may store:
- Any one individual file may be no larger than 15 GB.
Link to section 'Outside Collaborators' of 'Accounts on Box Research Lab Folders' Outside Collaborators
Within the bounds of your data use agreements, IRB protocols, or technology control plans, REED data folders may be shared with Purdue or non-Purdue collaborators.
Link to section 'Login to Box to Access your Research Lab Folder' of 'Accounts on Box Research Lab Folders' Login to Box to Access your Research Lab Folder
Access to your Box Research Lab Folder is done using your Purdue Career Account by visiting purdue.box.com.
Link to section 'Transferring Files into Box' of 'Accounts on Box Research Lab Folders' Transferring Files into Box
Files may be up/downloaded from Box via FTPS.
Frequently Asked Questions
Some common questions, errors, and problems are categorized below. Click the Expand Topics link in the upper right to see all entries at once. You can also use the search box above to search the user guide for any issues you are seeing.
About Box Research Lab Folder
Frequently asked questions about Box Research Lab Folder.
Does Purdue have a Business Associate Agreement with Box?
Box supports the HIPAA and HITECH regulations, as well as the ability to sign HIPAA Business Associate Agreements (BAAs) with customers. Customers who are required by law to comply with HIPAA, such as HIPAA Covered Entities and HIPAA Business Associates, must have an Enterprise or Elite account with Box and sign a HIPAA Business Associate Agreement (BAA).
- Purdue University signed a Business Associate Agreement with Box, and Box accounts are Enterprise.
- Box provides the ability to use O365 online office application to open and work with content. Purdue University has signed a Business Associate Agreement with Microsoft.
- All other third-party service providers with our instance of Box Enterprise are not covered by a Business Associate Agreement (BAA).
Do not use third party party applications to process data considered Restricted via your Purdue managed Box account. Purdue University does not maintain a contractual agreement with the vendor, and the required security controls are not in place.
A Box Managed account is your Purdue managed Box account. A REED folder is a project folder created in secure folder structure within Box.
What is a Box Managed Account and REED Folder?
A Box Managed account is your Purdue managed Box account. A REED folder is a project folder created in secure folder structured within Box.
Data
Frequently asked questions about data and data management.
Can I integrate 3rd-Party Apps with my Box folder?
Third-Party Apps for Box
While official Box add-on applications are approved for use with Purdue Box.com folders, third-party apps have not been reviewed for impact to security and are not approved for use until a security review has been completed by IT Security and Policy. If the official Box apps cannot provide the functionality you need, you may request a security review of the third-party app you are considering by submitting an email including the app name and the functionality you are addressing with the app to itpolicyreq@purdue.edu.
Can I use Unmanaged Box accounts, and other Cloud Storage Options?
Unmanaged (free and commercial) Box accounts and all other Cloud Storage options are not approved for storing or sharing sensitive or restricted data. Purdue University does not maintain a contractual agreement with the vendor, and the required security controls are not in place. Personal use of cloud storage can continue, however sensitive and restricted data must not be stored in the account. The table below will help you determine where to store your data.
Classification | Personal Box Folder | REED Folder |
---|---|---|
Individually Identifiable Health Information | No | Determined by Review |
Limited Data Set | No | Yes |
De-Identified Data | No | Yes |
De-Identified Data with Contractual Requirements | No | Yes |
Should I use Box Drive if I work with Restricted Data?
Currently, Box Drive is not recommended for use to work with restricted data. However, if you have a business need for Box Drive, contact IT Security and Policy so we can help you understand the risk and ensure you have the appropriate controls in place to protect the data.
Will Box Drive or Box Edit cache files on the local machine?
Box Drive does locally cache files that you have opened. Box Drive's cache size limit is based on your free disk space (50% of available space) and has a maximum limit of 25 GB. If you reach this limit, Box Drive begins removing files, starting with those files files that have gone the longest without your accessing them. Also, if a cached file has a new version created on Box, Box Drive discards the locally cached version. Do not open data in Box Drive containing restricted data. More details: Technical Information for Box Drive Administrators
Box Edit does download the file to a cache when you are modifying a file. The cache can be cleared. See: Clear Your Box Edit Cache
Can I use FTP to access Box?
No, FTP is disabled.
Can I delete my files permanently from Box?
Individuals can delete a file or folder, and the item will be placed in the Trash. Individuals will not be able to permanently delete the items. An automated process is in place to remove data older than 90 days. Once the item is purged, it can't be recovered using the built in Box tools.
What are the recommended permission levels?
Role | Permission Level |
---|---|
Project Team member who needs full control | Editor |
Project Team members who just needs to work within Box | Viewer Uploader |
Project Team member who needs Read/Download access | Viewer |
Partner who needs Read access | Previewer |
Partner who need to upload data | Uploader |
How do I host sensitive or restricted data in Box?
Storage of restricted data in Box is subject to review from Purdue's IT Security and Policy group (ITSP). Access may also require approval from campus offices and committees responsible for contractual compliance and research regulatory affairs.
Purdue’s managed Box environment can be used to store, de-identified data and limited data sets in scope of HIPAA for research. Fully identified data is subject to a security review and approval process.
All restricted data must be stored in a REED Folder. Do not use Box for an actual medical practice.
See the data map below for more details:
Scratch User Guide
For Scratch Storage, each cluster is assigned a default Lustre or GPFS parallel filesystem. The parallel filesystems provide work-area storage optimized for a wide variety of job types, and are designed to perform well with data-intensive computations, while scaling well to large numbers of simultaneous connections.
Scratch Overview
Scratch Storage currently consists of several redundant, high-availability disk spaces and is a central component of the research system's infrastructure. All scratch tier resources are high-performance, large capacity, and subject to scheduled purging of old files.
Link to section 'Gilbreth:' of 'Scratch Overview' Gilbreth:
- Scratch filesystem for Gilbreth.
- Gilbreth scratch consists of 2.3PB of redundant, high-availability disk space.
- The quota on Gilbreth scratch is 200TB and 2,000,000 files.
Link to section 'Brown:' of 'Scratch Overview' Brown:
- Scratch filesystem for Brown.
- Brown scratch consists of 3.4PB of redundant, high-availability disk space.
- The quota on Brown scratch is 200TB and 2,000,000 files.
Files in scratch directories are not backed up or recoverable. If you accidentally delete a file, old files are purged, or the filesystem crashes, they cannot be restored. All important files should be backed up to the Fortress HPSS Archive on a regular basis.
If you need more space in your scratch directories, please contact us.
Home Directory User Guide
/home is the primary space used to permanently hold files for a given user.
Home Overview
Your Home Directories for all RCAC resources is provided by a DDN GS7KX filesystem appliance.
/home, the primary space used to permanently hold files for a given user, has a 25 GB quota which can be monitored at any time by using a myquota command.
Home Directories spaces currently reside on a self-contained ZFS storage system that provides redundant, high-availability disk space and is a central component of RCAC's research systems infrastructure.
RCAC uses network attached storage (NAS) appliances from DDN to provide scale-out Home Directories space to cluster systems. This storage is reliable, backed-up (via snapshots), and is globally available on all RCAC systems. Your Home Directories is medium-performance, non-purged space suitable for tasks like sharing data, editing files, developing and building software, and many other uses.
Your Home Directories is not designed or intended for use as high-performance working space for running jobs.
File Storage and Transfer
Learn more about file storage transfer for Home Directories.
Link to section 'Archive and Compression' of 'Archive and Compression' Archive and Compression
There are several options for archiving and compressing groups of files or directories. The mostly commonly used options are:
Link to section 'tar' of 'Archive and Compression' tar
See the official documentation for tar for more information.
Saves many files together into a single archive file, and restores individual files from the archive. Includes automatic archive compression/decompression options and special features for incremental and full backups.
Examples:
(list contents of archive somefile.tar)
$ tar tvf somefile.tar
(extract contents of somefile.tar)
$ tar xvf somefile.tar
(extract contents of gzipped archive somefile.tar.gz)
$ tar xzvf somefile.tar.gz
(extract contents of bzip2 archive somefile.tar.bz2)
$ tar xjvf somefile.tar.bz2
(archive all ".c" files in current directory into one archive file)
$ tar cvf somefile.tar *.c
(archive and gzip-compress all files in a directory into one archive file)
$ tar czvf somefile.tar.gz somedirectory/
(archive and bzip2-compress all files in a directory into one archive file)
$ tar cjvf somefile.tar.bz2 somedirectory/
Other arguments for tar can be explored by using the man tar command.
Link to section 'gzip' of 'Archive and Compression' gzip
The standard compression system for all GNU software.
Examples:
(compress file somefile - also removes uncompressed file)
$ gzip somefile
(uncompress file somefile.gz - also removes compressed file)
$ gunzip somefile.gz
Link to section 'bzip2' of 'Archive and Compression' bzip2
See the official documentation for bzip for more information.
Strong, lossless data compressor based on the Burrows-Wheeler transform. Stronger compression than gzip.
Examples:
(compress file somefile - also removes uncompressed file)
$ bzip2 somefile
(uncompress file somefile.bz2 - also removes compressed file)
$ bunzip2 somefile.bz2
There are several other, less commonly used, options available as well:
- zip
- 7zip
- xz
Link to section 'Sharing Files from Home Directories' of 'Sharing' Sharing Files from Home Directories
Home Directories supports several methods for file sharing. Use the links below to learn more about these methods.
Link to section 'Sharing Data with Globus' of 'Globus' Sharing Data with Globus
Data on any RCAC resource can be shared with other users within Purdue or with collaborators at other institutions. Globus allows convenient sharing of data with outside collaborators. Data can be shared with collaborators' personal computers or directly with many other computing resources at other institutions.
To share files, login to https://transfer.rcac.purdue.edu, navigate to the endpoint (collection) of your choice, and follow instructions as described in Globus documentation on how to share data:
See also RCAC Globus presentation.
File Transfer
Home Directories supports several methods for file transfer. Use the links below to learn more about these methods.
SCP
SCP (Secure CoPy) is a simple way of transferring files between two machines that use the SSH protocol. SCP is available as a protocol choice in some graphical file transfer programs and also as a command line program on most Linux, Unix, and Mac OS X systems. SCP can copy single files, but will also recursively copy directory contents if given a directory name.
After Aug 17, 2020, the community clusters will not support password-based authentication for login. Methods that can be used include two-factor authentication (Purdue Login) or SSH keys. If you do not have SSH keys installed, you would need to type your Purdue Login response into the SFTP's "Password" prompt.
Link to section 'Command-line usage:' of 'SCP' Command-line usage:
You can transfer files both to and from Home Directories while initiating an SCP session on either some other computer or on Home Directories (in other words, directionality of connection and directionality of data flow are independent from each other). The scp
command appears somewhat similar to the familiar cp
command, with an extra user@host:file
syntax to denote files and directories on a remote host. Either Home Directories or another computer can be a remote.
-
Example: Initiating SCP session on some other computer (i.e. you are on some other computer, connecting to Home Directories):
(transfer TO Home Directories) (Individual files) $ scp sourcefile myusername@data.rcac.purdue.edu:somedir/destinationfile $ scp sourcefile myusername@data.rcac.purdue.edu:somedir/ (Recursive directory copy) $ scp -pr sourcedirectory/ myusername@data.rcac.purdue.edu:somedir/ (transfer FROM Home Directories) (Individual files) $ scp myusername@data.rcac.purdue.edu:somedir/sourcefile destinationfile $ scp myusername@data.rcac.purdue.edu:somedir/sourcefile somedir/ (Recursive directory copy) $ scp -pr myusername@data.rcac.purdue.edu:sourcedirectory somedir/
The -p flag is optional. When used, it will cause the transfer to preserve file attributes and permissions. The -r flag is required for recursive transfers of entire directories.
-
Example: Initiating SCP session on Home Directories (i.e. you are on Home Directories, connecting to some other computer):
(transfer TO Home Directories) (Individual files) $ scp myusername@$another.computer.example.com:sourcefile somedir/destinationfile $ scp myusername@$another.computer.example.com:sourcefile somedir/ (Recursive directory copy) $ scp -pr myusername@$another.computer.example.com:sourcedirectory/ somedir/ (transfer FROM Home Directories) (Individual files) $ scp somedir/sourcefile myusername@$another.computer.example.com:destinationfile $ scp somedir/sourcefile myusername@$another.computer.example.com:somedir/ (Recursive directory copy) $ scp -pr sourcedirectory myusername@$another.computer.example.com:somedir/
The -p flag is optional. When used, it will cause the transfer to preserve file attributes and permissions. The -r flag is required for recursive transfers of entire directories.
Link to section 'Software (SCP clients)' of 'SCP' Software (SCP clients)
Linux and other Unix-like systems:
- The
scp
command-line program should already be installed.
Microsoft Windows:
- MobaXterm
Free, full-featured, graphical Windows SSH, SCP, and SFTP client. - Command-line
scp
program can be installed as part of Windows Subsystem for Linux (WSL), or Git-Bash.
Mac OS X:
- The
scp
command-line program should already be installed. You may start a local terminal window from "Applications->Utilities". - Cyberduck is a full-featured and free graphical SFTP and SCP client.
Globus
Link to section 'Globus' of 'Globus' Globus
Globus, previously known as Globus Online, is a powerful and easy to use file transfer service for transferring files virtually anywhere. It works within RCAC's various research storage systems; it connects between RCAC and remote research sites running Globus; and it connects research systems to personal systems. You may use Globus to connect to your home, scratch, and Fortress storage directories. Since Globus is web-based, it works on any operating system that is connected to the internet. The Globus Personal client is available on Windows, Linux, and Mac OS X. It is primarily used as a graphical means of transfer but it can also be used over the command line.
Link to section 'Link to section 'Globus Web:' of 'Globus' Globus Web:' of 'Globus' Link to section 'Globus Web:' of 'Globus' Globus Web:
- Navigate to http://transfer.rcac.purdue.edu
- Click "Proceed" to log in with your Purdue Career Account.
- On your first login it will ask to make a connection to a Globus account. Accept the conditions.
- Now you are at the main screen. Click "File Transfer" which will bring you to a two-panel interface (if you only see one panel, you can use selector in the top-right corner to switch the view).
- You will need to select one collection and file path on one side as the source, and the second collection on the other as the destination. This can be one of several Purdue endpoints, or another University, or even your personal computer (see Personal Client section below).
The RCAC collections are as follows. A search for "Purdue" will give you several suggested results you can choose from, or you can give a more specific search.
- Home Directory storage: "Purdue Research Computing - Home Directories", however, you can start typing "Purdue" and "Home Directories" and it will suggest appropriate matches.
- Weber scratch storage: "Purdue Weber Cluster", however, you can start typing "Purdue" and "Weber and it will suggest appropriate matches. From here you will need to navigate into the first letter of your username, and then into your username.
- Research Data Depot: "Purdue Research Computing - Data Depot", a search for "Depot" should provide appropriate matches to choose from.
- Fortress: "Purdue Fortress HPSS Archive", a search for "Fortress" should provide appropriate matches to choose from.
From here, select a file or folder in either side of the two-pane window, and then use the arrows in the top-middle of the interface to instruct Globus to move files from one side to the other. You can transfer files in either direction. You will receive an email once the transfer is completed.
Link to section 'Link to section 'Globus Personal Client setup:' of 'Globus' Globus Personal Client setup:' of 'Globus' Link to section 'Globus Personal Client setup:' of 'Globus' Globus Personal Client setup:
Globus Connect Personal is a small software tool you can install to make your own computer a Globus endpoint on its own. It is useful if you need to transfer files via Globus to and from your computer directly.
- On the "Collections" page from earlier, click "Get Globus Connect Personal" or download a version for your operating system it from here: Globus Connect Personal
- Name this particular personal system and follow the setup prompts to create your Globus Connect Personal endpoint.
- Your personal system is now available as a collection within the Globus transfer interface.
Link to section 'Link to section 'Globus Command Line:' of 'Globus' Globus Command Line:' of 'Globus' Link to section 'Globus Command Line:' of 'Globus' Globus Command Line:
Globus supports command line interface, allowing advanced automation of your transfers.
To use the recommended standalone Globus CLI application (the globus command):
- First time use: issue the globus login command and follow instructions for initial login.
- Commands for interfacing with the CLI can be found via Using the Command Line Interface, as well as the Globus CLI Examples pages.
Link to section 'Link to section 'Sharing Data with Outside Collaborators' of 'Globus' Sharing Data with Outside Collaborators' of 'Globus' Link to section 'Sharing Data with Outside Collaborators' of 'Globus' Sharing Data with Outside Collaborators
Globus allows convenient sharing of data with outside collaborators. Data can be shared with collaborators' personal computers or directly with many other computing resources at other institutions. See the Globus documentation on how to share data:
For links to more information, please see Globus Support page and RCAC Globus presentation.
FTP / SFTP
FTP is not supported on any research systems because it does not allow for secure transmission of data. Use SFTP instead, as described below.
SFTP (Secure File Transfer Protocol) is a reliable way of transferring files between two machines. SFTP is available as a protocol choice in some graphical file transfer programs and also as a command-line program on most Linux, Unix, and Mac OS X systems. SFTP has more features than SCP and allows for other operations on remote files, remote directory listing, and resuming interrupted transfers. Command-line SFTP cannot recursively copy directory contents; to do so, try using SCP or graphical SFTP client.
After Aug 17, 2020, the community clusters will not support password-based authentication for login. Methods that can be used include two-factor authentication (Purdue Login) or SSH keys. If you do not have SSH keys installed, you would need to type your Purdue Login response into the SFTP's "Password" prompt.
Link to section 'Command-line usage' of 'FTP / SFTP' Command-line usage
You can transfer files both to and from Home Directories while initiating an SFTP session on either some other computer or on Home Directories (in other words, directionality of connection and directionality of data flow are independent from each other). Once the connection is established, you use put
or get
subcommands between "local" and "remote" computers. Either Home Directories or another computer can be a remote.
-
Example: Initiating SFTP session on some other computer (i.e. you are on another computer, connecting to Home Directories):
$ sftp myusername@data.rcac.purdue.edu (transfer TO Home Directories) sftp> put sourcefile somedir/destinationfile sftp> put -P sourcefile somedir/ (transfer FROM Home Directories) sftp> get sourcefile somedir/destinationfile sftp> get -P sourcefile somedir/ sftp> exit
The -P flag is optional. When used, it will cause the transfer to preserve file attributes and permissions.
-
Example: Initiating SFTP session on Home Directories (i.e. you are on Home Directories, connecting to some other computer):
$ sftp myusername@$another.computer.example.com (transfer TO Home Directories) sftp> get sourcefile somedir/destinationfile sftp> get -P sourcefile somedir/ (transfer FROM Home Directories) sftp> put sourcefile somedir/destinationfile sftp> put -P sourcefile somedir/ sftp> exit
The -P flag is optional. When used, it will cause the transfer to preserve file attributes and permissions.
Link to section 'Software (SFTP clients)' of 'FTP / SFTP' Software (SFTP clients)
Linux and other Unix-like systems:
- The
sftp
command-line program should already be installed.
Microsoft Windows:
- MobaXterm
Free, full-featured, graphical Windows SSH, SCP, and SFTP client. - Command-line
sftp
program can be installed as part of Windows Subsystem for Linux (WSL), or Git-Bash.
Mac OS X:
- The
sftp
command-line program should already be installed. You may start a local terminal window from "Applications->Utilities". - Cyberduck is a full-featured and free graphical SFTP and SCP client.
Lost File Recovery
Home Directories is protected against accidental file deletion through a series of snapshots taken every night just after midnight. Each snapshot provides the state of your files at the time the snapshot was taken. It does so by storing only the files which have changed between snapshots. A file that has not changed between snapshots is only stored once but will appear in every snapshot. This is an efficient method of providing snapshots because the snapshot system does not have to store multiple copies of every file.
These snapshots are kept for a limited time at various intervals. RCAC keeps nightly snapshots for 7 days, weekly snapshots for 4 weeks, and monthly snapshots for 3 months. This means you will find snapshots from the last 7 nights, the last 4 Sundays, and the last 3 first of the months. Files are available going back between two and three months, depending on how long ago the last first of the month was. Snapshots beyond this are not kept.
Only files which have been saved during an overnight snapshot are recoverable. If you lose a file the same day you created it, the file is not recoverable because the snapshot system has not had a chance to save the file.
Snapshots are not a substitute for regular backups. It is the responsibility of the researchers to back up any important data to the Fortress Archive. Home Directories does protect against hardware failures or physical disasters through other means however these other means are also not substitutes for backups.
Files in scratch directories are not recoverable. Files in scratch directories are not backed up. If you accidentally delete a file, a disk crashes, or old files are purged, they cannot be restored.
Home Directories offers several ways for researchers to access snapshots of their files.
flost
If you know when you lost the file, the easiest way is to use the flost command. This tool is available from any RCAC resource. If you do not have access to a compute cluster, any Data Depot user may use an SSH client to connect to data.rcac.purdue.edu and run this command.
To run the tool you will need to specify the location where the lost file was with the -w argument:
$ flost -w /depot/mylab
Replace mylab with the name of your lab's Home Directories directory. If you know more specifically where the lost file was you may provide the full path to that directory.
This tool will prompt you for the date on which you lost the file or would like to recover the file from. If the tool finds an appropriate snapshot it will provide instructions on how to search for and recover the file.
If you are not sure what date you lost the file you may try entering different dates into the flost to try to find the file or you may also manually browse the snapshots as described below.
Manual Browsing
You may also search through the snapshots by hand on the Home Directories filesystem if you are not sure what date you lost the file or would like to browse by hand. Snapshots can be browsed from any RCAC resource. If you do not have access to a compute cluster, any Home Directories user may use an SSH client to connect to data.rcac.purdue.edu and browse from there. The snapshots are located at /depot/.snapshots
on these resources.
You can also mount the snapshot directory over Samba (or SMB, CIFS) on Windows or Mac OS X. Mount (or map) the snapshot directory in the same way as you did for your main Home Directories space substituting the server name and path for \\datadepot.rcac.purdue.edu\depot\.winsnaps
(Windows) or smb://datadepot.rcac.purdue.edu/depot/.winsnaps
(Mac OS X).
Once connected to the snapshot directory through SSH or Samba, you will see something similar to this:
SSH to data.rcac.purdue.edu | Samba mount on datadepot.rcac.purdue.edu |
---|---|
|
![]() |
Each of these directories is a snapshot of the entire Home Directories filesystem at the timestamp encoded into the directory name. The format for this timestamp is year, two digits for month, two digits for day, followed by the time of the day.
You may cd into any of these directories where you will find the entire Home Directories filesystem. Use cd to continue into your lab's Home Directories space and then you may browse the snapshot as normal.
If you are browsing these directories over a Samba network drive you can simply drag and drop the files over into your live Data Depot folder.
Once you find the file you are looking for, use cp to copy the file back into your lab's live Home Directories space. Do not attempt to modify files directly in the snapshot directories.
Windows
If you use Home Directories through "network drives" on Windows you may recover lost files directly from within Windows:
- Open the folder that contained the lost file.
- Right click inside the window and select "Properties".
- Click on the "Previous Versions" tab.
- A list of snapshots will be displayed.
- Select the snapshot from which you wish to restore.
- In the new window, locate the file you wish to restore.
- Simply drag the file or folder to their correct locations.
In the "Previous Versions" window the list contains two columns. The first column is the timestamp on which the snapshot was taken. The second column is the date on which the selected file or folder was last modified in that snapshot. This may give you some extra clues to which snapshot contains the version of the file you are looking for.
Mac OS X
Mac OS X does not provide any way to access the Home Directories snapshots directly. To access the snapshots there are two options: browse the snapshots by hand through a network drive mount or use an automated command-line based tool.
To browse the snapshots by hand, follow the directions outlined in the Manual Browsing section.
To use the automated command-line tool, log into a compute cluster or into the host data.rcac.purdue.edu (which is available to all Home Directories users) and use the flost tool. On Mac OS X you can use the built-in SSH terminal application to connect.
- Open the Applications folder from Finder.
- Navigate to the Utilities folder.
- Double click the Terminal application to open it.
- Type the following command when the terminal opens.
Replace myusername with your Purdue career account username and provide your password when prompted.$ ssh myusername@data.rcac.purdue.edu
Once logged in use the flost tool as described above. The tool will guide you through the process and show you the commands necessary to retrieve your lost file.
Frequently Asked Questions
Some common questions, errors, and problems are categorized below. Click the Expand Topics link in the upper right to see all entries at once. You can also use the search box above to search the user guide for any issues you are seeing.
About Home Directories
Frequently asked questions about Home Directories.
Do I need to do anything to my firewall to access Home Directories?
No firewall changes are needed to access Home Directories. However, to access data through Network Drives (i.e., CIFS, "Z: Drive"), you must be on a Purdue campus network or connected through VPN.
Data
Frequently asked questions about data and data management.
Can I store Export-controlled data on Home Directories?
The Home Directories is not approved for storing data requiring Export control including ITAR, FISMA, DFAR-7012, NIST 800-171. Please contact the Export Control Office to discuss technology control plans and data storage appropriate for export controlled projects.
Can I store HIPAA data on Home Directories?
The Home Directories is not approved for storing data covered by HIPAA. Please contact the HIPAA Compliance Office to discuss HIPAA-compliant data storage.
Can I share data with outside collaborators?
Yes! Globus allows convenient sharing of data with outside collaborators. Data can be shared with collaborators' personal computers or directly with many other computing resources at other institutions. See the Globus documentation on how to share data:
Services
-High-Performance Computing
High-performance computing (HPC) is the ability to process data and perform complex calculations at high speeds. To put it into perspective, a laptop or desktop with a 3 GHz processor can perform around 3 billion calculations per second. While that is much faster than any human can achieve, it pales in comparison to HPC solutions that can perform quadrillions of calculations per second.
One of the best-known types of HPC solutions is the supercomputer. A supercomputer contains thousands of compute nodes that work together to complete one or more tasks. This is called parallel processing. It’s similar to having thousands of PCs networked together, combining compute power to complete tasks faster.
HPC solutions have three main components:
- Compute
- Network
- Storage
To build a high-performance computing architecture, compute servers are networked together into a cluster. Software programs and algorithms are run simultaneously on the servers in the cluster. The cluster is networked to the data storage to capture the output. Together, these components operate seamlessly to complete a diverse set of tasks.
Link to section 'Compute' of 'High-Performance Computing' Compute
Link to section 'Compute nodes' of 'High-Performance Computing' Compute nodes
A compute node is the place where all the computing is performed. Most of the nodes in a cluster are ordinarily compute nodes. With a specific end goal to give a general arrangement, a compute node can execute one or more tasks, taking into account the scheduling system.
Link to section 'Front-ends' of 'High-Performance Computing' Front-ends
Clusters are complex environments, and administration of each individual segment is essential. The front-end node gives numerous capacities, including: observing the status of individual nodes and issuing orders to individual nodes to execute jobs.
Link to section 'Storage' of 'High-Performance Computing' Storage
Applications that keep running on a cluster, compute nodes must have quick, dependable, and concurrent access to a storage framework. Storage gadgets are specifically joined to the nodes.
Services Guides
Information about using complementary RCAC resources can be found in the links below.
GitHub User Guide
A local instance of GitHub is available. This instance offers all the features of github.com, however, under the control of and hosted by Purdue IT and is integrated with Purdue Career Accounts. Each research group can be provided with and given full control over an organization within the GitHub instance where repositories can be created and access configured as desired. Repositories can also be made private to groups to protect work not meant to be publicly available. It provides the full functionality of paid memberships with github.com, at no cost to Purdue researchers.
There are many resources available on the Internet about using GitHub. Most guides and tutorials for github.com will apply to our instance of GitHub. Some links to external documentation are provided below, as well as several pages within our site describing GitHub.
Tutorials provided by GitHub covering the basics of using Git and GitHub.
Getting Started
The GitHub instance is offered at no cost to Purdue research groups, current students and faculty, or any Purdue person performing academic not-for-profit research. To get started with GitHub head to the github.itap.purdue.edu to access the system.
Access to this service is only available from campus networks. You will need to use Purdue's VPN to access this service from off campus.
Managing Users
All users of your GitHub repositories will need to have a Purdue Career Account. Any non-Purdue collaborators will need to have a Request for Privileges (R4P) filed to create a Purdue Career Account for them before they can be given access to any repositories on the Purdue GitHub. If your project is largely a shared, multi-institution project, you should strongly consider use of github.com instead of Purdue's private GitHub.
Creating repositories
You may create repositories to share within your organization, or create personal repositories. Head to the GitHub instance and click the New Repository
button. Choose your organization or your username and give it a name. Select either Public
or Private
access for your repository. Public repositories are visible to anyone on the internet. Private repositories are only visible to those you choose. In either, only those you choose are able to modify the repository. Commit privileges and private repository viewing access requires a Purdue Career Account.
Organization and Teams
Essentially, an organization is a place to house your research group's work. Multiple repositories may be created inside the organization. You may create teams, or lists of users, for each project. You can then assign the team to a repository, and give the team appropriate permissions to the repository. Organizations and teams are further described by GitHub.
Link to section 'Creating an Organization' of 'Organization and Teams' Creating an Organization
To create an organization start by going to your user settings by clicking your profile picture in the top right of the UI and selecting "Settings"
Next, click the 'Organizations' tab in the user settings sidebar.
Then, click 'New Organization' in the top right.
Follow the wizard to finish creating an organization.
Link to section 'Managing Organizations and Teams' of 'Organization and Teams' Managing Organizations and Teams
To add members to a team, begin by switching your dashboard context to the organization. This can be done by clicking your username in the left sidebar and switching the context to that of your organization.
Next, click "View organization" in the sidebar to be brought to the organization home page.
From here, click the "People" tab
Using the 'Add member' button, new users can be added to your organization. Due to the way our authentication mechanism is tied to Purdue Career accounts, the user must have logged in to the Github instance at least once to be invited to an organization. All currently existing members of the github.rcac.purdue.edu instance have been already added to this instance.
Promoting and Demoting Managers/Owners
GitHub organizations each have one or more managers, or owners. Owners of an organization have more permissions within that organization than regular members. Compare permission levels to determine which is best for all members of your research group.
Any existing member of an organization can be promoted to Owner status by an existing Owner. Users will need to be a member of the organization before they can be promoted.
To promote an existing Organization member:
- Navigate to github.itap.purdue.edu
- From the dropdown (typically defaults to your username), select your Organization
- Click the "View Organization" button in the upper right
- Alternatively, navigate directly to github.itap.purdue.edu/yourorganization
- Select the People tab (next to Repositories)
- Find the member to be promoted, and select Owner from the dropdown now labeled "Member"
To demote an existing Organization owner:
- Repeat steps above, except change Owner dropdown to Member
Using Git
GitHub provides an excellent guide on the basics of using Git and Github. Guides are also available on other topics. All of these guides are applicable to our instance, except you will use https://github.itap.purdue.edu as the URL instead of https://github.com.
Purdue IT Github vs github.com
The GitHub Enterprise instance is a licensed product offering from GitHub itself. It is an instance of the GitHub product on github.com, and is completely independent. The system is controlled and hosted by Purdue IT and uses Purdue Career Accounts for access control. It provides the full functionality of paid memberships with github.com, at no cost to Purdue researchers.
Citing code in academic literature
Projects within GitHub can be tagged with a Digital Object Identifier (DOI) to make your code citable in academic literature.
Using SVN
Native Subversion repositories are no longer offered. However, if you are more comfortable using SVN commands, or have code that requires it, GitHub repositories may be interacted with using Subversion commands.
Regular maintenance
Regular maintenance on Purdue IT GitHub is scheduled for the first Wednesday of every month, 3:00pm to 5:00pm. Operations requiring connection to the central server (e.g. new check-outs or pull requests, etc) may time out during the maintenance period. Operations with your own local repository will not be affected.
Moving Repositories
Follow the steps below to move a full Git repository, with history, from one remote server to another. You can move an entire repository or allow choose which branches and tags to include.
For the sake of this tutorial, the original repository will be https://github.rcac.purdue.edu/foo/bar
and it will be moving to https://github.itap.purdue.edu/foo/bar
.
1) Create an empty repo on the new Git server:
You need to have an empty target repository to push your cloned repository to. Do not add any of the suggested README
or LICENSE
auto-generated files as they will not be needed.
Link to section 'Entire Repository' of 'Moving Repositories' Entire Repository
If you want to copy the entire repository you can use the following steps instead.
2) Create a local repository. In this example, we're cloning into a directory called temp-dir
:
git clone --mirror https://github.rcac.purdue.edu/foo/bar temp-dir
Note: git clone –mirror
implies –bare
and does not generate a working copy.
3) Go into the temp-dir
directory.
cd temp-dir
4) Link your local repository to the newly created repository using the following command:
git remote set-url origin https://github.itap.purdue.edu/foo/bar
5) Push all branches and tags:
git push --mirror https://github.itap.purdue.edu/foo/bar
A Note on Pull Refs
When migrating a repo, you may errors like the following:
! [remote rejected] refs/pull/100/head -> refs/pull/100/head (deny updating a hidden ref)
! [remote rejected] refs/pull/101/head -> refs/pull/101/head (deny updating a hidden ref)
You will see this refs/pull
errors if your repository has ever had a Pull Request. refs/pull
is a private, read-only ref created by GitHub, in part, to allow for linking back to a Pull Request and its discussion thread, etc. These references cannot be mirrored but they do not prevent mirroring the rest of the repo and its history. These errors can be safely ignored.
Link to section 'Selective Branches' of 'Moving Repositories' Selective Branches
2) Create a local repository. In this example, we're cloning into a directory called temp-dir
:
git clone https://github.rcac.purdue.edu/foo/bar temp-dir
3) Go into the temp-dir
directory.
cd temp-dir
4) To see available branches of https://github.rcac.purdue.edu/foo/bar
:
git branch -a
5) Checkout all the branches that you want to copy:
git checkout branch-name
6) Fetch all the tags from https://github.rcac.purdue.edu/foo/bar
:
git fetch --tags
7) Check that your local branches and tags look correct:
git tag
git branch -a
8) Link your local repository to the newly created repository using the following command:
git remote set-url origin https://github.itap.purdue.edu/foo/bar
9) Push all branches and tags:
git push origin --all
git push --tags
There should now be a full copy of the repository at https://github.itap.purdue.edu/foo/bar
.
It's a good idea to archive the old repository at https://github.rcac.purdue.edu/foo/bar
to avoid having other users making commits to the old repository. See below for more information on how to archive a repository on GitHub.
Link to section 'Moving GitHub Wikis' of 'Moving Repositories' Moving GitHub Wikis
You can also migrate GitHub wikis using the above procedure because wikis are simply Git repositories that follow the special naming convention [repo-name].wiki.git
. As with repositories, an empty wiki at the destination (e.g., https://github.itap.purdue.edu/[owner]/[repo-name]/wiki
) must first be created before you try pushing to it.
Link to section 'Archiving GitHub repositories' of 'Moving Repositories' Archiving GitHub repositories
Archiving a repository lets users know that the repository is no longer used. When archiving a repository, all of its issues, pull requests, code, labels, milestones, projects, wiki, releases, commits, tags, branches, reactions, and comments become read-only. To make any changes to an archived repository it must be unarchived first.
To archive a repository, navigate to the main page of the repository and click the Settings
tab for the repository. Scroll down and find the Danger Zone
where you should click the Archive this repository
button. Read the warnings and type the name of the repository foo/bar
in the confirmation box and click the button to archive the repository.
To unarchive a repository, follow the same instructions as archiving the repository, all the buttons will be replaced with ones to unarchive the repository.
For more information about archiving repositories you can visit GitHub's documentation and click the button to archive the repository.
Slurm
The Simple Linux Utility for Resource Management (SLURM) is a system providing job scheduling and job management on compute clusters. With SLURM, a user requests resources and submits a job to a queue. The system will then take jobs from queues, allocate the necessary nodes, and execute them.
Running Jobs
SLURM performs job scheduling. Jobs may be any type of program. You may use either the batch or interactive mode to run your jobs. Use the batch mode for finished programs; use the interactive mode only for debugging.
In this section, you'll find a few pages describing the basics of creating and submitting SLURM jobs. As well, a number of example SLURM jobs that you may be able to adapt to your own needs.
PBS to Slurm
This is a reference for the most common command, environment variables, and job specification options used by the workload management systems and their equivalents.
Quick Guide
This table lists the most common command, environment variables, and job specification options used by the workload management systems and their equivalents (adapted from http://www.schedmd.com/slurmdocs/rosetta.html).
User Commands | PBS/Torque | Slurm |
---|---|---|
Job submission | qsub [script_file] |
sbatch [script_file] |
Interactive Job | qsub -I |
sinteractive |
Job deletion | qdel [job_id] |
scancel [job_id] |
Job status (by job) | qstat [job_id] |
squeue [-j job_id] |
Job status (by user) | qstat -u [user_name] |
squeue [-u user_name] |
Job hold | qhold [job_id] |
scontrol hold [job_id] |
Job release | qrls [job_id] |
scontrol release [job_id] |
Queue info | qstat -Q |
squeue |
Queue access | qlist |
slist |
Node list | pbsnodes -l |
sinfo -N scontrol show nodes |
Cluster status | qstat -a |
sinfo |
GUI | xpbsmon |
sview |
Environment | PBS/Torque | Slurm |
Job ID | $PBS_JOBID |
$SLURM_JOB_ID |
Job Name | $PBS_JOBNAME |
$SLURM_JOB_NAME |
Job Queue/Account | $PBS_QUEUE |
$SLURM_JOB_ACCOUNT |
Submit Directory | $PBS_O_WORKDIR |
$SLURM_SUBMIT_DIR |
Submit Host | $PBS_O_HOST |
$SLURM_SUBMIT_HOST |
Number of nodes | $PBS_NUM_NODES |
$SLURM_JOB_NUM_NODES |
Number of Tasks | $PBS_NP |
$SLURM_NTASKS |
Number of Tasks Per Node | $PBS_NUM_PPN |
$SLURM_NTASKS_PER_NODE |
Node List (Compact) | n/a | $SLURM_JOB_NODELIST |
Node List (One Core Per Line) | LIST=$(cat $PBS_NODEFILE) |
LIST=$(srun hostname) |
Job Array Index | $PBS_ARRAYID |
$SLURM_ARRAY_TASK_ID |
Job Specification | PBS/Torque | Slurm |
Script directive | #PBS |
#SBATCH |
Queue | -q [queue] |
-A [queue] |
Node Count | -l nodes=[count] |
-N [min[-max]] |
CPU Count | -l ppn=[count] |
-n [count] Note: total, not per node |
Wall Clock Limit | -l walltime=[hh:mm:ss] |
-t [min] OR-t [hh:mm:ss] OR-t [days-hh:mm:ss] |
Standard Output FIle | -o [file_name] |
-o [file_name] |
Standard Error File | -e [file_name] |
-e [file_name] |
Combine stdout/err | -j oe (both to stdout) OR-j eo (both to stderr) |
(use -o without -e) |
Copy Environment | -V |
--export=[ALL | NONE | variables] Note: default behavior is ALL |
Copy Specific Environment Variable | -v myvar=somevalue |
--export=NONE,myvar=somevalue OR--export=ALL,myvar=somevalue |
Event Notification | -m abe |
--mail-type=[events] |
Email Address | -M [address] |
--mail-user=[address] |
Job Name | -N [name] |
--job-name=[name] |
Job Restart | -r [y|n] |
--requeue OR--no-requeue |
Working Directory | --workdir=[dir_name] |
|
Resource Sharing | -l naccesspolicy=singlejob |
--exclusive OR--shared |
Memory Size | -l mem=[MB] |
--mem=[mem][M|G|T] OR--mem-per-cpu=[mem][M|G|T] |
Account to charge | -A [account] |
-A [account] |
Tasks Per Node | -l ppn=[count] |
--tasks-per-node=[count] |
CPUs Per Task | --cpus-per-task=[count] |
|
Job Dependency | -W depend=[state:job_id] |
--depend=[state:job_id] |
Job Arrays | -t [array_spec] |
--array=[array_spec] |
Generic Resources | -l other=[resource_spec] |
--gres=[resource_spec] |
Licenses | --licenses=[license_spec] |
|
Begin Time | -A "y-m-d h:m:s" |
--begin=y-m-d[Th:m[:s]] |
See the official Slurm Documentation for further details.
Notable Differences
-
Separate commands for Batch and Interactive jobs
Unlike PBS, in Slurm interactive jobs and batch jobs are launched with completely distinct commands.
Usesbatch [allocation request options] script
to submit a job to the batch scheduler, andsinteractive [allocation request options]
to launch an interactive job. sinteractive accepts most of the same allocation request options as sbatch does. -
No need for
cd $PBS_O_WORKDIR
In Slurm your batch job starts to run in the directory from which you submitted the script whereas in PBS/Torque you need to explicitly move back to that directory with
cd $PBS_O_WORKDIR
. -
No need to manually export environment
The environment variables that are defined in your shell session at the time that you submit the script are exported into your batch job, whereas in PBS/Torque you need to use the
-V
flag to export your environment. -
Location of output files
The output and error files are created in their final location immediately that the job begins or an error is generated, whereas in PBS/Torque temporary files are created that are only moved to the final location at the end of the job. Therefore in Slurm you can examine the output and error files from your job during its execution.
See the official Slurm Documentation for further details.
Depot Object User Guide
Depot Object storage is a high-capacity, fast, reliable and secure object storage service designed, configured and operated for the needs of Purdue researchers in any field and shareable with both on-campus and off-campus collaborators.Depot Object Overview
As with the community clusters, research labs will be able to easily purchase capacity in the Depot Object Store through the Data Depot Purchase page on this site. For more information, please contact us.
Link to section 'Depot Object Features' of 'Depot Object Overview' Depot Object Features
The Depot Object Store offers research groups in need of centralized object storage unique features and benefits:
- Available
To any Purdue research group as a purchase in increments of 1 TB at a competitive annual price or you may request a 100 GB trial space free of charge. Participation in the Community Cluster program is not required.
- Accessible
- Access to the Depot Object Store is via S3-compatible APIs. Applications requiring POSIX filesystem access should continue to use the Data Depot Filesystem.
- Capable
The Depot Object Store facilitates joint work on shared files across your research group, avoiding the need for numerous copies of datasets across individuals' home or scratch directories. It is an ideal place to store group datasets, models, or raw data.
- Controllable Access
Access management is under your direct control. Unix groups can be created for your group and staff can assist you in setting appropriate permissions to allow exactly the access you want and prevent any you do not. Easily manage who has access through a simple web application — the same application used to manage access to Community Cluster queues.
- Data Retention
All data kept in the Depot Object Store remains owned by the research group's lead faculty. When researchers or students leave your group, any files left in their home directories may become difficult to recover. Files kept in the Depot Object Store remain with the research group, unaffected by turnover, and could head off potentially difficult disputes.
- Never Purged
Depot Object is never subject to purging.
- Reliable
Depot Object is redundant and protected against hardware failures by Ceph replication and erasure coding.
- Restricted Data
Depot object is suitable for non-HIPAA human subjects data. See the Data Depot FAQ for a data security statement for your IRB documentation. The Data Depot is not approved for regulated data, including HIPAA, ePHI, FISMA, or ITAR data.
Link to section 'Depot Object Hardware Details' of 'Depot Object Overview' Depot Object Hardware Details
The Depot Object Store is built from a high-performance Ceph storage solution with an initial total capacity of over 4 PB. This storage is redundant and reliable, with APIs axcessible from any Purdue network .
Depot Object Concepts
Depot Object Storage is a highly scalable, durable, and secure S3 object store that allows users to store and serve large amounts of data. Depot Object is an S3 compatible object storage service base on Ceph. S3 storage usage is ubiquitous in enterprise and cloud computing environments and many of these same use cases apply to scientific data storage.
Depot Object Storage is fully integrated into Purdue's Globus insfrastructure for data movement and sharing.
Link to section 'Key Concepts' of 'Depot Object Concepts' Key Concepts
S3 stores data as objects, which can be up to 5 TB in size. Objects are stored in buckets, which are similar to folders.
- Buckets: A bucket is the top-level container for storing objects in S3. Each bucket has a unique name and can be used to store an unlimited number of objects.
- Objects: An object is a file or a collection of files stored in a bucket. Objects can be stored in a variety of formats, including text, images, videos, and more.
- Keys: A key is the unique identifier for an object within a bucket. Keys are used to retrieve objects from S3 and can be thought of as the "filename" for an object.
- Metadata: Metadata is additional information about an object that is stored along with the object itself. This can include things like the object's content type and last modified date.
Link to section 'Authentication and Authorization' of 'Depot Object Concepts' Authentication and Authorization
Access Keys are used to authenticate and authorize requests to access your Depot Object resources. There are two types of access keys:
- Access Key ID: A unique identifier for your Depot Object account, which is used in conjunction with the Secret Access Key.
- Secret Access Key: A secret key that is used to sign requests to Depot Object, ensuring that only authorized users can access your resources.
Access keys are used for:
- Programmatic access: Scripting or coding interactions with Depot Object using SDKs or APIs.
- CLI access: Using the command line tools to manage your Depot Object resources.
- Third-party integrations: Integrating Depot Object with third-party applications or services that require authentication.
Link to section 'Use Cases for S3 Storage' of 'Depot Object Concepts' Use Cases for S3 Storage
- Public dataset hosting: S3 is a popular choice for hosting static websites that can be used to share datasets publicly.
- Cross resource workflows: S3 can be used to easily access and process data across multiple RCAC resources, including cloud based platforms like Geddes.
- Cold storage tier: S3 can be used as a cold storage tier for datasets that are not ready to be stored on Fortress.
- AI/ML: Many machine learning libraries natively support S3 access for data input for training. Inference engines support accessing trained models directly from S3.
- Data lakes: S3 is a popular choice for building data lakes, which are centralized repositories that store raw, unprocessed data in its native format.
Managing Buckets and Objects
Link to section 'Accessing Depot Object Storage' of 'Managing Buckets and Objects' Accessing Depot Object Storage
The S3 endpoint provided by Depot Object can be accessed in multiple ways. Two popular options for interacting with S3 storage via the command line and GUI are listed below.
Endpoint: s3.rcac.purdue.edu
Link to section 's3cmd User Guide' of 'Managing Buckets and Objects' s3cmd User Guide
s3cmd is a free command line tool for managing data in S3 compatible storage resources that works on Linux, Mac and Windows. This section provides a basic overview of using s3cmd to manage Depot Object storage.
Link to section 'Table of Contents' of 'Managing Buckets and Objects' Table of Contents
Link to section 'Installation' of 'Managing Buckets and Objects' Installation
To use s3cmd, first ensure you have it installed on your system. You can install it via pip:
pip install s3cmd
Link to section 'Authentication' of 'Managing Buckets and Objects' Authentication
Before using s3cmd to interact with your S3 storage, you need to configure your .s3cfg
file in your home directory.
The s3cmd configuration file should have the following format. Access keys and secret keys can be obtained via rcac-help@purdue.edu.
[default] host_base = s3.rcac.purdue.edu host_bucket = s3.rcac.purdue.edu access_key = <your access key> secret_key = <your secret key>
Link to section 'Basic Commands' of 'Managing Buckets and Objects' Basic Commands
s3cmd ls
: This lists all the buckets associated with your account.s3cmd sync
: Syncs directories on your machine to or from S3.s3cmd put
: Uploads an object to S3.s3cmd get
: Downloads an object from S3.
Link to section 'Bucket Management' of 'Managing Buckets and Objects' Bucket Management
s3cmd mb s3://<bucket>
: Creates a new bucket.s3cmd rb s3://<bucket>
: Deletes an entire bucket, including all objects within it. This is irreversible, so use with caution.
Link to section 'Object Management' of 'Managing Buckets and Objects' Object Management
s3cmd put <file> s3://<bucket>
: This is used for uploading files to your bucket.s3cmd get s3://<bucket>/path/to/object
: Use this command to download an object from S3, specifying both the bucket and object name where necessary.
Link to section 'More Information' of 'Managing Buckets and Objects' More Information
For detailed usage and options, run `s3cmd --help` from your terminal/command prompt.
- Download: https://s3tools.org/download
- How-To Documentation: https://s3tools.org/s3cmd-howto
Link to section 'Cyberduck' of 'Managing Buckets and Objects' Cyberduck
Cyberduck is a free server and cloud storage browser that can be used on Windows and Mac.
-
Launch Cyberduck
-
Click + Open Connection at the top of the UI.
-
Select S3 from the dropdown menu
-
Fill in Server, Access Key ID and Secret Access Key fields
-
Click Connect
-
You can now right click to bring up a menu of actions that can be performed against the storage endpoint
Further information about using Cyberduck can be found on the Cyberduck documentation site.
Rowdy User Guide
Rowdy is a technology testbed intended for evaluation and benchmarking of new computing, networking and storage architectures, including accelerators and CPUs.Overview of Rowdy
Link to section 'Overview of Rowdy' of 'Overview of Rowdy' Overview of Rowdy
Rowdy is a technology testbed intended for evaluation and benchmarking of new computing, networking and storage architectures, including accelerators and CPUs.
Access to Rowdy is available by request.
Link to section 'Rowdy Namesake' of 'Overview of Rowdy' Rowdy Namesake
Rowdy is named in honor of "Rowdy", the lovable inflatable mascot at Purdue from 1997-2007. Rowdy photo credit to HolmanPhotos.com.
Link to section 'Rowdy Specifications' of 'Overview of Rowdy' Rowdy Specifications
Rowdy compute nodes have various core counts and memory sizes, and a 100 Gbps HDR100 Infiniband interconnect.
Front-Ends | Number of Nodes | Processors per Node | Cores per Node | Memory per Node |
---|---|---|---|---|
1 | One ARM Ampere CPU | 128 | 1 TB |
Sub-Cluster | Number of Nodes | Processors per Node | Cores per Node | Memory per Node |
---|---|---|---|---|
A | 4 | One Nvidia Grace Hopper GH200 Superchip | 72 | 480 GB |
B | 2 | Two Nvidia Grace ARM CPUs | 144 | 500 GB |
Rowdy nodes run Rocky Linux 9 and use Slurm (Simple Linux Utility for Resource Management) as the batch scheduler for resource and job management. The application of operating system patches occurs as security needs dictate. All nodes allow for unlimited stack usage, as well as unlimited core dump size (though disk space and server quotas may still be a limiting factor).
Link to section 'Software catalog' of 'Overview of Rowdy' Software catalog
Environment Management with the Module Command
Our clusters provide a number of software packages to users of the system via the module
command.
Link to section 'Environment Management with the Module Command' of 'Environment Management with the Module Command' Environment Management with the Module Command
The module command is the preferred method to manage your processing environment. With this command, you may load applications and compilers along with their libraries and paths. Modules are packages that you load and unload as needed.
Please use the module command and do not manually configure your environment, as staff may make changes to the specifics of various packages. If you use the module command to manage your environment, these changes will not be noticeable.
Link to section 'Hierarchy' of 'Environment Management with the Module Command' Hierarchy
Many modules have dependencies on other modules. For example, a particular openmpi module requires a specific version of the Intel compiler to be loaded. Often, these dependencies are not clear to users of the module, and there are many modules which may conflict. Arranging modules in a hierarchical fashion makes this dependency clear. This arrangement also helps make the software stack easy to understand - your view of the modules will not be cluttered with a bunch of conflicting packages.
Your default module view on ${resource.name} will include a set of compilers and a set of basic software that has no dependencies (such as Matlab and Fluent). To make software available that depends on a compiler, you must first load the compiler, and then software which depends on it becomes available to you. In this way, all software you see when doing module avail is completely compatible with each other.
Link to section 'Using the Hierarchy' of 'Environment Management with the Module Command' Using the Hierarchy
Your default module view on ${resource.name} will include a set of compilers and a set of basic software that has no dependencies (such as Matlab and Fluent).
To see what modules are available on this system by default:
$ module avail
To see which versions of a specific compiler are available on this system:
$ module avail gcc
$ module avail intel
To continue further into the hierarchy of modules, you will need to choose a compiler. As an example, if you are planning on using the Intel compiler you will first want to load
the Intel compiler:
$ module load intel
With intel
loaded, you can repeat the avail
command, and at the bottom of the output you will see the section of additional software that the intel module provides:
$ module avail
Several of these new packages also provide additional software packages, such as MPI libraries. You can repeat the last two steps with one of the MPI packages such as openmpi
and you will have a few more software packages available to you.
If you are looking for a specific software package and do not see it in your default view, the module command provides a search function for searching the entire hierarchy tree of modules without the need for you to manually load
and avail
on every module.
Link to section 'Load / Unload a Module' of 'Environment Management with the Module Command' Load / Unload a Module
All modules consist of both a name and a version number. When loading a module, you may use only the name to load the default version, or you may specify which version you wish to load.
For each cluster, RCAC makes a recommendation regarding the set of compiler, math library, and MPI library for parallel code. To load the recommended set:
$ module load rcac
To verify what you loaded:
$ module list
To load the default version of a specific compiler, choose one of the following commands:
$ module load gcc
$ module load intel
When running a job, you must use the job submission file to load on the compute node(s) any relevant modules. Loading modules on the front end before submitting your job makes the software available to your session on the front-end, but not to your job submission script environment. You must load the necessary modules in your job submission script.
To unload a compiler or software package you loaded previously:
$ module unload gcc
$ module unload intel
$ module unload matlab
To unload all currently loaded modules and reset your environment:
$ module purge
Link to section 'Show Module Details' of 'Environment Management with the Module Command' Show Module Details
To learn more about what a module does to your environment, you may use the module show command.
$ module show matlab
For more information about Lmod:
Protected Data Filesystem User Guide
The Protected Data Filesysem (PDFS) is a high-capacity, fast, reliable and secure data storage service designed, configured and operated for the needs of Purdue researchers requiring high-performance computing to work with sensitive and restricted data.
Protected Data Filesystem Overview
As with the community clusters, research labs will be able to easily purchase capacity in the PDFSthrough the PDFS Purchase page on this site. For more information, please contact us.
Link to section 'Protected Data Filesystem Features' of 'Protected Data Filesystem Overview' Protected Data Filesystem Features
The Protected Data Filesystem (PDFS) offers research groups in need of centralized data storage unique features and benefits:
- Available
To any Purdue research group working with sensitive or restricted data as a purchase in increments of 1 TB at a competitive annual price or you may request a 100 GB trial space free of charge. Participation in the Community Cluster program is not required.
- Accessible
- Directly on Community Cluster nodes.
- From other universities or labs through Globus High Assurance.
- Capable
The PDFS facilitates joint work on protected datasets across your research group, providing a central place for datasets requiring higher levels of security to meet sponsor requirements.
- Controllable Access
Access management is under your direct control. Unix groups can be created for your group and staff can assist you in setting appropriate permissions to allow exactly the access you want and prevent any you do not. Easily manage who has access through a simple web application — the same application used to manage access to Community Cluster queues.
- Data Retention
All data kept in the PDFS remains owned by the research group's lead faculty. When researchers or students leave your group, any files left in their home directories may become difficult to recover. Files kept in PDFS remain with the research group, unaffected by turnover, and could head off potentially difficult disputes.
- Never Purged
The PDFS is never subject to purging.
- Reliable
The PDFS is redundant and protected against hardware failures.
- Restricted Data
The PDFS is suitable for sensitive and restricted datasets. Example datasets that have been reviewed and approved include NIH Database of Genotypes and Phenotypes (dbGaP), licensed datasets such as the UK Biobank, and deidentified human genomic data. The PDFS is not approved for export controlled data subject to ITAR, or CUI.
Link to section 'Protected Data Filesystem Hardware Details' of 'Protected Data Filesystem Overview' Protected Data Filesystem Hardware Details
The PDFS uses an enterprise-class Lustre storage solution with an initial total capacity of over 2 PB. This storage is redundant and reliable, and is available today on the Negishi cluster. The PDFS is non-purged space suitable for tasks such as hosting datasets, processing protected data, editing files, developing and building software, and many other uses. The PDFS is built on Data Direct Networks' 400NVX2 storage platform.
Data Security Standards
The Protected Data Filesystem (PDFS) has been reviewed by the Purdue System Security Information Assurance team and found to meets or exceeds the requirements for controlled access to dbGaP data, human genomics data, and similar levels of data protection.
The PDFS is a shared Linux computing system physically located in the Purdue Research Data Center. Purdue IT facilities are configured with a high-level of physical security. All building access is controlled by the Purdue card office, badged and logged. All Purdue IT facilities and processes are certified ISO 9000, 27001, and 20000-1.
All data is stored on RAID arrays attached to file servers on a private, non-routable network. protected data sets are only accessible from within Purdue HPC systems. None of those servers are directly accessible from the Internet. All of the community cluster internal networks are isolated from the Internet, on private networks and with login nodes by firewall rules. The research network entry points are further protected with intrusion detection systems. All servers within RCAC are additionally protected by local firewalls.
All data is stored in directories (folders) with Linux file access controls restricting access to owner and group. Group membership is set by the owner. The top-level permissions on these directories are set by the system and unchangeable by individuals. Groups and accounts are reviewed annually by the primary investigator.
All user access to the system is password controlled. All users of the system are bound by Purdue IT Policies and Standards. Remote access to the servers is via encrypted transport (i.e. SSH). No data is exported to non-compliant systems.
Privileged access accounts are approved by the RCAC staff, documented and restricted to the specific staff members responsible for maintaining the cluster. All privileged access is logged. All system components are kept up-to-date with security patches.
Link to section 'Specific Example Approved Datasets (as of Summer 2024)' of 'Data Security Standards' Specific Example Approved Datasets (as of Summer 2024)
- NIH dbGaP
- UK Biobank
- Human Genomic Data
Link to section 'Cybersecurity Standards and Dataset Approval Process' of 'Data Security Standards' Cybersecurity Standards and Dataset Approval Process
Purdue IT high-performance computing resources are built and operated in line with the NIST 800-233 "High-Performance Computing Security: Architecture, Threat Analysis, and Security Posture" best practices, approved for data subject to the NIH Genomic Data Sharing Policy, and certified ISO 27001.
Data security requirements are driven by the contract and data use/material transfer/data transfer agreement. New data use agreements are reviewed by contract analysts and Purdue System Security (PSS) Information Assurance analysts, and matched to IT resources.
Sponsor-specifc data security requirements must be reviewed by PSS analysts prior to upload into the PDFS.
Protected Data Archive User Guide
The Protected Data Archive system is a large, long-term, multi-tiered file caching and storage system utilizing both online disk and robotic tape drives, intended for storing sensitve or restricted datasets.
Protected Data Archive Overview
The Protected Data Archive (PDA) uses a Spectra Logic TFinity robotic tape library with a capacity of over 200 PB.
Storage Subsystem | Current Capacity | Hardware |
---|---|---|
Disk Cache | Over 800 TB | 2 Seagate 5U84 Storage arrays |
Long-Term Storage | Over 200 PB | LTO-9 Robotic Tape Library |
All files stored on the Protected Data Archive appear in at least two separate storage devices:
- Two copies is permanently on tape.
Both primary and secondary copies of larger files reside on separate tape cartridges in the robotic tape library. After a period of inactivity, HPSS will migrate files from disk cache to tape.
The PDA writes two copies of every file either to two tapes, or to disk and a tape, to protect against medium errors. Unfortunately, HPSS does not automatically switch to the alternate copy when it has trouble accessing the primary. If it seems to be taking an extraordinary amount of time to retrieve a file (hours), please contact support. We can then investigate why it is taking so long. If it is an error on the primary copy, we will instruct the PDA to switch to the alternate copy as the primary and recreate a new alternate copy.
Link to section 'Sensitive and Restricted Data on the Protected Data Archive' of 'Protected Data Archive Overview' Sensitive and Restricted Data on the Protected Data Archive
The Protected Data Archive is suitable for sensitive and restricted datasets. Example datasets that have been reviewed and approved include NIH Database of Genotypes and Phenotypes (dbGaP), licensed datasets such as the UK Biobank, and deidentified human genomic data. The PDA is not approved for export controlled data subject to ITAR, or CUI.
Link to section 'Protected Data Archive Storage Quota' of 'Protected Data Archive Overview' Protected Data Archive Storage Quota
There is currently no quota on PDA disk use. Archive users will receive a monthly email report showing your current PDA usage.
Files belonging to deleted accounts will also be retained, but inaccessible except by special request after the accounts have been terminated. The files will be kept for no more than ten years or the usability of the media on which they are stored, whichever comes first.
Link to section 'Proteced Data Archive File Recovery' of 'Protected Data Archive Overview' Proteced Data Archive File Recovery
Data on the PDA is not backed up elsewhere in a traditional sense. New and modified files in the disk cache are migrated to tape within 30 minutes, and Fortress maintains two copies of every file on different media to protect against media failures, but there is no backup protecting against accidental deletions.
If you remove or overwrite a file on the PDA, it is gone. You cannot request to have it retrieved.
Accounts on the Protected Data Archive
Link to section 'Obtaining an Account' of 'Accounts on the Protected Data Archive' Obtaining an Account
Any Purdue PI requiring large-scale archival storage of sensitive or restricted data may obtain access to the Protected Data Archive.
Research groups are assigned a group data storage space within ${resource.name} group space. Faculty should request a Data Depot trial to create a shared PDA space for their research group.
The Protected Data Archive is suitable for sensitive and restricted datasets. Example datasets that have been reviewed and approved include NIH Database of Genotypes and Phenotypes (dbGaP), licensed datasets such as the UK Biobank, and deidentified human genomic data. The PDA is not approved for export controlled data subject to ITAR, or CUI.
The PDA sets no limits on the amount or number of files that you may store. However, there are several restrictions on the nature of files you may store:
- Many small files: The PDA is a tape archive and works best with a few, large files. Large sets of small files should be compressed into archives with utilities such as htar. Other technical limitations are detailed on the Fortress FAQs.
- Backing up individual or departmental computers. The PDA is intended to be a research data store for sensitive and restricted data, and not a personal or enterprise backup solution.
Storing more than 50 TB of data will incur a cost recovery charge for tape media.
Link to section 'Login & Keytabs' of 'Accounts on the Protected Data Archive' Login & Keytabs
It is not possible to login directly to ${resource.name} via SSH or SCP. Access to the PDA is via Globus High Assurance.
File Storage and Transfer
Learn more about file storage transfer for the Protected Data Archive.
Your home directory on the PDA is the default directory that in which your archive files are stored.
On the PDA, your home directory will appear as /home/myusername, but this is not the same directory as your home directory on any other Purdue IT systems.
The following link will take you to more information about transferring files in and out of the PDA.
Globus High Assurance
Globus, previously known as Globus Online, is a powerful and easy to use file transfer service for transferring files virtually anywhere. It works within RCAC's various research storage systems; it connects between RCAC and remote research sites running Globus; and it connects research systems to personal systems. You may use Globus to connect to your Protected Data Archive storage space. The Protected Data Archive uses Globus' high assurance subscription, providing additional controls and security options to allow Globus to be used for datasets that contain protected data, in compliance with the data’s security requirements
Since Globus is web-based, it works on any operating system that is connected to the internet. The Globus Personal client is available on Windows, Linux, and Mac OS X. It is primarily used as a graphical means of transfer but it can also be used over the command line.
Link to section 'Globus Web:' of 'Globus High Assurance' Globus Web:
- Navigate to http://transfer.rcac.purdue.edu
- Click "Proceed" to log in with your Purdue Career Account.
- On your first login it will ask to make a connection to a Globus account. Accept the conditions.
- Now you are at the main screen. Click "File Transfer" which will bring you to a two-panel interface (if you only see one panel, you can use selector in the top-right corner to switch the view).
- You will need to select one collection and file path on one side as the source, and the second collection on the other as the destination. This can be one of several Purdue endpoints, or another University, or even your personal computer (see Personal Client section below).
The collection providing access to the PDA is:
- PDA: "Purdue Protected Data HPSS Archive", a search for "PDA" should provide appropriate matches to choose from.
From here, select a file or folder in either side of the two-pane window, and then use the arrows in the top-middle of the interface to instruct Globus to move files from one side to the other. You can transfer files in either direction. You will receive an email once the transfer is completed.
Link to section 'Globus Personal Client setup:' of 'Globus High Assurance' Globus Personal Client setup:
Globus Connect Personal is a small software tool you can install to make your own computer a Globus endpoint on its own. It is useful if you need to transfer files via Globus to and from your computer directly.
- On the "Collections" page from earlier, click "Get Globus Connect Personal" or download a version for your operating system it from here: Globus Connect Personal
- Name this particular personal system and follow the setup prompts to create your Globus Connect Personal endpoint.
- Your personal system is now available as a collection within the Globus transfer interface.
Link to section 'Globus Command Line:' of 'Globus High Assurance' Globus Command Line:
Globus supports command line interface, allowing advanced automation of your transfers.
To use the recommended standalone Globus CLI application (the globus command):
- First time use: issue the globus login command and follow instructions for initial login.
- Commands for interfacing with the CLI can be found via Using the Command Line Interface, as well as the Globus CLI Examples pages.
Link to section 'Sharing Data with Outside Collaborators' of 'Globus High Assurance' Sharing Data with Outside Collaborators
Globus allows convenient sharing of data with outside collaborators. Data can be shared with collaborators' personal computers or directly with many other computing resources at other institutions. See the Globus documentation on how to share data:
For links to more information, please see Globus Support page and RCAC Globus presentation.
Purdue GenAI Studio
Purdue GenAI Studio is an GenAI service that makes open-source models like LLaMA accessible to Purdue researchers. It is hosted entirely with on-prem resources at Purdue, not only providing democratized access but adding another layer of control compared to commercial services. Chats, documents, and models are not shared between users nor used for training.
There are two modalities for interacting with GenAI Studio, UI, and API, and additional functionality for both modalities is under active development. This system is integrated with a PostgreSQL vector database in the backend to enable retrieval-augmented generation (RAG) functionality.
Note this service is a pilot and provides only limited safety measures. Models may hallucinate or generate offensive content. GenAI Studio should not be used for any illegal, harmful, or violent purposes.
Do not input, by any method, any data into these systems that your research institution would consider sensitive or proprietary. Do not input, by any method, any data into these systems that is regulated by State or Federal Law. This includes, but is not limited to, HIPAA data, Export Controlled data, personal identification numbers (e.g. SSNs) or biometric data.
Link to section 'Access' of 'Purdue GenAI Studio' Access
Navigate to https://genai.rcac.purdue.edu/ and log in using CILogon and your standard Purdue SSO account.
User Interface
Link to section 'Chat Interface' of 'User Interface' Chat Interface
Link to section 'Model Selection' of 'User Interface' Model Selection
The chat interface allows you to select from a list of available models to interface with. This list includes both base models available to all users as well as any custom models you have created (covered below).
You may also select multiple models to compare the output for any prompt. In this case, your prompt will be sent to all selected models and the results displayed side-by-side.
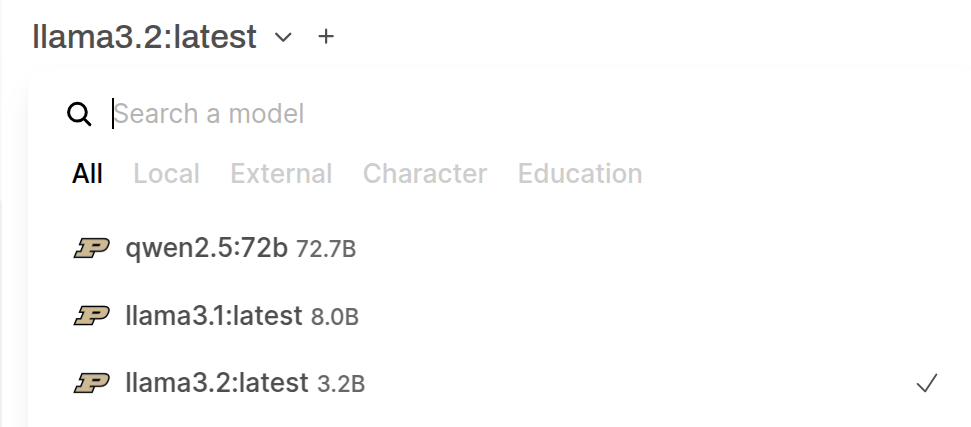
If there are additional open-source models you would like to use that are not available in the drop-down, please send in a ticket request. We are able to provide access to most open-source models.
Link to section 'Sending a Message' of 'User Interface' Sending a Message
Sending a prompt is as simple as adding text into the message bar. By clicking the microphone or headset buttons you can also use a speech-to-text service or have a “call” with the model where you can speak it the model will speak back.
You can also add a document to give the model specific context to respond to the prompt with. If you would like this context to be persisted, upload it as Knowledge or add it to a custom model as discussed below. To query an existing document that was previously uploaded into the document interface to the context of a chat, use ‘#’ and then the name of the Knowledge base before entering your prompt.
When you get a model response, you can take various action including editing, copying, or reading the response out loud, and view statistics about the generation with the options available at the bottom of the response.
Link to section 'Other Controls' of 'User Interface' Other Controls
From the top right of the screen, you can access various controls which enable you to tweak the parameters and system prompt for the chat. To persist this information, use a custom model. From these options you are also able to download chats and change other settings related to the UI.
On the left of the screen you can create new chats, access your chat history, and access the Workspace where you can upload documents and create custom models.
Link to section 'Workspace' of 'User Interface' Workspace
From the workspace you can create Knowledge Bases, upload documents and create models with a RAG functionality.
Link to section 'Knowledge' of 'User Interface' Knowledge
In the Knowledge tab, use the ‘+’ button to upload documents to a knowledge base. Set the Visibility to private if you don't want other users of GenAI Studio to see your documents.
If you would like to share your Knowledge with your research lab, department or other GenAI Studio users, groups can be used. To create a group or add members to a group please contact rcac-help@purdue.edu.
Select documents using the upload functionality to add them to a Knowledge base. Different Knowledge bases can be used to create different collections of documents, for example to create custom RAGs focusing on different tasks. Please do not upload documents with sensitive information or that are subject to regulations.
Link to section 'Models' of 'User Interface' Models
To create a custom model, navigate to the Models tab in the Workspace and click the + button on the right side of the window.
From here, you can customize a model by specifying the base model, system prompt, and any Knowledge, among other customizations. Knowledge and documents must be uploaded in the document tab before they will be accessible here. Once the model has been created it will show up in the model list in the chat interface.
API
To use the API, you wil first need to find your API key, accessible from the UI. Click on your user avatar and navigate to Settings, and then the Account page in settings. Here you will be able to see the API Keys. Expand this section and copy either the JWT token or create an API key.
Use the following endpoint to connect to the API.
For an OpenAI API-compatible response, use https://genai.rcac.purdue.edu/api/chat/completions
For a streaming response, this will return results formated like:
data: {"id": "llama3.1:latest-dd3f8a12-a36f-4fcc-9537-02a9e7c1d9c8", "created": 1749755137, "model": "llama3.1:latest", "choices": [{"index": 0, "logprobs": null, "finish_reason": null, "delta": {"content": "I"}}], "object": "chat.completion.chunk"}
For a non-streaming response, this will return results formated like:
{"id":"llama3.1:latest-703c01b4-c58d-4f78-b7e3-f0df34a91ede","created":1749755251,"model":"llama3.1:latest","choices":[{"index":0,"logprobs":null,"finish_reason":"stop","message":{"content":"I don't have a personal name. I'm an AI designed to assist and communicate with users, so I'm often referred to as a \"chatbot\" or simply \"Assistant.\" If you'd like, I can generate a unique identifier for our conversation, but it won't be a traditional name. How can I help you today?","role":"assistant"}}],"object":"chat.completion","usage":{"response_token/s":180.38,"prompt_token/s":13403.27,"total_duration":397326193,"load_duration":13348992,"prompt_eval_count":15,"prompt_tokens":15,"prompt_eval_duration":1119130,"eval_count":69,"completion_tokens":69,"eval_duration":382515521,"approximate_total":"0h0m0s","total_tokens":84,"completion_tokens_details":{"reasoning_tokens":0,"accepted_prediction_tokens":0,"rejected_prediction_tokens":0}}}
To use the endpoint, insert your API key in the example Python code below:
import requests
url = "https://genai.rcac.purdue.edu/api/chat/completions"
headers = {
"Authorization": f"Bearer {jwt_token_or_api_key}",
"Content-Type": "application/json"
}
body = {
"model": "llama3.1:latest",
"messages": [
{
"role": "user",
"content": "What is your name?"
}
],
"stream": True
}
response = requests.post(url, headers=headers, json=body)
if response.status_code == 200:
print(response.text)
else:
raise Exception(f"Error: {response.status_code}, {response.text}")
This will return output in a JSON format along with metadata.
Our clusters provide a number of software packages to users of the system via the module
command.
Link to section 'Environment Management with the Module Command' of 'Environment Management with the Module Command' Environment Management with the Module Command
The module command is the preferred method to manage your processing environment. With this command, you may load applications and compilers along with their libraries and paths. Modules are packages that you load and unload as needed.
Please use the module command and do not manually configure your environment, as staff may make changes to the specifics of various packages. If you use the module command to manage your environment, these changes will not be noticeable.
Link to section 'Hierarchy' of 'Environment Management with the Module Command' Hierarchy
Many modules have dependencies on other modules. For example, a particular openmpi
module requires a specific version of the Intel compiler to be loaded. Often, these dependencies are not clear to users of the module, and there are many modules which may conflict. Arranging modules in a hierarchical fashion makes this dependency clear. This arrangement also helps make the software stack easy to understand - your view of the modules will not be cluttered with a bunch of conflicting packages.
Your default module view on ${resource.name} will include a set of compilers and a set of basic software that has no dependencies (such as Matlab and Fluent). To make software available that depends on a compiler, you must first load the compiler, and then software which depends on it becomes available to you. In this way, all software you see when doing module avail is completely compatible with each other.
Link to section 'Using the Hierarchy' of 'Environment Management with the Module Command' Using the Hierarchy
Your default module view on ${resource.name} will include a set of compilers and a set of basic software that has no dependencies (such as Matlab and Fluent).
To see what modules are available on this system by default:
$ module avail
To see which versions of a specific compiler are available on this system:
$ module avail gcc
$ module avail intel
To continue further into the hierarchy of modules, you will need to choose a compiler. As an example, if you are planning on using the Intel compiler you will first want to load
the Intel compiler:
$ module load intel
With intel
loaded, you can repeat the avail
command, and at the bottom of the output you will see the section of additional software that the intel module provides:
$ module avail
Several of these new packages also provide additional software packages, such as MPI libraries. You can repeat the last two steps with one of the MPI packages such as openmpi
and you will have a few more software packages available to you.
If you are looking for a specific software package and do not see it in your default view, the module command provides a search function for searching the entire hierarchy tree of modules without the need for you to manually load
and avail
on every module.
Link to section 'Load / Unload a Module' of 'Environment Management with the Module Command' Load / Unload a Module
All modules consist of both a name and a version number. When loading a module, you may use only the name to load the default version, or you may specify which version you wish to load.
For each cluster, RCAC makes a recommendation regarding the set of compiler, math library, and MPI library for parallel code. To load the recommended set:
$ module load rcac
To verify what you loaded:
$ module list
To load the default version of a specific compiler, choose one of the following commands:
$ module load gcc
$ module load intel
When running a job, you must use the job submission file to load on the compute node(s) any relevant modules. Loading modules on the front end before submitting your job makes the software available to your session on the front-end, but not to your job submission script environment. You must load the necessary modules in your job submission script.
To unload a compiler or software package you loaded previously:
$ module unload gcc
$ module unload intel
$ module unload matlab
To unload all currently loaded modules and reset your environment:
$ module purge
Link to section 'Show Module Details' of 'Environment Management with the Module Command' Show Module Details
To learn more about what a module does to your environment, you may use the module show command.
$ module show matlab
For more information about Lmod:
Our clusters provide a number of software packages to users of the system via the module
command.
Link to section 'Environment Management with the Module Command' of 'Environment Management with the Module Command' Environment Management with the Module Command
The module command is the preferred method to manage your processing environment. With this command, you may load applications and compilers along with their libraries and paths. Modules are packages that you load and unload as needed.
Please use the module command and do not manually configure your environment, as staff may make changes to the specifics of various packages. If you use the module command to manage your environment, these changes will not be noticeable.
Link to section 'Hierarchy' of 'Environment Management with the Module Command' Hierarchy
Many modules have dependencies on other modules. For example, a particular openmpi
module requires a specific version of the Intel compiler to be loaded. Often, these dependencies are not clear to users of the module, and there are many modules which may conflict. Arranging modules in a hierarchical fashion makes this dependency clear. This arrangement also helps make the software stack easy to understand - your view of the modules will not be cluttered with a bunch of conflicting packages.
Your default module view on ${resource.name} will include a set of compilers and a set of basic software that has no dependencies (such as Matlab and Fluent). To make software available that depends on a compiler, you must first load the compiler, and then software which depends on it becomes available to you. In this way, all software you see when doing module avail is completely compatible with each other.
Link to section 'Using the Hierarchy' of 'Environment Management with the Module Command' Using the Hierarchy
Your default module view on ${resource.name} will include a set of compilers and a set of basic software that has no dependencies (such as Matlab and Fluent).
To see what modules are available on this system by default:
$ module avail
To see which versions of a specific compiler are available on this system:
$ module avail gcc
$ module avail intel
To continue further into the hierarchy of modules, you will need to choose a compiler. As an example, if you are planning on using the Intel compiler you will first want to load
the Intel compiler:
$ module load intel
With intel
loaded, you can repeat the avail
command, and at the bottom of the output you will see the section of additional software that the intel module provides:
$ module avail
Several of these new packages also provide additional software packages, such as MPI libraries. You can repeat the last two steps with one of the MPI packages such as openmpi
and you will have a few more software packages available to you.
If you are looking for a specific software package and do not see it in your default view, the module command provides a search function for searching the entire hierarchy tree of modules without the need for you to manually load
and avail
on every module.
Link to section 'Load / Unload a Module' of 'Environment Management with the Module Command' Load / Unload a Module
All modules consist of both a name and a version number. When loading a module, you may use only the name to load the default version, or you may specify which version you wish to load.
For each cluster, RCAC makes a recommendation regarding the set of compiler, math library, and MPI library for parallel code. To load the recommended set:
$ module load rcac
To verify what you loaded:
$ module list
To load the default version of a specific compiler, choose one of the following commands:
$ module load gcc
$ module load intel
When running a job, you must use the job submission file to load on the compute node(s) any relevant modules. Loading modules on the front end before submitting your job makes the software available to your session on the front-end, but not to your job submission script environment. You must load the necessary modules in your job submission script.
To unload a compiler or software package you loaded previously:
$ module unload gcc
$ module unload intel
$ module unload matlab
To unload all currently loaded modules and reset your environment:
$ module purge
Link to section 'Show Module Details' of 'Environment Management with the Module Command' Show Module Details
To learn more about what a module does to your environment, you may use the module show command.
$ module show matlab
For more information about Lmod:
Link to section 'Profilers' of 'Profilers' Profilers
Link to section 'NVIDIA Nsight Tools Overview' of 'NVIDIA Nsight Tools Overview' NVIDIA Nsight Tools Overview
Link to section 'Table of Contents' of 'NVIDIA Nsight Tools Overview' Table of Contents
Link to section 'Overview' of 'NVIDIA Nsight Tools Overview' Overview
NVIDIA Nsight tools are a powerful suite of libraries, SDKs, and developer tools designed for profiling, debugging, and optimizing software that utilizes NVIDIA’s latest accelerated computing hardware. These tools support both different platforms targets which incorporate NVIDIA GPU computations, and they assist developers in CUDA development as well as visual computing applications.
Multiple NVIDIA Nsight Developer Tools are available to assist developers with debugging and profiling, with a complete list accessible here. This user guide focuses on NVIDIA Nsight Systems and NVIDIA Nsight Compute.
See the list of available Nsight tools installed on RCAC clusters here.
Link to section 'Available Tools' of 'NVIDIA Nsight Tools Overview' Available Tools
Link to section 'NVIDIA Nsight Systems' of 'NVIDIA Nsight Tools Overview' NVIDIA Nsight Systems
Nsight Systems is a system-wide performance analysis tool that provides insight into CPU/GPU interactions, memory transfers, and API calls.
Key Profiling Features- CPU/GPU utilization tracking
- API call monitoring
- Memory copy analysis
- System-wide performance bottlenecks detection
- Visualizing how data moves through the system
- Parallelization insights for task execution
- Starting point for performance analysis
- Identifying system-wide bottlenecks
- Understanding CPU/GPU interactions
- Analyzing memory transfer patterns
Link to section 'NVIDIA Nsight Compute' of 'NVIDIA Nsight Tools Overview' NVIDIA Nsight Compute
An interactive kernel profiler for CUDA applications, providing detailed performance metrics and API debugging capabilities.
Key Features- Detailed kernel performance metrics
- API debugging capabilities
- Low-level GPU optimization insights
- Guided performance analysis
- Line-by-line code analysis
- Customizable profiling configurations
- Optimizing specific CUDA kernels
- Deep-diving into GPU performance
- Debugging CUDA code
- Fine-tuning kernel configurations
For more information, refer to the NVIDIA Nsight Compute user guide here.
Link to section 'Additional Resources' of 'NVIDIA Nsight Tools Overview' Additional Resources
Source: NVIDIA Tools Ecosystem SessionLink to section 'NVIDIA Nsight Tools on RCAC Clusters' of 'NVIDIA Nsight Tools on RCAC Clusters' NVIDIA Nsight Tools on RCAC Clusters
Link to section 'Tools installed along with CUDA on RCAC clusters' of 'NVIDIA Nsight Tools on RCAC Clusters' Tools installed along with CUDA on RCAC clusters
NVIDIA Nsight tools are installed along with CUDA on the clusters. Below is a list of the tools that can be found on the clusters. Some of these tools have both command-line interface (CLI) and graphical user interface (GUI) versions; for example, nsys
has a corresponding GUI version called nsys-ui
.
Tool in bin/ |
Corresponding NVIDIA Profiling Tool | Purpose |
---|---|---|
nsys |
Nsight Systems (CLI) | System-wide performance profiling for CPUs & GPUs |
nsys-ui |
Nsight Systems (GUI) | Graphical user interface for Nsight Systems |
ncu |
Nsight Compute (CLI) | Kernel-level CUDA performance profiling |
ncu-ui |
Nsight Compute (GUI) | Graphical user interface for Nsight Compute |
nvprof |
Deprecated CUDA Profiler | Legacy CUDA profiling tool (replaced by Nsight Compute & Nsight Systems) |
nvvp |
Nsight Visual Profiler (deprecated) | GUI tool for visualizing nvprof results |
compute-sanitizer |
Compute Sanitizer | Memory and correctness checks for CUDA applications |
cuda-gdb |
CUDA-GDB | Debugging CUDA applications using GDB |
cuda-gdbserver |
CUDA-GDB Server | Remote CUDA debugging |
nsight-sys |
Not recommended, use nsys or nsys-ui |
(Alias for nsys , use CLI or GUI version instead) |
Link to section 'Versions Available on RCAC Clusters' of 'NVIDIA Nsight Tools on RCAC Clusters' Versions Available on RCAC Clusters
Cluster | CUDA | Nsight Systems (nsys ) |
Nsight Compute (ncu ) |
Compute Sanitizer (compute-sanitizer ) |
nvprof (nvprof ) |
CUDA GDB (cuda-gdb ) |
---|---|---|---|---|---|---|
Gautschi | 12.6.0 | 2024.4.2 | 2024.3.0 | 2024.3.0 | 12.6.37 | 12.6 (Based on GDB 13.2) |
Anvil | 12.0.1 | 2022.4.2 | 2022.4.1 | 2022.4.1 | 12.0.147 | 12.0 (Based on GDB 12.1) |
Anvil | 11.4.2 | 2021.3.2 | 2021.2.2 | 2021.2.1 | 11.4.120 | 11.4 (Based on GDB 10.1) |
Anvil | 11.2.2 | 2020.4.3 | 2020.3.1 | 2020.3.1 | 11.2.152 | 11.2 (Based on GDB 8.3.1) |
Anvil | 11.0.3 | 2020.3.2 | 2020.1.2 | 2020.1.2 | 11.0.221 | 11.0 (Based on GDB 8.2) |
Gilbreth | 12.6.0 | 2024.4.2 | 2024.3.0 | 2024.3.0 | 12.6.37 | 12.6 (Based on GDB 13.2) |
Gilbreth | 12.1.1 | 2023.1.2 | 2023.1.1 | 2023.1.1 | 12.1.105 | 12.1 (Based on GDB 12.1) |
Scholar | 12.1.0 | 2024.4.2 | 2024.3.0 | 2024.3.0 | 12.6.37 | 12.6 (Based on GDB 13.2) |
Link to section 'Where to Find Nsight Tools' of 'NVIDIA Nsight Tools on RCAC Clusters' Where to Find Nsight Tools
When you load a CUDA module, the NVIDIA Nsight tools path will be set depending on the CUDA module you are loading. After loading the CUDA module, you can find the corresponding Nsight tools using commands like which nsys
. For example, here is the path on Gautschi:
$ ml spider cuda
----------------------------------------------------------------------------
cuda: cuda/12.6.0
----------------------------------------------------------------------------
Other possible modules matches:
cuda-quantum
This module can be loaded directly: module load cuda/12.6.0
----------------------------------------------------------------------------
To find other possible module matches execute:
$ module -r spider '.*cuda.*'
$ ml cuda/12.6.0
$ which nsys
/apps/gautschi/cuda/12.6/bin/nsys
Link to section 'NVIDIA Nsight Systems' of 'NVIDIA Nsight Systems' NVIDIA Nsight Systems
Link to section 'Table of Contents' of 'NVIDIA Nsight Systems' Table of Contents
- Overview
- Supported Platforms
- Installation
- Profiling and Analysis
- Troubleshooting
- Additional Resources
Link to section 'Overview' of 'NVIDIA Nsight Systems' Overview
NVIDIA Nsight Systems is a powerful tool that enables tracing of any NVIDIA GPU-accelerated application. It generates a holistic, easy-to-understand, and visually pleasing timeline view of system metrics, including all available hardware resources and active processes. This helps developers find performance issues and identify optimization opportunities. Beyond performance investigations, Nsight Systems is an ideal debugging tool for asynchronous GPU computing. Nsight Systems is available as both a CLI and GUI application, useful to different user needs.
Link to section 'Quick Start Guide' of 'NVIDIA Nsight Systems' Quick Start Guide
- Installation: Load the CUDA module on your system:
ml cuda/12.6
- Basic Profiling: Profile your application using the CLI:
nsys profile -o output_name ./your_application
- View Results: Either use the CLI to view stats:
nsys stats output_name.nsys-rep
nsys-ui
For more detailed instructions, see the sections below.
Link to section 'Supported Platforms' of 'NVIDIA Nsight Systems' Supported Platforms
Nsight Systems supports profiling on various platforms and can be used both locally—to profile code running on your machine—and remotely by connecting to targets over SSH or Bluetooth. For more details on which hosts can profile which targets, refer to the table below and visit NVIDIA's website for additional information.
Link to section 'Installation' of 'NVIDIA Nsight Systems' Installation
- If you have an NVIDIA GPU on your local system, you can install Nsight Systems by visiting this link. With your local installation, you can collect profiling data from GPU-accelerated applications running on your system or connect to other devices via Bluetooth (for devices like Jetson) or SSH to profile code on remote computers.
- On RCAC clusters with NVIDIA GPUs (Gautschi, Gilbreth, Anvil, and Scholar), Nsight Systems is typically installed alongside CUDA in the same binary directory. Loading the CUDA module will automatically set the paths for both the CLI tool (
nsys
) and the UI tool (nsys-ui
) in your environment.
For example, on Gilbreth:
$ ml cuda/12.6
$ which nsys
/apps/spack/gilbreth-r9/apps/cuda/12.6.0-gcc-11.5.0-a7cv7sp/bin/nsys
In some cases, the tool may also exist separately within the CUDA directory. For example, we have another installation of Nsight Systems in the CUDA directory:
$ pwd
/apps/spack/gilbreth-r9/apps/cuda/12.6.0-gcc-11.5.0-a7cv7sp
$ ls
bin gds nsight-compute-2024.3.0 pkgconfig tools
compute-sanitizer gds-12.6 nsightee_plugins README version.json
DOCS include nsight-systems-2024.4.2 share
EULA.txt lib64 nvml src
extras libnvvp nvvm targets
$ ls ./nsight-systems-2024.4.2/bin/nsys-ui
./nsight-systems-2024.4.2/bin/nsys-ui
Link to section 'Profiling and Analysis' of 'NVIDIA Nsight Systems' Profiling and Analysis
Link to section 'NVIDIA Nsight System CLI (nsys)' of 'NVIDIA Nsight Systems' NVIDIA Nsight System CLI (nsys)
First, ensure you have been allocated access to GPUs via the nvidia-smi
command.
Provided you have access to GPUs, you can profile a GPU-enabled application from the command line using the nsys
command:
nsys profile -o output_name ./your_application
Common options:
--gpu-metrics-device=all
– Collects GPU performance metrics (only works for Turing and newer architectures)--trace=cuda,nvtx,osrt
– Specify tracing for CUDA, NVTX, and OS runtime
For example, we can track GPU usage in machine learning training with:
nsys profile --trace=cuda,nvtx,osrt -o my_profile python train_model.py
This will create two files, my_profile.sqlite
and my_profile.qdrep
(or my_profile.nsys-rep
depending on the version) which store the results of the profiling. To analyze results directly on the command line, you can then run the following command:
nsys stats my_profile.nsys-rep
This command will output several tables that may be of interest for GPU optimization. For the purposes of GPU benchmarking, the CUDA API summary table will contain the statistics for the runtimes of CUDA API functions:
** CUDA API Summary (cuda_api_sum):
Time (%) Total Time (ns) Num Calls Avg (ns) Med (ns) Min (ns) Max (ns) StdDev (ns) Name
-------- --------------- --------- ----------- ----------- --------- ---------- ----------- ------------------------------
87.9 94,864,569 2,560 37,056.5 7,260.5 5,496 15,974,993 575,765.3 cudaLaunchKernel
3.9 4,182,395 484 8,641.3 9,363.5 3,857 42,452 4,478.2 cudaMemcpyAsync
3.6 3,890,901 484 8,039.1 7,569.5 1,997 65,340 3,191.9 cudaStreamSynchronize
1.9 2,021,777 2 1,010,888.5 1,010,888.5 2,769 2,019,008 1,425,696.3 cudaFree
1.4 1,477,196 1 1,477,196.0 1,477,196.0 1,477,196 1,477,196 0.0 cudaHostAlloc
1.1 1,206,049 8 150,756.1 147,012.0 4,340 394,302 139,253.6 cudaMalloc
0.2 178,292 810 220.1 146.0 77 6,701 267.8 cuGetProcAddress_v2
0.0 46,514 36 1,292.1 373.0 334 15,280 2,696.0 cudaEventCreateWithFlags
0.0 11,710 2 5,855.0 5,855.0 4,559 7,151 1,832.8 cudaStreamIsCapturing_v10000
0.0 9,806 3 3,268.7 2,574.0 2,232 5,000 1,509.1 cuInit
0.0 2,630 3 876.7 506.0 117 2,007 998.0 cuModuleGetLoadingMode
0.0 2,384 2 1,192.0 1,192.0 744 1,640 633.6 cudaGetDriverEntryPoint_v11030
If your program is frequently transferring a large amount of data to and from the GPU (for example, loading batches of training data), it may be useful to inspect the "host to device" and "device to host" memory copy times:
** CUDA GPU MemOps Summary (by Time) (cuda_gpu_mem_time_sum):
Time (%) Total Time (ns) Count Avg (ns) Med (ns) Min (ns) Max (ns) StdDev (ns) Operation
-------- --------------- ----- -------- -------- -------- -------- ----------- ----------------------------
65.7 466,364 324 1,439.4 1,408.5 1,343 2,016 62.3 [CUDA memcpy Host-to-Device]
34.3 243,199 160 1,520.0 1,504.0 1,472 2,944 120.4 [CUDA memcpy Device-to-Host]
** CUDA GPU MemOps Summary (by Size) (cuda_gpu_mem_size_sum):
Total (MB) Count Avg (MB) Med (MB) Min (MB) Max (MB) StdDev (MB) Operation
---------- ----- -------- -------- -------- -------- ----------- ----------------------------
0.247 324 0.001 0.000 0.000 0.005 0.001 [CUDA memcpy Host-to-Device]
0.001 160 0.000 0.000 0.000 0.000 0.000 [CUDA memcpy Device-to-Host]
- An
nsys
report is a database of collected samples and derived metrics. Using thensys
CLI, you can export the report into various formats, including SQLite, JSON, and more. - For more profiling options visit Nvidia Nsight documentation.
Link to section 'Limitations in Profiling' of 'NVIDIA Nsight Systems' Limitations in Profiling
For security reasons, some profiling options and results are not available for public use. Particularly, anything that utilizes performance counters on GPUs, or performs CPU sampling, are disabled. This means that capture options utilizing performance counters, or that otherwise require root access, will not be operational for general users. These options include:
--gpu-metrics-device=XXX
--gpu-metrics-set=XXX
--sample=system-wide
--event-sample=system-wide
--cpu-core-events=XXX
--cpu-core-metrics=XXX
--cpuctxsw=system-wide
--run-as=XXX
--ftrace=XXX
Link to section 'NVIDIA Nsight System GUI (nsys-ui)' of 'NVIDIA Nsight Systems' NVIDIA Nsight System GUI (nsys-ui)
If you'd prefer to analyze via a graphical interface, you can use nsys-ui
both to load the profiling results and to profile.
Link to section 'Launching GUI' of 'NVIDIA Nsight Systems' Launching GUI
RCAC Clusters' nsys-ui App Launcher
In some of our clusters, we have an app-launcher for this tool:
If the system provides an app launcher for nsys-ui, you can simply open it through the graphical interface without manually loading modules. Search for Nsight Systems UI in the application menu.
Launching Without an App Launcher
If an app launcher is unavailable, you may need to manually load the required modules before running nsys-ui.
- Prerequisite Modules:
# On Gilbreth: $ ml cuda $ ml qt $ ml xcb-util-cursor $ which nsys-ui $ nsys-ui # $ ml libxcb # $ ml mesa-glu|mesa # (Likely required)
Link to section 'Profiling' of 'NVIDIA Nsight Systems' Profiling
- Load the modules and launch Nsight Systems UI
nsys-ui
. - Specify the Profiling Target, which is the system where your CUDA application will run.
- You have the option to choose a remote machine with SSH and USB connection options. However, since this tutorial is focused on profiling applications running on the RCAC cluster, you need to connect to the Localhost, as it is already one of the RCAC nodes.
- Connect to the target machine.
- Set up profiling configuration:
- Command Line with Arguments: Provide the path to the executable file. This is the only mandatory setting.
- Working Directory: Automatically set but can be changed if needed.
- Data Collection Settings:
- Note: Choosing too many metrics can introduce overhead, so enable only necessary ones. Consider tracing different events across multiple runs to minimize overhead.
- There are plenty of customization options, and you will need to enable only a small subset of collection metrics.
- An explanation of each option is available in the Nsight Systems documentation.
- Click Start to begin data collection.
- Then, it will create a Nsight Systems report after executing the application and display the collected metrics inside the GUI.
Link to section 'Loading Profiling results' of 'NVIDIA Nsight Systems' Loading Profiling results
- Load dependent graphical utilities:
module load xcb-util-cursor xcb-util-image xcb-util-keysyms xcb-util-renderutil xcb-util-wm
, and launch the Graphical interface withnsys-ui
(Or use the app launcher described above). - Open the
.nsys-rep
or.qdrep
with File > Open. - A timeline of the processes will load. The X axis represents the runtime, and the Y axis has a breakdown of each process. Within each process, you can view the memory copy and CUDA API events.
- You can highlight a portion of the timeline and right-click to zoom to selection.
- Right-clicking any row on the Y axis and clicking "show in events view" will provide a summary in the bottom of the window.
Link to section 'Interpreting Results' of 'NVIDIA Nsight Systems' Interpreting Results
Link to section 'Understanding Timeline View' of 'NVIDIA Nsight Systems' Understanding Timeline View
- CPU Rows: Show thread activity, API calls, and synchronization points
- GPU Rows: Display kernel executions, memory transfers, and hardware metrics
- Memory Rows: Indicate memory operations between host and device
- CUDA API Rows: Show CUDA API calls and their duration
Link to section 'Common Performance Patterns' of 'NVIDIA Nsight Systems' Common Performance Patterns
- GPU Idle Periods
- Look for gaps in GPU timeline
- Check for CPU bottlenecks or synchronization issues
- Consider using CUDA streams for better overlap
- Memory Transfer Bottlenecks
- Large host-to-device or device-to-host transfers
- Consider using pinned memory or unified memory
- Look for opportunities to reduce data movement
- API Call Overhead
- Frequent small kernel launches
- Consider kernel fusion or batching
- Look for unnecessary synchronization points
Link to section 'Troubleshooting' of 'NVIDIA Nsight Systems' Troubleshooting
Link to section 'Common Issues' of 'NVIDIA Nsight Systems' Common Issues
- Unable to Launch nsys-ui
- Ensure all required modules are loaded
- Check X11 forwarding if running remotely
- Verify GPU driver compatibility
- Profile Collection Fails
- Verify GPU allocation and access
- Check available disk space
- Ensure proper permissions
- High Overhead During Profiling
- Reduce the number of metrics collected
- Use shorter collection duration
- Apply appropriate filters
Link to section 'Error Messages' of 'NVIDIA Nsight Systems' Error Messages
- "No CUDA application was profiled": Ensure your application is using CUDA and GPU resources are available
- "Failed to load driver": Check CUDA installation and driver compatibility
- "Unable to connect to target": Verify SSH settings and network connectivity for remote profiling
Link to section 'FAQs' of 'NVIDIA Nsight Systems' FAQs
- Where are the logs stored?
- By default,
nsys
generates.qdrep
(or.nsys-rep
) and.sqlite
files, which store data in a format that thensys-ui
application can easily understand. If you'd like to export the data in a friendlier CSV format, you can use the following:
nsys stats --export=csv -o output.csv my_profile.nsys-rep
- By default,
For more details on using Nsight Systems, please check the NVIDIA Nsight Systems documentation and the User Guide.